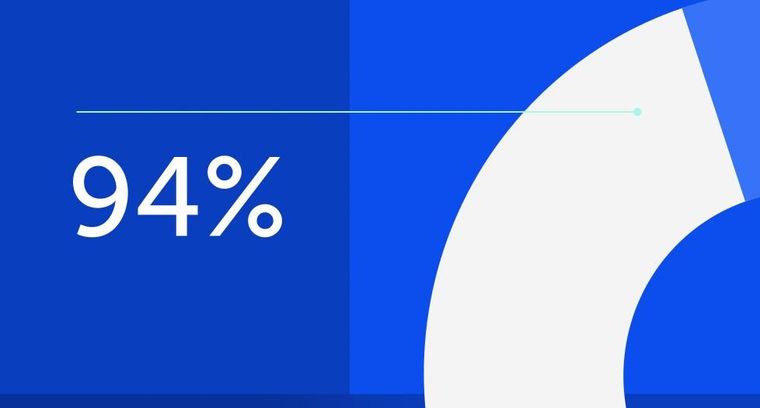
94% of researchers rate our articles as excellent or good
Learn more about the work of our research integrity team to safeguard the quality of each article we publish.
Find out more
ORIGINAL RESEARCH article
Front. Immunol., 21 January 2022
Sec. Systems Immunology
Volume 13 - 2022 | https://doi.org/10.3389/fimmu.2022.801639
Background: Human papillomavirus-positive (HPV+) cervical cancers are highly heterogeneous in molecular and clinical features. However, the molecular classification of HPV+ cervical cancers remains insufficiently unexplored.
Methods: Based on the expression profiles of 50 genes having the largest expression variations across the HPV+ cervical cancers in the TCGA-CESC dataset, we hierarchically clustered HPV+ cervical cancers to identify new subtypes. We further characterized molecular, phenotypic, and clinical features of these subtypes.
Results: We identified two subtypes of HPV+ cervical cancers, namely HPV+G1 and HPV+G2. We demonstrated that this classification method was reproducible in two validation sets. Compared to HPV+G2, HPV+G1 displayed significantly higher immune infiltration level and stromal content, lower tumor purity, lower stemness scores and intratumor heterogeneity (ITH) scores, higher level of genomic instability, lower DNA methylation level, as well as better disease-free survival prognosis. The multivariate survival analysis suggests that the disease-free survival difference between both subtypes is independent of confounding variables, such as immune signature, stemness, and ITH. Pathway and gene ontology analysis confirmed the more active tumor immune microenvironment in HPV+G1 versus HPV+G2.
Conclusions: HPV+ cervical cancers can be classified into two subtypes based on the expression profiles of the 50 genes with the largest expression variations across the HPV+ cervical cancers. Both subtypes have significantly different molecular, phenotypic, and clinical features. This new subtyping method captures the comprehensive heterogeneity in molecular and clinical characteristics of HPV+ cervical cancers and provides potential clinical implications for the diagnosis and treatment of this disease.
Cervical cancer is the most common gynecological malignancy (1), of which around 90% are squamous cell carcinomas and 10% are adenocarcinomas. Infection by the human papillomavirus (HPV) is the major risk factor for cervical cancer, and about 70% of HPV-related cervical cancer is caused by HPV-16 or HPV-18 (2). Furthermore, previous studies have revealed that HPV-18 infection is an adverse prognostic parameter and that HPV-16 infection has no significant association with survival prognosis in cervical cancer (3). Most of cervical squamous cell carcinoma (CESC) patients are HPV-positive, while about 25% of cervical adenocarcinoma patients are HPV-negative (4). Previous studies have revealed that cervical cancer is highly heterogeneous in clinical and molecular profiles (5). The Cancer Genome Atlas (TCGA) network grouped cervical cancer into four subtypes: HPV clade A9, A7, HPV-negative, and other (5). Besides, TCGA identified three subtypes of cervical cancer, namely keratin-low squamous, keratin-high squamous, and adenocarcinoma-rich by an integrative analysis of copy number, methylation, mRNA and microRNA profiles (5). In addition, TCGA identified three clusters of cervical cancer by reverse phase protein array (RPPA) analysis of 155 samples with 192 antibodies; these clusters included hormone, epithelial-mesenchymal transition (EMT), and PI3K-AKT, of which the EMT cluster showed the worst five-year overall survival outcome (5).
Currently, surgery, chemotherapy and radiotherapy are three major therapeutic options for cervical cancer, although they have limited efficiency for advanced or recurrent cervical cancers (6). Recently, immunotherapies, particularly immune checkpoint inhibitors (ICIs) (7), exhibit efficiency for various solid tumors, such as melanoma, lung cancer, head and neck cancer, kidney cancer, bladder cancer, triple-negative breast cancer, cervical cancer, liver cancer, prostate cancer, and gastrointestinal cancers with mismatch repair deficiency (dMMR). ICIs can induce the regression of certain virus infection-related epithelial malignancies, such as HPV-related cervical (8), head and neck (9), and anal (10) cancers. In fact, the use of ICIs for treating recurrent or metastatic cervical cancers has recently approved by FDA, although less than 20% of cancer patients have an active response to ICIs.
In this study, we identified subtypes of HPV-positive (HPV+) cervical cancers based on gene expression profiles in cervical cancers. Furthermore, we compared molecular and clinical features between the HPV+ cervical cancer subtypes. We also compared molecular features between HPV+ and HPV-negative (HPV-) cervical cancers. This study aimed to explore a new subtyping method for HPV+ cervical cancers and provide potential clinical implications for the diagnosis and treatment of HPV+ cervical cancers.
We downloaded three datasets of gene expression profiles in cervical cancer, including TCGA-CESC (5), GSE29570 (11), and GSE39001 (12). The TCGA-CESC dataset was from the genomic data commons (GDC) data portal (https://portal.gdc.cancer.gov/), and GSE30784 and GSE39366 were from the NCBI gene expression omnibus (https://www.ncbi.nlm.nih.gov/geo/). From GDC, we also downloaded the protein expression, somatic mutation, and somatic copy number alteration (CNA) profiles and clinical data in TCGA-CESC. We took TCGA-CESC as the discovery set and performed main analyses in this dataset. The other two datasets were validation sets. These datasets contained only data of human mRNA expression, but not data of the expression of infecting HPV variants. The data on the mRNA expression of HPV alpha-7 and alpha-9 clades were not available in any of these datasets. A summary of these datasets is shown in Table 1.
