- 1Nature Research Centre, Akademijos 2, Vilnius, Lithuania
- 2ANSES, INRAE, Ecole Nationale Vétérinaire d’Alfort, UMR BIPAR, Laboratoire de Santé Animale, Maisons-Alfort, France
- 3SEPPIC Paris La Défense, La Garenne Colombes, France
- 4School of Environmental Sciences, University of Guelph, Guelph, ON, Canada
Animal and human pathogens that are transmitted by arthropods are a global concern, particularly those vectored by mosquitoes (e.g., Plasmodium spp. and dengue virus). Vector microbiota may hold the key to vector-borne pathogen control, as mounting evidence suggests that the contributions of the vector microbiota to vector physiology and pathogen life cycle are so relevant that vectorial capacity cannot be understood without considering microbial communities within the vectors. Anti-tick microbiota vaccines targeting commensal bacteria of the vector microbiota alter vector feeding and modulate the taxonomic and functional profiles of vector microbiome, but their impact on vector-borne pathogen development within the vector has not been tested. In this study, we tested whether anti-microbiota vaccination in birds targeting Enterobacteriaceae within mosquito midguts modulates the mosquito microbiota and disrupt Plasmodium relictum development in its natural vector Culex quinquefasciatus. Domestic canaries (Serinus canaria domestica) were experimentally infected with P. relictum and/or immunized with live vaccines containing different strains of Escherichia coli. Immunization of birds induced E. coli-specific antibodies. The midgut microbial communities of mosquitoes fed on Plasmodium-infected and/or E. coli-immunized birds were different from those of mosquitoes fed on control birds. Notably, mosquito midgut microbiota modulation was associated with a significant decrease in the occurrence of P. relictum oocysts and sporozoites in the midguts and salivary glands of C. quinquefasciatus, respectively. A significant reduction in the number of oocysts was also observed. These findings suggest that anti-microbiota vaccines can be used as a novel tool to control malaria transmission and potentially other vector-borne pathogens.
Introduction
Mosquitoes are vectors of major human diseases such as dengue (caused by dengue virus), and malaria (caused by Plasmodium spp.) (1). According to literature, there are more than 50 avian Plasmodium species and new species are discovered every year (2) and infection by these parasites is common in some bird species (3). Among the Plasmodium species affecting birds, P. relictum is listed among the most invasive organisms in the world, infecting more than 300 bird species and is prevalent all around the world (4). Plasmodium relictum is transmitted mostly by Culex mosquitoes, including Culex pipiens and Culex quinquefasciatus (5). The midgut is the first organ in which P. relictum ingested with the host blood can survive (3). From the midgut lumen, the parasite traverses the peritrophic membrane and epithelial layer of the midgut and develops to oocysts (3). The oocysts invade other vector tissues such as salivary glands to complete the sporogonic development (3). In general, the susceptibility of mosquitoes to Plasmodium parasites infection is under genetic control (6–8), but the large variability in oocyst number among closely related mosquitoes indicates that environmental factors also play a role. Of special interest are the interactions between the vector, its microbiota and transmitted pathogens, since commensal bacteria interact with mosquito-borne pathogens (9) and can facilitate (10) or compete (11) with pathogen colonization and development within the vector midguts, prompting research into microbiota manipulation and transmission-blocking strategies (12). Depleting vector microbiota from bacteria that facilitates pathogen development could be exploited as a mean for blocking transmission. For example, the bacterium Asaia bogorensis increases midgut pH promoting Plasmodium berghei gametogenesis within Anopheles stephensi (10), and high abundance of Enterobacteriaceae increases Plasmodium falciparum infection in Anopheles gambiae midgut (13), making the reduction of these bacterial species a sound strategy to reduce pathogen infection in the vector and potentially block transmission to the host.
Although targeting specific commensal bacteria could block pathogen transmission, the lack of tools for the precise manipulation of the vector microbiota is currently a major limitation to developing novel transmission-blocking strategies. Specific host antibodies (Abs) are easily induced by vaccination, and once taken with the blood meal, they remain functional within the vector tissues and can bind symbionts (14) and other bacterial microbiota (15) within hematophagous arthropods (16). Surprisingly, host Abs specific to bacterial microbiota had never been used for microbiota manipulation and transmission blocking strategies (16). It was recently shown that anti-microbiota vaccines modulate the tick microbiota in a taxon-specific manner (17). Firstly, combining next-generation sequencing (NGS) and network analysis, Enterobacteriaceae was identified as a keystone taxon (i.e., highly connected taxa driving community composition and function) in the microbiota of Ixodes ricinus ticks (17, 18). Secondly, the abundance of vector-associated Enterobacteriaceae decreased in ticks fed on mice immunized with a live bacteria vaccine containing Escherichia coli (17). Thirdly, vaccination against Enterobacteriaceae had cascading ecological impacts on the whole tick microbiome by reducing bacterial diversity and modulating the functional profiles of the microbiome (17). Fourthly, decreased Enterobacteriaceae abundance was correlated with high levels of E. coli-specific Abs (17). Last but not least, no mortality or sign of pain were observed after the vaccination in the mice (17, 18) and the fecal microbiota of immunized mice showed no significant alterations (17). Here we tested whether modulation of mosquito microbiota by anti-microbiota vaccination of host birds against commensal Enterobacteriaceae disrupts P. relictum development within midguts and salivary glands of the vector C. quinquefasciatus.
Results
Anti-Microbiota Vaccination in Birds Interferes With Plasmodium-Induced Modulation of Mosquito Midgut Microbiota
Immunization was followed by experimental malaria infection and mosquito infestation (Figure 1). Anti-microbiota vaccination of birds with E. coli O86:B7 or E. coli BL21 increased the levels of IgY specific to E. coli (Figure 2A). Anti-E. coli IgY also increased in the sera of birds that received both anti-microbiota vaccines and infection with P. relictum (Figure 2A). No significant change was observed in the levels of anti-E. coli Abs in birds that received the mock vaccination (PBS) or those only infected with Plasmodium (Figure 2A). Following the bacterial immunization and P. relictum infection in birds, C. quinquefasciatus mosquitoes were allowed to feed on the birds to acquire the parasites and/or anti-E. coli Abs. The midguts of fed mosquitoes were dissected and used for microbiota analysis.
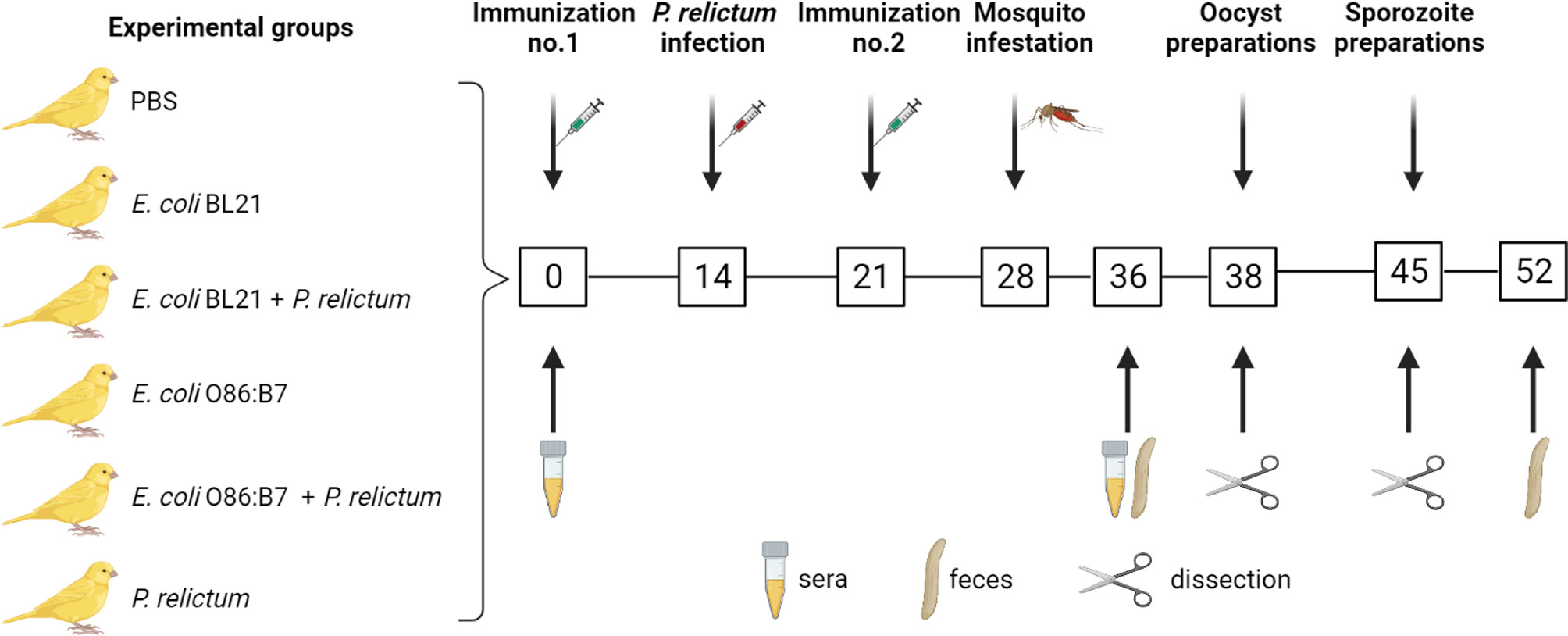
Figure 1 Experimental design and sample collection. Canaries were immunized twice (at day 0 and day 21) with a live vaccine containing E. coli BL21 (n = 7) or E. coli O86:B7 (n =7) or with a mock vaccine (PBS) (n = 3). A group of canaries (n = 5) and part of the birds vaccinated with E. coli BL21 (n = 4) or E. coli O86:B7 (n = 4) were infected with P. relictum at day 14. The birds were used as donors to feed C. quinquefasciatus (n = 20-50 mosquitoes per bird). The midgut of mosquitoes was dissected for microbiota (day 38 and day 45) and oocyst (day 38) analysis and salivary glands for sporozoite (day 45) analysis. Bird sera (used for ELISA and immunofluorescence) and fecal (used for bird gut microbiota analysis) samples were collected at different time points as indicated.
