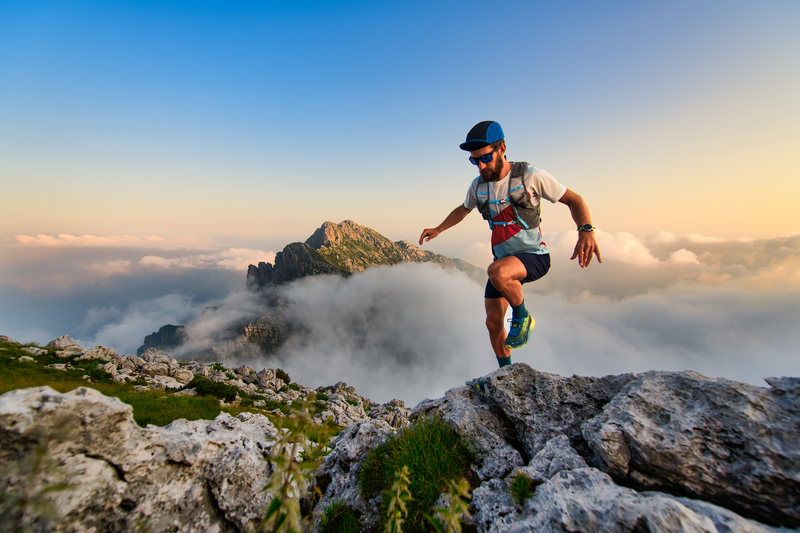
94% of researchers rate our articles as excellent or good
Learn more about the work of our research integrity team to safeguard the quality of each article we publish.
Find out more
ORIGINAL RESEARCH article
Front. Immunol. , 29 July 2022
Sec. Autoimmune and Autoinflammatory Disorders
Volume 13 - 2022 | https://doi.org/10.3389/fimmu.2022.930087
This article is part of the Research Topic Recent Advances in Antiphospholipid Syndrome View all 17 articles
Background: Ischemic stroke (IS) is the most common and life-threatening arterial manifestation of antiphospholipid syndrome (APS). It is related to high mortality and severe permanent disability in survivors. Thus, it is essential to identify patients with APS at high risk of IS and adopt individual-level preventive measures. This study was conducted to identify risk factors for IS in patients with APS and to develop a nomogram specifically for IS prediction in these patients by combining the adjusted Global Anti-Phospholipid Syndrome Score (aGAPSS) with additional clinical and laboratory data.
Methods: A total of 478 consecutive patients with APS were enrolled retrospectively. All patients were randomly assigned to the training and validation cohorts. Univariate and multivariate binary logistic analyses were conducted to identify predictors of IS in the training cohort. Then, a nomogram was developed based on these predictors. The predictive performance of the nomogram for the training and validation cohorts was evaluated by determining areas under the receiver operating characteristic curve (AUROC) and creating calibration plots. A decision curve analysis (DCA) was conducted to compare the potential net benefits of the nomogram with those of the aGAPSS.
Results: During a mean follow-up period of 2.7 years, 26.9% (129/478) of the patients were diagnosed with IS. Binary logistic regression analysis revealed that five risk factors were independent clinical predictors of IS: age (P < 0.001), diabetes (P = 0.030), hyperuricemia (P < 0.001), the platelet count (P = 0.001), and the aGAPSS (P = 0.001). These predictors were incorporated into the nomogram, named the aGAPSS-IS. The nomogram showed satisfactory performance in the training [AUROC = 0.853 (95% CI, 0.802–0.896] and validation [AUROC = 0.793 (95% CI, 0.737–0.843)] cohorts. Calibration curves showed good concordance between observed and nomogram-predicted probability in the training and validation cohorts. The DCA confirmed that the aGAPSS-IS provided more net benefits than the aGAPSS in both cohorts.
Conclusion: Age, diabetes, hyperuricemia, the platelet count, and the aGAPSS were risk factors for IS in patients with APS. The aGAPSS-IS may be a good tool for IS risk stratification for patients with APS based on routinely available data.
Antiphospholipid syndrome (APS) is an autoimmune disorder characterized by recurrent thrombotic events and pregnancy morbidity associated with the persistent presence of antiphospholipid antibodies (aPLs) (1). Ischemic stroke (IS) is one of the most common central nervous system manifestations and the most life-threatening complication of APS (2, 3). It accounts for nearly half of the arterial events caused by APS (4). Nearly 20% of cerebral strokes in patients younger than 50 years have been suggested to be associated with APS (5). In addition, cerebral infarction was reported in 19.8% of a cohort of 1000 European patients with APS and accounted for 11.8% of all deaths that occurred during a 10-years follow-up period (6). IS is associated with a high mortality rate and severe permanent disability in survivors (7). In 2019, stroke led to 6.55 million deaths and 143 million disability-adjusted life years on average, 62.4% of which were ischemic strokes (8). Thus, it is essential to identify patients with APS at high risk of IS and adopt individual-level preventive measures.
