- 1Department of Neurology, Odense University Hospital, Odense, Denmark
- 2Institute of Clinical Research, University of Southern Denmark, Odense, Denmark
- 3Institute of Molecular Medicine, University of Southern Denmark, Odense, Denmark
- 4Department of Mathematics and Computer Science, University of Southern Denmark, Odense, Denmark
- 5Chair of Computational Systems Biology, University of Hamburg, Hamburg, Germany
Multiple sclerosis (MS) is an inflammatory demyelinating and degenerative disease of the central nervous system (CNS). Although inflammatory responses are efficiently treated, therapies for progression are scarce and suboptimal, and biomarkers to predict the disease course are insufficient. Cure or preventive measures for MS require knowledge of core pathological events at the site of the tissue damage. Novelties in systems biology have emerged and paved the way for a more fine-grained understanding of key pathological pathways within the CNS, but they have also raised questions still without answers. Here, we systemically review the power of tissue and single-cell/nucleus CNS omics and discuss major gaps of integration into the clinical practice. Systemic search identified 49 transcriptome and 11 proteome studies of the CNS from 1997 till October 2021. Pioneering molecular discoveries indicate that MS affects the whole brain and all resident cell types. Despite inconsistency of results, studies imply increase in transcripts/proteins of semaphorins, heat shock proteins, myelin proteins, apolipoproteins and HLAs. Different lesions are characterized by distinct astrocytic and microglial polarization, altered oligodendrogenesis, and changes in specific neuronal subtypes. In all white matter lesion types, CXCL12, SCD, CD163 are highly expressed, and STAT6- and TGFβ-signaling are increased. In the grey matter lesions, TNF-signaling seems to drive cell death, and especially CUX2-expressing neurons may be susceptible to neurodegeneration. The vast heterogeneity at both cellular and lesional levels may underlie the clinical heterogeneity of MS, and it may be more complex than the current disease phenotyping in the clinical practice. Systems biology has not solved the mystery of MS, but it has discovered multiple molecules and networks potentially contributing to the pathogenesis. However, these results are mostly descriptive; focused functional studies of the molecular changes may open up for a better interpretation. Guidelines for acceptable quality or awareness of results from low quality data, and standardized computational and biological pipelines may help to overcome limited tissue availability and the “snap shot” problem of omics. These may help in identifying core pathological events and point in directions for focus in clinical prevention.
1 Introduction
Multiple sclerosis (MS) is a common cause of neurological disability among young adults that evolves in clinically different stages termed radiologically isolated syndrome (RIS), clinically isolated syndrome (CIS), relapsing-remitting MS (RRMS), secondary progressive phase (SPMS), and primary progressive MS (PPMS). However, this classification may not directly reflect the pathological mechanisms similarly to another classification that only considers clinical/radiological activity and disability progression (1).
MS has a heterogeneous, multifactorial origin that involves interactions between the immune and nervous system impacted by the genetic background (2) and by the environment (3, 4). The main pathological features are accumulation of lesions in the grey and white matter (GM, WM). These are characterized by different degrees of inflammation, demyelination, neuronal and axonal degeneration, oligodendrocyte loss, gliosis/glia activity, and remyelination. Additional features are diffuse inflammation in the normal-appearing (NA) tissues, meningeal infiltrates, and global CNS atrophy (5). Especially in early relapsing MS, influx of systemic immune cells into the CNS induces inflammatory demyelinating lesions (6, 7). As the disease progresses, the number of chronic active lesions increases, and they inversely correlate with the number of remyelinating/repairing lesions (8–10). Lesions in cortical and deep GM areas and neuronal loss become prominent in the progressive phase (11). At this stage, inflammation becomes more compartmentalized and is governed primarily by microglia, astrocytes, and tissue-resident lymphocytes (12, 13).
Approved MS treatments impact systemic adaptive immune responses and work effectively in the early phase (14). However, their passage through the blood-brain barrier is limited, and most of them do not affect innate immune responses in the CNS. Their effect on compartmentalized immune responses is largely unknown. Such limitations are also reflected by their poor impact in the progressive phase. Neuro- and oligodendrocyte-protective treatments that inhibit or reverse degenerative processes are basically missing. To develop efficient treatments for the progressive phase, understanding the molecular mechanisms of pathological events within the CNS is essential. This has shifted focus of MS research to CNS-specific events. Recent advances in omics will hopefully integrate several levels of spatiotemporal data, and may help to understand, how multiple factors can converge into phenotypically similar disease states. Such knowledge may also fuel novel treatments (15, 16). To accomplish such goals, several challenges have to be overcome, e.g. experimental and computational pipelines have to be standardized, and large amount of descriptive biological data should be functionally interpreted. Here, we systemically review the transcriptome and proteome studies in the MS brain and discuss gaps and obstacles.
2 Methods
2.1 Search Strategies
A systematic electronic search was conducted in PubMed with the following search terms from as far back as possible (earliest identified study was from 1997) to October 2021: category one “multiple sclerosis”; category two “brain”, “lesions”, “white matter”, “grey matter”; category three “omics”, “profiling”, “transcriptome”, “array”, “next generation sequencing” “proteome”; category four “human” and NOT “review”. The search was also complemented by reference lists of articles identified by this search strategy.
2.2 Selection Criteria
Studies were included, if they fulfilled the following criteria: (i) the study was performed on human brain tissue from patients with MS; (ii) the study used next-generation sequencing, mass spectrometry or arrays on the human brain tissue; (iii) article written in English.
Studies were excluded if the study design was not clearly stated.
3 Results
Omics studies on MS brain tissue are few. An overview of the different methods is illustrated in Figure 1. Advantages and disadvantages of different omics techniques are listed in Table 1. A flowchart summarizing the identification of relevant studies according to PRISMA is presented in Figure 2.
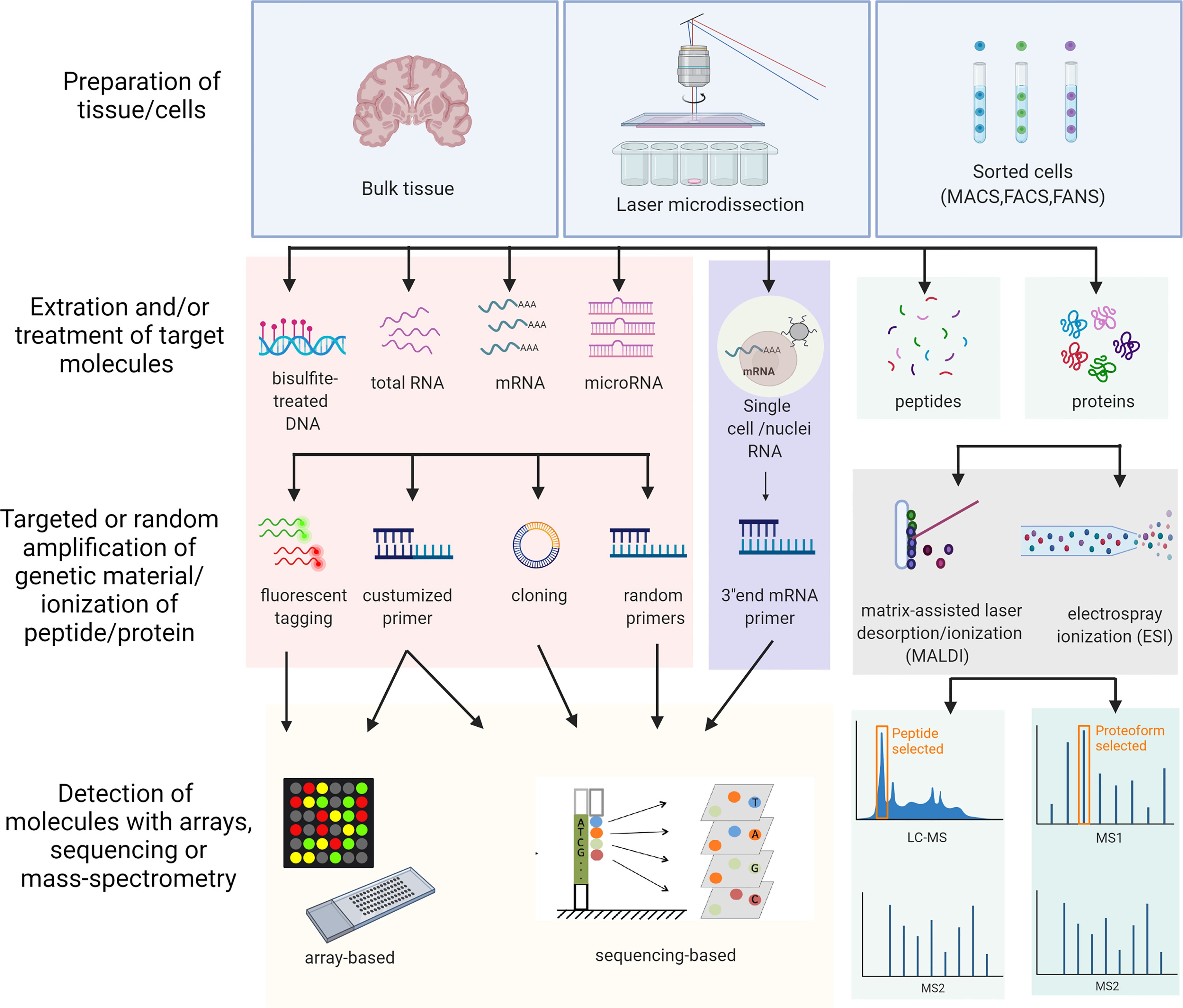
Figure 1 Schematic overview of the different omics approaches used in the study. The overview includes all the different methods used in the studies included in this review. MACS, Magnetic-activated cell sorting; FACS, Fluorescence-activated cell sorting; FANS, Fluorescence-activated nucleus sorting; LC-MS, Liquid chromatography–mass spectrometry. Created with BioRender.com.
