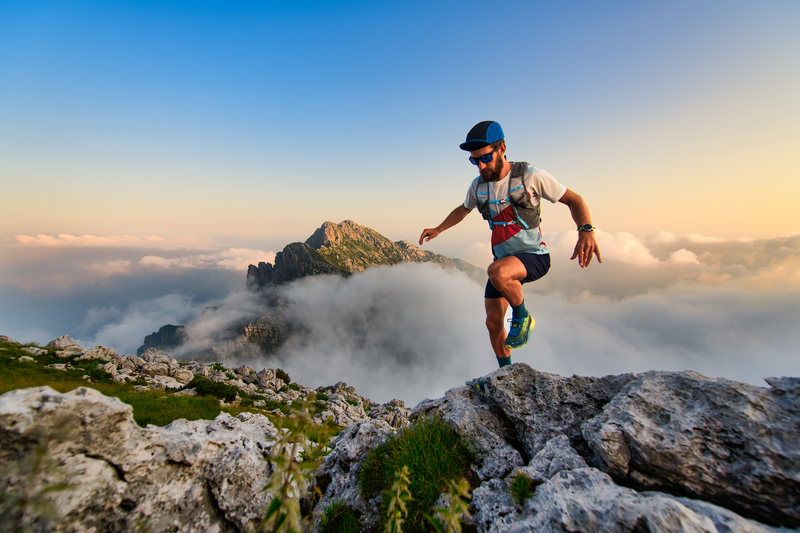
95% of researchers rate our articles as excellent or good
Learn more about the work of our research integrity team to safeguard the quality of each article we publish.
Find out more
ORIGINAL RESEARCH article
Front. Immunol. , 19 May 2022
Sec. Cancer Immunity and Immunotherapy
Volume 13 - 2022 | https://doi.org/10.3389/fimmu.2022.877689
This article is part of the Research Topic Tumor Microenvironment Immunophenotypes and Disease Progression View all 12 articles
Tertiary lymphoid structure (TLS) and tumor-resident memory T cells (TRM) play crucial roles in the anti-tumor immune response, facilitating a good prognosis in patients with cancer. However, there have been no reports on the relationship between TRM and TLS maturity. In this study, we detected TRM and the maturity of TLS by immunofluorescence staining and analyzed the relationship between their distribution and proportion in patients with lung adenocarcinoma (LUAD). The proportion of TRM within TLSs was significantly higher than that outside and was positively correlated with the survival of patients. In addition, the proportions of CD4+CD103+ TRM and CD8+CD103+ TRM were significantly increased with the gradually maturation of TLSs. We divided the patients into three levels (grade 1, grade 2, and grade 3) according to the presence of increasing maturation of TLSs. The proportion of CD103+ TRM in grade 3 patients was significantly higher than that in grade 1 and grade 2 patients, suggesting a close relationship between CD103+ TRM and TLS maturity. Furthermore, positive prognosis was associated with grade 3 patients that exhibited CD103+ phenotype.
Immunotherapy, e.g., treatment by immune checkpoint inhibitors (ICIs), has revolutionized therapeutic strategies for treating cancer, including non-small cell lung cancer (NSCLC) (1, 2). Previous studies on the mechanisms of ICIs have largely focused on tumor-infiltrating T cells (3). However, recently the findings of three independent studies have indicated that tertiary lymphoid structures (TLSs) and B cell signatures in the tumor site are key determinants of ICI therapeutic efficacy (4–6).
TLSs are ectopic immune cell aggregates that develop in peripheral tissues in response to a wide range of chronic inflammatory conditions, including tumors (7). The structure of TLSs includes B-cell- and T-cell-enriched areas; they have been reported to be the local site of initiation and maintenance of humoral and cellular immune responses for anti-tumor immunity (8). The activity and function of TLSs differ according to their cellular composition and maturation status. Well-developed TLSs composed of mature dendritic cell (DC)/T cell clusters and CD20+ B cell follicles are characterized by the presence of both a CD21+ follicular-DC (FDC) network and Ki67+ proliferating germinal center (GC)-B cells (9, 10). The density of mature TLS is associated with improved prognosis and is an effective predictive biomarker in cancer patients (11, 12). Researchers can synthesize tumor-specific antibodies, which are considered specific markers for prognosis (6). Moreover, B cells in TLSs can function as antigen-presenting cells and are associated with the induction of cytotoxic T cells (13). Therefore, these structures are major sources of tumor-infiltrating lymphocytes (TILs) and regulate the anti-tumor response (14).