No widely accepted predictive tool or model has been established for aPL-positive patients. The Global Antiphospholipid Syndrome Score (GAPSS) and antiphospholipid score (aPL-S) are used to predict thrombosis in patients with APS (9, 10). Although their utility has been validated with various external cohorts, they rely on aPL quantification (11–13), which is difficult due to the problems with the standardization of aPL criteria and the difficulty of interpreting lupus anticoagulant results (14). Furthermore, these scoring systems require data on laboratory parameters not routinely measured in daily clinical practice. GAPSS modifications, including the adjusted Global Antiphospholipid Syndrome Score (aGAPSS) and aGAPSS specific for cardiovascular disease (aGAPSSCVD), have been proposed (15, 16), and patients with primary APS who had experienced IS were found to have higher aGAPSS (17). However, little is known about the efficiency of the aGAPSS for the prediction of IS in patients with APS. Traditional thrombotic risk factors, including obesity, smoking habit, and diabetes, also increase the risk of thrombosis in these patients (18).
In the present study, we evaluated the risk factors for IS in patients with APS. We also developed a new nomogram specifically for IS prediction in these patients by combining the aGAPSS with additional risk factors.
Consecutive patients with APS who attended Peking University People’s Hospital between 1 January 2005 and 1 March 2021 were enrolled retrospectively in this study. All participants met the 2006 Sydney classification criteria for APS (1). The exclusion criteria were: 1) IS occurrence before APS onset; 2) other coagulation disorders, such as severe hepatic diseases and malignancy; and 3) incomplete medical records. The following clinical data were collected at the time of APS diagnosis: age, sex, body mass index, time from first APS event, history of autoimmune disease (e.g., systemic lupus erythematosus, Sjögren’s syndrome), vascular thrombosis, pregnancy morbidity, hypertension, hyperlipidemia, diabetes, chronic obstructive pulmonary disease (COPD), chronic kidney disease, hyperuricemia, smoking, laboratory data, and treatment. Patients were followed by telephone interviews or clinic visits every three months.
According to the guidelines of the American Stroke Association (12), hypertension, diabetes, smoking, and hyperlipidemia were considered to be traditional risk factors for IS. Hypertension, diabetes, and smoking were assessed according to the guidelines of the National Institute for Health and Care Excellence (19). Hypertension was defined as high blood pressure at two or more random time points or the use of antihypertensive medication. Diabetes was defined as a fasting blood glucose level > 7.0 mmol/L on more than two occasions or the use of insulin or oral antidiabetic drugs. Smoking status was determined by self-reports of tobacco consumption. According to the Chinese Guideline for the Management of Dyslipidemia in Adults (20), hyperlipidemia was defined by any of the following criteria: 1) triglyceride level > 2.3 mmol/L, 2) high-density lipoprotein level < 1.0 mmol/L, 3) low-density lipoprotein level > 4.1 mmol/L, and 4) total cholesterol level > 6.2 mmol/L. Hyperuricemia was established when fasting serum urate levels equaled to or exceeded 420 μmol/L (21). Thrombocytopenia was defined as a platelet count <100 × 109/L (1). In addition, diagnoses of COPD and chronic kidney disease, recently accepted as risk factors for stroke (22–24), were confirmed by medical record review.
Anti-cardiolipin (aCL) and anti-β2-glycoprotein I antibody (aβ2GPI) were detected by enzyme-linked immunosorbent assay as described previously (25). Values for aCL > 12 IU/mL and aβ2GPI > 27 RU/mL were considered positive based on local cut-off. The lupus anticoagulant (LAC) assay was performed using Stago STA Compact Hemostasis System as described previously (25). The simplified Dilute Russell’s Viper Venom Test (dRVVT) was considered positive if the dRVVT ratios were > 1.2.
The aGAPSS was calculated as previously reported by adding corresponding points to the risk factors: 3 for hyperlipidemia, 1 for arterial hypertension, 5 for aCL, 4 for aβ2GPI, and 4 for LAC (10).
Two experienced neurologists assigned patients to IS and non-IS groups based on clinical manifestations and neuroimaging (magnetic resonance imaging or computed tomography) findings. The diagnostic criteria for ischemic stroke are as follows: (1) acute onset; (2) focal neurological deficit (weakness or numbness of one side of the face or limb, speech impairment, etc.); (3) presence of a responsible lesion on imaging or signs/symptoms lasting more than 24 h; (4) exclusion of non-vascular causes; and (5) exclusion of cerebral hemorrhage by neuroimaging (26). Any inconsistency in the definition of IS was resolved by a senior neurologist.
