Commentary: Identification of Genes Linking Natural Killer Cells to Apoptosis in Acute Myocardial Infarction and Ischemic Stroke
- 1Department of Cardiovascular Surgery, Xijing Hospital, The Fourth Military Medical University, Xi’an, China
- 2National Translational Science Center for Molecular Medicine and Department of Cell Biology, Fourth Military Medical University, Xi’an, China
- 3Department of Breast and Thyroid Surgery, Xijing Hospital, Fourth Military Medical University, Xi’an, China
- 4Department of Geriatrics, Xijing Hospital, The Fourth Military Medical University, Xi’an, China
- 5Department of Internal Medicine, Central Health Center of Huilong Town, Shangluo, China
- 6College of Life Science, Northwest University, Xi’an, China
- 7The Second Clinical Medicine College, Shaanxi University of Chinese Medicine, Xianyang, China
Natural killer (NK) cells are a type of innate lymphoid cell that are involved in the progression of acute myocardial infarction and ischemic stroke. Although multiple forms of programmed cell death are known to play important roles in these diseases, the correlation between NK cells and apoptosis-related genes during acute myocardial infarction and ischemic stroke remains unclear. In this study, we explored the distinct patterns of NK cell infiltration and apoptosis during the pathological progression of acute myocardial infarction and ischemic stroke using mRNA expression microarrays from the Gene Expression Omnibus database. Since the abundance of NK cells correlated positively with apoptosis in both diseases, we further examined the correlation between NK cell abundance and the expression of apoptosis-related genes. Interestingly, APAF1 and IRAK3 expression correlated negatively with NK cell abundance in both acute myocardial infarction and ischemic stroke, whereas ATM, CAPN1, IL1B, IL1R1, PRKACA, PRKACB, and TNFRSF1A correlated negatively with NK cell abundance in acute myocardial infarction. Together, these findings suggest that these apoptosis-related genes may play important roles in the mechanisms underlying the patterns of NK cell abundance and apoptosis in acute myocardial infarction and ischemic stroke. Our study, therefore, provides novel insights for the further elucidation of the pathogenic mechanism of ischemic injury in both the heart and the brain, as well as potential useful therapeutic targets.
Introduction
Cardiovascular and cerebrovascular diseases are associated with high mobility and mortality and are the leading causes of death worldwide (1). It was recently reported that cardiovascular diseases (CVDs), which include coronary heart disease, heart failure, stroke, and hypertension, have a prevalence of 49.2% in adults which increases with age in both males and females (2). It is therefore highly important to study the mechanisms underlying CVDs in order to develop improved methods of treatment and prevention.
Both acute myocardial infarction (AMI) and ischemic stroke (IS) are characterized by ischemia and hypoxia in the target organ, as well as inflammation and multiple forms of cell death. Immune cells play important roles in the occurrence, progression, and outcome of CVDs (3, 4). In particular, studies have reported that natural killer (NK) cells are crucially involved in AMI and IS (5, 6). NK cells are type I innate lymphoid cells that exhibit a lymphoid cellular morphology without antigen specificity, express the transcription factor T-bet, and produce IFN-γ, perforin, and granzyme B (7). Patients with coronary artery disease showed a significant reduction in the number of circulating NK cells compared with healthy controls (4, 8, 9). In IS, NK cells display distinct patterns of infiltration characterized by an increased accumulation in the brain and decreased accumulation in the peripheral blood (5). However, few studies have compared the differences in immune cell infiltration during AMI and IS. Furthermore, the role of NK cells in AMI and IS remains poorly characterized.
Since the mid-1980s, when apoptosis was the only well-defined form of regulated cell death, more than 10 other mechanistically distinct forms of programmed cell death have been recognized (10). Most recently, Gasdermin D(GSDMD)-mediated cardiomyocyte pyroptosis was reported to contribute toward heart damage during myocardial infarction and reperfusion injury (11). Although various therapies targeting these different forms of cell death have produced favorable results in preclinical studies and can effectively reduce adverse remodeling after AMI (12, 13), the correlations between immune cell infiltration and programmed cell death in AMI and IS and their pathological mechanisms remain unclear.
In this study, we performed bioinformatics analyses to compare the patterns of immune cell infiltration and cell death in patients with AMI or IS at the transcriptional level. Moreover, we identified correlations between NK cell infiltration and the expression of specific apoptosis-related genes. Together, the novel analyses performed in this study may provide new avenues for the study of AMI and IS, as well as the development of beneficial clinical treatments.
Materials and Methods
Data Collection and Processing
The overall design of our study is illustrated in Figure 1. First, we acquired the gene expression profiles of patients with AMI and IS from the Gene Expression Omnibus (GEO) database (http://www.ncbi.nlm.nih.gov/geo). Five mRNA microarray datasets were downloaded: GSE59867, GSE22255, GSE58294, GSE48060, and GSE16561. These datasets met the following criteria: (1) data acquired using microarray platforms detecting >15,000 genes; (2) ≥ 40 patients recruited; (3) all samples from Homo sapiens.
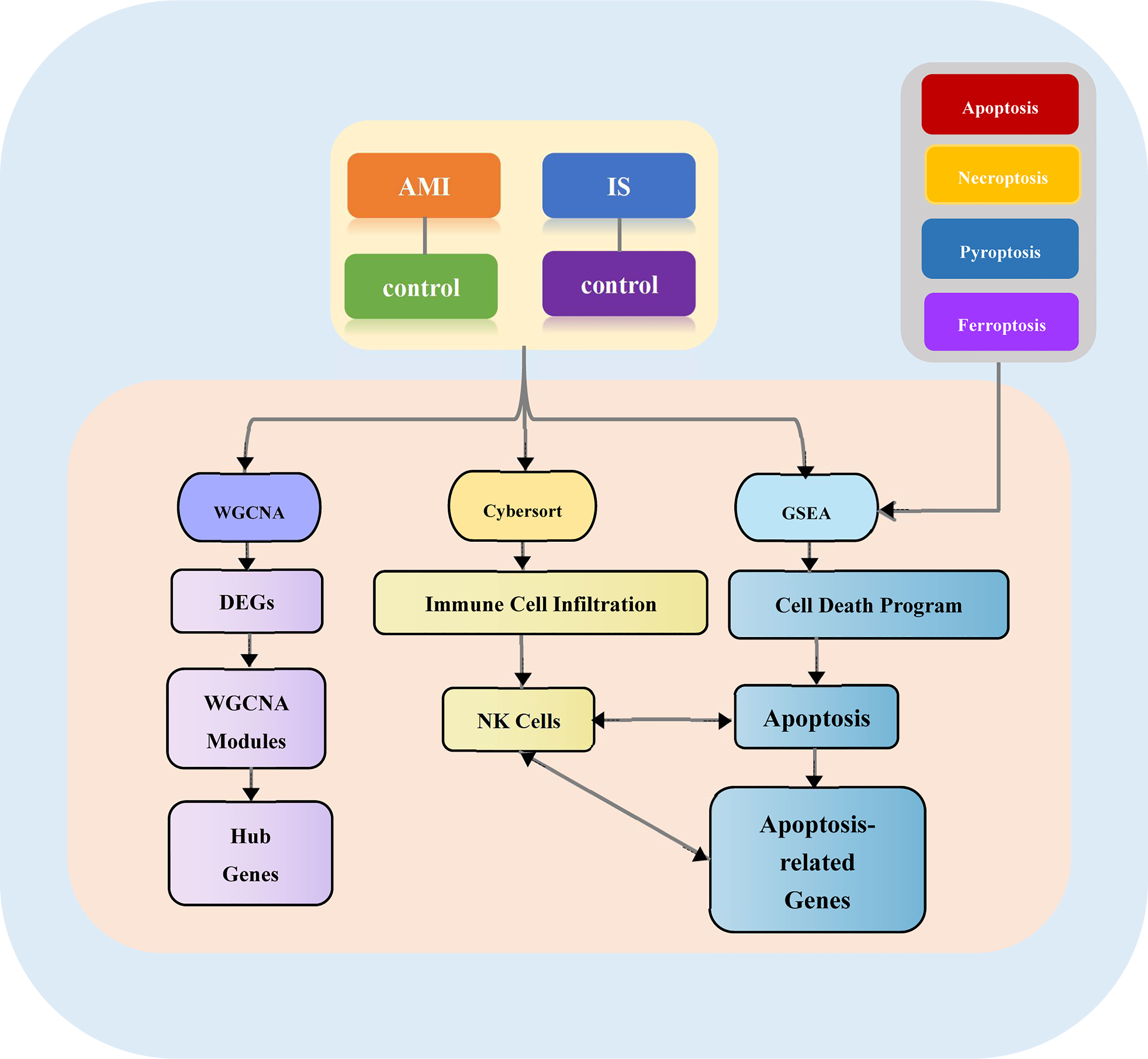
Figure 1 Study flowchart. AMI, acute myocardial infarction; DEGs, differentially expressed genes; WGCNA, weighted gene co-expression network analysis; GSEA, gene set enrichment analysis; NK cells, natural killer cells.
GSE59867 was based on the Affymetrix Human Gene 1.0 ST Array [transcript (gene) version]; 111 AMI and 46 control samples derived from peripheral blood mononuclear cells (PBMCs) were analyzed. GSE22255, GSE58294, and GSE48060 were based on Affymetrix Human Genome U133 Plus 2.0 Arrays. For GSE22255, gene expression profiling was performed on PBMCs from 20 patients with IS and 20 sex-and age-matched controls. For GSE58294, 23 control samples and 69 cardioembolic stroke samples were assayed. For GSE48060, blood samples from 31 patients with AMI and 21 controls were analyzed. GSE16561 was based on the Illumina HumanRef-8 v3.0 expression beadchip analysis of total RNA extracted from the whole blood of 39 patients with IS and 24 healthy controls. GSE22255, GSE59867, and GSE58294 were used as basic datasets for screening differentially expressed genes (DEGs), immune cell infiltration analysis, and functional enrichment analysis. GSE48060 and GSE16561 were used as the validation cohorts. The details of these datasets are provided in Table 1.
Data Merging
The GEO datasets were merged using the “sva” R package (19). GSE22255 and GSE58294 were merged as the input data for DEG analysis, whereas GSE22255, GSE58294, andGSE59867 were merged as the input data for weighted gene co-expression network analysis (WGCNA) and to evaluate immune cell infiltration.
DEG Screening
DEG analysis was performed on the GEO array data using the “Limma” R package (20). Genes with a P-value < 0.05 and |log2fold change (FC)| > log2(1.2) were considered DEGs.
Functional Enrichment Analysis
Gene ontology (GO) analysis, Kyoto encyclopedia of genes and genomes (KEGG) analysis, and gene set enrichment analysis (GSEA) (21) were performed using the “clusterProfiler” (22) and “GSEABase” R packages.
WGCNA
WGCNA was performed using the “WGCNA” (23) R package with the top 5000 DEGs as input genes. Hub genes in the WGCNA modules were identified using Cytoscape (24).
Evaluation of Immune Cell Infiltration
The infiltration of 22 immune cell types in the AMI and IS samples used in this study was analyzed using CIBERSORT (25). The expression data were imported into CIBERSORT using R and then iterated 1000 times to estimate the relative proportion of each immune cell type.