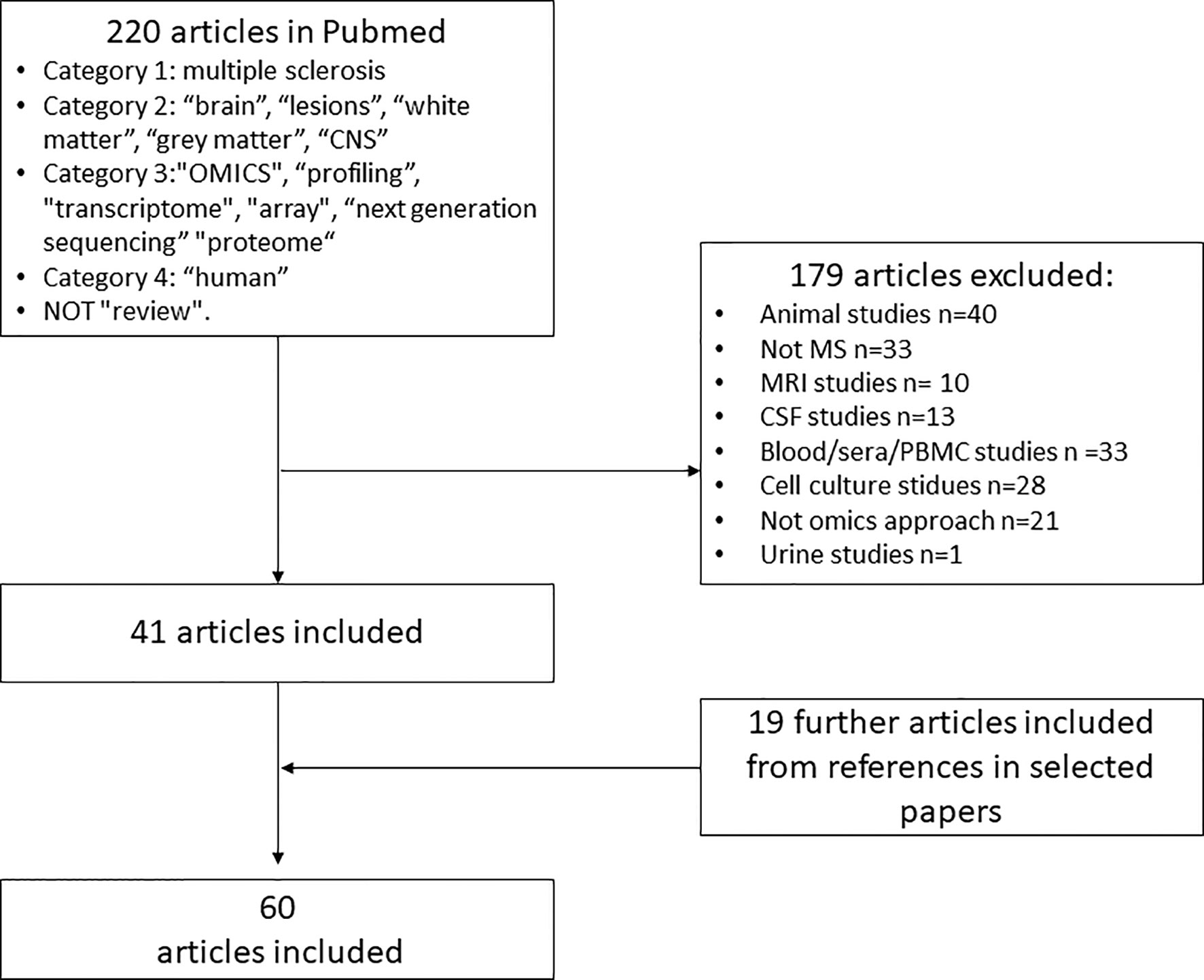
Figure 2 Flowchart for identification and inclusion of relevant studies for systematic review. PMD, postmortem-delay; RIN, RNA integrity number.
3.1 Transcriptional (mRNA, ncRNA, MicroRNA) Approaches to Examine Pathological Mechanisms in the MS Brain Tissue
In the late 1990s, the first large-scale gene expression profiles were performed on different WM lesions from both autopsies and biopsies using microarrays (Table 2). They revealed alterations in cell metabolism, shifts in cytokines and cell adhesion molecules (17, 18), new inflammatory (19, 20, 44) and oxidative damage markers (23). In the 2010s, the number of samples increased, and microdissected tissues were also analyzed in designed systems biology studies; these included vessels near lesions (27), chronic active rim areas (33), or specific cell types like astrocytes (32). Single-cell/nucleus technologies, such as single-cell/nucleus RNA sequencing (sc/nRNA-seq, spatial-seq) that promote identification of novel cell types and cell state transitions have been published since 2019 (56–58).

Table 2 An overview of the studies (n=49) that examined the transcriptome profile in human MS brain tissue.
We highlight the main findings in eight sections based on tissue types: (i) brain regional differences; (ii) NAWM; (iii) NAGM; (iv) WM lesions; (v) GM lesions; (vi) cell-specific changes; (vii) non-human transcripts, (viii) databases.
3.1.1 Brain Regional Differences
Corpus callosum and optic chiasm were the most significantly affected CNS regions in a study, and myelinating oligodendrocytes were most enriched with differentially expressed genes (51). Heat shock proteins were upregulated in all examined brain regions (HSPA1A, HSPA1B, HSPA7, HSPA6, HSPH1, HSPA4L) (52). Genes important in antigen-presentation, inflammation and hypoxia-induced responses were altered in the corpus callosum and optic chiasm (TAPBP, IRF4, CTSB, CD79A), while STAT6 and HLA-DRB5 were only increased in the optic chiasm. However, these regional differences may also reflect the presence of different cell types expressing different types of regional specific “housekeeping genes” with distinct physiological functions and purpose.
DNA methylation was altered, and RNA levels of DNA mehyltransferase were increased in MS hippocampus following demyelination (40). This study identified hypomethylation upstream of six genes including ANKA, a major regulator of CD40-CD40L, and hypermethylation upstream of ten genes e.g. WDR81, NHLH2, PLCH1 involved in neuronal survival, synaptic density and memory.
In the choroid plexus (CP), 17 genes were significantly upregulated in progressive MS patients (54). These genes were related to hypoxia, neuroprotection and secretion (e.g. CXCL2, LYVE1, SNHG15, MT1X, non-coding HIFA1-AS3), while strong inflammatory reactions were absent.
3.1.2 NAWM
Comparing NAWM to control WM, 465 genes were differentially expressed (48). Among the top ten upregulated genes were immune-related (IGHG1, HLA-DRB5, GPNMB, CD163) and mitochondria-related (MTRNR2L12, MTRNR2L8). NAWM was also different from control WM by a global defense against oxidative stress based on upregulation of STAT6, HIFα and its target genes (21, 26, 61). Genes in the STAT-6 signaling were upregulated in oligodendrocytes (61) (Figure 3). These alterations were accompanied by upregulation of nNOS, HO-1 and HLA-DR, suggesting an inflammatory and oxidative-stress related reaction in oligodendrocytes outside of lesions. A combined methylome and transcriptome study found downregulation and hypermethylation of oligodendrocyte survival genes in NAWM (BCL2L2, NBRG1) (46). Besides oligodendrocytes, several dysregulated genes in MS suggested alterations in subcortical WM neurons (21).
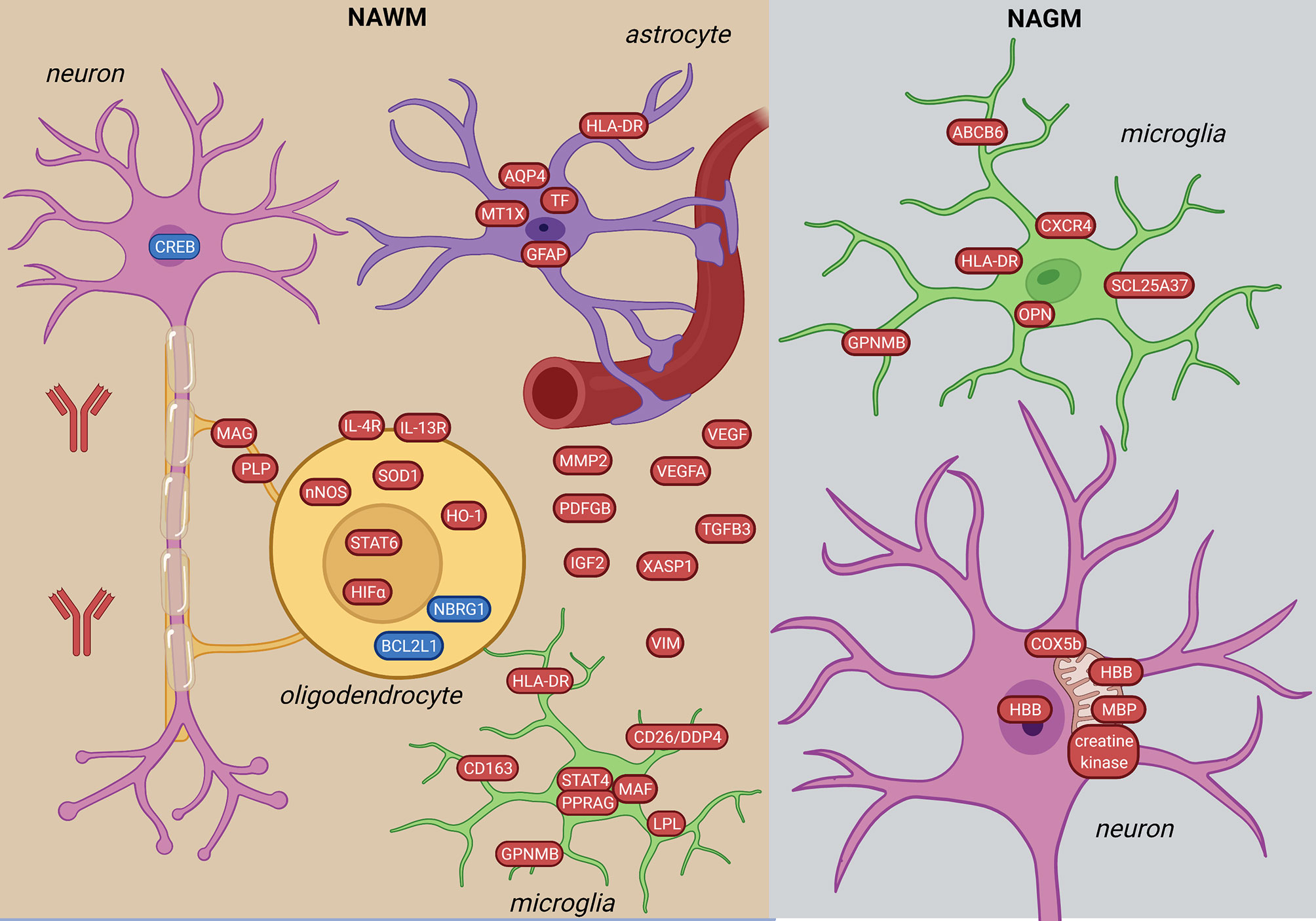
Figure 3 Signature of NAWM and NAGM in the MS brain based on transcriptome and proteome studies. In the NAWM, alterations in all brain resident cells were observed. Oligodendrocytes are characterized by altered myelin transcripts and upregulate anti-inflammatory and hypoxia-induced pathways (STAT6-, HIFα-signaling). Microglia upregulate pro-inflammatory molecules (STAT4-signaling, HLA-DR, GPNMB, CD163). Inflammatory astrocytes have iron- and oxidative stress-related profiles. In the NAGM, microglia have a distinct inflammation-induced neurodegenerative profile from NAWM (CXCR4, ABCB6, SCL25A37). Neurons in the NAGM express hemoglobin β (HBB) and have alterations in mitochondrial proteins. The figure was created by compiling data from several articles, and therefore molecules may not be expressed at the same time. Created with BioRender.com.
