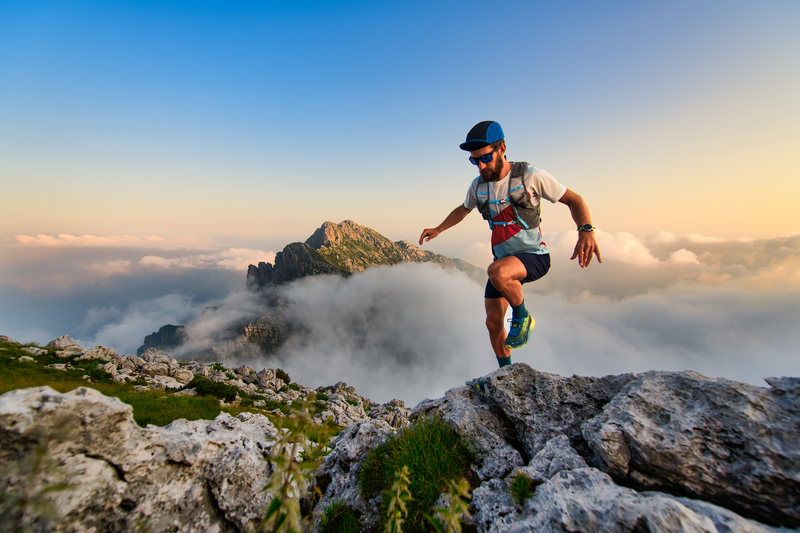
95% of researchers rate our articles as excellent or good
Learn more about the work of our research integrity team to safeguard the quality of each article we publish.
Find out more
ORIGINAL RESEARCH article
Front. Immunol. , 09 June 2022
Sec. Vaccines and Molecular Therapeutics
Volume 13 - 2022 | https://doi.org/10.3389/fimmu.2022.887219
This article is part of the Research Topic Insights in Vaccines and Molecular Therapeutics: 2021 View all 11 articles
Concerted efforts to fight malaria have caused significant reductions in global malaria cases and mortality. Sustaining this will be critical to avoid rebound and outbreaks of seasonal malaria. Identifying predictive attributes that define clinical malaria will be key to guide development of second-generation tools to fight malaria. Broadly reactive antibodies against variable surface antigens that are expressed on the surface of infected erythrocytes and merozoites stage antigens are targets of naturally acquired immunity and prime candidates for anti-malaria therapeutics and vaccines. However, predicting the relationship between the antigen-specific antibodies and protection from clinical malaria remains unresolved. Here, we used new datasets and multiple approaches combined with re-analysis of our previous data to assess the multi-dimensional and complex relationship between antibody responses and clinical malaria outcomes. We observed 22 antigens (17 PfEMP1 domains, 3 RIFIN family members, merozoite surface protein 3 (PF3D7_1035400), and merozoites-associated armadillo repeats protein (PF3D7_1035900) that were selected across three different clinical malaria definitions (1,000/2,500/5,000 parasites/µl plus fever). In addition, Principal Components Analysis (PCA) indicated that the first three components (Dim1, Dim2 and Dim3 with eigenvalues of 306, 48, and 29, respectively) accounted for 66.1% of the total variations seen. Specifically, the Dim1, Dim2 and Dim3 explained 52.8%, 8.2% and 5% of variability, respectively. We further observed a significant relationship between the first component scores and age with antibodies to PfEMP1 domains being the key contributing variables. This is consistent with a recent proposal suggesting that there is an ordered acquisition of antibodies targeting PfEMP1 proteins. Thus, although limited, and further work on the significance of the selected antigens will be required, these approaches may provide insights for identification of drivers of naturally acquired protective immunity as well as guide development of additional tools for malaria elimination and eradication.
There is a common agreement that sustainable elimination of malaria will require multiple approaches (1). The need to develop efficacious vaccines remains an integral component of this fight, supported by strong evidence showing that naturally acquired protective immunity against Plasmodium falciparum is acquired among individuals living in malaria-endemic regions (2, 3). Specifically, antibody-mediated immunity against P. falciparum malaria is acquired with age and repeated exposure (2). This immunity mainly targets antigens of the parasite asexual blood-stages; however, the complete repertoire of the specific targets has not been unequivocally defined. Based on this understanding, several approaches are being applied to select the most appropriate vaccine candidates.
Focused and unbiased immuno-epidemiology studies have reported, identified, or characterized most of the leading candidate vaccines in the developmental pipeline. Most recently, high-throughput immunoscreening to simultaneously investigate proteins as potential vaccine candidates or as immune correlates of protection has been a major strategy (4–7) with antigens such as PfRh5, CelTOS, MSP3, and GLURP (7–9) being identified. These antigens are in the vaccine development pipeline. However, most data, to date has limited our ability to prioritize antigens, enrich the pool of vaccine candidates, and link immunological data with clinical outcomes.
Over the years, we have developed and optimized a robust and high-throughput eukaryotic wheat germ cell-free protein synthesis system (WGCFS) coupled with a homogeneous high-throughput AlphaScreen platform for antibody profiling and mechanistic characterization of proteins that have a role in merozoites invasion of erythrocytes, or induction of protective immunity in malaria naturally exposed individuals (9–11). Leveraging this approach, we have prioritized, from hundreds of parasite proteins expressed in multiple parasite stages, several P. falciparum antigens for inclusion in the vaccine development pipeline. Recently, a total of 1,827 recombinant proteins drawn from different P. falciparum stages (sporozoites, merozoites, trophozoites, schizonts, and gametocytes) were used to probe individual serum samples obtained from residents of a malaria endemic region in Uganda. Protein immunoreactivity was observed at 54% with 128 antigens inducing antibody responses that significantly associated with reduced risk to clinical malaria episodes (defined as fever ≥37.5 ˚C and asexual parasitemia of ≥2,500/µl of blood) during a 12-months follow-up period. Of these antigens, 53 were down-selected as the most viable vaccine candidates by virtue of having a signal peptide (SP) and/or transmembrane domain (TM) (4, 5, 7) suggesting their putative expression on the surface of merozoites and/or sporozoites, or on the infected erythrocytes. Similarly, by focusing on parasite protein families that are exported to the surface of infected red blood cells such as erythrocyte membrane protein 1 (PfEMP1), repetitive interspersed family (RIFIN) proteins, subtelomeric variable open reading frame (STEVOR), and surface-associated interspersed gene family (SURFIN) (12), we observed that more than 95% of the antigens were reactive with serum samples obtained from Uganda (11, 13). These studies demonstrated that the repertoire of potentially protective antigens that correlated with protective immunity against clinical malaria is wider than thought and offers multiple options for the identification of malaria vaccine candidates, aside from those that are currently under clinical or pre-clinical evaluation (9).
