- 1Laboratory of Transplantation Immunobiology, Institute of Biomedical Sciences, Department of Immunology, University of São Paulo, São Paulo, Brazil
- 2Laboratory of Biochemical Genetics, Department of Biochemistry and Immunology, Institute of Biomedical Sciences, Federal University of Minas Gerais, Belo Horizonte, Brazil
- 3Laboratory of Clinical and Experimental Immunology, Department of Nephrology, Escola Paulista de Medicina, Federal University of São Paulo, São Paulo, Brazil
Acute rejection (AR) is a process triggered via the recognition of grafted organ-derived antigens by the immune system, which could present as a life-threatening condition. In the context of a kidney transplant, despite improvement with immunosuppressive therapies, AR maintains a significant incidence of 10%, and currently available drugs generally act in similar and canonical pathways of lymphocyte activation. This prompted the research for different approaches to identify potential novel targets that could improve therapeutic interventions. Here, we conducted a transcriptome analysis comparing groups of acute rejection (including T cell-mediated rejection and antibody-mediated rejection) to stable grafts that included differentially expressed genes, transcription factor and kinase enrichment, and Gene Set Enrichment Analysis. These analyses revealed inflammasome enhancement in rejected grafts and AIM2 as a potential component linked to acute rejection, presenting a positive correlation to T-cell activation and a negative correlation to oxidative phosphorylation metabolism. Also, the AIM2 expression showed a global accuracy in discerning acute rejection grafts (area under the curve (AUC) = 0.755 and 0.894, p < 0.0001), and meta-analysis comprising different studies indicated a considerable enhancement of AIM2 in rejection (standardized mean difference (SMD) = 1.45, [CI 95%, 1.18 to 1.71]), especially for T cell-mediated rejection (TCMR) (SMD = 2.01, [CI 95%, 1.58 to 2.45]). These findings could guide future studies of AIM2 as either an adjuvant target for immunosuppression or a potential biomarker for acute rejection and graft survival.
1 Introduction
Kidney transplant has become the key therapeutic strategy for organ failure, especially in the context of chronic diseases such as diabetes, lupus, polycystic kidney disease, and hypertension (1–3). Since its beginning in the 20th century, advances in surgical and conservation techniques allowed an increase in its numbers worldwide: from 2000 to 2020, the total sum of kidney transplants has drastically increased (from 23,084 to above 100,000), as well as its rate per population (from 9.8 to 14.01 per million) (4). These values, however, are below the general demand (5, 6), which prompted a continuous search for strategies that allowed a greater acquisition and offer of organs—such as the use of expanded criteria donor and a central distribution strategy—as well as interventions that reduce organ rejection and dysfunction (7–9).
During transplantation, the kidney is subjected to ischemia (10). This phenomenon leads to graft inflammation and necrosis, resulting in the release of damage-associated molecular patterns (DAMPs) (11). After graft reperfusion, the presence of DAMPs triggers an immune response from the host, leading to immune cell migration and adaptive response, which could contribute to acute rejection (AR) (12, 13).
AR can manifest as an early (<12 months) or late (>12 months) complication of kidney transplant (14). It can be divided according to Banff criteria histopathological findings in antibody-mediated rejection (ABMR), T cell-mediated rejection (TCMR), and borderline for TCMR. TCMR is initiated via the presentation of donor alloantigens to recipient T lymphocytes, leading to T-cell (CD4+ and CD8+) and natural killer recruitment to the allograft, accumulating in kidney tubules, the interstitium, and vascular areas. This process leads to histologic features that allow its classification via Banff criteria in interstitial inflammation, tubulitis, or intimal arteritis (15). The activation of T helper cells leads to the release of soluble factors that support the activation of B cells that could trigger ABMR. ABMR is a severe form of acute rejection and can occur with or without TCMR. After recognition and presentation of recipient HLA and DAMPs, T helper cells interact with B cells, leading to antibody affinity maturation and plasma cell activation. In the graft, antibodies formed to activate the complement pathway and trigger neutrophil recruitment, leading to the endothelial lesion and cell death (16, 17). In the Banff criteria, ABMR is characterized for vascular inflammation—utilized as evidence of acute tissue injury—current antibody and endothelial interaction and serologic markers of donor-specific antibodies (18).
A common mechanism that connects both TCMR and ABMR is the innate inflammatory response triggered upon recognition of DAMPs and mismatched HLA. In this sense, one potential component of innate immunity that has been recently put in perspective in the context of transplant rejection is the inflammasomes (19). Inflammasomes are multimeric protein complexes that are activated via PRR located in the cytosol, recruiting and activating caspase precursors, leading to IL-1β and IL-18 production and consequent induction of proinflammatory immune responses (20).
Another current therapy target is immunomodulation via metabolism reprogramming. Studies regarding T-cell metabolism showed that glycolysis is linked to an increase in inflammation, with Th1 and Th17 mostly relying on glycolytic activity and the mammalian target of rapamycin complex I (MTORC1) pathway to shape an effector response (21, 22). However, OXPHOS and lipid metabolism are linked to immunosuppressive response, with the products of these metabolic pathways being used to produce immunosuppressive cytokines as well as the persistence of memory T cells and generation of T regulatory cells (Tregs) (21–24). For graft rejection, animal studies have shown that the inhibition of glycolysis could prevent or delay rejection by blocking T-cell polarization and increasing relative Treg frequency (25).
Here we performed a transcriptomics analysis, where we aimed to evaluate non-canonical genes and pathways linked to AR in the context of human kidney transplant in comparison to a non-rejecting group. Our analysis indicated possible associations related to the regulation of the inflammatory response and metabolism that could drive graft rejection, which might pave the way for new therapeutic approaches to the patients.
2 Materials and methods
2.1 Microarray data curation
A query was made in the National Center for Biotechnology Information (NCBI) open-source Gene Expression Omnibus (GEO2R) platform (https://www.ncbi.nlm.nih.gov/geo/) for the term “kidney transplant” filtering study type to “expression profile by array” and limiting results to “Homo sapiens” organism. Inclusion criteria comprised kidney tissue samples of acute rejection datasets that presented stable grafts as control. Exclusion criteria included co-transplanted receptors, concomitant chronic rejection, previous meta-analysis, and studies evaluating treatment interventions.
2.2 Differentially expressed gene filtering
Three selected datasets (GSE25902, GSE36059, and GSE129166), all from Affymetrix Human Genome U133 2.0 Array platform, were employed for initial screening. Acute rejection samples were compared to stable grafts by applying the GEO2R online tool (26), which enables to identify differences in gene expression using GEOquery and limma (Linear Models for Microarray Analysis) R packages (27). The threshold for differentially expressed genes (DEGs) was set for a false discovery rate (FDR) <0.05, considering upregulated the genes with logFC > 1 and downregulated the ones presenting logFC < −1. Subsequently, to visualize DEG similarity between datasets, the UpsetR graph and Venn diagram were performed using UpsetR v.1.4.0 (28) and VennDiagram v.1.6.20 (29) packages for R.
2.3 Transcription factor and kinase enrichment analyses
The X2K Appyter (Expression2Kinases) tool was used to perform transcription factor and kinase enrichment analyses based on the DEG list obtained. This tool predicts upstream regulatory networks associated with inputted sets of genes. Discrete query gene sets were compared first to ChEA3 libraries of transcription factor target gene sets assembled from orthogonal “omics” datasets. Afterward, ChEA3 results were put through a protein–protein interaction database to determine the transcription factor intermediate protein interactors. Finally, protein interactors were compared to the KEA3 background database—which contains measured and predicted kinase–substrate interactions, kinase–protein interactions, and interactions supported by co-expression and co-occurrence data—to determine which kinases may be most closely associated with the transcription factor intermediate protein interactors. The top 10 transcription factors and kinases were selected from the enrichment analysis and displayed in the bar charts (Supplementary Figure 2).
2.4 Gene enrichment and functional analyses
2.4.1 Common differentially expressed gene enrichment analysis
To characterize commonly upregulated genes in all three datasets (GSE25902, GSE36059, and GSE129166), we used Venn diagram intersections obtained at jvenn: an interactive Venn diagram viewer (inra.fr) (30). We performed ClusterProfiler analysis in R software version 4.0.2. Out of 52 commonly upregulated genes, we used “ClusterProfiler” to generate enrichment plots of gene ontology analysis through “DOSE”, “enrichplot”, and “reactomePA” packages (31). We generated color-themed dot plots of enriched terms and used them to illustrate statistical biological processes, cellular components, molecular function, and overall representation. We used FDR < 0.05 as a criterion to select enriched terms.
2.4.2 Gene set enrichment analysis
To perform this analysis, we used the computational program Gene Set Enrichment Analysis (GSEA) (gsea-msigdb.org) (32) to upload expression values obtained from the raw data of each dataset. We phenotyped samples as “Acute Rejection” and “Rejection Free” for comparisons and gene set as the permutation type. We selected enriched pathways based on the following criteria: nominal p-value <0.05 and FDR < 0.25. To perform correlation analysis, we set AIM2 gene as the phenotype label to compare with the whole dataset. We also altered the metric for ranking genes for Pearson’s correlation to provide statistical analysis. We selected the top 10 negatively correlated genes present in the oxidative phosphorylation term to be plotted. We generated heatmaps using the Morpheus software (https://software.broadinstitute.org/morpheus) (33).
2.5 Clinical outcome measurements
To assess AIM2 accuracy in identifying acute kidney rejection, the receiver operating characteristic (ROC) curve with multivariate logistic regression was performed on four chosen datasets (GSE25902, GSE36059, GSE129166, and GSE21374). With the use of a cutoff value comprising 72.55% sensitivity and 54.11% specificity for failure risk, samples of GSE21374 were classified into low or high AIM2 expression, aiming to further compare graft survival by the Kaplan–Meier method and log-rank test. Graphs were performed in GraphPad Prism 8.0.1 (GraphPad Software, San Diego, CA, USA), and differences were considered statistically significant for p-value <0.05.
For external validation, a meta-analysis was designed to include 16 of the 18 filtered studies, excluding two of Zhejiang university human 449 oligonucleotide array platforms that did not identify AIM2 gene. Groups had standardized mean difference (SMD) comparison evaluated with random-effects models using the meta v.4.19-1 R package, as previously described for microarray samples (34, 35). Heterogeneity evaluation was performed with InfluenceAnalysis of dmetar v.0.0.9000 package (36).