GSEA
GSEA was performed to find enriched terms in four cell death pathways. Differences were considered statistically significant if p < 0.05 or FDR (false discovery rate) < 0.25 and |NES| >1.
Gene Set Variation Analysis (GSVA)
GSVA (26) was performed to transform the GEO gene expression matrices into programmed cell death enrichment matrices to detect subtle pathway activity changes over a sample population. And the results were used to analyse the relationship between immune infiltration and the cell death.
Results
Identifying DEGs in AMI and IS
To identify DEGs in the basic datasets obtained from the GEO, we first merged the expression matrices to eliminate between-batch differences. For AMI, 1002 DEGs were obtained, 429 of which were significantly upregulated and 573 of which were downregulated. Meanwhile, 2488 DEGs were obtained for IS, of which 1133 were significantly upregulated and 1355 were significantly downregulated. Volcano plots and heatmaps of these DEGs are shown in Figures 2A, B, D, E. To further investigate the functions of these DEGs, we conducted GO and KEGG enrichment analyses. The top 10 enriched terms are listed (Figures 2C, F). Notably, the DEGs in both AMI and IS were mainly associated with immune system activation, immune cell activities, inflammatory cytokine release, and inflammation-related pathways. Together, these findings suggest that the DEGs are likely to be associated with inflammatory processes (Figures 2C, F).
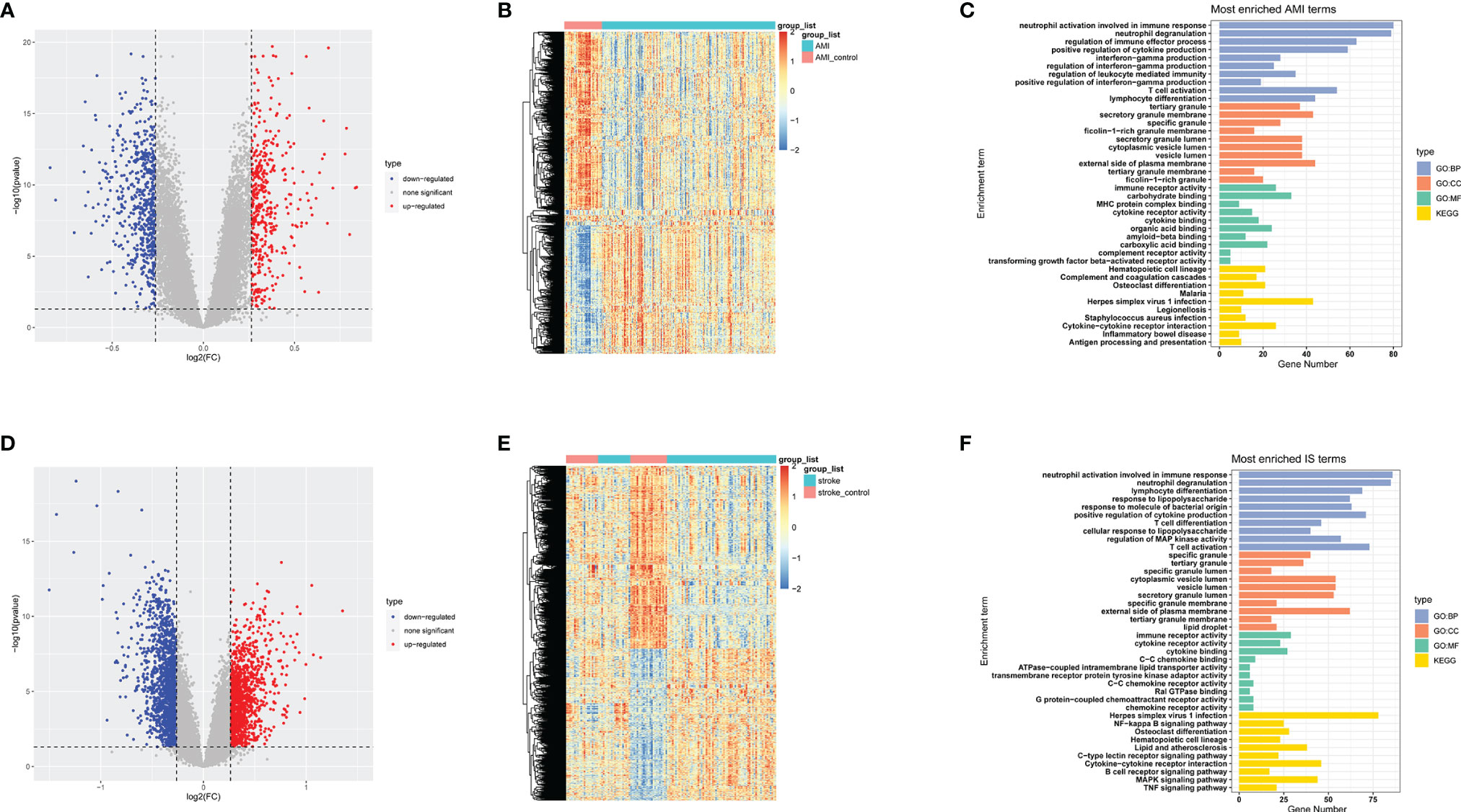
Figure 2 Data preprocessing, DEG identification, and enrichment analyses. (A) Volcano plots of DEGs in AMI. (B) Heatmaps of DEGs in AMI. (C) GO and KEGG enrichment analyses in AMI. (D) Volcano plots of DEGs in IS. (E) Heatmaps of DEGs in IS. (F) GO and KEGG enrichment analysis in IS. Statistical significance was determined by hypergeometric distribution analysis and the P-values were calculated by Fisher’s exact test. CC, cell component; BP, biological process; MF, molecular function.
WGCNA and Identification of Key Modules
Next, we performed WGCNA to identify key modules and hub genes in AMI and IS. After the outliers had been removed, we drew a sample clustering tree (Figure 3A) and constructed a scale-free network (Figures 3B, C) with a soft threshold of 9 (R2 = 0.98). An adjacency matrix was then built and a topological overlap matrix was constructed. A total of 13 modules were identified based on average hierarchical clustering and dynamic tree clipping (Figure 3D). The yellow module was strongly and positively related to both AMI and IS, whereas the red module was positively and negatively related to AMI and IS, respectively. Although the pink and green-yellow modules correlated significantly with AMI, they did not correlate significantly with IS. Conversely, the tan and magenta modules correlated significantly with IS but not AMI. Consequently, these six modules were selected as clinically important modules for further analysis.
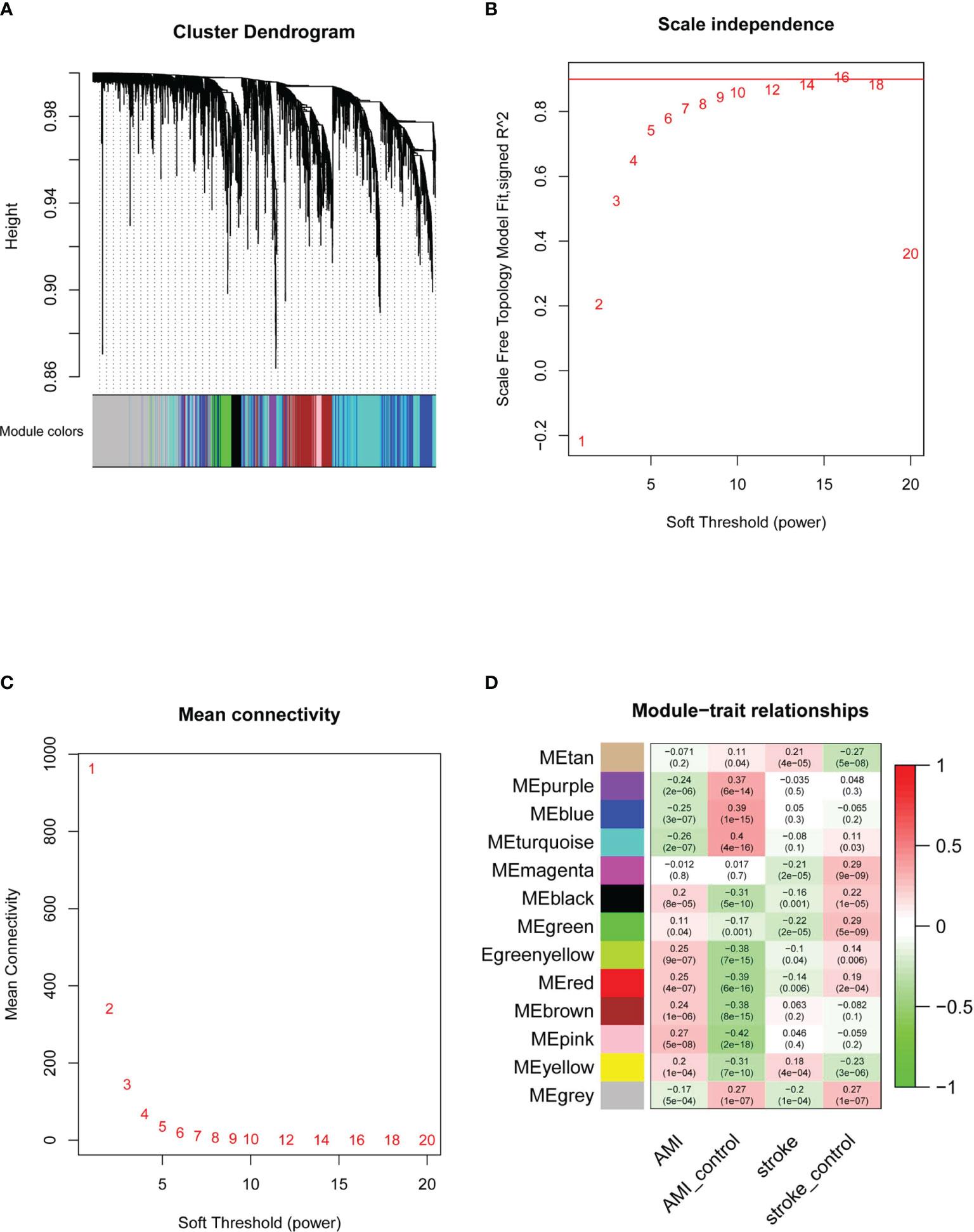
Figure 3 Determination of WGCNA soft-threshold power and identification of modules associated with AMI and IS. (A) Dendrogram of DEGs clustered on the basis of the measurement of dissimilarity (1-TOM). The color band indicates the results from automatic single-block analysis. (B) Scale-free index analysis for various soft-threshold powers. (C) Mean connectivity analysis for various soft-threshold powers. (D) Heatmap of the correlation between modules and diseases. TOM, topological overlap matrix; ME, module eigengene. Statistical significance was determined by t-test.
GO enrichment analyses for cell component (CC), biological process (BP), and molecular function (MF) in these modules were performed using clusterProfiler. In the yellow module, the BP were mainly associated with neutrophil activation, the CC were mainly associated with multiple cellular granules and membranes, and the MF were significantly related to the receptor for advanced glycation end products (RAGE). Together, these results suggest that the yellow module is mainly involved in the activation of inflammation. In the red module, the BP were mainly related to the electron transport chain and ATP metabolic processes, the CC were related to mitochondrial activities, and the MF were associated with electron transfer activity, which demonstrated that the genes of the red module were mainly involved in the regulation of mitochondrial activities. Further information about each module and its hub genes are included in the Supplementary Data. Taken together, the findings of these analyses suggest that these six modules are highly associated with clinical situations and that the activation of inflammation and mitochondrial activity could play important roles in the development of AMI and IS.