Previous studies with our follow-up cohort in Uganda (14) and similar immunoepidemiology studies (4, 7, 15) have largely focused on a single definition of clinical malaria based on the incidence within a specified geographic area. However, ongoing field trials/studies further strengthen the argument that clinical definitions of malaria are also influenced by factors that are related to host immunity (age, transmission, co-infections, etc), and parasitaemia accompanied by symptoms do not necessarily imply clinical malaria especially in endemic areas (16–19). Moreover, malaria transmission heterogeneity (ranging from high, stable, and declining), as well as absence of robust correlates of protection highlights the complexity involved in evaluating new and future interventions. In this study, to obtain a wider picture, and considering the declining malaria transmission in endemic areas, we sought to re-evaluate the previously published data together with new data sets generated against merozoites stage antigens that are considered potential targets of protective immunity, to assess the relationship between antibody responses and clinical malaria outcomes categorized by different clinical malaria definitions. Further, using the Principal Component Analysis (PCA) for the antibody responses we highlight antigens that are robustly identified by different protein based global immunological screens.
Serum samples used in this study were obtained from residents of Lira Municipality, Northern Uganda, that were taking part in a prospective study of 66 non-vaccinated participants aged 6–20 years. The region was characterized by high malaria transmission (14, 20–23). Study protocols and permission to use the samples were approved by the Institutional Research and Ethics Committee of Lacor Hospital (LHIREC 023/09/13), Uganda National Council for Science and Technology (HS1403) in Uganda; and Ethics Committees of the Research Institute for Microbial Diseases, Osaka University, and Ehime University, Japan. Written informed consents were obtained from all participants and/or their parents or guardians before the study. Aside from parental consent, assent was obtained from children aged 8-17 years. The study was conducted in compliance with the International Conference on Harmonisation Good Clinical Practices guidelines and the Declaration of Helsinki.
The comprehensive protein library consisted of 579 proteins representing the asexual erythrocytic stages of P. falciparum (Table S1). Newly synthesized asexual blood-stage proteins (BSP: n = 46) selected from our previous study based on their significant immunoreactivities (9) were asssessd together with data from previously published libraries of proteins derived from cysteine-rich interdomain regions of P. falciparum erythrocyte membrane protein 1 (PfEMP1) (CIDR: n = 108), Duffy binding–like domains of PfEMP1 (DBL: n = 163) (11), repetitive interspersed family proteins (RIFIN: n = 176), subtelomeric variable open reading frame proteins (STEVOR: n = 53), and surface-associated interspersed gene family proteins (SURFIN: n = 33) (13). All proteins were synthesized using the optimized WGCFS protocol and assayed alongside biotinylated rabbit IgG standard for plate-to-plate and day-to-day normalization (11).
All the proteins were expressed from sequences derived from the P. falciparum 3D7 reference strain. Briefly, the DNA sequences representing the ectodomains while excluding the SP and/or TM domains were amplified by using high fidelity PrimeSTAR Max DNA polymerase (Takara Bio, Kusatsu, Japan) and cloned into the WGCFS dedicated pEU plasmid vector (CellFree Sciences, Matsuyama, Japan) using the In-Fusion HD Cloning Kit (Takara Bio). A semi-automated GenDecoder1000 robotic protein synthesizer (CellFree Sciences) was used for in vitro transcription and mono-biotinylated recombinant protein synthesis with WGCFS.
We used the AlphaScreen platform to assess serum antibodies in malaria exposed individuals as described (9, 24). The system exploits the existence of mono-biotinylation on each recombinant P. falciparum protein. Specifically, the proteins were dispensed into a 384-well OptiPlate using a JANUS Automated Workstation dispenser (PerkinElmer, Waltham, MA) and mixed with 10 μl of 4000-fold diluted sera in reaction buffer (100 mM Tris-HCl [pH 8.0], 0.01% [v/v] Tween-20, and 0.1% [w/v] bovine serum albumin). After 30 min incubation at 26°C, 10 μl of detection mixture containing streptavidin-coated donor beads (PerkinElmer) and protein G (Thermo Scientific, Waltham, MA) conjugated acceptor beads (PerkinElmer) were added, to make a final concentration of 12 μg/ml for both beads. The plate was then incubated in the dark for 1 hr at 26°C to allow optimal binding of the donor and acceptor beads to the biotinylated protein and human antibody, respectively. Luminescence emitted by acceptor beads upon excitation of the donor beads was detected using an EnVision plate reader (PerkinElmer) and captured as AlphaScreen Counts (ASC). To account for day-to-day and plate-to-plate assay variability, serially diluted biotinylated rabbit IgG (PerkinElmer) was included in each plate, and subsequently used to generate a 5-parameter logistic standardization curve. The assays were randomized to minimize experimental bias.
All data analyses were performed using R software (Version 4.0.1, R Foundation for Statistical Computing). As previously described, protein seropositivity cut off point to human sera was set at half the lowest non-negative ASC value from that of the assayed samples (25), and a protein was considered immunoreactive if more than 10% of the volunteers had ASC levels above the seropositivity cut-off.
For survival analysis, the time-to-first clinical malaria episode from baseline (defined as the time from first sampling when all enrolled individuals were blood-smear negative) was used as the endpoint (14). For detailed analyses, three different definitions for clinical malaria were used: fever ≥37.5°C and asexual parasitemia of (i) ≥1,000/μl, (ii) ≥2,500/μl, and (iii) ≥5,000/μl blood, with no sign(s) of complicated disease. Although some children may have multiple parasitic or febrile malaria episodes as defined above, only the first febrile episode was considered in the analysis. To assess whether the presence of antigen-specific antibodies was associated with overall survival, Cox proportional hazards model was used to calculate hazard ratios (HR) and 95% confidence intervals (CIs) between ‘High Responders’ (individuals with an ASC value above the population median for that antigen) or ‘Low Responders’ (7, 9, 26, 27). Potential protective efficacy (PPE) relative to different definitions of malaria was computed as 1- hazard ratio (PPE% = (1- HR) × 100%). For multivariate survival analysis, data was adjusted for bednet use and age as a categorical variable (6-10 years, 11-15 years, and 16-20 years).