We first identified 50 genes having the largest expression variations across the HPV+ cervical cancers in TCGA-CESC. Based on the expression profiles of the 50 genes, we performed the hierarchical clustering of the HPV+ cervical cancers in the three datasets, respectively.
We quantified the enrichment level of an immune signature or phenotypic feature in a tumor sample by the single-sample gene-set enrichment analysis (ssGSEA) of its marker gene set (13). The ssGSEA calculates the enrichment score of a gene set in a sample based on its expression profiles. The ratios of immunostimulatory/immunosuppressive signatures were the base-2 log-transformed values of the geometric mean expression levels of all marker genes of immunostimulatory signatures divided by those of immunosuppressive signatures. The marker gene sets of immune signatures or phenotypic features are shown in Supplementary Table S1.
We first identified the genes differentially expressed between two classes of samples using the Student’s t test with a threshold of adjusted P value < 0.05 and fold change of their mean expression levels > 2. By inputting the differentially expressed genes into the GSEA web tool (14), we identified KEGG (15) pathways highly enriched in one class versus another class using a threshold of adjusted P value < 0.05. We used the weighted gene co-expression network analysis (WGCNA) (16) to identify gene modules significantly enriched in subtypes. Based on the expression correlations between the gene modules’ hub genes, we identified GO terms having significant correlations with specific trait by WGCNA.
We used the ESTIMATE algorithm (17) to calculate tumor immune score, stromal score, and tumor purity based on gene expression profiles. The immune score, stromal score, and tumor purity indicate the level of tumor-infiltrating lymphocytes, proportion of stromal component, and proportion of tumor cells in a bulk tumor sample. The stemness score was calculated by the ssGSEA of its marker gene set (18) and defined the level of tumor stem cell-like phenotypic feature. We evaluated the ITH level by the DEPTH algorithm (19), which quantifies ITH based on transcriptomic alterations in the tumor.
We used Kaplan–Meier curves to display the survival time difference between cervical cancer subtypes, whose significance was evaluated by the log-rank test. The function “survfit” in the R package “survival” was utilized to perform this analysis. In addition, we performed multivariate survival analysis to investigate the correlation between cervical cancer subtypes and survival prognosis after adjusting for confounding variables, including immune score, stemness score, and ITH score. All these variables are continuous variables. The Cox proportional hazards model was utilized to implement the multivariate survival analysis with the function “coxph” in the R package “survival”.
The TMB was defined as the total number of somatic mutations in the tumor. We used GISTIC2 (20) to calculate G-scores for cervical cancer subtypes. The G-score represents the amplitude of the CNA and the frequency of its occurrence across a group of samples (20).
We utilized the Random Forest (RF) algorithm (21) to predict cervical cancer subtypes. In the RF, the number of trees was set to 500, and the features included the 50 genes with the largest expression variations across the HPV+ cervical cancers in TCGA-CESC. We reported sensitivity, specificity, and area under the receiver operating characteristic curve (AUC) to evaluate the prediction performance. The R package “randomForest” was utilized to perform the RF algorithm. The R package “PreHPVcc” for predicting HPV+ cervical cancer subtypes is available in the GitHub repository (https://github.com/WangX-Lab/PreHPVcc).
In class comparisons, we used the Mann–Whitney U test or Kruskal–Wallis (K–W) test for not normally distributed data (Shapiro test, P < 0.05) and Student’s t test or one-way analysis of variance (ANOVA) test for normally distributed data. We utilized the chi-square test to analyze contingency tables. We used the Benjamini-Hochberg method (22) to adjust for P values in multiple tests. We performed all statistical analyses in the R programming environment (version 4.0.2).
We hierarchically clustered HPV+ cervical cancers based on the expression profiles of 50 genes which had the largest expression variations across the HPV+ cervical cancers. We obtained two clear clusters, termed HPV+G1 and HPV+G2 (Figure 1A). We confirmed that this classification was reproducible in two other datasets (GSE29570 and GSE39001) (Figure 1A). We found that HPV+G1 had a significantly higher disease-free survival (DFS) rate than HPV+G2 (log-rank test, P = 0.01) (Figure 1B). Interestingly, HPV+G1 showed significantly higher enrichment levels of various immune signatures than HPV+G2, including CD8+ T cells, B cells, M1 macrophages, cytolytic activity, IFN response, CD4+ regulatory T cells, pro-inflammatory cytokines, T cell exhaustion, MDSCs, PD-L1 expression, and anti-inflammatory cytokines (one-tailed Mann–Whitney U test, P < 0.01) (Figure 1C). Moreover, the ratios of immunostimulatory/immunosuppressive signatures (M1/M2 macrophages and pro/anti-inflammatory cytokines) were higher in HPV+G1 than in HPV+G2 (Figure 1C). We further used the ESTIMATE algorithm (17) to calculate the immune score representing the tumor immune infiltration level. As expected, immune scores were significantly higher in HPV+G1 than in HPV+G2 (P < 0.001) (Figure 1D), while tumor purity was significantly lower in HPV+G1 than in HPV+G2 (P < 0.001) (Figure 1E). These results suggest that HPV+G1 has a more active tumor immune microenvironment than HPV+G2. In addition, we found that stromal scores were significantly higher in HPV+G1 than in HPV+G2 (P = 0.002) (Figure 1F). HPV+G1 had significantly lower stemness scores than HPV+G2 (P = 0.005) (Figure 1G). Moreover, HPV+G1 had significantly lower ITH scores than HPV+G2 (P = 0.005) (Figure 1H).
Figure 1 Subtyping of HPV+ cervical cancers based on gene expression profiles. (A) Based on the expression levels of the 50 genes having the largest expression variations across the TCGA HPV+ cervical cancers, hierarchical clustering analyses identify two subtypes of HPV+ cervical cancers: HPV+G1 and HPV+G2, consistently in three different datasets. (B) HPV+G1 showing significantly higher disease-free survival rate than HPV+G2. The log-rank test P value is shown. (C) HPV+G1 showing significantly higher enrichment levels of various immune signatures than HPV+G2. The one-tailed Mann–Whitney U test or two-tailed Student’s t test P values are indicated. Comparisons of immune scores (D), tumor purity (E), stromal scores (F), stemness scores (G), and intratumor heterogeneity (ITH) scores (H) between HPV+G1 and HPV+G2. The one-tailed Mann–Whitney U test P values are shown. (I) Cox proportional hazards regression analysis showing that the subtype HPV+G2 is a risk factor for disease-free survival prognosis in HPV+ cervical cancers after adjusting for tumor immune signatures, stemness, and ITH. HR, hazard ratio; CI, confidence interval; *P < 0.05, **P < 0.01, ***P < 0.001 (they also apply to the following figures).
