- 1Department of Thyroid and Breast Surgery, Tongji Hospital, Tongji Medical College, Huazhong University of Science and Technology, Wuhan, China
- 2Department of Breast Surgical Oncology, National Cancer Center/National Clinical Research Center for Cancer/Cancer Hospital, Chinese Academy of Medical Sciences and Peking Union Medical College, Beijing, China
- 3Department of Thyroid & Breast, Burn and Plastic Surgery, Tongren City People’s Hospital, Tongren, China
- 4Center on Smart and Connected Health Technologies, Mays Cancer Center, School of Nursing, University of Texas (UT) Health San Antonio, San Antonio, TX, United States
- 5Melbourne School of Population and Global Health, The University of Melbourne, Melbourne, VIC, Australia
- 6Centre of Cancer Research, Victorian Comprehensive Cancer Centre, Melbourne VIC, Australia
- 7School of Population Medicine and Public Health, Chinese Academy of Medical Sciences and Peking Union Medical College, Beijing, China
Objective: This study aims at investigating the potential prognostic significance of the breast immune prognostic index (BIPI) in breast cancer patients who received neoadjuvant chemotherapy (NACT).
Methods: The optimal cutoff value was calculated through the receiver operating characteristic curve (ROC). The correlations between BIPI and clinicopathologic characteristics were determined by the chi-square test or Fisher’s exact test. The Kaplan–Meier method was used to estimate the survival probability, and the log-rank test was used to analyze the differences in the survival probability among patients. The univariate and multivariate Cox proportional hazard regression model was used to screen the independent prognostic factors. A prognostic nomogram for disease-free survival (DFS) and overall survival (OS) was built on the basis of the multivariate analyses. Furthermore, the calibration curve and decision curve analysis (DCA) were used to assess the predictive performance of the nomogram.
Results: All enrolled patients were split into three subgroups based on the BIPI score. The mean DFS and OS of the BIPI score 0 group and BIPI score 1 group were significantly longer than those of the BIPI score 2 group (42.02 vs. 38.61 vs. 26.01 months, 77.61 vs. 71.83 vs. 53.15 months; p < 0.05). Univariate and multivariate analyses indicated that BIPI was an independent prognostic factor for patients’ DFS and OS (DFS, hazard ratio (HR): 6.720, 95% confidence interval (CI): 1.629–27.717; OS, HR: 8.006, 95% CI: 1.638–39.119). A nomogram with a C-index of 0.873 (95% CI: 0.779–0.966) and 0.801 (95% CI: 0.702–0.901) had a favorable performance for predicting DFS and OS survival rates for clinical use by combining immune scores with other clinical features. The calibration curves at 1-, 3-, and 5-year survival suggested a good consistency between the predicted and actual DFS and OS probability. The DCA demonstrated that the constructed nomogram had better clinical predictive usefulness than only BIPI in predictive clinical applications of 5-year DFS and OS prognostic assessments.
Conclusions: The patients with low BIPI score have better prognoses and longer DFS and OS. Furthermore, the BIPI-based nomogram may serve as a convenient prognostic tool for breast cancer and help in clinical decision-making.
Introduction
Breast cancer (BC) is a fatal disease—it is the most common female malignancy and the primary cause of cancer-related death worldwide (1). Although the prognosis of breast cancer is relatively satisfactory in contrast to other tumors, such as gastrointestinal tumor and lung cancer, the survival outcome of patients with advanced breast cancer or with distant metastasis is still very poor (2). Moreover, more than half of breast cancer deaths are caused by distant metastasis (2). In the past, chemotherapy is the main treatment for advanced breast cancer or recurrent breast cancer (3). Over the past few decades, a great deal of molecular target drugs, for instance, monoclonal HER2-targeting antibodies (trastuzumab and pertuzumab) and antibody-drug conjugates (ADCs) (trastuzumab deruxtecan and trastuzumab emtansine), had been approved for the treatment of HER2-positive breast cancer or metastatic breast cancer (4, 5). Furthermore, immunotherapy (immune-checkpoint inhibitors) has been the focus of attention, and its effectiveness in the treatment of breast cancer has been reported (6, 7). The emergence and rise of these therapeutic agents have significantly improved the treatment of breast cancer.
Recently, some oncologists begin to focus on antitumor immune responses, which may become fundamental markers in cancer immunotherapy (8). Immune checkpoint inhibitors (ICIs), such as programmed cell death 1 (PD-1), programmed cell death ligand 1 (PD-L1), and cytotoxic T lymphocyte antigen 4 (CTLA-4), have indicated remarkable improvement in the prognosis for the treatment of dozens of cancers (9). However, mixed findings are also present in the immunotherapy literature, with the most noticeable one being that substantial heterogeneity in response is observed among different tumors (10). To address this issue, potential predictive biomarkers such as gene signatures and multi-omics have been used to further evaluate the prognosis of different tumors (11). However, obtaining and analyzing these biomarkers are often time-consuming, inconvenient, and expensive, which in turn could limit their clinical applications. Hence, it is necessary to develop effective and efficient indicators to evaluate the effect of immune status on the prognosis for breast cancer patients.
A systemic immune and inflammatory status in the body is of importance in cancer prognosis (12). The peripheral blood biomarkers representing inflammation and tumor burden have been increasingly studied in order to predict the treatment effect for breast cancer (13). Currently, some reports have shown that the neutrophil to lymphocyte ratio (NLR), monocyte to lymphocyte ratio (MLR), platelet to lymphocyte ratio (PLR), prognostic nutritional index (PNI), systemic immune inflammation index (SII), and systemic inflammation response index (SIRI) were used to reflect the patients’ immune and inflammatory status (14–19). Furthermore, a derived score composed of the white cell and neutrophil counts which are divided by absolute white cell count minus absolute neutrophil count (dNLR) is similar to NLR and can evaluate the prognosis of tumors (20). The baseline serum lactate dehydrogenase (LDH) level is also an independent prognostic factor for evaluating the survival outcomes in different cancer types, such as non-small cell lung cancer (NSCLC), metastatic melanoma, and colorectal cancer (21–23). Furthermore, the immune prognostic index (IPI) based on the LDH and the dNLR level can also help clinicians to examine and evaluate the prognosis in NSCLC (24). Moreover, the IPI stratified patients into poor, intermediate, and good prognostic groups to further improve the breast cancer diagnostic procedure (25). However, due to lack of research insights, whether the IPI is useful for the prognosis of breast cancer remains unclear, especially in breast cancer patients who received neoadjuvant chemotherapy (NACT). Therefore, to bridge the research gap, in the present study, we aim to gain insights into the clinical prognostic significance of the breast immune prognostic index (BIPI) as a useful prognostic factor in breast cancer patients undergoing NACT.
Methods
Ethics Approval and Consent to Participate
The present study was retrospectively conducted and approved by the institutional review board of the Cancer Hospital Chinese Academy of Medical Sciences in China. All processes performed in the study were conducted in accordance with the standards of the institutional research committee and with the declaration of 1964 Helsinki as well as its later amendments or comparable ethical standards. Individual patient information has been protected and not been shown.
Study Population and Data Collection
We conducted a retrospective study of breast cancer undergoing NACT diagnosed and treated at the Cancer Hospital Chinese Academy of Medical Sciences between June 2009 and December 2015. Using the electronic medical records, we collected and searched the clinical and demographic data on every patient.
Inclusion Criteria and Exclusion Criteria
Participants who met the following inclusion criteria were included in the study: 1) all enrolled breast patients who received NACT; 2) pathologically confirmed breast cancer, and underwent surgery after NACT; 3) no preoperative antitumor therapy or anti-infection treatments; 4) complete follow-up information and available clinical data; and 5) peripheral blood samples collected before treatment. The patients were excluded in the study if they have the following: 1) lack of clear and definite pathological diagnosis and medical history information; 2) with other malignant tumors except breast cancer or with distant metastasis; 3) suffer from autoimmune diseases or chronic inflammatory; and 4) with history of blood transfusion before treatment.
Calculation of the Breast Immune Prognostic Index
The BIPI was an indicator that combined the LDH level and the dNLR level. The dNLR was defined as neutrophil count/(white blood cell count – neutrophil count). The dNLR had been recently identified as a prognostic factor of immune checkpoint inhibitor therapy (26). The optimal cutoff values of LDH and dNLR were assessed by the ROC curve (Figure S1). The optimal cutoff values of LDH and dNLR were 203.5 U/l (range: 105–715 U/l) and 1.67 (range: 0.07–4.36), respectively. Moreover, all patients were assigned to three groups: 1) BIPI score 0 (Good): LDH < 203.5 U/l and dNLR < 1.67; 2) BIPI score 1 (Intermediate): LDH ≥203.5 U/l and dNLR < 1.67, or LDH < 203.5 U/l and dNLR ≥ 1.67; and 3) BIPI score 2 (Poor): LDH ≥203.5 U/l and dNLR ≥ 1.67. According to the BIPI score, 43 (41.3%), 46 (44.2%), and 15 14.4%) breast cancer patients were classified into the BIPI score 0 group, BIPI score 1 group, and BIPI score 2 group, respectively.
Follow-Up
All enrolled patients had routine inpatient, outpatient, and/or telephone follow-up after operation. Follow-up evaluations were performed every 3 months for the first to the second year, every 6 months for the third to the fifth year, and then yearly thereafter. Disease-free survival (DFS) was defined as the time lapsed from surgery to progression with regard to the distant disease metastasis, death from any cause, or last follow-up. Overall survival (OS) was defined as the time lapsed from surgery to the date of death from any cause or last follow-up.
Statistical Analysis
The baseline characteristics data were presented as absolute value and percentage (%), compared between groups using the chi-square test or Fisher’s exact test. The optimal cutoff value was calculated using the receiver operating characteristic curve (ROC). The Kaplan–Meier method was used to estimate the survival probability, and the log-rank test was used to compare survival distributions of the individual index level. The univariate and multivariate Cox proportional hazard regression model was used to evaluate the independent prognostic factors. The hazard ratios (HRs) and 95% confidence intervals (CIs) were performed to evaluate the association between the clinicopathological data. The prognostic nomogram for DFS and OS was established on the multivariate analyses. The calibration curve and decision curve analysis (DCA) were further used to assess the predictive performance. All statistical analyses were performed using the SPSS software (version 17.0; SPSS Inc., Chicago, IL, USA), GraphPad Prism software (version 8.0; GraphPad Inc., La Jolla, CA, USA), and R (version 3.6.0; Vienna, Austria. URL: http://www.R-project.org/). Alpha was set at the 0.05 level, and a two-tailed p < 0.05 indicated statistical significance.