To simultaneously evaluate antibodies developed in response to different proteins among patients with and without clinical malaria, we used Principal Component Analysis (PCA). PCA essentially reduces the dimensionality of antibody responses by generating fewer composite variables to capture as much variance in that dataset. The model then assesses and compares the relationship between the scores obtained for each of these components per subject. To generate the global antigen derived principal components, all immunoreactive antigens were used without prespecified groupings (BSP, PfEMP1-CIDR, PfEMP1-DBL, RIFIN, STEVOR, and SURFIN) to determine group-based variance. To identify PCA derived clusters of antibody responses that were involved in protection against clinical malaria, we selected principal components where at least three variables were loaded, and the eigenvalue was greater than 2 or the proportion of variance explained was >5% (28). Individuals displaying outlier PCA coordinates were excluded. Individual contributions to the PCA were assessed in association with age, gender, and clinical malaria outcome.
We assessed the human antibody reactivities to the protein library (Table S1) obtained from the samples (n = 66) taken at the beginning of the study, prior to the rainy season. All the blood-stage proteins (BSP) were newly measured in this study and they were all immunoreactive with seroprevalence above the threshold cut-off point of 10%. Seroprevalence of the other protein groups was reported previously (11, 13). Briefly, 99% immunoreactivity was observed for PfEMP1 (CIDR and DBL), RIFIN, STEVORs, and SURFINs (11, 13) (Figure 1). The seroprevalence varied widely among the protein groups/families: BSP (16-100%), CIDR domains (22-92%), DBL domains (12-100%), RIFIN (10-93%), STEVORs (12-83%) and SURFINs (21-100%) (Kruskal-Wallis Test, p = 0.001) with STEVORs having significantly lower median levels, and SURFINs the highest compared to other groups. Although this may represent cross-reactivity within the assessed families (12), a strong Spearman’s rank correlation between antibody levels measured in the samples and across different groups, as shown by the correlation matrix (Figure S1) suggests that antibodies to different antigens are co-acquired in this population.
Figure 1 Seroprevalence of antibodies to different P. falciparum proteins. Antibodies immunoreactive to BSP (blood-stage proteins), CIDR (cysteine-rich interdomain regions of PfEMP1), DBL (Duffy binding–like domains of PfEMP1), RIFIN (repetitive interspersed family proteins), STEVOR (subtelomeric variable open reading frame proteins), and SURFIN (surface-associated interspersed gene family proteins) are shown. Box plots illustrate medians with 25th and 75th and whiskers for 10th and 90th percentiles with the horizontal line in each group denoting the overall median per group. The dashed red horizontal line indicates 10% seroprevalence that was set as protein immunoreactivity cut-off point. The data in BSP were newly measured in this study. The data in other protein groups, CIDR and DBL from PfEMP1, RIFIN, STEVOR and SURFIN were reported previously (11, 13).
To gain further insight into the relationship between individual protein reactivity and clinical malaria outcomes, we determined the hazard ratios based on time-to-event using three parasite thresholds for clinical malaria in univariate analysis. Based on the lower threshold of 1,000 parasites/µl blood, antigen-specific antibodies to 43 proteins associated with protection. This changed to 26 and 32 proteins when assessed at 2,500 parasites/µl blood and a higher threshold of 5,000 parasites/µl blood, respectively (Figure 2A). In the multivariable-adjusted survival analysis, the correlation between antibodies and the risk of febrile malaria was generally reduced. Twenty-two antigens were selected across the three definitions (Table 1) by the unadjusted analysis. The 22 antigens included 17 PfEMP1 domains, 3 RIFIN family members, merozoite surface protein 3 (PF3D7_1035400), and merozoite-associated armadillo repeats protein (PF3D7_1035900). The number of antigens that remained significantly associated with reduced risk of clinical malaria after adjusting for age (24) and bed-net use was 2 for 1,000 parasites/µl blood, 1 for 2,500 parasites/µl blood, and 2 antigens for 5,000 parasites/µl blood (summarized in Figure 2B).
Figure 2 Associations between antibody levels and risk of P. falciparum febrile malaria. Complete list of the data for potential protective efficacy (PPE), and 95% confidence interval, is provided in Table 1. (A) Unadjusted association between antibody levels and risk of P. falciparum febrile malaria for each of the antigens tested in the cohort. The top-50 antigens are ranked - top to bottom - by the strength of their PPE. PPE for each antigen was derived from the hazard ratio (HR) calculated by the unadjusted Cox-regression hazard model analysis (comparing children with high vs. low antibody responses). Black dots indicate the percentage protection, and error bars indicate the 95% confidence interval. The red vertical line represents a PPE of 0% (i.e. HR = 1). The 22 cross-selected antigens are labeled in bold. (B) The bars represent the number of antigens that associated with protection to clinical malaria in univariate and multivariate analysis. Red bar: Adjusted, Blue bar: Unadjusted.
Since the antibody responses and the corresponding semi-protective immunity involve numerous antibodies acting simultaneously but targeting different proteins (26, 29), we performed PCA with all the proteins assayed and with different protein families to capture the effects of all antibodies in a single analysis and extract important associations. The analysis indicated that the first three components (with eigenvalues of 306, 48, and 29, respectively) accounted for 66.1% of the total variation in these data (Figure 3A). The analysis showed that the participants who did not experience clinical malaria were more dispersed while those with malaria episodes clustered together (Figures 3B–D). The first component (Dim1) explained 52.8% of variability and gave the greatest weights to antibodies against PfEMP1 domains [PF3D7_1240300_DBLβ8 (contributing 0.3), PF3D7_0632500_DBLe2 (0.3), PF3D7_0200100_CIDRα2.2 (0.29), PF3D7_1000300 (0.29) and PF3D7_1373500_CIDRβ6 (0.28)] (Table 2; Figure S2), while principal component 2 (Dim2) was mainly reflective of anti-PF3D7_1372800 (0.78), PF3D7_0401400 (0.77), PF3D7_0632100 (0.74), PF3D7_0500400 (0.72), and PF3D7_0425900 (0.67) and accounted for 8.2% of the variation. The third component (Dim3), which explained slightly above 5.0% of data variability, gave the strongest weight to antibodies against PF3D7_1200100_DBLα0.9 (2.44), PF3D7_1150400_CIDRγ2 (2.33), PF3D7_0712300_CIDRα0.1 (2.21), PF3D7_0400400_DBLδ1 (2.07), and PF3D7_1200400_DBLγ14 (1.86) (Table 2; Figure S2). These findings suggest that PfEMP1 domains are major determinants of the variability observed in individuals experiencing clinical malaria and are consistent with the findings of the Cox analysis model (Figure 2A). Specifically, the PF3D7_0425800_DBLβ3 was the only domain selected by the multi-approach analysis and appeared as well in the top 50 antigens by PCA.
