- 1School of Public Health, Zhejiang Chinese Medical University, Hangzhou, China
- 2The Fourth College of Clinical Medicine, Zhejiang Chinese Medical University, Hangzhou, China
- 3School of Life Sciences, Westlake University, Hangzhou, China
Background: Inflammation plays a pivotal role in the pathogenesis of cancer. Though previous studies have reported a link between several inflammatory biomarkers and risk of certain types of cancer, there is a lack of systematic investigation. Therefore, we aimed to assess the role of circulating cytokines on the risk of cancer using a two-sample Mendelian randomization (MR) approach.
Method: We used genetic variants associated with circulating levels of cytokines from a meta-analysis of genome-wide association studies (GWASs) of 8,293 Finns as instrumental variables. Summary level data of 20 site-specific cancer were obtained from the UK BioBank including up to 456,348 participants of European ancestry. We performed two-sample MR analyses using inverse-variance weighted (IVW) method as the main method, followed by weighted-median and likelihood-based methods as sensitivity analysis. Pleiotropic and outlier variants were assessed by MR-Egger regression and MR Pleiotropy RESidual Sum and Outlier (MR-PRESSO) test.
Results: 224 genetic variants associated with 27 circulating cytokines achieving genome-wide significance (P<5×10-8) were used as IVs. After Bonferroni correction, genetically predicted high levels of interleukin-18 (IL-18) were associated with a decreased risk of acute myeloid leukemia (odds ratio (OR) per 1 standard deviation (SD) increase = 0.55, 95% confidence interval (CI):0.43-0.69, P=5.39×10-7), and circulating levels of IL-17 were associated with altered stomach cancer risk (OR per 1 SD increase = 0.15, 95% CI: 0.07-0.36, P=1.25×10-5) by IVW. Results were stable across sensitivity analyses, and MR-Egger regression did not suggest the presence of directional pleiotropy. Additionally, we found suggestive evidence for 48 cytokine-cancer associations including tumor necrosis factor related apoptosis-inducing ligand (TRAIL) and cutaneous T-cell attracting chemokine (CTACK) with the risk of several types of cancer (9.26×10-5≤P<0.05).
Conclusions: By using a genetic epidemiological approach, our study systematically evaluated the role of circulating cytokines on the risk of cancer, and provided clues for potential therapeutic targets. However, the exact underlying biological mechanism warrants further investigation.
Introduction
Cancer is a leading cause of death before the age of 70 years in 183 countries in 2019 (1). Globally, an estimated 19.3 million new cancer cases and approximately 10.0 million cancer deaths occurred in 2020 (2). Established risk factors for cancer include obesity, smoking, alcohol consumption, and infection (3–7). Inflammation has been demonstrated to play a pivotal role in carcinogenesis, since the reactive oxygen/nitrogen species from inflammation damage not only DNA but also other biomacromolecules, such as proteins and lipids, may result in their dysfunction, thus exerting cancer promoting effects (8). Previous studies have shown that NLRP3 inflammasome and cytosolic multi-protein complexes involved in innate immune response (9), can promote the development of several malignancies, including head and neck squamous cell carcinoma, fibrosarcoma, melanoma, stomach cancer and lung cancer (10–15). Observational data also indicated that chronic inflammation can increase the risk of certain types of cancer. For example, chronic prostatitis can increase the risk of prostate cancer by 2-3 times, and ulcerative colitis can increase the risk of colon cancer by 6-19 times (16). In addition, several inflammatory markers have been tested in relation to cancer incidence. For instance, a meta-analysis showed that higher concentrations of circulating C-reactive protein (CRP), a non-specific marker of systemic inflammation, were associated with a higher risk of breast cancer (hazard ratio (HR)=1.14, 95% confidence interval (CI) 1.01-1.28), lung cancer (HR=2.03, 95% CI 1.59-2.60), colorectal cancer (OR=1.34, 95% CI 1.11-1.60) and prostate cancer (HR=1.09, 95% CI 1.03-1.15) (17). These findings suggested that cytokines may play pivotal roles in carcinogenesis, and intervening on these inflammatory biomarkers in cancer may help prevent its incidence and aid in the development of novel therapeutic targets (17).
Mendelian Randomization (MR) utilizes genetic variants as instrumental variables (IVs) to estimate the potential causal relationship between the exposure (i.e., circulating cytokines) and the outcome (i.e., cancer risk) (18). Since genotypes are randomly distributed in the process of gamete formation, the causal inference from MR analyses are less susceptible to common confounding factors in conventional observational studies, such as postnatal environment, socio-economic status, and behavioral factors (19). Moreover, since genotypes preceded the onset of diseases, MR analyses are less prone to reverse causation in traditional observational studies. Therefore, it becomes widely used to assess the potential causal associations between exposures and diseases.
In the present study, by leveraging large-scale data of genome-wide association studies (GWAS) from the UK BioBank, we implemented a two-sample MR design to systematically investigate the role of circulating cytokines on the risk of different cancer types.
Method
Study Design and Data Source
The overall design of the present study is shown in Figure 1. The detailed information on the summary-level data of GWASs on cytokines and 20 site-specific cancer from the UK BioBank are summarized in Table S1. Briefly, all data in our study were based on participants of European ancestry. The GWAS meta-analysis of cytokines included 8,293 Finnish individuals from three independent population cohorts: the Cardiovascular Risk in Young Finns Study, FINRISK1997, and FINRISK2002 Study (20). The effect estimates of each genetic variants retrieved were calculated using a additive genetic model and adjusted for the first ten genetic principal components, age, sex, and body mass index.