Results
Patients’ General Characteristics in the Study
A total of 104 breast cancer patients who received NACT were included in the present study. The median age was 46 years (range from 27 to 73 years). On the basis of the eighth edition of the TNM classification, 3 (2.9%), 39 (37.5%), and 62 (59.6%) breast cancer patients before treatment were classified as stages I, II, and III, respectively. After operation, 2 (1.9%), 16 (15.4%), 38 (36.5%), and 48 (46.2%) breast cancer patients were classified as stages Tis/T0, I, II, and III, respectively. In terms of ABO blood type, A type was 28 cases (26.9%), B type was 34 cases (32.7%), O type was 28 cases (26.9%), and AB type was 14 cases (13.5%). The PD-1 and PD-L1 protein expressions in tumor cells by immunohistochemistry (IHC) assay were 38 cases with PD-1 low expression, 66 cases with PD-1 high expression, 61 cases with PD-L1 low expression, and 43 cases with PD-L1 high expression, respectively. BIPI was associated with type of surgery (p = 0.032). The clinical characteristics are summarized in Table 1.
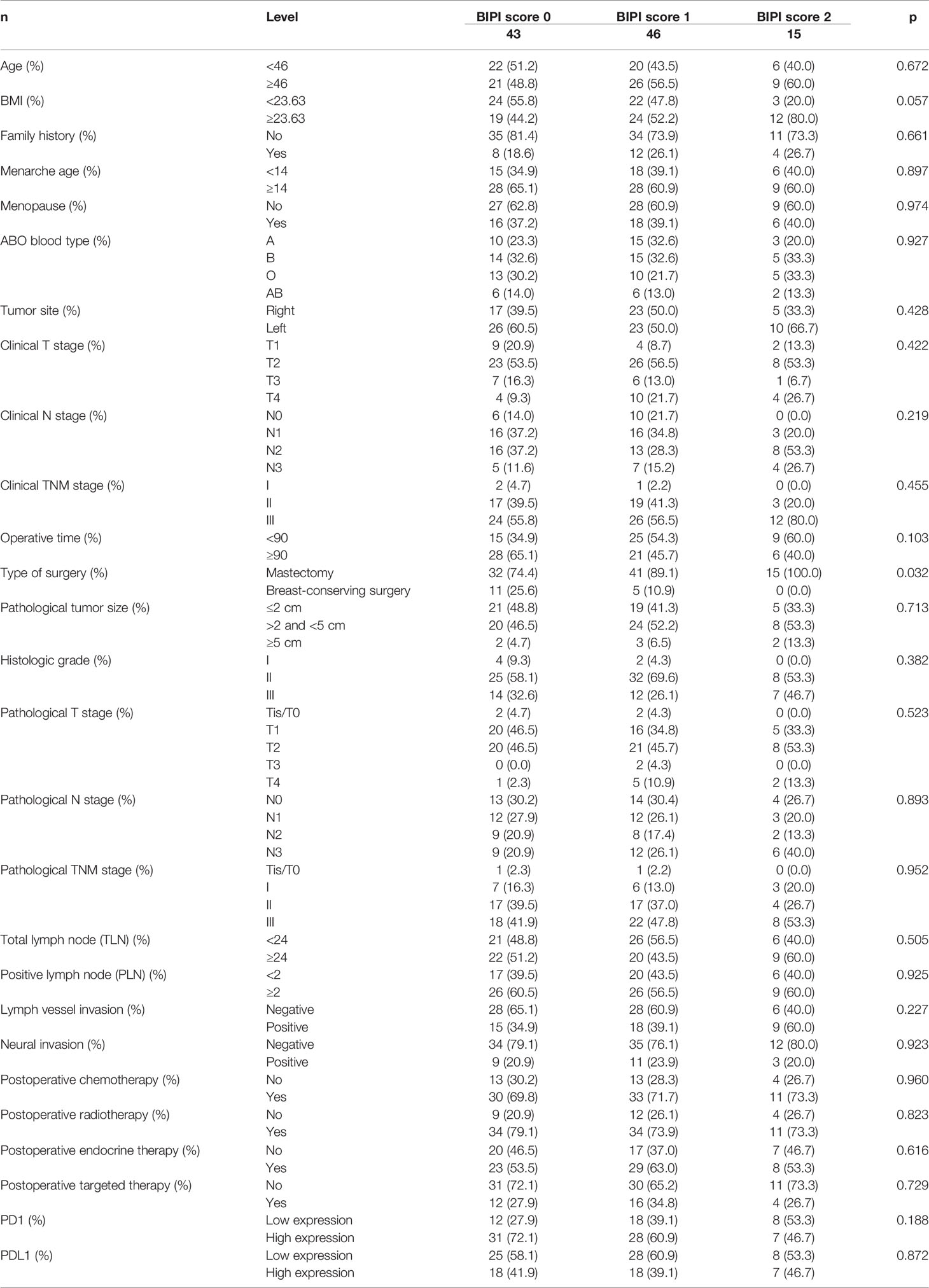
Table 1 Patients’ characteristics for all patients in accordance with breast immune prognostic index (BIPI).
Association Between BIPI and the Patients’ Pathology Parameters in the Study
There were 8 patients (7.7%) with Luminal A type, 14 patients (13.5%) with Luminal B HER2 (+) type, 35 patients (33.7%) with Luminal B HER2 (-) type, 15 patients (14.4%) with HER2-enriched type, and 32 patients (30.8%) with triple-negative type before NACT. Moreover, 17 patients (16.3%) with Luminal A type, 9 patients (8.7%) with Luminal B HER2 (+) type, 23 patients (22.1%) with Luminal B HER2 (-), 18 patients (17.3%) with HER2-enriched type, and 37 patients (35.6%) with triple-negative type after operation. BIPI was associated with P53 (p = 0.010). Detailed information is shown in Table 2.
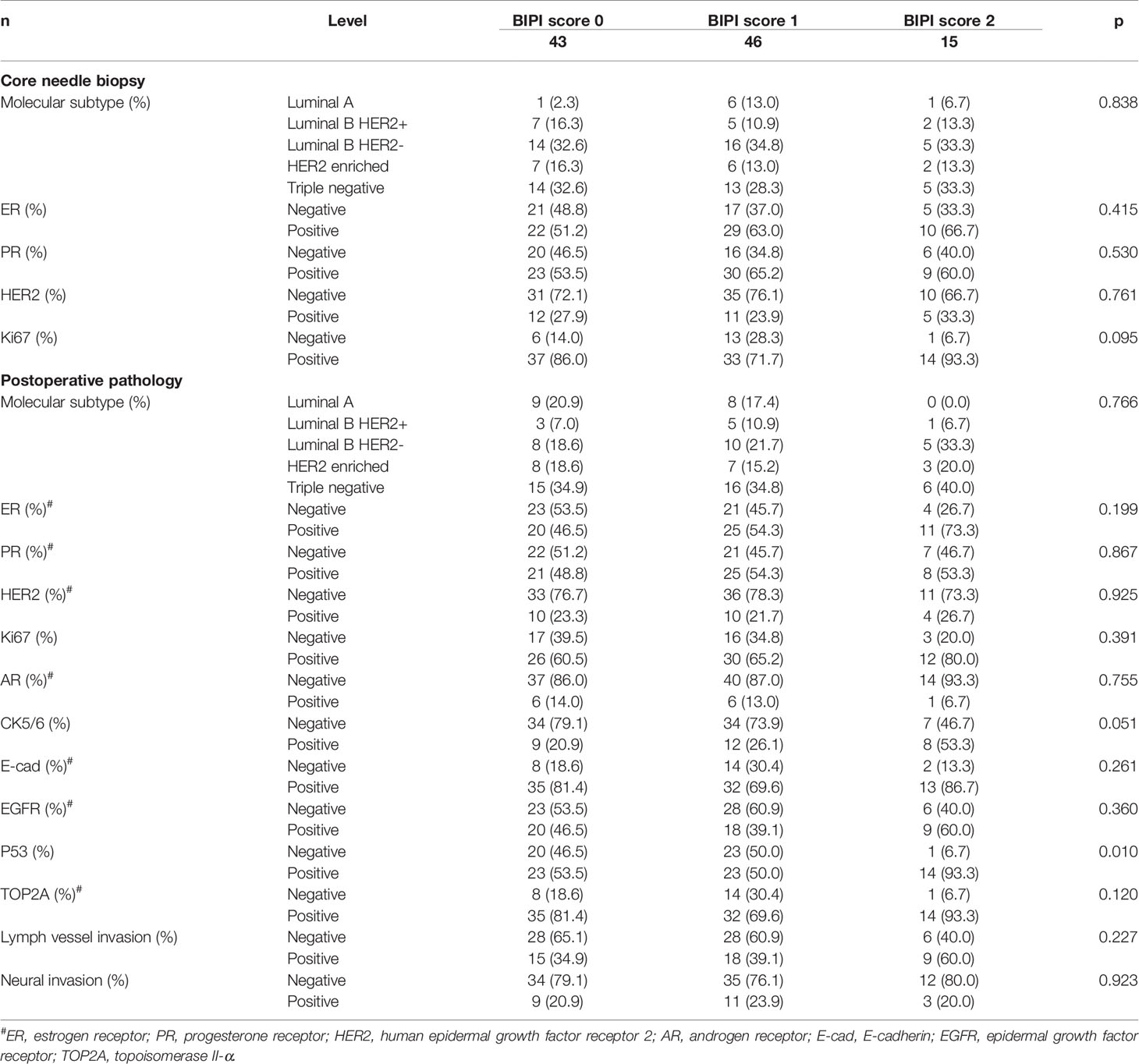
Table 2 Patients’ pathology parameters for all patients in accordance with breast immune prognostic index (BIPI).
Association Between BIPI and the Patients’ Chemotherapy in the Study
Based on the RECIST guidelines, there were 60 patients (57.7%) with partial responses (PRs), 43 patients (41.3%) with stable disease (SD), and one patient (1.0%) with progressive disease (PD) after two chemotherapy cycles. According to Miller–Payne grade (MPG), there were 9 cases (8.7%) with MPG 1, 42 cases (40.4%) with MPG 2, 48 cases (46.2%) with MPG 3, one case (1.0%) with MPG 4, and 4 cases (3.8%) with MPG 5. However, no significant correlations between BIPI and the patients’ chemotherapy were found (p > 0.05). Detailed information is shown in Table 3.