The statistical analyses were performed using IBM SPSS Statistics (version 22.0), MedCalc software (version 20.1.0), and R software (version 4.1.2). By using a computer random number generator, one-half of the patients were randomized into the training cohort to construct the predictive nomogram, and the remaining patients were assigned to the validation cohort to evaluate the performance of the nomogram. Group comparisons were performed using the unpaired t-test (for normally distributed data) and Mann–Whitney U test (for non-normally distributed data) for quantitative variables, and Fisher’s exact test and the chi-squared test for categorical variables. Receiver operating characteristic (ROC) curve analysis was used to determine the cutoff aGAPSS for discrimination of the IS and non-IS groups.
For the training cohort, univariate logistic regression analysis was performed to screen for potential predictors of IS. To identify independent risk factors for IS, variables with P values < 0.05 in the univariate analysis were included in a multivariate regression model based on the training cohort. The variance inflation factor (VIF) was used to measure the impact of collinearity among the variables in the regression model. Then, a nomogram was built based on these independent predictors using the rms package of the R software. The area under the receiver operating characteristic curve (AUROC) was drawn to evaluate and compare the discrimination efficacy of the nomogram with that of the aGAPSS. To access the predictive accuracy of the nomogram, calibration curves were drawn by plotting the observed probability against the nomogram-predicted probability. Finally, a decision curve analysis (DCA) was conducted with the rmda package to evaluate and compare potential net benefits at different threshold probabilities. For all statistical tests, two-sided P values < 0.05 were significant.
Of 505 patients with APS initially identified, three whose IS preceded APS onset, six with other coagulation diseases (four with malignancies and two with severe hepatic disease), and 18 whose medical records were incomplete were excluded. The remaining 478 patients were assigned randomly to the training and validation cohorts (n = 239 each; Figure 1). Baseline clinical data did not differ between cohorts (Table 1). During a mean follow-up period of 2.7 years, 129 (26.9%) patients were diagnosed with IS. Among all the IS patients in our study, eight patients had no definite neurological deficit symptom. Their silent ischemic lesions were identified through MRI scan due to nonspecific symptom (e.g., headache, dizziness), which showed high signal on the diffusion-weighted image (DWI). The DWI sequence has high accuracy for diagnosing IS (88%−100% sensitivity and 95%−100% specificity) (27). The IS incidence rates were similar in the training and validation cohorts [n = 69 (28.9%) and n = 61 (25.5%), respectively].
In the training cohort, patients with IS were older than those without IS [54.0 (43.0–64.0) vs. 37.0 (30.0–50.0) years, P < 0.01] and the proportion of males was larger in the former group (36.2% vs. 21.2%, P = 0.02). aGAPSS were higher for patients with than for those without IS [13.0 (11.0–16.0) vs. 9.0 (5.3–13.0), P < 0.01]. Greater prevalence of hypertension (50.7% vs. 24.1%, P < 0.01), diabetes (30.4% vs. 7.1%, P < 0.01), hyperlipidemia (65.2% vs. 50%, P = 0.03), and hyperuricemia (26.1% vs. 4.7%, P < 0.01) were also observed in patients with IS than in those without IS. Among laboratory parameters, the platelet count [114.9 (63.0–172.0) vs. 180.1 (104.3–237.5) ×109/L, P < 0.01] was lower in the IS than in the non-IS group. The rate of aCL positivity (73.9% vs. 58.8%, P = 0.03), LAC positivity (79.7% vs. 53.5%, P < 0.01), and triple aPL positivity (44.9% vs. 25.9%, P < 0.01), were also higher in the IS than in the non-IS group (Table 2).
In the univariate analysis, IS was associated with age (P < 0.001), sex (P = 0.017), diabetes (P < 0.001), hyperuricemia (P < 0.001), autoimmune disease (P = 0.020), the platelet count (P < 0.001), and the aGAPSS (P < 0.001; Table 2). For the training cohort, the AUROC for the ability of the aGAPSS to predict IS was 0.686 [95% confidence interval (CI), 0.623–0.744; P < 0.001]. The ROC curve analysis showed that the cut-off aGAPSS for IS prediction was 10, with a sensitivity of 75.4% and a specificity of 60%. In the multivariable regression analysis, independent predictors of IS in the training cohort were age (P < 0.001), diabetes (P = 0.030), hyperuricemia (P < 0.001), the platelet count (P = 0.001), and aGAPSS > 10 (P = 0.001; Table 3). All VIF values were below 1.26, indicating low degrees of collinearity among variables. We built a predictive nomogram for IS (the aGAPSS-IS) based on these five independent predictors (Figure 2). For each patient, we added up the points identified on the points scale for the five risk factors. Then, the risk probability of IS was obtained according to the “Total Points” axis of the nomogram.