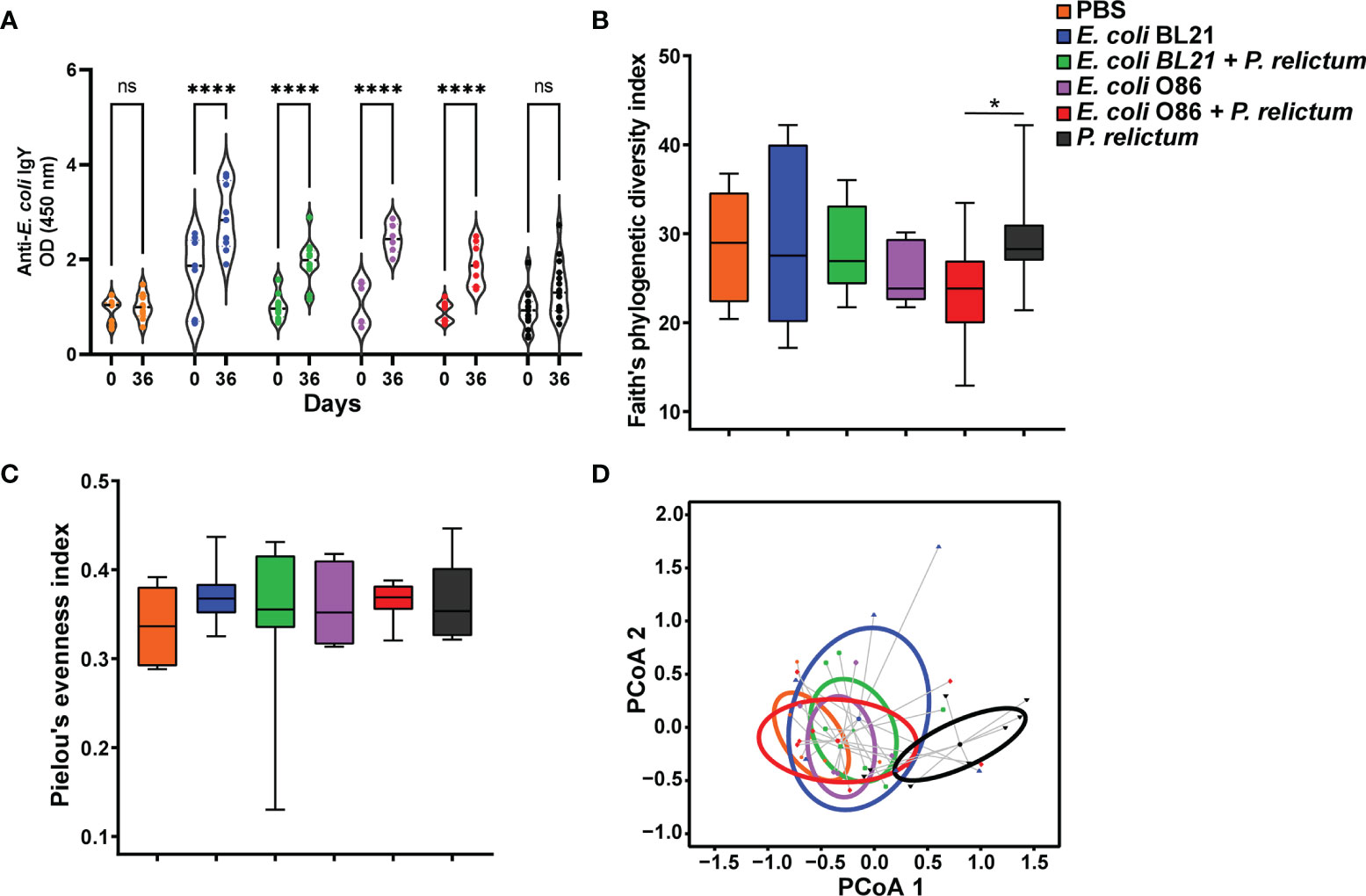
Figure 2 Impact of anti-microbiota vaccine and P. relictum infection on bird antibody response and mosquitoes’ microbial diversity (A). Levels of IgY specific to E. coli proteins were measured by semiquantitative ELISA in sera of canaries before immunization (day 0) and in sera of canaries immunized with a mock vaccine, E. coli BL21 and E. coli O86:B7 alone or challenged with P. relictum (day 36 after first vaccination) (****p < 0.00001; ns, not significant). (B) Faith’s phylogenetic diversity and (C) Pielou’s evenness indexes were used to measure the richness and evenness, respectively, of microbiota of mosquitoes fed on canaries immunized with a mock vaccine, E. coli BL21 and E. coli O86:B7 alone or challenged with P. relictum. The asterisk indicates significative differences between groups (pairwise Kruskal-wallis, p = 0.03). (D) Beta diversity of mosquito microbiota in the different experimental conditions represented in PCoA plot obtained by Betadisper function. There were no differences in the intragroup dispersions (variances) (ANOVA, p = 0.426), whereas differences were found in the community composition between groups (PERMANOVA, p = 0.001), specifically the group infected with P. relictum alone was different from the other groups. Taxonomic profiles at the level of ASVs, used to measure the alpha and beta diversity, were obtained from 16S rRNA sequences from midgut of mosquitoes fed on mock-immunized (n = 5 mosquitoes midgut pool), E. coli O86:B7-immunized (n = 5 mosquitoes midgut pool), E. coli O86:B7-immunized and challenged with P. relictum (n = 8 mosquitoes midgut pool) and challenged only with P. relictum (n = 8 mosquitoes midgut pool).
Bacterial community composition and diversity were compared between groups to assess the impact of anti-microbiota vaccines and P. relictum infection on the mosquito midgut microbiota. Analysis of alpha diversity indexes showed that overall, the phylogenetic richness (Figure 2B) and evenness (Figure 2C) did not differ between experimental groups (Kruskal-Wallis, p > 0.05). However, pairwise comparisons between groups revealed a reduced taxonomic richness in mosquitoes from E. coli O86:B7 + P. relictum group compared to P. relictum-infected mosquitoes (Kruskal-Wallis, p = 0.036, Figure 2B). Beta diversity analysis revealed a significant difference in Bray Curtis dissimilarity index between the groups (PERMANOVA, F = 2.40, p = 0.001). Specifically, Plasmodium-infected mosquito microbiota shows a tendency to separate from the other groups. Furthermore, dispersion analysis of Bray Curtis dissimilarity index did not show significant differences between groups (BetaDisper F= 1.01, p = 0.426, Figure 2D).
Further characterization of the impact of anti-E. coli O86:B7 immunization and Plasmodium infection on mosquito midgut microbiota was achieved using differential abundance analysis. Significant changes in the abundance of 20 and 7 bacterial genera were detected in the midgut microbiota of mosquitoes fed on P. relictum-infected (Figures 3A, B) or E. coli O86:B7-immunized canaries (Figures 3C, D), respectively, compared to those fed on mock-immunized canaries. This suggests that P. relictum infection and the anti-microbiota vaccine disturb the mosquito microbiota. We next asked whether anti-microbiota vaccination could interfere with pathogen-induced modulation of the mosquito microbiota. The midgut microbiota of mosquito exposed to both P. relictum infection and anti-E. coli O86:B7 Abs had 23 and four taxa with significant changes in abundance compared to mosquitoes fed on Plasmodium-infected (Figures 3E, F), and E. coli O86:B7-immunized canaries (Figures 3G, H), respectively. Vaccination with E. coli BL21 was also associated with changes in the abundance of several bacterial taxa, compared with the control mock-vaccinated and with Plasmodium infection groups (Figure 4). Notably, compared with the mock-vaccinated group, the bacterial taxa affected by infection or vaccination alone were different between them and from those affected simultaneously by vaccination and infection (Figure 5). These results suggest that P. relictum infection and the anti-microbiota vaccines modulate the mosquito midgut microbiota in different ways and that the anti-microbiota vaccines interfere with the Plasmodium-induced modulation of the mosquito microbiota.
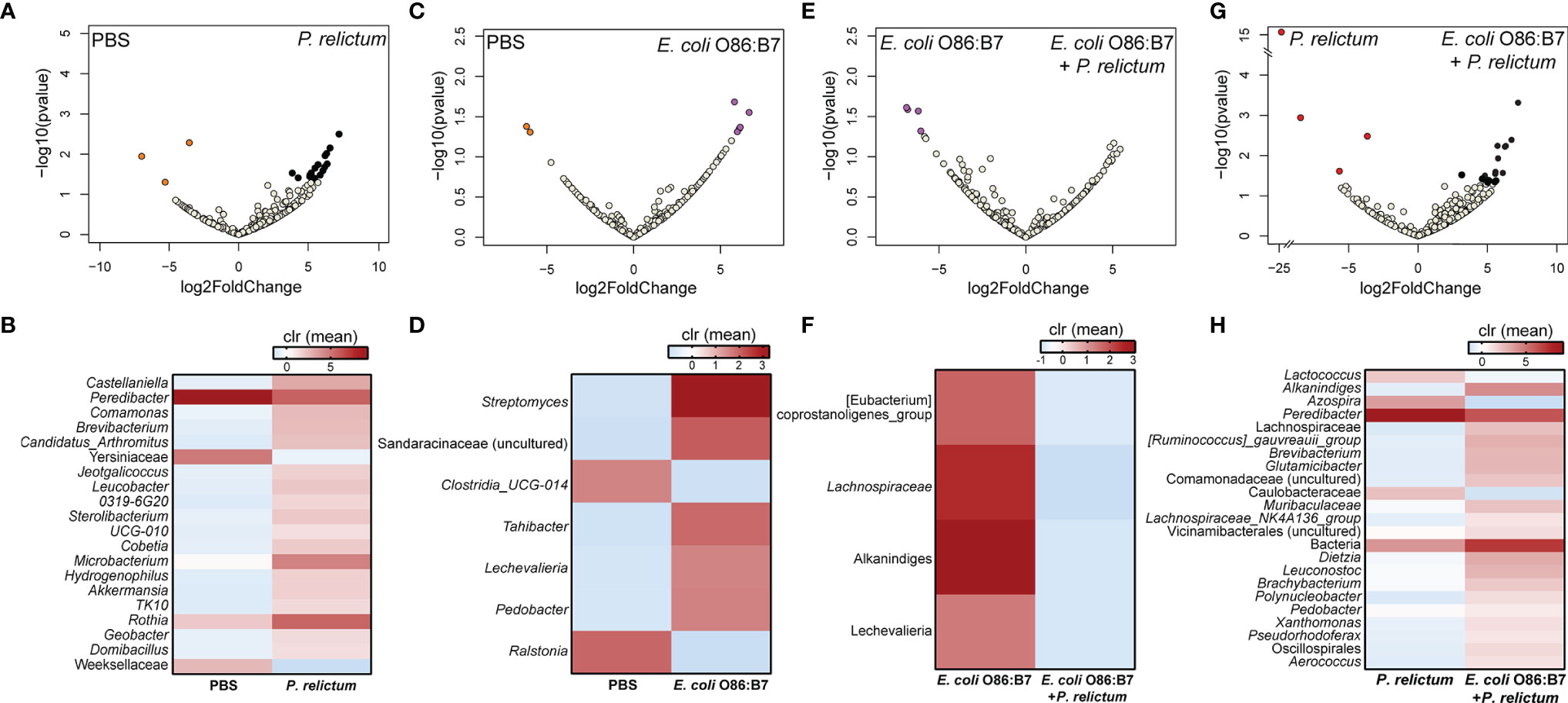
Figure 3 Impact of E. coli O86:B7 vaccine and P. relictum infection on the taxonomic profiles of mosquito microbiota. Volcano plot showing the differential bacterial abundance in mosquito microbiota between different experimental groups (A) PBS vs. P. relictum, (C) PBS vs. E. coli O86:B7, (E) E. coli O86:B7 vs. E. coli O86:B7+P. relictum and (G) P. relictum vs. E. coli O86:B7+P. relictum. Taxa with significant differences (Wald test, p < 0.05) between the groups are represented with colored dots (i.e., orange, purple, red and black). The gray dots represent taxa with no significant differences between groups. Heatmaps representing the relative abundance (expressed as clr) of taxa with significant differences between (B) PBS vs. P. relictum, (D) PBS vs. E. coli O86:B7, (F) E. coli O86:B7 vs. E. coli O86:B7+P. relictum and (H) P. relictum vs. E. coli O86:B7+P. relictum. Taxa with significant differences in their abundance were identified using DeSeq2 algorithm. The taxonomic profiles were obtained from 16S rRNA sequences from midgut of mosquitoes fed on mock-immunized (n = 5 mosquitoes midgut pool), E. coli O86:B7-immunized (n = 5 mosquitoes midgut pool), E. coli O86:B7-immunized and challenged with P. relictum (n = 8 mosquitoes midgut pool) and challenged only with P. relictum (n = 8 mosquitoes midgut pool).