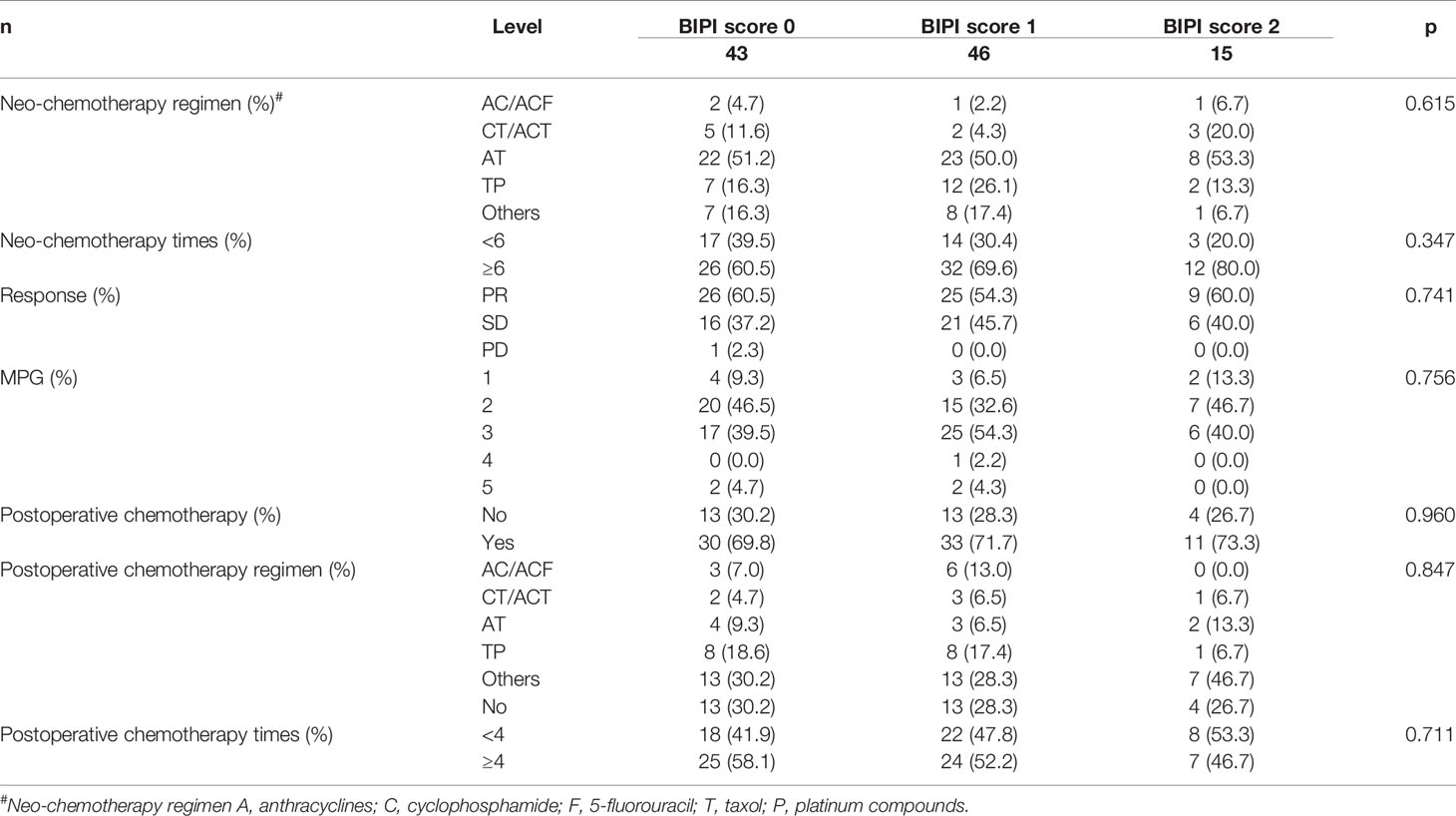
Table 3 Patients’ chemotherapy for all patients in accordance with breast immune prognostic index (BIPI).
Association Between BIPI and the Patients’ Side Effects of Chemotherapy in the Study
The hematologic reactions and gastrointestinal reactions myelosuppression and hepatic dysfunction were the common adverse events (AEs) among the NACT treatment. Nevertheless, no significant correlations between BIPI and side effects of chemotherapy were found (p > 0.05). Detailed information is shown in Table 4.
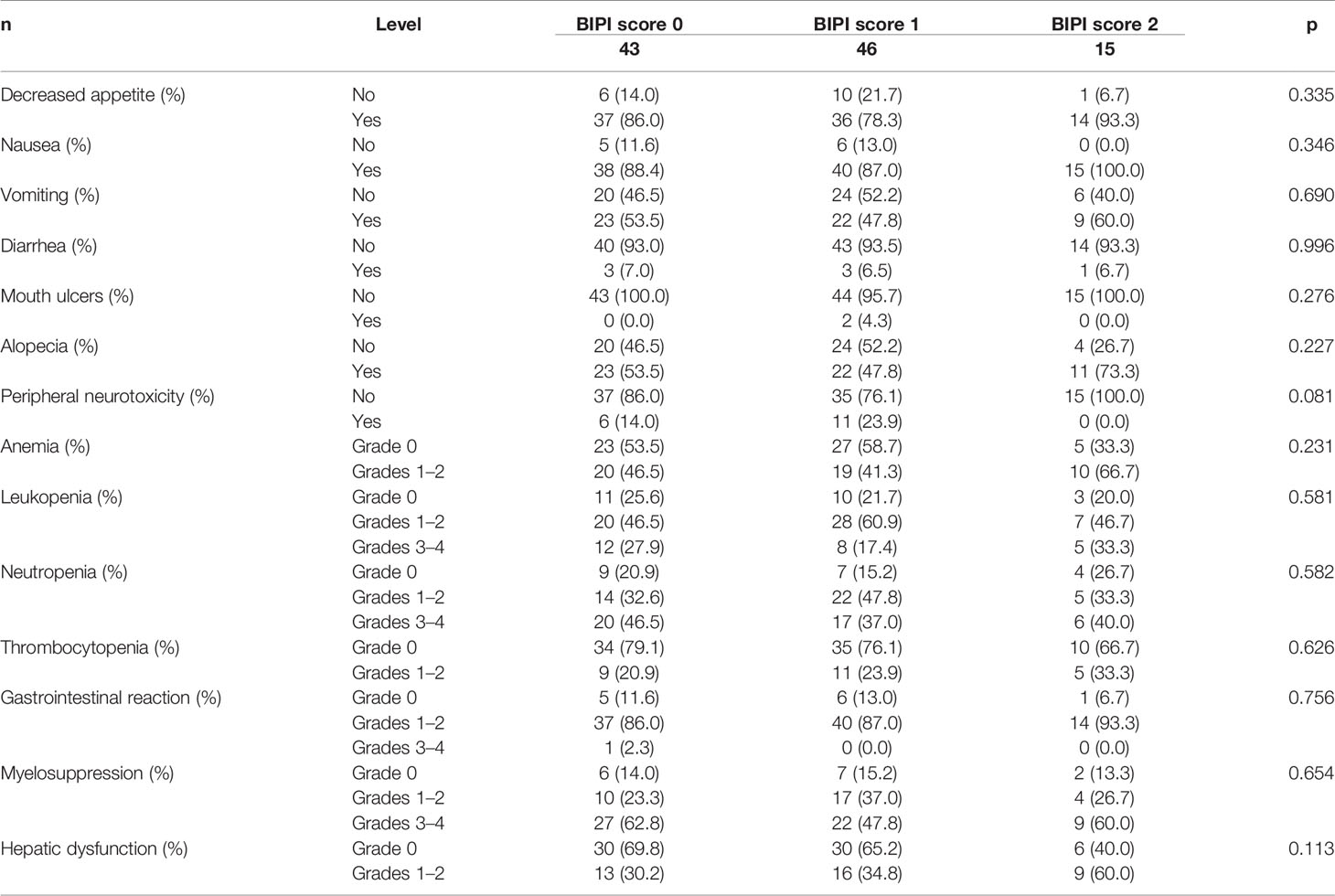
Table 4 Patients’ side effects of chemotherapy for all patients in accordance with breast immune prognostic index (BIPI).
Univariate and Multivariate Analyses for DFS and OS
The univariate analysis indicated that LDH, dNLR, BIPI, PD-L1, ABO blood type, pathological N stage, total lymph node (TLN), PR, Ki67, CK5/6, E-cadherin (E-cad), postoperative chemotherapy, postoperative endocrine therapy, and postoperative targeted therapy were associated with the prognosis of breast cancer patients for DFS; however, the multivariate analysis found that only LDH, BIPI, PD-L1, ABO blood type, PR, E-cad, postoperative chemotherapy, postoperative endocrine therapy, and postoperative targeted therapy were the independent prognostic factors for DFS (Table 5). Moreover, the results were displayed using forest plots and are shown in Figure S2A.