Figure 2 The nomogram for predicting the risk of IS in the training cohort. For each patient, we added up the points identified on the points scale for the five risk factors. Then, the risk probability of IS was obtained according to the “Total Points” axis of the nomogram. APS, antiphospholipid syndrome; IS, ischemic stroke; aGAPSS, adjusted Global Anti-Phospholipid Syndrome Score; PLT, platelet count.
For the training and validation cohorts, the AUROCs for the aGAPSS-IS were larger than those for the aGAPSS [0.853 (95% CI, 0.802–0.896) vs. 0.686 (95% CI, 0.623–0.744) and 0.793 (95% CI, 0.737–0.843) vs. 0.624 (95% CI, 0.560–0.656), respectively, both P < 0.001], meaning that the aGAPSS-IS showed better discriminative capacity (Figures 3A, B). The calibration plot for the training cohort showed optimal agreement between the aGAPSS-IS–predicted probability and the observed probability of IS; the mean absolute error was 0.015 (Figure 4A). The plot for the validation cohort also showed excellent concordance between these probabilities, with a mean absolute error of 0.028 (Figure 4B). For the training cohort, the DCA demonstrated that the aGAPSS-IS provided more net benefits than the aGAPSS for IS prediction when the threshold probability was >2% (Figure 5A). Similarly, the aGAPSS-IS always had marked net benefits over the aGAPSS for IS prediction when the threshold probability was >4% (Figure 5B).
Figure 3 Comparison of the area under the receiver operating characteristic curve values between aGAPSS-IS score and aGAPSS in the training and the validation cohort. (A) In the training cohort, the aGAPSS-IS score had a larger AUROC than the aGAPSS [0.853 (95% CI, 0.802-0.896) vs. 0.686 (95% CI, 0.623-0.744), P < 0.001]; (B) In the validation cohort, the AUROC of aGAPSS-IS score was larger than the aGAPSS [0.793 (95% CI, 0.737-0.843) vs. 0.624 (95% CI, 0.560-0.656), P < 0.001]. aGAPSS, adjusted Global Anti-Phospholipid Syndrome Score.
Figure 4 The calibration curve of the aGAPSS-IS score in the training and the validation cohort. (A) mean absolute error = 0.015 (training cohort); (B) mean absolute error = 0.028 (validation cohort).
Figure 5 Comparison of the decision curves between the aGAPSS-IS score and aGAPSS in the training and the validation cohort. (A) For a threshold probability > 2%, application of the aGAPSS-IS score would add more net benefit to patients compared to the use of the aGAPSS in the training cohort. (B) For a threshold probability > 4%, the aGAPSS-IS score would provide more net benefit to APS patients than the aGAPSS in the validation cohort. aGAPSS adjusted Global Anti-Phospholipid Syndrome Score.
In the present study, we developed the clinical nomogram aGAPSS-IS for IS risk stratification for patients with APS. This nomogram was based on easily accessible data, including patient age, diabetes, hyperuricemia, platelet count, and aGAPSS. It showed better performance than the aGAPSS for IS prediction in an APS cohort. It could facilitate rheumatologists in making individualized decisions about the clinical management of patients with APS (e.g., whether neuroimaging is indicated during hospitalization) by weighing the probability of IS occurrence.
IS represents the most common and disabling arterial involvement in APS (28). It is vital to identify APS patients at high risk of stroke and adopt timely prophylactic treatment measures. The aGAPSS, based on conventional cardiovascular risk factors and aPL profile, was a widely accepted risk stratification score (10, 16, 29). Consistent with this, our results demonstrated that aGAPSS > 10 could predict IS in APS patients with a sensitivity of 75.4% and a specificity of 60%. However, the performance of aGAPSS in discriminating IS was dissatisfactory, perhaps due to the lack of consideration of some critical risk factors.