Immune Cell Infiltration Analysis
To further analyze the immune cell infiltration characteristics of AMI and IS, we compared the patterns of immune cell infiltration. Monocytes, NK cells, CD8+T cells, CD4+ memory T cells, CD4+ naïve T cells, neutrophils, and naïve B cells were the main immune cell types in both AMI and IS according to the estimated profiles (Figure 4A). However, the distribution of some immune cell subsets with low abundance was not fully revealed due to the limitations of the CIBERSORT algorithm. As reported previously (3, 24, 25), the proportion of monocytes, which play crucial roles in inflammation and tissue repair, increased greatly after both IS and AMI (3, 27, 28). Most immune cell types displayed similar patterns and infiltration extent between AMI and IS, except for NK cells. As shown in Figure 4A, despite the estimated proportions of NK cells being lower in both AMI and IS than in the controls, the difference between patients with AMI and the controls is much more significant. The general distribution of immune cells in each sample is shown in Figure 4B, in which each immune cell type is represented by a different color and the height represents the percentage of cells in the sample. We also calculated the correlations between these immune cells (Figure 4C), finding that monocytes correlated positively with plasma cells, CD4+ T memory cells, dendritic cells, mast cells, and neutrophils, but correlated negatively with naïve B cells, CD8+T cells, NK cells, and M2 macrophages. Furthermore, NK cells correlated positively with CD8+ T cells and M2 macrophages (data not shown). The alterations in NK cell abundance were significantly different between AMI and IS, while other cell types showed no or smaller differences. Consequently, we decided to examine the patterns of NK cell infiltration in AMI and IS in more detail.
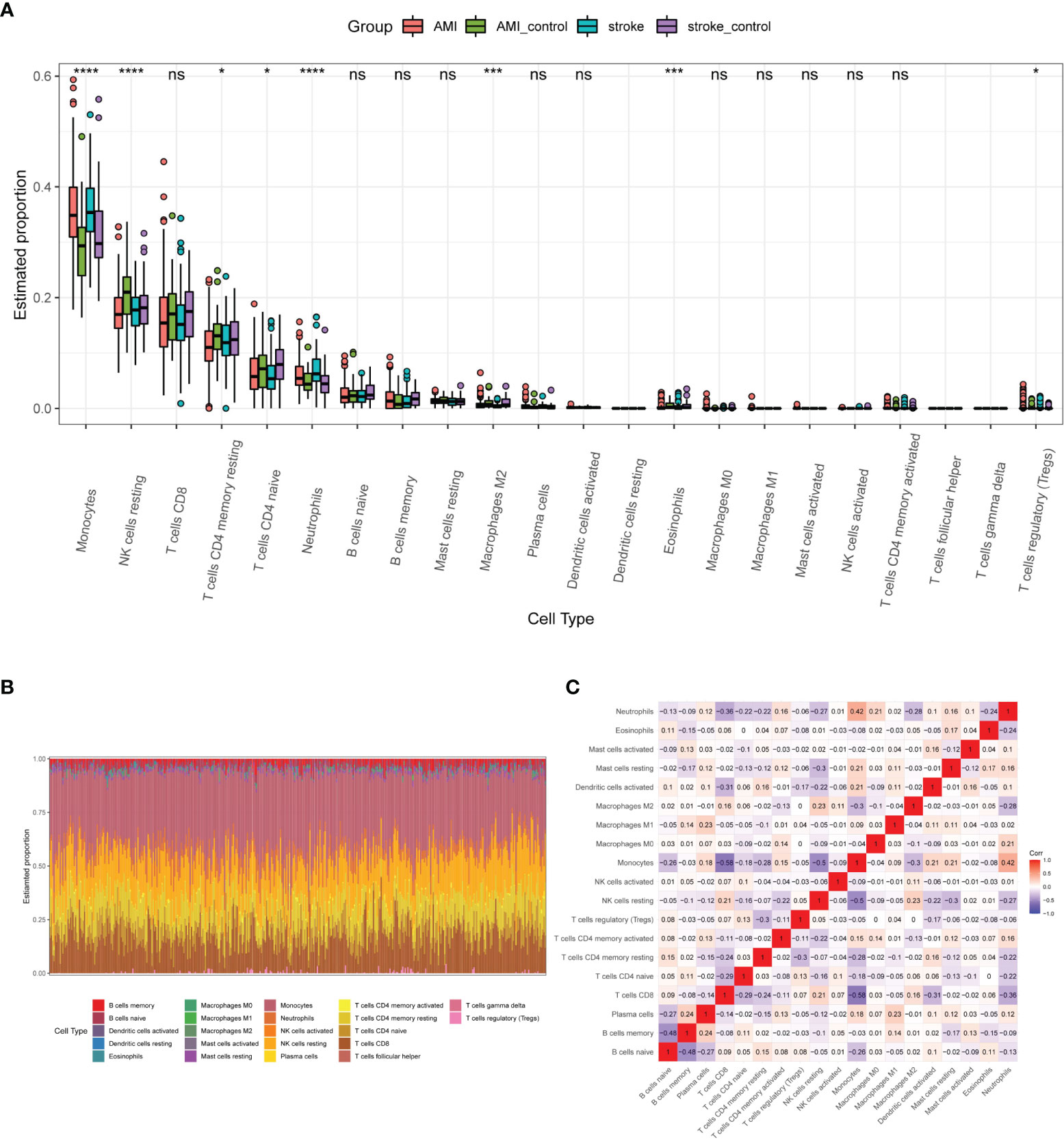
Figure 4 Profiles of immune cell infiltration patterns in the basic datasets. (A) Estimated proportions of 22 immune cell types in AMI and IS. Statistical significance was determined by Kruskal test analysis. * P-value < 0.05; *** P-value < 0.001, **** P-value < 0.0001. NS, not significant. (B) Heatmap of the immune cell proportions in each sample. (C) Correlation heatmap of 19 immune cell types (three were excluded as their proportion was zero).
Programmed Cell Death Analysis
Besides inflammation, the GO analyses of the DEGs indicated that programmed cell death may also participate in the pathology of AMI and IS (Supplementary Material Figure 1), as reported previously (11, 29–31). Therefore, we conducted GSEA to explore the mechanisms underlying cell death in AMI and IS. Due to their prevalence, we decided to focus on apoptosis, ferroptosis, necroptosis, and pyroptosis. As shown in Figure 5, apoptosis appeared to play an important role in IS (p < 0.05) but not in AMI (Figures 5A, B) (p > 0.05). Ferroptosis was not involved in either AMI or IS (Figures 5C, D) (p > 0.05), whereas necroptosis and pyroptosis were significantly involved in both (Figures 5E–H) (p < 0.05).
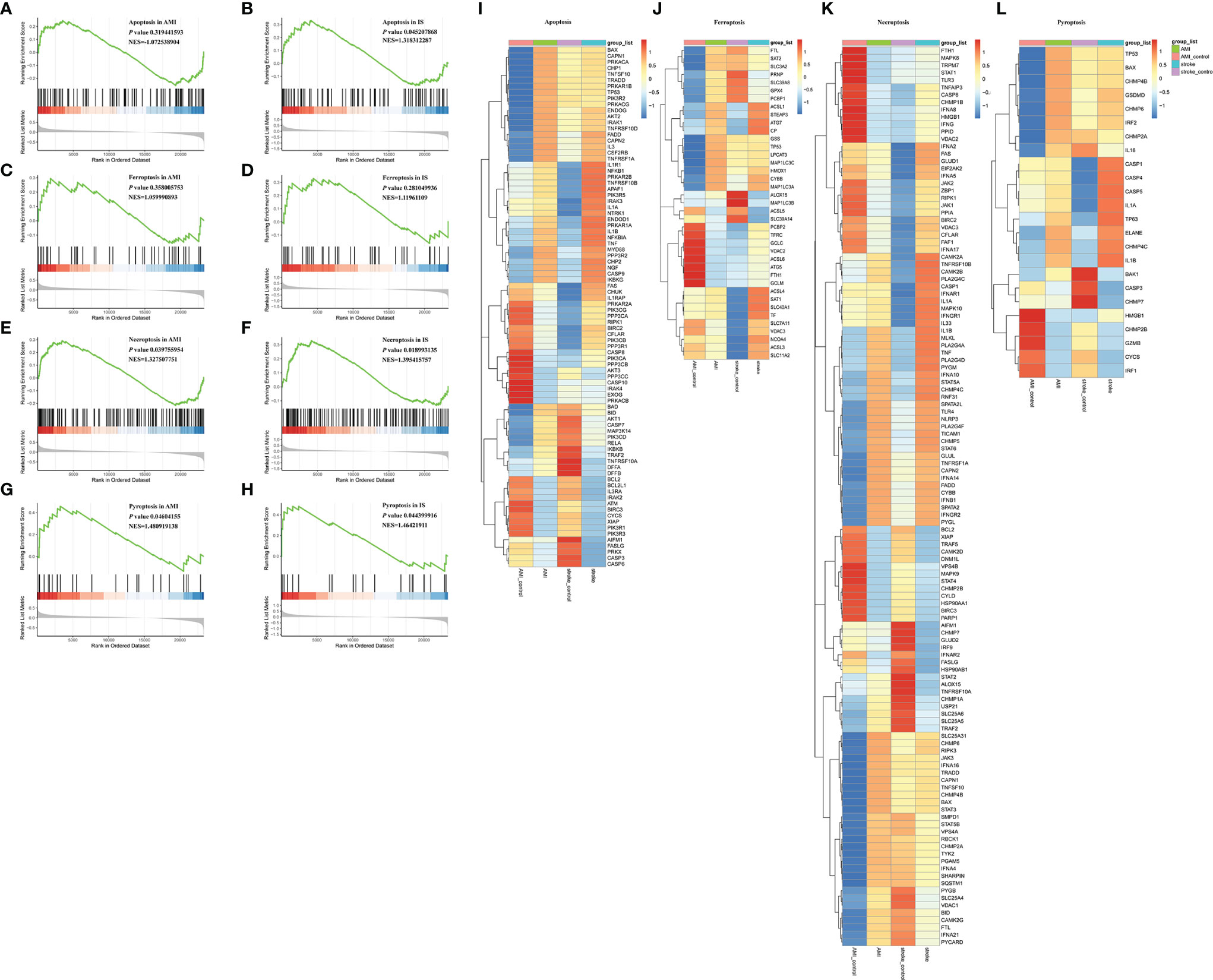
Figure 5 GSEA of apoptosis, necroptosis, ferroptosis, and pyroptosis. (A) GSEA of apoptosis in AMI. (B) GSEA of apoptosis in IS. (C) GSEA of ferroptosis in AMI. (D) GSEA of ferroptosis in IS. (E) GSEA of necroptosis in AMI. (F) GSEA of necroptosis in IS. (G) GSEA of pyroptosis in AMI. (H) GSEA of pyroptosis in IS. Statistically significant associations were determined by permutation test. (I) Heatmaps of DEGs in apoptosis. (J) Heatmaps of DEGs in necroptosis. (K) Heatmaps of DEGs in ferroptosis. (L) Heatmaps of DEGs in pyroptosis.