Tissue-resident memory T (TRM) cells are tumor antigen-reactive TILs that produce a magnitude of cytotoxic mediators, such as granzyme B and perforin, as well as cytokines, such as interferon-gamma (IFN-γ) and tumor necrosis factor (TNF), in the tumor microenvironment (TME) (15, 16). TRM is a newly discovered subset of long-lived memory T cells that reside permanently in peripheral tissues without recirculation (17). In the tumor tissue, they mediate regional tumor surveillance and exhibit a protected anti-tumor function (15, 18). The permanence of TRM in NSCLC is mainly mediated by the expression of integrin αE (CD103) β7, which binds to E-cadherin in epithelial cells (19, 20). TRM is positively correlated with the survival of patients with cancer, including lung cancer (21, 22). The presence of intra-tumor CD8+CD103+TRM cells could predict a good clinical response in PD-1/PD-L1 blockade immunotherapy (23). CD8+ TRM cells have been mainly located around TLSs—both are associated with a better prognosis in patients with gastric cancer (24). These results indicate that tumor-resident T cells may have a close relationship with TLSs. However, there have been no reports on the association of TRM subset distribution with TLS maturation and their relationship with the prognosis of patients.
Because patients with stage III NSCLC usually have quite heterogeneous prognoses, we selected patients with stage III lung adenocarcinoma (LUAD) for the current study. The aim of this study was to investigate the clinical significance of TLS maturation in patients with LUAD, its association with the spatial distribution of distinct TRM subsets in LUAD.
Forty-nine patients with stage III primary LUAD who underwent surgical resection at Tianjin Medical University Cancer Institute and Hospital between January 2015 and May 2016 were enrolled in this retrospective study. Pathological TNM staging was histologically diagnosed based on the 7th edition of the Union for International Cancer Control TNM classification. The inclusion criterion was complete clinical data, standardized postoperative treatment and accurate pathological diagnosis. All patients underwent surgical resection of R0, and adjuvant therapy was mainly platinum-based chemotherapy, supplemented by radiotherapy or targeted therapy, when necessary. The exclusion criteria were those who had received anti-cancer treatment before surgery, had a second primary tumor, or were lacking follow-up. In this study, TLS positive tissues were selected for subsequent experiments which confirmed through hematoxylin and eosin (HE) staining slices. Formalin-fixed paraffin-embedded tumor tissues were collected from 49 patients for subsequent immunohistochemical staining and multiple immunofluorescence staining (Table 1). The study was approved by the Ethics Committee of the Tianjin Medical University Cancer Institute and Hospital. All patients signed relevant informed consent forms.
Multiple immunofluorescence staining was performed using a PerkinElmer Opal 7-color Technology Kit (NEL81001KT). The tumor specimens in paraffin-embedded blocks were cut into 4-μm-thick sections. The sections were deparaffinized in xylene and rehydrated in ethanol. Microwave repair was performed using EDTA buffer (PH=9.0) for 20 min. After cooling, the tissue was sealed with an antibody blocker at room temperature. The sections were then incubated overnight with primary antibody in a refrigerator at 4°C, and on the second day, the sections were co-incubated with poly-HRP-MS/Rb for 10 min at room temperature. Visualization was performed using Opal TSA (1:100). EDTA buffer was then heated by MWT to remove the AB-TSA complex. These steps were repeated for each round of the multiple staining. TSA-stained sections were washed with MWT and counterstained with DAPI (1:100) for 10 min. Using this staining method, all samples were stained with the primary antibody for CD20 (1:600 dilution, clone L26, Abcam) visualized with Opal520 TSA, CD3 (1:400 dilution, clone SP162, Abcam) visualized with Opal540 TSA, CD103 (1:500 dilution, clone EPR4166(2), Abcam) visualized with Opal570 TSA, Bcl6 (1:200 dilution, clone LN22, Novus) visualized with Opal620 TSA, CD4 (1:1000 dilution, clone EPR6855, Abcam) visualized with Opal650 TSA, CD21(1:800 dilution, clone EP3093, Abcam) visualized with Opal690 TSA. Finally, the sections were covered with an anti-fluorescence attenuating tablet and cover glass.
Tumor sections were scanned using a PerkinElmer Mantra Quantitative Pathology Imaging System at 200× magnification. Multispectral images were obtained using PerkinElmer inform Image Analysis software (version 2.4.0). Spectral libraries were built from the images of single-stained tissues with each antibody. The TLSs were then manually distinguished. We collected all TLSs of every tumor section and randomly collected three to five fields from areas outside the TLSs. A total of 958 fields were collected, including 807 TLSs and 151 outside fields of TLS.
The density of TLS was calculated as the number of TLSs per mm2 of the tumor region in the sections. Immune subsets were determined by antibody expression, including CD4+ T cells, CD8+ T lymphocytes (CD3+CD4-), B cells (CD20+), FDC (CD21+), CD3+ TRM (CD3+CD103+), CD4+ TRM (CD4+CD103+), CD8+ TRM (CD3+CD4-CD103+), and GC reaction (CD20+Bcl-6+) (25). The proportion of the immune subsets in each TLS (or field) was calculated as the percentage of this subpopulation to all nucleated cells in the TLS (or field). The proportion of the immune subsets in each patient was calculated by the average proportion in all fields (within the TLS and outside the TLS) across the entire section.
Disease-free survival (DFS) was defined as the time from the date of surgery to tumor recurrence. The surv_cutpoint function in the survminer R package (version 4.1.2) was used to obtain the cutoff value of immune subsets proportion. Then the different immune subsets inside and outside TLS were divided into “high” and “low” groups. Kaplan-Meier curve was drawn with the survminer R package (4.1.2). The log-rank test in survival R package (4.1.2) was used to calculate the P value. Both the survminer and survival R package were downloaded from the public resource website: https://cran.r-project.org/. When comparing the prognostic differences of more than two of sub-groups after combining TLS score and TRM, P value and HR ratio was calculated with log-rank test in GraphPad Prism software.