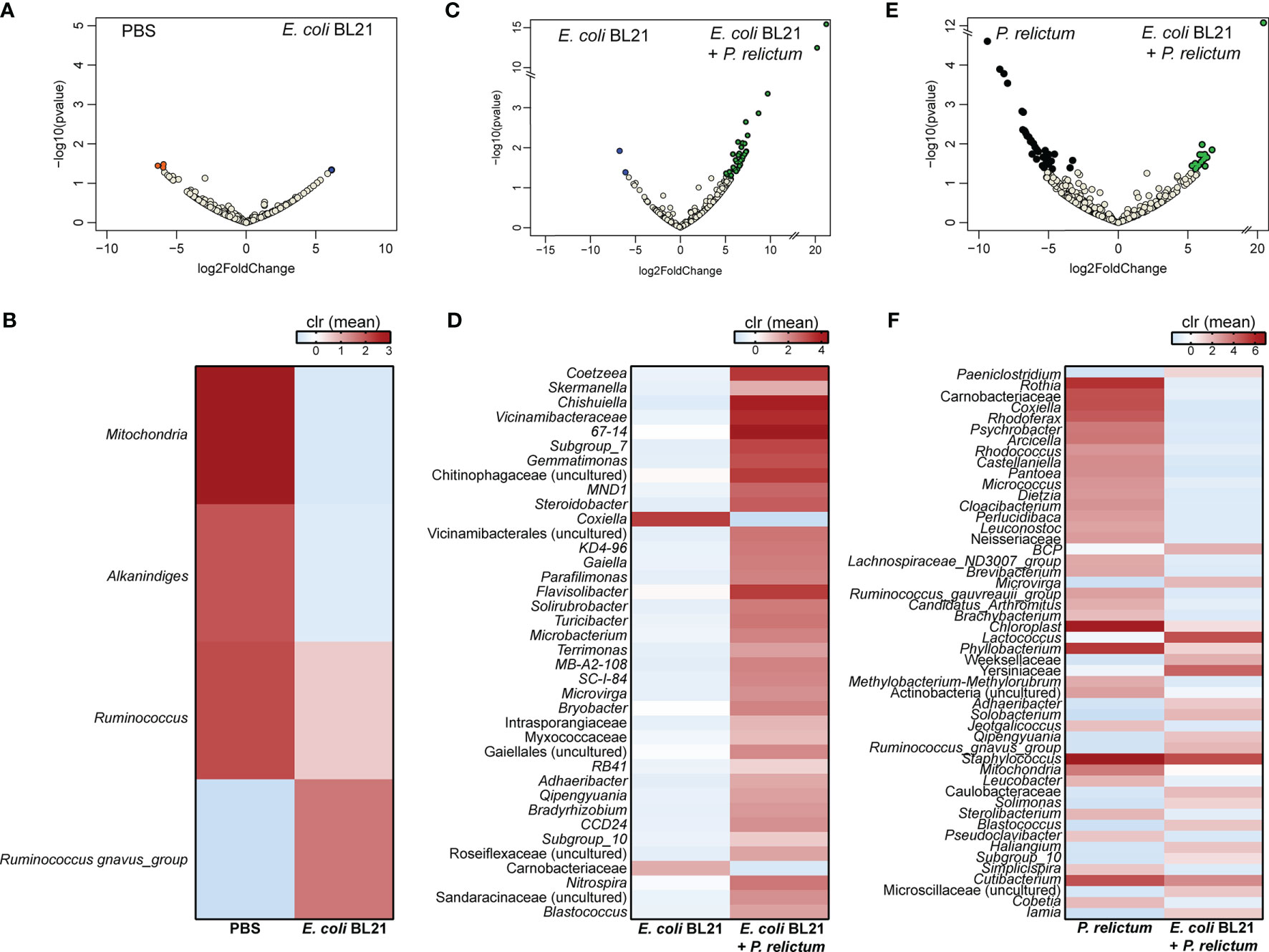
Figure 4 Impact of E. coli BL21 vaccine and P. relictum infection on the taxonomic profiles of mosquito microbiota. Volcano plot showing the differential bacterial abundance in microbiota of mosquitoes from different experimental groups (A) PBS vs. E. coli BL21, (C) E. coli BL21 vs. E. coli BL21+P. relictum, (E) P. relictum vs. E. coli BL21+P. relictum. Taxa with significant differences (Wald test, p < 0.05) between the groups are represented with colored dots (i.e., orange, blue, green and black). The gray dots represent taxa with no significant differences between groups. Heatmaps represent the relative abundance (expressed as clr) of taxa with significant differences in the comparisons (B) PBS vs. E. coli BL21, (D) E. coli BL21 vs. E. coli BL21+P. relictum, and (F) P. relictum vs. E. coli BL21+P. relictum. Taxa with significant differences in their abundance were identified using DeSeq2 algorithm. The taxonomic profiles were obtained from 16S rRNA sequences from midgut of mosquitoes fed on mock-immunized (n = 5 mosquitoes midgut pool), E. coli BL21-immunized (n = 7 mosquitoes midgut pool), E. coli BL21-immunized and challenged with P. relictum (n = 7 mosquitoes midgut pool) and challenged only with P. relictum (n = 8 mosquitoes midgut pool).
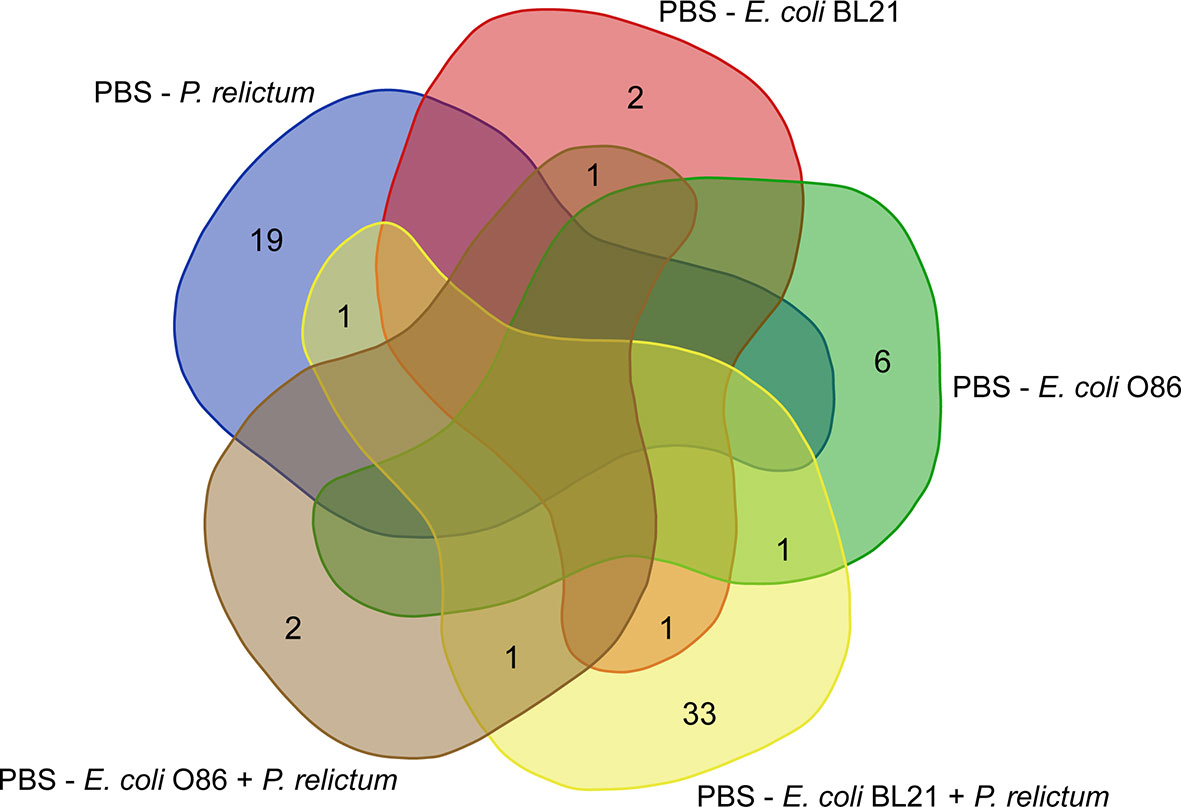
Figure 5 Comparison of unique and shared taxa across different experimental groups. Venn diagram showing the common and different taxa with significant changes in their abundance between vaccinated and infected groups, compared to the mock-vaccinated group (PBS).
To rule out a negative effect of anti-microbiota vaccine on host microbiota, we collected bird feces after mosquitoes infestation (d36) and at the end of the experiment (d52). We compared fecal microbiota of mock-immunized, E. coli BL21-immunized and E. coli O86:B7-immunized birds. The results showed non-significant differences in the microbial richness (Kruskal-Wallis, p > 0.05), as measured by Faith’s phylogenetic index (Supplementary Figure S1A). Similarly, species evenness did not differ between the different experimental groups (Kruskal-Wallis, p > 0.05, Supplementary Figure S1B). Regarding the beta diversity of the microbial communities in bird guts, Bray Curtis dissimilarity index did not show significant differences among mock-immunized, E. coli BL21-immunized and E. coli O86:B7-immunized birds (PERMANOVA, F = 1.35, p = 0.25).
Anti-Microbiota Vaccination Re-Structures the Microbial Communities in Midgut of Plasmodium-Infected Mosquitoes
Bacteria co-occurrence networks were used to further characterize the impact of Plasmodium infection and anti-microbiota vaccines on the mosquito microbiota. Visual inspection of the taxonomic networks revealed that anti-microbiota vaccines and malaria infection, together and separately, changes network topology, compared with the control mock vaccine group (Figure 6 and Table 1). Jaccard index was used to test for similarities (Jacc = 0, lowest similarity and Jacc = 1, highest similarity) in selected local network centrality measures (i.e., hub taxa, degree, betweenness centrality, closeness centrality, and eigenvector centrality) of the different networks. The observed Jaccard index for the betweenness centrality was higher than expected by random for the comparison between E. coli BL21 and E. coli O86:B7 networks (Jacc = 0,418, p = 0.01) (Supplementary Table S1). In addition, except for the Jaccard index of betweenness centrality in the PBS - P. relictum (Jacc = 0,432, p = 0.004), PBS - E. coli BL21 (Jacc = 0,512, p = 0.000002) and PBS - E. coli O86:B7 (Jacc = 0,522, p = 0. 000001) network comparisons, the observed Jaccard indexes of the other centrality measures were lower than expected by random in most networks (Supplementary Table S1). This suggests low similarity between compared networks. Topological differences, together with dissimilarity in local network centrality measures, indicate a major shift in the community structure induced by anti-microbiota vaccines and malaria infection.
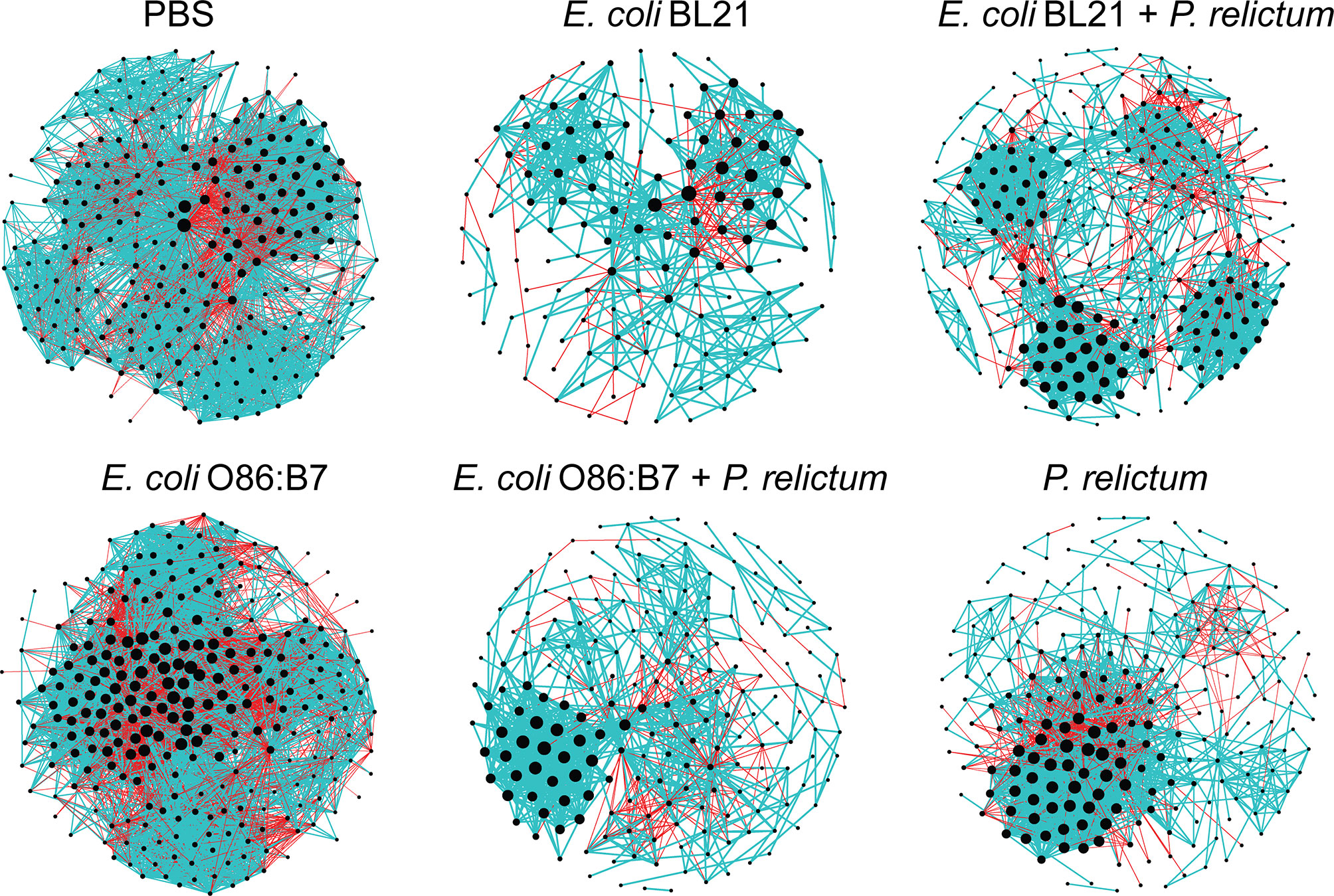
Figure 6 Co-occurrence networks of C. quinquefasciatus microbiota in the different experimental groups. Bacterial co-occurrence networks were inferred from the microbiota of mosquitoes fed on control (PBS), vaccinated and/or infected birds. Nodes represent bacterial taxa and connecting edges stand for a co-occurrence correlation (SparCC > 0.75). Node sizes are proportional to the eigenvector centrality value. Edges representing positive or negative correlations were colored in blue and red, respectively. Only nodes with at least one connection are displayed.