To further explore the DEGs in each of these forms of cell death, we collected genes related to apoptosis, necroptosis, ferroptosis, and pyroptosis from the Molecular Signatures Database (MSigDB) and conducted GSEA. A total of 88 necroptosis-related genes, 69 apoptosis-related genes, and 17 pyroptosis-related genes were found to be significantly differentially expressed between AMI and IS (Figures 5I–L). To narrow the scope down, we decided to validate the expression patterns of these genes before further investigating their relationship with NK cells.
External Validation of Alterations in Apoptosis-Related Genes
To confirm the relationship between these DEGs and apoptosis, we analyzed their expression in the validation datasets and selected genes with the same patterns of alterations in the basic datasets (Figure 6). In particular, APAF1, CAPN1, IL1B, IRAK3, and PRKACA were significantly upregulated in IS but not in AMI (Figures 6A–E), whereas BAX and TNFRSF1A were markedly upregulated in AMI but not in IS (Figures 6F, G). Meanwhile, the expression of IL1R1 was elevated in both IS and AMI (Figure 6H), and that of PRKX was reduced in IS but not in AMI (Figure 6I). Conversely, PRKACB expression was suppressed in AMI but not in IS (Figure 6J). Additionally, ATM expression was decreased in both IS and AMI (Figure 6K). As such, these genes are likely to be involved in the distinct patterns of NK cell infiltration and apoptosis between AMI and IS.
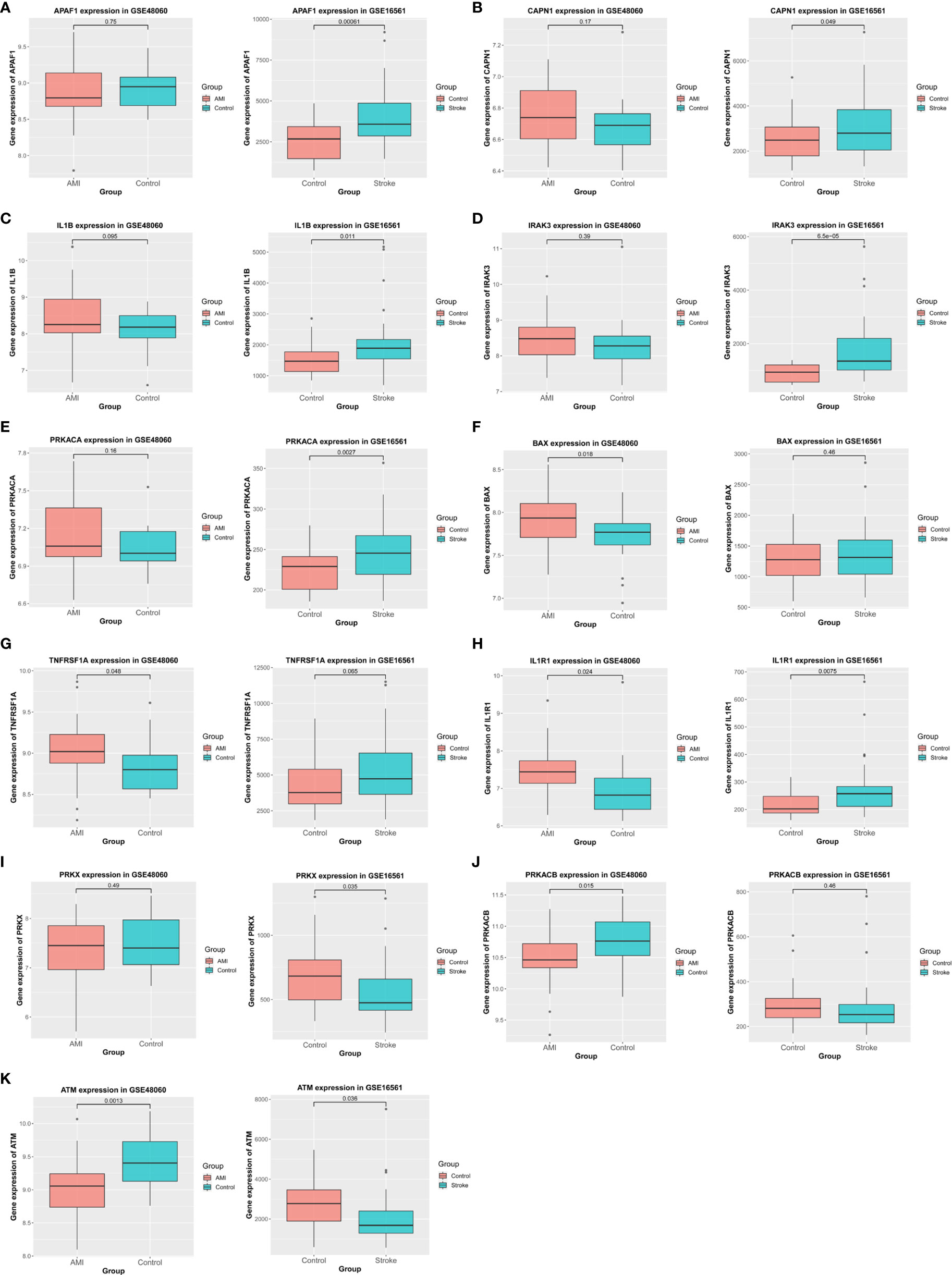
Figure 6 Verification using validation datasets. (A–K) Verification of APAF1, CAPN1, IL1B, IRAK3, PRKACA, BAX, TNFRSF1A, IL1R1, PRKX, PRKACB, and ATM expression in the validation cohort. Statistical significance was determined by t-test. P-values of < 0.05 were considered statistically significant.
Correlation Between Immune Infiltration and Cell Death
Based on our observations, monocytes, NK cells, CD8+ T cells, CD4+ memory T cells, CD4+ naïve T cells, neutrophils, and naïve B cells were the main immune cell types in both AMI and IS. The remaining cell types were estimated to have a low abundance. Then the GEO gene expression matrices were transformed into programmed cell death enrichment matrices by using the GSVA package. We next analyzed the relationship between the patterns of immune cell infiltration, particularly for NK cells, and the types of programmed cell death in AMI and IS (Figure 7). Although the proportions of monocytes and neutrophils correlated positively with all four types of cell death, their changing patterns in Figure 4 show no difference between AMI and IS. Moreover, the proportion of NK cells was positively correlated with apoptosis and negatively correlated with the other three types of cell death. Figures demonstrating the exact correlations and their corresponding P-values are included in the Supplementary Materials (Figures 2, 3) Thus, the lack of NK cells in AMI appears to be consistent with the deficiency in apoptosis in AMI, meaning that the number of NK cells may be associated with apoptosis in cells within the target organ.
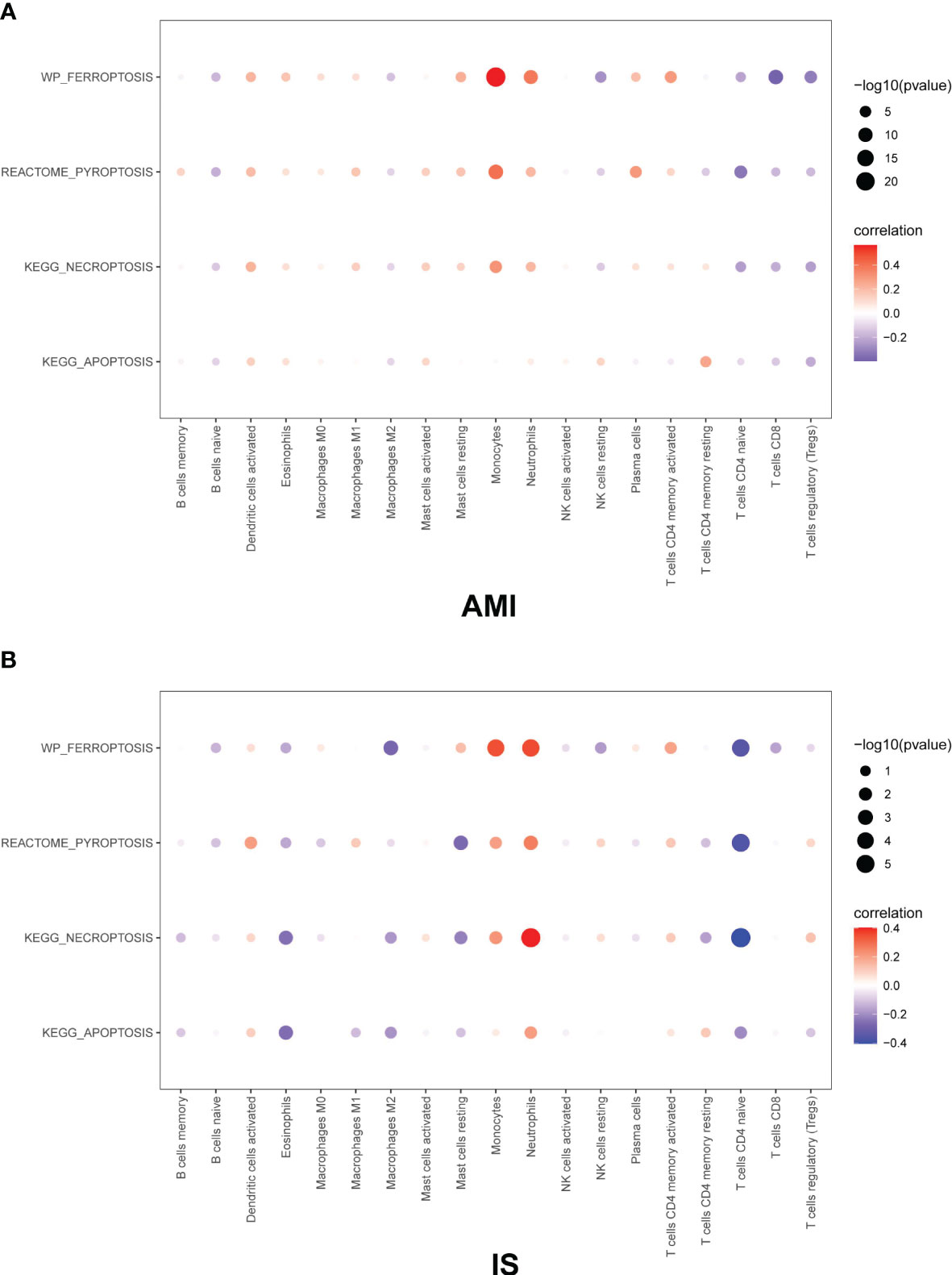
Figure 7 Pearson correlation coefficient between immune cells and cell death in (A) AMI and (B) IS. The Pearson correlation coefficient was determined by Pearson correlation analysis and the P-values were calculated by t-test.