NAWM microglia upregulated STAT4 and HLA-DRα (26), and had a lipid metabolic gene expression profile (e.g. EEPD1, PPARG, LPL) with unchanged expression of the homeostatic signature (P2RY12 and TMEM119) (50). Additionally, a subtype of microglia (48) had increased expression of CD26/DPP4 in the NAWM (46). Astrocytic markers (GFAP, AQP4) were also altered in the NAWM (61) (Figure 3).
Genes of several chemokines and cytokines (21, 26) were upregulated in NAWM reflecting the low level inflammation even without lesion formation. A mild disease course was also associated with a different molecular profile with altered expression of genes related to immune-regulation, myelination, anti-oxidative mechanism and neuroprotection together with a high hypothalamus-pituitary-adrenal (HPA) axis activity (35).
3.1.3 NAGM
The difference in WM vs. GM microglia gene expression was significantly lower in MS compared to non-neurological disease brains. This suggests that microglia cells are losing region-specific profile in MS (50). However, while NAWM microglia have a lipid signature, NAGM microglia have increased expression of genes related to glycolysis and iron homeostasis (SCL25A37, ABCB6) and a neurodegenerative profile (CXCR4, GPNMB, OPN/SPP1) (Figure 3). Furthermore, in HLADRB1*15:01 positive patients, HLA-DRB1 and B5 were the highest expressed genes in NAGM (37).
3.1.4 WM Lesions
A continuum of dysfunctional homeostasis (e.g. VIM, HBB, MAF) and inflammatory changes (e.g. CASP1, IRF5, MMP2) between active lesions and NAWM supports the concept of MS involving the whole CNS (24). However, the lesions differed from NAWM by high expression of genes related to immunoglobulin synthesis (IGKC, IGL, IGGL1, ILR6) and neuroglial differentiation (SNAP25, CAP2, NFL/M) (24). Upregulated genes in active lesions compared to NAWM also included chemokine genes and receptors (MIP-a, RANTES, CCR1, CCR4, CCR5, VLA-4, CCR8) genes, interferon- and tumor-necrosis factor receptors (17), and cytokines (TGFB, IL-3, OPN, IL-5, IL6) (18, 44, 53) (Figure 4). Two highly expressed genes encoded the Th cell marker (CD4) and the antigen-presenting gene (HLA-DRa) (18). Additionally, CD8+ T cells containing cytotoxic granules were suggested to communicate with mononuclear phagocyte cell expressing CD163 and CD11b in the lesions (49). Genes encoding multiple autoantigens were also found in MS lesions indicating a secondary autoimmune stimulation that could exacerbate the ongoing inflammation (43).
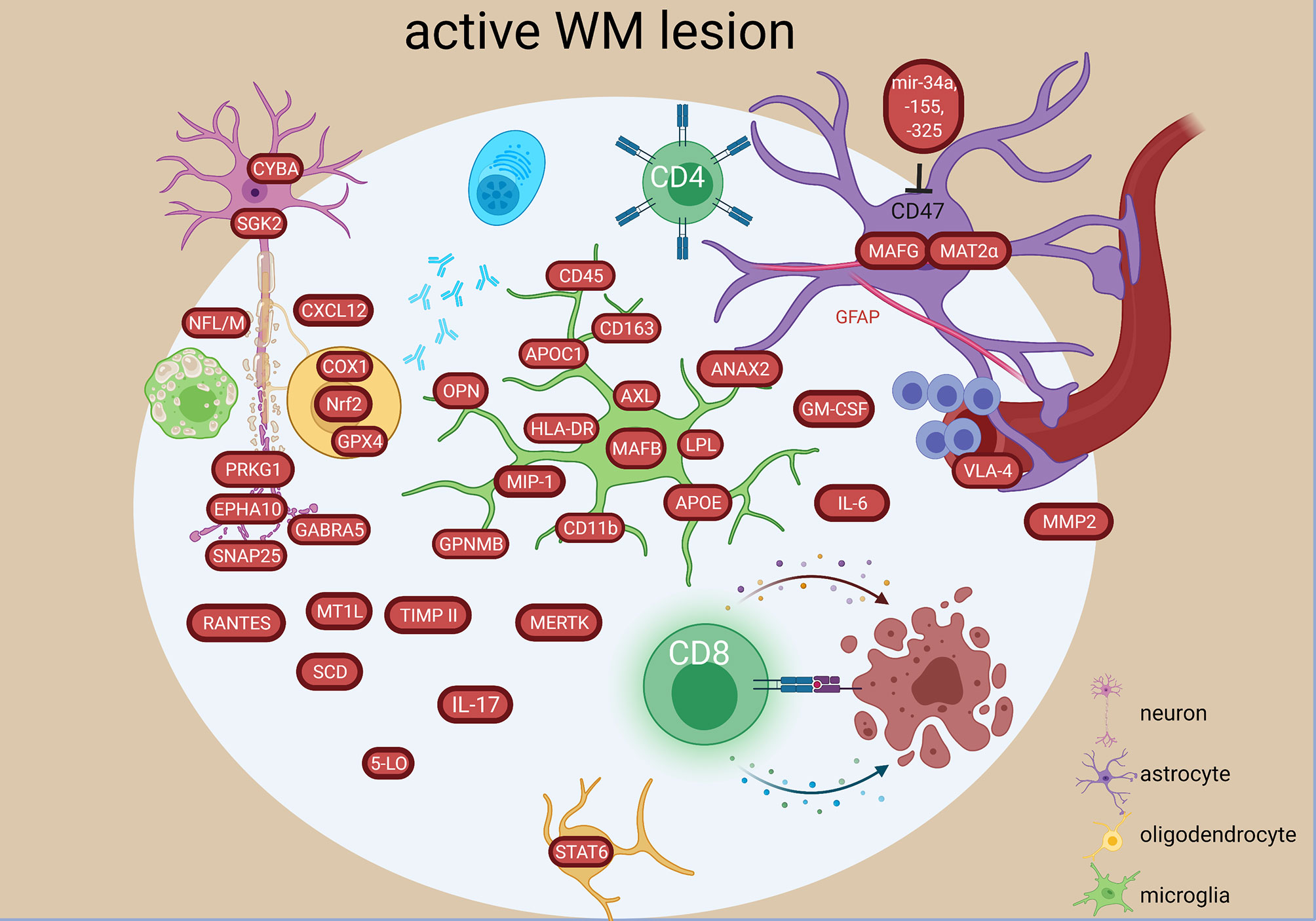
Figure 4 Signature of active WM lesion in the MS brain based on transcriptome and proteome studies. In the active lesion, an increase in both innate and adaptive inflammatory responses are present characterized by different molecular components in resident and infiltrating cells. An oxidative stress and degenerative profile especially in the oligodendrocytes and neurons have also been detected. The figure was created by compiling data from several articles, and therefore molecules may not be expressed at the same time. Created with BioRender.com.
Mitochondrial injury in initial WM lesions was indicated by increase of ND1-6, CYTB, COX1, CYBA, MPO, PTGS1, PXDN, GPX4, PRDX1, SGK2, ALOX12, EPHX2 expression, which were related to degeneration of oligodendrocytes and neurons and contributed to reactive oxygen species production by activated microglia and macrophages (28) (Figure 4).
Active and chronic active lesions shared upregulation of a number of genes coding for e.g. iron-binding protein (TF), chemokine and its receptor important for T cell accumulation in CNS (CXCR3, CXCL10), the myelin-binding protein (MBP), the first subcomponent of the complement system (C1QB), oxidative protection (GPX1, SOD1) and cytokines (IL-6, IL-17, INFg) (20, 23). However, 70 uniquely differentially expressed genes were also found: e.g. coding for the receptor related to differentiation (EPHB6), the granulocyte-macrophage colony-stimulating factor (GM-CSF), and a MHC class I molecule (HLA-A) in active lesions or e.g. genes coding for the chaperone protein (HSPA1A), component of MHC class I (B2M) or complement factor 4B (C4B) in chronic active lesions.
Differences have also been found on an epigenetic level, as the microRNA profile was different between active and inactive lesions (39). In the active lesions, microRNA-34a, -155 and -326 were all upregulated and targeted the CD47 in brain resident cells to release inhibitor control and promote phagocytosis (Figure 4). Moreover, upregulated miR-22, miR-320 in active lesion and upregulated miR-30d in inactive lesions (39) were related to pathogenic changes (41), while downregulation of miR-18a, miR23b in inactive lesions (39) were related to protective changes correlating with MRI abnormalities (41).