High variation of local connectedness of Escherichia-Shigella was observed in co-occurrence sub-networks of experimental groups (Figure 7). Specifically, the number of direct neighbors co-occurring with Escherichia-Shigella in the microbiota of mosquitoes fed on birds of the different experimental groups decreased in comparison to the sub-network of the microbiota of mosquitoes fed on mock-vaccinated birds. Although the number of edges directly connected to Escherichia-Shigella was similar in the sub-networks of the microbiota of mosquitoes fed on mock-immunized (47 co-occurring taxa) and E. coli O86:B7-immunized birds (45 co-occurring taxa), an increase of negative co-occurrence correlation was observed in the latter compared to the former group. A detailed analysis of the nodes connected to Escherichia-Shigella revealed the inexistence of shared taxa among the different sub-networks (Supplementary Figure S2). In addition, we used the eigenvector centrality metric to evaluate the “keystoneness” (importance of a node within the network) of Escherichia-Shigella in the different microbial networks. Eigenvector centrality value of Escherichia-Shigella in the sub-network of mosquitoes fed on E. coli BL21-immunized (eigenvector 0.03) and E. coli O86:B7-immunized birds (eigenvector 1) decreased and increased, respectively, compared to that in the control sub-network (i.e., mock vaccine group, eigenvector 0.29). Interestingly, eigenvector centrality values of Escherichia-Shigella decreased dramatically in the groups E. coli BL21+P. relictum (eigenvector 0.07), E. coli O86:B7+P. relictum (eigenvector 0), compared with the P. relictum infected mosquito group (eigenvector 0.16). Variable local interactions and “keystoneness” of Escherichia-Shigella between networks suggest that this taxon is affected by anti-microbiota vaccines and Plasmodium infection. More importantly, Escherichia-Shigella losses importance in the microbiota of mosquito exposed simultaneously to anti-E. coli Abs and P. relictum.
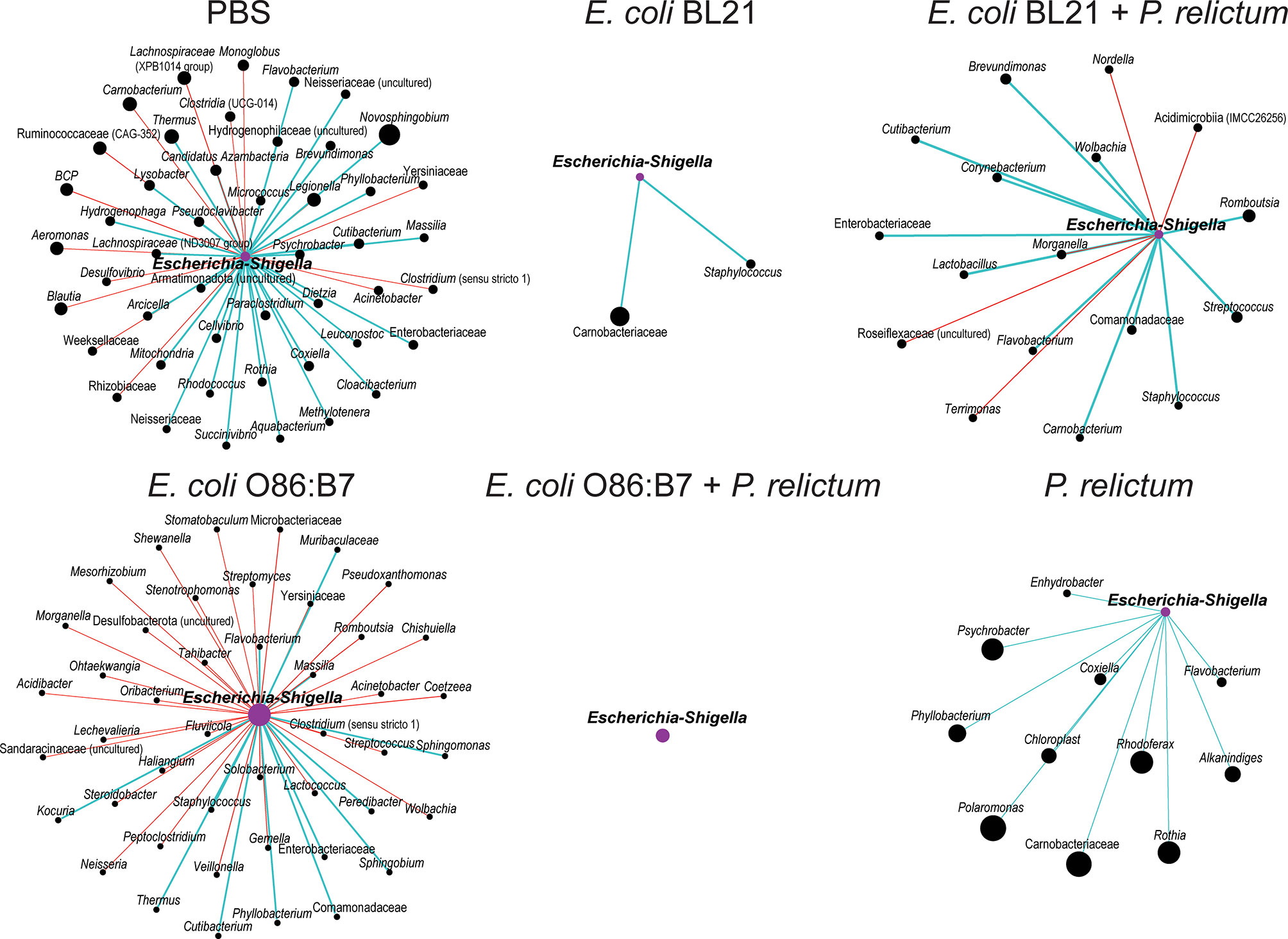
Figure 7 Local connectivity of Escherichia-Shigella in the co-occurrence networks of C. quinquefasciatus microbiota in the different experimental groups. The nodes/taxa linked to Escherichia-Shigella (purple node) were identified in the bacterial co-occurrence networks of mosquitoes fed on mock-immunized (PBS), E. coli-immunized and infected birds. Node sizes are proportional to the eigenvector centrality value. Only nodes with at least one connection are displayed. Connecting edges stand for a co-occurrence correlation (SparCC > 0.75), representing positive (blue edges) and negative (red edges) interactions between bacteria. BCP – Burkholderia Caballeronia Paraburkholderia.
Anti-Microbiota Vaccination Reduces Plasmodium Infection Within Mosquito Tissues
Mosquito survival was recorded and compared between groups. Results showed a significant difference in survival rate between the groups (Fisher’s exact test, p = 0.013). However, pairwise comparison of individual groups revealed significant differences only in the survival of mosquitoes fed on birds of the E. coli BL21 and E. coli BL21-P. relictum groups between them (p = 0.02) and with the others (Figure 8). Mosquitoes in the E. coli BL21 and E. coli BL21+P. relictum groups had the highest and lowest survival rates.
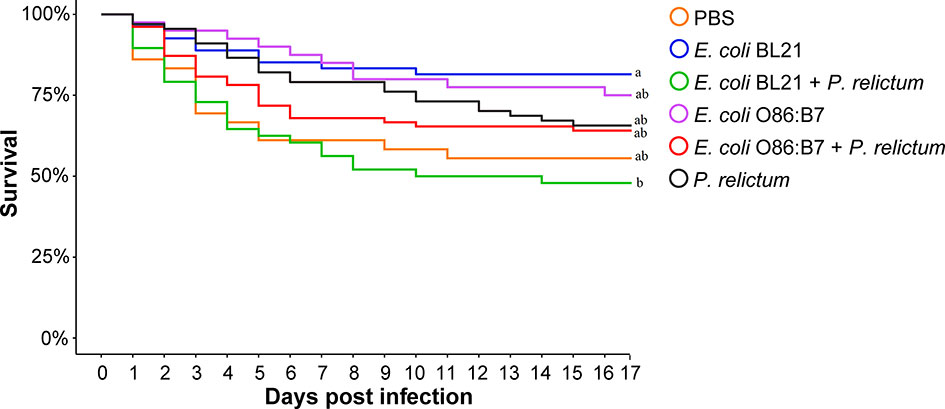
Figure 8 Survival of blood-fed mosquitoes throughout the experiment. The highest mortality was observed in the mosquito group fed on donors vaccinated with E. coli BL21 and infected with P. relictum, which significantly differed from the group fed on the birds vaccinated with E. coli BL21 (p = 0.02). Survival of mosquitoes between other groups did not differ significantly. Survival of mosquitoes was estimated by Fisher’s exact test with Bonferroni correction. Different letters at the end of the curves indicate statistically significant differences in survival rate (p < 0.05).
The occurrence (i.e., number of mosquitoes in which at least one oocyst was found) and number of P. relictum oocysts in midguts and occurrence of sporozoites in salivary glands of mosquitoes were measured. The results showed a significant overall difference in the occurrence of oocysts between the groups (Fisher’s exact test, p = 0.03). Pairwise comparisons revealed that the occurrence of Plasmodium oocysts was significantly lower in the midguts of mosquitoes fed on birds vaccinated with E. coli O86:B7 (p = 0.01), but not with E. coli BL21 (p > 0.05), compared with mosquitoes infected with P. relictum and not exposed to anti-E. coli Abs (Figure 9A). The number of oocysts was significantly lower in midguts of mosquitoes fed on birds vaccinated with both E. coli O86:B7 (p = 0.01) and E. coli BL21 (p = 0.001), compared with control mosquitoes (Figure 9B). We then hypothesized that decreased oocysts load in midguts might be associated with lower sporozoite infection in salivary glands. Results showed a significant difference between sporozoite occurrence between the groups (Fisher’s exact test, p < 0.001). The occurrence of sporozoites in salivary glands of mosquitoes fed on E. coli O86:B7-immunized, but not E. coli BL21-immunized (p > 0.05), birds was significantly lower (p = 0.001) than the control group (i.e., P. relictum infection alone) (Figure 9C).
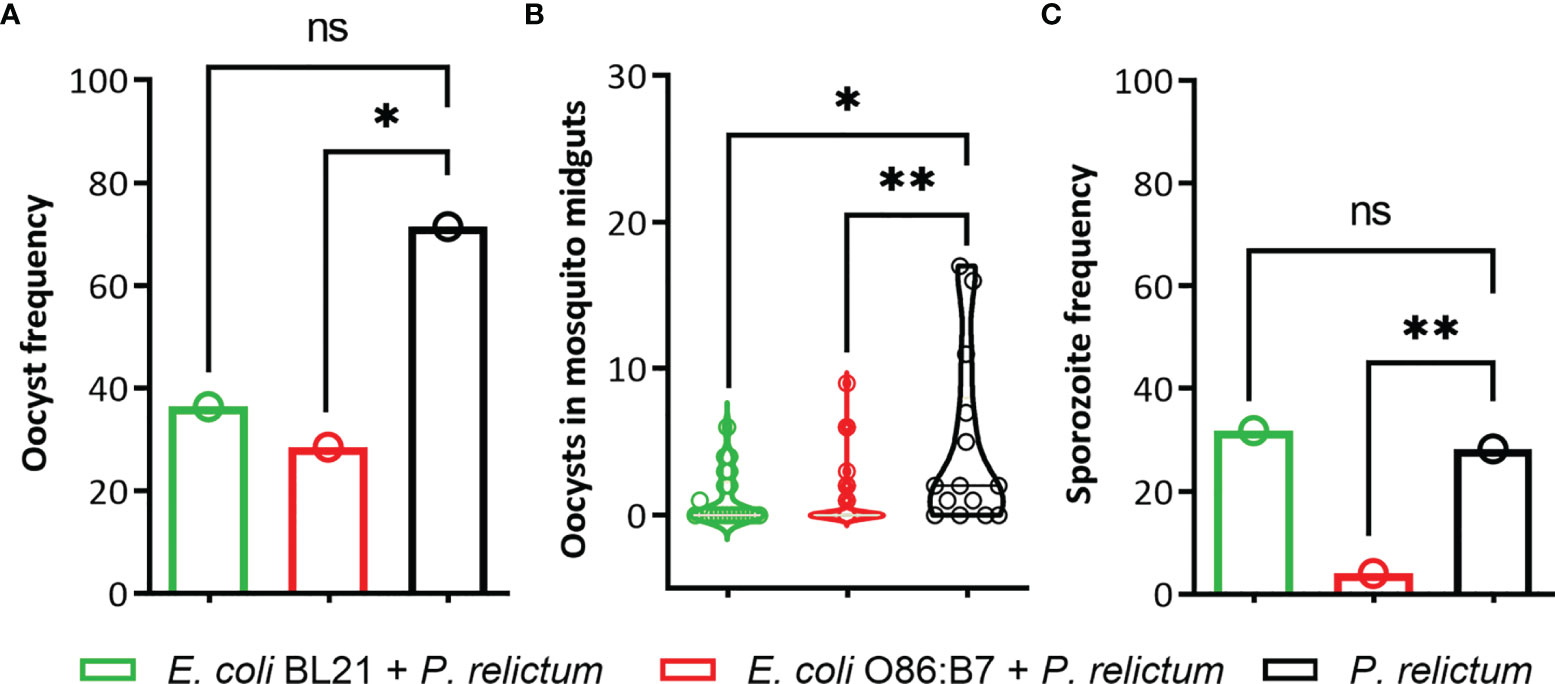
Figure 9 Impact of anti-microbiota vaccines on P. relictum development in infected mosquitoes. Three groups of P. relictum-infected birds were used as infection donors for mosquitoes. One of the groups was vaccinated with E. coli BL21, the other with E. coli O86:B7, and a third group was a control (only infection). (A) The percentage of mosquitoes in which at least one oocyst was found in the midgut by microscopy and compared between the groups was measured. (B) The number of oocysts in the midgut of P. relictum-infected mosquitoes was calculated and compared between the groups. (C) The percentage of mosquitoes in which sporozoites were detected in salivary glands by microscopy or PCR was measured and compared between the groups. The parameters were compared between groups by Fisher’s exact test with Bonferroni correction (oocyst and sporozoite frequency) and the Mann-Whitney U test (number of oocyst). (*p <0.05; **p <0.001; ns, not significant; n = 14-53 mosquitoes per group).