Because tumor immune signatures, stemness, and ITH are associated with clinical outcomes in cancer (18, 23, 24) and had significantly different scores between the HPV+ cervical cancer subtypes, the survival difference between the subtypes could be impacted by these confounding variables. To explore the possibility, we performed multivariate (immune score, stemness score, ITH score, and subtype) survival analysis with the multivariate Cox proportional hazards model. The result showed that the subtype HPV+G2 remained a significant risk factor (P = 0.007; hazard ratio (HR) = 3.76 and its 95% confidence interval (CI) (3):) (Figure 1I). It suggests that the DFS difference between both subtypes is independent of these confounding variables.
Genomic instability often leads to increased TMB and CNAs (25). We found that HPV+G1 had higher TMB than HPV+G2 (one-tailed Mann–Whitney U test, P = 0.057) (Figure 2A). Homologous recombination deficiency (HRD) may lead to aneuploidy, namely CNAs (25). We obtained the HRD scores for the TCGA cervical cancers from the publication by Knijnenburg et al., which were the combined scores of HRD loss of heterozygosity, large-scale state transitions, and the number of telomeric allelic imbalances (25). We found that HPV+G1 had significantly higher HRD scores than HPV+G2 (one-tailed Mann–Whitney U test, P = 0.015) (Figure 2B). We found that the G-scores of copy number amplifications and deletions were significantly higher in HPV+G1 than in HPV+G2 cervical cancers (Figure 2C). Taken together, these results indicated that HPV+G1 was more genomically instable than HPV+G2.
Figure 2 Comparisons of genomic and epigenomic profiles between the HPV+ cervical cancer subtypes. HPV+G1 having higher TMB (A), homologous recombination deficiency (HRD) scores (B), and G-scores of copy number amplifications and deletions (C), and lower global methylation levels (D) than HPV+G2. The one-tailed Mann–Whitney U test P values are shown in (A, B, D). The G-score calculated by GISTIC2 (20) represents the amplitude of the copy number alteration and the frequency of its occurrence across a group of samples.
We compared global methylation levels (26) between both subtypes and found that HPV+G1 had significantly lower global methylation levels than HPV+G2 (one-tailed Mann–Whitney U test, P = 0.011) (Figure 2D). This result conforms with that low methylation levels is associated with increased TMB and CNAs in cancer (26). Strikingly, we found that 5367 genes showed significantly lower methylation levels in HPV+G1 than in HPV+G2 (FDR < 0.05), while there was no any gene showing significantly higher methylation levels in HPV+G1 than in HPV+G2. These results indicate a significant difference in epigenomic profiles between both subtypes.
We compared gene expression profiles between HPV+G1 and HPV+G2 and identified significantly upregulated genes in both subtypes. Based on these upregulated genes, we identified KEGG pathways highly enriched in HPV+G1 and HPV+G2, respectively, using the GSEA web tool (14). Many of the pathways especially enriched in HPV+G1 were involved in immune signatures, including cytokine-cytokine receptor interaction, cell adhesion molecules, complement and coagulation cascades, chemokine signaling, Toll-like receptor signaling, Fc gamma R-mediated phagocytosis, leukocyte transendothelial migration, intestinal immune network for IgA production, natural killer cell-mediated cytotoxicity, NOD-like receptor signaling, and Jak-STAT signaling (Figure 3A). It confirms the more active tumor immune microenvironment in HPV+G1 versus HPV+G2.
Figure 3 Pathways and gene ontology (GO) enriched in the HPV+ cervical cancer subtypes. (A) The immune-related pathways enriched in HPV+G1 versus HPV+G2. (B) Nine gene modules and their representative GO terms significantly differentiating cervical cancers by subtype and survival. OS, overall survival; DFS, disease-free survival.
WGCNA (16) identified nine gene modules significantly differentiating cervical cancers by subtype (Figure 3B). The representative GO terms for the gene modules upregulated in HPV+G1 while downregulated in HPV+G2 included immune response (in brown) and epidermal and epithelial cell differentiation (in black). In contrast, the representative GO terms for the gene modules upregulated in HPV+G2 while downregulated in HPV+G1 included intracellular non-membrane-bounded organelle (in green) and microvillus (in pink). Again, these results confirm that HPV+G1 has a more active tumor immune microenvironment versus HPV+G2.
We compared the expression levels of 192 proteins between HPV+G1 and HPV+G2 and identified significantly upregulated proteins in both subtypes (two-tailed Student’s t test, P < 0.05). We found 22 proteins having significantly higher expression levels in HPV+G1, including NDRG1_pT346, Notch1, EGFR, Annexin-1, CD49b, PI3K-p85, EGFR_pY1068, Caveolin-1, YB-1_pS102, Src_pY416, Bad_pS112, MEK1, Myosin-IIa_pS1943, PAI-1, YAP_pS127, YAP, Bcl-2, Fibronectin, MYH11, GSK3_pS9, Syk, and VHL (Figure 4). Among them, Annexin A1, also known as lipocortin I, plays a role in the regulation of innate and adaptive immunity (27). CD49b (cluster of differentiation 49b) is an integrin alpha subunit expressed on various cell types, including T cells and NK cells (28). YAP is involved in the Hippo signaling pathway, which plays a role in tumor immune regulation (29). Bcl-2 is a positive regulator of apoptosis, which is associated with anti-tumor immunity (30). Collectively, these results again confirmed the more active tumor immune microenvironment in HPV+G1 versus HPV+G2.