An in-depth investigation of different lesion types (active, early remyelinating, chronic active, inactive) in the WM showed extreme diverse events at transcriptome level. More differential expressed genes were unique than shared. Among the 282 altered genes common to all lesion types were genes related to inflammation (STAT6, CXCL12, TNFs, DPP4/CD26, ITGA4, GPNMB, IL16, HLA-DRB5, MAFB, IGHG1, IGF2, MMP2), phagocytosis (SCD, CD163, MERTK) complement pathway (CFH, C7, CFI), apoptosis/necroptosis (FADS1, CASP1,-4, MLKL) (48). Immunoglobulin genes were among the top 10 in all WM MS tissues, but the most heterogeneous expression pattern was detected in early remyelinating lesions. TGFBR2 was the major molecular hub of the largest shared lesion network and was highly expressed in remyelinating lesions by astrocytes (48) (Figure 4). The most different signatures were found between remyelinating and chronic active lesions. Chronic active lesions had the highest number of unique genes reflecting intrinsic neuronal alterations, and de novo networks suggested an end-stage exhaustion (48). Most of the uniquely expressed genes in the early remyelinating lesions were non-coding RNAs, while others were related to lymphocytes and NKT cells (e.g. CD8a, TIAM1, CTSW, CCL5/RANTES), growth and development (e.g. PEG10, BMP4, GDF10), vascular changes and remodeling (e.g. PLAU, VEGFA, CTGF), mitochondria and protective stress responses (e.g. NDUFA4, NOSTRIN), lipid metabolism (e.g. ACACA, ACOX2, ADH6, CA3), and neurons (e.g. NEUROD1, NLGN1, GRIA3) (Figure 5). Another study found CXCL12, SCD, STAT6 increased in all lesion types, and transcriptional differences between lesion types reflected a heterogeneous oligodendrogliogenesis (34). Quantitative changes of oligodendrocyte regulators were also found in remyelinating lesions (30). Compared to demyelinating lesions, remyelination was accompanied by significant changes in the expression of myelin proteins (CNP, MAG, MBP, MOBP, MOG, OMG, PLP1), anti-inflammatory IL10, and semaphorins (SEMA3C, SEMA4D, SEMA6A, SEMA6D, SEMA7A) (Figure 5). The growth factor gene FGF1 was significantly increased in remyelinating lesions compared to both control WM and demyelinating lesions. In functional experiments, FGF1 promoted both developmental myelination and remyelination by inducing LIF and CXCL8 in astrocytes to recruit oligodendrocytes. GFAP was also significantly increased in active and remyelinating lesions (55) (Figures 4, 5). The glia receptor protein tyrosine phosphatase gene PTPRG was increased in all MS WM tissues, and was also significantly increased in the CSF of MS patients compared to healthy and other neurological disease controls (55). CHI3L1 was increased in astrocytes in the chronic active lesion rim (55), and by microglia in active lesions compared to NAWM (50).
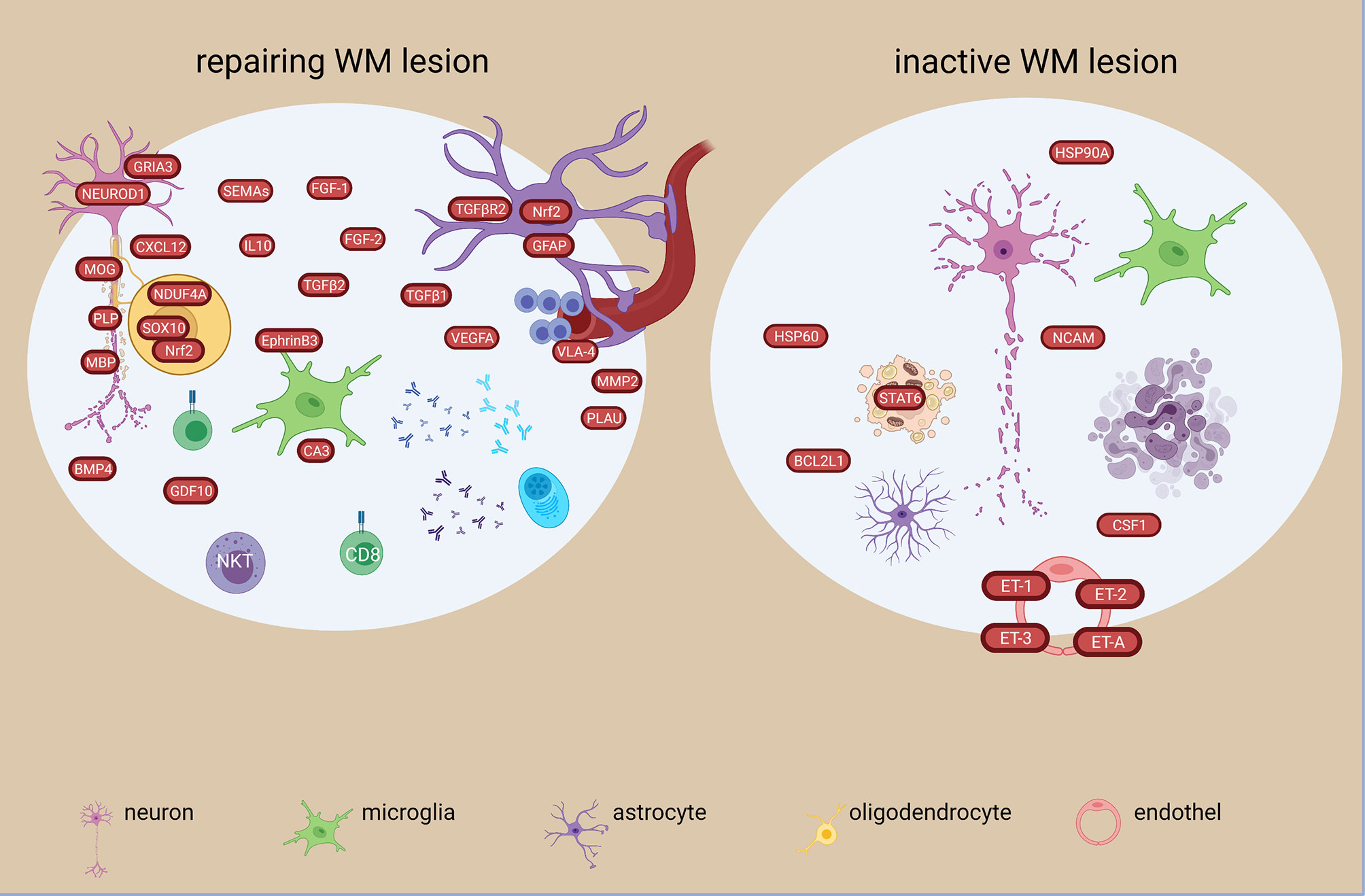
Figure 5 Signatures of repairing/remyelinating and inactive WM lesion types in the MS brain based on transcriptome and proteome studies. Remyelinating signatures are characterized among others by soluble growth factors and reparatory molecules such as FGF-1, -2, TGFB1,-2, BMP4 and GDF10. Oxidative and anti-oxidative responses are present, as well as a heterogenous immune response. In the inactive lesion, different heat shock proteins are present together with changes in endothelin transcripts. The figure was created by compiling data from several articles, and therefore molecules may not be expressed at the same time. Created with BioRender.com.
In a single-nucleus study of WM lesions, the majority of cells were oligodendrocytes, and oligodendrocytes represented the most heterogenous cell population (56). One of the seven oligodendrocyte populations was termed immune oligodendroglia (imOLG) due to expression of APOE and CD74. OPCs were reduced in lesions and NAWM compared to control WM. One oligodendrocyte population was depleted, whereas three others and imOLG were enriched in MS. Several myelin protein genes were upregulated in mature oligodendrocytes in MS, however some of those (e.g. CNP, MAG) were downregulated in remyelinating lesions.
Excessive expression of the antioxidant transcription factor NRF2 in oligodendrocytes indicated oxidative stress and degeneration at sites of initial demyelination in active lesions (31). NRF2 in astrocytes and macrophages were mainly seen in the later stages of active lesions with profound loss of oligodendrocytes. NRF2 in neurons was low or absent despite NRF2-positve oligodendrocytes in close proximity indicating cellular differences in reaction to oxidative stress and inflammation (Figure 4).
In chronic active lesions,14 genes were significantly upregulated in the rim vs the center (e.g. IFNG, NGF2, CD4, CASP9, MAPKK1) (22, 25, 29) (Figure 6). Inflammatory genes were upregulated in chronic active lesion center (CCL4, IL6, CD27, TNFA) (Figure 6), while upregulation of NCAM, CSF1, HSP60, HSP90A, BCL2L1 in inactive lesion center and rim highlighted different inflammatory responses, beside apoptosis and stress (Figure 5). Heat shock protein genes in inactive lesions (48) and in the rim of chronic active lesions were upregulated, especially the heat shock factor 4 (HSF4) (29).