Figure 3 Principal Components Analysis (PCA) of the antibody responses. (A) Distribution of principal components (first 10 dimensions) that explained the highest variance of the antibody responses derived from all the immunoreactive proteins. (B–D) The plots of the distribution of individuals by type of antibody responses to different proteins. The principal components (Dim)1 vs 2 (B), Dim1 vs 3 (C), and Dim2 vs 3 (D) that explained the highest percentage of the variance (percentage in parenthesis) of the antibody responses are presented. Green and red represent malaria (M) and no malaria (NM) cases, respectively. Light green and light red eclipses represent distribution of individuals with and without clinical malaria episodes, respectively.
Table 2 Top 50 antigens based on respective contributions to variability in principal components 1, 2 and 3 (Dim1, Dim2, Dim3).
When assessing the representation of antibody responses to different protein groups based on Dim1 and Dim2, we observed that most clinical malaria cases clustered together irrespective of the protein group or family (Figure S3); and both components are the major drivers of the observed variability. This was again in agreement with the observation in Cox analysis which suggested that multiple, and probably specific antigens may be associated with protective immunity (Figure 2A).
We then assessed the relationship between the scores obtained from PCA on one hand and age and malaria clinical outcome to identify patterns of specific antibody responses that could be important in malaria. Age was significantly positively correlated with the scores of the first principal component (Dim1, R = 0.5; P <0.001) but not with Dim2 (R = 0.04, P = 0.8) or Dim3 (R = -0.05, P = 0.7) (Figure 4). This, further strengthens the observation on previous studies that age is an important factor on naturally acquired immunity (2).
Figure 4 Associations between the antibody responses and age. Correlation between the scores of the principal components 1–3 and age (in years) of the participants. Blue line represent linear regression lines and shading represents 95% confidence intervals. Only the first principal component (Dim1) correlated with age.
Here, using antibody response data from our previous publications on multi-gene families (9, 11, 13) plus newly generated data from merozoite surface antigens, and expanding the analysis to include wider definition of clinical malaria (categorized according to different parasitemia thresholds), we re-assessed antibody responses in a malaria exposed population in Northern Uganda to capture as much immunological information as possible within the context of protective immunity from one population. This comprehensive approach would help identify further key antigenic targets or signature(s) of protective immunity. We observed 22 antigens, that included 17 PfEMP1 domains, 3 RIFIN family members, merozoite surface protein 3 (MSP3; PF3D7_1035400), and merozoite-associated armadillo repeats protein (PfMAAP; PF3D7_1035900), selected by the three clinical malaria definitions (1,000/2,500/5,000 parasites/µl blood plus fever). This suggest that these proteins have a key role in protection against clinical malaria.
We observed strong immune responses with high seroprevalence in the different protein families. This could be due to high level of exposure to these abundantly expressed proteins or cross-reactivity among the conserved regions of the domains (12). Although it could be argued that, likely, only highly expressed proteins can be flagged down in terms of antigen discovery and could be restrictive, the potential cross-reactivity could also be a strong point in terms of cross-protection. For instance, recent data suggests that despite their huge sequence diversity, CIDRα1 are structurally and functionally conserved for binding to EPCR (30, 31). Importantly, CIDRα1-EPCR interaction can be blocked by antibodies obtained from individuals naturally infected with malaria in Tanzania (30). The function of other multi-gene families in malaria infection and pathogenicity, to date, remains unclear but it is expected that the parasite could be using these proteins as alternative ligands to evade human immune system for host colonization.
Among them, PF3D7_0425800_DBLβ3, a PfEMP1 domain was selected by the multi-definition analyses, and appeared as well in the top 50 antigens by PCA. The molecule is a domain cassette 4 (DC4) family member consisting of a combination of short tandems that is associated with severe malaria (32–34). Antibodies against DBLβ3 can broadly inhibit PfEMP1 binding to intercellular adhesion molecule 1 (ICAM1) and are cross-reactive to DC4 derived from genetically distant parasite isolates (35). DBLβ3 was also identified in the previous analyses (32, 34) and the approach used here affirmed the importance of this domain in protection against symptomatic malaria. In addition, 3 members of the RIFIN family were selected. This is consistent with recent data which pointed to an important role of these molecules as ligands for opsonization of infected RBCs (36). Studies have suggested that anti-RIFIN antibodies correlate with parasite clearance, abrogation of symptoms of clinical malaria in children (37), and have a role in protection against severe malaria in Tanzanian patients (38). However, we do note that targeting RIFIN alone, as with PfEMP1 domains, for vaccine/as possible intervention tools may not be sufficient or highly effective due to the clonal expression of these proteins (39). We hypothesize that cross-conserved domains, either linear or structural, within the RIFINs may offer better and wider protective base than targeting only a single protein or domain. A recent study has pointed that this is indeed possible as broadly reactive antibodies were generated through insertion of a large DNA fragment between V and DJ segments of antigen binding domains able to recognize RIFINs of different P. falciparum isolates (36). Identification and characterization of the cross-protective mechanisms need to be critically pursued.
MSP3 was first identified in 1994 (40), has been a well-known target of naturally acquired immunity, and has been considered to be a promising asexual blood-stage malaria vaccine candidate (41). After the decades of research and development efforts, MSP3 is now under the clinical development as GMZ2, an asexual blood-stage malaria vaccine in combination with another surface antigen, P. falciparum glutamate-rich protein (GLURP) (42–44). PfMAAP was recently identified by Aniweh et al. (45). Recently studied for its viability as vaccine candidate, PfMAAP was found localized to the apical region of merozoites, anti-PfMAAP antibodies inhibited merozoite invasion of erythrocytes in vitro, and naturally acquired human antibodies to the conserved N- and C- terminal regions of PfMAAP were associated with reduced risk to malaria. Independent analyses and association with protection in this study supports the vaccine candidacy of both MSP3 and PfMAAP, similar to the highly immunogenic erythrocyte surface antigens.