2.6 Statistical analysis
Statistical analysis was performed to AIM2 level expression and correlations in GraphPad Prism 8.0.1 (GraphPad Software, San Diego, CA, USA). For expression comparison, unpaired t-test and one-way ANOVA followed by the Bonferroni test were used, respectively, for two and three or more group analyses. The plots represented mean ± standard deviation (SD). For correlation measurement, data were initially sorted according to normality distribution using the Kolmogorov–Smirnov test. In normalized samples, correlations were accessed using Pearson’s parametric test, while non-normalized groups were analyzed with non-parametric Spearman’s rank correlation. Correlations’ results were represented by dot plot using the “ggplot2” package (37). Results were considered statistically significant when the p-value <0.05.
3 Results
3.1 Study curation and common differentially expressed gene identification
A systematic search was performed on the Gene Expression Omnibus platform identifying 18 datasets of acute kidney rejection (Supplementary Figure 1). Of them, three did not differentiate acute rejection subtypes, three presented exclusively TCMR grafts, one presented just ABMR rejection, one presented only borderline change, and 10 embraced distinct proportions of TCMR, ABMR, borderline, mixed, or non-specific rejections (Table 1). To avoid distinct readouts according to platforms and to embrace common processes of different rejections types, three studies (GSE25902, GSE36059, and GSE129166) were selected for initially DEG analysis, including, respectively, the datasets with a higher sample number of TCMR, ABMR, or borderline rejection.
In total, 52 genes were found commonly upregulated when comparing acute rejection to stable grafts (Figure 1). However, no overlapping could be defined for downregulated genes, which were found mostly differentially expressed in the GSE25902 dataset (6869 genes) but discreetly changed for GSE129166 (one gene, RBP4) and absent for GSE36059 (no genes).
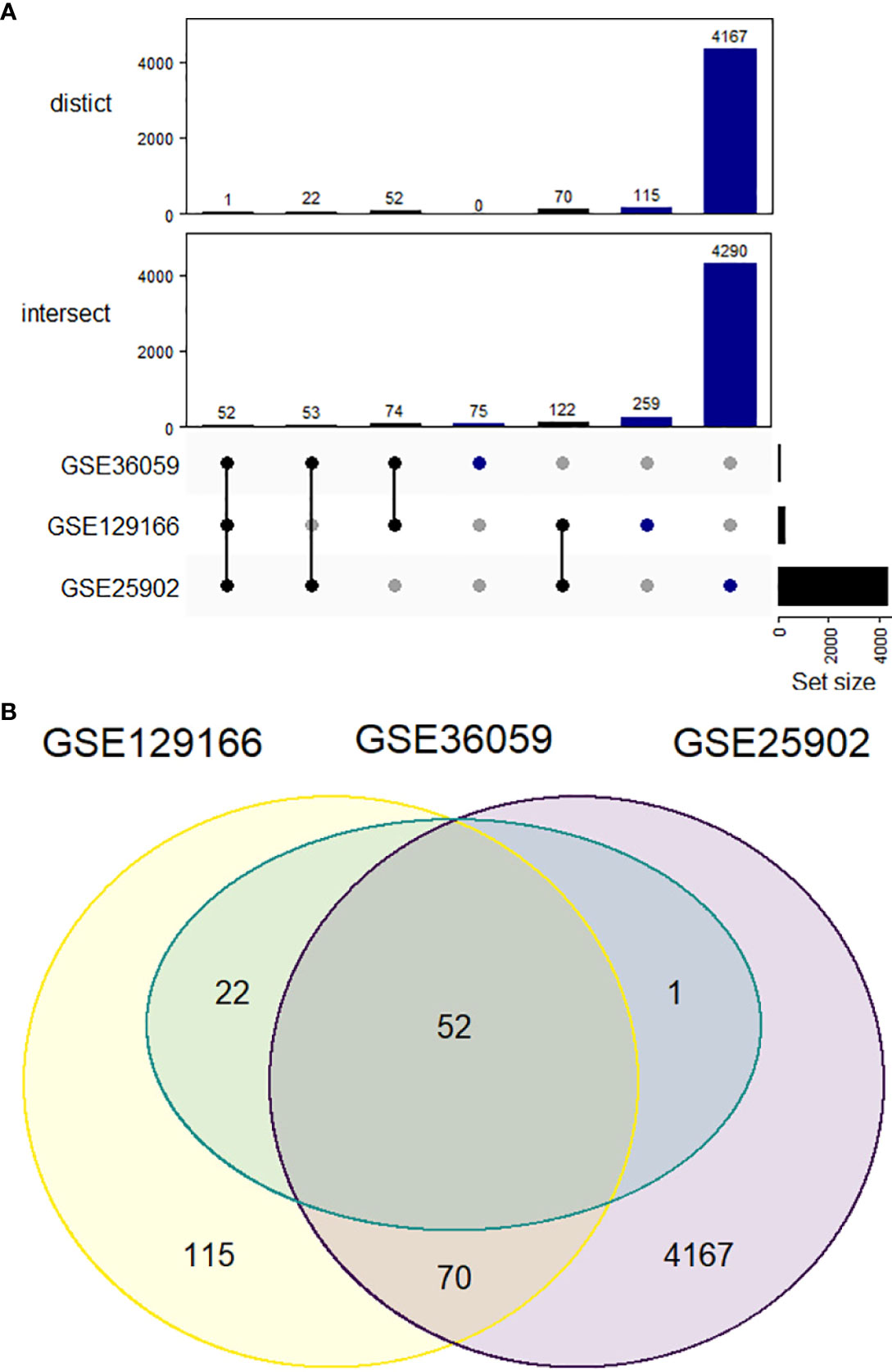
Figure 1 Upregulated differentially expressed genes comparing acute kidney rejection vs. stable graft. (A) Datasets represented by Venn diagram showing 52 overlapped genes between GSE25902, GSE36059, and GSE129166. (B) UpSetR representation of both intersect and distinct forms. The intersect method indicates whole gene similarity between compared studies, while distinct analysis plots the genes shared exclusively among each group of datasets.
3.2 Inflammatory and immune pathways are enriched upon graft rejection
To better discern roles underlying the 52 upregulated genes, GO enrichment analysis was employed for Biological process, Molecular function, and Cellular components, revealing majorly immune-related terms (Figure 2) (Supplementary Figure 2) including interferon signaling (GO:0034341 GO:0034341), T-cell response (GO:0051251, GO:0042098), and CARD-binding domains (GO:0050700), processes that are in line with previous studies results showing both lymphocyte and interferon-gamma enhancement upon kidney rejection (38, 39). Potential upstream targets of the DEG list were concomitantly accessed by the X2K tool and indicated consistent Mitogen-activated protein kinases enrichment (MAPK1, MAPK8, MAPK9, MAPK10, and MAPK14) and transcription factors befitting inflammatory and lymphocyte pathways (TBX21, SP100, IRF8, and BATF) activation (Supplementary Figure 3), highlighting a possible role for these mechanisms in the context of acute rejection.
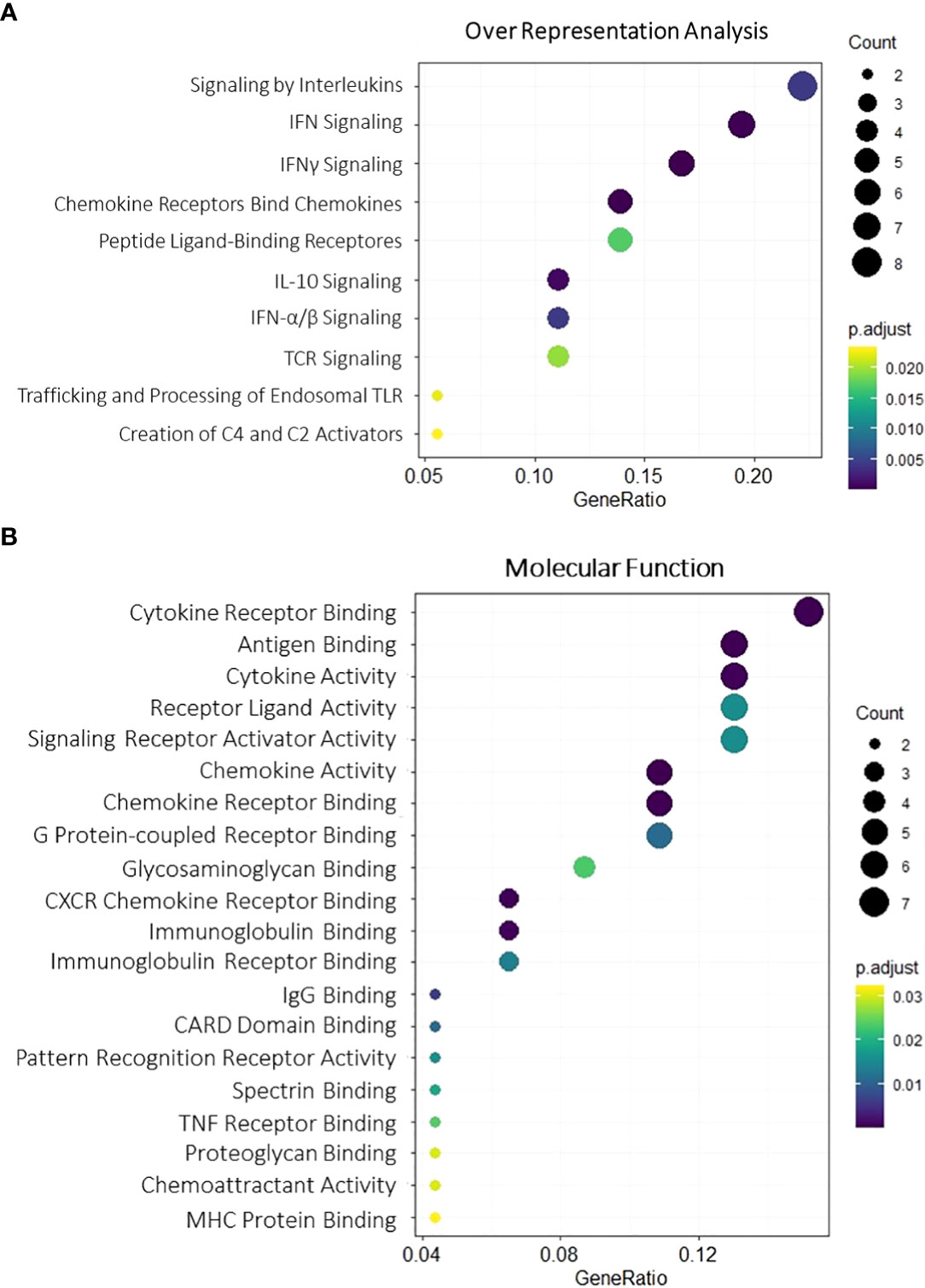
Figure 2 Gene Ontology enrichment for overlapping upregulated DEGs. Molecular function (A) and overall representation (B) for the 52 overlapping upregulated genes. Dot size is proportional to gene count, and color gradients follow adjusted p-value range, assuming higher significance for darker tones and lower significance for light ones. All terms were filtered for adjusted p-value < 0.05. DEGs, differentially expressed genes.