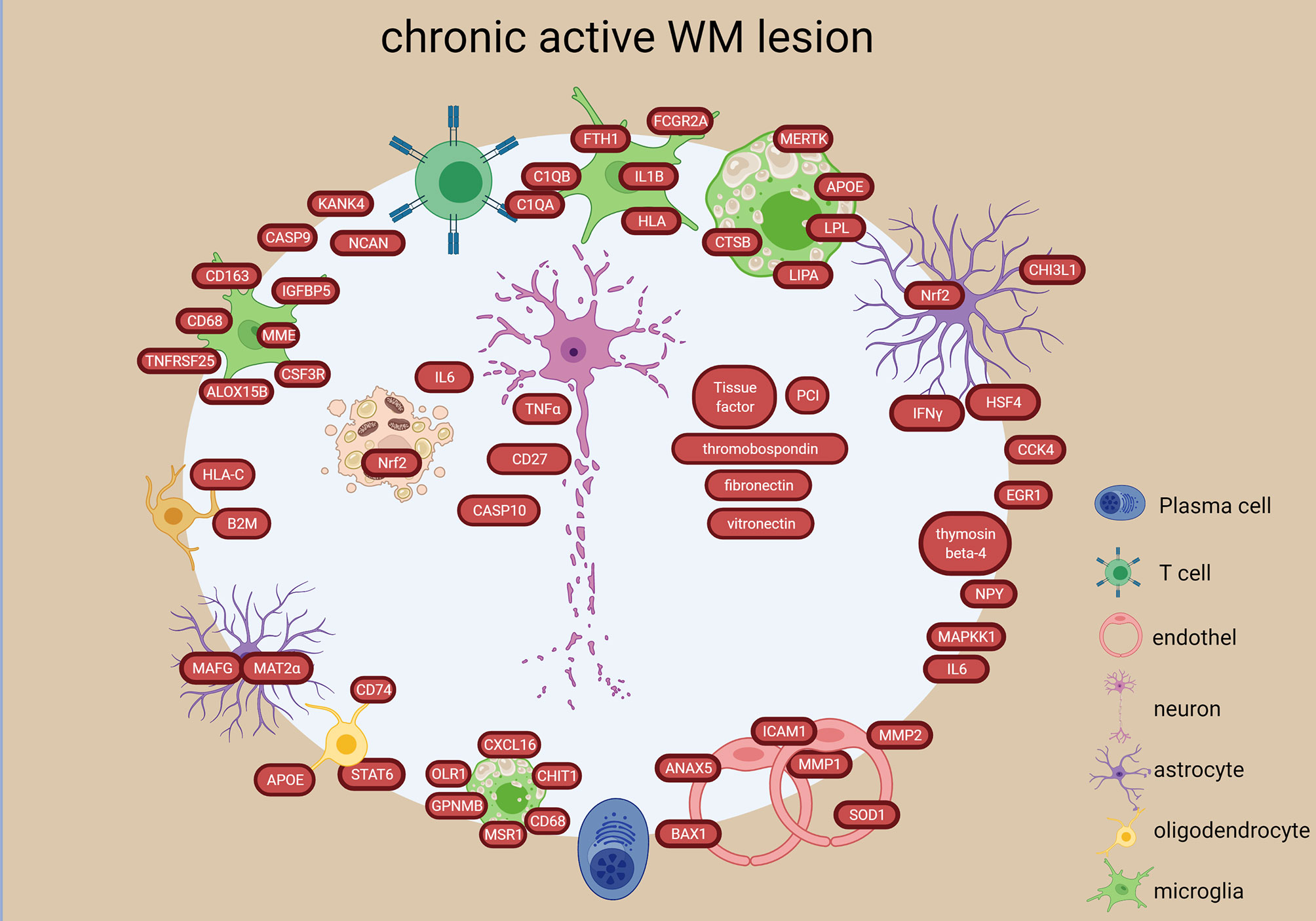
Figure 6 Signatures of chronic active lesion in the WM lesion types in the MS brain based on transcriptome and proteome studies. Chronic active lesion has a different molecular profile in rim vs center. Most activity is present in the rim with stressed astrocytes and oligodendroglia, proinflammatory microglial polarization and foamy macrophages. Additionally, presence of coagulation factors and endothelial alterations are detected. The chronic active lesion displayed the highest number of neuronal/axonal intracellular components. The figure was created by compiling data from several articles, and therefore molecules may not be expressed at the same time. Created with BioRender.com.
Upregulation of 165 genes and downregulation of 35 genes were identified in the chronic active lesion/slowly expanding lesions compared to inactive as well as NAWM (38). The upregulated genes suggested accumulation of microglia with proinflammatory differentiation at the lesion edge (e.g. CD163, CD68, CSF3R, IGFBP5, ALOX15B, MME, TNFRSF25) (Figure 6). A study that investigated the rim and peri-lesional regions of both chronic active and inactive lesions, found upregulation of previously not reported genes in the rim of chronic active lesions (NPY, KANK4, NCAN, TKTL1, ANO4) (33) (Figure 6). They also found that foamy macrophages in the rim upregulated genes involved in lipid binding and uptake indicating the expansion of demyelination (e.g. MSR1, CD68, CXCL16, OLR1, CHIT1, GPNMB all (Figure 6). Stressed oligodendrocytes with iron overload, reactive astrocytes and activated phagocytosing cells were also detected in the rim of chronic active lesions (58). These findings were confirmed and elaborated in a recent snRNA-study, where they found immunological-active OPCs, inflamed astrocytes (AIMS) and microglia (MIMS) in the chronic active rim (60). These were strongly connected to a high number of T cells and plasma cells suggesting an active role of the adaptive immune system in lesion expansion in collaboration with the glia cells in the smoldering inflammatory lesions (60). Microglia consisted of two distinct functional subtypes: the MIMS-foamy characterized by myelin phagocytosis and clearance properties, and the MIMS-iron, characterized by expression of complement C1q-complex, antigen-presentation and direct propagation of inflammatory damage at the lesion edge. The inflamed astrocytes were enriched for response to lipid, corticosteroids, wounding and expression of C3 similar to the A1 phenotype identified in the GM (62).
3.1.5 GM Lesions
A combined microRNA and mRNA profiling in GM lesions vs NAGM found significantly regulated microRNAs in GM lesions, which target genes of axonal guidance, TGFβ-signaling and FOXO signaling (63). Out of 27 significantly altered microRNAs, four microRNAs (mir149, mir20a, mir29c, mir25) and their targets (e.g. HIF1A, VEGFA, TGFBR1, TGFBR2, NFKBIB, FGFR1, TNFSF10, BCL2, MAP2K4, STAT3, MMP2, PTEN, CD44) were associated with GM atrophy (Figure 7) (63). Three of the 27 significantly altered microRNAs were also detected in another GM lesional microarray study (mir181b, mir129-5p, mir1180) (64). Additionally, miR-330-3p, miR-4286, miR-4488, let-7e-5p and miR-432-5p shared the same mRNA target, the Syt7 gene coding for the neuroaxonal protein normally transported to synapses. These 5 microRNAs may be protective against Syt7 accumulation in the soma resulting in disturbed axonal transport.
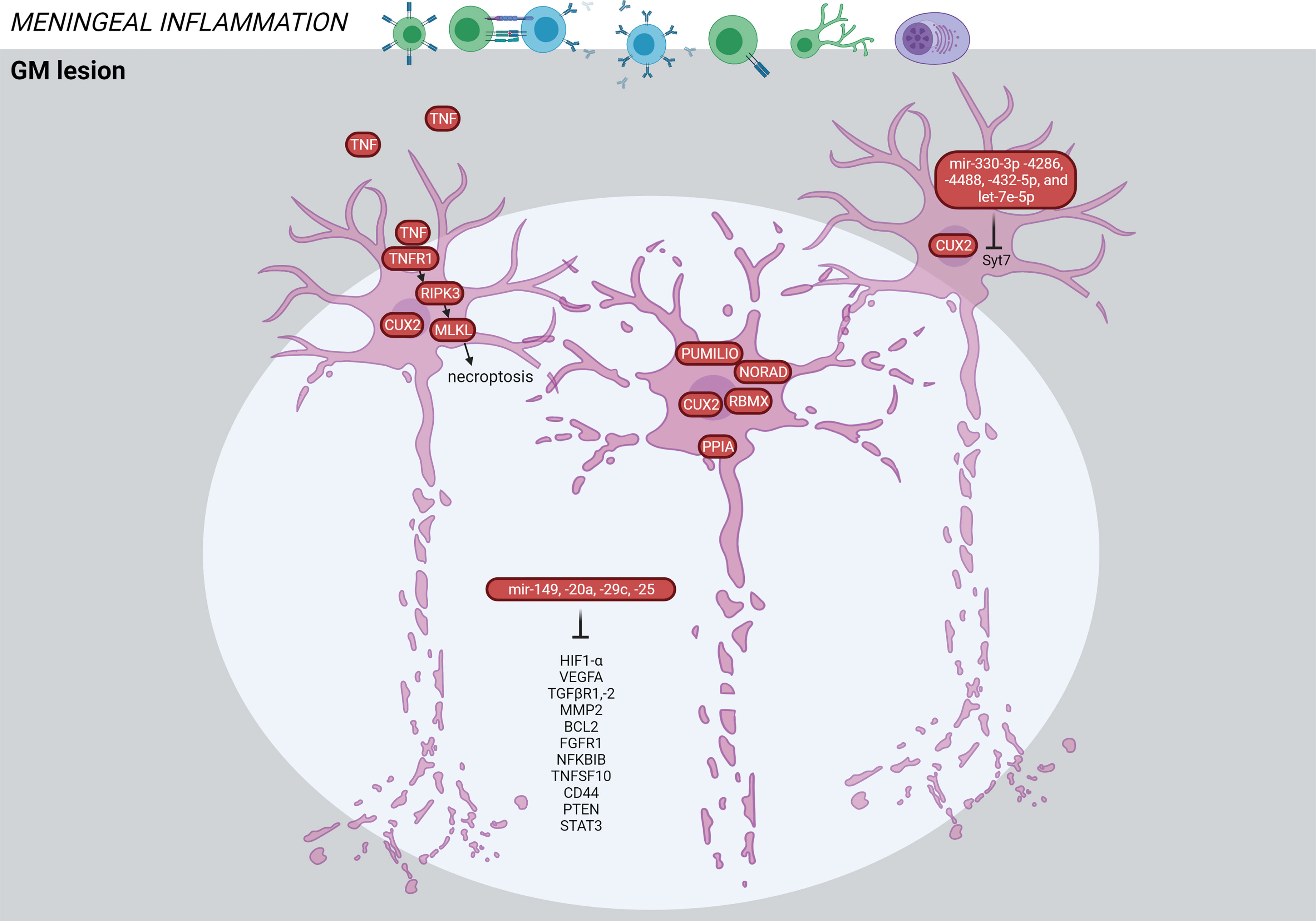
Figure 7 Signature of the GM lesion in the MS brain based on transcriptome and proteome studies. GM lesions are characterized by neuronal death mediated through TNF signaling. The CUX2-expressing cells are particularly vulnerable for degeneration. Alterations in microRNAs have been detected in the GM lesions associated with cortical atrophy. The figure was created by compiling data from several articles, and therefore molecules may not be expressed at the same time. Created with BioRender.com.