The need for continued development of robust malaria intervention tools including vaccines across different malaria endemicities (46) calls for “flexible” definitions of clinical malaria (47). Moreover, given that malaria can be defined in very diverse ways since clinical presentations vary widely, it is not obvious what aspects are key to supporting accurate monitoring in the face of changing epidemiology (48). We show that three different parasitemia cut-off points yielded almost similar results (Figure 2A) suggesting that, for immunological studies, combination of antigens provide an important signature that can be true in different geographical settings. We do acknowledge that there are challenges to attribute fever (or other symptoms) with observed parasitemia (49) and additional studies will be needed to validate or optimize our findings in different settings and in a larger dataset.
We investigated whether a specific combination of antibody responses to the recombinant proteins evaluated could be associated with key malaria severity factors such as age. PCA is useful for exploring multi-dimensional data, mixed infections and complex host-parasite interactions. To this end, and to capture the joint effects of all antibodies in a single analysis in biological conditions, we investigated the relationship between the overall antibody responses by PCA, a factor analysis approach accommodating the fact that protective antibody responses are likely to involve a number of antibodies working together. With the current dataset, the first three principal components accounted for the majority of the outcomes observed (Figure 3A). The analyses only showed a significant relationship between first (Dim1) component scores and age; with PfEMP1 domains being the top contributing variables (Figure 4; Table 2). This is consistent with a recent proposal suggesting that there are ordered acquisition of antibodies targeting PfEMP1 (50). Taken together, these findings suggest that antibodies to multiple proteins may be acquired with age, and continuous exposure is a key factor in protection against clinical disease and protective immunity (2).
Multiple P. falciparum protein microarrays have been designed over the past decade to identify malaria vaccine candidate antigens. One of the most important characteristic in this study is the use of WGCFS for recombinant protein production. In contrast to E. coli- (5, 51) and mammalian cell-based protein microarray (52) systems which may cause an artefactual change in protein folding (53), WGCFS can express large and complex non-glycosylated proteins in their near-native forms without codon optimization (54). The AlphaScreen immunoscreening platform does not require protein purification and conjugation. Based on these characteristics, therefore, WGCFS/AlphaScreen platform provides a rapid, straightforward tool for screening and identification of parasite antigenic targets as well as protein-protein interactions important for immunity and pathogenesis (55–57), overcoming most challenges attributed to the E. coli-based systems (58, 59) resulting to robust evaluations of immunoreactivities across different proteins families and groups.
In conclusion, the large platform of malaria antigens and the analysis approach applied in this study improved our ability to interrelate immunological data with clinical outcomes and highlighted antigens for future work aimed towards developing additional tools for malaria elimination and eradication.
The original contributions presented in the study are included in the article/Supplementary Material. Further inquiries can be directed to the corresponding authors.
The studies involving human participants were reviewed and approved by Institutional Review Committees of Ehime University and Institutional Review Committees of Research Institute for Microbial Diseases, Osaka University, Japan. In Uganda, the studies were reviewed and approved by Lacor Hospital (LHIREC 023/09/13), and Uganda National Council for Science and Technology (HS866, HS1403). Written informed consent to participate in this study was provided by the participants’ legal guardian/next of kin.
ET and BK conceived and designed experiments. HN, MM, and IH conducted experiments. ET, BK, and TT analyzed the data. ET, BK, NP, TE, TH, JG, and TT wrote the manuscript. All authors discussed and edited the manuscript.
This work was funded in part by JSPS KAKENHI (grant numbers JP20H03481, JP21H02724, JP21KK0138) and in part by Strategic Promotion of International Cooperation to Accelerate Innovation in Africa by MEXT, Japan. BK is an EDCTP Fellow under EDCTP2 programme supported by the European Union grant number TMA2020CDF-3203. The funders had no role in study design, data collection and analysis, decision to publish, or preparation of the manuscript.
The authors declare that the research was conducted in the absence of any commercial or financial relationships that could be construed as a potential conflict of interest.
All claims expressed in this article are solely those of the authors and do not necessarily represent those of their affiliated organizations, or those of the publisher, the editors and the reviewers. Any product that may be evaluated in this article, or claim that may be made by its manufacturer, is not guaranteed or endorsed by the publisher.
We appreciate the study volunteers from Lira, Northern Uganda; and thank the research teams from Med Biotech Laboratories, Lira Medical Center, Uganda, and Research Institute for Microbial Diseases, Osaka University for their technical assistance in obtaining the field samples.
The Supplementary Material for this article can be found online at: https://www.frontiersin.org/articles/10.3389/fimmu.2022.887219/full#supplementary-material
1. WHO. Global Technical Strategy for Malaria 2016-2030, 2021 Update Vol. Vol. 2021. Geneva, Switzerland: World Health Organization (2021).