3.3 Inflammasome expression is enhanced upon rejection and correlates with lymphocyte populations
From the previous analysis, we identified CARD-binding domains as enriched terms. CARD activation is traditionally reported on direct or indirect caspase-1 recruitment by inflammasomes (40). In this sense, we first evaluated whether the classically studied inflammasomes NLRP1, NLRP3, NLRC4, and AIM2 could participate in acute rejection. Accordingly, our representative heatmap of the GSE25902, GSE36059, and GSE129166 datasets showed reproducibility and increased expression, especially of AIM2 and NLRP3 (Figure 3A). Also, scatter plot visualization discerns that this enhancement was significant for AIM2 in TCMR (MD = 1.401, 1.426, and 3.248, p < 0.0001) and ABMR (MD = 1.113 and 1.848, p < 0.0001) when compared to stable grafts, with TCMR reaching statistically higher levels among rejection groups (Figure 3B).
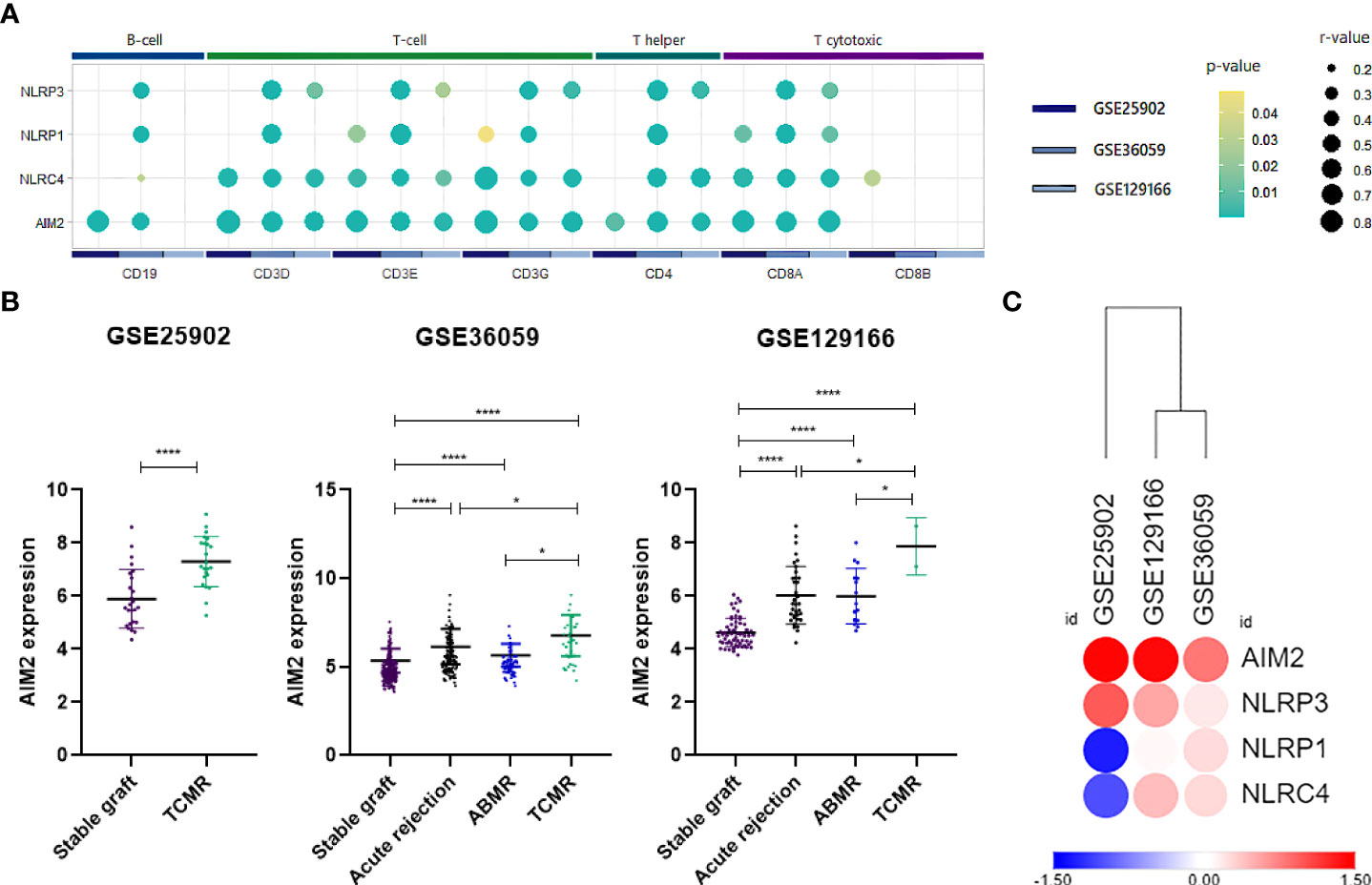
Figure 3 AIM2 and inflammasomes expression. (A) Log2 fold-change heatmap for inflammasomes comparing acute rejection samples to stable grafts. (B) Scatter plot of AIM2 distribution for three selected datasets (GSE25902, GSE36059, and GSE129166), considering overall acute rejection and its subdivisions TCMR and ABMR. (C) Summarized dot plot representing inflammasome correlations between lymphocyte surface markers. Size is designed proportionally to r-value. Yellow color is attributed to less significant correlations, and green tones are attributed to more significant ones. Correlations that did not reach a p-value <0.05 were omitted from the scheme. TCMR, T cell-mediated rejection; ABMR, antibody-mediated rejection.
Since the products of inflammasome activation, such as cytokines and antigens, have been shown to provide signals for T-cell activation, serving as potential interfaces between innate and adaptive immunity (41, 42), we therefore performed correlations between inflammasome genes and surface markers of T cells and B cells. Our analysis outlined a positive association mainly between NLRC4 and AIM2 for CD3, CD4, and CD8A. Additionally, NLRP1 and NLRP3, albeit attained fewer and weaker correlations, were still significant for major T-cell molecules (Figure 3C) (Supplementary Figure 4). Given that AIM2 presented the most significant correlations with T-cell populations, we then hypothesized that AIM2 inflammasome could play a significant role in the regulation of T lymphocytes during allograft rejection.
3.4 AIM2 is associated with allograft rejection and oxidative phosphorylation gene sets
To better understand the role of AIM2 in acute rejection and cellular dysfunction, we performed a GSEA (43) using the three initial datasets to compare the AIM2 phenotype with Hallmarks gene sets. The analysis indicated allograft rejection, interferon, and inflammatory response as the main processes positively regulated for the AIM2 phenotype, while oxidative phosphorylation (OXPHOS) and fatty acid metabolism were most negatively regulated (Supplementary Table 1). When comparing acute rejection to stable graft phenotype, similar results were reproduced, particularly concerning positive processes and the OXPHOS enrichment in non-rejected kidneys (Supplementary Table 2).
Considering that allograft rejection and OXPHOS were the main regulated pathways for both the AIM2 phenotype and acute rejection group and that the current literature supports intracellular metabolism as a potential driver of different leukocytes responses (23), we aimed to access how the central genes of these processes were expressed according to rejection type and lymphocyte infiltration. For that, the top 10 most enriched genes linked to allograft rejection or OXPHOS were selected for a log2FC heatmap (Figure 4) (Supplementary Figure 5), which demonstrated a reproductive different expression, mainly on TCMR subgroups. When analyzing associations to lymphocyte markers, the OXPHOS genes NDUFC1, ETFA, and COX7B were the most negatively correlated to CD3 subunits, CD8A and CD4 (Figure 5) (Supplementary Figure 6), suggesting that a decrease in OXPHOS could either arose from the metabolic shift in T-cell populations or be influenced by these cells’ infiltration.
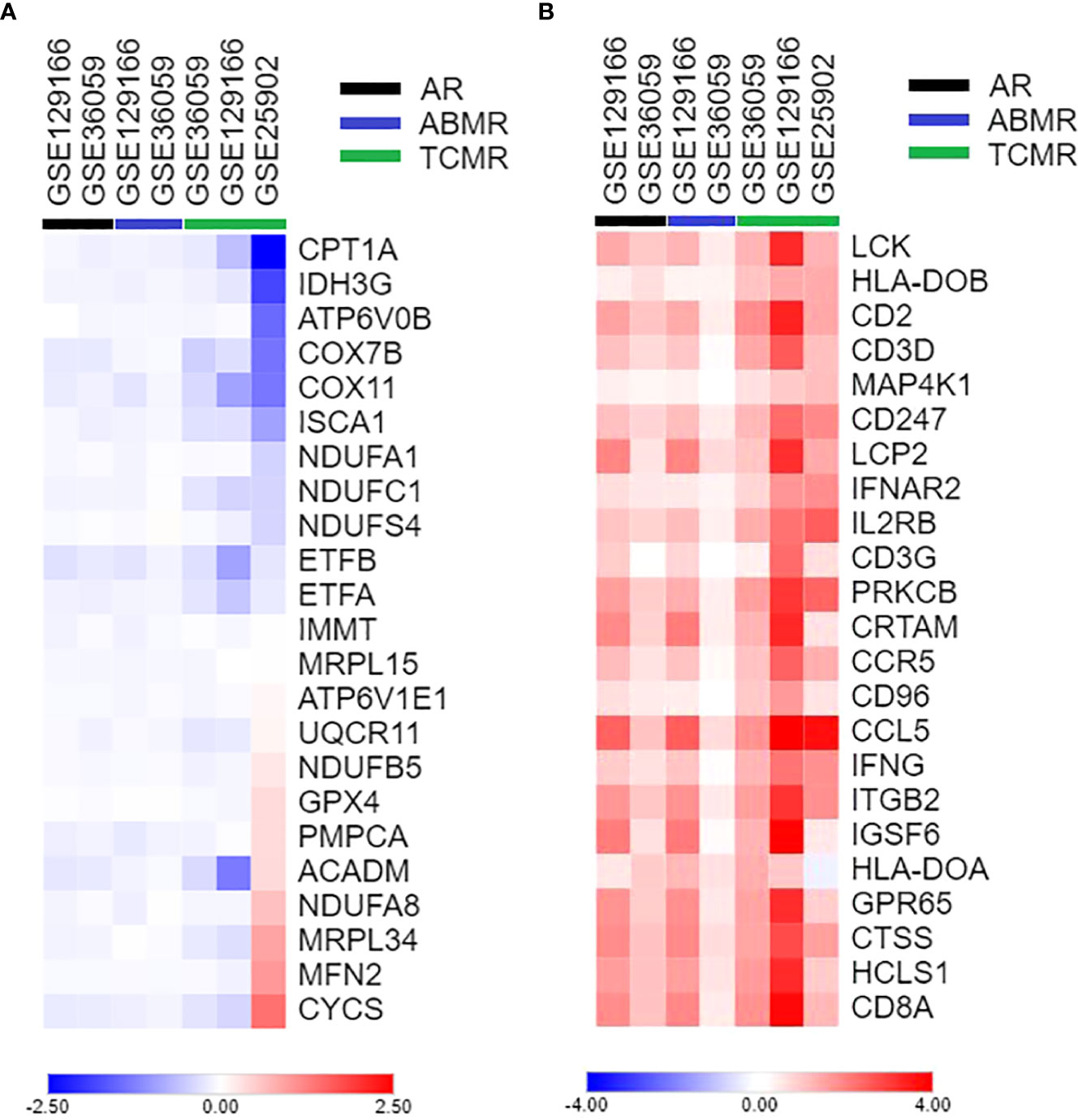
Figure 4 Heatmap of differentially expressed genes between acute rejection and stable graft groups. (A) Top 10 genes of allograft rejection GSEA gene set and inflammasomes. (B) Top 10 genes of oxidative phosphorylation GSEA gene set and inflammasomes. GSEA, Gene Set Enrichment Analysis.