TNF signaling was also significantly increased in GM lesions. Increased meningeal inflammation was associated with a shift from TNFR1/TNFR2 and NFkB-mediated anti-apoptotic pathways towards TNFR1- and RIPK3-mediated pro-apoptotic/pro-necroptotic signaling (36) (Figure 7). TNFR1 was expressed by neurons and oligodendrocytes, while TNFR2 was predominantly expressed by astrocytes and microglia. The authors suggest that immune cells in meninges generate a milieu of increased demyelination and neurodegeneration by changing the balance of TNF signaling.
Another study found a selective loss of neurons expressing the transcription factor CUX2 in upper cortical layer lesions associated with pronounced meningeal B cell infiltration (58). These neurons expressed markers of cellular stress (PPIA, NORAD, PUMILIO, RBMX), and their loss may be a key event in MS progression and cortical atrophy (Figure 7).
3.1.6 Cell-Specific Changes
A study focused on endothelial cells in vessels found 52 genes significantly altered in chronic active or inactive lesions compared to control WM or NAWM (27). The majority of these genes belonged to endothelial cell activation, while VEGFA was the only one belonging to angiogenesis. Most of the genes were highly expressed in chronic active lesions compared to control WM (ANXA5, CSF3, FGF1,-2, FLT1,-4, ICAM1, MMP1, -2) (Figure 6) and compared to NAWM (FGF2, FLT1,-3, MMP14, PLAU, RIPK1). Several endothelin genes (1,2,3,A) involved in constriction of blood vessels and supply were increased in inactive lesions compared to NAWM (Figure 5).
Transcriptional profiling of isolated astrocytes in NAWM also revealed increased gene expression related to iron metabolism, oxidative stress, and inflammatory response (32) (Figure 3). An astrocyte single-cell study identified an expanded astrocyte population in active lesions characterized by decreased NRF2 and increased MAFG, GM-CSF signaling, pro-inflammatory pathway activity and DNA methylation (DNMT1) (59) (Figure 4). This astrocyte population is characterized by a MAFG/MAT2α-driven pro-inflammatory genomic program contributing to the pathology and may be induced by GM-CSF produced by infiltrating T cells (Figure 4). This corresponds to the high GM-CSF in active lesions (23), and low NRF2 in astrocytes in initial demyelinating lesions (31).
Seven microglia cell populations expressing the core microglial genes (TMEM119, P2RY12) in the WM were discovered in a single-cell study (57). Two of these clusters were enriched in brains of MS patients and one was associated with MS. These three populations had increased levels of APOE and MAFB (Figures 4, 8). The MS-associated microglia subset highly expressed CTSD, APOC1, GPNMB, ANAX2, LGALS1, while the two MS-enriched clusters showed high expression of either CD74, HLA-DRA, HLA-DRB1 or OPN/SPP1, PADI2, LPL (Figure 8). These findings suggest distinct disease-related subtypes of microglia in the MS brain, which were similar to microglia subtypes in a demyelination model. However, subsets of microglia varied substantially between individual patients indicating high inter-individual heterogeneity. Additionally, the different microglia populations appeared as a transcriptional continuum of the local populations, which could reflect the ability of microglia to easily adapt to changes in the surroundings.
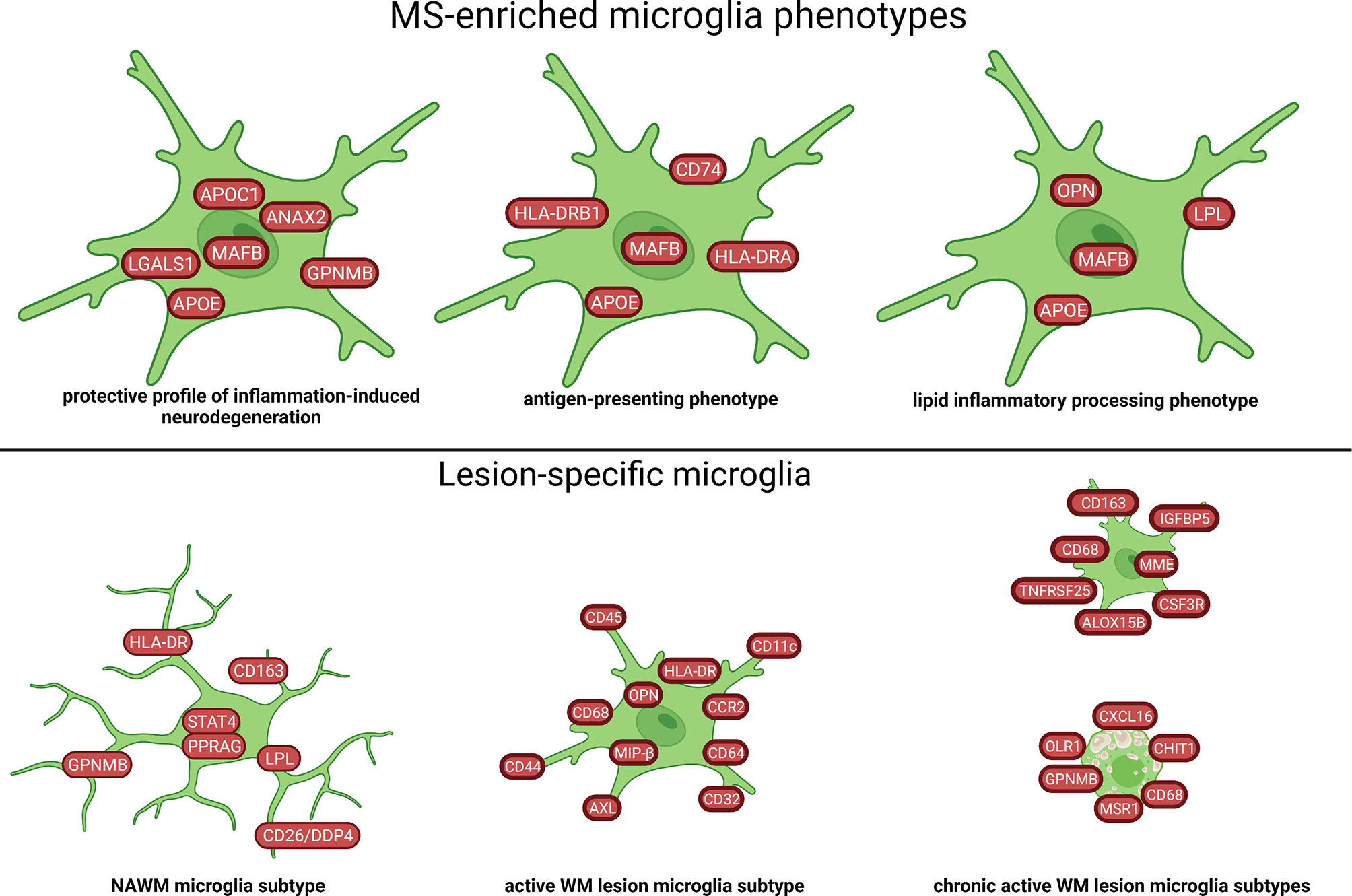
Figure 8 Signature of microglia subtypes in the WM of MS brain based on transcriptome and proteome studies. Different profiles of microglia in WM tissue of MS have been identified. The NAWM microglia subtype in the figure was created by compiling data from several articles, and therefore molecules may not be expressed at the same time. Created with BioRender.com.
Methylome changes within neuronal nuclei in WM suggested alterations in axonal guidance, synaptic plasticity and CREB signaling in MS (42). The CREB activity was reduced in NAWM compared to WM neurons suggesting alteration of CREB signaling prior focal tissue damage (Figure 3). Neurons from MS patients displayed epigenetic alterations affecting several genes of the glutamate/GABA signaling along with interconnected cellular networks (semaphoring/plexin, Slit/ROBO, Shh/Wnt signaling). Lesion-associated changes in genes implicated neuronal projections and synaptic processes (e.g. GABRA5, PRKG1, DLGAP3/SAPAP3) (42) (Figure 4).
3.1.7 Non-Human Transcripts
Amplicon next-generation sequencing of the human endogenous retrovirus (HERV)-W group found very similar transcript level between of WM lesions and control WM but evidence for interindividual differences in HERV-W transcript levels (45). In another study, genome-wide HERVs expression level between MS WM and control WM was not different (65). However, transcripts of HERV-W were reduced in chronic active and repairing lesions. Additionally, four different transcripts of HERV-W on chromosome 7 were only present in the MS brain (65). Another study found HERVs significantly overexpressed in demyelinating brain tissue including several retroviral domains (core, envelope, integrase, reverse transcriptase) (47). However, the overexpression was small. Due to multiple similar HERV transcripts incorporated and spread out throughout the human genome, examination of them is difficult.
Presence of microbial RNA sequences and bacterial antigens were associated with demyelinating brain lesions (66). In the study, they found 29 MS microbial candidate genera from 11 different phyla, most of which were anaerobic.
3.1.8 Databases
Based on these transcriptomics data, novel interactive online databases were generated. The MS Atlas (www.msatlas.dk), comprises comprehensive high-quality transcriptomic profiles of 98 different WM lesion types (53). The user-friendly MS Atlas was designed to provide information about significant expression of candidate genes and their participation in de novo protein-protein interaction networks in different MS lesions (53, 67). The OligoInternode database (https://ki.se/en/mbb/oligointernode), and the single cell expression atlas (https://www.ebi.ac.uk/gxa/sc/experiments/E-HCAD-35/results/tsne) give information about gene expression from single cells in MS lesions.
3.2 Systems Proteomics to Examine Pathological Mechanisms in the MS Brain Tissue
Proteomics has also been developed as a large-scale unbiased tool for identifying final products of cells and post-translational modifications such as phosphorylation, glycosylation and acetylation associated with MS (68, 69). Despite various proteome studies in brains of animal models of MS, only a few proteome studies of MS CNS tissue have been performed (Table 3).