Summary-level data for the association between genetic variants and 20 site-specific cancer were obtained from the UK BioBank (UKBB), which is a large-scale cohort study of around 500,000 adults aged 40 to 69 years across the UK from 2006 to 2010 (21). In the present analyses, we included genetic data of up to 456,348 participants from UKBB (Table S1).
Selection of Genetic Variants
Single nucleotide polymorphisms (SNPs) associated with circulating levels of cytokines were selected at the genome-wide significance level (P<5×10-8). We then pruned these SNPs in linkage disequilibrium (LD) using an r2 threshold < 0.1, and selected the SNPs with the lowest P-value. A total of 958 independent SNPs were selected as IVs. Among them, 129 SNPs were associated with more than one cytokine and were thus excluded. Among the remaining 829 SNPs, 605 were not available in the outcome datasets. Finally, 224 SNPs associated with circulating levels of 27 cytokines were included in the subsequent MR analyses. Detailed information of the 27 cytokines and the SNPs used as IVs are displayed in Table S2.
Statistical Analysis
First, we calculated F-statistics to quantify the strength of the IVs, with the equation of F=R2×(N−1−k)/(1−R2), in which R2 represents the variance explained by the IVs, N indicates the sample size, and k is the number of SNPs included in the instrument (22).
We then used inverse-variance-weighted (IVW) method as the main MR analysis to evaluate the potential causal effects of the 27 cytokines on the risk of 20 site-specific cancer (19, 23). Cocrane’s Q test was applied to assess the heterogeneity between the SNPs, and a fixed-effects model was used when there was no evidence of heterogeneity; otherwise, a random-effects model was used. In addition, to assess the robustness of our main findings, we used a series of sensitivity analyses, including the weighted-median and likelihood-based methods. Specifically, the weighted-median method combines the unweighted or weighted estimation with the median. As long as the weight of the causal effect calculated by the effective instrumental variable reaches 50%, a consistent estimation of the causal effect can be obtained (22). Meanwhile, the likelihood-based method evaluates the potential causal relationship under the assumption of a linear association between the risk factor and the outcome variables (24). Moreover, MR-Egger regression was performed to assess the potential directional pleiotropy. The slope of MR-Egger regression can suggest pleiotropy corrected causal estimates, and the value of the intercept can provide an estimate of the degree of pleiotropy (25). The Mendelian randomization pleiotropy residual sum and outlier (MR-PRESSO) test was also used to detect and correct for horizontal pleiotropic outliers. It conducts a global test of heterogeneity by regressing the SNP-outcome associations on the SNP-exposure associations and comparing the observed distance of each SNP from the regression with the distance expected under the null hypothesis of no pleiotropy (26).
Furthermore, to minimize the influence of pleiotropic instruments on MR estimates, we manually scanned the SNPs used as IVs in the GWAS Catalog, and excluded those associated with secondary phenotypes at genome-wide significance. We then reran the MR analyses using the updated IVs. Additionally, to test for the stability of our findings, we performed “leave-one-out” analyses, which excluded one single SNP at a time, and re-run the MR analysis using IVW method with the remaining IVs.
All analyses were performed using R (version 3.6.0) and related R packages (MendelianRandomization and MR-PRESSO). Associations with P values<9.26×10-5 (P = 0.05/27 cytokines/20 site-specific cancers) were considered statistically significant after Bonferroni correction for 27 cytokines and 20 site-specific cancer. A P-value < 0.05, but above the Bonferroni correction threshold, was considered suggestive evidence for a potential causal association.
Results
Table S3 presents the detailed information of the IVs used for circulating levels of the 27 cytokines and growth factors. The median of F-statistics ranged from 30 to 788.96, satisfying the threshold of >10, suggesting that the IVs used in our study were unlikely to suffer from weak instrument bias (27).
The MR association estimates of the 27 cytokines with the risk of 20-site specific cancer are shown in Figure 2. Specifically, genetically predicted higher levels of circulating IL-18 was associated with a decreased risk of acute myeloid leukemia (OR=0.55, 95% CI: 0.43-0.69, P=5.39×10-7, per 1 standard deviation (SD) increase). Detailed information of the IVs used for circulating IL-18 levels and their associations with the risk of acute myeloid leukemia (AML) are presented in Table 1. In sensitivity analysis using different MR methods, the association remained statistically significant and the effect estimates were similar (OR=0.44, 95% CI: 0.32-0.60, P=1.23×10-7 for the weighted-median method; OR=0.55, 95% CI: 0.44-0.70, P=1.06×10-6 for the maximum-likelihood method). Moreover, there was no evidence for the presence of directional pleiotropy (P for MR-Egger intercept =0.095; P for MR-PRESSO global test=0.381; and number of outlier SNPs=0), and the results from MR-PRESSO test was similar (OR=0.55, 95% CI: 0.43-0.69, P=1.55×10-4). None of the SNPs used as IVs had documented pleiotropy as we searched the GWAS Catalog (last accessed on January 31, 2022). In the leave-one-out sensitivity analysis, the association estimates of genetically predicted IL-18 levels with the risk of AML did not change substantially after excluding one single SNP at a time (Figure S1).