Age is a robust non-modifiable risk factor for IS in the general population; the risk of IS doubles every 10 years after the age of 55, and almost 75% of strokes occur in people aged > 65 years (30). Patients with diabetes are more prone to atherosclerosis and microangiopathy than are healthy people, and these vascular diseases deteriorate rapidly, leading to cardiovascular accidents. The prevalence of diabetes among patients experiencing IS is estimated to be 33% and was found to be associated closely with poor outcomes and stroke recurrence in this population (31). Thus, aging and diabetes contribute to the incidence of IS in patients with APS. Hyperuricemia has a dose-response relationship to cardiovascular disease (23) and is an accepted risk factor for venous thromboembolism (32, 33). According to a longitudinal study including 15773 participants, a 59.5-μmol/l increase in uric acid was associated with a 28% increase in total and cardiovascular mortality during ten years of follow-up (34). Monosodium urate crystals can induce neutrophil extracellular trap release (35), a very important mechanism for thrombosis in APS (36). Hence, hyperuricemia is another independent risk factor for IS in patients with APS. Thrombocytopenia is related significantly to IS and is a risk factor for thrombosis in patients with APS (37) and aPL carriers (12). A prospective study including 228 APS patients demonstrated that patients with thrombocytopenia had a higher risk of thrombotic events than those without [HR = 2.93, (95%CI:1.31-6.56)] (38). Phospholipids are integral parts of the platelet membrane, and the binding of aPL leads to the destruction of platelets and the release of microparticles, which play a procoagulant role in APS-related thrombotic events (39, 40). In our study, it was also an essential risk factor for arterial complications in the central nervous system.
The nomogram makes clinicians realize the critical role of traditional cardiovascular disease (CVD) risk factors for IS. CVD, especially stroke and coronary artery disease, is a leading cause of morbidity and mortality in APS (6). In the recent European League Against Rheumatism recommendations for managing CVD, the screening and strict control of traditional cardiovascular risk were highlighted in APS (41). However, CVD factors’ (e.g., hypertension and dyslipidemia) target achievement was suboptimal in APS, especially in high/very high-risk patients (42). Therefore, modifiable risk factors for arterial events, such as hypertension, diabetes, and hyperuricemia, should be strictly monitored and controlled in patients with APS to reduce the risk of IS.
This study has several limitations. First, it was retrospective rather than prospective, which may have attenuated the significance of the findings. Second, to make the nomogram convenient in clinical practice, we did not incorporate valuable data from other antibodies (e.g., anti-phosphatidylserine/prothrombin), which may lead to some information loss. Thirdly, in our study, only 53.9% (184/334) of thrombotic APS were under long-term anticoagulation. Similarly, in a prospective study of 1000 APS patients, 40.2% received oral anticoagulants during the first 5 years and 37.0% during the second 5 years of the follow-up period (6). Low rates of anticoagulation prescription in patients at high risk of thrombosis (e.g., APS, atrial fibrillation combined with IS) may exist in different regions (6, 43–45), posing a challenge for thrombosis prevention. The possible reasons may be lower levels of education, lower income, and prior antiplatelet use (43). There is no sufficient evidence that anticoagulation reduces the risk of IS in our study. Thus, anticoagulation was not included in the nomogram. More prospective studies are expected to explore the association between anticoagulation and IS incidence. Finally, this study was based on clinical information from a single center’s database and lacked external validation. Hence, we encourage the performance of multicenter studies to further validate the reliability and applicability of the aGAPSS-IS.
The aGAPSS-IS may be a good tool for the identification of patients with APS at high risk of IS based on routinely available data. It may aid physicians in making individualized treatment decisions for patients with APS by weighing the probability of IS occurrence.
The raw data supporting the conclusions of this article will be made available by the authors, without undue reservation.
The studies involving human participants were reviewed and approved by ethics committee at Peking University People’s Hospital. The patients/participants provided their written informed consent to participate in this study.
All authors were involved in the design of this study. CL and JZ conceived the original idea, supervised, and interpreted the result of this work. XS and YF performed the statistical analysis and wrote the manuscript. XS, YF, and JZ were involved in the assessment of ischemic stroke. YJ, GL, and ML contributed to clinical data collection pre-processing. YX gave advice in the statistical analysis and data interpretation. All authors contributed to the article and approved the submitted version.
This work was supported in part by China International Medical Foundation (No. Z-2018-40-2101), National Natural Science Foundation of China (No.81871281), and Beijing Natural Science Foundation (7192211).
The authors of this study would like to thank all the study participants.
The authors declare that the research was conducted in the absence of any commercial or financial relationships that could be construed as a potential conflict of interest.
All claims expressed in this article are solely those of the authors and do not necessarily represent those of their affiliated organizations, or those of the publisher, the editors and the reviewers. Any product that may be evaluated in this article, or claim that may be made by its manufacturer, is not guaranteed or endorsed by the publisher.