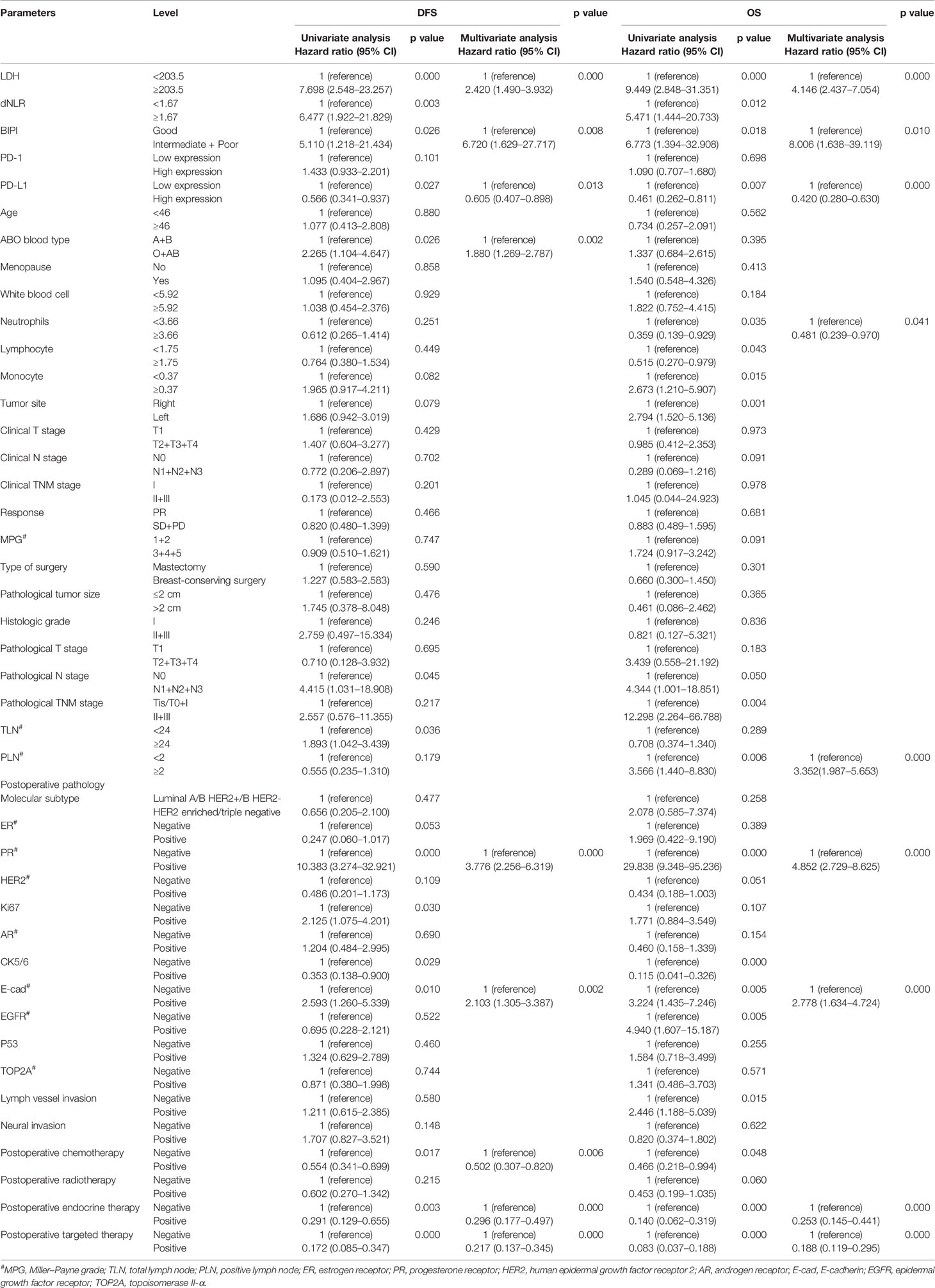
Table 5 Univariate and multivariate cox proportional hazard regression model for disease-free survival (DFS) and overall survival (OS).
Moreover, the univariate analysis showed that LDH, dNLR, BIPI, PD-L1, neutrophils, lymphocyte, monocyte, tumor site, pathological N stage, pathological TNM stage, positive lymph node (PLN), PR, CK5/6, E-cad, epidermal growth factor receptor (EGFR), lymph vessel invasion (LVI), postoperative chemotherapy, postoperative endocrine therapy, and postoperative targeted therapy were associated with the prognosis of breast cancer patients for OS; however, the multivariate analysis found that only LDH, BIPI, PD-L1, neutrophils, PLN, PR, E-cad, postoperative endocrine therapy, and postoperative targeted therapy were the independent prognostic factors for OS (Table 5). Moreover, the results were displayed using forest plots and are shown in Figure S2B. BIPI was an independent prognostic factor for patients’ DFS and OS (DFS, hazard ratio (HR): 6.720, 95% confidence interval (CI): 1.629–27.717; OS, HR: 8.006, 95% CI: 1.638–39.119).
Survival Analysis
The mean DFS was 42.02 months (range from 6.33 to 107.77 months) in the BIPI score 0 group, 38.61 months (range from 4.67 to 101.30 months) in the BIPI score 1 group, and 26.01 months (range from 6.23 to 56.77 months) in the BIPI score 2 group, respectively. Overall, significant differences were found when comparing the three BIPI groups (p < 0.001). Moreover, the mean DFS of the BIPI score 0 group and BIPI score 1 group was significantly longer than that of the BIPI score 2 group (p = 0.007, and p = 0.025), respectively (Figure 1A). Furthermore, the mean OS was 77.61 months (range from 6.43 to 148.03 months) in the BIPI score 0 group, 71.83 months (range from 14.47 to 137.90 months) in the BIPI score 1 group, 53.15 months (range from 10.77 to 93.00 months) in the BIPI score 2 group, respectively. Analyses showed that there was a significant difference among the three groups compared (p < 0.001). Moreover, the mean OS of the BIPI score 0 group and BIPI score 1 group was significantly longer than that of the BIPI score 2 group (p = 0.011, and p = 0.041), respectively (Figure 1B).
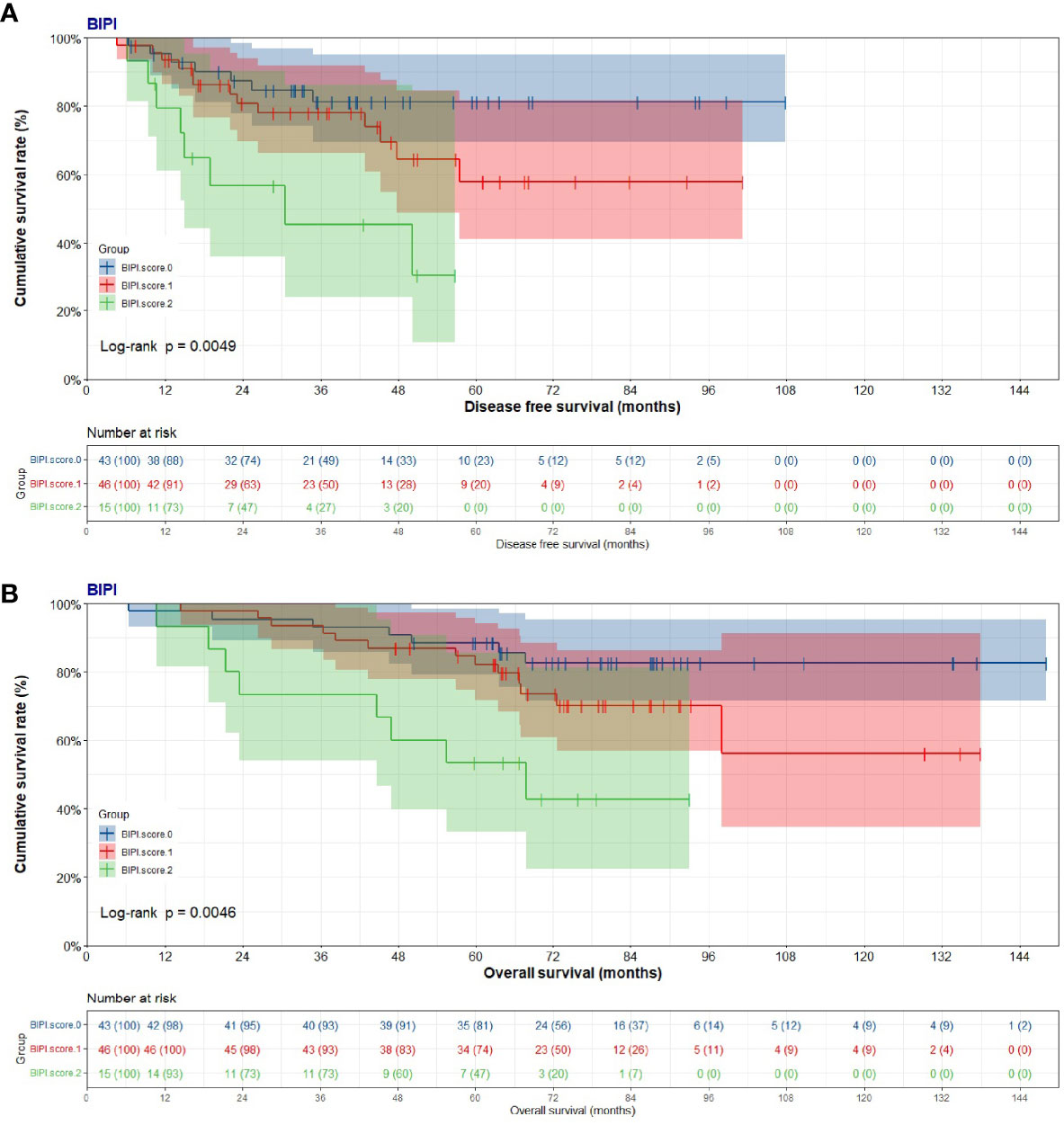
Figure 1 Kaplan–Meier curves for disease-free survival (DFS) and overall survival (OS). (A) Kaplan–Meier curves for DFS for breast immune prognostic index (BIPI). (B) Kaplan–Meier curves for OS for breast immune prognostic index (BIPI).
According to the pretreatment optimal cutoff values of LDH and dNLR, we also analyzed the survival. A total of 83 cases (79.8%) had LDH <203.5 U/l, and 21 (20.2%) had LDH ≥203.5 U/l. Compared with the two groups, patients with low LDH had longer DFS and OS than those with high LDH (p = 0.018, and p = 0.011) (Figure S3A). A total of 49 cases (47.1%) had dNLR <1.67, and 55 cases (52.9%) had dNLR ≥1.67. Compared with the two groups, patients with low dNLR had longer DFS and OS than those with high dNLR (p = 0.039, and p = 0.043) (Figure S3B).
Establishment and Validation of the Nomogram
According to the results of the univariate and multivariate Cox proportional hazard model, we constructed an effective and novel nomogram for the individualized assessment of DFS and OS after NACT and operation. In the nomogram, variables were imputed into weighted points, the sum of which was subsequently utilized to predict the 1-, 3-, and 5-year survival probabilities for DFS, and 1-, 3-, 5-, and 10-year survival probabilities for OS. A higher patient grade is associated with a lower survival probability. The nomogram for DFS had unique features, and integrated LDH, BIPI, PD-L1, ABO blood type, PR, E-cad, postoperative chemotherapy, postoperative endocrine therapy, and postoperative targeted therapy; it was generated as shown in Figure 2A. Moreover, the nomogram for OS had unique features, and integrated LDH, BIPI, PD-L1, neutrophils (N), PLN, PR, E-cad, postoperative endocrine therapy, and postoperative targeted therapy; it was generated as shown in Figure 2B. A nomogram with a C-index of 0.873 (95% CI: 0.779–0.966) and 0.801 (95% CI: 0.702–0.901) had a favorable performance for predicting DFS and OS survival rates for clinical use by combining immune scores with other clinical features. Moreover, we also conducted the dynamic nomogram, and the results are as shown in Figures S4A, B.