Correlation Between NK Cells and Apoptosis-Related Genes
To further explore the relationship between NK cells and genes related to cell death, we calculated the Pearson correlation coefficient between immune cells and apoptosis-related genes (Figure 8). The values of the Pearson correlation coefficient between each gene and NK cell abundance in AMI and IS as well as the corresponding P-values are shown in the Supplementary Materials (Figures 4–9). The following genes were negatively related to NK cell abundance in AMI and IS: TNFRSF1A (AMI: p < 0.05, IS: p > 0.05), PRKACA (AMI: p < 0.05, IS: p > 0.05), IRAK3 (AMI: p < 0.05, IS: p < 0.05), IL1R1 (AMI: p < 0.05, IS: p > 0.05), and APAF1 (AMI: p < 0.05, IS: p < 0.05). Conversely, the following genes were positively related to changes in NK cell abundance in both AMI and IS: PRKX (AMI: p > 0.05, IS: p > 0.05), PRKACB (AMI: p < 0.05, IS: p > 0.05), IRAK3 (AMI: p > 0.05, IS: p > 0.05), and ATM (AMI: p < 0.05, IS: p > 0.05). The expression of several genes also correlated negatively with NK cell abundance in AMI but positively in IS: IL1B (AMI: p < 0.05, IS: p > 0.05), CAPN1 (AMI: p < 0.05, IS: p > 0.05), and BAX (AMI: p > 0.05, IS: p > 0.05). Thus, the correlation between NK cell abundance and the expression of the genes PRKX and BAX was not significant. On the contrary, there was a significant relationship between the expression level of the genes TNFRSF1A, PRKACA, PRKACB, IRAK3, IL1R1, IL1B, CAPN1, ATM, and APAF1 and NK cell abundance. Consequently, these genes could play key roles in the relationship between NK cell abundance and apoptosis in AMI and IS.
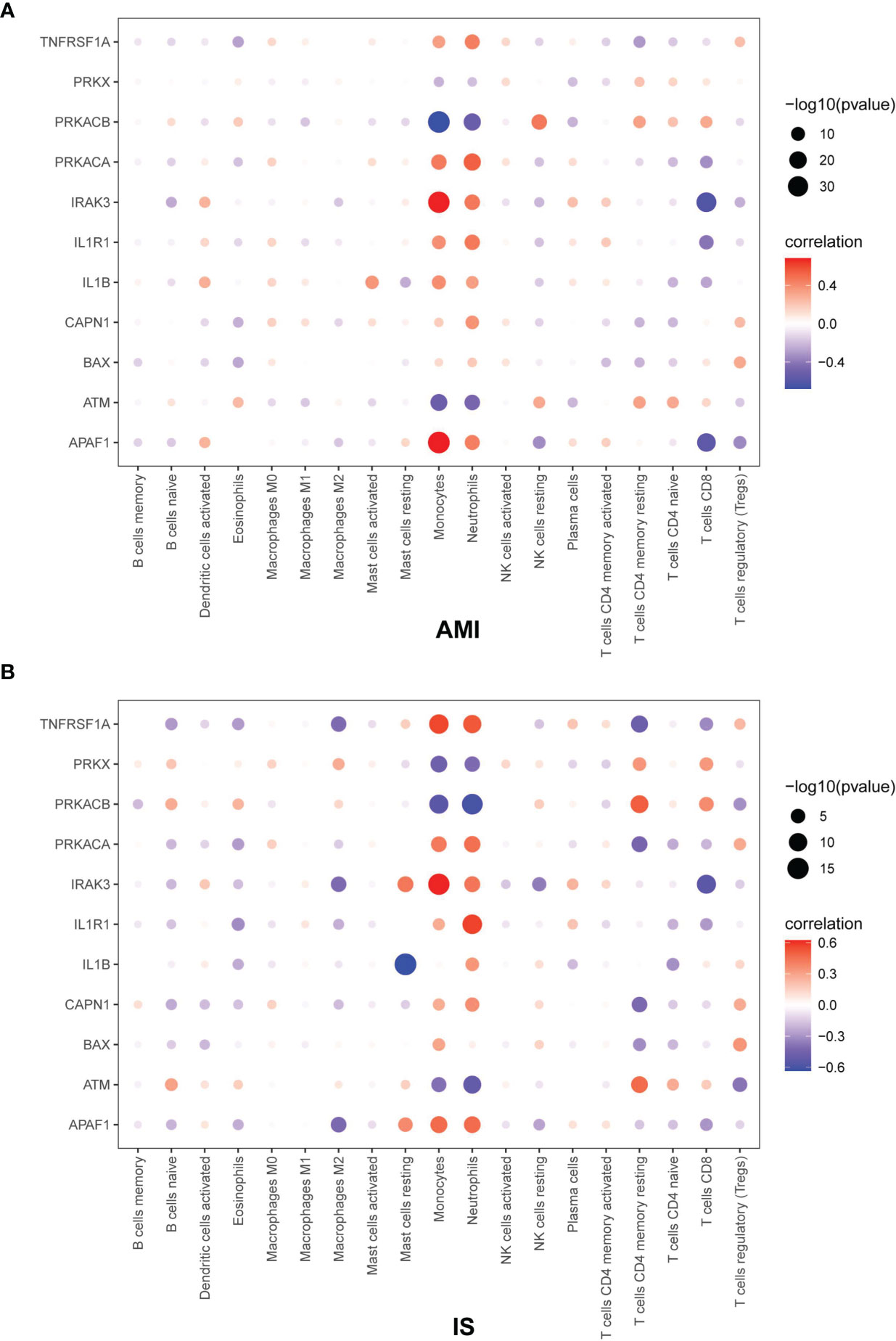
Figure 8 Pearson correlation coefficient between immune cells and apoptosis-related genes in (A) AMI and (B) IS. The Pearson correlation coefficient was determined by Pearson correlation analysis and the P-values were calculated by t-test.
Discussion
Since cardiovascular and cerebrovascular diseases are the leading causes of death worldwide (1), with a high prevalence that increases with age, new approaches to improve cardiac healing are urgently required. Recent studies have highlighted the crucial role that the immune system plays in orchestrating angiogenesis, the resolution of inflammation, the regeneration of injured tissues, and post-infarction tissue remodeling after ischemic injury, including AMI and IS (5, 32). However, inflammation can also facilitate cell death in target organs and infarct expansion, thereby determining the degree of injury and course of the disease. Immune cells can remove necrotic cell debris and produce a milieu that is vital for the reconstruction of the extracellular matrix, neo-vascularization, and subsequent organ recovery. In particular, NK cells possess both direct and indirect cytotoxic effector mechanisms (33). In addition to the exocytosis of lytic granules, activated NK cells can mediate apoptosis by expressing TRAIL and/or Fas ligand (FasL) to engage TRAIL-R1/-R2 or CD95/Fas, respectively, on the surface of diseased cells (33). Moreover, NK cells can interact with inflammatory macrophages via the IFN-γ/TNF-α/IL-12 cytokine axis in order to potentiate one another’s activity and increase inflammation in the infarcted zone (6). However, it has also been reported that NK deficiency during AMI is correlated with reduced cardiac cell apoptosis in post-infarcted myocardium (34). In this study, we compared the different expression patterns of genes in AMI and IS. And according to the results of GO and KEGG enrichment, the DEGs were mainly enriched in activities of immune cells and related to the process of cell death. Consequently, we compared the patterns of immune cell infiltration and cell death in patients with AMI or IS. Surprisingly, the alterations in NK cell abundance were significantly different between AMI and IS, while other cell types showed no or smaller differences. Moreover, apoptosis was only statistically significant in IS. After the analyses of the correlation between immune infiltration and cell death as well as the relationship between NK cell abundance and apoptosis related genes. We confirmed for the first time that the proportion of NK cells and the activation of apoptosis are highly correlated. This observation may be partially explained by apoptosis-related genes whose expression clearly correlated with NK cell abundance in AMI and IS.
Tumor necrosis factor (TNF) receptor superfamily member 1A (TNFRSF1A) is a member of the TNF superfamily that encodes the type 1 TNF receptor (TNFR1, TNFRSF1A, p55, CD120A) (35). In this study, we found that it correlated negatively with NK cell abundance in both AMI and IS. Moreover, it was upregulated in these diseases. Heterozygous mutations in TNFRSF1A on chromosome 12p13 have been shown to induce TNF receptor-associated periodic syndrome (TRAPS), a rare dominantly inherited disease characterized by recurrent episodes of fever and generalized/localized inflammation (35, 36). Others have also found an association between the R92Q polymorphism of TNFRSF1A and the development of atherosclerosis as well as inconsistency between the presence of the R92Q in patients with AMI and IS and disease occurrence (37). TNFRSF1A has also been shown to mediate the protective role of cerebellar fastigial nucleus stimulation in reducing the severity of post-stroke depression (38). Together, these findings and researches of others indicate that TNFRSF1A may present genetic changes or polymorphism, thereby leading to different patterns of NK cell infiltration and apoptosis in AMI and IS.
Protein kinase CAMP-activated catalytic subunit alpha (PRKACA) encodes the PKA catalytic subunit alpha (Cα) isoform which has three alternately spliced transcripts in humans: Cα1, Cα2 (Cαs), and Cα3 (39). PRKACB is an important paralog of this gene that encodes Cβ of PKA. The targeted deletion of PRKACA in mice has been found to result in growth retardation in those that survive, while Cα deficiency has been linked to spinal neural tube defects (40, 41). PRKACA has also been reported to be involved in various cancers (42–45). The functions of PRKACA and PRKACB are profoundly associated with those of PKA, a serine/threonine kinase that is responsible for phosphorylating various downstream substrates. Here, we found that PRKACA expression was elevated after IS but not AMI and correlated positively with the abundance of NK cells in IS but negatively with their abundance in AMI. In contrast, PRKACB was downregulated after AMI but not IS and correlated positively with the abundance of NK cells in both AMI and IS. It has been reported that Cα but not Cβ is required for normal immune reactivity, demonstrating that PKA catalytic subunits can exert isoform-specific functions in the same cell (46). However, whether the isoform-specific functions also exist in NK cells or target organ cells during AMI and IS remains unclear.
Interleukin 1 receptor associated kinase 3 (IRAK3) is a member of the IRAK family that encodes IRAK-M, which is only expressed in human monocytes and macrophages in an inducible manner (47–49). IRAK3 has been reported to negatively regulate TLR signaling (50) as well as the alternative NF-κB pathway in a TLR2-specific manner (51). Moreover, silencing IRAK3 impedes cardiac rupture and ventricular remodeling by inactivating the NF-κB signaling pathway (52), while IRAK-M deficiency was shown to increase infarct volume, exacerbate brain edema, elevate the incidence of hemorrhage transformation, and increase inflammatory responses (53). Downregulation of IRAK3 may lead to lesions in the heart and brain caused by hypoxia injury through inflammatory pathways. In our study, we found that IRAK3 was downregulated in both AMI and IS, and correlated negatively with the changes in NK cell abundance.
Interleukin 1 receptor type 1 (IL1R1) belongs to the interleukin 1 receptor family, whose members sense the barrier integrity and fitness of cells (54). IL-1R1 mediates IL-1-dependent activation via IL-1α and IL-1β (55). In addition, IL-1R1 has a soluble isoform that inhibits the interaction of soluble IL-1 ligands with IL-1R1 (56), which regulates post-MI remodeling via the IL-1R1/cardiac fibroblast signaling axis (57). Brain endothelial and neuronal (cholinergic) IL-1R1 has also been shown to mediate the detrimental effects of IL-1 in the brain during an ischemic stroke (58). In the present study, we showed that IL1R1 was upregulated in both diseases and correlated negatively with NK cell abundance in both AMI and IS. Our findings point to the possibility of different IL1R isoforms being involved in AMI and IS.