1. Miyakis S, Lockshin MD, Atsumi T, Branch DW, Brey RL, Cervera R, et al. International consensus statement on an update of the classification criteria for definite antiphospholipid syndrome (APS). J Thromb Haemost (2006) 4(2):295–306. doi: 10.1111/j.1538-7836.2006.01753.x
2. Muscal E, Brey RL. Neurologic manifestations of the antiphospholipid syndrome: integrating molecular and clinical lessons. Curr Rheumatol Rep (2008) 10(1):67–73. doi: 10.1007/s11926-008-0012-y
3. Cervera R, Piette JC, Font J, Khamashta MA, Cervera R, Piette JC, et al. Antiphospholipid syndrome - clinical and immunologic manifestations and patterns of disease expression in a cohort of 1,000 patients. Arthritis Rheumatol (2002) 46(4):1019–27. doi: 10.1002/art.10187
4. Asherson RA, Khamashta MA, Ordiros J, Derksen R, Machin SJ, Barquinero J, et al. The primary antiphospholipid syndrome - major clinical and serological features. Med (Baltimore) (1989) 68(6):366–74. doi: 10.1097/00005792-198911000-00004
5. Sciascia S, Sanna G, Khamashta MA, Cuadrado MJ, Erkan D, Andreoli L, et al. The estimated frequency of antiphospholipid antibodies in young adults with cerebrovascular events: a systematic review. Ann Rheum Dis (2015) 74(11):2028–33. doi: 10.1136/annrheumdis-2014-205663
6. Cervera R, Serrano R, Pons-Estel GJ, Ceberio-Hualde L, Shoenfeld Y, de Ramon E, et al. Morbidity and mortality in the antiphospholipid syndrome during a 10-year period: a multicentre prospective study of 1000 patients. Ann Rheum Dis (2015) 74(6):1011–8. doi: 10.1136/annrheumdis-2013-204838
7. Collaborators GS. Global, regional, and national burden of stroke, 1990-2016: a systematic analysis for the global burden of disease study 2016. Lancet Neurol (2019) 18(5):439–58. doi: 10.1016/s1474-4422(19)30034-1
8. Collaborators GS. Global, regional, and national burden of stroke and its risk factors, 1990-2019: a systematic analysis for the global burden of disease study 2019. Lancet Neurol (2021) 20(10):795–820. doi: 10.1016/s1474-4422(21)00252-0
9. Otomo K, Atsumi T, Amengual O, Fujieda Y, Kato M, Oku K, et al. Efficacy of the antiphospholipid score for the diagnosis of antiphospholipid syndrome and its predictive value for thrombotic events. Arthritis Rheumatol (2012) 64(2):504–12. doi: 10.1002/art.33340
10. Sciascia S, Sanna G, Murru V, Roccatello D, Khamashta MA, Bertolaccini ML. GAPSS: the global anti-phospholipid syndrome score. Rheumatology (2013) 52(8):1397–403. doi: 10.1093/rheumatology/kes388
11. Sciascia S, Sanna G, Murru V, Roccatello D, Khamashta MA, Bertolaccini ML. The global anti-phospholipid syndrome score in primary APS. Rheumatol (Oxford England) (2015) 54(1):134–8. doi: 10.1093/rheumatology/keu307
12. Hisada R, Kato M, Sugawara E, Fujieda Y, Oku K, Bohgaki T, et al. Thrombotic risk stratification by platelet count in patients with antiphospholipid antibodies: a longitudinal study. J Thromb Haemostasis: JTH (2017) 15(9):1782–7. doi: 10.1111/jth.13763
13. Oku K, Amengual O, Yasuda S, Atsumi T. How to identify high-risk aps patients: clinical utility and predictive values of validated scores. Curr Rheumatol Rep (2017) 19(8):51. doi: 10.1007/s11926-017-0674-4
14. Pierangeli SS, PGd G, Dlott J, Favaloro E, Harris EN, Lakos G, et al. ‘Criteria’ aPL tests: report of a task force and preconference workshop at the 13th international congress on antiphospholipid antibodies, galveston, texas, april 2010. Lupus (2011) 20(2):182–90. doi: 10.1177/0961203310395055
15. Minno MNDD, Scalera A, Tufano A, Ambrosino P, Bettiol A, Silvestri E, et al. The association of adjusted global antiphospholipid syndrome score (agapss) with cardiovascular disease in subjects with antiphospholipid antibodies. Atherosclerosis (2018) 278:60–5. doi: 10.1016/j.atherosclerosis.2018.09.010
16. Radin M, Sciascia S, Erkan D, Pengo V, Tektonidou MG, Ugarte A, et al. The adjusted global antiphospholipid syndrome score (aGAPSS) and the risk of recurrent thrombosis: Results from the APS ACTION cohort. Semin Arthritis Rheumat (2019) 49(3):464–8. doi: 10.1016/j.semarthrit.2019.04.009