Discussion
In this study, we showed that anti-microbiota vaccination of birds with two strains of E. coli O86:B7 and BL21 modulate C. quinquefasciatus midgut microbiota. These results concur with a previous report in which live bacteria vaccine were used as a tool for the manipulation of tick microbiome (17, 18). During feeding, hematophagous ectoparasites ingest blood from the vertebrate host, along with host immune molecules. Host Abs and/or complement proteins have been detected in the guts of ticks (19–24), mosquitoes (25, 26), sandflies (27, 28), and tsetse flies (29). Once ingested, host immune components can remain active from a few hours to months depending on the species of blood-sucking arthropod, raising the possibility that vertebrate Abs could interact with pathogens and microbiota (16). Empirical work shows that host Abs can target vector-borne pathogens within ticks (30) and mosquitoes (31–33). Targeting pathogen proteins expressed within the arthropod vectors is the rationale behind transmission-blocking vaccines (32–34). Functional host Abs have also been shown to interact with symbionts in Rhodnius prolixus (14) and Glossina morsitans (35), as well as with bacterial microbiota in mosquitoes (15) and ticks (17, 18). Vaccination of mice with E. coli BL21 induced anti-E. coli Abs that when ingested with the blood decreased Enterobacteriaceae abundance within the tick microbiota (17). Targeting commensal Escherichia in ticks with anti-E. coli Abs reduced the connectivity of this taxon in the co-occurring networks (17). Similarly, in this study we observed reduced connectivity of Escherichia in the co-occurring networks of mosquitoes fed on birds vaccinated with E. coli BL21, but not in those vaccinated against E. coli O86:B7. However, we did observe a switch in the bacterial connectivity pattern of mosquitoes fed on E. coli O86:B7 compared to the control group PBS. In the control group, the majority of Escherichia correlations with its direct neighbor nodes were positive, while in the E. coli O86:B7 group most correlations were negative. These results show that antibody-mediated disturbance of the microbiome had cascading ecological impacts on the whole tick microbiome with strong impact on the structure of the microbial community of the vectors.
Recent research on vector-pathogen-microbiota interactions shows that microbial communities within vectors strongly influence pathogen colonization and transmission (36). Despite recent advances in vector microbiota research, the lack of tools for the precise and selective manipulation of the vector microbiome is currently a major limitation to disrupt pathogen-microbiome interactions in a taxon-specific manner (12, 37). Here we showed that mosquitoes fed on birds immunized with the Enterobacteriaceae bacteria E. coli O86:B7 and E. coli BL21 had reduced numbers of P. relictum oocysts in the midguts. The results support the potential role of commensal Enterobacteriaceae as a key player in the early development of Plasmodium within the vector. In agreement with this, high abundance of Enterobacteriaceae was previously associated with increase P. falciparum infection in A. gambiae midgut (13), and several studies showed that the ookinete–oocyst transition of Plasmodium spp. is strongly influenced by resident midgut microbiota in different mosquito species (38–41). However, the directionality (facilitation vs. competition) of midgut microbiota contribution to infection cannot be simplified to the abundance of a single taxon, while neglecting global changes of the microbial community as a whole. For example, experimental exposure of mosquitoes to a cocktail of penicillin and streptomycin (PS) reduced the proportion of microbiota-resident Enterobacteriaceae 92-fold, while simultaneous exposure to PS and Plasmodium infection increased the prevalence and intensity of P. berghei oocyst in A. gambiae midguts (42). This is not expected if Enterobacteriaceae facilitates Plasmodium infection (13). However, regardless that the impact of Enterobacteriaceae on parasite infectivity may differ between Plasmodium species (e.g., P. berghei vs. P. falciparum), the decreased of Enterobacteriaceae has been associated with a 19-fold increase of Asaia sp. in PS-exposed A. gambiae (42). Commensal Asaia bogorensis remodels glucose metabolism and increases midgut pH which in turn induces P. berghei gametogenesis and facilitates parasite infection of A. stephensi (10). Thus, the decrease of Enterobacteriaceae abundance may be balanced by the increase of another taxon (e.g., Asaia sp.) that also facilitates infection with a net effect of increased Plasmodium infectivity. Our results showed that anti-microbiota vaccines altered the Plasmodium-induced modulation of the mosquito microbiota, resulting in a global microbial community transformation and the reduction of Plasmodium infectivity.
We also found a significant reduction of sporozoites occurrence in the salivary glands of mosquitoes fed on E. coli O86:B7-immunized birds, but no in those fed on E. coli BL21-immunized birds. This could be explained by differences in the levels of the glycan Galα1-3Gal (α-Gal) in these two E. coli strains. The strain E. coli O86:B7 expresses high levels of α-Gal (43), which is not the case for E. coli BL21 (44, 45). Even when E. coli BL21 produces low levels of α-Gal, immunization with this bacterium induces anti-α-Gal (18), as low-abundant antigens could also induce immune responses. However, immunization with E. coli O86:B7 may induce anti-α-Gal Abs at levels even higher than E. coli BL21. This is relevant because as previously shown in ticks (18), α-1,3-galactosyltransferase genes, and possibly α-Gal, may be widely distributed in mosquito bacterial microbiota, increasing the number of bacterial taxa targeted by Abs induced by E. coli-vaccination. Another point to consider is that α-Gal has been detected on the surface of P. falciparum, P. berghei and Plasmodium yoelii sporozoites (43). Whether P. relictum sporozoites express α-Gal within C. quinquefasciatus salivary glands and whether anti-α-Gal Abs could target this Plasmodium stage within mosquito salivary glands remain to be tested.
Interestingly, mosquitoes fed on birds immunized with E. coli BL21 or E. coli O86:B7 had the highest survival rates, although the differences were significant only for mosquitoes in the E. coli BL21 group, compared with the P. relictum+E. coli BL21 group. Similar impact on survival was observed on mosquitoes fed on blood supplemented with PS (42). Exposure of A. gambiae to PS increased survival and fecundity, which are known to augment vectoral capacity (42). Our results can be interpreted as if microbiota modulation by E. coli BL21 vaccination: (i) increases C. quinquefasciatus fitness, and/or as if microbiota modulation by E. coli BL21 vaccination together with Plasmodium infection (ii) increases P. relictum virulence on mosquitoes and/or (iii) induces changes in the microbiome that impose a high cost on mosquito midguts homeostasis.
Conclusions
The vector microbiome can be assembled in different possible states, some of which may be incompatible with pathogen infection and/or transmission, while others increase vector competence or could increase or reduce vector fitness. Unraveling how to modulate these different states in a precise manner offers a powerful tool to develop novel transmission-blocking vaccines (16). Our results support the use of anti-microbiota vaccines to target vector commensal bacteria that facilitate pathogen infection (16, 46). In addition to taxon-specific effects, the community-level effects and cascading ecological impact of anti-microbiota vaccines on vector microbiota might induce infection-refractory states in the vector microbiome. Effective infection by vector-borne pathogens involves competent vectors, infective pathogens, and an infection-compatible microbiome (16). Mismatch of at least one of these components can result in an impaired ability of the vector to support the pathogen life cycle. For example, one strategy used to reduce the vector competence for pathogens is the genetic modification of insects that no longer transmit pathogens (47). Our results provide strong evidence that alterations in the vector midgut microbiomes, without the need to altering vector and/or pathogen genetics, affect pathogen infection in the vector. Therefore, deviations from infection-compatible microbiomes could block transmission and disease development. Anti-microbiota vaccines can be used as a microbiome manipulation tool for the induction of infection-refractory states in the vector microbiome for the control of major vector-borne pathogens such as malaria.
Material and Methods
Ethical Statement
All procedures were performed at the Nature Research Centre in Vilnius, Lithuania, according to Lithuanian and International Guiding Principles for Biomedical Research Involving Animals (2012). Infection experiments and other procedures were reviewed and approved by the Lithuanian State Food and Veterinary Service, Ref. No. 2020/07/24-G2-84. The assessment of the animal health and all described procedures were implemented by trained professionals (under licenses 2012/02/06-No-208, and 2016/01.29-No-344).
Birds and Housing Conditions
Seven-months-old domestic canaries were purchased commercially and kept for adaptation for one-month before experimental procedures. Birds were kept at the Nature Research Centre vivarium (License No. LT-61-13-003) under standard living conditions for birds. Experimental animals were housed in cages, up to three birds per cage. The facilities were under a controlled temperature of 21°C, which was maintained throughout the time that the experimentation lasted. Animals were supplied with a standard food for canaries and water ad libitum.
Experimental Design
Domestic canaries (Serinus canaria domestica) were used as a model for avian malaria infection, anti-microbiota vaccination and as donors of P. relictum to mosquitoes. Before the experimental procedures began, birds were randomly separated into six groups as displayed in Figure 1. Birds in one group received a mock vaccination containing PBS and adjuvant. Another group of birds was only infected with P. relictum. All birds in the other four groups received anti-microbiota vaccines and in two of these groups, birds were additionally infected with P. relictum. After Plasmodium infection and antibody titers to anti-microbiota vaccines were confirmed, C. quinquefasciatus mosquito females were fed on the birds for pathogen acquisition experiment. In total, 22 experimental birds from all groups were exposed to mosquito bites as described in the sections below. Engorged mosquitoes (n = 663) were used for midgut and/or salivary glands preparations. Mosquito tissues were used for oocyst (midguts) and sporoites (salivary glands) counting. Mosquito midguts were also used for DNA extraction and microbiota analysis using bacterial 16S rRNA amplicon sequencing. Bird feces were collected to test for an impact of anti-microbiota vaccines on bird gut microbiota. Experimental procedures are described below.
Bacterial Cultures and Live Bacteria Immunization
Representative bacteria of the genus Escherichia-Shigella were selected to be included in live bacteria vaccine formulations as previously reported (17, 18). Anti-microbiota vaccinations were used to test the impact of host immune response against targeted bacteria on mosquito microbiota composition and structure, mosquito survival and Plasmodium infection. The Escherichia coli strains BL21 (DE3, Invitrogen, Carlsbad, CA, USA), and O86:B7 (ATCC® 12701TM) were selected. The two bacterial strains were prepared as previously described (17, 18). Briefly, E. coli was grown on Luria Broth (LB, Sigma-Aldrich, St. Louis, MO, USA) at 37°C under vigorous agitation, washed with phosphate buffer saline (PBS) 10 mM NaH2PO4, 2.68 mM KCl, 140 mM NaCl, pH 7.2 (Thermo Scientific, Waltham, MA, USA), resuspended at 3.6 × 104 colony-forming unit (CFU)/mL, and homogenized using a glass homogenizer. Eight-month-old, canaries were immunized subcutaneously with Escherichia sp. in 50 µL (4, 1 × 106 CFU per bird) of a water-in-oil emulsion containing 70% (w/w) Montanide™ ISA 71 VG adjuvant (Seppic, Paris, France), with a booster dose two weeks after the first dose. Control birds received a mock vaccine containing PBS and adjuvant. All reagents used for bacterial preparation were apyrogenic.
Bacterial Protein Extraction
Escherichia coli strains were washed twice with PBS, centrifuged at 1,000× g for 5 min at 4°C, resuspended in 1% Trion-PBS lysis buffer (Sigma-Aldrich, St. Louis, MO, USA) and homogenized with 20 strokes using a glass balls homogenizer. The homogenate was then centrifuged at 300× g for 5 min at 4°C and the supernatant was collected. Protein concentration was determined using the Bradford Protein Assay (Thermo Scientific, San Jose, CA, USA) with Bovine Serum Albumin (BSA) as standard. Bacterial protein extracts were used in the indirect ELISA to measure anti-E. coli Abs in bird sera.