Interleukin 1 beta (IL1β) is a central mediator of innate immunity and inflammation (59) that was first identified as being responsible for resistance against microbes (60). IL-1β is produced by various types of cells, including monocytes, macrophages, skin dendritic cells, and brain microglia, in response to Toll-like receptors (TLR), activated complement components, other cytokines (e.g., TNF-α), and IL-1 itself (61). The active form of IL-1β is released into the extracellular space when its precursor is cleaved by caspase-1, which requires proenzyme (procaspase-1) cleavage by the inflammasome. IL1β has been strongly associated with cancers (62, 63) and one IL-1β haplotype has been linked to the risk of stroke in small vessels (64). In this study, we found that IL1β was positively correlated with the abundance of NK cells in IS, but negatively correlated with their abundance in AMI.
Calpain 1 (CAPN1) is a member of the calpain family of at least 15 enzymes (65), among which calpain-1 (μ-calpain) and calpain-2 (m-calpain) are the most ubiquitous in all tissues and organs. Activated calpain-1 may exert neuroprotective effects by cleaving PHLPP1, which activates Akt and leads to neuronal survival (66, 67). In addition, CAPN1 mutations have been reported to induce cerebellar ataxia in some human families and missense mutations in calpain-1 have been found to cause spinocerebellar ataxia in dogs (66, 68, 69). Although some studies have documented that calpains can play crucial roles in ischemia/reperfusion injury in the heart and other organs (70–74), few studies have investigated the role of calpain-1 in AMI and IS. Here, we demonstrated that CAPN1 was upregulated after IS but not in AMI, and correlated positively with the abundance of NK cells in IS but negatively with their abundance in AMI.
Ataxia telangiectasia mutated (ATM) is a protein-coding gene that causes the autosomal recessive disease, ataxia telangiectasia. ATM kinases play key roles in orchestrating signaling cascades that lead to programmed cell death, such as the DNA damage response, DNA repair pathways, and cell cycle checkpoint regulation (75–81). ATM also exerts dual roles in neuronal protection during ischemic preconditioning and the promotion of neuronal death in lethal ischemic injury (82). Similarly, ATM deficiency has been observed to attenuate cardiac dysfunction early post-MI but exacerbate cardiac remodeling during late post-MI by affecting cardiac function, fibrosis, apoptosis, and myocyte hypertrophy (83, 84). Moreover, ATM can mediate the spontaneous regression of Eμ-myc-driven murine B-cell leukemia in a natural killer and T cell-dependent manner (85). In this study, we found that the apoptosis-related gene ATM was significantly downregulated both in AMI and IS. Further analysis revealed that ATM correlated positively with the abundance of NK cells in AMI but not in IS, consistent with our hypothesis that ATM is linked with apoptosis in AMI and IS via its effects on NK cell abundance.
Apoptotic peptidase activating factor 1(APAF1) is a key component of the apoptosome which also contains cytochrome c, (d)ATP, and procaspase-9 (86). Under normal conditions, Apaf-1 is stored in an inactive monomeric form in the cytosol. Intracellular stressors such as hypoxia, growth factor deprivation, cell detachment, and stress signals, cause cytochrome c to be released from the mitochondrial intermembrane space into the cytosol where it interacts with and activates Apaf-1, leading to apoptosome formation (86). Apaf-1 has been reported to mediate the beneficial effect of miR-136 during aging (87) and interventions targeting Apaf-1 have demonstrated protective effects in AMI and IS (88–90). Here, we observed a negative correlation between APAF1 expression and changes in NK cell abundance in AMI and IS.
In summary, we hypothesize that apoptosis-related DEGs including TNFRSF1A, PRKACB, PRKACA, IRAK3, IL1R1, IL1B, CAPN1, ATM, and APAF1, together with their correlations with NK cell abundance, likely play important roles in mechanisms underlying the distinct patterns of apoptosis in AMI and IS. However, the function of these genes in NK cells and whether they play different roles in AMI and IS remain to be investigated. Thus, our findings provide potential avenues for the further elucidation of pathogenic mechanisms of ischemic injury in both the heart and brain, as well as promising therapeutic targets for the clinical treatment of AMI and IS.
Data Availability Statement
Publicly available datasets were analyzed in this study. This data can be found here: http://www.ncbi.nlm.nih.gov/geo.
Author Contributions
Study design, WY, LL, and YS. Writing—original draft preparation, LF, RT, and XM. Writing—review and editing, CC, YZ, JC, YJS, YL, MZ, and LS. Supervision, WY and LL. All authors contributed to the article and approved the submitted version.
Funding
This work was supported by the National Natural Science Foundation of China (grants no. 81770345 and 81870266) and the National High Level Talents Special Support Plan.
Conflict of Interest
The authors declare that the research was conducted in the absence of any commercial or financial relationships that could be construed as a potential conflict of interest.
Publisher’s Note
All claims expressed in this article are solely those of the authors and do not necessarily represent those of their affiliated organizations, or those of the publisher, the editors and the reviewers. Any product that may be evaluated in this article, or claim that may be made by its manufacturer, is not guaranteed or endorsed by the publisher.
Supplementary Material
The Supplementary Material for this article can be found online at: https://www.frontiersin.org/articles/10.3389/fimmu.2022.817377/full#supplementary-material
Supplementary Figure 1 | GO enrichment analysis in IS. BP, biological process.
Supplementary Figure 2–3 | Pearson correlation coefficient between immune cells and cell death in (Left) AMI and (Right) IS. The Pearson correlation coefficient was determined by Pearson correlation analysis and the P-values were calculated by t-test.
Supplementary Figure 4–9 | Pearson correlation coefficient between immune cells and apoptosis-related genes in (Left) AMI and (Right) IS. The Pearson correlation coefficient was determined by Pearson correlation analysis and the P-values were calculated by t-test.
References
1. Mozaffarian D, Benjamin EJ, Go AS, Arnett DK, Blaha MJ, Cushman M, et al. Heart Disease and Stroke Statistics–2015 Update: A Report From the American Heart Association. Circulation (2015) 131(4):e29–322. doi: 10.1161/cir.0000000000000152
2. Virani SS, Alonso A, Aparicio HJ, Benjamin EJ, Bittencourt MS, Callaway CW, et al. Heart Disease and Stroke Statistics-2021 Update: A Report From the American Heart Association. Circulation (2021) 143(8):e254–743. doi: 10.1161/cir.0000000000000950
3. Jian Z, Liu R, Zhu X, Smerin D, Zhong Y, Gu L, et al. The Involvement and Therapy Target of Immune Cells After Ischemic Stroke. Front Immunol (2019) 10:2167. doi: 10.3389/fimmu.2019.02167
4. Kologrivova I, Shtatolkina M, Suslova T, Ryabov V. Cells of the Immune System in Cardiac Remodeling: Main Players in Resolution of Inflammation and Repair After Myocardial Infarction. Front Immunol (2021) 12:664457. doi: 10.3389/fimmu.2021.664457
5. Chen C, Ai QD, Chu SF, Zhang Z, Chen NH. NK Cells in Cerebral Ischemia. BioMed Pharmacother (2019) 109:547–54. doi: 10.1016/j.biopha.2018.10.103
6. Knorr M, Münzel T, Wenzel P. Interplay of NK Cells and Monocytes in Vascular Inflammation and Myocardial Infarction. Front Physiol (2014) 5:295. doi: 10.3389/fphys.2014.00295
7. Ong S, Rose NR, Čiháková D. Natural Killer Cells in Inflammatory Heart Disease. Clin Immunol (2017) 175:26–33. doi: 10.1016/j.clim.2016.11.010
8. Jonasson L, Backteman K, Ernerudh J. Loss of Natural Killer Cell Activity in Patients With Coronary Artery Disease. Atherosclerosis (2005) 183(2):316–21. doi: 10.1016/j.atherosclerosis.2005.03.011
9. Li W, Lidebjer C, Yuan XM, Szymanowski A, Backteman K, Ernerudh J, et al. NK Cell Apoptosis in Coronary Artery Disease: Relation to Oxidative Stress. Atherosclerosis (2008) 199(1):65–72. doi: 10.1016/j.atherosclerosis.2007.10.031
10. Galluzzi L, Vitale I, Aaronson SA, Abrams JM, Adam D, Agostinis P, et al. Molecular Mechanisms of Cell Death: Recommendations of the Nomenclature Committee on Cell Death 2018. Cell Death Differ (2018) 25(3):486–541. doi: 10.1038/s41418-017-0012-4
11. Jia XF, Liang FG, Kitsis RN. Multiple Cell Death Programs Contribute to Myocardial Infarction. Circ Res (2021) 129(3):397–9. doi: 10.1161/circresaha.121.319584
12. Marunouchi T, Ito T, Onda S, Kyo L, Takahashi K, Uchida M, et al. Effects of 17-AAG on the RIP1/RIP3/MLKL Pathway During the Development of Heart Failure Following Myocardial Infarction in Rats. J Pharmacol Sci (2021) 147(2):192–9. doi: 10.1016/j.jphs.2021.06.009
13. Yu Y, Liu H, Yang D, He F, Yuan Y, Guo J, et al. Aloe-Emodin Attenuates Myocardial Infarction and Apoptosis via Up-Regulating miR-133 Expression. Pharmacol Res (2019) 146:104315. doi: 10.1016/j.phrs.2019.104315
14. Maciejak A, Kiliszek M, Michalak M, Tulacz D, Opolski G, Matlak K, et al. Gene Expression Profiling Reveals Potential Prognostic Biomarkers Associated With the Progression of Heart Failure. Genome Medicine (2015) 7(1)::26. doi: 10.1186/s13073-015-0149-z