Chi-square (and Fisher’s exact) test was used to evaluate the relationship between grade score, CD3+ CD103+ TRM and clinicopathological features. Wilcoxon rank test (paired nonparametric t test) was used to compare the difference of CD103+subsets inside and outside TLS. Kruskal-Wallis H test was used to compare the differences of immune subsets proportion among different sub-group. All statistical analyses, except survival analyses, were performed with GraphPad Prism (version 9.1.0, US). P values of < 0.05 were considered statistically significant.
According to the increasing prevalence of FDCs and the maturation of B cells, TLSs were classified into three maturity stages: 1) early TLS (E-TLS), characterized by dense lymphocytic aggregates without CD21 and Bcl-6 expression (Figure 1A); 2) primary follicle-like TLS (PFL-TLS), characterized by lymphocytic clusters with central network CD21 expression, but no GC reaction (Bcl-6-) (Figure 1B); and 3) secondary follicle-like TLS (SFL-TLS), characterized by lymphocytic clusters with GC reaction (CD20+Bcl-6+) (Figure 1C).
Figure 1 Representative images of TLS maturity (magnification, ×200). The slide was stained with CD3 (orange), CD4 (purple), CD20 (green), CD21 (brown), Bcl-6 (red), and DAPI (blue). (A), E-TLS, both FDC and Bcl-6 markers were negative. (B), PFL-TLS, FDC positive and Bcl-6 negative. (C), SFL-TLS, both FDC and Bcl-6 markers were positive.
For the first time, we divided patients into three levels based on the maturity of TLSs: 1) grade 1: patients with TLSs characterized by only E-TLSs, and without PFL-TLSs and SFL-TLSs; 2) grade 2: patients with TLSs characterized by E-TLSs and at least one PFL-TLS, but no SFL-TLS; and 3) grade 3: patients with TLSs characterized by at least one SFL-TLS in the tumor tissue (Table 2).
We first evaluated the prognostic impact of the number and density of TLSs in patients. Kaplan–Meier analysis showed that patients with higher numbers of TLSs had a much better prognosis (median DFS 18.7 months vs. 7.4 months, P = 0.011, Figure 2A, left). A higher density of TLS was also positively associated with a good DFS (median 17.3 months vs. 12.4 months, P = 0.009, Figure 2A, right).
Figure 2 Prognosis impact of the number and density of TLS and patients score. (A) Kaplan–Meier survival curves showing DFS according to the number of TLS (P =0.011) and the density of TLS (P =0.009). (B) Kaplan–Meier survival curves showing DFS according to patients score. P values were calculated by the log-rank test.
Furthermore, we analyzed the prognosis of patients with different grades. The results showed that the prognosis of grade 3 patients was significantly higher than that of those in grade 1 (median DFS 19.5 months vs. 4.3 months, P <0.001, Figure 2B). The grade 2 patients also had a better DFS than those in grade 1 (median 12.6 months vs. 4.3 months, P =0.039). The prognosis of grade 3 patients tended to be better than those in grade 2 (median DFS 19.5 months vs. 12.6 months, P =0.059, Figure 2B). These results indicate that the maturity of TLS is crucial for the prognosis of patients.
By comparing the difference in the proportion of TRM inside and outside the TLS, we determined that all TRM subsets were mainly located in TLS, especially CD4+ TRM (Figures 3A, B). The proportion of CD3+ TRM in TLS (mean ± SD: 1.34% ± 1.13%) was significantly higher than that outside (mean ± SD: 0.71% ± 0.84%), P <0.001. The proportion of CD4+ TRM in TLS (mean ± SD: 0.83% ± 0.75%) was significantly higher than that outside (mean ± SD: 0.26% ± 0.33%), P <0.001. The proportion of CD8+ TRM in TLS (mean ± SD: 0.78% ± 0.72%) was significantly higher than that outside (mean ± SD: 0.55% ± 0.67%), P <0.05.
Figure 3 TRM distribution and its association with prognosis. (A) Representative images of TRM inside (left) and outside (right) TLSs. (B) Comparison of CD3+CD103+ TRM (left, P < 0.001), CD4+CD103+ TRM (middle, P < 0.001), and CD8+CD103+ TRM (right, P < 0.001) distribution inside and outside TLS. TRM were mainly located in TLS. C, Influence of TRM inside and outside of TLS on patient prognosis. TRM inside TLS predicted a better prognosis.
Survival analysis showed that both CD3+ TRM and CD8+ TRM in TLS could predict longer survival (median DFS 17.3 months vs. 6.7 months, 17.5 months vs. 12.7 months, P<0.05; Figure 3C). Likewise, CD4+ TRM within TLS tended to prolong DFS of patients although there was no significant difference between groups (median 15.2 months vs. 6.9 months, P =0.078, Figure 3C). However, TRM outside the TLS had no effect on prognosis.