In contrast, 25 proteins showed significantly higher expression levels in HPV+G2 than in HPV+G1 (Figure 4). These proteins included TIGAR, XRCC1, AMPK-α, Claudin-7, Bcl-xL, PCNA, p27, SCD1, TAZ, INPP4B, Ku80, ASNS, AMPK_pT172, MSH2, Acetyl-a-Tubulin-Lys40, 53BP1, Rab25, MIG-6, MSH6, mTOR, Chk2, ER-α, c-Kit, PKC-delta_pS664, and Transglutaminase. Among them, many proteins are involved in DNA repair, including XRCC1, PCNA, Ku80, MSH2, and MSH6, confirming that HPV+G2 was more genomically stable than HPV+G2.
Although HPV+G2 had lower enrichment levels of immune signatures than HPV+G1, it showed significantly higher enrichment levels of various immune signatures than HPV- tumors, including
NK cells, M1 macrophages, IFN response, CD4+ regulatory T cells, and M2 macrophages (Supplementary Figure S1A). Moreover, the ratios of immunostimulatory/immunosuppressive signatures (CD8+ T cells/MDSCs) were significantly higher in HPV+G2 than in HPV- cervical cancers (Supplementary Figure S1B). Most of the human leukocyte antigen (HLA) genes, which encode major histocompatibility complex (MHC) proteins and play essential roles in the regulation of the immune system, displayed significantly higher expression levels in HPV+G2 than in HPV- cervical cancers (two-tailed Student’s t test, FDR < 0.02; fold change (FC) > 1.5) (Supplementary Figure S1C). Immune scores were significantly higher in HPV+G2 than in HPV- cervical cancers (P = 0.04) (Supplementary Figure S1D). These results support that HPV infection results in a more active tumor immune microenvironment in cervical cancer.
Both ITH and stemness scores followed the pattern: HPV+G1 < HPV+G2 < HPV-, while global methylation levels were higher in HPV+G2 than in both HPV+G1 and HPV- (Supplementary Figure S1E). In addition, although TMB and HRD scores were higher in HPV+G1 than in HPV+G2, they were not significantly different between HPV+G2 and HPV- (P > 0.2). Furthermore, we did not observe significantly different OS or DFS rate between the HPV+ subtypes and HPV- (log-rank test, P > 0.2).
We used TCGA-CESC as the training set and the other two datasets as test sets. The 10-fold cross-validation (CV) sensitivity, specificity, and AUC in TCGA-CESC were 99.1%, 95.0%, and 100.0%, respectively. The prediction sensitivity, specificity, and AUC in GSE29570 were 100%, 80.0%, and 90.0%, respectively, and in GSE39001 were 92.0%, 100%, and 96.0%, respectively (Figure 5). These results suggest that our subtyping method for HPV+ cervical cancers is highly reproducible and predictable. In the prediction model, we found that the 10 features (genes) with the highest importance weights included DSG3, DSC3, CLCA2, LASS3, CALML3, SERPINB13, IVL, PROM1, AGR3, and TMPRSS11D (Table 2).
Figure 5 Prediction of the HPV+ cervical cancer subtypes using the 50 genes having the largest expression variations across the TCGA HPV+ cervical cancers by random forest. TCGA-CESC was the training set and GSE29570 and GSE39001 were test sets. The sensitivity, specificity, and AUC are shown. AUC, area under the receiver operating characteristic curve; CV, cross validation.
We investigated the relationship between our subtyping method and other cervical cancer subtyping methods (5). We found that squamous cell carcinomas constituted 98% of HPV+G1 tumors versus 34% of HPV+G2 tumors (chi-square test, P < 0.001) (Figure 6). In contrast, adenocarcinomas constituted 63% of HPV+G2 tumors versus 2% of HPV+G1 tumors. The TCGA network classified cervical cancers into three subtypes by unsupervised clustering of variable DNA-methylation probes (5). The three subtypes included: CpG island hypermethylated (CIMP-high), CIMP-intermediate, and CIMP-low. We found that 4% of HPV+G1 tumors were CIMP-high, compared to 53% of HPV+G2 tumors being CIMP-high, and that 46% of HPV+G1 tumors were CIMP-low versus 29% of HPV+G2 tumors being CIMP-low (P < 0.001) (Figure 6). It is consistent with the lower overall DNA methylation level in HPV+G1 relative to HPV+G2. In addition, we found 20% of HPV+G1 tumors being Clade A7 versus 50% of HPV+G2 tumors and 78% of HPV+G1 tumors being Clade A9 versus 50% of HPV+G2 tumors (P = 0.002) (Figure 6). The different distribution of HPV clades between HPV+G1 and HPV+G2 indicates a better prognosis in HPV+G1 versus HPV+G2 since HPV clade A7 cervical cancers are more aggressive than clade A9 cancer (31). RPPA-based clustering identified three clusters: hormone, EMT, and PI3K-AKT (5). We found that 34% of HPV+G1 tumors were in the EMT cluster versus 8% of HPV+G2 tumors (P = 0.002) (Figure 6). It is justified since EMT represents a stromal signature and HPV+G1 has higher stromal scores than HPV+G2. In addition, 32% of HPV+G1 tumors were in the PI3K-AKT cluster versus 17% of HPV+G2 tumors, and 34% of HPV+G1 tumors were in the hormone cluster versus 75% of HPV+G2 tumors. It is consistent with previous results that PI3K-p85 was more abundant in HPV+G1 while ER-α was more abundant in HPV+G2. Furthermore, 37% of HPV+G1 tumors were HPV-16 positive versus 32% of HPV+G2 tumors, and 5% of HPV+G1 tumors were HPV-18 positive versus 25% of HPV+G2 tumors. It indicates that a significantly higher proportion of HPV+G2 tumors are HPV-18 positive compared to HPV+G1 tumors (P < 0.001). This result supports that HPV-18 infection is an adverse prognostic factor in cervical cancer (3), while HPV-16 infection is a positive prognostic factor in cervical cancer (32).
Based on the expression profiles of the 50 genes with the largest expression variations across the HPV+ cervical cancers in TCGA-CESC, we identified two subtypes of HPV+ cervical cancers, namely HPV+G1 and HPV+G2. We demonstrated that this classification method was reproducible in two validation sets. Compared to HPV+G2, HPV+G1 displayed significantly higher immune infiltration level and stromal content, lower tumor purity, lower stemness scores and ITH scores, higher level of genomic instability, lower DNA methylation level, as well as more favorable prognosis. The multivariate survival analysis suggests that the survival difference between both subtypes is independent of confounding variables, such as immune signature, stemness, and ITH. It is interesting to observe that HPV+G1 has a better DFS prognosis than HPV+G2, while HPV+G1 is more genomically instable compared to HPV+G2. Genomic instability is a common characteristic of cancers that drives cancer development (33). Nevertheless, increased TMB resulting from genomic instability may have generated more neoantigens that were shown to promote antitumor immune response (34). This could explain why patients in group HPV+G1 have a more favorable prognosis than in HPV+G2, even though the former display a higher level of genomic instability than the latter (35).