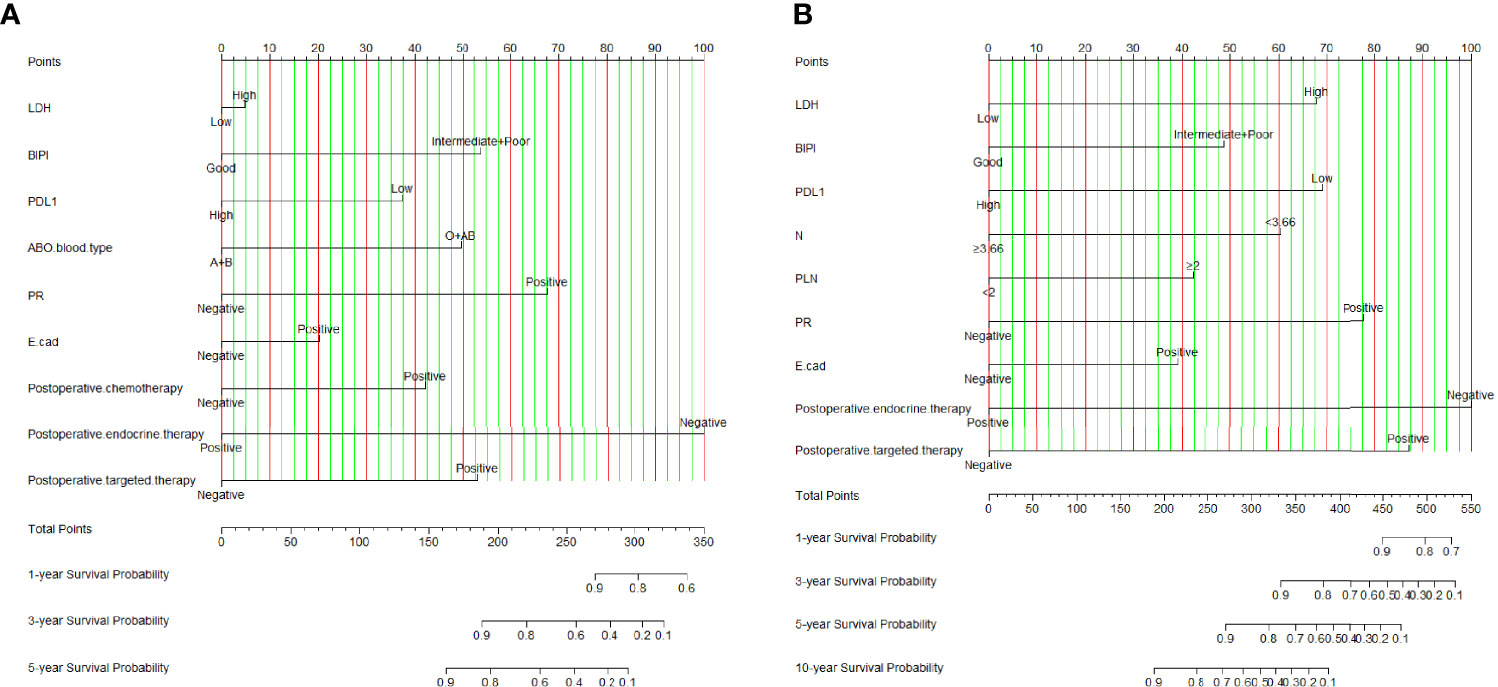
Figure 2 Breast immune prognostic index (BIPI)-based nomogram for predicting disease-free survival (DFS) and overall survival (OS). A straight upward line is drawn to determine the points for every predictor. The sum of these points is situated on the total points axis, and a straight downward line shows the 1-, 3-, and 5-year DFS estimated rates and 1-, 3-, 5-, and 10-year OS estimated rates. (A) BIPI-based nomogram for predicting disease-free survival (DFS). (B) BIPI-based nomogram for predicting and overall survival (OS). E-cad, E-cadherin; N, neutrophils; PLN, positive axillary lymph node.
Furthermore, the calibration curves (1,000 bootstrap resamples) were used to assess the performance of the nomogram for the predicted and the actual probability of DFS and OS. The prediction line matched the reference line well for postoperative 1-, 3-, and 5-year survival DFS, which was an indication of good performance of the nomogram, especially for the 5-year DFS category (Figures 3A–C). Moreover, the prediction line matched the reference line well for postoperative 1-, 3-, 5-, and 10-year survival OS, showing good performance of the nomogram, especially in 3-year OS (Figures 3D–F). However, the prediction line matched the reference line not well for postoperative 10-year survival OS (Figure 3G).
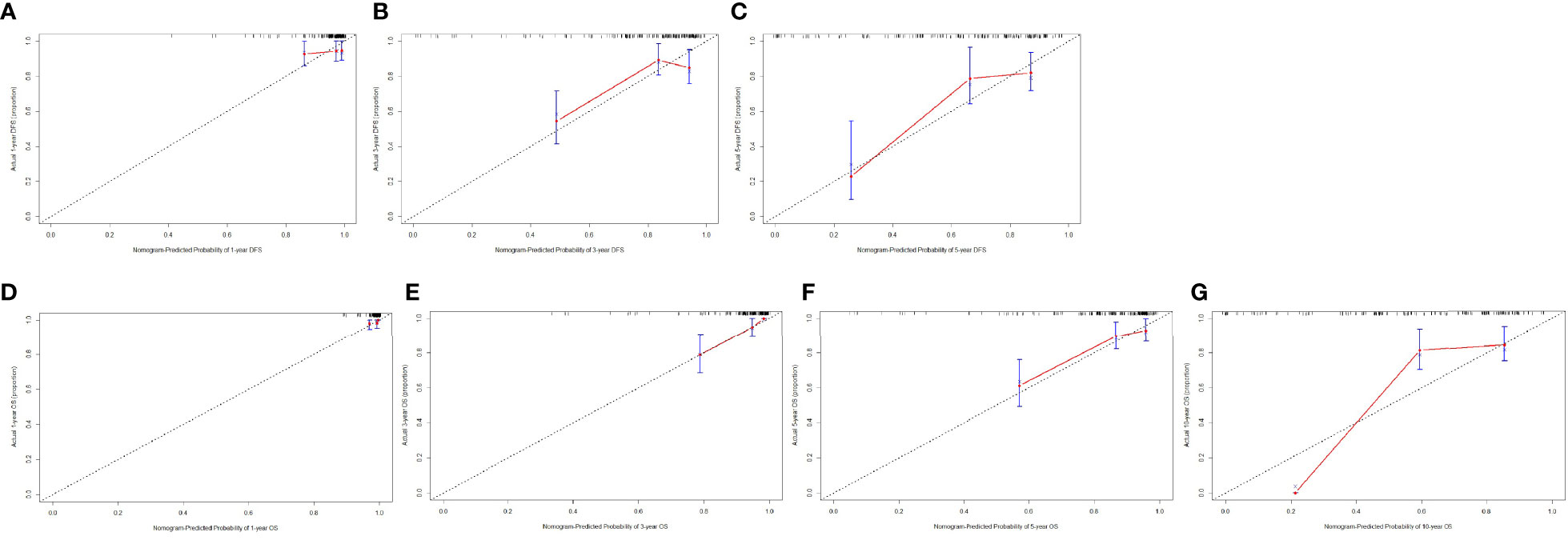
Figure 3 The calibration curves for predicting the 1-, 3-, and 5-year DFS rates and 1-, 3-, 5-, and 10-year OS rates. The X-axis presents the nomogram-predicted probability of disease-free survival (DFS) and overall survival (OS), and the Y-axis shows the actual DFS and OS. (A) The calibration curves for predicting the 1-year DFS rate in patients with breast cancer. (B) The calibration curves for predicting the 3-year DFS rate in patients with breast cancer. (C) The calibration curves for predicting the 5-year DFS rate in patients with breast cancer. (D) The calibration curves for predicting the 1-year OS rate in patients with breast cancer. (E) The calibration curves for predicting the 3-year OS rate in patients with breast cancer. (F) The calibration curves for predicting the 5-year OS rate in patients with breast cancer. (G) The calibration curves for predicting the 10-year OS rate in patients with breast cancer.
Comparison of Predictive Accuracy for DFS and OS Between Nomogram and BIPI by DCA
We used DCA to evaluate the clinical utility between the nomogram and BIPI by quantifying the net benefits at different threshold probabilities. A higher threshold probability represented better estimation for decision outcomes. Compared with BIPI, the nomogram model yielded the best net benefit across in the range of threshold probability for 5-year DFS and OS, indicating that its ability for clinical decision-making was better than only BIPI (Figure 4). The blue line represented BIPI with other independent prognostic factors by the COX proportional hazard regression model, and the green line represented only BIPI. Moreover, compared with the two lines, the blue line was obviously higher than the green line, which meant that the BIPI with other independent prognostic factors which were used to evaluate the prognosis showed better performance than only BIPI. Moreover, we also analyzed the clinical utility between BIPI and other factors (pathological N stage, pathological TNM stage) by DCA. The DCA demonstrated that the BIPI nomogram displayed better clinical predictive usefulness than the pathological N stage or pathological TNM stage alone (Figure S5).