2. Doolan DL, Dobano C, Baird JK. Acquired Immunity to Malaria. Clin Microbiol Rev (2009) 22:13–36. doi: 10.1128/CMR.00025-08
3. Barry A, Hansen D. Naturally Acquired Immunity to Malaria. Parasitology (2016) 143:125–8. doi: 10.1017/S0031182015001778
4. Osier FH, Mackinnon MJ, Crosnier C, Fegan G, Kamuyu G, Wanaguru M, et al. New Antigens for a Multicomponent Blood-Stage Malaria Vaccine. Sci Transl Med (2014) 6:247ra102. doi: 10.1126/scitranslmed.3008705
5. Crompton PD, Kayala MA, Traore B, Kayentao K, Ongoiba A, Weiss GE, et al. A Prospective Analysis of the Ab Response to Plasmodium falciparum Before and After a Malaria Season by Protein Microarray. Proc Natl Acad Sci USA (2010) 107:6958–63. doi: 10.1073/pnas.1001323107
6. Doolan DL, Mu Y, Unal B, Sundaresh S, Hirst S, Valdez C, et al. Profiling Humoral Immune Responses to P. falciparum Infection With Protein Microarrays. Proteomics (2008) 8:4680–94. doi: 10.1002/pmic.200800194
7. Richards JS, Arumugam TU, Reiling L, Healer J, Hodder AN, Fowkes FJ, et al. Identification and Prioritization of Merozoite Antigens as Targets of Protective Human Immunity to Plasmodium falciparum Malaria for Vaccine and Biomarker Development. J Immunol (2013) 191:795–809. doi: 10.4049/jimmunol.1300778
8. Dodoo D, Aikins A, Kusi KA, Lamptey H, Remarque E, Milligan P, et al. Cohort Study of the Association of Antibody Levels to AMA1, MSP119, MSP3 and GLURP With Protection From Clinical Malaria in Ghanaian Children. Malaria J (2008) 7:142. doi: 10.1186/1475-2875-7-142
9. Kanoi BN, Takashima E, Morita M, White MT, Palacpac NM, Ntege EH, et al. Antibody Profiles to Wheat Germ Cell-Free System Synthesized Plasmodium falciparum Proteins Correlate With Protection From Symptomatic Malaria in Uganda. Vaccine (2017) 35:873–81. doi: 10.1016/j.vaccine.2017.01.001
10. Ntege EH, Takashima E, Morita M, Nagaoka H, Ishino T, Tsuboi T. Blood-Stage Malaria Vaccines: Post-Genome Strategies for the Identification of Novel Vaccine Candidates. Expert Rev Vaccines (2017) 16:769–79. doi: 10.1080/14760584.2017.1341317
11. Kanoi BN, Nagaoka H, Morita M, White MT, Palacpac NMQ, Ntege EH, et al. Comprehensive Analysis of Antibody Responses to Plasmodium falciparum Erythrocyte Membrane Protein 1 Domains. Vaccine (2018) 36:6826–33. doi: 10.1016/j.vaccine.2018.08.058
12. Chan JA, Fowkes FJ, Beeson JG. Surface Antigens of Plasmodium falciparum-Infected Erythrocytes as Immune Targets and Malaria Vaccine Candidates. Cell Mol Life Sci (2014) 71:3633–57. doi: 10.1007/s00018-014-1614-3
13. Kanoi BN, Nagaoka H, White MT, Morita M, Palacpac NMQ, Ntege EH, et al. Global Repertoire of Human Antibodies Against Plasmodium falciparum RIFINs, SURFINs, and STEVORs in a Malaria Exposed Population. Front Immunol (2020) 11:893. doi: 10.3389/fimmu.2020.00893
14. Palacpac NM, Ntege E, Yeka A, Balikagala B, Suzuki N, Shirai H, et al. Phase 1b Randomized Trial and Follow-Up Study in Uganda of the Blood-Stage Malaria Vaccine Candidate BK-Se36. PLoS One (2013) 8:e64073. doi: 10.1371/journal.pone.0064073
15. Stanisic DI, Fowkes FJ, Koinari M, Javati S, Lin E, Kiniboro B, et al. Acquisition of Antibodies Against Plasmodium falciparum Merozoites and Malaria Immunity in Young Children and the Influence of Age, Force of Infection, and Magnitude of Response. Infect Immun (2015) 83:646–60. doi: 10.1128/IAI.02398-14
16. Bejon P, Williams TN, Liljander A, Noor AM, Wambua J, Ogada E, et al. Stable and Unstable Malaria Hotspots in Longitudinal Cohort Studies in Kenya. PLoS Med (2010) 7:e1000304. doi: 10.1371/journal.pmed.1000304
17. Greenwood BM, Armstrong JR. Comparison of Two Simple Methods for Determining Malaria Parasite Density. Trans R Soc Trop Med Hyg (1991) 85:186–8. doi: 10.1016/0035-9203(91)90015-Q
18. Schellenberg JR, Smith T, Alonso PL, Hayes RJ. What Is Clinical Malaria? Finding Case Definitions for Field Research in Highly Endemic Areas. Parasitol Today (1994) 10:439–42. doi: 10.1016/0169-4758(94)90179-1
19. Mwangi TW, Ross A, Snow RW, Marsh K. Case Definitions of Clinical Malaria Under Different Transmission Conditions in Kilifi District, Kenya. J Infect Dis (2005) 191:1932–9. doi: 10.1086/430006
20. Egwang TG, Apio B, Riley E, Okello D. Plasmodium falciparum Malariometric Indices in Apac District, Northern Uganda. East Afr Med J (2000) 77:413–6.
21. Proietti C, Pettinato DD, Kanoi BN, Ntege E, Crisanti A, Riley EM, et al. Continuing Intense Malaria Transmission in Northern Uganda. Am J Trop Med Hyg (2011) 84:830–7. doi: 10.4269/ajtmh.2011.10-0498
22. Yeka A, Gasasira A, Mpimbaza A, Achan J, Nankabirwa J, Nsobya S, et al. Malaria in Uganda: Challenges to Control on the Long Road to Elimination: I. Epidemiology and Current Control Efforts. Acta Trop (2012) 121:184–95. doi: 10.1016/j.actatropica.2011.03.004
23. Okello PE, Van Bortel W, Byaruhanga AM, Correwyn A, Roelants P, Talisuna A, et al. Variation in Malaria Transmission Intensity in Seven Sites Throughout Uganda. Am J Trop Med Hyg (2006) 75:219–25. doi: 10.4269/ajtmh.2006.75.219
24. Matsuoka K, Komori H, Nose M, Endo Y, Sawasaki T. Simple Screening Method for Autoantigen Proteins Using the N-Terminal Biotinylated Protein Library Produced by Wheat Cell-Free Synthesis. J Proteome Res (2010) 9:4264–73. doi: 10.1021/pr9010553
25. Longley RJ, White MT, Takashima E, Morita M, Kanoi BN, Li Wai Suen CSN, et al. Naturally Acquired Antibody Responses to More Than 300 Plasmodium Vivax Proteins in Three Geographic Regions. PloS Negl Trop Dis (2017) 11:e0005888. doi: 10.1371/journal.pntd.0005888
26. Osier FH, Fegan G, Polley SD, Murungi L, Verra F, Tetteh KK, et al. Breadth and Magnitude of Antibody Responses to Multiple Plasmodium falciparum Merozoite Antigens are Associated With Protection From Clinical Malaria. Infect Immun (2008) 76:2240–8. doi: 10.1128/IAI.01585-07
27. Rono J, Osier FH, Olsson D, Montgomery S, Mhoja L, Rooth I, et al. Breadth of Anti-Merozoite Antibody Responses is Associated With the Genetic Diversity of Asymptomatic Plasmodium falciparum Infections and Protection Against Clinical Malaria. Clin Infect Dis (2013) 57:1409–16. doi: 10.1093/cid/cit556
28. Jolliffe I. Principal Component Analysis. In: Encyclopedia of Statistics in Behavioral Science (New York, NY: Springer) (2005).