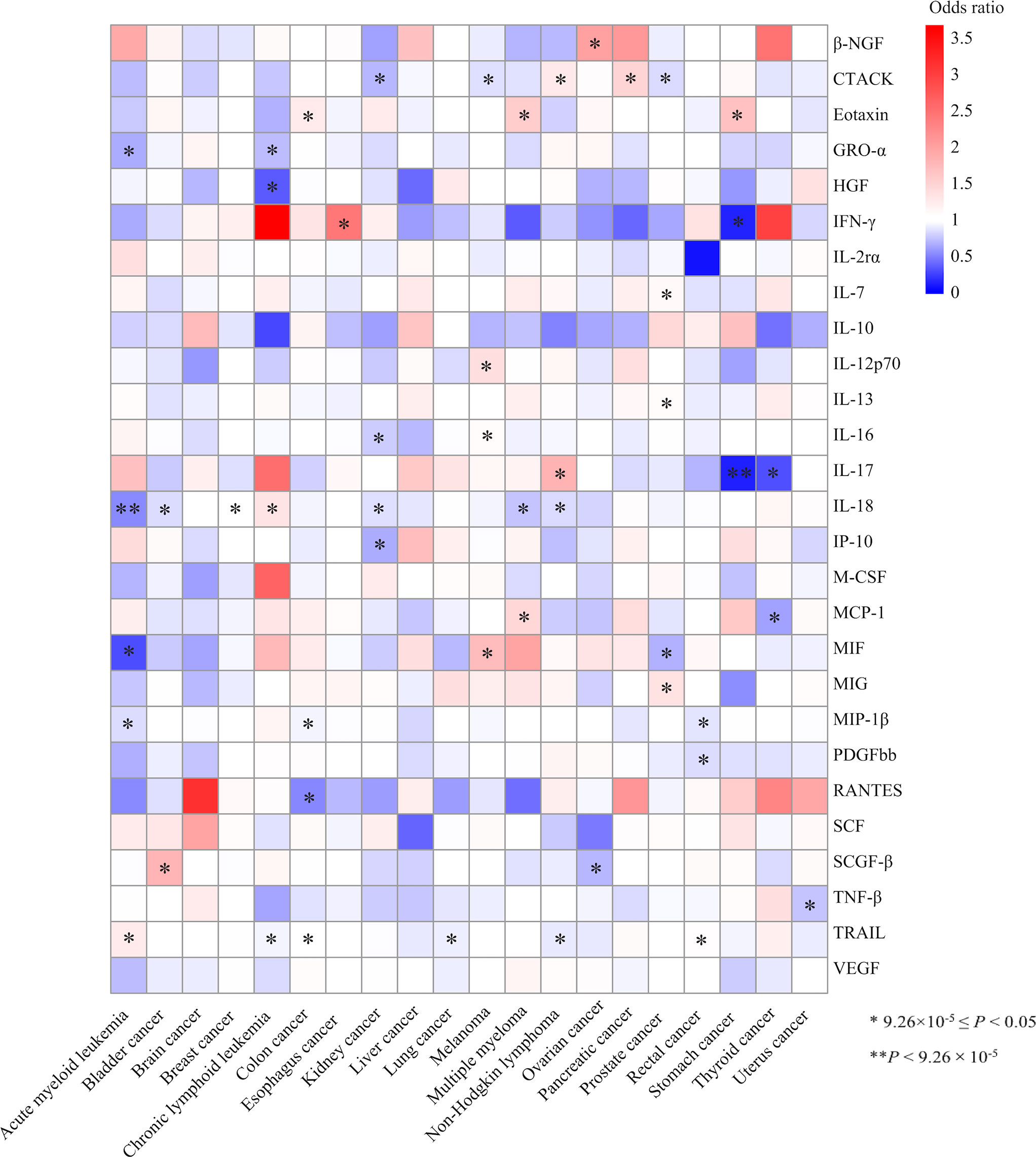
Figure 2 Heatmap of the associations of the 27 cytokines with the risk of 20 site-specific cancer from the inverse variance weighted (IVW) method. One asterisk indicates the suggestive evidence for a potential causal association (9.26×10-5≤ P <0.05), while two asterisks denote that the associations were statistically significant after multiple comparison correction (P<9.26×10-5). Colour is scaled based on the odd ratio (OR) of the MR association estimate.