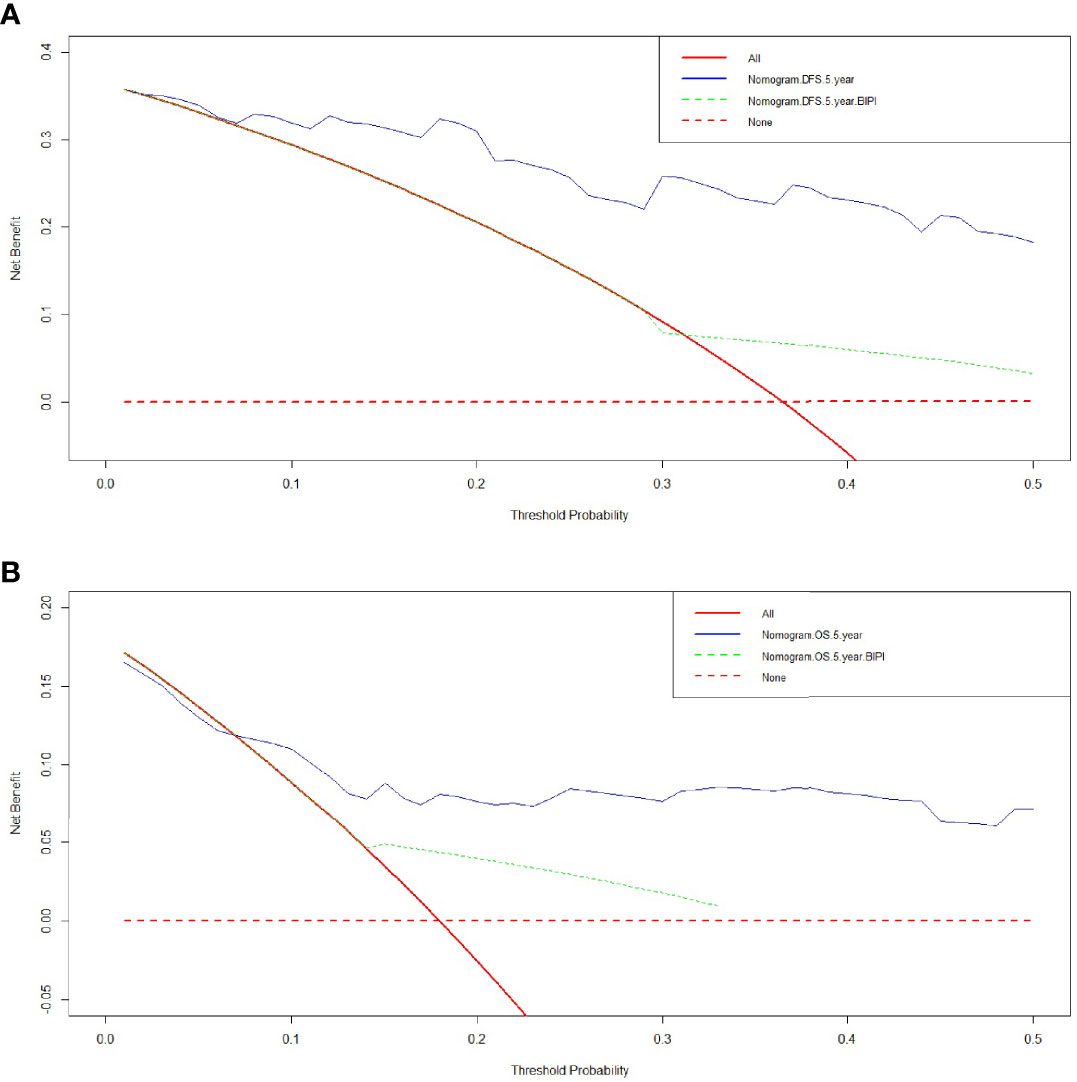
Figure 4 Decision curve analysis (DCA) of the nomogram and breast immune prognostic index (BIPI) for predicting the disease-free survival (DFS) and overall survival (OS). The X-axis represents the threshold probability, and the Y-axis shows the net benefit. The lines between the X-axis and the Y-axis display the benefit of different predictive variables. The red dotted line suggests that no patient has poor prognosis, while the red line indicates that all patients have poor prognosis. The blue line represents BIPI with other independent prognostic factors by the COX proportional hazard regression model, and the green line represents only BIPI. (A) DCA of the nomogram and BIPI for predicting the 5-year DFS. (B) DCA of the nomogram and BIPI for predicting the 5-year OS.
Discussion
This study aimed at investigating the potential prognostic significance of BIPI in breast cancer patients who received NACT to address research gaps in the literature. The present study demonstrated for the first time that BIPI was an independent prognostic factor by univariate and multivariate analyses for DFS and OS. According to the optimal cutoff values of LDH and dNLR, the BIPI was classified into three groups: BIPI score 0 (Good), BIPI score 1 (Intermediate), and BIPI score 2 (Poor). Overall, significant differences were found when comparing the three BIPI groups. Moreover, the mean DFS and OS of the BIPI score 0 group and BIPI score 1 group were significantly longer than those of the BIPI score 2 group. In order to more accurately predict the survival of breast cancer patients, we constructed a prognostic nomogram based on the independent prognostic factors by the Cox proportional hazard model. The nomogram predicts the 1-, 3-, and 5-year survival probabilities. Moreover, the prediction line matches the reference line well for postoperative survival DFS and OS survival by calibration curves, especially in 5-year DFS and 3-year OS. Furthermore, the nomogram model produced the best net benefit across in the range of threshold probability for 5-year DFS and OS than only by BIPI and could effectively provide the clinical decision-making for breast cancer.
Although the clinical application of multimodal treatments has been advancing, including surgery, chemotherapy, radiotherapy, and immunotherapy, the treatment and prognosis of advanced breast cancer are still not satisfactory (27). Hence, looking for the optimal individualized treatment and providing the appropriate prognostic indicators for breast cancer have become a research hotspot. In recent years, more attention has been paid to the immune response status in tumor cells, such as PD-1 and PD-L1, for mediating tumor progression and metastasis (28). It is well known that abnormal immune surveillance and immune escape of tumor cells play a critical role in affecting antitumor immune response and carcinogenesis (29, 30). Furthermore, the tumor immune microenvironment (TIME), including immune cells and inflammatory cells, influences the prognosis and effectiveness of treatment (31). Nevertheless, not all patients with a positive expression of PD-1 and PD-L1 can benefit from immunotherapy (32). Inflammation not only is of vital importance at different stages of tumor development and progression but also may have a negative or positive impact on tumor treatment response and immune monitoring (33, 34). It is also unknown whether combining BIPI and PD-L1 expression can lead to better prognoses for breast cancer patients. Therefore, accurate prognostic evaluation is an important prerequisite for the selection of appropriate treatments.
The BIPI score is composed of two values, namely, the levels of LDH and dNLR in the peripheral blood. The LDH is an enzyme responsible for the conversion of pyruvate to lactic acid during glycolysis, coded by two different genes LDH-A and LDH-B, and five isozymes (LDH1 to LDH5) with selective distribution among different tissues in serum are constructed (35, 36). LDH is a marker of inflammation, hemolysis, tissue injury, and myocardial infarction (37). Furthermore, it is elevated in many types of cancers as a potential diagnostic marker and has been linked to tumor growth, maintenance, and invasion (38). The dNLR has also been reported as a novel potential biomarker associated with different types of malignant tumors (39, 40). Moreover, a combination of the two parameters as an immune prognostic index (IPI)-based scoring system was used to evaluate the prognosis in various cancers, such as NSCLC (41). In Meyers’ study, the lung immune prognostic index (LIPI) correlates with survival outcomes in patients with NSCLC treated with immune checkpoint inhibitors (ICIs), and the intermediate and poor LIPI were independently prognostic of OS compared to good LIPI (42).
There are several plausible mechanisms to evaluate the relationship between BIPI and the prognosis of tumors. Hypoxia-inducible factor 1 (HIF-1) can be activated by the glycolytic metabolites, and it further upregulates angiogenic factors, leading to a feedforward stimulatory loop in cancer cells (43). Moreover, LDH is an enzyme involved in anaerobic glycolysis and gluconeogenesis, regulated by key oncogenic processes, such as phosphatidylinositol 3-kinase (PI3K), the target of rapamycin (TOR) kinase, and tumor hypoxia and necrosis (44). Hence, LDH is linked to angiogenesis and cancer progression, also depending on nutrient availability. Furthermore, LDH-A is very important in c-MYC-mediated cell transformation, and LDH-B is also critical in m-TOR-mediated tumorigenesis (45, 46). In recent clinical trials, serum LDH is a predictor of worse survival in diffuse large B-cell lymphoma (DBCL), advanced or metastatic breast cancer, and hepatocellular carcinoma (HCC) (47–49). Peripheral venous blood analysis can reveal the condition of the immune system. Available evidence has indicated that systemic inflammation is related to the prognosis of tumors and contributes to the pathogenesis and progression of cancers (50, 51). The dNLR was calculated by white blood cell and neutrophil. It is critical that neutrophil is the first line of human defense against infection and responds to different inflammatory signals (52). The neutrophil is an indicator of immune response and inflammatory and is involved in almost every stage of tumorigenesis and paradoxically shows antitumor and pro-tumor characteristics (53). The neutrophil also interacted with immune cells in the tumor microenvironment (TME) and peripheral blood (54). Furthermore, several studies also indicate that dNLR is an inflammation marker that can predict and reflect the prognosis of systematic inflammation in different types of tumors, for instance, non-colorectal gastrointestinal cancer and non-small cell lung cancer (NSCLC) (55, 56).
However, the present study had several limitations. First, this study is a retrospective study with a relatively small sample of breast cancer patients. Second, due to the presence of the eligibility criteria, the selection bias is difficult to eliminate. Third, while in line with the scope of the research question raised, some potential critical parameters associated with clinical prognosis have not been evaluated in the study, and the constructed nomogram was assessed by limited independent factors. Finally, as BIPI is a non-specific tumor marker, further study should consider further examining the correlation between BIPI and cancer prognosis in a prospective study.
Conclusion
BIPI is found to be a significant prognostic factor and predictive biomarker for breast cancer patients. Patients with low immune scores are significantly related to better DFS and OS. Moreover, a novel nomogram based on immune scores may serve as a prognostic stratification tool to promote clinical decision-making.
Data Availability Statement
The raw data supporting the conclusions of this article will be made available by the authors, without undue reservation.
Ethics Statement
This study was approved by the ethics committee of Cancer Hospital Chinese Academy of Medical Sciences. The patients/participants provided their written informed consent to participate in this study.
Author Contributions
Writing—original draft and writing—review and editing: LC, SH, and XK. Formal analysis: LC and XK. Data curation and investigation: LC and ZS. Methodology and supervision: YF and LZ. Resources, funding acquisition, and project administration: XL and JW. All authors contributed to the article and approved the submitted version.
Funding
The work is partly supported by research grants from the National Nature Science Foundation of China (No. 81872160, No. 82072940, No. 82103047, No. 82102887, and No. 81802676), the Beijing Nature Science Foundation of China (No. 7191009, No. 7204293), the National Key R&D Program of China (No. 2018YFC1312100), the China National Key R&D (or Research and Development) Program (Nos. 2020AAA0105000 and 2020AAA0105004), the Special Research Fund for Central Universities, Peking Union Medical College (No. 3332019053), the Beijing Hope Run Special Fund of Cancer Foundation of China (No. LC2020L01, No. LC2019B03, No. LC2019L07), the Wuhan Youth Cadre Project (2017zqnlxr01 and 2017zqnlxr02), the Clinical Research Physician Program of Tongji Medical College, HUST (5001540018), the Golden Bridge Project Seed Fund of Beijing Association for Science and Technology (No. ZZ20004), the Chinese Young Breast Experts Research project (No. CYBER-2021-005), the 2021 Chaoyang District Social Development Science and Technology Plan Project (Medical and Health Field) (No. CYSF2115), the Beijing Xisike Clinical Oncology Research Foundation (No. Y-Young2021-0017), and the XianSheng Clinical Research Special Fund of China International Medical Foundation (No. Z-2014-06-2103).