Thawing the Cryopreserved Avian Malaria Sample
The cryopreserved P. relictum strain SGS1 was thawed and used to infect donor canaries. Tubes containing Plasmodium-infected avian blood, conserved frozen in liquid nitrogen, were thawed as described by Dimitrov et al. (48). Briefly, thawed samples were mixed with 12% NaCl (1/3 of thawed sample amount). After 5 min equilibration, one volume of 1.6% of NaCl was added followed by centrifugation at 10,000 rpm for 5 min. After centrifugation, the supernatant was removed and 1.6% NaCl (1/3 of original sample) was added and centrifuged again. The same procedure was repeated three times with 0.9% NaCl solution. The final mixture was diluted with 0.9% NaCl and sub-inoculated into two canaries.
Experimental Infection of Birds
All birds from the mock-immunized group and birds randomly selected (n = 4) from E. coli BL21-immunized and E. coli O86:B7-immunized groups were experimentally infected with P. relictum (SGS1) using the protocol described by Palinauskas et al. (49). Each experimental bird was sub-inoculated with a mixture (0.10 mL) of infected blood, 3.7% sodium citrate and 0.9% saline in proportion 4:1:5 into the pectoral muscles. Each bird received approximately 1 x 105 of mature P. relictum meronts. The duration of experimental time before exposure to the mosquitoes was 13 days post inoculation (dpi). This time was sufficient to develop higher parasitemia in the blood for malaria parasites. Birds with suitable infection levels were exposed to feed mosquitoes. Parasitemia was examined every 4 days by taking blood from the brachial vein as described in the section below.
Maintenance of Mosquitoes
For experimental infection of P. relictum in mosquitoes, we used the P. B. Šivickis parasitology laboratory-reared C. quinquefasciatus mosquitoes. The colony was maintained as described in Žiegytė et al. (50). Mosquitoes were kept in a nylon netted cage (65×65×65 cm) under controlled conditions (room temperature 23 ± 1°C; humidity 75-80%; photoperiod 17:7 light:dark). Adult insects were provided with cotton wools saturated with 5% saccharose solution. Mosquito females were randomly separated from the main colony into smaller cages (about 300 mosquitoes) for each experimental group. For experimental infection we used insects of the same age, approximately one week after hatching.
Mosquito Exposure to Vaccinated Birds and Infection With Plasmodium
We evaluated gametocytemia in all donor birds immediately after mosquito exposure. The gametocytemia of P. relictum-infected donor birds varied between 0.005% – 1.5%, E. coli BL21-immunized birds – 0.02% – 2% and E. coli O86:B7-immunized birds – 0.01% – 3.5%. For experimental exposure to mosquito bites, the donor bird was carefully immobilized and fixed in a paper tube, leaving only the legs exposed for the mosquitoes (51). The tube was placed into a separate mosquito cage with separated female mosquitoes taken from the main colony. The bird was kept up to one hour, or when approximately 40 fully saturated mosquitoes were counted. Engorged insects were separated into small cages (17.4 × 17.5 × 17.5 cm) and kept there up to 17 days post exposure (dpe) under the same rearing conditions as described above. All cages were additionally provided with cups containing water for oviposition. Mosquitoes were exposed to donor birds immunized with E. coli BL21, E. coli O86:B7 and mock vaccine 28 days post first vaccination (dpvI). Plasmodium-infected donor birds were used for mosquito infestation 28 dpvI (i.e., 14 dpi). Exposed mosquitoes were dissected gradually for preparations of different sporogonic stages and sampling for microbiota analysis.
Blood Sample and Bird Feces Collection
Blood samples were taken from birds by puncturing brachial vein using microcapillaries. A small drop of blood was used to make smears for microscopy to estimate the development of parasites in the blood. Smears were air-dried, fixed with absolute methanol and stained as described by Valkiūnas et al. (52). A fraction of blood (20-30 μL) was placed in SET-buffer for molecular analysis (PCR, see below) to confirm the lineage in recipient birds. The rest of the blood (100 μL) was used to obtain serum for immunological analysis. Before centrifugation the blood was incubated for 2 h at room temperature, allowing it to coagulate. Then samples were centrifuged at 5,000x g for 5 min and serum separated in microtube and kept in a freezer at -15°C until processing. The blood for smears and SET-buffer was collected on days 0, 4, 8, 12, after inoculation of parasites. The blood for serum was taken on days 0 and 36 after the first vaccine inoculation. Fresh feces were collected from each bird in sterile tubes on days 36 and 52 and were stored at -20°C before genomic DNA extraction.
Microscopic Examination
We used an Olympus BX61 light microscope (Olympus, Japan) to examine blood smears and preparations of mosquito tissues. Parasitemia was calculated as a percentage by actual counting of the number of parasites per 10,000 erythrocytes as described by Godfrey et al. (53). The infection intensity in the mosquito was evaluated by counting the oocysts in the midgut. Successful sporogony was determined by examining salivary glands preparations and confirming sporozoite development.
Indirect ELISA
The levels of Abs reactive to bacterial proteins were measured in bird sera as previously reported (17, 18), with small modifications. The 96-well ELISA plates (Thermo Scientific, Waltham, MA, USA) were coated with 50 ng/mL (100 µL/well) of E. coli BL21 protein extracts in carbonate/bicarbonate buffer (0.05 M, pH 9.6) and incubated for 2 h with 100 rpm shaking at RT. Subsequently, plates were incubated overnight at 4°C. Wells were washed three times with 100 µL of PBS containing 0.05% (vol/vol) Tween 20 (PBST), and then blocked by adding 100 µL of 1% Human Serum Albumin (HSA)/PBS for 1 h at RT and 100 rpm shaking. After three washes, sera samples, diluted at 1:200 in 0.5% HSA/PBS, were added to the wells and incubated for 1 h at 37°C and 100 rpm shaking. The plates were washed three times and HRP-conjugated Abs (goat anti-turkey IgG) (MyBioSource, San Diego, CA, USA) were added at 1:1,000 dilution in 0.5% HSA/PBST (100 µL/well) and incubated for 1 h at RT with shaking. The plates were washed three times and the reaction was developed with 100 µL ready-to-use TMB solution (Promega, Madison, WI, USA) at RT for 20 min in the dark, and then stopped with 50 µL of 4% H2SO4. Optimal antigen concentration and dilutions of sera and conjugate were defined using titration assays. The optical density (OD) was measured at 450 nm using an ELISA plate reader (Filter-Max F5, Molecular Devices, San Jose, CA, USA). All samples were tested in triplicate and the average value of three blanks (no Abs) was subtracted from the reads. The cut-off was determined as two times the mean OD value of the blank controls.
Evaluation of Mosquito Survival and Collection of Midguts and Salivary Glands for Testing P. relictum Development and Midgut Microbiota Analyses
The survival of mosquitoes was estimated by daily checking the mosquito cages at 10 am and counting dead insects until 17 dpe. On 10 dpe, mosquito midguts were dissected for estimation of developed oocysts and microbiota analysis. Before dissection, mosquitoes were euthanized by shaking vigorously to stun them in insect aspirator. Wings and legs of the insects were removed before dissection, which was performed under the binocular stereoscopic microscope. Each mosquito was carefully separated in two segments, the thorax with head and abdomen. The abdomen was placed in the drop of saline and the midgut of the mosquito was extracted. The midgut was stained according to Kazlauskienė et al. (51) for counting oocysts of Plasmodium parasite. For microbiota analysis, unstained midguts were pooled up to 10 in sterile microtubes and frozen at -20C. To eliminate contamination of samples, new dissecting needles were used for each dissected insect. On 17 dpe, each mosquito was carefully separated in two segments, the thorax with head and abdomen. Salivary glands were extracted from the thorax, placed in a separate sterile drop of saline and grinded to make a smear to record the presence of sporozoites (51). The remnants of salivary gland preparations and thorax were fixed in SET-buffer for PCR analysis. The abdomen, the same as on day 10, was placed in a drop of saline to prepare the samples for the midgut microbiota analysis.
DNA Extraction and PCR for P. relictum Identification
Total DNA for PCR analysis was extracted from the blood remnants of mosquitoes using an ammonium acetate extraction protocol by Sambrook & Russel (54). A nested PCR protocol described by Hellgren et al. (55) was used to confirm P. relictum infection in the donor birds and test mosquitoes for positive parasite sporozoite development. For the first PCR we used the primers HaemNFI [5’-CATATATTAAGAGAAITATGGAG-3’] and HaemNR3 [5’-ATAGAAAGATAAGAAATACCATTC-3’] (55). In the second PCR a mitochondrial cyt b gene (478 bp) was amplified using the primers HaemF [5’-ATGGTGCTTTCGATATATGCATG-3’] and HaemR2 [5’-GCATTATCTGGATGTGATAATGGT-3’] (56). For PCR mix we used 12.5 μl of DreamTaq Master Mix (Thermo Fisher Scientific, Lithuania), 8.5 μl of nuclease-free water, 1 μl of each primer and 2 μl of template DNA (extracted DNA or products of first PCR). P. relictum-positive samples were determined by running 2 μl of second PCR product on 2% agarose gel. For parasite lineage confirmation, samples containing parasite DNA were sequenced from the 5` end using the HAEMF primer on an ABI PRISM TM 3100 capillary sequencing robot (Applied Biosystems, USA) as described by Bensch et al. (56). The BLAST search tool (National Centre for Biotechnology Information website: http://www.ncbi.nlm.nih.gov/BLAST) was used to determine SGS1 lineage.
DNA Extraction and 16S rRNA Sequencing for Microbiota Analysis
Genomic DNA for microbiota analysis was extracted from frozen midguts of engorged mosquitoes and from fecal samples of birds using a Pure Link Microbiome DNA Purification Kit (Invitrogen, Thermo Fisher Scientific, CA, USA). Each DNA sample was eluted in 100 µl of elution buffer. Genomic DNA quality (OD260/280 between 1.8 –2.0) was measured with NanoDrop™ One (Thermo Scientific, Waltham, MA, USA). More than 850ng of DNA at ≥ 8.5 ng/μL concentration were sent for amplicon sequencing of the bacterial 16S rRNA gene, which was commissioned to Novogene Bioinformatics Technology Co. (London, UK). Libraries were prepared with NEBNext® Ultra™ IIDNA Library Prep Kit (New England Biolabs, MA, USA). A single lane of Illumina MiSeq system was used to generate 251-base paired-end reads from the V4 variable region of the 16S rRNA gene using barcoded universal primers (515F/806R) in samples from mosquitoes engorged on E. coli BL21-immunized (n = 7 midgut pools), E. coli BL21-immunized and Plasmodium-infected (n = 7), E. coli O86:B7-immunized (n = 5), E. coli O86:B7-immunized and Plasmodium-infected (n = 8), Plasmodium-infected (n = 8) or mock-immunized (n = 5) birds. The raw 16S rRNA sequences obtained from mosquito samples were deposited in the SRA repository, Bioproject No. PRJNA778616. One extraction reagent control was set in which the different DNA extraction and amplification steps were performed using the same conditions as for the samples but using water as template.
16S rRNA Sequences Processing
The analysis of 16S rRNA sequences was performed using QIIME 2 pipeline (v. 2021.4) (57). The sequences in the fastq files were denoised and merged using the DADA2 software (58) as implemented in QIIME 2. The amplicon sequence variants (ASVs) were aligned with q2-alignment of MAFFT (59) and used to construct a phylogeny (60). Taxonomy was assigned to ASVs using a classify-sklearn naïve Bayes taxonomic classifier (61) based on SILVA database (release 132) (62). The taxonomic data tables were collapsed at genus level and filtered excluding taxa with less than 10 total reads and present in less than 30% of samples of each dataset.
Bacterial Co-Occurrence Networks
Co-occurrence networks were inferred for each experimental condition based on taxonomic profiles. Correlation matrices were calculated using the Sparse Correlations for Compositional data (SparCC) method (63), implemented in the R Studio (64). Network visualization and calculation of topological features and taxa connectedness (i.e., the number of nodes and edges, network diameter, average degree, weighted degree, average path length, modularity, number of modules, average clustering coefficient) was performed using the software Gephi 0.9.2 (65).