The above findings suggested that TRM is mainly located within the TLS, and the TRM within the TLS can affect prognosis. Therefore, we next focused our research on TRM inside TLS. The relationships between patient score, CD3+ TRM within TLS, and clinical features of patients including sex, age, TNM stage, micropapillary, EGFR mutation, and smoking are shown in Table 3. The results showed that patients with stage IIIA LUAD had more mature TLS than patients with IIIB LUAD (P =0.041). There were no significant associations between other clinical features and patient scores, as well as between CD3+ TRM and TLS.
Table 3 The relationship between patients score, CD3+CD103+TRM within TLS and various clinical parameters in patients with stage III LUAD (n=49).
Moreover, we analyzed the clinical and immune characteristics that affected the DFS of patients in this study. The results identified some univariate factors that could affect the DFS of patients, including the TLS number, TLS density, patient score, CD20+ B cells in TLS, FDC in TLS, CD103+ TRM, and CD8+CD103+ TRM in TLS, average CD3+ CD103+ TRM, average CD4+CD103+ TRM, and average CD8+CD103+ TRM (Table 4).
Table 4 Univariate analysis of clinical and immune characteristics affecting DFS of patients in the study.
We analyzed the distribution of immune subsets in the TLS in different grades of patients. The results showed that the proportion of CD20+ B cells in grade 3 patients was higher than that in grade 2 and grade 1 patients (mean ± SD: 21.08% ± 6.72% vs. 15.59% ± 4.47%, 21.08% ± 6.72% vs. 11.41% ± 7.84%, P =0.037, and P =0.008, respectively; Figure 4A). The proportion of CD3+ TRM in grade 3 patients was higher than that in grade 2 and grade 1 patients (mean ± SD: 1.98% ± 1.23% vs. 1.10% ± 0.96%, 1.98% ± 1.23% vs. 0.57% ± 0.38%, P =0.027, and P =0.019, respectively; Figure 4A). Patients in grade 3 had higher CD4+ TRM than patients in grade 1 (mean ± SD: 1.33% ± 0.87% vs. 0.59% ± 0.53%, P =0.005; Figure 4A). The proportion of CD8+ TRM in grade 3 patients was higher than that in grade 1 (mean ± SD: 1.06% ± 0.84% vs. 0.69% ± 0.63%, P =0.039, Figure 4A).
Figure 4 The relationship between TRM distribution and TLSs maturation. (A) The distributions of immune subsets within TLS in patients with different TLS scores. The proportion of CD20+ B cell and CD3+CD103+ TRM within TLS in grade 3 patients was significantly higher than that in grade 2 and grade 1 respectively. The proportion of CD4+CD103+ TRM and CD8+CD103+ TRM within TLS in grade 3 patients was significantly higher than that in grade 1. (B) The distribution of TRM in E-TLS, PFL-TLS, and SFL-TLS. The proportions of CD3+CD103+ TRM, CD4+CD103+ TRM, and CD8+CD103+ TRM within SFL-TLS were significantly higher than those in E-TLS and PFL-TLS, respectively. ns, non-significant.
Next, we analyzed the proportion of TRM in all 807 TLSs, including E-TLSs, PFL-TLSs, and SFL-TLSs. The proportion of CD3+ TRM in SFL-TLS was significantly higher than that of E-TLS and PFL-TLS (mean ± SD: 3.60% ± 6.80% vs. 1.57% ± 2.71%, 3.60% ± 6.80% vs. 1.23% ± 1.62%, P<0.001; Figure 4B), respectively. The proportion of CD4+ TRM in SFL-TLS was significantly higher than that in E-TLS and PFL-TLS (mean ± SD: 2.17% ± 2.19% vs. 0.98% ± 2.21%, 2.17% ± 2.19% vs. 0.67% ± 1.19%, P<0.001; Figure 4B), respectively. The proportion of CD8+ TRM in SFL-TLS was significantly higher than that in E-TLS and PFL-TLS (mean ± SD: 1.30% ± 1.78% vs. 0.98% ± 2.46%, 1.30% ± 1.78% vs. 0.81% ± 1.41%, P<0.001; Figure 4B), respectively.
Patients were stratified into four groups according to the proportion of CD103+ TRM and patient scores. The prognosis of patients in the group of CD3CD103High and grade 3 was significantly higher than that of CD3CD103High and grade 1 + 2 (median DFS 19.7 months vs. 12.7 months, P =0.046) and that of CD3CD103Low and grade 1 + 2 (median DFS 19.7 months vs. 7.2 months, P =0.003), respectively (Figure 5A). Patients in the CD4CD103High and grade 3 groups had a significantly better prognosis than those in CD4CD103High and grade 1 + 2 (median DFS 19.5 months vs. 12.6 months, P =0.037) and in CD4CD103Low and grade 1 + 2 (median DFS 19.5 months vs. 6.9 months, P =0.011), respectively (Figure 5B). Similarly, the prognosis of patients in the group of CD8CD103High and grade 3 tended to be better than that of CD8CD103High and grade 1 + 2 (median DFS 19.7 months vs. 12.8 months, P =0.052), and significantly higher than that of CD8CD103Low and grade 1 + 2 (median DFS 19.7 months vs. 7.3 months, P <0.001; Figure 5C). However, there was no significant difference in prognosis between patients in the CD103High and grade 1 + 2 groups and the CD103Low and grade 1 + 2 groups, regardless of the CD3+ TRM, CD4+ TRM, or CD8+ TRM subsets.