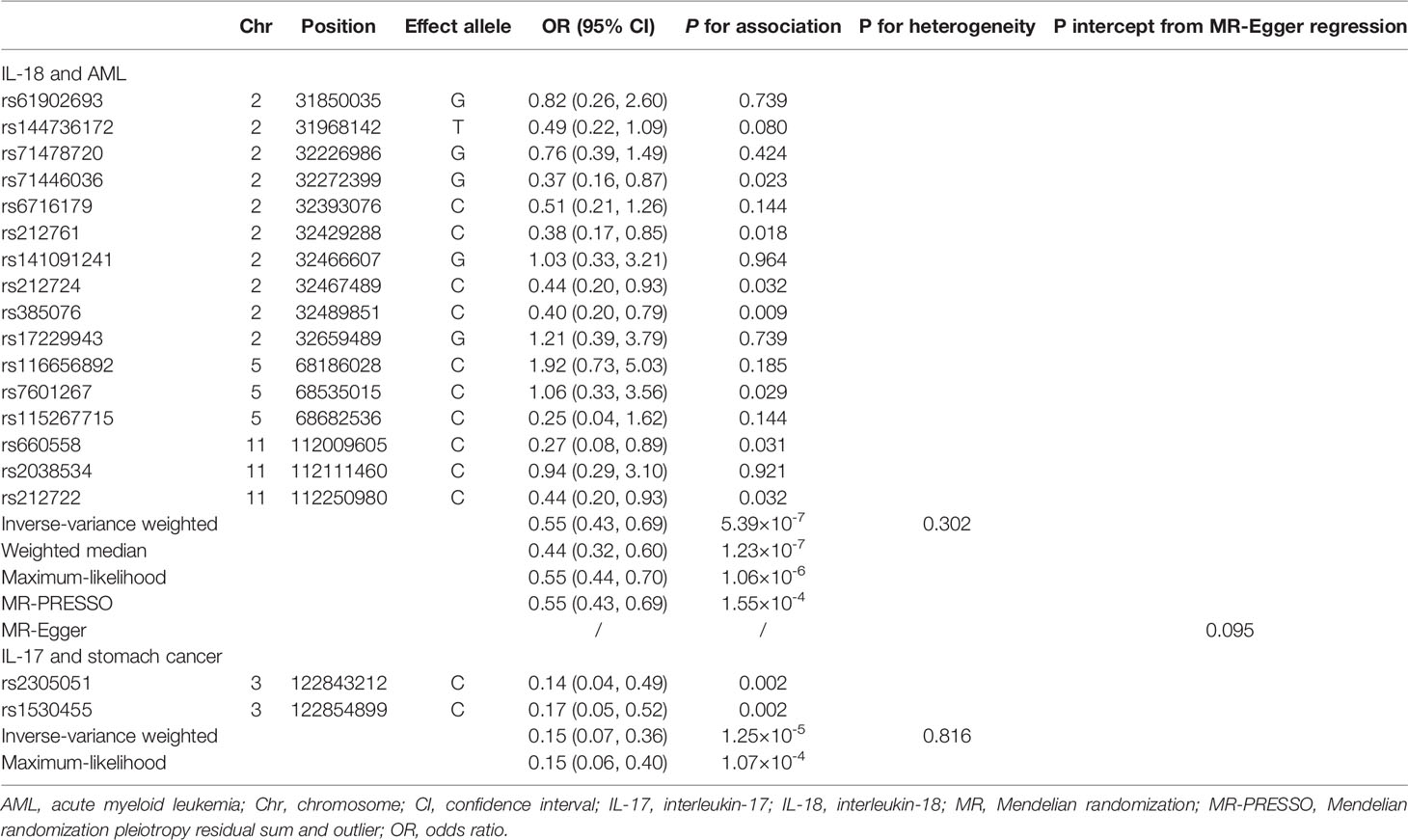
Table 1 MR effect estimates for associations of circulating IL-18 with the risk of AML and IL-17 with stomach cancer.
Meanwhile, we found an inverse association between circulating levels of IL-17 and the risk of stomach cancer using the IVW method (per 1 SD increase OR=0.15, 95% CI: 0.07-0.36, P=1.25×10-5, Table 1, Figure 2). One SNP, rs1530455, was found to be associated with other phenotypes at genome-wide significance level (Table S4). After excluding this SNP, the effect estimate did not change essentially (OR=0.14, 95% CI: 0.04-0.49, P=0.002).
In addition, we found 48 suggestive associations of a specific cytokine with the risk of site-specific cancer (9.26×10-5≤ P <0.05). The detailed results are shown in Figure 2 and Table S5. Among them, IL-18 was nominally associated with the risk of six types of cancer, including bladder cancer (OR=0.86, 95% CI: 0.79-0.93, P=3.30×10-4), breast cancer (OR=1.05, 95% CI: 1.01-1.09, P=0.027), chronic lymphoid leukemia (CLL) (OR=1.39, 95% CI: 1.29-1.71, P=0.002), kidney cancer (OR=0.87, 95% CI: 0.77-0.99, P=0.028), multiple myeloma (OR=0.76, 95% CI: 0.63-0.90, P=0.002), non-Hodgkin lymphoma (OR=0.84, 95% CI: 0.74-0.96, P=0.008). Similarly, genetically determined circulating levels of tumor necrosis factor related apoptosis-inducing ligand (TRAIL) were nominally associated with the risk AML (OR=1.27, 95% CI: 1.06-1.52, P=0.011), colon cancer (OR=1.07, 95% CI: 1.01-1.13, P=0.021), CLL (OR=1.27, 95% CI: 1.06-1.52, P=0.011), lung cancer (OR=0.93, 95% CI: 0.87-0.99, P=0.034), non-Hodgkin lymphoma (OR=0.91, 95% CI: 0.84-0.99, P=0.034), and rectal cancer (OR=1.08, 95% CI: 1.01-1.15, P=0.032). Moreover, circulating cutaneous T-cell attracting chemokine (CTACK) levels were nominally associated with the risk of kidney cancer (OR=0.72, 95% CI: 0.64-0.85, P=1.64×10-4), melanoma (OR=0.87, 95% CI: 0.79-0.95, P=0.002), non-Hodgkin lymphoma (OR=1.29, 95% CI: 1.11-1.49, P=6.90×10-4), pancreatic cancer (OR=1.50, 95% CI: 1.20-1.87, P=4.17×10-4) and prostate cancer (OR=0.85, 95% CI: 0.79-0.92, P=1.58×10-5).