29. Fowkes FJ, Richards JS, Simpson JA, Beeson JG. The Relationship Between Anti-Merozoite Antibodies and Incidence of Plasmodium falciparum Malaria: A Systematic Review and Meta-Analysis. PLoS Med (2010) 7:e1000218. doi: 10.1371/journal.pmed.1000218
30. Lau CK, Turner L, Jespersen JS, Lowe ED, Petersen B, Wang CW, et al. Structural Conservation Despite Huge Sequence Diversity Allows EPCR Binding by the PfEMP1 Family Implicated in Severe Childhood Malaria. Cell Host Microbe (2015) 17:118–29. doi: 10.1016/j.chom.2014.11.007
31. Turner L, Lavstsen T, Berger SS, Wang CW, Petersen JE, Avril M, et al. Severe Malaria is Associated With Parasite Binding to Endothelial Protein C Receptor. Nature (2013) 498:502–5. doi: 10.1038/nature12216
32. Jensen AT, Magistrado P, Sharp S, Joergensen L, Lavstsen T, Chiucchiuini A, et al. Plasmodium falciparum Associated With Severe Childhood Malaria Preferentially Expresses PfEMP1 Encoded by Group A Var Genes. J Exp Med (2004) 199:1179–90. doi: 10.1084/jem.20040274
33. Tonkin-Hill GQ, Trianty L, Noviyanti R, Nguyen HHT, Sebayang BF, Lampah DA, et al. The Plasmodium falciparum Transcriptome in Severe Malaria Reveals Altered Expression of Genes Involved in Important Processes Including Surface Antigen-Encoding Var Genes. PLoS Biol (2018) 16:e2004328. doi: 10.1371/journal.pbio.2004328
34. Bengtsson A, Joergensen L, Rask TS, Olsen RW, Andersen MA, Turner L, et al. A Novel Domain Cassette Identifies Plasmodium falciparum PfEMP1 Proteins Binding ICAM-1 and Is a Target of Cross-Reactive, Adhesion-Inhibitory Antibodies. J Immunol (2013) 190:240–9. doi: 10.4049/jimmunol.1202578
35. Lennartz F, Bengtsson A, Olsen RW, Joergensen L, Brown A, Remy L, et al. Mapping the Binding Site of a Cross-Reactive Plasmodium falciparum PfEMP1 Monoclonal Antibody Inhibitory of ICAM-1 Binding. J Immunol (2015) 195:3273–83. doi: 10.4049/jimmunol.1501404
36. Tan J, Pieper K, Piccoli L, Abdi A, Perez MF, Geiger R, et al. A LAIR1 Insertion Generates Broadly Reactive Antibodies Against Malaria Variant Antigens. Nature (2016) 529:105–9. doi: 10.1038/nature16450
37. Abdel-Latif MS, Dietz K, Issifou S, Kremsner PG, Klinkert MQ. Antibodies to Plasmodium falciparum Rifin Proteins are Associated With Rapid Parasite Clearance and Asymptomatic Infections. Infect Immun (2003) 71:6229–33. doi: 10.1128/IAI.71.11.6229-6233.2003
38. Saito F, Hirayasu K, Satoh T, Wang CW, Lusingu J, Arimori T, et al. Immune Evasion of Plasmodium falciparum by RIFIN via Inhibitory Receptors. Nature (2017) 552:101–5. doi: 10.1038/nature24994
39. Fernandez V, Hommel M, Chen Q, Hagblom P, Wahlgren M. Small, Clonally Variant Antigens Expressed on the Surface of the Plasmodium falciparum-Infected Erythrocyte are Encoded by the Rif Gene Family and are the Target of Human Immune Responses. J Exp Med (1999) 190:1393–404. doi: 10.1084/jem.190.10.1393
40. Oeuvray C, Bouharoun-Tayoun H, Grass-Masse H, Lepers JP, Ralamboranto L, Tartar A, et al. A Novel Merozoite Surface Antigen of Plasmodium falciparum (MSP-3) Identified by Cellular-Antibody Cooperative Mechanism Antigenicity and Biological Activity of Antibodies. Mem Inst Oswaldo Cruz (1994) 89 Suppl 2:77–80. doi: 10.1590/S0074-02761994000600018
41. Singh S, Soe S, Mejia JP, Roussilhon C, Theisen M, Corradin G, et al. Identification of a Conserved Region of Plasmodium falciparum MSP3 Targeted by Biologically Active Antibodies to Improve Vaccine Design. J Infect Dis (2004) 190:1010–8. doi: 10.1086/423208
42. Sirima SB, Mordmuller B, Milligan P, Ngoa UA, Kironde F, Atuguba F, et al. A Phase 2b Randomized, Controlled Trial of the Efficacy of the GMZ2 Malaria Vaccine in African Children. Vaccine (2016) 34:4536–42. doi: 10.1016/j.vaccine.2016.07.041
43. Dassah S, Adu B, Sirima SB, Mordmuller B, Ngoa UA, Atuguba F, et al. Extended Follow-Up of Children in a Phase2b Trial of the GMZ2 Malaria Vaccine. Vaccine (2021) 39:4314–9. doi: 10.1016/j.vaccine.2021.06.024
44. Dejon-Agobe JC, Ateba-Ngoa U, Lalremruata A, Homoet A, Engelhorn J, Nouatin OP, et al. Controlled Human Malaria Infection of Healthy Adults With Lifelong Malaria Exposure to Assess Safety, Immunogenicity, and Efficacy of the Asexual Blood Stage Malaria Vaccine Candidate Gmz2. Clin Infect Dis (2019) 69:1377–84. doi: 10.1093/cid/ciy1087
45. Aniweh Y, Nyarko PB, Charles-Chess E, Ansah F, Osier FHA, Quansah E, et al. Plasmodium falciparum Merozoite Associated Armadillo Protein (PfMAAP) Is Apically Localized in Free Merozoites and Antibodies Are Associated With Reduced Risk of Malaria. Front Immunol (2020) 11:505. doi: 10.3389/fimmu.2020.00505
46. Nasir SMI, Amarasekara S, Wickremasinghe R, Fernando D, Udagama P. Prevention of Re-Establishment of Malaria: Historical Perspective and Future Prospects. Malaria J (2020) 19:452. doi: 10.1186/s12936-020-03527-8