Figure 5 The relationship between TRM, patient score and prognosis. DFS was shown with Kaplan–Meier plots according to the combination of TRM and patient score. (A) DFS of patients in the group of CD3CD103High and grade 3 (median 19.7 months) was significantly higher than that of CD3CD103High and grade 1 + 2 (median 12.7 months) and that of CD3CD103Low and grade 1 + 2 (median 7.2 months), respectively, P<0.05. (B) DFS of patients in the group of CD4CD103High and grade 3 (median 19.5 months) was significantly higher than that of CD4CD103High and grade 1 + 2 (median 12.6 months) and that of CD4CD103Low and grade 1 + 2 (median 6.9 months), respectively, P<0.05. (C). DFS of patients in the group of CD8CD103High and grade 3 tended to be better than that of CD8CD103High and grade 1 + 2 (median 19.7 months vs. 12.8 months, P=0.052), and significantly higher than that of CD8 CD103Low and grade 1 + 2 (median DFS 19.7 months vs. 7.3 months, P < 0.001).
In the present study, we evaluated the relationship among TLS maturity, clinical characteristics, and prognosis of patients with stage III LUAD. Although there is no standardized classification of TLS maturity, we used the classification method of TLS that Winder (26) had reported in colorectal carcinoma, and classified the TLSs into three mature stages, including E-TLSs, PFL-TLSs, and SFL-TLSs. For the first time, we divided patients into three levels based on the mature state of TLSs: 1) grade 1: only E-TLSs with no PFL-TLSs and SFL-TLSs; 2) grade 2: E-TLSs and PFL-TLSs in the tumor, and without SFL-TLS; and 3) grade 3: possess at least one SFL-TLS in the tumor tissue. The results showed that patients in grade 3 had the best DFS, followed by grade 2. The DFS of patients in grade 1 was the worst. This was consistent with the findings in colorectal cancer and lung squamous cell carcinoma that found that patients with GC reaction had a better prognosis (26, 27). This indicates that B cell maturity and humoral immunity play pivotal roles in the anti-tumor immune response.
In addition, we evaluated the distribution of CD4+ TRM cells and CD8+ TRM cells in the tumor tissue and found that the proportion of TRM within TLSs was significantly higher than that outside, especially CD4+ TRM. The proportion of TRM within TLSs was positively correlated with the prognosis of patients, while there was no significant association between the proportion of TRM outside TLSs and prognosis. Furthermore, we compared the proportions of different immune subsets in LUAD patients of different grades. The proportions of CD20+ B cells and CD3+ TRM in grade 3 patients were significantly higher than those in grade 1 and grade 2, respectively. The proportions of CD4+CD103+ TRM and CD8+CD103+ TRM were significantly higher in grade 3 patients than in grade 1 patients (P<0.05). We then analyzed the proportion difference of TRM in different maturities of TLSs. The proportions of CD3+ TRM, CD4+ TRM, and CD8+ TRM in SFL-TLSs were significantly higher than those in E-TLSs and PFL-TLSs, respectively (P<0.05). All these results indicate that there is a close relationship between TRM and TLS maturity.
In the subsequent prognosis analysis, the data showed that patients with both more mature TLSs and a higher proportion of CD103+ TRM had a much better prognosis. CD3+ TRM, CD4+ TRM, and CD8+ TRM showed similar results. The data further confirmed that CD103+ TRM was closely related to the maturation of TLSs.
Although the exact mechanism by which TRM preferentially located into TLS had not been clarified, it was reported that CXCL13 was the key molecular determinant of TLS formation in the TME (27–30). Activated CD103+CTLs were involved in the migration of B cells to tumor via production of CXCL13. The high mutation load and CD8+ T cell–rich tumors showed higher expression of CXCL13 and ITGAE (CD103) and that they presented with significantly higher numbers of B cells in a variety of tumors (30). A previous study on the distribution of CD8+CD103+ TRM in gastric carcinoma reported similar results. CD103+ T cells were located around TLSs, and patients with CD103High had more TLSs (24). Furthermore, patients who were CD103high and TLSrich had a better prognosis than other groups (24). However, this study mainly focused on CD8+ subsets and there was no analysis of the relationship between TLS maturity and CD103+ TRM. Another study identified a new subset of CD4+ Th-CXCL13 with tumor-resident gene characteristics in NPC (31). CD4+ Th-CXCL13 recruits tumor-associated B cells and induces plasma cell differentiation and immunoglobulin production through interleukin-21 (IL-21) secretion and CD84 interactions in TLSs. In a mouse model of influenza viral infection, Young Min Son et al. reported a population of lung-resident helper CD4+ T cells (CD4+ TRH) that developed after viral clearance. They found that the formation of CD4+ TRH is dependent on transcription factors involved in the feather of follicular T cells and resident T cells, including BCL6 and Bhlhe40. CD4+ TRH could promote the development of protective B cells and CD8+ T cell responses through IL-21 dependent mechanism (32). Moreover, B cells in TLSs can function as antigen-presenting cells; they highly express the co-stimulatory molecules CD86 and CD80 and facilitate tumor antigen-specific T-cell responses, including CD8+ TIL and CD4+ TIL responses (33). Bradley et al. have demonstrated that B cells play important roles in memory CD4+ T cell generation and differentiation because mice in a B cell knockout model did not develop memory CD4+ T cells (34). These results indicated that there might be synergy function between TRM and TLSs in the antitumor response.