We also identified nominal associations of genetically predicted circulating levels of macrophage inflammatory protein-1β (MIP1β) with the risk of AML (OR=0.86, 95% CI: 0.75-0.99, P=0.048), colon cancer (OR=0.95, 95% CI: 0.90-0.99, P=0.015) and rectal cancer (OR=0.89, 95% CI: 0.84-0.94, P=2.75×10-5), as well as eotaxin with the risk of colon cancer (OR=1.28, 95% CI: 1.09-1.49, P=0.003), multiple myeloma (OR=1.58, 95% CI: 1.08-2.29, P=0.017), and stomach cancer (OR=1.68, 95% CI: 1.16-2.44, P=0.006).
Discussion
In this study, we adopted a two-sample MR approach and systematically evaluated the potential causal effect of 27 circulating cytokines and growth factors on the risk of 20 site-specific cancer. We found that genetically predicted higher levels of IL-18 were associated with an decreased risk of AML, and IL-17 was associated with the risk of stomach cancer. Results were stable in sensitivity analyses using different MR methods and different IV sets. Additionally, we found nominal associations of some cytokine-cancer pairs, suggesting the potential role of these inflammatory biomarkers in the development of certain types of cancer.
IL-18 is a proinflammatory cytokine of the IL-1 family, which can stimulate interferon gamma production and regulate both T helper (Th) 1 and Th2 responses, thus having multiple biological functions (28). IL-18 can promote the proliferation of activated T cells, activation of natural killer cells and cytokine production (29). Protective effects of IL-18 in cancer have been reported in different murine models. For instance, mice receiving IL-18 before or after challenge with CL8-1, both regimens significantly suppressed tumor growth and reduced the number of mice with growth of tumor from 60% (3/5) to 20% (1/5) (30). Hitzler et al. (31) found that IL-18 counteracts IL-1-driven inflammation and limits Helicobacter pathogenic effect. However, observational studies on the association between circulating IL-18 levels and AML are limited to date. A case-control study involving 70 patients and 50 controls reported that there were no differences in the expression level of IL-18 in patients with AML compared to healthy controls (P=0.100) (32), while another study of 47 patients with AML found that IL-18 was associated with unfavorable prognostic factors of AML (33). The potential mechanism may be that IL-18 plays an important role in anti-tumor immunity through enhancing interferon-γ production and Fas ligand dependent cytotoxicity of immune cells, and the dose of IL-18 was correlated with the level of serum IFN-γ. Additionally, IL-18 can induce tumor Hapten by activating natural killer (NK) cells, and NK-mediated cytotoxicity of tumor cells, which exert an important role in immune response (30, 34).
As for IL-17, previous observational studies have reported a positive association of circulating IL-17 levels with the risk of stomach cancer. For example, a case-control study including 76 patients and 30 healthy age- and sex-matched controls reported that the median serum levels of IL-17 in patients with stomach cancer were higher than those of controls (9.04 vs. 8.07 pg/ml, P=0.010), but serum IL-17 levels were not associated with tumor stage (small (1-2 stage) vs. large (3-4 stage): 9.20 vs. 8.27 pg/ml, P=0.410) (35). Another case-control study involving 50 patients and 50 controls reported similar results (36). However, Carneiro et al. reported that IL-17 levels in stomach patients were lower than that in the control group (median: 404.2 vs. 573.9 pg/ml, P<0.004) (37). Our MR analysis found an inverse association of circulating IL-17 levels and the risk of stomach cancer. The conflicting results from observational studies may be related to the study design, study population, the stage and type of stomach cancer investigated. For example, a case-control study found that patients with early gastric carcinoma had higher levels of IL-17, whereas individuals with advanced gastric carcinoma had mean IL-17 concentrations comparable to those observed in healthy individuals (38). Another case-only study including 70 patients reported that the expression of IL-17 was lower in patients with diffuse type of stomach cancer compared with those with intestinal type (P=0.001) (39). Moreover, Chen et al. found that intratumoral IL-17 expression was an independent factor affecting the five-year overall survival probability in patients with gastric adenocarcinoma (HR: 0.52; 95% CI: 0.33-0.82; P=0.005) (40). The potential biological mechanism of IL-17 mediated tumor immunity may be that IL-17 can absorb Th1-related chemokines, such as CXC19 and CXC110, which can promote the migration of effector T cells to tumor sites and increase the number of CD8+ T cells (41).
In addition, we noted nominal cytokine-cancer associations including CTACK and MIP-1β with the risk of several types of cancer. MIP-1β is a member of the chemokine family, and it is related to leukocyte traffic, angiogenesis and metastasis of various types of cancer (42, 43). Observational studies have suggested that the serum concentration of MIP-1β was lower in patients with colorectal cancer as compared to controls (44, 45), which was consistent with our findings. MIP-1β can attract several types of immune cells, including macrophages and T cells. Thus, lower levels of MIP-1β in patients with colon and rectal cancer may be associated with decreased CD 68+ tumor-associated macrophage in the invasive margin (44, 46). Similarly, CTACK is a member of the CC chemokine family, which is crucial for the functioning of immune system cells (47). Our study also provided suggestive evidence for potential causal associations of genetically determined circulating levels of CTACK with the risk of prostate, kidney and pancreatic cancer, as well as the risk of melanoma and non-Hodgkin lymphoma. However, observational evidence on CTACK and these types of cancer was limited to date. There was a case-report showing that the pancreatic islets in patients with pancreatic cancer tested positive for CTACK expression (48).