Statistical Analysis
Statistical analysis was performed using R program (version 4.0.4) (66). Differences in oocyst and sporozoite frequency between the groups of infected mosquitoes were compared using Fisher’s exact test with Bonferroni comparison tests. The numbers of oocysts formed in mosquito midguts were compared between infected groups by the Mann-Whitney U test. Differences in relative Ab levels (i.e., OD) among groups of immunized birds in the different time points were compared using two-way ANOVA with Bonferroni multiple comparison tests applied for individual comparisons. Microbial diversity analyses were carried out on rarefied ASV tables, calculated using the q2-diversity plugins. The alpha diversity (richness and evenness) was explored using Faith’s phylogenetic alpha diversity index (67) and Pielou’s evenness index (68). Differences in α-diversity metric between groups were assessed using Kruskal-Wallis test (alpha= 0.05). Bacterial β-diversity was assessed using the Bray Curtis dissimilarity (69) and compared between groups using the PERMANOVA test. Betadisper function was used for the construction of PCoA plot, and an ANOVA test was used to compare the dispersion of the samples by groups. The differential features were detected by comparing the log2 fold change (LFC) using the Wald test as implemented in the compositional data analysis method DESeq2 (70). The number of shared co-occurring taxa among different experimental groups was done in R studio using the package “Venn”.
Differential Network Analysis
Comparison of the similarity of the most central nodes between two networks was done with the package “NetCoMi” (71) in R studio using the read count taxonomic tables. “Most central” nodes are defined as those nodes with a centrality value above the empirical 75% quartile. The comparison returns Jaccard’s indexes for each of four local measures (i.e., degree, betweenness centrality, closeness centrality, eigenvector centrality) of the sets of most central nodes as well as for the sets of hub taxa between the two networks compared. Thus, the Jaccard’s index express the similarity of the sets of most central nodes as well as the sets of hub taxa between the two networks. Jaccard index of 0 indicates completely different sets while a value of 1 indicates equal sets of most central nodes or hub taxa between the compared networks. The two p-values P(J ≤ j) and P(J ≥ j) for each Jaccard’s index are the probability that the observed value of Jaccard’s index is ‘less than or equal’ or ‘higher than or equal’, respectively, to the Jaccard value expected at random, which is calculated taking into account the present total number of taxa in both sets [based on Real and Vargas (72)].
Data Availability Statement
The datasets presented in this study can be found in online repositories. The names of the repository/repositories and accession number(s) can be found below: https://www.ncbi.nlm.nih.gov/sra, PRJNA778616.
Ethics Statement
All procedures were performed at the Nature Research Centre in Vilnius, Lithuania, according to Lithuanian and International Guiding Principles for Biomedical Research Involving Animals (2012). Infection experiments and other procedures were reviewed and approved by the Lithuanian State Food and Veterinary Service, Ref. No. 2020/07/24-G2-84. The assessment of the animal health and all described procedures were implemented by trained professionals (under licenses 2012/02/06-No-208, and 2016/01.29-No-344).
Author Contributions
AC-C and VP conceived the study. JA, RŽ, JM, EP, LM-H, and VP performed the experiments and acquired the data. JA, AW-C, and DO analyzed the data. JA, AW-C, and AC-C prepared the figures. AC-C, VP, and JM contributed reagents and other resources. AC-C, VP, and DO supervised the work. JA, AW-C, AC-C, and VP drafted the first version of the manuscript. All authors contributed to the article and approved the submitted version.
Funding
This research was funded by the French Government’s Investissement d’Avenir program, Laboratoire d’Excellence “Integrative Biology of Emerging Infectious Diseases” (grant no. ANR-10-LABX-62-IBEID). Funding for VP from European Social Fund (project No 09.3.3-LMT-K-712-01-0016) under grant agreement with the Research Council of Lithuania (LMTLT). AW-C was supported by Programa Nacional de Becas de Postgrado en el Exterior “Don Carlos Antonio López” (Grant No. 205/2018).
Conflict of Interest
The authors declare that the research was conducted in the absence of any commercial or financial relationships that could be construed as a potential conflict of interest.
Publisher’s Note
All claims expressed in this article are solely those of the authors and do not necessarily represent those of their affiliated organizations, or those of the publisher, the editors and the reviewers. Any product that may be evaluated in this article, or claim that may be made by its manufacturer, is not guaranteed or endorsed by the publisher.
Supplementary Material
The Supplementary Material for this article can be found online at: https://www.frontiersin.org/articles/10.3389/fimmu.2022.841835/full#supplementary-material.
Supplementary Figure S1 | Impact of anti-microbiota vaccines on fecal microbiota of birds. Comparison of ASV richness and evenness, measured with (A) Faith’s phylogenetic diversity index and (B) Pielou’s evenness index, respectively, among the microbiota of mock-immunized, E. coli BL21-immunized and E. coli O86:B7-immunized birds.
Supplementary Figure S2 | Number of shared co-occurring taxa of Escherichia-Shigella among the sub-networks of different experimental group. Venn diagram showing the number of bacteria that are common or unique among the taxa that co-occur directly to Escherichia-Shigella in the different experimental group.
Supplementary Table S1 | Jaccard indexes of local centrality measures. Jaccard’s indexes for each of local centrality measures (i.e., degree, betweenness centrality, closeness centrality, eigenvector centrality and hub taxa) of the sets of most central nodes for pairwise network comparisons. The two p-values, P(J ≤ j) and P(J ≥ j), for each Jaccard’s index were added.
References
1. Lee H, Halverson S, Ezinwa N. Mosquito-Borne Diseases. Prim Care (2018) 45(3):393–407. doi: 10.1016/j.pop.2018.05.001
2. Valkiūnas G, Iezhova TA. Keys to the Avian Malaria Parasites. Malar J (2018) 17:212. doi: 10.1186/s12936-018-2359-5
3. Valkiunas G. Avian Malaria Parasites and Other Haemosporidia. 1st. Boca Raton, FL: CRC Press (2004).
4. Martínez-de la Puente J, Santiago-Alarcon D, Palinauskas V, Bensch S. Plasmodium Relictum. Trends Parasitol (2021) 37(4):355–6. doi: 10.1016/j.pt.2020.06.004
5. Santiago-Alarcon D, Palinauskas V, Schaefer HM. Diptera Vectors of Avian Haemosporidian Parasites: Untangling Parasite Life Cycles and Their Taxonomy. Biol Rev Camb Philos Soc (2012) 87(4):928–64. doi: 10.1111/j.1469-185X.2012.00234.x
6. Blandin SA, Wang-Sattler R, Lamacchia M, Gagneur J, Lycett G, Ning Y, et al. Dissecting the Genetic Basis of Resistance to Malaria Parasites in Anopheles Gambiae. Science (2009) 326:147–50. doi: 10.1126/science.1175241
7. Collins FH, Sakai RK, Vernick KD, Paskewitz S, Seeley DC, Miller LH, et al. Genetic Selection of a Plasmodium-Refractory Strain of the Malaria Vector Anopheles Gambiae. Science (1986) 234:607–10. doi: 10.1126/science.3532325
8. Vernick KD, Oduol F, Lazzaro BP, Glazebrook J, Xu J, Riehle M, et al. Molecular Genetics of Mosquito Resistance to Malaria Parasites. Curr Top Microbiol Immunol (2005) 295:383–415. doi: 10.1007/3-540-29088-5_15
9. Hajkazemian M, Bossé C, Mozūraitis R, Emami SN. Battleground Midgut: The Cost to the Mosquito for Hosting the Malaria Parasite. Biol Cell (2021) 113(2):79–94. doi: 10.1111/boc.202000039
10. Wang M, An Y, Gao L, Dong S, Zhou X, Feng Y, et al. Glucose-Mediated Proliferation of a Gut Commensal Bacterium Promotes Plasmodium Infection by Increasing Mosquito Midgut Ph. Cell Rep (2021) 35(3):108992. doi: 10.1016/j.celrep.2021.108992
11. Bando H, Okado K, Guelbeogo W, Badolo A, Aonuma H, Nelson B, et al. Intra-Specific Diversity of Serratia Marcescens in Anopheles Mosquito Midgut Defines Plasmodium Transmission Capacity. Sci Rep (2013) 3:1641. doi: 10.1038/srep01641
12. Shaw WR, Catteruccia F. Vector Biology Meets Disease Control: Using Basic Research to Fight Vector-Borne Diseases. Nat Microbiol (2019) 4:20–34. doi: 10.1038/s41564-018-0214-7
13. Boissière A, Tchioffo MT, Bachar D, Abate L, Marie A, Nsango SE, et al. Midgut Microbiota of the Malaria Mosquito Vector Anopheles Gambiae and Interactions With Plasmodium Falciparum Infection. PloS Pathog (2012) 8(5):e1002742. doi: 10.1371/journal.ppat.1002742
14. Ben-Yakir D. Growth Retardation of Rhodnius Prolixus Symbionts by Immunizing Host Against Nocardia (Rhodococcus) Rhodnii. J Insect Physiol (1987) 33:379–83. doi: 10.1016/0022-1910(87)90015-1
15. Noden BH, Vaughan JA, Pumpuni CB, Beier JC. Mosquito Ingestion of Antibodies Against Mosquito Midgut Microbiota Improves Conversion of Ookinetes to Oocysts for Plasmodium Falciparum, But Not P. yoelii Parasitol Int (2011) 60(4):440–6. doi: 10.1016/j.parint.2011.07.007
16. Maitre A, Wu-Chuang A, Aželytė J, Palinauskas V, Mateos-Hernández L, Obregon D, et al. Vector Microbiota Manipulation by Host Antibodies: The Forgotten Strategy to Develop Transmission-Blocking Vaccines. Parasit Vectors (2021) 15:4. doi: 10.1186/s13071-021-05122-5
17. Mateos-Hernández L, Obregón D, Wu-Chuang A, Maye J, Bornères J, Versille N, et al. Anti-Microbiota Vaccines Modulate the Tick Microbiome in a Taxon-Specific Manner. Front Immunol (2021) 12:704621. doi: 10.3389/fimmu.2021.704621
18. Mateos-Hernández L, Obregón D, Maye J, Borneres J, Versille N, de la Fuente J, et al. Anti-Tick Microbiota Vaccine Impacts Ixodes Ricinus Performance During Feeding. Vaccines (Basel) (2020) 8(4):702. doi: 10.3390/vaccines8040702
19. Ackerman S, Clare FB, McGill TW, Sonenshine DE. Passage of Host Serum Components, Including Antibody, Across the Digestive Tract of Dermacentor Variabilis (Say). J Parasitol (1981) 67(5):737–40. doi: 10.2307/3280459
20. Ben-Yakir D, Fox CJ, Homer JT, Barker RW. Quantification of Host Immunoglobulin in the Hemolymph of Ticks. J Parasitol (1987) 73(3):669–71. doi: 10.2307/3282157
21. Wang H, Nuttall PA. Excretion of Host Immunoglobulin in Tick Saliva and Detection of IgG-Binding Proteins in Tick Haemolymph and Salivary Glands Parasitology. Parasitology (1994) 109(4):525–30. doi: 10.1017/s0031182000080781
22. Willadsen P. Novel Vaccines for Ectoparasites. Vet Parasitol (1997) 71(2-3):209–22. doi: 10.1016/s0304-4017(97)00028-9
23. Rathinavelu S, Broadwater A, de Silva AM. Does Host Complement Kill Borrelia Burgdorferi Within Ticks? Infect Immun (2003) 71(2):822–9. doi: 10.1128/IAI.71.2.822-829.2003
24. Galay RL, Matsuo T, Hernandez EP, Talactac MR, Kusakisako K, Umemiya-Shirafuji R, et al. Immunofluorescent Detection in the Ovary of Host Antibodies Against a Secretory Ferritin Injected Into Female Haemaphysalis Longicornis Ticks. Parasitol Int (2018) 67(2):119–22. doi: 10.1016/j.parint.2017.10.006