17. Radin M, Schreiber K, Cecchi I, Roccatello D, Cuadrado MJ, Sciascia S. The risk of ischaemic stroke in primary antiphospholipid syndrome patients: a prospective study. Eur J Neurol (2018) 25(2):320–5. doi: 10.1111/ene.13499
18. Danowski A, MNLd A, JAdS P, Petri M. Determinants of risk for venous and arterial thrombosis in primary antiphospholipid syndrome and in antiphospholipid syndrome with systemic lupus erythematosus. J Rheumatol (2009) 36(6):1195–9. doi: 10.3899/jrheum.081194
19. D’Agostino RB, Vasan RS, Pencina MJ, Wolf PA, Cobain M, Massaro JM, et al. General cardiovascular risk profile for use in primary care: the framingham heart study. Circulation (2008) 117(6):743–53. doi: 10.1161/circulationaha.107.699579
20. revision Jcfg. 2016 Chinese Guidelines for the management of dyslipidemia in adults. J Geriatric Cardiol: JGC (2018) 15(1):1–29. doi: 10.11909/j.issn.1671-5411.2018.01.011
21. Dalbeth N, Gosling AL, Gaffo A, Abhishek A. Gout. Lancet (2021) 397(10287):1843–55. doi: 10.1016/s0140-6736(21)00569-9
22. Morgan AD, Sharma C, Rothnie KJ, Potts J, Smeeth L, Quint JK. Chronic obstructive pulmonary disease and the risk of stroke. Ann Am Thorac Soc (2017) 14(5):754–65. doi: 10.1513/AnnalsATS.201611-932SR
23. Padda J, Khalid K, Padda S, Boddeti NL, Malhi BS, Nepal R, et al. Hyperuricemia and its association with ischemic stroke. Cureus (2021) 13(9):e18172. doi: 10.7759/cureus.18172
24. Chelluboina B, Vemuganti R. Chronic kidney disease in the pathogenesis of acute ischemic stroke. J Cereb Blood Flow Metab (2019) 39(10):1893–905. doi: 10.1177/0271678x19866733
25. Li C, Zuo Y, Zhang S, Makris UE, Karp DR, Li Z. Additional risk factors associated with thrombosis and pregnancy morbidity in a unique cohort of antiphospholipid antibody-positive patients. Chin Med J (2022). doi: 10.1097/cm9.0000000000001964
26. Hasan TF, Rabinstein AA, Middlebrooks EH, Haranhalli N, Silliman SL, Meschia JF, et al. Diagnosis and management of acute ischemic stroke. Mayo Clinic Proc (2018) 93(4):523–38. doi: 10.1016/j.mayocp.2018.02.013
27. Latchaw RE, Alberts MJ, Lev MH, Connors JJ, Harbaugh RE, Higashida RT, et al. Recommendations for imaging of acute ischemic stroke: a scientific statement from the American heart association. Stroke (2009) 40(11):3646–78. doi: 10.1161/strokeaha.108.192616
28. Markousis-Mavrogenis G, Sfikakis PP, Mavrogeni SI, Tektonidou MG. Combined brain/heart magnetic resonance imaging in antiphospholipid syndrome-two sides of the same coin. Clin Rheumatol (2021) 40(7):2559–68. doi: 10.1007/s10067-020-05498-2
29. Uludag O, Bektas M, Cene E, Sezer M, Sahinkaya Y, Gul A, et al. Validation of the adjusted global antiphospholipid syndrome score in a single centre cohort of APS patients from Turkey. J Thromb Thrombol (2021) 51(2):466–74. doi: 10.1007/s11239-020-02195-4
30. Yousufuddin M, Young N. Aging and ischemic stroke. Aging (2019) 11(9):2542–4. doi: 10.18632/aging.101931
31. Lau LH, Lew J, Borschmann K, Thijs V, Ekinci EI. Prevalence of diabetes and its effects on stroke outcomes: A meta-analysis and literature review. J Diabetes Investig (2019) 10(3):780–92. doi: 10.1111/jdi.12932
32. Li L, McCormick N, Sayre EC, Esdaile JM, Lacaille D, Xie H, et al. Trends of venous thromboembolism risk before and after diagnosis of gout: a general population-based study. Rheumatol (Oxford England) (2020) 59(5):1099–107. doi: 10.1093/rheumatology/kez398
33. Lucchi LD, Nardin C, Sponchiado A, Raggi D, Faggin E, Martini E, et al. Serum uric acid levels and the risk of recurrent venous thromboembolism. J Thromb Haemostasis: JTH (2021) 19(1):194–201. doi: 10.1111/jth.15139
34. Stack AG, Hanley A, Casserly LF, Cronin CJ, Abdalla AA, Kiernan TJ, et al. Independent and conjoint associations of gout and hyperuricaemia with total and cardiovascular mortality. QJM: Month J Assoc Phys (2013) 106(7):647–58. doi: 10.1093/qjmed/hct083
35. Jeong JH, Choi SJ, Ahn SM, Oh JS, Kim Y-G, Lee C-K, et al. Neutrophil extracellular trap clearance by synovial macrophages in gout. Arthritis Res Ther (2021) 23(1):88. doi: 10.1186/s13075-021-02472-4