Our study had several limitations. First, our MR estimates may be biased by potential pleiotropy. Therefore, we used different MR approaches and alternative IV sets to assess the influence of pleiotropic SNPs on our MR results. For example, for the association between CTACK and pancreatic cancer, though the MR-Egger regression suggested evidence of pleiotropy (P for MR-Egger intercept=0.048), none of the SNPs used as IVs for CTACK have documented associations with other secondary traits, suggesting our results may be biased by unrecognized pleiotropic IVs. Additionally, the GWAS summary statistics used in the present study were all from participants of European ancestry, which may limit the inference of findings to other populations with different ethnicities. Finally, the statistical power may be inadequate in the analyses of certain types of cancer with a limited number of cases, and therefore we may have overlooked potential weak associations.
In conclusion, our findings provide evidence to support potential causal associations of IL-18 with AML and IL-17 with stomach cancer. Further studies are warranted to elucidate underlying biological mechanism and to explore the potential therapeutic targets.
Data Availability Statement
The original contributions presented in the study are included in the article/Supplementary Material. Further inquiries can be directed to the corresponding authors.
Author Contributions
JS and AL performed the literature review, conducted data analysis, interpreted findings, and drafted the manuscript. LL and BL carried out data analysis and interpreted findings. YQ and DY conducted data analysis, interpreted findings and revised the manuscript. JS, AL and BL took responsibility for the statistical reports, tables and figures of the data analysis. YM and XS directed analytic strategy, supervised the study from conception to completion and revised drafts of the manuscript. All authors read and approved the final version of the manuscript.
Funding
This work was jointly supported by grants from National Natural Science Foundation of China (82103936, 82174208, and 81973663) and Natural Science Foundation of Zhejiang Province (LQ20H260008 and LQ21H260001). The funder had no role in the study design, data analysis, interpretation of data, or preparation of the manuscript.
Conflict of Interest
The authors declare that the research was conducted in the absence of any commercial or financial relationships that could be construed as a potential conflict of interest.
Publisher’s Note
All claims expressed in this article are solely those of the authors and do not necessarily represent those of their affiliated organizations, or those of the publisher, the editors and the reviewers. Any product that may be evaluated in this article, or claim that may be made by its manufacturer, is not guaranteed or endorsed by the publisher.
Acknowledgments
The author sincerely thanks the researchers and participants of the original GWAS for their collection and management of large-scale data resources, as well as those who actively participated in this study.
Supplementary Material
The Supplementary Material for this article can be found online at: https://www.frontiersin.org/articles/10.3389/fimmu.2022.886144/full#supplementary-material
Glossary
References
1. (WHO). WHO. Global Health Estimates 2020: Deaths by Cause, Age, Sex, by Country and by Region, 2000-2019. Global Cancer Observatory: WHO (2020).
2. Sung H, Ferlay J, Siegel RL, Laversanne M, Soerjomataram I, Jemal A, et al. Global Cancer Statistics 2020: GLOBOCAN Estimates of Incidence and Mortality Worldwide for 36 Cancers in 185 Countries. CA Cancer J Clin (2021) 71(3):209–49. doi: 10.3322/caac.21660
3. Salaun H, Thariat J, Vignot M, Merrouche Y, Vignot S. [Obesity and Cancer]. Bull Canc (2017) 104(1):30–41. doi: 10.1016/j.bulcan.2016.11.012
4. Parkin DM. 1. The Fraction of Cancer Attributable to Lifestyle and Environmental Factors in the UK in 2010. Br J Canc (2011) 105 Suppl 2:S2–5. doi: 10.1038/bjc.2011.474
5. Bagnardi V, Rota M, Botteri E, Tramacere I, Islami F, Fedirko V, et al. Alcohol Consumption and Site-Specific Cancer Risk: A Comprehensive Dose-Response Meta-Analysis. Br J Canc (2015) 112(3):580–93. doi: 10.1038/bjc.2014.579
6. Humans IWGotEoCRt. Personal Habits and Indoor Combustions. Volume 100 E. A Review of Human Carcinogens. IARC Monogr Eval Carcinog Risks Hum (2012) 100(Pt E):1–538.