Among the 50 genes for clustering analysis, 37 showed higher expression levels in HPV+G1 versus HPV+G2. These genes included four members of the keratin gene family: KRT1, KRT4, KRT13, and KRT14. It supports previous findings that keratin expression plays a role in cervical cancer classification (36). The 37 genes also included two genes encoding calmodulin-like proteins: CALML3 and CALML5. CALML5 has been identified as a tumor suppressor gene in squamous cell carcinoma of uterine cervix (37). It consistent with that CALML5 is upregulated in HPV+G1 which has a more favorable prognosis versus HPV+G2. CALML3 and CALML5 are involved in the Ras, Rap1, calcium, neurotrophin, and estrogen signaling pathways, which plays important roles in cervical cancer development (38–42). In addition, the 37 genes included six members of the small proline-rich protein gene family: SPRR1A, SPRR1B, SPRR2A, SPRR2D, SPRR2E, and SPRR3. Previous studies have revealed that many small proline-rich protein genes were downregulated in cervical cancer relative to normal cervical tissue (43, 44), suggesting their tumor suppressor roles. It conforms to the better prognosis in HPV+G1 versus HPV+G2. 13 of the 50 genes for clustering analysis were more highly expressed in HPV+G2 than in HPV+G1, including MUC5B, BPIFB1, PIGR, MUC13, SLC34A2, TFF3, AQP5, LTF, PROM1, GABRP, AGR3, PAX7, and TSPAN8. Among them, MUC5B and MUC13 belong to the mucin gene family, which plays oncogenic roles in various cancers (45, 46). Previous studies also showed that mucin genes were associated with subtyping of cervical cancer (5). PAX7 is a member of the paired box (PAX) family of transcription factors and is oncogenic in a variety of cancers, including cervical cancer (47). Again, PAX7 upregulation in HPV+G2 relative to HPV+G1 is in line with the better prognosis in HPV+G1 versus HPV+G2. Interestingly, 47 of the 50 genes for clustering analysis were differentially expressed between HPV-16 positive HPV+G1 and HPV-18 positive HPV+G2 tumors (FDR < 0.01, FC > 2). The 47 genes included 34 genes which were more highly expressed in HPV-16 positive HPV+G1 versus HPV-18 positive HPV+G2 tumors and were also more highly expressed in HPV+G1 versus HPV+G2 tumors. In contrast, the other 13 genes were more highly expressed in HPV-18 positive HPV+G2 versus HPV-16 positive HPV+G1 tumors and were also more highly expressed in HPV+G2 versus HPV+G1 tumors. These data suggest that the HPV genotype could exert a significant effect on the expression pattern of most of the 5o genes since the HPV-18 genotype has a significantly different distribution between HPV+G1 and HPV+G2. In fact, previous studies have shown that HPV infection is able to cause global gene expression changes at the precancerous and cancerous stages of cervical cancer (48–50). For example, HPV-16 and HPV-18 E6 oncoproteins promote the deregulation of tumor suppressor genes, such as TP53 and RB1, to induce the expression changes of their target genes (51–53). Interestingly, some of the 50 genes, such as DSG3 and CLCA2, have been identified as targets of p53 (54, 55).
The TCGA network also performed mRNA clustering analysis to identify cervical cancer subtypes using the uncentered correlation and centroid linkage method (5). This method discovered three cervical cancer subtypes: C1, C2, and C3. We found that 95% of HPV+G2 tumors belonged to C1 and that 72% and 25% of HPV+G1 tumors belonged to C2 and C3, respectively. It indicates that HPV+G2 is nearly equivalent to C1 and that HPV+G1 is the combination of C2 and C3. However, survival analysis showed that there was no significant difference in DFS among C1, C2, and C3, compared to significant difference in DFS between HPV+G1 and HPV+G2. It suggests that our mRNA-based subtyping method is more reasonable than that method in terms of the prognostic relevance.
HPV+ cervical cancers can be classified into two subtypes based on the expression profiles of the 50 genes with the largest expression variations across the HPV+ cervical cancers. Both subtypes have significantly different immune and stromal microenvironment, tumor purity, stemness, ITH, genomic instability, DNA methylation level, as well as survival prognosis. This new subtyping method captures the comprehensive heterogeneity in molecular and clinical characteristics of HPV+ cervical cancers and provides potential clinical implications for the management of this disease.
Publicly available datasets were analyzed in this study. This data can be found here: TCGA-CESC (https://portal.gdc.cancer.gov) and the NCBI gene expression omnibus (https://www.ncbi.nlm.nih.gov/geo/) under the accession numbers GSE30784 and GSE39366.
XZ and SL, software, validation, formal analysis, investigation, data curation, and visualization. JL, investigation and data curation. XY, formal analysis and investigation. ZL, YW, MZ, and TZ, formal analysis. PJ, investigation, resources, supervision, funding acquisition. XW, conceptualization, methodology, resources, investigation, writing - original draft, writing - review and editing, supervision, and project administration. All authors contributed to the article and approved the submitted version.
This work was supported by the National Natural Science Foundation of China (grant number 81902625).
The authors declare that the research was conducted in the absence of any commercial or financial relationships that could be construed as a potential conflict of interest.
All claims expressed in this article are solely those of the authors and do not necessarily represent those of their affiliated organizations, or those of the publisher, the editors and the reviewers. Any product that may be evaluated in this article, or claim that may be made by its manufacturer, is not guaranteed or endorsed by the publisher.