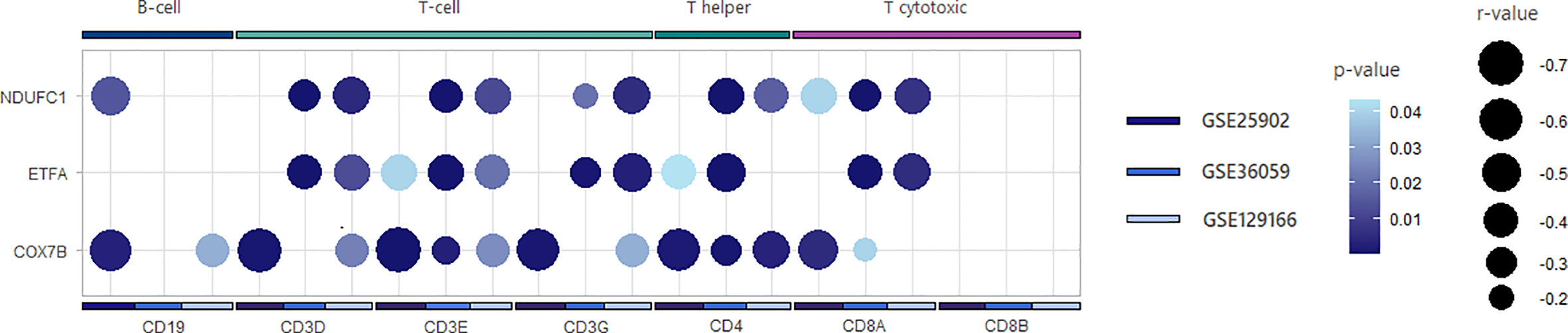
Figure 5 Correlation plot between oxidative phosphorylation genes and lymphocyte receptors genes. Summarized plot representing correlations’ magnitude and significance. Dot size is inversely proportional to r coefficient. Dark blue color indicates more significant correlations, while light tones indicate less significant p-values. Correlations that did not reach p-value <0.05 were suppressed from the plot.
3.5 AIM2 is accurate in discerning acute rejection grafts and implies lower graft survival
After discerning potential molecular processes aligned to AIM2, we enquired whether this gene could reach clinical relevance in identifying acute rejection grafts. In this sense, we employed ROC curves for GSE36059, GSE129166, and GSE25902 (Figure 6A), indicating global accuracy to discern overall rejections (area under the curve (AUC) = 0.755 and 0.894, p < 0.0001) possibly in a more pertinent fashion for TCMR (AUC = 0.865, p < 0.0001) than for ABMR (AUC = 0.657, p < 0.0001), even though just a single dataset enabled their simultaneously comparison.
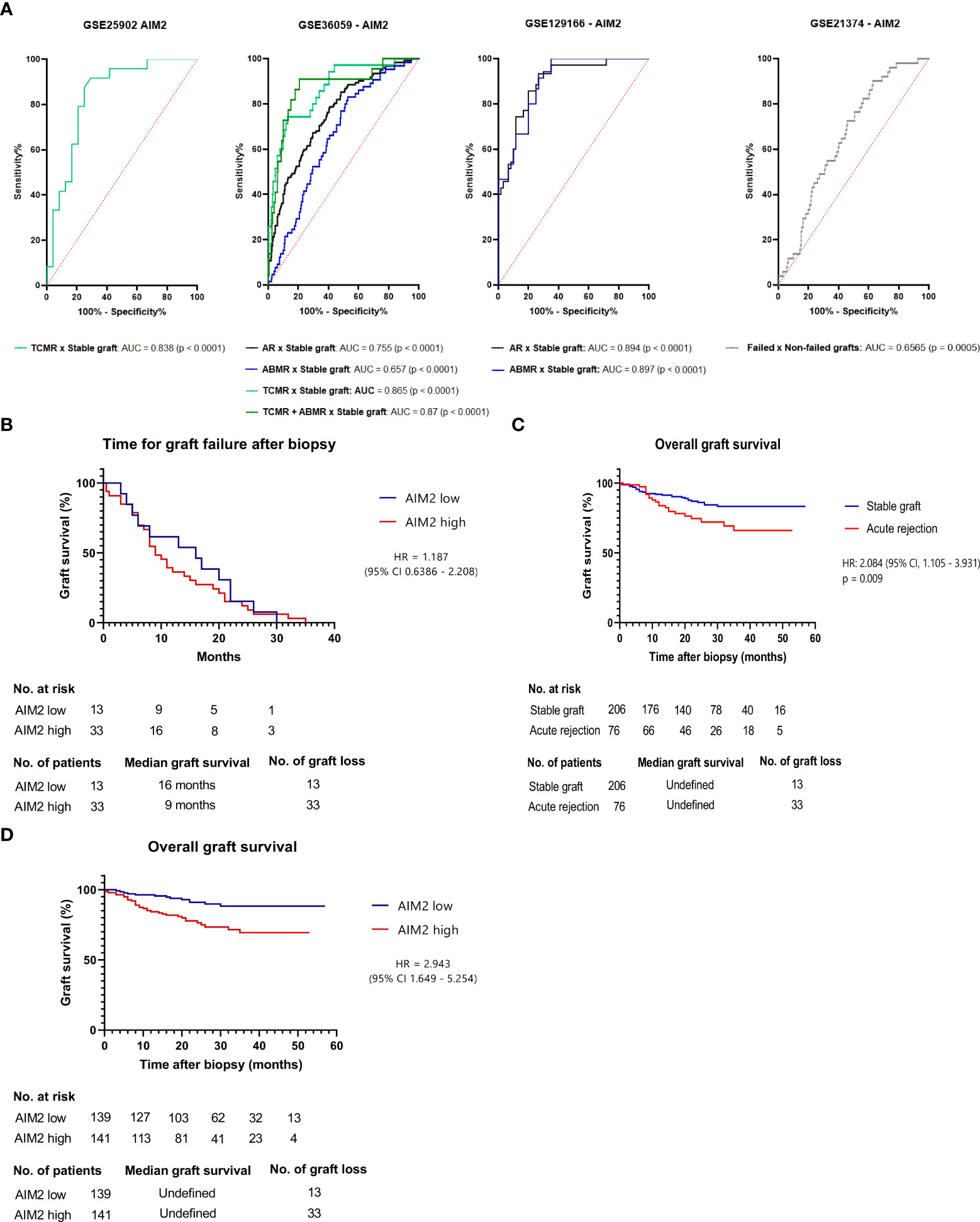
Figure 6 Clinical outcomes linked to AIM2 (A) Receiver Operating Characteristic Curve (ROC) depicting AIM2 accuracy for GSE25902, GSE36059, GSE129166, GSE21374 and its respective TCMR or ABMR samples (B) Graft survival for GSE21374 stable graft and acute rejection samples predicted by Kaplan-Meier curve on log-rank (Mantel-Cox) test. Groups were separated in low or high AIM2 levels according to a 5.738 cut-off that showed 78.95% sensitivity and 71.84% specificity for acute rejection prediction on ROC curve (C) Graft survival for acute rejected samples of GSE21374, applying same 5.738 threshold to determine AIM2 high or low groups. Statistics were determined by the log-rank test (D) Time for graft failure after biopsy for GSE21374 dataset.
To investigate if patients’ outcomes could also be influenced by the gene, an additional dataset (GSE21374) was used to assess graft survival rate based on pre-failure AIM2 expression—this dataset was not added in the previous genome analysis due to the fact that it investigated gene expression prior to transplant; hence, it could not be included in the analysis of “acute rejection versus no-rejection”. Interestingly, we found that AIM2 is indeed useful to determine failure susceptibility even in biopsies taken before graft dysfunction (AUC = 0.6565, p = 0.0005) (Figure 6A). In this line, a value comprising 72.55% sensitivity and 54.11% specificity for failure risk was defined to classify grafts in AIM2-high or AIM2-low expressions, in order to evaluate long-term graft survival. Accordingly, the Kaplan–Meier curves for both groups demonstrated that higher AIM2 grafts achieved shorter survival periods than the lower-expression group (hazard ratio (HR) = 2.943, 95% CI = 1.649–5.254, p < 0.0005) (Figure 6B). Interestingly, this survival difference was more pronounced and statistically significant than the one measured between acute rejection and stable grafts (HR = 2.084, 95% CI = 1.105–3.931 p < 0.009) (Figure 6C), suggesting that AIM2-related failure could potentially act regardless of rejection development. However, when a similar curve is performed including only thefailed grafts, no difference could be observed in survival ratesaccording to AIM2 expression (HR = 1.187, 95% CI = 0.6386–2.208, p = 0.5813) (Figure 6D), indicating that AIM2 is possibly involved in triggering graft loss, but not on its failure time course.