7. Mantovani A. Cancer: Inflammation by Remote Control. Nature (2005) 435(7043):752–3. doi: 10.1038/435752a
8. Murata M. Inflammation and Cancer. Environ Health Prev Med (2018) 23(1):50. doi: 10.1186/s12199-018-0740-1
9. Place DE, Kanneganti TD. Recent Advances in Inflammasome Biology. Curr Opin Immunol (2018) 50:32–8. doi: 10.1016/j.coi.2017.10.011
10. Dunn JH, Ellis LZ, Fujita M. Inflammasomes as Molecular Mediators of Inflammation and Cancer: Potential Role in Melanoma. Cancer Lett (2012) 314(1):24–33. doi: 10.1016/j.canlet.2011.10.001
11. Zitvogel L, Kepp O, Galluzzi L, Kroemer G. Inflammasomes in Carcinogenesis and Anticancer Immune Responses. Nat Immunol (2012) 13(4):343–51. doi: 10.1038/ni.2224
12. Terlizzi M, Casolaro V, Pinto A, Sorrentino R. Inflammasome: Cancer's Friend or Foe? Pharmacol Ther (2014) 143(1):24–33. doi: 10.1016/j.pharmthera.2014.02.002
13. Karki R, Man SM, Kanneganti TD. Inflammasomes and Cancer. Cancer Immunol Res (2017) 5(2):94–9. doi: 10.1158/2326-6066.CIR-16-0269
14. Grivennikov SI, Greten FR, Karin M. Immunity, Inflammation, and Cancer. Cell (2010) 140(6):883–99. doi: 10.1016/j.cell.2010.01.025
15. Bae JY, Lee SW, Shin YH, Lee JH, Jahng JW, Park K. P2X7 Receptor and NLRP3 Inflammasome Activation in Head and Neck Cancer. Oncotarget (2017) 8(30):48972–82. doi: 10.18632/oncotarget.16903
16. Schetter AJ, Heegaard NH, Harris CC. Inflammation and Cancer: Interweaving microRNA, Free Radical, Cytokine and P53 Pathways. Carcinogenesis (2010) 31(1):37–49. doi: 10.1093/carcin/bgp272
17. Michels N, van Aart C, Morisse J, Mullee A, Huybrechts I. Chronic Inflammation Towards Cancer Incidence: A Systematic Review and Meta-Analysis of Epidemiological Studies. Crit Rev Oncol Hematol (2021) 157:103177. doi: 10.1016/j.critrevonc.2020.103177
18. Katan MB. Commentary: Mendelian Randomization, 18 Years on. Int J Epidemiol (2004) 33(1):10–1. doi: 10.1093/ije/dyh023
19. Lawlor DA, Harbord RM, Sterne JA, Timpson N, Davey Smith G. Mendelian Randomization: Using Genes as Instruments for Making Causal Inferences in Epidemiology. Stat Med (2008) 27(8):1133–63. doi: 10.1002/sim.3034
20. Ahola-Olli AV, Wurtz P, Havulinna AS, Aalto K, Pitkanen N, Lehtimaki T, et al. Genome-Wide Association Study Identifies 27 Loci Influencing Concentrations of Circulating Cytokines and Growth Factors. Am J Hum Genet (2017) 100(1):40–50. doi: 10.1016/j.ajhg.2016.11.007
21. Sudlow C, Gallacher J, Allen N, Beral V, Burton P, Danesh J, et al. UK Biobank: An Open Access Resource for Identifying the Causes of a Wide Range of Complex Diseases of Middle and Old Age. PloS Med (2015) 12(3):e1001779. doi: 10.1371/journal.pmed.1001779
22. Bowden J, Davey Smith G, Haycock PC, Burgess S. Consistent Estimation in Mendelian Randomization With Some Invalid Instruments Using a Weighted Median Estimator. Genet Epidemiol (2016) 40(4):304–14. doi: 10.1002/gepi.21965
23. Burgess S, Butterworth A, Thompson SG. Mendelian Randomization Analysis With Multiple Genetic Variants Using Summarized Data. Genet Epidemiol (2013) 37(7):658–65. doi: 10.1002/gepi.21758
24. Burgess S, Scott RA, Timpson NJ, Davey Smith G, Thompson SG, Consortium E-I. Using Published Data in Mendelian Randomization: A Blueprint for Efficient Identification of Causal Risk Factors. Eur J Epidemiol (2015) 30(7):543–52. doi: 10.1007/s10654-015-0011-z
25. Bowden J, Davey Smith G, Burgess S. Mendelian Randomization With Invalid Instruments: Effect Estimation and Bias Detection Through Egger Regression. Int J Epidemiol (2015) 44(2):512–25. doi: 10.1093/ije/dyv080
26. Verbanck M, Chen CY, Neale B, Do R. Detection of Widespread Horizontal Pleiotropy in Causal Relationships Inferred From Mendelian Randomization Between Complex Traits and Diseases. Nat Genet (2018) 50(5):693–8. doi: 10.1038/s41588-018-0099-7
27. Palmer TM, Lawlor DA, Harbord RM, Sheehan NA, Tobias JH, Timpson NJ, et al. Using Multiple Genetic Variants as Instrumental Variables for Modifiable Risk Factors. Stat Methods Med Res (2012) 21(3):223–42. doi: 10.1177/0962280210394459
28. Yasuda K, Nakanishi K, Tsutsui H. Interleukin-18 in Health and Disease. Int J Mol Sci (2019) 20(3):E649. doi: 10.3390/ijms20030649
29. Srivastava S, Pelloso D, Feng H, Voiles L, Lewis D, Haskova Z, et al. Effects of Interleukin-18 on Natural Killer Cells: Costimulation of Activation Through Fc Receptors for Immunoglobulin. Cancer Immunol Immunother (2013) 62(6):1073–82. doi: 10.1007/s00262-013-1403-0
30. Osaki T, Peron JM, Cai Q, Okamura H, Robbins PD, Kurimoto M, et al. IFN-Gamma-Inducing Factor/IL-18 Administration Mediates IFN-Gamma- and IL-12-Independent Antitumor Effects. J Immunol (1998) 160(4):1742–9.
31. Hitzler I, Sayi A, Kohler E, Engler DB, Koch KN, Hardt WD, et al. Caspase-1 has Both Proinflammatory and Regulatory Properties in Helicobacter Infections, Which Are Differentially Mediated by its Substrates IL-1beta and IL-18. J Immunol (2012) 188(8):3594–602. doi: 10.4049/jimmunol.1103212
32. Saadi MI, Ramzi M, Hosseinzadeh M, Ebrahimi N, Owjfard M, Abdolyousefi EN, et al. Expression Levels of Il-6 and Il-18 in Acute Myeloid Leukemia and Its Relation With Response to Therapy and Acute GvHD After Bone Marrow Transplantation. Indian J Surg Oncol (2021) 12(3):465–71. doi: 10.1007/s13193-021-01358-w
33. Zhang B, Wang Y, Zheng GG, Ma XT, Li G, Zhang FK, et al. Clinical Significance of IL-18 Gene Over-Expression in AML. Leuk Res (2002) 26(10):887–92. doi: 10.1016/S0145-2126(02)00025-5
34. Micallef MJ, Tanimoto T, Kohno K, Ikeda M, Kurimoto M. Interleukin 18 Induces the Sequential Activation of Natural Killer Cells and Cytotoxic T Lymphocytes to Protect Syngeneic Mice From Transplantation With Meth A Sarcoma. Cancer Res (1997) 57(20):4557–63.