In conclusion, our data highlight the proportion of TRM within TLSs was significantly increased with the maturation of TLSs. When we divided patients into three levels including grade 1, grade 2 and grade 3 according to the presence of different maturity of TLSs, the proportions of CD4+CD103+TRM and CD8+CD103+TRM in grade 3 of patients were significantly higher than grade 1 and grade 2. These results indicate a close relationship between CD103+TRM and TLS maturity. Furthermore, patients with a combination feature of grade 3 and CD103+ exhibited a good prognosis. The combination of TLS maturity and CD103+ TRM proportion could be used as a biomarker to predict the prognosis of LUAD patients.
The original contributions presented in the study are included in the article/Supplementary Material. Further inquiries can be directed to the corresponding authors.
The studies involving human participants were reviewed and approved by Ethics Committee of the Tianjin Medical University Cancer Institute and Hospital. The patients/participants provided their written informed consent to participate in this study.
HZ and XR designed the experiments. HW performed the experiments. HZ and HW performed the analyses and wrote the manuscript. HW and YZ collected clinical data. QS and XR revised the manuscript. All authors have commented on and approved the manuscript. HZ and HW contributed equally to this work. All authors contributed to the article and approved the submitted version.
The work in this study was supported by a grant from the National Natural Science Foundation of China (Grant No. U20A20375).
The authors declare that the research was conducted in the absence of any commercial or financial relationships that could be construed as a potential conflict of interest.
All claims expressed in this article are solely those of the authors and do not necessarily represent those of their affiliated organizations, or those of the publisher, the editors and the reviewers. Any product that may be evaluated in this article, or claim that may be made by its manufacturer, is not guaranteed or endorsed by the publisher.
We sincerely appreciate the colleagues in the Department of Pathology for their help in the pathological diagnosis and tumor section preparation. The authors would like to thank all the patients for their consent to participate in this study.
The Supplementary Material for this article can be found online at: https://www.frontiersin.org/articles/10.3389/fimmu.2022.877689/full#supplementary-material
1. Hsu ML, Naidoo J. Principles of Immunotherapy in non-Small Cell Lung Cancer. Thorac Surg Clin (2020) 30(2):187–98. doi: 10.1016/j.thorsurg.2020.01.009
2. Suresh K, Naidoo J, Lin CT, Danoff S. Immune Checkpoint Immunotherapy for non-Small Cell Lung Cancer: Benefits and Pulmonary Toxicities. Chest (2018) 154(6):1416–23. doi: 10.1016/j.chest.2018.08.1048
3. Tumeh PC, Harview CL, Yearley JH, Shintaku IP, Taylor EJ, Robert L, et al. PD-1 Blockade Induces Responses by Inhibiting Adaptive Immune Resistance. Nature (2014) 515(7528):568–71. doi: 10.1038/nature13954
4. Helmink BA, Reddy SM, Gao J, Zhang S, Basar R, Thakur R, et al. B Cells and Tertiary Lymphoid Structures Promote Immunotherapy Response. Nature (2020) 577(7791):549–55. doi: 10.1038/s41586-019-1922-8
5. Cabrita R, Lauss M, Sanna A, Donia M, Skaarup Larsen M, Mitra S, et al. Tertiary Lymphoid Structures Improve Immunotherapy and Survival in Melanoma. Nature (2020) 577(7791):561–5. doi: 10.1038/s41586-019-1914-8
6. Petitprez F, de Reyniès A, Keung EZ, Chen TW, Sun CM, Calderaro J, et al. B Cells are Associated With Survival and Immunotherapy Response in Sarcoma. Nature (2020) 577(7791):556–60. doi: 10.1038/s41586-019-1906-8
7. Sautès-Fridman C, Petitprez F, Calderaro J, Fridman WH. Tertiary Lymphoid Structures in the Era of Cancer Immunotherapy. Nat Rev Cancer (2019) 19(6):307–25. doi: 10.1038/s41568-019-0144-6