36. Tambralli A, Gockman K, Knight JS. NETs in APS: current knowledge and future perspectives. Curr Rheumatol Rep (2020) 22(10):67. doi: 10.1007/s11926-020-00936-1
37. Pardos-Gea J, Marques-Soares JR, Buján S, Ordi-Ros J, Alijotas-Reig J. Persistent thrombocytopenia predicts poor long-term survival in patients with antiphospholipid syndrome: a 38-year follow-up study. Rheumatol (Oxford England) (2022) 61(3):1053–61. doi: 10.1093/rheumatology/keab475
38. Shi Y, Zhao J, Jiang H, Huang C, Qi W, Song Y, et al. Thrombocytopenia in primary antiphospholipid syndrome: Association with prognosis and clinical implications. Rheumatol (Oxford) (2022) 135(6):658–64. doi: 10.1093/rheumatology/keac264
39. Artim-Esen B, Diz-Kucukkaya R, Inanc M. The significance and management of thrombocytopenia in antiphospholipid syndrome. Curr Rheumatol Rep (2015) 17(3):10. doi: 10.1007/s11926-014-0494-8
40. Niccolai E, Squatrito D, Emmi G, Silvestri E, Emmi L, Ciucciarelli L, et al. A new cytofluorimetric approach to evaluate the circulating microparticles in subjects with antiphospholipid antibodies. Thromb Res (2015) 136(6):1252–8. doi: 10.1016/j.thromres.2015.10.018
41. Drosos GC, Vedder D, Houben E, Boekel L, Atzeni F, Badreh S, et al. EULAR recommendations for cardiovascular risk management in rheumatic and musculoskeletal diseases, including systemic lupus erythematosus and antiphospholipid syndrome. Ann Rheum Dis (2022) 81(6):768–79. doi: 10.1136/annrheumdis-2021-221733
42. Bolla E, Tentolouris N, Sfikakis PP, Tektonidou MG. Cardiovascular risk management in antiphospholipid syndrome: trends over time and comparison with rheumatoid arthritis and diabetes mellitus. Lupus Sci Med (2021) 8(1):e000579. doi: 10.1136/lupus-2021-000579
43. Gu HQ, Yang X, Wang CJ, Zhao XQ, Wang YL, Liu LP, et al. Assessment of trends in guideline-based oral anticoagulant prescription for patients with ischemic stroke and atrial fibrillation in china. JAMA Netw Open (2021) 4(7):e2118816. doi: 10.1001/jamanetworkopen.2021.18816
44. Djokovic A, Stojanovich L, Stanisavljevic N, Djokic S, Filipovic B, Matic P, et al. Cardiac manifestations in primary antiphospholipid syndrome and their association to antiphospholipid antibodies’ types and titers-cross-sectional study of Serbian cohort. Clin Rheumatol (2022) 41(5):1447–55. doi: 10.1007/s10067-022-06056-8
Keywords: antiphospholipid syndrome, adjusted Global Anti-Phospholipid Syndrome Score, ischemic stroke, nomogram, risk stratification
Citation: Song X, Fan Y, Jia Y, Li G, Liu M, Xu Y, Zhang J and Li C (2022) A novel aGAPSS-based nomogram for the prediction of ischemic stroke in patients with antiphospholipid syndrome. Front. Immunol. 13:930087. doi: 10.3389/fimmu.2022.930087
Received: 27 April 2022; Accepted: 13 July 2022;
Published: 29 July 2022.
Edited by:
Rohan Willis, University of Texas Medical Branch at Galveston, United StatesReviewed by:
Aleksandra Djokovic, Bezanijska Kosa, University Medical Center, SerbiaCopyright © 2022 Song, Fan, Jia, Li, Liu, Xu, Zhang and Li. This is an open-access article distributed under the terms of the Creative Commons Attribution License (CC BY). The use, distribution or reproduction in other forums is permitted, provided the original author(s) and the copyright owner(s) are credited and that the original publication in this journal is cited, in accordance with accepted academic practice. No use, distribution or reproduction is permitted which does not comply with these terms.
*Correspondence: Chun Li, MTM4MTExOTAwOThAMTYzLmNvbQ==; Jun Zhang, emhhbmdqdW5AcGt1cGguZWR1LmNu
†These authors have contributed equally to this work and share first authorship
Disclaimer: All claims expressed in this article are solely those of the authors and do not necessarily represent those of their affiliated organizations, or those of the publisher, the editors and the reviewers. Any product that may be evaluated in this article or claim that may be made by its manufacturer is not guaranteed or endorsed by the publisher.
Research integrity at Frontiers
Learn more about the work of our research integrity team to safeguard the quality of each article we publish.