35. Karabulut M, Usul Afsar C, Serimez M, Karabulut S. Serum IL-17 Levels Can Be Diagnostic for Gastric Cancer. J BUON (2019) 24(4):1601–9.
36. Meng XY, Zhou CH, Ma J, Jiang C, Ji P. Expression of Interleukin-17 and Its Clinical Significance in Gastric Cancer Patients. Med Oncol (2012) 29(5):3024–8. doi: 10.1007/s12032-012-0273-1
37. Carneiro B, Petroianu A, Machado JAN, Dos Anjos PMF, da Silva FR, Alberti LR, et al. Clinical and Immunological Allergy Assessment in Cancer Patients. Sci Rep (2021) 11(1):18110. doi: 10.1038/s41598-021-97200-y
38. Blogowski W, Madej-Michniewicz A, Marczuk N, Dolegowska B, Starzynska T. Interleukins 17 and 23 in Patients With Gastric Neoplasms. Sci Rep (2016) 6:37451. doi: 10.1038/srep37451
39. Pavlovic M, Gajovic N, Jurisevic M, Mitrovic S, Radosavljevic G, Pantic J, et al. Diverse Expression of IL-32 in Diffuse and Intestinal Types of Gastric Cancer. Gastroenterol Res Pract (2018) 2018:6578273. doi: 10.1155/2018/6578273
40. Chen JG, Xia JC, Liang XT, Pan K, Wang W, Lv L, et al. Intratumoral Expression of IL-17 and Its Prognostic Role in Gastric Adenocarcinoma Patients. Int J Biol Sci (2011) 7(1):53–60. doi: 10.7150/ijbs.7.53
41. Kryczek I, Banerjee M, Cheng P, Vatan L, Szeliga W, Wei S, et al. Phenotype, Distribution, Generation, and Functional and Clinical Relevance of Th17 Cells in the Human Tumor Environments. Blood (2009) 114(6):1141–9. doi: 10.1182/blood-2009-03-208249
42. Hua F, Tian Y. CCL4 Promotes the Cell Proliferation, Invasion and Migration of Endometrial Carcinoma by Targeting the VEGF-A Signal Pathway. Int J Clin Exp Pathol (2017) 10(11):11288–99.
43. Blum DL, Koyama T, M'Koma AE, Iturregui JM, Martinez-Ferrer M, Uwamariya C, et al. Chemokine Markers Predict Biochemical Recurrence of Prostate Cancer Following Prostatectomy. Clin Cancer Res (2008) 14(23):7790–7. doi: 10.1158/1078-0432.CCR-08-1716
44. Vayrynen JP, Kantola T, Vayrynen SA, Klintrup K, Bloigu R, Karhu T, et al. The Relationships Between Serum Cytokine Levels and Tumor Infiltrating Immune Cells and Their Clinical Significance in Colorectal Cancer. Int J Canc (2016) 139(1):112–21. doi: 10.1002/ijc.30040
45. Shirasuna K, Ito M, Matsuda T, Enomoto T, Ohara Y, Yamamoto M, et al. Correlation Analysis of the Proportion of Monocytic Myeloid-Derived Suppressor Cells in Colorectal Cancer Patients. PloS One (2020) 15(12):e0243643. doi: 10.1371/journal.pone.0243643
46. Maurer M, von Stebut E. Macrophage Inflammatory Protein-1. Int J Biochem Cell Biol (2004) 36(10):1882–6. doi: 10.1016/j.biocel.2003.10.019
47. Cogliano VJ, Baan R, Straif K, Grosse Y, Lauby-Secretan B, El Ghissassi F, et al. Preventable Exposures Associated With Human Cancers. J Natl Cancer Inst (2011) 103(24):1827–39. doi: 10.1093/jnci/djr483
Keywords: mendelian randomization, inflammation, cytokines, cancer, single nucleotide polymorphism
Citation: Song J, Li A, Qian Y, Liu B, Lv L, Ye D, Sun X and Mao Y (2022) Genetically Predicted Circulating Levels of Cytokines and the Risk of Cancer. Front. Immunol. 13:886144. doi: 10.3389/fimmu.2022.886144
Received: 14 March 2022; Accepted: 08 June 2022;
Published: 05 July 2022.
Edited by:
Fabiola Castro, University of São Paulo, BrazilReviewed by:
Armando Rojas, Catholic University of the Maule, ChileTathiane Malta, University of São Paulo, Brazil
Copyright © 2022 Song, Li, Qian, Liu, Lv, Ye, Sun and Mao. This is an open-access article distributed under the terms of the Creative Commons Attribution License (CC BY). The use, distribution or reproduction in other forums is permitted, provided the original author(s) and the copyright owner(s) are credited and that the original publication in this journal is cited, in accordance with accepted academic practice. No use, distribution or reproduction is permitted which does not comply with these terms.
*Correspondence: Xiaohui Sun, 20191030@zcmu.edu.cn; Yingying Mao, myy@zcmu.edu.cn
†These authors have contributed equally to this work