8. Zhao H, Wang H, Zhou Q, Ren X. Insights Into Tertiary Lymphoid Structures in the Solid Tumor Microenvironment: Anti-Tumor Mechanism, Functional Regulation, and Immunotherapeutic Strategies. Cancer Biol Med (2021) 18(4):981–91. doi: 10.20892/j.issn.2095-3941.2021.0029
9. Dieu-Nosjean MC, Antoine M, Danel C, Heudes D, Wislez M, Poulot V, et al. Long-Term Survival for Patients With non-Small-Cell Lung Cancer With Intratumoral Lymphoid Structures. J Clin Oncol (2008) 26(27):4410–7. doi: 10.1200/JCO.2007.15.0284
10. Kroeger DR, Milne K, Nelson BH. Tumor-Infiltrating Plasma Cells are Associated With Tertiary Lymphoid Structures, Cytolytic T-Cell Responses, and Superior Prognosis in Ovarian Cancer. Clin Cancer Res (2016) 22(12):3005–15. doi: 10.1158/1078-0432.CCR-15-2762
11. Rodriguez AB, Engelhard VH. Insights Into Tumor-Associated Tertiary Lymphoid Structures: Novel Targets for Antitumor Immunity and Cancer Immunotherapy. Cancer Immunol Res (2020) 8(11):1338–45. doi: 10.1158/2326-6066.CIR-20-0432
12. Munoz-Erazo L, Rhodes JL, Marion VC, Kemp RA. Tertiary Lymphoid Structures in Cancer – Considerations for Patient Prognosis. Cell Mol Immunol (2020) 17(6):570–5. doi: 10.1038/s41423-020-0457-0
13. Yamakoshi Y, Tanaka H, Sakimura C, Deguchi S, Mori T, Tamura T, et al. Immunological Potential of Tertiary Lymphoid Structures Surrounding the Primary Tumor in Gastric Cancer. Int J Oncol (2020) 57(1):171–82. doi: 10.3892/ijo.2020.5042
14. Martinet L, Garrido I, Filleron T, Le Guellec SL, Bellard E, Fournie JJ, et al. Human Solid Tumors Contain High Endothelial Venules: Association With T- and B-Lymphocyte Infiltration and Favorable Prognosis in Breast Cancer. Cancer Res (2011) 71(17):5678–87. doi: 10.1158/0008-5472.CAN-11-0431
15. Djenidi F, Adam J, Goubar A, Durgeau A, Meurice G, de Montpréville V, et al. CD8+CD103+ Tumor-Infiltrating Lymphocytes are Tumor-Specific Tissue-Resident Memory T Cells and a Prognostic Factor for Survival in Lung Cancer Patients. J Immunol (2015) 194(7):3475–86. doi: 10.4049/jimmunol.1402711
16. Ganesan AP, Clarke J, Wood O, Garrido-Martin EM, Chee SJ, Mellows T, et al. Tissue-Resident Memory Features are Linked to the Magnitude of Cytotoxic T Cell Responses in Human Lung Cancer. Nat Immunol (2017) 18(8):940–50. doi: 10.1038/ni.3775
17. Schenkel JM, Masopust D. Tissue-Resident Memory T Cells. Immunity (2014) 41(6):886–97. doi: 10.1016/j.immuni.2014.12.007
18. Park SL, Gebhardt T, Mackay LK. Tissue-Resident Memory T Cells in Cancer Immunosurveillance. Trends Immunol (2019) 40(8):735–47. doi: 10.1016/j.it.2019.06.002
19. Oja AE, Piet B, van der Zwan D, Blaauwgeers H, Mensink M, de Kivit S, et al. Functional Heterogeneity of CD4+ Tumor-Infiltrating Lymphocytes With a Resident Memory Phenotype in NSCLC. Front Immunol (2018) 9:2654. doi: 10.3389/fimmu.2018.02654
20. Gaudreau PO, Negrao MV, Mitchell KG, Reuben A, Corsini EM, Li J, et al. Neoadjuvant Chemotherapy Increases Cytotoxic T Cell, Tissue Resident Memory T Cell, and B Cell Infiltration in Resectable NSCLC. J Thorac Oncol (2021) 16(1):127–39. doi: 10.1016/j.jtho.2020.09.027
21. Ida S, Takahashi H, Kawabata-Iwakawa R, Mito I, Tada H, Chikamatsu K. Tissue-Resident Memory T Cells Correlate With the Inflammatory Tumor Microenvironment and Improved Prognosis in Head and Neck Squamous Cell Carcinoma. Oral Oncol (2021) 122:105508. doi: 10.1016/j.oraloncology.2021.105508
22. Savas P, Virassamy B, Ye C, Salim A, Mintoff CP, Caramia F, et al. Single-Cell Profiling of Breast Cancer T Cells Reveals a Tissue-Resident Memory Subset Associated With Improved Prognosis. Nat Med (2018) 24(7):986–93. doi: 10.1038/s41591-018-0078-7
23. Banchereau R, Chitre AS, Scherl A, Wu TD, Patil NS, de Almeida P, et al. Intratumoral CD103+ CD8+ T Cells Predict Response to PD-L1 Blockade. J Immunother Cancer (2021) 9(4):e002231. doi: 10.1136/jitc-2020-002231
24. Mori T, Tanaka H, Suzuki S, Deguchi S, Yamakoshi Y, Yoshii M, et al. Tertiary Lymphoid Structures Show Infiltration of Effective Tumor-Resident T Cells in Gastric Cancer. Cancer Sci (2021) 112(5):1746–57. doi: 10.1111/cas.14888