47. Hay SI, Okiro EA, Gething PW, Patil AP, Tatem AJ, Guerra CA, et al. Estimating the Global Clinical Burden of Plasmodium falciparum Malaria in 2007. PLoS Med (2010) 7:e1000290. doi: 10.1371/journal.pmed.1000290
48. Nkumama IN, O'Meara WP, Osier FH. Changes in Malaria Epidemiology in Africa and New Challenges for Elimination. Trends Parasitol (2016) 33(2):128–40. doi: 10.1016/j.pt.2016.11.006
49. Koram KA, Molyneux ME. When Is “Malaria” Malaria? The Different Burdens of Malaria Infection, Malaria Disease, and Malaria-Like Illnesses. Am J Trop Med Hyg (2007) 77:1–5. doi: 10.4269/ajtmh.77.6.suppl.1
50. Thomson-Luque R, Votborg-Novel L, Ndovie W, Andrade CM, Niangaly M, Attipa C, et al. Plasmodium falciparum Transcription in Different Clinical Presentations of Malaria Associates With Circulation Time of Infected Erythrocytes. Nat Commun (2021) 12:4711. doi: 10.1038/s41467-021-25062-z
51. Finney OC, Danziger SA, Molina DM, Vignali M, Takagi A, Ji M, et al. Predicting Antidisease Immunity Using Proteome Arrays and Sera From Children Naturally Exposed to Malaria. Mol Cell Proteomics (2014) 13:2646–60. doi: 10.1074/mcp.M113.036632
52. Kamuyu G, Tuju J, Kimathi R, Mwai K, Mburu J, Kibinge N, et al. KILchip V1.0: A Novel Plasmodium falciparum Merozoite Protein Microarray to Facilitate Malaria Vaccine Candidate Prioritization. Front Immunol (2018) 9:2866. doi: 10.3389/fimmu.2018.02866
53. Romanov V, Davidoff SN, Miles AR, Grainger DW, Gale BK, Brooks BD. A Critical Comparison of Protein Microarray Fabrication Technologies. Analyst (2014) 139:1303–26. doi: 10.1039/C3AN01577G
54. Kanoi BN, Nagaoka H, Morita M, Tsuboi T, Takashima E. Leveraging the Wheat Germ Cell-Free Protein Synthesis System to Accelerate Malaria Vaccine Development. Parasitol Int (2021) 80:102224. doi: 10.1016/j.parint.2020.102224
55. Nagaoka H, Kanoi BN, Ntege EH, Aoki M, Fukushima A, Tsuboi T, et al. Antibodies Against a Short Region of PfRipr Inhibit Plasmodium falciparum Merozoite Invasion and PfRipr Interaction With Rh5 and SEMA7A. Sci Rep (2020) 10:6573. doi: 10.1038/s41598-020-63611-6
56. Nagaoka H, Sasaoka C, Yuguchi T, Kanoi BN, Ito D, Morita M, et al. PfMSA180 Is a Novel Plasmodium falciparum Vaccine Antigen That Interacts With Human Erythrocyte Integrin Associated Protein (CD47). Sci Rep (2019) 9:5923. doi: 10.1038/s41598-019-42366-9
57. Yuguchi T, Kanoi BN, Nagaoka H, Miura T, Ito D, Takeda H, et al. Plasmodium Yoelii Erythrocyte Binding Like Protein Interacts With Basigin, an Erythrocyte Surface Protein. Front Cell Infect Microbiol (2021) 11:656620. doi: 10.3389/fcimb.2021.656620
58. Arumugam TU, Ito D, Takashima E, Tachibana M, Ishino T, Torii M, et al. Application of Wheat Germ Cell-Free Protein Expression System for Novel Malaria Vaccine Candidate Discovery. Expert Rev Vaccines (2014) 13:75–85. doi: 10.1586/14760584.2014.861747
Keywords: Plasmodium falciparum, variable surface antigens, PfEMP1, RIFIN, malaria immunity, PCA
Citation: Takashima E, Kanoi BN, Nagaoka H, Morita M, Hassan I, Palacpac NMQ, Egwang TG, Horii T, Gitaka J and Tsuboi T (2022) Meta-Analysis of Human Antibodies Against Plasmodium falciparum Variable Surface and Merozoite Stage Antigens. Front. Immunol. 13:887219. doi: 10.3389/fimmu.2022.887219
Received: 01 March 2022; Accepted: 11 May 2022;
Published: 09 June 2022.
Edited by:
Denise L. Doolan, James Cook University, AustraliaReviewed by:
Virander Singh Chauhan, International Centre for Genetic Engineering and Biotechnology, IndiaCopyright © 2022 Takashima, Kanoi, Nagaoka, Morita, Hassan, Palacpac, Egwang, Horii, Gitaka and Tsuboi. This is an open-access article distributed under the terms of the Creative Commons Attribution License (CC BY). The use, distribution or reproduction in other forums is permitted, provided the original author(s) and the copyright owner(s) are credited and that the original publication in this journal is cited, in accordance with accepted academic practice. No use, distribution or reproduction is permitted which does not comply with these terms.
*Correspondence: Eizo Takashima, dGFrYXNoaW1hLmVpem8ubXpAZWhpbWUtdS5hYy5qcA==; Takafumi Tsuboi, dHN1Ym9pLnRha2FmdW1pLm1iQGVoaW1lLXUuYWMuanA=
†These authors have contributed equally to this work
Disclaimer: All claims expressed in this article are solely those of the authors and do not necessarily represent those of their affiliated organizations, or those of the publisher, the editors and the reviewers. Any product that may be evaluated in this article or claim that may be made by its manufacturer is not guaranteed or endorsed by the publisher.
Research integrity at Frontiers
Learn more about the work of our research integrity team to safeguard the quality of each article we publish.