3.6 Meta-analysis for AIM2 expression supports its major enhancement on T cell-mediated rejection grafts
To support data confidence, a meta-analysis was conducted for 15 previously selected studies of either TCMR, ABMR, or borderline patients, totalizing 575 acute rejection samples and 1,214 stable grafts. The result was calculated for the SMD variable and selecting the random-effects model, due to expected differences in rejection types and demographics, features that were not standardized in the dataset description. Indeed, primary analysis showed considerable AIM2 enhancement upon rejection (SMD = 1.45, [CI 95%, 1.18 to 1.71]) followed by significant high heterogeneity (I2 = 75%, p < 0.01) (Figure 7A). Subgroup analysis indicated a higher to AIM2 upregulation on TCMR (SMD = 2.01, [CI 95%, 1.58 to 2.45]) when compared to ABMR (SMD = 1.02, [CI 95%, 0.45 to 1.60]), although maintaining an elevated heterogeneity (TCMR I2 = 76%, p < 0.01; ABMR I2 = 90%, p < 0.01) (Figures 7B, C).
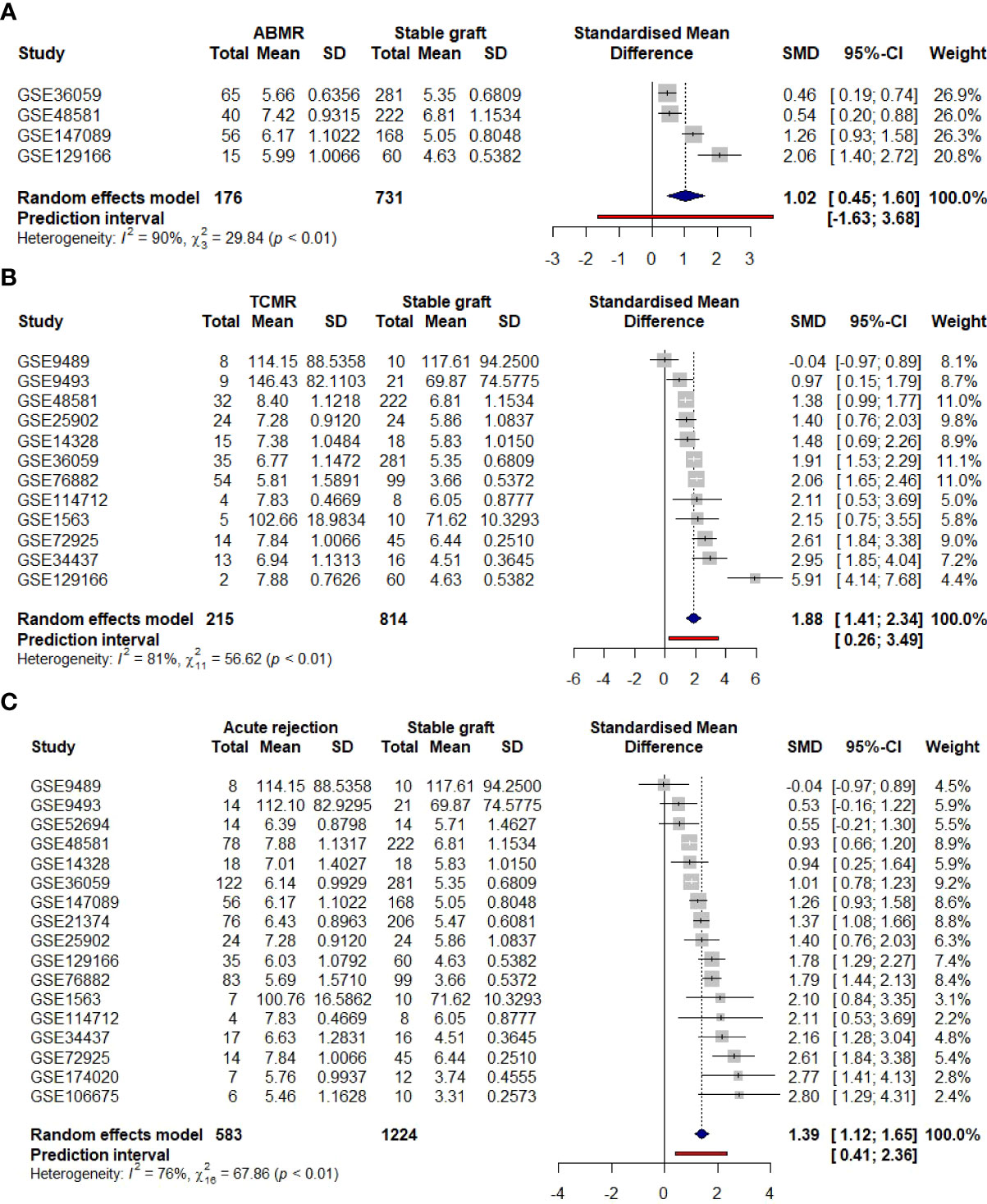
Figure 7 Clinical meta-analysis for AIM2 employing a random-effects model. Lines correspond to 95% confidence intervals. Square size is proportional to study weight on overall effect. Right axis indicates AIM2 enhancement on acute rejection, while left axis indicates an elevation of stable graft. (A) General comparison considering all acute rejections and stable graft samples. (B) Subgroup analysis of the 11 TCMR-only grafts. (C) Subgroup analysis of the four ABMR-only grafts. TCMR, T cell-mediated rejection; ABMR, antibody-mediated rejection.
To access each study collaboration on the computed heterogeneity, an additional test was performed on the 15 datasets with Influence Analysis, which re-calculates I2 values excluding one of the initial datasets. For the overall meta-analysis, GSE72925 and GSE76882 showed major contributions to heterogeneity, while for either TCMR or ABMR subgroups, GSE129166 was the main outline. Nevertheless, even with the respective studies’ omission, heterogeneity could just slightly improve, maintaining a high I2 value of 71%, 58%, and 87% (Supplementary Figures 7A-C).
4 Discussion
Acute rejection is a life-threatening condition affecting nearly 10% of kidney transplants of which 25% do not recover previous graft function (44, 45). Despite the improvement of immunosuppression, the currently available drugs show limitations in reducing these statistics, possibly due to targeting canonical pathways of lymphocyte activation, such as the mammalian target of rapamycin (mTOR), antigen presentation, and IL-2 transcription (46, 47). In this sense, the investigation of new processes involved in pathogenesis could act as adjuvant therapies, for either acute rejection prevention or treatment.
Here, using a transcriptomics-based approach, we innovatively suggest that inflammasomes could act as a complementary branch, reporting for the first time their enhancement and reproducibility on acute rejection, mainly for TCMR. Indeed, inflammasomes are known to be involved in renal homeostasis and inflammatory response (48, 49). Curiously, while NLRP3 has been the one most described in kidney acute and chronic injuries (48), we showed that its upregulation is less remarkable in acute rejection when compared to NLRC4 and AIM2. We hypothesize that this may reflect either the relevance of particular signals, for example, an NLRC4 activation by interferon-regulated genes (50), since interferon processes were enriched in our analyzed datasets, or an AIM2 stimuli secondary to dsDNA release, possibly donor-free DNA molecules (51–54) since apoptotic and necrotic cells are proven to be present in these grafts (55, 56).
Few studies have explored the association between inflammasome activation and the regulation of adaptive immunity. In this sense, it has been demonstrated that activated T cells display a complement C5 protein-dependent NLRP3 inflammasome assembly, which induces T helper 1 (Th1) responses (57), which are typically associated with autoimmune and infectious diseases. However, our group demonstrated that a gain of function in NLRP3 specifically in CD4+ T cells improved the clinical course of disease in a model of experimental autoimmune encephalomyelitis (EAE), which was associated with a reduction of Th1 and Th17 inflammatory responses and a shift toward a Th2 pattern (58). It is important to point out that inflammasome receptors can also modulate adaptive immune responses regardless of their canonical roles in inducing the assembly of the complexes. Accordingly, NLRP3 has been shown to translocate to the nucleus of Tregs and negatively regulate this population’s master transcriptional regulator FOXP3, impairing their differentiation efficiency (59). AIM2, in turn, has been shown to enhance the stability of Tregs through metabolic reprogramming toward beta-oxidation in the mitochondria, restraining autoimmune responses in an inflammasome-independent fashion (60). Altogether, these data indicate that inflammasome receptors are important players in the regulation of T-cell responses and suggest that these processes are context-dependent. Our results here provide evidence that AIM2 expression correlates positively with T cells and is linked to allograft rejection, highlighting a detrimental role for this molecule in the context of kidney transplantation, which could be better explored and targeted in further studies.
Additionally, T cells typically undergo metabolic rewiring after stimulation. The interplay between cell metabolism and effector immune function sets an emerging field termed “immunometabolism” (23), which is unraveling mechanisms that may propose promising therapeutic targets to dampen allograft rejection. Th1 and Th17 cells mostly rely on glycolytic activity in order to shape an effector response, and the MTORC1 and HIF-1α transcription factor are upregulated in both of these cells. However, Tregs usually activate the AMPK pathway, leading to lipid oxidation, OXPHOS, and suppression of immune responses (21, 22). In our results, the enrichment analysis showed that OXPHOS was negatively correlated to AIM2 expression and rejected grafts. We also observed that OXPHOS-related genes were negatively correlated to lymphocyte markers such as CD3 and CD4. Studies show that pharmacological glycolysis ablation can enhance levels of CD4+Foxp3+ cells and reduce CD8+IFN-γ+ cells in transplanted mice (25). Also, in graft-versus-host disease (GvDH), when T cells encounter alloantigens, they preferentially upregulate glycolytic metabolism to produce pro-inflammatory responses. Ablation of glycolysis activator MTORC1 alleviated GvDH progression in mouse models (61). Although our results do not directly provide evidence for different T lymphocyte subset metabolic profiles, they suggest a correlation with these studies in a way that T cells present in rejected kidney allografts may have reduced levels of OXPHOS and lipid oxidation, which may enhance their inflammatory phenotype and, therefore, increase transplant rejection. A proposed integrative mechanism for AIM2 based on our findings is demonstrated in Figure 8.