Conflict of Interest
The authors declare that the research was conducted in the absence of any commercial or financial relationships that could be construed as a potential conflict of interest.
The reviewer AL declared a shared affiliation with the authors LC, XK, YF, MZ, and JW at the time of review.
Publisher’s Note
All claims expressed in this article are solely those of the authors and do not necessarily represent those of their affiliated organizations, or those of the publisher, the editors and the reviewers. Any product that may be evaluated in this article, or claim that may be made by its manufacturer, is not guaranteed or endorsed by the publisher.
Supplementary Material
The Supplementary Material for this article can be found online at: https://www.frontiersin.org/articles/10.3389/fimmu.2022.831848/full#supplementary-material
Supplementary Figure 1 | Receiver operating characteristic curve (ROC) for LDH and dNLR.
Supplementary Figure 2 | Forest plots for multivariate Cox proportional hazards regression model. A) Multivariate analysis for Disease free survival; 2) Multivariate analysis for Overall survival.
Supplementary Figure 3 | Kaplan-Meier curves for disease-free survival (DFS) and overall survival (OS). (A) Kaplan-Meier curves for DFS for LDH; (B) Kaplan-Meier curves for OS for breast immune LDH; (C) Kaplan-Meier curves for DFS for dNLR; (D) Kaplan-Meier curves for OS for breast immune dNLR.
Supplementary Figure 4 | Dynamic Nomogram for predicting the survival. (A) Dynamic Nomogram for DFS; (B) Dynamic Nomogram for OS.
Supplementary Figure 5 | Decision curve analysis (DCA) of the BIPI and pathological N stage or pathological TNM stage for predicting the disease free survival (DFS) and overall survival (OS). The X axis represents threshold probability, and the Y axis shows net benefit. The lines between the X-axis and the Y-axis displays the benefit of different predictive variables. The red dotted line suggested that no patient has poor prognosis, while the red line indicated that all patients have poor prognosis. The blue line represented BIPI, and the green line represented only pathological N stage or pathological TNM stage. (A) DCA of the BIPI and pathological N stage for predicting the 5-year DFS; (B) DCA of the BIPI and pathological N stage for predicting the 5-year OS; (C) DCA of the BIPI and pathological TNM stage for predicting the 5-year DFS; (D) DCA of the BIPI and pathological TNM stage for predicting the 5-year OS.
References
1. Siegel RL, Miller KD, Fuchs HE, Jemal A. Cancer Statistics, 2021. CA Cancer J Clin (2021) 71(1):7–33. doi: 10.3322/caac.21654
2. Sung H, Ferlay J, Siegel RL, Laversanne M, Soerjomataram I, Jemal A, et al. Global Cancer Statistics 2020: GLOBOCAN Estimates of Incidence and Mortality Worldwide for 36 Cancers in 185 Countries. CA Cancer J Clin (2021) 71(3):209–49. doi: 10.3322/caac.21660
3. Shien T, Iwata H. Adjuvant and Neoadjuvant Therapy for Breast Cancer. Jpn J Clin Oncol (2020) 50(3):225–9. doi: 10.1093/jjco/hyz213
4. Takada M, Toi M. Neoadjuvant Treatment for HER2-Positive Breast Cancer. Chin Clin Oncol (2020) 9(3):32. doi: 10.21037/cco-20-123
5. Rachdi H, Mokrani A, Batti R, Ayadi M, Chraiet N, Mezlini A. Target Therapy for Metastatic Breast Cancer. Tunis Med (2018) 96(8-9):465–71.
6. Marra A, Viale G, Curigliano G. Recent Advances in Triple Negative Breast Cancer: The Immunotherapy Era. BMC Med (2019) 17(1):90. doi: 10.1186/s12916-019-1326-5
7. Arab A, Yazdian-Robati R, Behravan J. HER2-Positive Breast Cancer Immunotherapy: A Focus on Vaccine Development. Arch Immunol Ther Exp (Warsz) (2020) 68(1):2. doi: 10.1007/s00005-019-00566-1
8. Kennedy LB, Salama AKS. A Review of Cancer Immunotherapy Toxicity. CA Cancer J Clin (2020) 70(2):86–104. doi: 10.3322/caac.21596
9. Ribas A, Wolchok JD. Cancer Immunotherapy Using Checkpoint Blockade. Science (2018) 359(6382):1350–5. doi: 10.1126/science.aar4060
10. Thelen M, Wennhold K, Lehmann J, Garcia-Marquez M, Klein S, Kochen E, et al. Cancer-Specific Immune Evasion and Substantial Heterogeneity Within Cancer Types Provide Evidence for Personalized Immunotherapy. NPJ Precis Oncol (2021) 5(1):52. doi: 10.1038/s41698-021-00196-x
11. Xu H, Lien T, Bergholtz H, Fleischer T, Djerroudi L, Vincent-Salomon A, et al. Multi-Omics Marker Analysis Enables Early Prediction of Breast Tumor Progression. Front Genet (2021) 12:670749. doi: 10.3389/fgene.2021.670749
12. Greten FR, Grivennikov SI. Inflammation and Cancer: Triggers, Mechanisms, and Consequences. Immunity (2019) 51(1):27–41. doi: 10.1016/j.immuni.2019.06.025
13. Jiang X, Shapiro DJ. The Immune System and Inflammation in Breast Cancer. Mol Cell Endocrinol (2014) 382(1):673–82. doi: 10.1016/j.mce.2013.06.003
14. Grassadonia A, Graziano V, Iezzi L, Vici P, Barba M, Pizzuti L, et al. Prognostic Relevance of Neutrophil to Lymphocyte Ratio (NLR) in Luminal Breast Cancer: A Retrospective Analysis in the Neoadjuvant Setting. Cells (2021) 10(7):1685. doi: 10.3390/cells10071685
15. Deng JP, Hua X, Long ZQ, Zhang WW, Lin HX, He ZY. Prognostic Value of Skeletal Muscle Index and Monocyte-to-Lymphocyte Ratio for Lymph Node-Positive Breast Cancer Patients After Mastectomy. Ann Transl Med (2019) 7(23):775. doi: 10.21037/atm.2019.11.37
16. Yang L, Wang H, Ma J, Hao J, Zhang C, Ma Q, et al. Association Between the Platelet to Lymphocyte Ratio, Neutrophil to Lymphocyte Ratio and Axillary Lymph Node Metastasis in Ct1n0 Breast Cancer Patients. Am J Transl Res (2021) 13(3):1854–61.
17. Chen L, Bai P, Kong X, Huang S, Wang Z, Wang X, et al. Prognostic Nutritional Index (PNI) in Patients With Breast Cancer Treated With Neoadjuvant Chemotherapy as a Useful Prognostic Indicator. Front Cell Dev Biol (2021) 9:656741. doi: 10.3389/fcell.2021.656741
18. Chen L, Kong X, Wang Z, Wang X, Fang Y, Wang J. Pre-Treatment Systemic Immune-Inflammation Index Is a Useful Prognostic Indicator in Patients With Breast Cancer Undergoing Neoadjuvant Chemotherapy. J Cell Mol Med (2020) 24(5):2993–3021. doi: 10.1111/jcmm.14934
19. Dong J, Sun Q, Pan Y, Lu N, Han X, Zhou Q. Pretreatment Systemic Inflammation Response Index is Predictive of Pathological Complete Response in Patients With Breast Cancer Receiving Neoadjuvant Chemotherapy. BMC Cancer (2021) 21(1):700. doi: 10.1186/s12885-021-08458-4
20. Ren K, Yin Y, He F, Shao Y, Wang S. Prognostic Role of Derived Neutrophil-to-Lymphocyte Ratio in Surgical Triple-Negative Breast Cancer. Cancer Manag Res (2018) 10:4891–8. doi: 10.2147/CMAR.S180695
21. Huang W, Liu P, Zong M, Chen QQ, Zhou H, Kong H, et al. Combining Lactate Dehydrogenase and Fibrinogen: Potential Factors to Predict Therapeutic Efficacy and Prognosis of Patients With Small-Cell Lung Cancer. Cancer Manag Res (2021) 13:4299–307. doi: 10.2147/CMAR.S300153
22. Bustos MA, Gross R, Rahimzadeh N, Cole H, Tran LT, Tran KD, et al. A Pilot Study Comparing the Efficacy of Lactate Dehydrogenase Levels Versus Circulating Cell-Free microRNAs in Monitoring Responses to Checkpoint Inhibitor Immunotherapy in Metastatic Melanoma Patients. Cancers (Basel) (2020) 12(11):3361. doi: 10.3390/cancers12113361
23. Aday U, Böyük A, Akkoç H. The Prognostic Significance of Serum Lactate Dehydrogenase-to-Albumin Ratio in Colorectal Cancer. Ann Surg Treat Res (2020) 99(3):161–70. doi: 10.4174/astr.2020.99.3.161
24. Aldea M, Benitez JC, Mezquita L. The Lung Immune Prognostic Index (LIPI) Stratifies Prognostic Groups in Advanced non-Small Cell Lung Cancer (NSCLC) Patients. Transl Lung Cancer Res (2020) 9(4):967–70. doi: 10.21037/tlcr.2020.04.14
25. Mezquita L, Auclin E, Ferrara R, Charrier M, Remon J, Planchard D, et al. Association of the Lung Immune Prognostic Index With Immune Checkpoint Inhibitor Outcomes in Patients With Advanced Non-Small Cell Lung Cancer. JAMA Oncol (2018) 4(3):351–7. doi: 10.1001/jamaoncol.2017.4771
26. Lim JU, Kang HS, Yeo CD, Kim JS, Park CK, Kim JW, et al. Predictability of Early Changes in Derived Neutrophil-to-Lymphocyte Ratio and Neutrophil-to-Lymphocyte Ratio in Patients With Advanced Non-Small Cell Lung Cancer Treated With Immune Checkpoint Inhibitors. J Thorac Dis (2021) 13(5):2824–32. doi: 10.21037/jtd-20-3416