The Supplementary Material for this article can be found online at: https://www.frontiersin.org/articles/10.3389/fimmu.2022.801639/full#supplementary-material
Supplementary Figure 1 | Comparisons between the HPV+ cervical cancer subtypes and HPV- tumors. Higher enrichment levels of immune signatures (A), ratios of immunostimulatory/immunosuppressive signatures (CD8+ T cells/MDSCs) (B), expression levels of human leukocyte antigen (HLA) genes (C), and immune scores (D) in HPV+G2 than in HPV- cervical cancers. (E) Comparisons of ITH scores, stemness scores, and global methylation levels among cervical cancer subtypes. The K–W or one-way ANOVA test (A, C), two-tailed Student’s t test (B), and one-tailed Mann–Whitney U test (D, E) P values are indicated. * P < 0.05, ** P < 0.01, *** P < 0.001.
HPV, human papillomavirus; HPV+, Human papillomavirus-positive; HPV-, HPV-negative; CESC, cervical squamous cell carcinoma; EMT, epithelial-mesenchymal transition; dMMR, mismatch repair deficiency; TMB, tumor mutation burden; CNA, copy number alteration; ITH, intratumor heterogeneity; HRD, Homologous recombination deficiency; PR, progesterone receptor; ICIs, immune checkpoint inhibitors; RF, Random Forest; DFS, disease-free survival; HR, hazard ratio; HLA, human leukocyte antigen; MHC, major histocompatibility complex; CV, cross-validation; CIMP, CpG island hypermethylated; WGCNA, weighted gene co-expression network analysis.
1. Ferlay J, Soerjomataram I, Dikshit R, Eser S, Mathers C, Rebelo M, et al. Cancer Incidence and Mortality Worldwide: Sources, Methods and Major Patterns in GLOBOCAN 2012. Int J Cancer (2015) 136(5):E359–86. doi: 10.1002/ijc.29210
2. Burd EM. Human Papillomavirus and Cervical Cancer. Clin Microbiol Rev (2003) 16(1):1–17. doi: 10.1128/CMR.16.1.1-17.2003
3. Xu Y, Qiu Y, Yuan S, Wang H. Prognostic Implication of Human Papillomavirus Types in Cervical Cancer Patients: A Systematic Review and Meta-Analysis. Infect Agent Cancer (2020) 15(1):66. doi: 10.1186/s13027-020-00332-5
4. Xing B, Guo J, Sheng Y, Wu G, Zhao Y. Human Papillomavirus-Negative Cervical Cancer: A Comprehensive Review. Front Oncol (2020) 10:606335. doi: 10.3389/fonc.2020.606335
5. Cancer Genome Atlas Research Network, Albert Einstein College of Medicine, Analytical Biological Services, Barretos Cancer Hospital, Baylor College of Medicine, Beckman Research Institute of City of Hope. Integrated Genomic and Molecular Characterization of Cervical Cancer. Nature (2017) 543(7645):378–84. doi: 10.1038/nature21386
6. Das BC, Hussain S, Nasare V, Bharadwaj M. Prospects and Prejudices of Human Papillomavirus Vaccines in India. Vaccine (2008) 26(22):2669–79. doi: 10.1016/j.vaccine.2008.03.056
7. Liu Z, Li M, Jiang Z, Wang X. A Comprehensive Immunologic Portrait of Triple-Negative Breast Cancer. Transl Oncol (2018) 11(2):311–29. doi: 10.1016/j.tranon.2018.01.011
8. Stevanović S, Draper LM, Langhan MM, Campbell TE, Kwong ML, Wunderlich JR, et al. Complete Regression of Metastatic Cervical Cancer After Treatment With Human Papillomavirus-Targeted Tumor-Infiltrating T Cells. J Clin Oncol (2015) 33(14):1543–50. doi: 10.1200/JCO.2014.58.9093
9. Burtness B, Harrington KJ, Greil R, Soulières D, Tahara M, de Castro G Jr, et al. Pembrolizumab Alone or With Chemotherapy Versus Cetuximab With Chemotherapy for Recurrent or Metastatic Squamous Cell Carcinoma of the Head and Neck (KEYNOTE-048): A Randomised, Open-Label, Phase 3 Study. Lancet (2019) 394(10212):1915–28. doi: 10.1016/S0140-6736(19)32591-7
10. Yanik EL, Kaunitz GJ, Cottrell TR, Succaria F, McMiller TL, Ascierto ML, et al. Association of HIV Status With Local Immune Response to Anal Squamous Cell Carcinoma: Implications for Immunotherapy. JAMA Oncol (2017) 3(7):974–8. doi: 10.1001/jamaoncol.2017.0115
11. Guardado-Estrada M, Medina-Martínez I, Juárez-Torres E, Roman-Bassaure E, Macías L, Alfaro A, et al. The Amerindian mtDNA Haplogroup B2 Enhances the Risk of HPV for Cervical Cancer: De-Regulation of Mitochondrial Genes may be Involved. J Hum Genet (2012) 57(4):269–76. doi: 10.1038/jhg.2012.17
12. Espinosa AM, Alfaro A, Roman-Basaure E, Guardado-Estrada M, Palma I, Serralde C, et al. Mitosis Is a Source of Potential Markers for Screening and Survival and Therapeutic Targets in Cervical Cancer. PloS One (2013) 8(2):e55975. doi: 10.1371/journal.pone.0055975
13. Hanzelmann S, Castelo R, Guinney J. GSVA: Gene Set Variation Analysis for Microarray and RNA-Seq Data. BMC Bioinf (2013) 14:7. doi: 10.1186/1471-2105-14-7