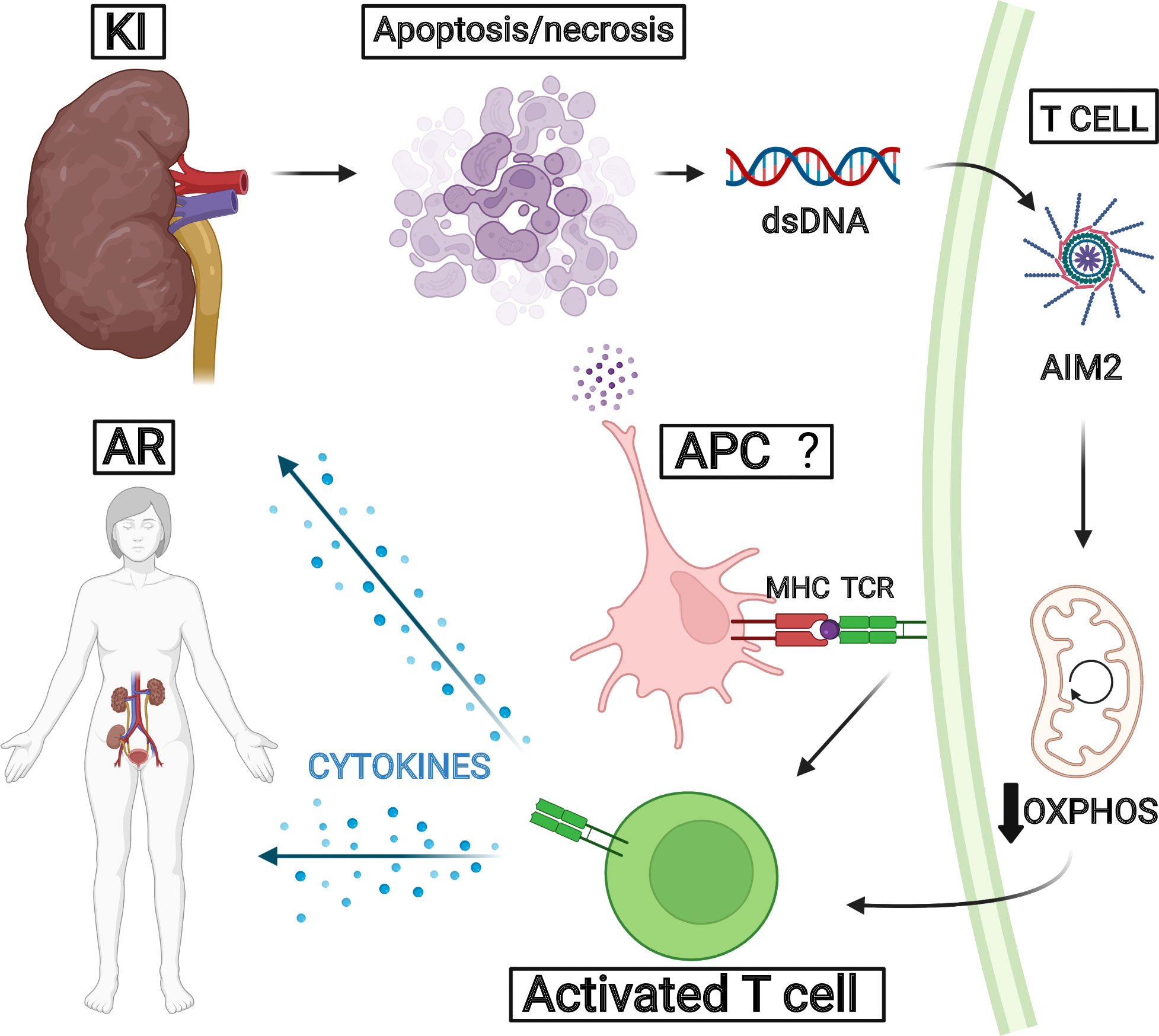
Figure 8 Proposed pathway for AIM2 in acute rejection. We hypothesize that kidney injury secondary to transplantation could trigger tissue apoptosis and necrosis, leading to donor dsDNA release. This molecule could be recognized by AIM2 collaborating for T-cell activation related to OXPHOS decrease. The inflammatory processes reinforce kidney injuries and favor acute rejection development.
Finally, meta-analysis is a strategy to quantify a combined effect size of different studies (62), in the case of bioinformatics, usually employing the standardized mean difference (SMD) variable, which was also applied to remove batch effects, in order to reduce heterogeneity (63–65). In our data, AIM2 attained a significant SMD in the TCMR subgroup, in accordance with our individual-dataset heatmap, suggesting a probable confident non-random finding. Since demographic information is usually incompletely available either in the GEO platform or in published transcriptome papers, our analyzed microarrays could have embraced grafts from different donor types, distinct ischemia periods, and immunosuppressive schemes, which are conditions that affect acute rejection development, potentially modifying our measured effect (66–68). In spite of diagnostic heterogeneity, expression profiling by array has intrinsic variations that may also contribute to this observation. It has been noted that microarrays carried out at different time points but performed in the same laboratory still show discrepancies among them (69). Since the datasets analyzed in our work were retrieved from different centers, we standardized the type of annotation that the microarray was provided, which aids in reducing batch effects (69, 70). In this line, further clinical studies are desired to overcome the intrinsic heterogeneity of in silico studies (65), supporting and refining information concerning which grafts are more susceptible to AIM2 signaling and, thus, identifying the patients who could benefit from drug trials targeting the inflammasomes as adjuvant therapies (71–73).
Currently, acute rejection is suspected with parameters for acute kidney injury—rise in 48 h of serum creatinine >0.3 mg/dl or >50% or oliguria < 0.5 ml/kg/h for more than 6 h, with the diagnosis being made by the realization of biopsy to directly identify allograft rejection (74, 75). These criteria, however, present some clinical challenges: serum creatinine is not specific for allograft rejection and can be elevated in both acute rejection and progression of the disease or new pathologic processes in the kidney (76). Furthermore, the confirmation via kidney biopsy brings financial limitations—since it is necessary for the patient to be hospitalized—as well as the bias of sampling error or variability between professionals (77). This context led to the search for non-invasive biomarkers that could predict acute rejection, specifically in blood and urine samples that were linked to TCMR, like CXCL10, TNF-alpha, and miR-155-5p (74, 75). Our findings for AIM2 expression in the tissue and accuracy for graft failure could prompt new analysis for its expression in patient biosamples.
Even though AIM2 has been in recent discussions as a potential target to understand and regulate the progression of inflammatory conditions (78), we were unable to find references that directly linked the molecule to acute rejection both inside and outside the context of a kidney transplant. Going further from the potential mechanism linked to AIM2 and acute rejection previously discussed, markers that permit identifying and predicting acute rejection in kidney transplants are still being researched. In summary, potential non-invasive markers bring a new perspective to the diagnosis of acute rejection as well as a potential way of introducing different interventions according to the findings. In this sense, AIM2 could be a potential new biomarker, especially for TCMR. Even though the previously cited markers showed a good overall diagnostic accuracy with a more practical clinical approach since they are collected from the blood and urine; for acute rejection, here we also include the potential use of AIM2 as a predictor for overall graft survival and not only acute rejection. This could prompt the evaluation of AIM2 as a marker in blood and urine as well.
Data availability statement
The datasets presented in this study can be found in online repositories. The names of the repository/repositories and accession number(s) can be found in the article/Supplementary Material.
Author contributions
NF and JV selected datasets and wrote the first draft of the manuscript. LE and BG contributed writing sections of the manuscript. NF and LE performed computational analysis. NF, JV and BG designed correlations. NF, JV, LE, IZ, GR and BG collaborate in data interpretation and visualization. NO conceived and supervised the study. All authors contributed to edition, critical revision and approved the submitted version.
Funding
The research was supported financially by São Paulo Research Foundation (FAPESP) (18/21844-6, 2019/22409-4, 17/05264-7) and National Council for Scientific and Technological Development (CNPq) and CAPES (financial code 001) grants.
Conflict of interest
The authors declare that the research was conducted in the absence of any commercial or financial relationships that could be construed as a potential conflict of interest.
Publisher’s note
All claims expressed in this article are solely those of the authors and do not necessarily represent those of their affiliated organizations, or those of the publisher, the editors and the reviewers. Any product that may be evaluated in this article, or claim that may be made by its manufacturer, is not guaranteed or endorsed by the publisher.
Supplementary material
The Supplementary Material for this article can be found online at: https://www.frontiersin.org/articles/10.3389/fimmu.2022.839359/full#supplementary-material
Supplementary Figure 1 | Query strategy. Flowchart for systematic studies selection, adapted from Reporting Items for Systematic Reviews and Meta-Analyses (PRISMA) 2020 guidelines.
Supplementary Figure 2 | Transcription factor and kinase enrichment analysis. (A). The bar chart is representative of the mean rank of the highest ranked transcription factors from ChEA3. The y-axis displays the different transcription factors, and the x-axis displays the average mean rank of a transcription factor across all the available libraries. (B). The bar chart is a mean rank bar chart for the top ranked kinases from KEA3. The y-axis displays the different kinases, and the x-axis displays the mean rank of a specific kinase across all the available libraries.
Supplementary Figure 3 | Gene Ontology enrichment for overlapping up-regulated DEGs. Biological processes and cellular components representation for the 52 overlapping up-regulated genes. Dot size is proportional to gene count, and color gradients follow adjusted p-value range, assuming higher significance for darker tones, and lower significance for light ones. All terms were filtered for adjusted p-value < 0.05.
Supplementary Figure 4 | Correlation plot between lymphocyte receptors genes and AIM2 expression (A) CD3 subunits used as general T-cell markers (B) CD4 expression used as T-helper signature (C) CD8 subunits used to represent T-cytotoxic cells (D) CD19 expression used for B-cell assessment.
Supplementary Figure 5 | Log-2-fold-change heatmap of differentially expressed genes between acute rejection and stable graft groups for all datasets (A) Top 10 genes of allograft rejection GSEA geneset and inflammasomes (B) Top 10 genes of oxidative phosphorylation GSEA geneset and inflammasomes.
Supplementary Figure 6 | Correlation plot between lymphocyte receptors genes and oxidative phosphorylation related genes (A) CD3 subunits used as general T-cell markers (B) CD4 expression used as T-helper signature (C) CD8 subunits used to represent T-cytotoxic cells (D) CD19 expression used for B-cell assessment.
Supplementary Figure 7 | Studies heterogeneity contribution accessed by InfluenceAnalysis (A) General comparison considering all acute rejections and stable graft samples (B) Subgroup analysis of the 11 TCMR-only grafts (C) Subgroup analysis of the 4 ABMR-only graft.
Supplementary Table 1 | Genesets related to AIM2. Top 5 genesets of GSEA Hallmark enrichment for AIM2 phenotype, indicating processes more positively or negatively correlated to AIM2.
Supplementary Table 2 | Genesets related to acute rejection. Top 5 genesets of GSEA Hallmark enrichment comparing acute rejection vs. stable graft phenotype.
Supplementary Table 3 | Hallmarks positively and negatively enriched in acute rejection.