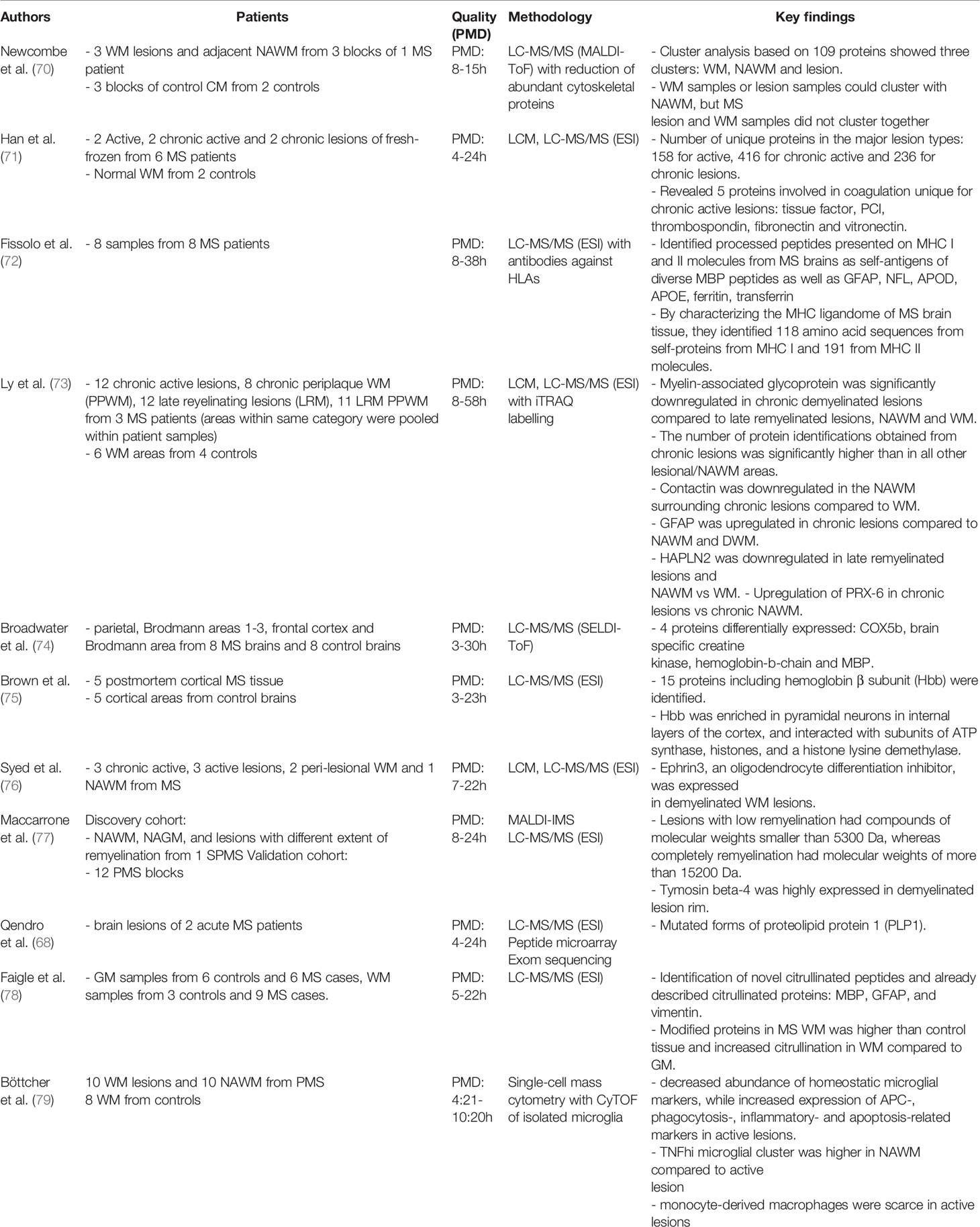
Table 3 An overview of the studies (n=11) that examined the proteome profile in human MS brain tissue.
3.2.1 WM Immune Activity
A proteome study found that 109 proteins could separate WM lesions from adjacent NAWM and control WM (70). Overlap was only observed between NAWM and WM lesions, but not between NAWM and control WM.
To characterize the MHC-bound peptide repertoire in MS brains, proteomics was performed on captured HLA-A, B, C, and DRs. 118 amino acid sequences from MHC I and 191 from MHC II were eluted corresponding to 174 identified proteins including both known and novel autoantigens (72). Some were involved in apoptosis (annexin A1, BCL2-associated TF1), enzymes (GDH, GS, G3PD, NADH dehydrogenase), cytoskeleton (actin, α-ubulin), immune responses (CXCR1, IL12R), CNS structure (NFL, GFAP, MBP, α-synuclein), and serum proteins/iron-related/coagulation (APOD, APOE, ferritin, transferrin, von Willebrand factor). These proteins within the MHC ligandome mirror the proteins involved in different features of the MS pathology.
Combined proteomics and genomics on two acute MS autopsied brain samples detected seven unique mutations of PLP1 (68). This was confirmed with in-depth genomic analysis on mRNA, but not in the genomic DNA, highlighting how results from integrative approaches can strengthen the discovery of specific and precise pathogenic mechanisms in MS.
Myeloid cells from active lesions, NAWM and WM in progressive MS (PMS) were analyzed using single-cell mass cytometry and found lower abundance of microglial homeostatic proteins in active lesions (P2Y12, TMEM119, CXC3R1, GPR56) (79). The myeloid cells in the active lesions were highly phagocytotic and activated indicated by upregulation of CD45, HLADR, CD44, CD114, CD11c, CD68, MS4A4A, CCR2, CD64, CD32, AXL, NFAT1, CD95, Clec7a, CD47, MIP-1β (CCL4) OPN (SPP1) (Figure 4). However, infiltrating myeloid cells were scarce in active lesions in PMS. Additionally, the TNFhi microglia population was reduced in active lesions compared to NAWM.
3.2.2 Two Proteins Important in Remyelination?
Unsupervised clustering of proteomics data led to discovery of cortical lesions, which were not detectable by routine histology (77). They identified tymosin beta-4 mainly expressed in macrophages and activated microglia at the rim of chronic active WM lesions and in the GM (Figure 6). Tymosin beta-4 is involved in neurite extension and plays a role in restoring and remodeling neurons and in remyelination.
Another study found upregulation of the receptor tyrosine kinase Ephrin3 in the MS lesions. Tissue extracts from MS lesions inhibited OPC, while antibody-mediated masking of EphrinB3 epitopes promoted it (76) (Figure 5). These proteomics studies suggest that EphrinB3 and tymosin beta-4 may be potential targets to promote remyelination.
3.2.3 Coagulation and Hemoglobin β
Proteomics of microdissected active, chronic active and inactive lesions showed that chronic active lesions displayed the highest number of uniquely dysregulated proteins, and proteins of unknown function made up more than half of the unique proteins (71). This was supported by an independent study in 2011 (73). Five proteins involved in coagulation were unique to chronic active lesions (tissue factor, PCI, thromobospondin, fibronectin, vitronectin) (71) (Figure 6). Coagulation factors in the CNS interfere with synaptic homeostasis and neuronal networks, and act pleiotropic on different receptors of both resident and circulating cells as well as the extracellular matrix (80).
Another study found dysregulated proteins associated with extracellular matrix, oxidative stress and myelin sheath (73). There was decreased abundance of MAG (oligodendrocytes) and contactin-1 (neurons), while increase in GFAP (astrocytes) in the chronic active lesions in a milieu with abundant anti-oxidant PRX6 and metabolic processes (alfa-enolase).
Proteome studies with co-immunoprecipitation have discovered that hemoglobin β may play a role in neuronal energetics by interacting with histones in the nucleus and by binding to proteins in mitochondria (74, 75) (Figure 7).
3.2.4 Post-Translational Protein Modifications – A Missing Link
Studies on post-translational modifications will be the next layer of valuable information. Recently, a comprehensive analysis of citrullinated peptides in WM and GM of MS patients identified novel citrullinated sites of MBP, GFAP and vimentin, but their functional role remains unknown (78).
4 Discussion
Omics studies of MS brain tissue in the last four decades support MS as a global brain disease with inflammation, iron-disturbances, cellular-stress and hypoxia. However, some regions are more affected than others and the biggest transcriptional changes were detected in the corpus callosum and the optic chiasm (51). While microglia seem to lose the regional specificity in MS, there are similarities between MS microglia phenotypes and the microglia phenotypes during de- and remyelination in the cuprizone model, which also affects mainly the corpus callosum (57, 81). The most affected cell type seems to be oligodendrocyte (30, 34, 51, 56). This may not be surprising as the disease is characterized by demyelination. However, there is a bias towards a higher number of studies investigating the WM than GM. Considering the altered genes, the cell type may be more important than the tissue location, although the local environment, architecture and milieu may continuously drive the cell types into different phenotypic and functional subsets to adapt to the local surroundings.
Molecular components of TGFβ signaling and CREB signaling are altered in addition to multiple changes in semaphorin-, heat shock-, myelin-, APO- and especially multiple types of HLA-transcripts/proteins. Key differentially expressed molecules found multiple times independent of lesion stage are related to inflammatory responses (CD163, OPN, GPNMB, MIP-α/β), lipid metabolism (SCD, LPL, SOD1) cell trafficking (MMP2, CXCL12, VEGFA), but there has been bias in the selection of the examined tissue/cell types.
4.1 Oligodendrocytes
Even in the NAWM, oligodendrocytes have a different molecular profile similar to a survival mode against virtual hypoxia. They upregulate the hypoxia induced HIFα-signaling pathway and the STAT6-signaling pathway, which is associated with anti-inflammatory IL-4 and IL-13 receptor expression (21, 26, 46). The STAT6-signaling seems to be even more increased in oligodendrocytes in all lesion types (34, 48). However, there is a heterogeneity of oligodendrocyte subtypes between different lesion types, where even an immunological phenotype appears (56, 58). This immunophenotypic OPC was also seen at the rim of chronic active lesions (60).