15. Krug T, Gabriel JP, Taipa R, Fonseca BV, Domingues-Montanari S, Fernandez-Cadenas I, et al. TTC7B Emerges as a Novel Risk Factor for Ischemic Stroke Through the Convergence of Several Genome-Wide Approaches. J Cereb Blood Flow Metab (2012) 32(6):1061–72. doi: 10.1038/jcbfm.2012.24
16. Stamova B, Jickling GC, Ander BP, Zhan X, Liu D, Turner R, et al. Gene Expression in Peripheral Immune Cells Following Cardioembolic Stroke is Sexually Dimorphic. PloS one (2014) 9(7):e102550. doi: 10.1371/journal.pone.0102550
17. Suresh R, Li X, Chiriac A, Goel K, Terzic A, Perez-Terzic C, et al. Transcriptome From Circulating Cells Suggests Dysregulated Pathways Associated With Long-Term Recurrent Events Following First-Time Myocardial Infarction. J Mol Cell Cardiol (2014) 74:13–21. doi: 1016/j.yjmcc.2014.04.017
18. O’Connell GC, Treadway MB, Petrone AB, Tennant CS, Lucke-Wold N, Chantler PD, et al. Peripheral Blood AKAP7 Expression as an Early Marker for Lymphocyte-Mediated Post-Stroke Blood Brain Barrier Disruption. Sci Rep (2017) 7(1):1172. doi: 10.1038/s41598-017-01178-5
19. Leek JT, Johnson WE, Parker HS, Jaffe AE, Storey JD. The Sva Package for Removing Batch Effects and Other Unwanted Variation in High-Throughput Experiments. Bioinformatics (2012) 28(6):882–3. doi: 10.1093/bioinformatics/bts034
20. Ritchie ME, Phipson B, Wu D, Hu Y, Law CW, Shi W, et al. Limma Powers Differential Expression Analyses for RNA-Sequencing and Microarray Studies. Nucleic Acids Res (2015) 43(7):e47. doi: 10.1093/nar/gkv007
21. Giebe S, Cockcroft N, Hewitt K, Brux M, Hofmann A, Morawietz H, et al. Cigarette Smoke Extract Counteracts Atheroprotective Effects of High Laminar Flow on Endothelial Function. Redox Biol (2017) 12:776–86. doi: 10.1016/j.redox.2017.04.008
22. Yu G, Wang LG, Han Y, He QY. clusterProfiler: An R Package for Comparing Biological Themes Among Gene Clusters. OMICS (2012) 16(5):284–7. doi: 10.1089/omi.2011.0118
23. Langfelder P, Horvath S. WGCNA: An R Package for Weighted Correlation Network Analysis. BMC Bioinf (2008) 9:559. doi: 10.1186/1471-2105-9-559
24. Shannon P, Markiel A, Ozier O, Baliga NS, Wang JT, Ramage D, et al. Cytoscape: A Software Environment for Integrated Models of Biomolecular Interaction Networks. Genome Res (2003) 13(11):2498–504. doi: 10.1101/gr.1239303
25. Gentles AJ, Newman AM, Liu CL, Bratman SV, Feng W, Kim D, et al. The Prognostic Landscape of Genes and Infiltrating Immune Cells Across Human Cancers. Nat Med (2015) 21(8):938–45. doi: 10.1038/nm.3909
26. Hänzelmann S, Castelo R, Guinney J. GSVA: Gene Set Variation Analysis for Microarray and RNA-Seq Data. BMC Bioinf (2013) 14:7. doi: 10.1186/1471-2105-14-7
27. Jung K, Kim P, Leuschner F, Gorbatov R, Kim JK, Ueno T, et al. Endoscopic Time-Lapse Imaging of Immune Cells in Infarcted Mouse Hearts. Circ Res (2013) 112(6):891–9. doi: 10.1161/circresaha.111.300484
28. Lee WW, Marinelli B, van der Laan AM, Sena BF, Gorbatov R, Leuschner F, et al. PET/MRI of Inflammation in Myocardial Infarction. J Am Coll Cardiol (2012) 59(2):153–63. doi: 10.1016/j.jacc.2011.08.066
29. Chen Z, Chua CC, Ho YS, Hamdy RC, Chua BH. Overexpression of Bcl-2 Attenuates Apoptosis and Protects Against Myocardial I/R Injury in Transgenic Mice. Am J Physiol Heart Circulatory Physiol (2001) 280(5):H2313–20. doi: 10.1152/ajpheart.2001.280.5.H2313
30. Lee P, Sata M, Lefer DJ, Factor SM, Walsh K, Kitsis RN. Fas Pathway is a Critical Mediator of Cardiac Myocyte Death and MI During Ischemia-Reperfusion In Vivo. Am J Physiol Heart Circulatory Physiol (2003) 284(2):H456–63. doi: 10.1152/ajpheart.00777.2002
31. Li W, Feng G, Gauthier JM, Lokshina I, Higashikubo R, Evans S, et al. Ferroptotic Cell Death and TLR4/Trif Signaling Initiate Neutrophil Recruitment After Heart Transplantation. J Clin Invest (2019) 129(6):2293–304. doi: 10.1172/jci126428
32. Gori AM, Cesari F, Marcucci R, Giusti B, Paniccia R, Antonucci E, et al. The Balance Between Pro- and Anti-Inflammatory Cytokines is Associated With Platelet Aggregability in Acute Coronary Syndrome Patients. Atherosclerosis (2009) 202(1):255–62. doi: 10.1016/j.atherosclerosis.2008.04.001
33. Prager I, Watzl C. Mechanisms of Natural Killer Cell-Mediated Cellular Cytotoxicity. J Leukoc Biol (2019) 105(6):1319–29. doi: 10.1002/JLB.MR0718-269R
34. Boukouaci W, Lauden L, Siewiera J, Dam N, Hocine HR, Khaznadar Z, et al. Natural Killer Cell Crosstalk With Allogeneic Human Cardiac-Derived Stem/Progenitor Cells Controls Persistence. Cardiovasc Res (2014) 104(2):290–302. doi: 10.1093/cvr/cvu208
35. McDermott MF, Aksentijevich I, Galon J, McDermott EM, Ogunkolade BW, Centola M, et al. Germline Mutations in the Extracellular Domains of the 55 kDa TNF Receptor, TNFR1, Define a Family of Dominantly Inherited Autoinflammatory Syndromes. Cell (1999) 97(1):133–44. doi: 10.1016/s0092-8674(00)80721-7
36. Cudrici C, Deuitch N, Aksentijevich I. Revisiting TNF Receptor-Associated Periodic Syndrome (TRAPS): Current Perspectives. Int J Mol Sci (2020) 21(9):3263. doi: 10.3390/ijms21093263
37. Poirier O, Nicaud V, Gariépy J, Courbon D, Elbaz A, Morrison C, et al. Polymorphism R92Q of the Tumour Necrosis Factor Receptor 1 Gene is Associated With Myocardial Infarction and Carotid Intima-Media Thickness–the ECTIM, AXA, EVA and GENIC Studies. Eur J Hum Genet (2004) 12(3):213–9. doi: 10.1038/sj.ejhg.5201143
38. Wang M, Guo J, Dong LN, Wang JP. Cerebellar Fastigial Nucleus Stimulation in a Chronic Unpredictable Mild Stress Rat Model Reduces Post-Stroke Depression by Suppressing Brain Inflammation via the microRNA-29c/TNFRSF1A Signaling Pathway. Med Sci Monit (2019) 25:5594–605. doi: 10.12659/msm.911835
39. Turnham RE, Scott JD. Protein Kinase A Catalytic Subunit Isoform PRKACA; History, Function and Physiology. Gene (2016) 577(2):101–8. doi: 10.1016/j.gene.2015.11.052
40. Skålhegg BS, Huang Y, Su T, Idzerda RL, McKnight GS, Burton KA. Mutation of the Calpha Subunit of PKA Leads to Growth Retardation and Sperm Dysfunction. Mol Endocrinol (2002) 16(3):630–9. doi: 10.1210/mend.16.3.0793
41. Huang Y, Roelink H, McKnight GS. Protein Kinase A Deficiency Causes Axially Localized Neural Tube Defects in Mice. J Biol Chem (2002) 277(22):19889–96. doi: 10.1074/jbc.M111412200
42. Moody SE, Schinzel AC, Singh S, Izzo F, Strickland MR, Luo L, et al. PRKACA Mediates Resistance to HER2-Targeted Therapy in Breast Cancer Cells and Restores Anti-Apoptotic Signaling. Oncogene (2015) 34(16):2061–71. doi: 10.1038/onc.2014.153
43. Honeyman JN, Simon EP, Robine N, Chiaroni-Clarke R, Darcy DG, Lim II, et al. Detection of a Recurrent DNAJB1-PRKACA Chimeric Transcript in Fibrolamellar Hepatocellular Carcinoma. Sci (New York NY) (2014) 343(6174):1010–4. doi: 10.1126/science.1249484
44. Yang F, Xie HY, Yang LF, Zhang L, Zhang FL, Liu HY, et al. Stabilization of MORC2 by Estrogen and Antiestrogens Through GPER1- PRKACA-CMA Pathway Contributes to Estrogen-Induced Proliferation and Endocrine Resistance of Breast Cancer Cells. Autophagy (2020) 16(6):1061–76. doi: 10.1080/15548627.2019.1659609
45. Singhi AD, Wood LD, Parks E, Torbenson MS, Felsenstein M, Hruban RH, et al. Recurrent Rearrangements in PRKACA and PRKACB in Intraductal Oncocytic Papillary Neoplasms of the Pancreas and Bile Duct. Gastroenterology (2020) 158(3):573–82.e2. doi: 10.1053/j.gastro.2019.10.028
46. Funderud A, Aas-Hanssen K, Aksaas AK, Hafte TT, Corthay A, Munthe LA, et al. Isoform-Specific Regulation of Immune Cell Reactivity by the Catalytic Subunit of Protein Kinase A (PKA). Cell Signal (2009) 21(2):274–81. doi: 10.1016/j.cellsig.2008.10.013
47. Cao Z, Henzel WJ, Gao X. IRAK: A Kinase Associated With the Interleukin-1 Receptor. Sci (New York NY) (1996) 271(5252):1128–31. doi: 10.1126/science.271.5252.1128
48. Rosati O, Martin MU. Identification and Characterization of Murine IRAK-M. Biochem Biophys Res Commun (2002) 293(5):1472–7. doi: 10.1016/s0006-291x(02)00411-4
49. Flannery S, Bowie AG. The Interleukin-1 Receptor-Associated Kinases: Critical Regulators of Innate Immune Signalling. Biochem Pharmacol (2010) 80(12):1981–91. doi: 10.1016/j.bcp.2010.06.020
50. Kobayashi K, Hernandez LD, Galán JE, Janeway CA Jr., Medzhitov R, Flavell RA. IRAK-M is a Negative Regulator of Toll-Like Receptor Signaling. Cell (2002) 110(2):191–202. doi: 10.1016/s0092-8674(02)00827-9
51. Su J, Zhang T, Tyson J, Li L. The Interleukin-1 Receptor-Associated Kinase M Selectively Inhibits the Alternative, Instead of the Classical NFkappaB Pathway. J Innate Immun (2009) 1(2):164–74. doi: 10.1159/000158541
52. Ge ZW, Wang BC, Hu JL, Sun JJ, Wang S, Chen XJ, et al. IRAK3 Gene Silencing Prevents Cardiac Rupture and Ventricular Remodeling Through Negative Regulation of the NF-kappaB Signaling Pathway in a Mouse Model of Acute Myocardial Infarction. J Cell Physiol (2019) 234(7):11722–33. doi: 10.1002/jcp.27827
53. Lyu C, Zhang Y, Gu M, Huang Y, Liu G, Wang C, et al. IRAK-M Deficiency Exacerbates Ischemic Neurovascular Injuries in Experimental Stroke Mice. Front Cell Neurosci (2018) 12:504. doi: 10.3389/fncel.2018.00504
54. Boraschi D, Italiani P, Weil S, Martin MU. The Family of the Interleukin-1 Receptors. Immunol Rev (2018) 281(1):197–232. doi: 10.1111/imr.12606