25. Yamaguchi K, Ito M, Ohmura H, Hanamura F, Nakano M, Tsuchihashi K, et al. Helper T Cell-Dominant Tertiary Lymphoid Structures are Associated With Disease Relapse of Advanced Colorectal Cancer. Oncoimmunology (2020) 9(1):1724763. doi: 10.1080/2162402X.2020.1724763
26. Posch F, Silina K, Leibl S, Mündlein A, Moch H, Siebenhüner A, et al. Maturation of Tertiary Lymphoid Structures and Recurrence of Stage II and III Colorectal Cancer. Oncoimmunology (2018) 7(2):e1378844. doi: 10.1080/2162402X.2017.1378844
27. Siliņa K, Soltermann A, Attar FM, Casanova R, Uckeley ZM, Thut H, et al. Germinal Centers Determine the Prognostic Relevance of Tertiary Lymphoid Structures and are Impaired by Corticosteroids in Lung Squamous Cell Carcinoma. Cancer Res (2018) 78(5):1308–20. doi: 10.1158/0008-5472.CAN-17-1987
28. Gräbner R, Lötzer K, Döpping S, Hildner M, Radke D, Beer M, et al. Lymphotoxin Beta Receptor Signaling Promotes Tertiary Lymphoid Organogenesis in the Aorta Adventitia of Aged ApoE-/- Mice. J Exp Med (2009) 206(1):233–48. doi: 10.1084/jem.20080752
29. Fleige H, Ravens S, Moschovakis GL, Bölter J, Willenzon S, Sutter G, et al. IL-17-Induced CXCL12 Recruits B Cells and Induces Follicle Formation in BALT in the Absence of Differentiated FDCs. J Exp Med (2014) 211(4):643–51. doi: 10.1084/jem.20131737
30. Workel HH, Lubbers JM, Arnold R, Prins TM, van der Vlies P, de Lange K, et al. A Transcriptionally Distinct Cxcl13+Cd103+Cd8+ T-Cell Population Is Associated With B-Cell Recruitment and Neoantigen Load in Human Cancer. Cancer Immunol Res (2019) 7(5):784–96. doi: 10.1158/2326-6066.CIR-18-0517
31. Li JP, Wu CY, Chen MY, Liu SX, Yan SM, Kang YF, et al. PD-1+CXCR5-CD4+ Th-CXCL13 Cell Subset Drives B Cells Into Tertiary Lymphoid Structures of Nasopharyngeal Carcinoma. J Immunother Cancer (2021) 9(7):e002101. doi: 10.1136/jitc-2020-002101
32. Son YM, Cheon IS, Wu Y, Li C, Wang Z, Gao X, et al. Tissue-Resident CD4+ T Helper Cells Assist the Development of Protective Respiratory B and CD8+ T Cell Memory Responses. Sci Immunol (2021) 6(55):eabb6852. doi: 10.1126/sciimmunol.abb6852
33. Behr DS, Peitsch WK, Hametner C, Lasitschka F, Houben R, Schönhaar K, et al. Prognostic Value of Immune Cell Infiltration, Tertiary Lymphoid Structures and PD-L1 Expression in Merkel Cell Carcinomas. Int J Clin Exp Pathol (2014) 7(11):7610–21.
Keywords: TRM, TLS, B cell, TIL, lung adenocarcinoma
Citation: Zhao H, Wang H, Zhao Y, Sun Q and Ren X (2022) Tumor-Resident T Cells, Associated With Tertiary Lymphoid Structure Maturity, Improve Survival in Patients With Stage III Lung Adenocarcinoma. Front. Immunol. 13:877689. doi: 10.3389/fimmu.2022.877689
Received: 17 February 2022; Accepted: 21 April 2022;
Published: 19 May 2022.
Edited by:
Mercedes Beatriz, CONICET Instituto de Biología y Medicina Experimental (IBYME), ArgentinaReviewed by:
Shahram Salek-Ardakani, Pfizer, United StatesCopyright © 2022 Zhao, Wang, Zhao, Sun and Ren. This is an open-access article distributed under the terms of the Creative Commons Attribution License (CC BY). The use, distribution or reproduction in other forums is permitted, provided the original author(s) and the copyright owner(s) are credited and that the original publication in this journal is cited, in accordance with accepted academic practice. No use, distribution or reproduction is permitted which does not comply with these terms.
*Correspondence: Xiubao Ren, cmVueGl1YmFvQHRqbXVjaC5jb20=; Qian Sun, c3VucWlhbjkyM0AxMjYuY29t
†These authors have contributed equally to this work
Disclaimer: All claims expressed in this article are solely those of the authors and do not necessarily represent those of their affiliated organizations, or those of the publisher, the editors and the reviewers. Any product that may be evaluated in this article or claim that may be made by its manufacturer is not guaranteed or endorsed by the publisher.
Research integrity at Frontiers
Learn more about the work of our research integrity team to safeguard the quality of each article we publish.