References
1. Thurlow JS, Joshi M, Yan G, Norris KC, Agodoa LY, Yuan CM, et al. Global epidemiology of end-stage kidney disease and disparities in kidney replacement therapy. Am J Nephrol (2021) 52(2):98–107. doi: 10.1159/000514550
2. Lorent M, Foucher Y, Kerleau K, Brouard S, Baayen C, Lebouter S, et al. The EKiTE network (epidemiology in kidney transplantation - a European validated database): An initiative epidemiological and translational European collaborative research. BMC Nephrol (2019) 20(1):1–10. doi: 10.1186/s12882-019-1522-8
3. eCFR. 42 CFR part 121 – organ procurement and transplantation network . Available at: https://www.ecfr.gov/current/title-42/chapter-I/subchapter-K/part-121.
4. Data (Charts and tables) - GODT . Available at: http://www.transplant-observatory.org/data-charts-and-tables/.
5. Soares LS da S, de Brito ES, Magedanz L, França FA, de Araújo WN, Galato D. Solid organ transplantation in Brazil: A descriptive study of distribution and access inequalities across the Brazilian territory, 2001-2017. Epidemiologia e Servicos saude : Rev do Sistema Unico Saude do Brasil (2020) 29(1):e2018512. doi: 10.5123/s1679-49742020000100014
6. Lewis A, Koukoura A, Tsianos GI, Gargavanis AA, Nielsen AA, Vassiliadis E. Organ donation in the US and Europe: The supply vs demand imbalance. Transplant Rev (2021) 35(2):100585. doi: 10.1016/j.trre.2020.100585
7. Lentine KL, Pastan S, Mohan S, Reese PP, Leichtman A, Delmonico FL, et al. A roadmap for innovation to advance transplant access and outcomes: A position statement from the national kidney foundation. Am J Kidney Dis (2021) 78(3):319–32. doi: 10.1053/j.ajkd.2021.05.007
8. Hariharan S, Israni AK, Danovitch G. Long-term survival after kidney transplantation. New Engl J Med (2021) 385(8):729–43. doi: 10.1056/NEJMra2014530
9. Abramowicz D, Oberbauer R, Heemann U, Viklicky O, Peruzzi L, Mariat C, et al. Recent advances in kidney transplantation: A viewpoint from the Descartes advisory board. Nephrol Dial Transplant (2018) 33(10):1699–707. doi: 10.1093/ndt/gfx365
10. Koo DDH, Welsh KI, Roake JA, Morris PJ, Fuggle S v. Ischemia/Reperfusion injury in human kidney transplantation: An immunohistochemical analysis of changes after reperfusion. Am J Pathol (1998) 153(2):557–66. doi: 10.1016/S0002-9440(10)65598-8
11. Nogueira E, Salomao R, Brunialti MKC, Ozaki KS, Marques GDM, Cenedeze MA, et al. Expression of TLR-4 and -2 in peripheral mononuclear cells in renal transplant patients with TLR-4 gene polymorphism. Int Immunopharmacol (2010) 10(12):1481–5. doi: 10.1016/j.intimp.2010.09.005
12. Land WG, Agostinis P, Gasser S, Garg AD, Linkermann A. Transplantation and damage-associated molecular patterns (DAMPs). Am J Transplantation (2016) 16(12):3338–61. doi: 10.1111/ajt.13963
13. Land WG, Agostinis P, Gasser S, Garg AD, Linkermann A, Abendroth DK, et al. DAMP-induced allograft and tumor rejection: The circle is closing. Am J Transplant (2016) 16(12):3322–37. doi: 10.1111/ajt.14012
14. Matas AJ, Smith JM, Skeans MA, Thompson B, Gustafson SK, Schnitzler MA, et al. OPTN/SRTR 2012 annual data report: Kidney. Am J Transplant (2014) 14 Suppl 1:11–44. doi: 10.1111/ajt.12579
15. Randhawa P. T-Cell-mediated rejection of the kidney in the era of donor-specific antibodies: Diagnostic challenges and clinical significance. Curr Opin Organ Transplant (2015) 20(3):325–32. doi: 10.1097/mot.0000000000000189
16. Kenta I, Takaaki K. Molecular mechanisms of antibody-mediated rejection and accommodation in organ transplantation. Nephron (2020) 144 Suppl 1:2–6. doi: 10.1159/000510747
17. Senev A, Coemans M, Lerut E, van Sandt V, Daniëls L, Kuypers D, et al. Histological picture of antibody-mediated rejection without donor-specific anti-HLA antibodies: Clinical presentation and implications for outcome. Am J Transplantation (2019) 19(3):763–80. doi: 10.1111/ajt.15074
18. Loupy A, Haas M, Roufosse C, Naesens M, Adam B, Afrouzian M, et al. The banff 2019 kidney meeting report (I): Updates on and clarification of criteria for T cell– and antibody-mediated rejection. Am J Transplant (2020) 20(9):2318–31. doi: 10.1111/ajt.15898
19. Dessing MC, Kers J, Damman J, Navis GJ, Florquin S, Leemans JC. Donor and recipient genetic variants in NLRP3 associate with early acute rejection following kidney transplantation. Sci Rep (2016) 6(1):1–9. doi: 10.1038/srep36315
20. Martinon F, Tschopp J. Inflammatory caspases and inflammasomes: master switches of inflammation. Cell Death Differentiation (2007) 14(1):10–22. doi: 10.1038/sj.cdd.4402038
21. Maciver NJ, Michalek RD, Rathmell JC. Metabolic regulation of T lymphocytes, (2013). 31:259–83. doi: 10.1146/annurev-immunol-032712-095956.
22. Buck MD, O’Sullivan D, Pearce EL. T Cell metabolism drives immunity. J Exp Med (2015) 212(9):1345–60. doi: 10.1084/jem.20151159
23. O’Neill LAJ, Kishton RJ, Rathmell J. A guide to immunometabolism for immunologists. Nat Rev Immunol (2016) 16(9):553–65. doi: 10.1038/nri.2016.70
24. Zasłona Z, O’Neill LAJ. Cytokine-like roles for metabolites in immunity. Mol Cell (2020) 78(5):814–23. doi: 10.1016/j.molcel.2020.04.002
25. Lee CF, Lo YC, Cheng CH, Furtmüller GJ, Oh B, Andrade-Oliveira V, et al. Preventing allograft rejection by targeting immune metabolism. Cell Rep (2015) 13(4):760–70. doi: 10.1016/j.celrep.2015.09.036
26. Sean D, Meltzer PS. GEOquery: A bridge between the gene expression omnibus (GEO) and BioConductor. Bioinformatics (2007) 23(14):1846–7. doi: 10.1093/bioinformatics/btm254
27. Smyth GK. Limma: Linear models for microarray data. In: Bioinformatics and computational biology solutions using r and bioconductor (2005) p. 397–420. Available at: vailable at: https://link.springer.com/chapter/10.1007/0-387-29362-0_23.
28. Conway JR, Lex A, Gehlenborg N. UpSetR: An r package for the visualization of intersecting sets and their properties. Bioinformatics (2017) 33(18):2938–40. doi: 10.1093/bioinformatics/btx364
29. Chen H, Boutros PC. VennDiagram: A package for the generation of highly-customizable Venn and Euler diagrams in r. BMC Bioinf (2011) 12:35. doi: 10.1186/1471-2105-12-35
30. Bardou P, Mariette J, Escudié F, Djemiel C, Klopp C. Jvenn: An interactive Venn diagram viewer. BMC Bioinf (2014) 15(1). doi: 10.1186/1471-2105-15-293
31. Yu G, Wang LG, Han Y, He QY. clusterProfiler: An r package for comparing biological themes among gene clusters. OMICS (2012) 16(5):284–7. doi: 10.1089/omi.2011.0118
32. Subramanian A, Tamayo P, Mootha VK, Mukherjee S, Ebert BL, Gillette MA, et al. Gene set enrichment analysis: A knowledge-based approach for interpreting genome-wide expression profiles. Proc Natl Acad Sci 102(43):15545–50. doi: 10.1073/pnas.0506580102
33. Starruß J, de Back W, Brusch L, Deutsch A. Morpheus: A user-friendly modeling environment for multiscale and multicellular systems biology. Bioinformatics (2014) 30(9):1331–2. doi: 10.1093/bioinformatics/btt772
34. He RQ, Wu PR, Xue XL, Yang X, Liang HW, Qiu XH, et al. Downregulated miR-23b-3p expression acts as a predictor of hepatocellular carcinoma progression: A study based on public data and RT-qPCR verification. Int J Mol Med (2018) 41(5):2813–31. doi: 10.3892/ijmm.2018.3513
35. Balduzzi S, Rücker G, Schwarzer G. How to perform a meta-analysis with r: A practical tutorial. Evid Based Ment Health (2019) 22(4):153–60. doi: 10.1136/ebmental-2019-300117
36. Harrer M, Cuijpers P, Ebert D. Companion r package for the guide doing meta-analysis in r. dmetar. (2021). Available at: http://dmetar.protectlab.org/.