14. Subramanian A, Tamayo P, Mootha VK, Mukherjee S, Ebert BL, Gillette MA, et al. Gene Set Enrichment Analysis: A Knowledge-Based Approach for Interpreting Genome-Wide Expression Profiles. Proc Natl Acad Sci USA (2005) 102(43):15545–50. doi: 10.1073/pnas.0506580102
15. Kanehisa M, Furumichi M, Tanabe M, Sato Y, Morishima K. KEGG: New Perspectives on Genomes, Pathways, Diseases and Drugs. Nucleic Acids Res (2017) 45(D1):D353–61. doi: 10.1093/nar/gkw1092
16. Langfelder P, Horvath S. WGCNA: An R Package for Weighted Correlation Network Analysis. BMC Bioinf (2008) 9:559. doi: 10.1186/1471-2105-9-559
17. Yoshihara K, Shahmoradgoli M, Martínez E, Vegesna R, Kim H, Torres-Garcia W, et al. Inferring Tumour Purity and Stromal and Immune Cell Admixture From Expression Data. Nat Commun (2013) 4:2612. doi: 10.1038/ncomms3612
18. Miranda A, Hamilton PT, Zhang AW, Pattnaik S, Becht E, Mezheyeuski A, et al. Cancer Stemness, Intratumoral Heterogeneity, and Immune Response Across Cancers. Proc Natl Acad Sci USA (2019) 116(18):9020–9. doi: 10.1073/pnas.1818210116
19. Li M, Zhang Z, Li L, Wang X. An Algorithm to Quantify Intratumor Heterogeneity Based on Alterations of Gene Expression Profiles. Commun Biol (2020) 3(1):505. doi: 10.1038/s42003-020-01230-7
20. Mermel CH, Schumacher SE, Hill B, Meyerson ML, Beroukhim R, Getz G. GISTIC2.0 Facilitates Sensitive and Confident Localization of the Targets of Focal Somatic Copy-Number Alteration in Human Cancers. Genome Biol (2011) 12(4):R41. doi: 10.1186/gb-2011-12-4-r41
22. Benjamini Y, Hochberg Y. Controlling the False Discovery Rate: A Practical and Powerful Approach to Multiple Testing. J R Stat Soc B (1995) 57:289–300. doi: 10.1111/j.2517-6161.1995.tb02031.x
23. Liu Q, Nie R, Li M, Li L, Zhou H, Lu H, et al. Identification of Subtypes Correlated With Tumor Immunity and Immunotherapy in Cutaneous Melanoma. Comput Struct Biotechnol J (2021) 19:4472–85. doi: 10.1016/j.csbj.2021.08.005
24. Li L, Chen C, Wang X. DITHER: An Algorithm for Defining IntraTumor Heterogeneity Based on EntRopy. Brief Bioinform (2021) 22(6):bbab202. doi: 10.1093/bib/bbab202
25. Knijnenburg TA, Wang L, Zimmermann MT, Chambwe N, Gao GF, Cherniack AD, et al. Genomic and Molecular Landscape of DNA Damage Repair Deficiency Across The Cancer Genome Atlas. Cell Rep (2018) 23(1):239–54.e6. doi: 10.1016/j.celrep.2018.03.076
26. Jung H, Kim HS, Kim JY, Sun JM, Ahn JS, Ahn MJ, et al. DNA Methylation Loss Promotes Immune Evasion of Tumours With High Mutation and Copy Number Load. Nat Commun (2019) 10(1):4278. doi: 10.1038/s41467-019-12159-9
27. Perretti M, D'Acquisto F. Annexin A1 and Glucocorticoids as Effectors of the Resolution of Inflammation. Nat Rev Immunol (2009) 9(1):62–70. doi: 10.1038/nri2470
28. Arase H, Saito T, Phillips JH, Lanier LL. Cutting Edge: The Mouse NK Cell-Associated Antigen Recognized by DX5 Monoclonal Antibody Is CD49b (Alpha 2 Integrin, Very Late Antigen-2). J Immunol (2001) 167(3):1141–4. doi: 10.4049/jimmunol.167.3.1141
29. He Y, Jiang Z, Chen C, Wang X. Classification of Triple-Negative Breast Cancers Based on Immunogenomic Profiling. J Exp Clin Cancer Res (2018) 37(1):327. doi: 10.1186/s13046-018-1002-1
30. Jiang Z, Liu Z, Li M, Chen C, Wang X. Increased Glycolysis Correlates With Elevated Immune Activity in Tumor Immune Microenvironment. EBioMedicine (2019) 42:431-42. doi: 10.1016/j.ebiom.2019.03.068
31. Gagliardi A, Porter VL, Zong Z, Bowlby R, Titmuss E, Namirembe C, et al. Analysis of Ugandan Cervical Carcinomas Identifies Human Papillomavirus Clade-Specific Epigenome and Transcriptome Landscapes. Nat Genet (2020) 52(8):800–10. doi: 10.1038/s41588-020-0673-7
32. Hang D, Jia M, Ma H, Zhou J, Feng X, Lyu Z, et al. Independent Prognostic Role of Human Papillomavirus Genotype in Cervical Cancer. BMC Infect Dis (2017) 17(1):391. doi: 10.1186/s12879-017-2465-y
33. Negrini S, Gorgoulis VG, Halazonetis TD. Genomic Instability–an Evolving Hallmark of Cancer. Nat Rev Mol Cell Biol (2010) 11(3):220–8. doi: 10.1038/nrm2858
34. Yarchoan M, Johnson BA 3rd, Lutz ER, Laheru DA, Jaffee EM. Targeting Neoantigens to Augment Antitumour Immunity. Nat Rev Cancer (2017) 17(4):209–22. doi: 10.1038/nrc.2016.154
35. Wood MA, Weeder BR, David JK, Nellore A, Thompson RF. Burden of Tumor Mutations, Neoepitopes, and Other Variants are Weak Predictors of Cancer Immunotherapy Response and Overall Survival. Genome Med (2020) 12(1):33. doi: 10.1186/s13073-020-00729-2
36. Smedts F, Ramaekers F, Troyanovsky S, Pruszczynski M, Link M, Lane B, et al. Keratin Expression in Cervical Cancer. Am J Pathol (1992) 141(2):497–511.