25. Hatfield PR. Detection and Localization of Antibody Ingested With a Mosquito Bloodmeal. Med Vet Entomol (1988) 2(4):339–45. doi: 10.1111/j.1365-2915.1988.tb00206.x
26. Lackie AM, Gavin S. Uptake and Persistence of Ingested Antibody in the Mosquito Anopheles Stephensi. Med Vet Entomol (1989) 3(3):225–30. doi: 10.1111/j.1365-2915.1989.tb00220.x
27. Tesh RB, Chen WR, Catuccio D. Survival of Albumin, IgG, IgM, and Complement (C3) in Human Blood After Ingestion by Aedes Albopictus and Phlebotomus Papatasi. Am J Trop Med Hyg (1988) 39(1):127–30. doi: 10.4269/ajtmh.1988.39.127
28. Saab NAA, Nascimento AAS, Queiroz DC, da Cunha IGM, Pereira AA, D'Ávila Pessoa GC, et al. How Lutzomyia Longipalpis Deals With the Complement System Present in the Ingested Blood: The Role of Soluble Inhibitors and the Adsorption of Factor H by Midgut. J Insect Physiol (2020) 120:103992. doi: 10.1016/j.jinsphys.2019.103992
29. Nogge G, Giannetti M. Specific Antibodies: A Potential Insecticide. Science (1980) 209(4460):1028–9. doi: 10.1126/science.7403865
30. Kumar M, Kaur S, Kariu T, Yang X, Bossis I, Anderson JF, et al. Borrelia Burgdorferi BBA52 is a Potential Target for Transmission Blocking Lyme Disease Vaccine. Vaccine (2011) 29(48):9012–9. doi: 10.1016/j.vaccine.2011.09.035
31. Tachibana M, Wu Y, Iriko H, Muratova O, MacDonald NJ, Sattabongkot J, et al. N-Terminal Prodomain of Pfs230 Synthesized Using a Cell-Free System is Sufficient to Induce Complement-Dependent Malaria Transmission-Blocking Activity. Clin Vaccine Immunol (2011) 18(8):1343–50. doi: 10.1128/CVI.05104-11
32. Chowdhury DR, Angov E, Kariuki T, Kumar N. A Potent Malaria Transmission Blocking Vaccine Based on Codon Harmonized Full Length Pfs48/45 Expressed in Escherichia Coli. PloS One (2009) 4(7):e6352. doi: 10.1371/journal.pone.0006352
33. Kapulu MC, Da DF, Miura K, Li Y, Blagborough AM, Churcher TS, et al. Comparative Assessment of Transmission-Blocking Vaccine Candidates Against Plasmodium Falciparum. Sci Rep (2015) 5:11193. doi: 10.1038/srep11193
34. de Silva AM, Telford SR 3rd, Brunet LR, Barthold SW, Fikrig E. Borrelia Burgdorferi OspA is an Arthropod-Specific Transmission-Blocking Lyme Disease Vaccine. J Exp Med (1996) 183(1):271–5. doi: 10.1084/jem.183.1.271
35. Nogge G. Aposymbiotic Tsetse Flies, Glossina Morsitans Morsitans Obtained by Feeding on Rabbits Immunized Specifically With Symbionts. J Insect Physiol (1978) 24(4):299–304. doi: 10.1016/0022-1910(78)90026-4
36. Wu-Chuang A, Hodzic A, Mateos-Hernandez L, Estrada-Peña A, Obregon D, Cabezas-Cruz A, et al. Current Debates and Advances in Tick Microbiome Research. CRPVBD (2021) 1:100036. doi: 10.1016/j.crpvbd.2021.100036
37. Narasimhan S, Swei A, Abouneameh S, Pal U, Pedra JHF, Fikrig E. Grappling With the Tick Microbiome. Trends Parasitol (2021) 37(8):722–33. doi: 10.1016/j.pt.2021.04.004
38. Pumpuni CB, Beier MS, Nataro JP, Guers LD, Davis JR. Plasmodium Falciparum: Inhibition of Sporogonic Development in Anopheles Stephensi by Gram-Negative Bacteria. Exp Parasitol (1993) 77(2):195–9. doi: 10.1006/expr.1993.1076
39. Pumpuni CB, Demaio J, Kent M, Davis JR, Beier JC. Bacterial Population Dynamics in Three Anopheline Species: The Impact on Plasmodium Sporogonic Development. Am J Trop Med Hyg (1996) 54(2):214–8. doi: 10.4269/ajtmh.1996.54.214
40. Straif SC, Mbogo CN, Toure AM, Walker ED, Kaufman M, Toure YT, et al. Midgut Bacteria in Anopheles Gambiae and An. Funestus (Diptera: Culicidae) From Kenya and Mali. J Med Entomol (1998) 35(3):222–6. doi: 10.1093/jmedent/35.3.222
41. Dong Y, Manfredini F, Dimopoulos G. Implication of the Mosquito Midgut Microbiota in the Defense Against Malaria Parasites. PloS Pathog (2009) 5(5):e1000423. doi: 10.1371/journal.ppat.1000423
42. Gendrin M, Rodgers F, Yerbanga R, Bosco J, Basañez MG, Cohuet A, et al. Antibiotics in Ingested Human Blood Affect the Mosquito Microbiota and Capacity to Transmit Malaria. Nat Commun (2015) 6:5921. doi: 10.1038/ncomms6921
43. Yilmaz B, Portugal S, Tran TM, Gozzelino R, Ramos S, Gomes J, et al. Gut Microbiota Elicits a Protective Immune Response Against Malaria Transmission. Cell (2014) 159(6):1277–89. doi: 10.1016/j.cell.2014.10.053
44. Mateos-Hernández L, Risco-Castillo V, Torres-Maravilla E, Bermúdez-Humarán LG, Alberdi P, Hodzic A, et al. Gut Microbiota Abrogates Anti-α-Gal IgA Response in Lungs and Protects Against Experimental Aspergillus Infection in Poultry. Vaccines (Basel) (2020) 8(2):285. doi: 10.3390/vaccines8020285
45. Cabezas-Cruz A, Mateos-Hernández L, Alberdi P, Villar M, Riveau G, Hermann E, et al. Effect of Blood Type on Anti-α-Gal Immunity and the Incidence of Infectious Diseases. Exp Mol Med (2017) 49(3):e301. doi: 10.1038/emm.2016.164
46. Wu-Chuang A, Obregon D, Mateos-Hernández L, Cabezas-Cruz A. Anti-Tick Microbiota Vaccines: How can This Actually Work? Biologia (2021). doi: 10.1007/s11756-021-00818-6
47. Burt A. Site-Specific Selfish Genes as Tools for the Control and Genetic Engineering of Natural Populations. Proc Biol Sci (2003) 270(1518):921–8. doi: 10.1098/rspb.2002.2319
48. Dimitrov D, Palinauskas V, Iezhova TA, Bernotiene R, Ilgunas M, Bukauskaite D, et al. Plasmodium Spp.: An Experimental Study on Vertebrate Host Susceptibility to Avian Malaria. Exp Parasitol (2015) 148:1–16. doi: 10.1016/j.exppara.2014.11.005
49. Palinauskas V, Valkiunas G, Bolshakov CV, Bensch S. Plasmodium Relictum (Lineage P-SGS1): Effects on Experimentally Infected Passerine Birds. Exp Parasitol (2008) 120:372–80. doi: 10.1016/j.exppara.2008.09.001
50. Žiegytė R, Bernotienė R, Bukauskaitė D, Palinauskas V, Iezhova T, Valkiūnas G. Complete Sporogony of Plasmodium Relictum (Lineages Psgs1 and Pgrw11) in Mosquito Culex Pipiens Pipiens Form Molestus, With Implications to Avian Malaria Epidemiology. J Parasitol (2014) 100:878–82. doi: 10.1645/13-469.1
51. Kazlauskiene R, Bernotiene R, Palinauskas V, Iezhova TA, Valkiunas G. Plasmodium Relictum (Lineages Psgs1 and Pgrw11): Complete Synchronous Sporogony in Mosquitoes Culex Pipiens Pipiens. Exp Parasitol (2013) 133:454–61. doi: 10.1016/j.exppara.2013.01.008
52. Valkiūnas G, Iezhova TA, Križanauskienė A, Palinauskas V, Sehgal RNM, Bensch S. A Comparative Analysis of Microscopy and PCR-Based Detection Methods for Blood Parasites. J Parasitol (2008) 94:1395–401. doi: 10.1645/GE-1570.1
53. Godfrey RD, Fedynich AM, Pence DB. Quantification of Hematozoa in Blood Smears. J Wildl Dis (1987) 23:558–65. doi: 10.7589/0090-3558-23.4.558
54. Sambrook J, Fritsch EF, Mamiatis T. Molecular Cloning: A Laboratory Manual. 3rd. New York, NY: Cold Spring Harbor Laboratory Press (2001).
55. Hellgren O, Waldenström J, Bensch S. A New PCR Assay for Simultaneous Studies of Leucocytozoon, Plasmodium, and Haemoproteus From Avian Blood. J Parasitol (2004) 90:797–802. doi: 10.1645/GE-184R1
56. Bensch S, Stjernman M, Hasselquist D, Orjan O, Hannson B, Westerdahl H, et al. Host Specificity in Avian Blood Parasites: A Study of Plasmodium and Haemoproteus Mitochondrial DNA Amplified From Birds. Proc R Soc B Biol Sci (2000) 267:1583–9. doi: 10.1098/rspb.2000.1181
57. Bolyen E, Rideout JR, Dillon MR, Bokulich NA, Abnet CC, Al-Ghalith GA, et al. Reproducible, Interactive, Scalable and Extensible Microbiome Data Science Using QIIME 2. Nat Biotechnol (2019) 37(8):852–57. doi: 10.1038/s41587-019-0209-9
58. Callahan BJ, McMurdie PJ, Rosen MJ, Han AW, Johnson AJA, Holmes SP. DADA2: High-Resolution Sample Inference From Illumina Amplicon Data. Nat Methods (2016) 13:581–3. doi: 10.1038/nmeth.3869
59. Katoh K. MAFFT: A Novel Method for Rapid Multiple Sequence Alignment Based on Fast Fourier Transform. Nucleic Acids Res (2002) 30:3059–66. doi: 10.1093/nar/gkf436
60. Price MN, Dehal PS, Arkin AP. FastTree 2 – Approximately Maximum-Likelihood Trees for Large Alignments. PloS One (2010) 5:e9490. doi: 10.1371/journal.pone.0009490
61. Bokulich NA, Kaehler BD, Rideout JR, Dillon M, Bolyen E, Knight R, et al. Optimizing Taxonomic Classification of Marker-Gene Amplicon Sequences With QIIME 2’s Q2-Feature-Classifier Plugin. Microbiome (2018) 6:90. doi: 10.1186/s40168-018-0470-z
62. Yarza P, Yilmaz P, Pruesse E, Glöckner FO, Ludwig W, Schleifer K-H, et al. Uniting the Classification of Cultured and Uncultured Bacteria and Archaea Using 16S rRNA Gene Sequences. Nat Rev Microbiol (2014) 12:635–45. doi: 10.1038/nrmicro3330
63. Friedman J, Alm EJ. Inferring Correlation Networks From Genomic Survey Data. PloS Comput Biol (2012) 8:1–11. doi: 10.1371/journal.pcbi.1002687
65. Bastian M, Heymann S, Jacomy M. Gephi: An Open Source Software for Exploring and Manipulating Networks. In: Proc Int AAAI Conf Weblogs Soc Media (2009) 3:1. doi: 10.13140/2.1.1341.1520
66. Wickham H, Grolemund G. R for Data Science: Import, Tidy, Transform, Visualize, and Model Data. 1st. Canada: O’Reilly (2016). Available at: http://r4ds.had.co.nz/.
67. Faith DP. Conservation Evaluation and Phylogenetic Diversity. Biol Conserv (1992) 61:1–10. doi: 10.1016/0006-3207(92)91201-3
68. Pielou EC. The Measurement of Diversity in Different Types of Biological Collections. J Theor Biol (1966) 13:131–44. doi: 10.1016/0022-5193(66)90013-0
69. Bray JR, Curtis JT. An Ordination of the Upland Forest Communities of Southern Wisconsin. Ecol Monogr (1957) 27:325–49. doi: 10.2307/1942268
70. Love MI, Huber W, Anders S. Moderated Estimation of Fold Change and Dispersion for RNA-Seq Data With Deseq2. Genome Biol (2014) 15:550. doi: 10.1186/s13059-014-0550-8
71. Peschel S, Müller CL, von Mutius E, Boulesteix AL, Depner M. NetCoMi: Network Construction and Comparison for Microbiome Data in R. Brief Bioinf (2021) 22:bbaa290. doi: 10.1093/bib/bbaa290
Keywords: anti-microbiota vaccines, avian malaria, mosquitoes, networks, microbiota
Citation: Aželytė J, Wu-Chuang A, Žiegytė R, Platonova E, Mateos-Hernandez L, Maye J, Obregon D, Palinauskas V and Cabezas-Cruz A (2022) Anti-Microbiota Vaccine Reduces Avian Malaria Infection Within Mosquito Vectors. Front. Immunol. 13:841835. doi: 10.3389/fimmu.2022.841835
Received: 22 December 2021; Accepted: 11 February 2022;
Published: 03 March 2022.
Edited by:
Kézia K.G. Scopel, Juiz de Fora Federal University, BrazilReviewed by:
Yukita Sato, Nihon University, JapanKrishnendu Mukherjee, University Hospital Münster, Germany
Copyright © 2022 Aželytė, Wu-Chuang, Žiegytė, Platonova, Mateos-Hernandez, Maye, Obregon, Palinauskas and Cabezas-Cruz. This is an open-access article distributed under the terms of the Creative Commons Attribution License (CC BY). The use, distribution or reproduction in other forums is permitted, provided the original author(s) and the copyright owner(s) are credited and that the original publication in this journal is cited, in accordance with accepted academic practice. No use, distribution or reproduction is permitted which does not comply with these terms.
*Correspondence: Vaidas Palinauskas, palinauskas@gmail.com; Alejandro Cabezas-Cruz, alejandro.cabezas@vet-alfort.fr
†These authors have contributed equally to this work