37. Create elegant data visualisations using the grammar of graphics. ggplot2. (2021). Available at: https://ggplot2.tidyverse.org/
38. Goerlich N, Brand HA, Langhans V, Tesch S, Schachtner T, Koch B, et al. Kidney transplant monitoring by urinary flow cytometry: Biomarker combination of T cells, renal tubular epithelial cells, and podocalyxin-positive cells detects rejection. Sci Rep (2020) 10(1):1–11. doi: 10.1038/s41598-020-57524-7
39. Halloran PF, Miller LW, Urmson J, Ramassar V, Zhu LF, Kneteman NM, et al. IFN-γ alters the pathology of graft rejection: Protection from early necrosis. J Immunol (2001) 166(12):7072–81. doi: 10.4049/jimmunol.166.12.7072
40. Zheng D, Liwinski T, Elinav E. Inflammasome activation and regulation: Toward a better understanding of complex mechanisms. Cell Discovery (2020) 6(1):1–22. doi: 10.1038/s41421-020-0167-x
41. Bandera A, Masetti M, Fabbiani M, Biasin M, Muscatello A, Squillace N, et al. The NLRP3 inflammasome is upregulated in HIV-infected antiretroviral therapy-treated individuals with defective immune recovery. Front Immunol (2018) 9(FEB). doi: 10.3389/fimmu.2018.00214
42. Deets KA, Vance RE. Inflammasomes and adaptive immune responses. Nat Immunol (2021) 22(4):412–22. doi: 10.1038/s41590-021-00869-6
43. Subramanian A, Tamayo P, Mootha VK, Mukherjee S, Ebert BL, Gillette MA, et al. Gene set enrichment analysis: A knowledge-based approach for interpreting genome-wide expression profiles. Proc Natl Acad Sci U.S.A. (2005) 102(43):15545–50. doi: 10.1073/pnas.0506580102
44. Clayton PA, McDonald SP, Russ GR, Chadban SJ. Long-term outcomes after acute rejection in kidney transplant recipients: An ANZDATA analysis. J Am Soc Nephrol (2019) 30(9):1697–707. doi: 10.1681/ASN.2018111101
45. Cooper JE. Evaluation and treatment of acute rejection in kidney allografts. Clin J Am Soc Nephrol (2020) 15(3):430–8. doi: 10.2215/CJN.11991019
46. Wiseman AC. Immunosuppressive medications. Clin J Am Soc Nephrol (2016) 11(2):332–43. doi: 10.2215/CJN.08570814
47. Neuwirt H, Rudnicki M, Schratzberger P, Pirklbauer M, Kronbichler A, Mayer G, et al. Mmunosuppression after renal transplantation. Imemo - Magazine Eur Med Oncol (2019) 12(3):216–21. doi: 10.1007/s12254-019-0507-4
48. Xiang H, Zhu F, Xu Z, Xiong J. Role of inflammasomes in kidney diseases via both canonical and non-canonical pathways. Front Cell Dev Biol (2020) 8:106. doi: 10.3389/fcell.2020.00106
49. Chun J, Chung H, Wang X, Barry R, Taheri ZM, Platnich JM, et al. NLRP3 localizes to the tubular epithelium in human kidney and correlates with outcome in IgA nephropathy. Sci Rep (2016) 6. doi: 10.1038/srep24667
50. Wen J, Xuan B, Liu Y, Wang L, He L, Meng X, et al. Updating the NLRC4 inflammasome: from bacterial infections to autoimmunity and cancer. Front Immunol (2021) 12:2634. doi: 10.3389/fimmu.2021.702527
51. Filev AD, Shmarina G v., Ershova ES, Veiko NN, Martynov A v., Borzikova MA, et al. Oxidized cell-free DNA role in the antioxidant defense mechanisms under stress. Oxid Med Cell Longevity (2019) 2019. doi: 10.1155/2019/1245749
52. Agbor-Enoh S, Shah P, Tunc I, Hsu S, Russell S, Feller E, et al. Cell-free DNA to detect heart allograft acute rejection. Circulation (2021) 143:1184–97. doi: 10.1161/CIRCULATIONAHA.120.049098
53. Tsuji N, Agbor-Enoh S. Cell-free DNA beyond a biomarker for rejection: Biological trigger of tissue injury and potential therapeutics. J Heart Lung Transplant (2021) 40(6):405–13. doi: 10.1016/j.healun.2021.03.007
54. Bloom RD, Bromberg JS, Poggio ED, Bunnapradist S, Langone AJ, Sood P, et al. Cell-free DNA and active rejection in kidney allografts. J Am Soc Nephrol (2017) 28(7):2221–32. doi: 10.1681/ASN.2016091034
55. Miller LW, Granville DJ, Narula J, McManus BM. APOPTOSIS IN CARDIAC TRANSPLANT REJECTION. Cardiol Clinics (2001) 19(1):141–54. doi: 10.1016/S0733-8651(05)70200-9
56. Wever PC, Aten J, Rentenaar RJ, Hack CE, Koopman G, Weening JJ, et al. Apoptotic tubular cell death during acute renal allograft rejection. Clin Nephrol (1998) 49(1):28–34.
57. Arbore G, West EE, Spolski R, Robertson AAB, Klos A, Rheinheimer C, et al. T Helper 1 immunity requires complement-driven NLRP3 inflammasome activity in CD4+ T cells. Science (2016) 352(6292). doi: 10.1126/science.aad1210
58. Braga TT, Brandao WN, Azevedo H, Terra FF, Melo ACL, Pereira FV, et al. NLRP3 gain-of-function in CD4+ T lymphocytes ameliorates experimental autoimmune encephalomyelitis. Clin Sci (2019) 133(17):1901–16. doi: 10.1042/CS20190506
59. Park SH, Ham S, Lee A, Möller A, Kim TS. NLRP3 negatively regulates treg differentiation through Kpna2-mediated nuclear translocation. J Biol Chem (2019) 294(47):17951–61. doi: 10.1074/jbc.RA119.010545
60. Chou WC, Guo Z, Guo H, Chen L, Zhang G, Liang K, et al. AIM2 in regulatory T cells restrains autoimmune diseases. Nature (2021) 591:7849. doi: 10.1038/s41586-021-03231-w
61. Nguyen HD, Chatterjee S, Haarberg KMK, Wu Y, Bastian D, Heinrichs J, et al. Metabolic reprogramming of alloantigen-activated T cells after hematopoietic cell transplantation. J Clin Invest (2016) 126(4):1337–52. doi: 10.1172/JCI82587
62. Thompson SG. Systematic review: Why sources of heterogeneity in meta-analysis should be investigated. BMJ (1994) 309(6965):1351–5. doi: 10.1136/bmj.309.6965.1351
63. Walsh CJ, Hu P, Batt J, Santos CCd, Li K, Shing K. Microarray meta-analysis and cross-platform normalization: Integrative genomics for robust biomarker discovery. Microarrays (Basel) (2015) 4(3):389–406. doi: 10.3390/microarrays4030389
64. Buckberry S, Bianco-Miotto T, Bent SJ, Dekker GA, Roberts CT. Integrative transcriptome meta-analysis reveals widespread sex-biased gene expression at the human fetal-maternal interface. Mol Hum Reprod (2014) 20(8):810–9. doi: 10.1093/molehr/gau035
65. Gao L, Xiong DD, He RQ, Yang X, Lai ZF, Liu LM, et al. MIR22HG as a tumor suppressive lncRNA in HCC: A comprehensive analysis integrating RT-qPCR, mRNA-seq, and microarrays. Onco Targets Ther (2019) 12:9827–48. doi: 10.2147/OTT.S227541
66. Al-Sheyyab A, Binari L, Shwetar M, Ramos E, Kapp ME, Bala S, et al. Association of medication non-adherence with short-term allograft loss after the treatment of severe acute kidney transplant rejection. BMC Nephrol (2019) 20(1). doi: 10.1186/s12882-019-1563-z
67. Pallardó Mateu LM, Sancho Calabuig A, Capdevila Plaza L, Esteve AF. Acute rejection and late renal transplant failure: Risk factors and prognosis. Nephrol Dial Transplant (2004) 19 Suppl 3. doi: 10.1093/ndt/gfh1013
68. Fu Q, Zhang H, Nie W, Deng R, Li J, Xiong Y, et al. Risk factors for acute rejection after deceased donor kidney transplantation in China. Transplant Proc (2018) 50(8):2465–8. doi: 10.1016/j.transproceed.2018.03.063
69. Tseng GC, Ghosh D, Feingold E. Comprehensive literature review and statistical considerations for microarray meta-analysis. Nucleic Acids Res (2012) 40(9):3785–99. doi: 10.1093/nar/gkr1265
70. Ramasamy A, Mondry A, Holmes CC, Altman DG. Key issues in conducting a meta-analysis of gene expression microarray datasets. PloS Med (2008) 5(9):e184. doi: 10.1371/journal.pmed.0050184
71. Kaneko N, Ito Y, Iwasaki T, Takeda H, Sawasaki T, Migita K, et al. Reconstituted AIM2 inflammasome in cell-free system. J Immunol Methods (2015) 426:76–81. doi: 10.1016/j.jim.2015.08.004
72. Honda H, Nagai Y, Matsunaga T, Okamoto N, Watanabe Y, Tsuneyama K, et al. Isoliquiritigenin is a potent inhibitor of NLRP3 inflammasome activation and diet-induced adipose tissue inflammation. J Leukocyte Biol (2014) 96(6):1087–100. doi: 10.1189/jlb.3A0114-005RR
73. Komada T, Muruve DA. The role of inflammasomes in kidney disease. Nat Rev Nephrol (2019) 15(8):501–20. doi: 10.1038/s41581-019-0158-z
74. Eikmans M, Gielis EM, Ledeganck KJ, Yang J, Abramowicz D, Claas FFJ. Non-invasive biomarkers of acute rejection in kidney transplantation: Novel targets and strategies. Front Med (2018) 5(JAN):358. doi: 10.3389/fmed.2018.00358
75. Guzzi F, Cirillo L, Buti E, Becherucci F, Errichiello C, Roperto RM, et al. Urinary biomarkers for diagnosis and prediction of acute kidney allograft rejection: A systematic review. Int J Mol Sci (2020) 21(18):6889. doi: 10.3390/ijms21186889
76. Josephson MA. Monitoring and managing graft health in the kidney transplant recipient. Clin J Am Soc Nephrol (2011) 6(7):1774–80. doi: 10.2215/CJN.01230211
77. Furness PN, Taub N. International variation in the interpretation of renal transplant biopsies: Report of the CERTPAP project. Kidney Int (2001) 60(5):1998–2012. doi: 10.1046/j.1523-1755.2001.00030.x
Keywords: kidney transplant, acute rejection (AR), AIM2 inflammasome, biomarker, bioinformatics, inflammasome
Citation: Franchon Marques Tejada N, Ziroldo Lopes JV, Duarte Gonçalves LE, Mamede Costa Andrade da Conceição I, Franco GR, Ghirotto B and Câmara NOS (2022) AIM2 as a putative target in acute kidney graft rejection. Front. Immunol. 13:839359. doi: 10.3389/fimmu.2022.839359
Received: 19 December 2021; Accepted: 06 September 2022;
Published: 30 September 2022.
Edited by:
Effie Wang Petersdorf, Fred Hutchinson Cancer Research Center, United StatesReviewed by:
Helong Dai, Second Xiangya Hospital, Central South University, ChinaWai Lim, University of Western Australia, Australia
Copyright © 2022 Franchon Marques Tejada, Ziroldo Lopes, Duarte Gonçalves, Mamede Costa Andrade da Conceição, Franco, Ghirotto and Câmara. This is an open-access article distributed under the terms of the Creative Commons Attribution License (CC BY). The use, distribution or reproduction in other forums is permitted, provided the original author(s) and the copyright owner(s) are credited and that the original publication in this journal is cited, in accordance with accepted academic practice. No use, distribution or reproduction is permitted which does not comply with these terms.
*Correspondence: Niels Olsen Saraiva Câmara, bmllbHNAaWNiLnVzcC5icg==; bmllbHMuY2FtYXJhQGdtYWlsLmNvbQ==
†These authors have contributed equally to this work
‡These authors share senior authorship