37. Kitazawa S, Takaoka Y, Ueda Y, Kitazawa R. Identification of Calmodulin-Like Protein 5 as Tumor-Suppressor Gene Silenced During Early Stage of Carcinogenesis in Squamous Cell Carcinoma of Uterine Cervix. Int J Cancer (2021) 149(6):1358–68. doi: 10.1002/ijc.33687
38. Feng Q, Song D, Wang X. Pan-Cancer Analysis Reveals That Neurotrophin Signaling Correlates Positively With Anti-Tumor Immunity, Clinical Outcomes, and Response to Targeted Therapies and Immunotherapies in Cancer. Life Sci (2021) 282:119848. doi: 10.1016/j.lfs.2021.119848
39. Mammas IN, Zafiropoulos A, Sifakis S, Sourvinos G, Spandidos DA. Human Papillomavirus (HPV) Typing in Relation to Ras Oncogene mRNA Expression in HPV-Associated Human Squamous Cervical Neoplasia. Int J Biol Markers (2005) 20(4):257–63. doi: 10.1177/172460080502000409
40. Pascoal-Xavier MA, Figueiredo AC, Gomes LI, Peruhype-Magalhães V, Calzavara-Silva CE, Costa MA, et al. RAP1 GTPase Overexpression is Associated With Cervical Intraepithelial Neoplasia. PloS One (2015) 10(4):e0123531. doi: 10.1371/journal.pone.0123531
41. Shen MR, Chou CY, Browning JA, Wilkins EJ, Ellory JC. Human Cervical Cancer Cells Use Ca2+ Signalling, Protein Tyrosine Phosphorylation and MAP Kinase in Regulatory Volume Decrease. J Physiol (2001) 537(Pt 2):347–62. doi: 10.1111/j.1469-7793.2001.00347.x
42. Zhai Y, Bommer GT, Feng Y, Wiese AB, Fearon ER, Cho KR. Loss of Estrogen Receptor 1 Enhances Cervical Cancer Invasion. Am J Pathol (2010) 177(2):884–95. doi: 10.2353/ajpath.2010.091166
43. Santin AD, Zhan F, Bignotti E, Siegel ER, Cané S, Bellone S, et al. Gene Expression Profiles of Primary HPV16- and HPV18-Infected Early Stage Cervical Cancers and Normal Cervical Epithelium: Identification of Novel Candidate Molecular Markers for Cervical Cancer Diagnosis and Therapy. Virology (2005) 331(2):269–91. doi: 10.1016/j.virol.2004.09.045
44. Yao S, Liu T. Analysis of Differential Gene Expression Caused by Cervical Intraepithelial Neoplasia Based on GEO Database. Oncol Lett (2018) 15(6):8319–24. doi: 10.3892/ol.2018.8403
45. Ringel J, Lohr M. The MUC Gene Family: Their Role in Diagnosis and Early Detection of Pancreatic Cancer. Mol Cancer (2003) 2:9. doi: 10.1186/1476-4598-2-9
46. Senapati S, Sharma P, Bafna S, Roy HK, Batra SK. The MUC Gene Family: Their Role in the Diagnosis and Prognosis of Gastric Cancer. Histol Histopathol (2008) 23(12):1541–52. doi: 10.14670/HH-23.1541
47. Su PH, Lai HC, Huang RL, Chen LY, Wang YC, Wu TI, et al. Paired Box-1 (PAX1) Activates Multiple Phosphatases and Inhibits Kinase Cascades in Cervical Cancer. Sci Rep (2019) 9(1):9195. doi: 10.1038/s41598-019-45477-5
48. Kang SD, Chatterjee S, Alam S, Salzberg AC, Milici J, van der Burg SH, et al. Effect of Productive Human Papillomavirus 16 Infection on Global Gene Expression in Cervical Epithelium. J Virol (2018) 92(20):e01261–18. doi: 10.1128/JVI.01261-18
49. Gius D, Funk MC, Chuang EY, Feng S, Huettner PC, Nguyen L, et al. Profiling Microdissected Epithelium and Stroma to Model Genomic Signatures for Cervical Carcinogenesis Accommodating for Covariates. Cancer Res (2007) 67(15):7113–23. doi: 10.1158/0008-5472.CAN-07-0260
50. Rosty C, Sheffer M, Tsafrir D, Stransky N, Tsafrir I, Peter M, et al. Identification of a Proliferation Gene Cluster Associated With HPV E6/E7 Expression Level and Viral DNA Load in Invasive Cervical Carcinoma. Oncogene (2005) 24(47):7094–104. doi: 10.1038/sj.onc.1208854
51. Dyson N, Howley PM, Münger K, Harlow E. The Human Papilloma Virus-16 E7 Oncoprotein is Able to Bind to the Retinoblastoma Gene Product. Science (1989) 243(4893):934–7. doi: 10.1126/science.2537532
52. Scheffner M, Werness BA, Huibregtse JM, Levine AJ, Howley PM. The E6 Oncoprotein Encoded by Human Papillomavirus Types 16 and 18 Promotes the Degradation of P53. Cell (1990) 63(6):1129–36. doi: 10.1016/0092-8674(90)90409-8
53. Werness BA, Levine AJ, Howley PM. Association of Human Papillomavirus Types 16 and 18 E6 Proteins With P53. Science (1990) 248(4951):76–9. doi: 10.1126/science.2157286
54. Rehman A, Cai Y, Hünefeld C, Jedličková H, Huang Y, Teck Teh M. The Desmosomal Cadherin Desmoglein-3 Acts as a Keratinocyte Anti-Stress Protein via Suppression of P53. Cell Death Dis (2019) 10(10):750. doi: 10.1038/s41419-019-1988-0
Keywords: human papillomavirus-positive cervical cancer, subtyping, clustering analysis, tumor immune microenvironment, multi-omics
Citation: Zhu X, Li S, Luo J, Ying X, Li Z, Wang Y, Zhang M, Zhang T, Jiang P and Wang X (2022) Subtyping of Human Papillomavirus-Positive Cervical Cancers Based on the Expression Profiles of 50 Genes. Front. Immunol. 13:801639. doi: 10.3389/fimmu.2022.801639
Received: 25 October 2021; Accepted: 05 January 2022;
Published: 21 January 2022.
Edited by:
Wei-Hua Yan, Wenzhou Medical University, ChinaReviewed by:
Maria Issagouliantis, Riga Stradiņš University, LatviaCopyright © 2022 Zhu, Li, Luo, Ying, Li, Wang, Zhang, Zhang, Jiang and Wang. This is an open-access article distributed under the terms of the Creative Commons Attribution License (CC BY). The use, distribution or reproduction in other forums is permitted, provided the original author(s) and the copyright owner(s) are credited and that the original publication in this journal is cited, in accordance with accepted academic practice. No use, distribution or reproduction is permitted which does not comply with these terms.
*Correspondence: Peiyue Jiang, NTMxNTAxNEB6anUuZWR1LmNu; Xiaosheng Wang, eGlhb3NoZW5nLndhbmdAY3B1LmVkdS5jbg==
†These authors have contributed equally to this work
Disclaimer: All claims expressed in this article are solely those of the authors and do not necessarily represent those of their affiliated organizations, or those of the publisher, the editors and the reviewers. Any product that may be evaluated in this article or claim that may be made by its manufacturer is not guaranteed or endorsed by the publisher.
Research integrity at Frontiers
Learn more about the work of our research integrity team to safeguard the quality of each article we publish.