27. Fisusi FA, Akala EO. Drug Combinations in Breast Cancer Therapy. Pharm Nanotechnol (2019) 7(1):3–23. doi: 10.2174/2211738507666190122111224
28. McKelvey KJ, Hudson AL, Back M, Eade T, Diakos CI. Radiation, Inflammation and the Immune Response in Cancer. Mamm Genome (2018) 29(11-12):843–65. doi: 10.1007/s00335-018-9777-0
29. Gil Del Alcazar CR, Alečković M, Polyak K. Immune Escape During Breast Tumor Progression. Cancer Immunol Res (2020) 8(4):422–7. doi: 10.1158/2326-6066.CIR-19-0786
30. Domschke C, Schneeweiss A, Stefanovic S, Wallwiener M, Heil J, Rom J, et al. Cellular Immune Responses and Immune Escape Mechanisms in Breast Cancer: Determinants of Immunotherapy. Breast Care (Basel) (2016) 11(2):102–7. doi: 10.1159/000446061
31. Petitprez F, Meylan M, de Reyniès A, Sautès-Fridman C, Fridman WH. The Tumor Microenvironment in the Response to Immune Checkpoint Blockade Therapies. Front Immunol (2020) 11:784. doi: 10.3389/fimmu.2020.00784
32. Gibney GT, Weiner LM, Atkins MB. Predictive Biomarkers for Checkpoint Inhibitor-Based Immunotherapy. Lancet Oncol (2016) 17(12):e542–51. doi: 10.1016/S1470-2045(16)30406-5
33. Mantovani A, Allavena P, Sica A, Balkwill F. Cancer-Related Inflammation. Nature (2008) 454(7203):436–44. doi: 10.1038/nature07205
34. Grivennikov SI, Greten FR, Karin M. Immunity, Inflammation, and Cancer. Cell (2010) 140(6):883–99. doi: 10.1016/j.cell.2010.01.025
35. Wulaningsih W, Holmberg L, Garmo H, Malmstrom H, Lambe M, Hammar N, et al. Serum Lactate Dehydrogenase and Survival Following Cancer Diagnosis. Br J Cancer (2015) 113(9):1389–96. doi: 10.1038/bjc.2015.361
36. Hirschhaeuser F, Sattler UG, Mueller-Klieser W. Lactate: A Metabolic Key Player in Cancer. Cancer Res (2011) 71(22):6921–5. doi: 10.1158/0008-5472.CAN-11-1457
37. Van Wilpe S, Koornstra R, Den Brok M, De Groot JW, Blank C, De Vries J, et al. Lactate Dehydrogenase: A Marker of Diminished Antitumor Immunity. Oncoimmunology (2020) 9(1):1731942. doi: 10.1080/2162402X.2020.1731942
38. Miao P, Sheng S, Sun X, Liu J, Huang G. Lactate Dehydrogenase A in Cancer: A Promising Target for Diagnosis and Therapy. IUBMB Life (2013) 65(11):904–10. doi: 10.1002/iub.1216
39. Yang T, Hao L, Yang X, Luo C, Wang G, Lin Cai C, et al. Prognostic Value of Derived Neutrophil-to-Lymphocyte Ratio (dNLR) in Patients With Non-Small Cell Lung Cancer Receiving Immune Checkpoint Inhibitors: A Meta-Analysis. BMJ Open (2021) 11(9):e049123. doi: 10.1136/bmjopen-2021-049123
40. Capone M, Giannarelli D, Mallardo D, Madonna G, Festino L, Grimaldi AM, et al. Baseline Neutrophil-to-Lymphocyte Ratio (NLR) and Derived NLR Could Predict Overall Survival in Patients With Advanced Melanoma Treated With Nivolumab. J Immunother Cancer (2018) 6(1):74. doi: 10.1186/s40425-018-0383-1
41. Xu Z, Yan Y, Wang X, Zeng S, Gong Z. Lung Immune Prognostic Index for Outcome Prediction to Immunotherapy in Patients With NSCLC. J Thorac Oncol (2019) 14(9):e207–8. doi: 10.1016/j.jtho.2019.04.027
42. Meyers DE, Stukalin I, Vallerand IA, Lewinson RT, Suo A, Dean M, et al. The Lung Immune Prognostic Index Discriminates Survival Outcomes in Patients With Solid Tumors Treated With Immune Checkpoint Inhibitors. Cancers (Basel) (2019) 11(11):1713. doi: 10.3390/cancers11111713
43. Kim JW, Tchernyshyov I, Semenza GL, Dang CV. HIF-1-Mediated Expression of Pyruvate Dehydrogenase Kinase: A Metabolic Switch Required for Cellular Adaptation to Hypoxia. Cell Metab (2006) 3(3):177–85. doi: 10.1016/j.cmet.2006.02.002
44. Armstrong AJ, George DJ, Halabi S. Serum Lactate Dehydrogenase Predicts for Overall Survival Benefit in Patients With Metastatic Renal Cell Carcinoma Treated With Inhibition of Mammalian Target of Rapamycin. J Clin Oncol (2012) 30(27):3402–7. doi: 10.1200/JCO.2011.40.9631
45. Lewis BC, Prescott JE, Campbell SE, Shim H, Orlowski RZ, Dang CV. Tumor Induction by the C-Myc Target Genes Rcl and Lactate Dehydrogenase a. Cancer Res (2000) 60(21):6178–83.
46. Zha X, Wang F, Wang Y, He S, Jing Y, Wu X, et al. Lactate Dehydrogenase B is Critical for Hyperactive mTOR-Mediated Tumorigenesis. Cancer Res (2011) 71(1):13–8. doi: 10.1158/0008-5472.CAN-10-1668
47. Nagle SJ, Woo K, Schuster SJ, Nasta SD, Stadtmauer E, Mick R, et al. Outcomes of Patients With Relapsed/Refractory Diffuse Large B-Cell Lymphoma With Progression of Lymphoma After Autologous Stem Cell Transplantation in the Rituximab Era. Am J Hematol (2013) 88(10):890–4. doi: 10.1002/ajh.23524
48. Brown JE, Cook RJ, Lipton A, Coleman RE. Serum Lactate Dehydrogenase Is Prognostic for Survival in Patients With Bone Metastases From Breast Cancer: A Retrospective Analysis in Bisphosphonate-Treated Patients. Clin Cancer Res (2012) 18(22):6348–55. doi: 10.1158/1078-0432.CCR-12-1397
49. Faloppi L, Scartozzi M, Bianconi M, Svegliati Baroni G, Toniutto P, Giampieri R, et al. The Role of LDH Serum Levels in Predicting Global Outcome in HCC Patients Treated With Sorafenib: Implications for Clinical Management. BMC Cancer (2014) 14:110. doi: 10.1186/1471-2407-14-110
50. Tuomisto AE, Mäkinen MJ, Väyrynen JP. Systemic Inflammation in Colorectal Cancer: Underlying Factors, Effects, and Prognostic Significance. World J Gastroenterol (2019) 25(31):4383–404. doi: 10.3748/wjg.v25.i31.4383
51. Umamaheswaran S, Dasari SK, Yang P, Lutgendorf SK, Sood AK. Stress, Inflammation, and Eicosanoids: An Emerging Perspective. Cancer Metastasis Rev (2018) 37(2-3):203–11. doi: 10.1007/s10555-018-9741-1
52. Giese MA, Hind LE, Huttenlocher A. Neutrophil Plasticity in the Tumor Microenvironment. Blood (2019) 133(20):2159–67. doi: 10.1182/blood-2018-11-844548
53. Mackey JBG, Coffelt SB, Carlin LM. Neutrophil Maturity in Cancer. Front Immunol (2019) 10:1912. doi: 10.3389/fimmu.2019.01912
54. Lecot P, Sarabi M, Pereira Abrantes M, Mussard J, Koenderman L, Caux C, et al. Neutrophil Heterogeneity in Cancer: From Biology to Therapies. Front Immunol (2019) 10:2155. doi: 10.3389/fimmu.2019.02155
55. Li S, Zou J, Liu C, Jiao X, Gong J, Li J, et al. Baseline Derived Neutrophil-to-Lymphocyte Ratio as a Prognostic Biomarker for Non-Colorectal Gastrointestinal Cancer Patients Treated With Immune Checkpoint Blockade. Clin Immunol (2020) 212:108345. doi: 10.1016/j.clim.2020.108345
56. Russo A, Franchina T, Ricciardi GRR, Battaglia A, Scimone A, Berenato R, et al. Baseline Neutrophilia, Derived Neutrophil-to-Lymphocyte Ratio (dNLR), Platelet-to-Lymphocyte Ratio (PLR), and Outcome in non Small Cell Lung Cancer (NSCLC) Treated With Nivolumab or Docetaxel. J Cell Physiol (2018) 233(10):6337–43. doi: 10.1002/jcp.26609
Keywords: breast cancer, breast immune prognostic index, nomogram, neoadjuvant chemotherapy, survival
Citation: Chen L, Kong X, Huang S, Su Z, Zhu M, Fang Y, Zhang L, Li X and Wang J (2022) Preoperative Breast Immune Prognostic Index as Prognostic Factor Predicts the Clinical Outcomes of Breast Cancer Patients Receiving Neoadjuvant Chemotherapy. Front. Immunol. 13:831848. doi: 10.3389/fimmu.2022.831848
Received: 09 December 2021; Accepted: 10 February 2022;
Published: 07 March 2022.
Edited by:
Xu Wang, Affiliated Hospital of Jiangsu University, ChinaReviewed by:
Qian Chen, Guangxi Medical University Cancer Hospital, ChinaQingyu Luo, Dana–Farber Cancer Institute, United States
Aiping Luo, Cancer Hospital, Chinese Academy of Medical Sciences and Peking Union Medical College, China
Copyright © 2022 Chen, Kong, Huang, Su, Zhu, Fang, Zhang, Li and Wang. This is an open-access article distributed under the terms of the Creative Commons Attribution License (CC BY). The use, distribution or reproduction in other forums is permitted, provided the original author(s) and the copyright owner(s) are credited and that the original publication in this journal is cited, in accordance with accepted academic practice. No use, distribution or reproduction is permitted which does not comply with these terms.
*Correspondence: Lin Zhang, dG9ueTE5ODIxMTBAZ21haWwuY29t; Xingrui Li, bGl4aW5ncnVpQHRqaC50am11LmVkdS5jbg==; Jing Wang, d2FuZ2ppbmdAY2ljYW1zLmFjLmNu
†ORCID: Li Chen, orcid.org/0000-0002-6989-1177
‡These authors have contributed equally to this work