55. Sims JE, March CJ, Cosman D, Widmer MB, MacDonald HR, McMahan CJ, et al. cDNA Expression Cloning of the IL-1 Receptor, a Member of the Immunoglobulin Superfamily. Sci (New York NY) (1988) 241(4865):585–9. doi: 10.1126/science.2969618
56. Svenson M, Hansen MB, Heegaard P, Abell K, Bendtzen K. Specific Binding of Interleukin 1 (IL-1) Beta and IL-1 Receptor Antagonist (IL-1ra) to Human Serum. High-Affinity Binding of IL-1ra to Soluble IL-1 Receptor Type I. Cytokine (1993) 5(5):427–35. doi: 10.1016/1043-4666(93)90032-z
57. Bageghni SA, Hemmings KE, Yuldasheva NY, Maqbool A, Gamboa-Esteves FO, Humphreys NE, et al. Fibroblast-Specific Deletion of Interleukin-1 Receptor-1 Reduces Adverse Cardiac Remodeling Following Myocardial Infarction. JCI Insight (2019) 5(17):e125074. doi: 10.1172/jci.insight.125074
58. Wong R, Lénárt N, Hill L, Toms L, Coutts G, Martinecz B, et al. Interleukin-1 Mediates Ischaemic Brain Injury via Distinct Actions on Endothelial Cells and Cholinergic Neurons. Brain Behav Immun (2019) 76:126–38. doi: 10.1016/j.bbi.2018.11.012
59. Garlanda C, Dinarello CA, Mantovani A. The Interleukin-1 Family: Back to the Future. Immunity (2013) 39(6):1003–18. doi: 10.1016/j.immuni.2013.11.010
60. van der Meer JW, Barza M, Wolff SM, Dinarello CA. A Low Dose of Recombinant Interleukin 1 Protects Granulocytopenic Mice From Lethal Gram-Negative Infection. Proc Natl Acad Sci U States America (1988) 85(5):1620–3. doi: 10.1073/pnas.85.5.1620
61. Dinarello CA. Interleukin-1 in the Pathogenesis and Treatment of Inflammatory Diseases. Blood (2011) 117(14):3720–32. doi: 10.1182/blood-2010-07-273417
62. Krelin Y, Voronov E, Dotan S, Elkabets M, Reich E, Fogel M, et al. Interleukin-1beta-Driven Inflammation Promotes the Development and Invasiveness of Chemical Carcinogen-Induced Tumors. Cancer Res (2007) 67(3):1062–71. doi: 10.1158/0008-5472.can-06-2956
63. Carmi Y, Dotan S, Rider P, Kaplanov I, White MR, Baron R, et al. The Role of IL-1β in the Early Tumor Cell-Induced Angiogenic Response. J Immunol (2013) 190(7):3500–9. doi: 10.4049/jimmunol.1202769
64. Bis JC, Heckbert SR, Smith NL, Reiner AP, Rice K, Lumley T, et al. Variation in Inflammation-Related Genes and Risk of Incident Nonfatal Myocardial Infarction or Ischemic Stroke. Atherosclerosis (2008) 198(1):166–73. doi: 10.1016/j.atherosclerosis.2007.09.031
65. Macqueen DJ, Wilcox AH. Characterization of the Definitive Classical Calpain Family of Vertebrates Using Phylogenetic, Evolutionary and Expression Analyses. Open Biol (2014) 4(4):130219. doi: 10.1098/rsob.130219
66. Wang Y, Briz V, Chishti A, Bi X, Baudry M. Distinct Roles for μ-Calpain and M-Calpain in Synaptic NMDAR-Mediated Neuroprotection and Extrasynaptic NMDAR-Mediated Neurodegeneration. J Neurosci (2013) 33(48):18880–92. doi: 10.1523/jneurosci.3293-13.2013
67. Wang Y, Hersheson J, Lopez D, Hammer M, Liu Y, Lee KH, et al. Defects in the CAPN1 Gene Result in Alterations in Cerebellar Development and Cerebellar Ataxia in Mice and Humans. Cell Rep (2016) 16(1):79–91. doi: 10.1016/j.celrep.2016.05.044
68. Shetty A, Gan-Or Z, Ashtiani S, Ruskey JA, van de Warrenburg B, Wassenberg T, et al. CAPN1 Mutations: Expanding the CAPN1-Related Phenotype: From Hereditary Spastic Paraparesis to Spastic Ataxia. Eur J Med Genet (2019) 62(12):103605. doi: 10.1016/j.ejmg.2018.12.010
69. Forman OP, De Risio L, Mellersh CS. Missense Mutation in CAPN1 is Associated With Spinocerebellar Ataxia in the Parson Russell Terrier Dog Breed. PloS One (2013) 8(5):e64627. doi: 10.1371/journal.pone.0064627
70. Iwamoto H, Miura T, Okamura T, Shirakawa K, Iwatate M, Kawamura S, et al. Calpain Inhibitor-1 Reduces Infarct Size and DNA Fragmentation of Myocardium in Ischemic/Reperfused Rat Heart. J Cardiovasc Pharmacol (1999) 33(4):580–6. doi: 10.1097/00005344-199904000-00010
71. Urthaler F, Wolkowicz PE, Digerness SB, Harris KD, Walker AA. MDL-28170, a Membrane-Permeant Calpain Inhibitor, Attenuates Stunning and PKC Epsilon Proteolysis in Reperfused Ferret Hearts. Cardiovasc Res (1997) 35(1):60–7. doi: 10.1016/s0008-6363(97)00099-0
72. Gao G, Dou QP. N-Terminal Cleavage of Bax by Calpain Generates a Potent Proapoptotic 18-kDa Fragment That Promotes Bcl-2-Independent Cytochrome C Release and Apoptotic Cell Death. J Cell Biochem (2000) 80(1):53–72. doi: 10.1002/1097-4644(20010101)80:1<53::aid-jcb60>3.0.co;2-e
73. Kohli V, Madden JF, Bentley RC, Clavien PA. Calpain Mediates Ischemic Injury of the Liver Through Modulation of Apoptosis and Necrosis. Gastroenterology (1999) 116(1):168–78. doi: 10.1016/s0016-5085(99)70241-6
74. Liu X, Rainey JJ, Harriman JF, Schnellmann RG. Calpains Mediate Acute Renal Cell Death: Role of Autolysis and Translocation. Am J Physiol Renal Physiol (2001) 281(4):F728–38. doi: 10.1152/ajprenal.2001.281.4.F728
75. Matsuoka S, Huang M, Elledge SJ. Linkage of ATM to Cell Cycle Regulation by the Chk2 Protein Kinase. Sci (New York NY) (1998) 282(5395):1893–7. doi: 10.1126/science.282.5395.1893
76. Lee JS, Collins KM, Brown AL, Lee CH, Chung JH. hCds1-Mediated Phosphorylation of BRCA1 Regulates the DNA Damage Response. Nature (2000) 404(6774):201–4. doi: 10.1038/35004614
77. Yang S, Kuo C, Bisi JE, Kim MK. PML-Dependent Apoptosis After DNA Damage is Regulated by the Checkpoint Kinase Hcds1/Chk2. Nat Cell Biol (2002) 4(11):865–70. doi: 10.1038/ncb869
78. Bakkenist CJ, Kastan MB. DNA Damage Activates ATM Through Intermolecular Autophosphorylation and Dimer Dissociation. Nature (2003) 421(6922):499–506. doi: 10.1038/nature01368
79. Lukas C, Falck J, Bartkova J, Bartek J, Lukas J. Distinct Spatiotemporal Dynamics of Mammalian Checkpoint Regulators Induced by DNA Damage. Nat Cell Biol (2003) 5(3):255–60. doi: 10.1038/ncb945
80. Roos WP, Kaina B. DNA Damage-Induced Cell Death by Apoptosis. Trends Mol Med (2006) 12(9):440–50. doi: 10.1016/j.molmed.2006.07.007
81. Guo QQ, Wang SS, Zhang SS, Xu HD, Li XM, Guan Y, et al. ATM-CHK2-Beclin 1 Axis Promotes Autophagy to Maintain ROS Homeostasis Under Oxidative Stress. EMBO J (2020) 39(10):e103111. doi: 10.15252/embj.2019103111
82. Xie GH, Dai HJ, Liu F, Zhang YP, Zhu L, Nie JJ, et al. A Dual Role of ATM in Ischemic Preconditioning and Ischemic Injury. Cell Mol Neurobiol (2020) 40(5):785–99. doi: 10.1007/s10571-019-00773-6
83. Daniel LL, Daniels CR, Harirforoosh S, Foster CR, Singh M, Singh K. Deficiency of Ataxia Telangiectasia Mutated Kinase Delays Inflammatory Response in the Heart Following Myocardial Infarction. J Am Heart Assoc (2014) 3(6):e001286. doi: 10.1161/jaha.114.001286
84. Daniel LL, Scofield SL, Thrasher P, Dalal S, Daniels CR, Foster CR, et al. Ataxia Telangiectasia-Mutated Kinase Deficiency Exacerbates Left Ventricular Dysfunction and Remodeling Late After Myocardial Infarction. Am J Physiol Heart Circulatory Physiol (2016) 311(2):H445–52. doi: 10.1152/ajpheart.00338.2016
85. Tang ML, Gasser S. ATM Activation Mediates Anticancer Immunosurveillance by Natural Killer and T Cells. Oncoimmunology (2013) 2(6):e24438. doi: 10.4161/onci.24438
86. Shakeri R, Kheirollahi A, Davoodi J. Contribution of Apaf-1 to the Pathogenesis of Cancer and Neurodegenerative Diseases. Biochimie (2021) 190:91–110. doi: 10.1016/j.biochi.2021.07.004
87. Zhang N, Zhu J, Ma Q, Zhao Y, Wang Y, Hu X, et al. Exosomes Derived From Human Umbilical Cord MSCs Rejuvenate Aged MSCs and Enhance Their Functions for Myocardial Repair. Stem Cell Res Ther (2020) 11(1):273. doi: 10.1186/s13287-020-01782-9
88. Gao Y, Liang W, Hu X, Zhang W, Stetler RA, Vosler P, et al. Neuroprotection Against Hypoxic-Ischemic Brain Injury by Inhibiting the Apoptotic Protease Activating Factor-1 Pathway. Stroke (2010) 41(1):166–72. doi: 10.1161/strokeaha.109.561852
89. Chen Q, Xu J, Li L, Li H, Mao S, Zhang F, et al. MicroRNA-23a/B and microRNA-27a/B Suppress Apaf-1 Protein and Alleviate Hypoxia-Induced Neuronal Apoptosis. Cell Death Dis (2014) 5(3):e1132. doi: 10.1038/cddis.2014.92
Keywords: natural killer cell, apoptosis, myocardial infarction, ischemic stroke, gene expression
Citation: Feng L, Tian R, Mu X, Chen C, Zhang Y, Cui J, Song Y, Liu Y, Zhang M, Shi L, Sun Y, Li L and Yi W (2022) Identification of Genes Linking Natural Killer Cells to Apoptosis in Acute Myocardial Infarction and Ischemic Stroke. Front. Immunol. 13:817377. doi: 10.3389/fimmu.2022.817377
Received: 18 November 2021; Accepted: 11 March 2022;
Published: 01 April 2022.
Edited by:
Marina Cella, Washington University in St. Louis, United StatesReviewed by:
Jeanette Villanueva, Victor Chang Cardiac Research Institute, AustraliaNoha Mousaad Elemam, University of Sharjah, United Arab Emirates
Copyright © 2022 Feng, Tian, Mu, Chen, Zhang, Cui, Song, Liu, Zhang, Shi, Sun, Li and Yi. This is an open-access article distributed under the terms of the Creative Commons Attribution License (CC BY). The use, distribution or reproduction in other forums is permitted, provided the original author(s) and the copyright owner(s) are credited and that the original publication in this journal is cited, in accordance with accepted academic practice. No use, distribution or reproduction is permitted which does not comply with these terms.
*Correspondence: Yang Sun, ZHJfeWFuZ3N1bkAxNjMuY29t; Ling Li, bGlsaW5nMjVAZm1tdS5lZHUuY24=; Wei Yi, eWl3ZWlAZm1tdS5lZHUuY24=
†These authors have contributed equally to this work