Myelin proteins are altered in all studies including even the GM mitochondrial proteome (74). Nevertheless, different studies showed contradicting results: myelin transcripts and proteins can be reduced in remyelinating lesions (34, 48), while others found them upregulated (30, 76). This could be due to the different stages of remyelinating and remodeling processes captured by omics studies as static snapshots. Understanding the molecular mechanisms in remyelinating lesion using omics may be complicated, as non-coding RNAs dominate and no known predefined pathways have been found (34, 48), but for OPC differentiation FGF1-signaling through astrocytes, EphrinB3 and thymosin beta-4 may be important (30, 76, 82). Mapping the genetic programs of OPC and oligodendrocyte development/polarization in MS may help to unlock and even direct the remyelination process.
4.2 Microglia
Microglia play a role during all stages of lesion evolution in both the GM and WM. Even far from lesions, there are highly activated distinct microglia subtypes (26, 48, 50). This suggests an early activation of their local function, most probably cleanup, which may have been catalyzed by low level of chemokines and cytokines detected throughout the brain. In active lesions, the microglia profile is highly activated, and seems to be the dominated by signal transduction (CD45), immunomodulation (OPN, CD11), antigen-presentation (HLADR) and phagocytotic properties (AXL, CD68, CD163) (79). The MS microglia expressing APOE and MAFB were divided into three subgroups: a protective profile of inflammation-induced neurodegeneration, an antigen-presenting phenotype and an inflammatory lipid-processing phenotype (57). However, there was a decrease in the TNFhigh microglia subgroup in active lesion compared to NAWM (79). In the rim of the chronic active lesions, microglia may have a damaging vs repairing functional phenotype, and by mapping the interactome, microglia strongly interacted with immune cells with involvement of the C1q providing evidence for a lymphocyte-glia axis of lesion progression (60).
4.3 Astrocytes
Being the most abundant cells in the CNS, astrocytes also have altered phenotypes in MS with spatial molecular differences (58). Astrocytes have multiple key functions depending on the surrounding cells and tissue architecture (83). In the NAWM, astrocytes express transcripts associated with iron homeostasis, oxidative stress and immune-related genes (32). GFAP is also increased in remyelinating WM lesions (30, 55). In the GM, astrocytes upregulate the NRF2 and its anti-oxidant target molecules, implying a reparatory and neuroprotective effect (31). However, a pathogenic pro-inflammatory subtype of astrocytes has also been detected and is characterized by reduced expression of NRF2 and increased expression of MAFG/MAT2α. In the chronic active rim, reactive and inflamed astrocytes (AIMS) were detected expressing C3 and an A1-proinflammatory profile and in close interaction with the inflammatory microglia (60). This suggests that astrocytes can polarize to very distinct activation states, which are either damaging or beneficial in the MS pathogenesis. A detailed description of processes towards astrocytic polarization and functional changes are needed, as they can promote brain repair.
4.4 Neurons
Neuronal pathology and axonal injury are hallmarks of MS and major contributors to progression and permanent disability. Neurons in the NAWM have altered expression of genes involved in axonal and synaptic guidance as well as the CREB-mediated neuroprotective signaling pathway (42). NFL and α-synuclein as autoantigens also suggest direct immune attack against neurons (72).
In the GM tissue, TNF signaling seem to play a crucial role, where released TNF binds to TNFR1 on neurons and oligodendrocytes and activates pro-apoptotic/pro-necroptotic pathways leading to brain atrophy (36, 84). CUX2-expressing neurons in the upper cortical layers are most vulnerable for cell stress and death (58). Hemoglobin β in the MS neurons works as an epigenetic regulator and interacts with mitochondrial proteins, both ultimately controlling the energy metabolism (75).
4.5 The Mystery of the Chronic Active Rim
The number of chronic active lesions is increased in the progressive phase and is associated with aggressive disease course and poor clinical prognosis (85). However, it is unclear if the active rim purely expands the lesion, or it represents a cellular/molecular wall to halt progression, or even a battle in between. Moreover, data suggest that even though chronic active lesions are histologically similar, there may be differences on a genomic programming level. As snapshots, omics studies cannot answer if such differences represent distinct molecular mechanisms leading to lesion evolution or rather halting those. Based on multiple transcriptome and proteome studies, chronic active lesion is the most unique WM lesion type: it has the highest number of differentially regulated genes and proteins that may represent end-stage exhaustion, and it differs the most from control WM on molecular levels (48, 71, 73). Some of the unique proteins in chronic active lesions are involved in anti-oxidation and coagulation (71, 73), while many of the transcripts are neuronal/axonal (48). The uniqueness of chronic active lesions has also been identified by distinct and diverse cell populations connected through a lymphocyte-microglia-astrocyte axis that may be responsible for the smoldering inflammation (60).
4.6 Unbalanced Rate of Discovery Research vs Functional Research
Omics studies of tissue alone are very unlikely to lead to new treatments. However, the rate for finding differentially expressed transcripts/proteins and molecular networks is much faster than establishing their functional roles in a specific cell and in a given context. Thus, interpretation can end up with crude functional annotations, and therefore may even confuse results. Interpretation of omics in MS is often annotated to immune cells or immunological properties, even though molecules may have different functions in the brain depending on cell type. Therefore, functional experiments can enhance the interpretation of omics findings in the context of CNS.
4.7 Limitations, Considerations, and Recommendations of Multi-Omics
At least four main problems need to be solved: (i) sample size and quality, (ii) the “snapshot” characteristics of omics (iii) analytic obstacles, integration and gaps of data, (iv) relationship between clinical/pathological classification and tissue systems biology (endophenotypes).
4.7.1 Quality
Sample size is often low due to high experimental cost, the need of specific laboratory equipment, and limited access to human MS brain tissue. Most studies conducted on brain tissue include a restricted number of patients, and overlapping these studies is also complicated due to inter-individual and inter-study variations. Additionally, availability of tissue from the early timeframe of the disease course or from the transition to progression is largely missing. Autopsy brain tissues often represent advanced stages of disease from older patients, while biopsy brain tissue is very limited, taken from specific sites and most often from patients with atypical MS. The postmortem delay of tissue varies considerably even within the same study (Tables 2, 3). In transcriptomic studies, the RNA integrity number (RIN) value is often not mentioned, but the threshold for integrity also depends on which downstream approach is used (Tables 2, 3). Qualities and quantities also differ, where most identified proteins are the highly abundant (86, 87), and low abundant proteins, likely to be involved in the distinct specific processes, remain to be discovered. Consequently, to find the true pathological signatures, reproducible and robust results are needed generated by well-designed studies including sample size power calculation, standardization of experimental as well as computational pipelines and independent validation. Furthermore, the high experimental costs and limited material demand consortiums and larger studies in collaborations across disciplines and nations using experimental and computational consensus pipelines. This kind of international network of MS experts have already begun as with the “Mystery Solved Project” (88).
4.7.2 “Snapshot”
Omics provides only static snapshots of cells at different states in a limited area: only a moment is captured of the highly dynamic variations derived from the cell state kinetics, daily biological rhythms and even stratification of patient populations over time. Longitudinal studies or individual cell trajectory tools might be helpful, but the same cell can only be measured once. To overcome this, increasing the data size by learning a latent factor model would be necessary, which encodes some unknown cell state coupled with the cell type for deconvolution. This leads to another problem, where the rapidly produced comprehensive omics data challenge the current computational methods and tools for integrative analyses.
4.7.3 Analytic Obstacles, Integration and Gaps of Data
There is a danger that too much trust is given to the output data without comprehending, how those data were obtained. Especially, there is no criteria for the sample size, the quality and standardized computational pipelines. Difficulties in combining different datasets have also been emphasized by a comparison of proteome, mRNA and protein abundance profiles of oligodendrocytes and myelin (89). The challenges to develop true robust integrative methods include different modalities, batch effects between experiments, low sequencing depth and high-modality interactions.
Furthermore, directly translate changes in the transcriptome to the dysregulated proteome is improper due to posttranscriptional regulations and spatial and temporal differences in the production of RNA and proteins. On top of that, protein function and turnover are intensely regulated by posttranslational modifications. Phosphorylation and cysteine modifications regulate protein activity; glycosylation affects protein-protein interactions; and ubiquitination affects protein localization and turnover. Activity of a protein, and its abundance in a cell cannot be deduced with certainty from the level of the corresponding mRNA.
Another challenge is to clarify, how single features are associated through multiple interactions across distinct systems and networks, and how to validate them in simplified “artificial” functional assays and models. Functional follow-up studies of the discovered networks and molecules in the right context are required to obtain specific functional annotations as discussed in 4.6. A potential approach to gain full mechanistic insight will require coordinated sets of molecular and cellular multilayer omics data obtained at multiple time points and collected from disease-relevant tissues representing different stages of damage or repair. Additionally, combination of different omics in different human compartments, and combination of omics in the human disease with animal models may help to assess the biological significance (55, 81). However, such combination of omics techniques needs high-level integration. Combination of data-driven and knowledge-driven models into integrative models may define, whether the altered pathways are related to cause or effect. Here, in situ RNAseq will also help in elucidating these aspects of cell-cell interaction without the need of artificial in silico and in vitro modeling.
With the rapid acquirement of data, the concept to understand the heterogeneity of MS may change, starting from the causative molecular signature rather than the clinical phenotype (90). The classic approach (analytical forward approach) (Figure 9) applies omics of a patient group with a particular phenotype and determines, which variants these people have in common. In contrast, analyses may also start from large omics datasets by examination what human variants have in common in a clinical setting and connect it to endophenotypes (biological reverse approach) (Figure 9). Applying this latter strategy for understanding the mechanism behind MS phenotypes, the interaction of functional subsets of single cells and their unique intracellular systems should be analyzed, where macromolecules and key hubs interact with each other in networks. The observed heterogeneity of cell subtypes (endophenotypes) in individual MS lesions may be responsible for the evolution of different lesion types, and the heterogenous composition of these lesion types may contribute to changes in specific brain networks that are ultimately responsible for the clinical heterogeneity (Figure 9). However, here the snapshot problem will also still be an obstacle.
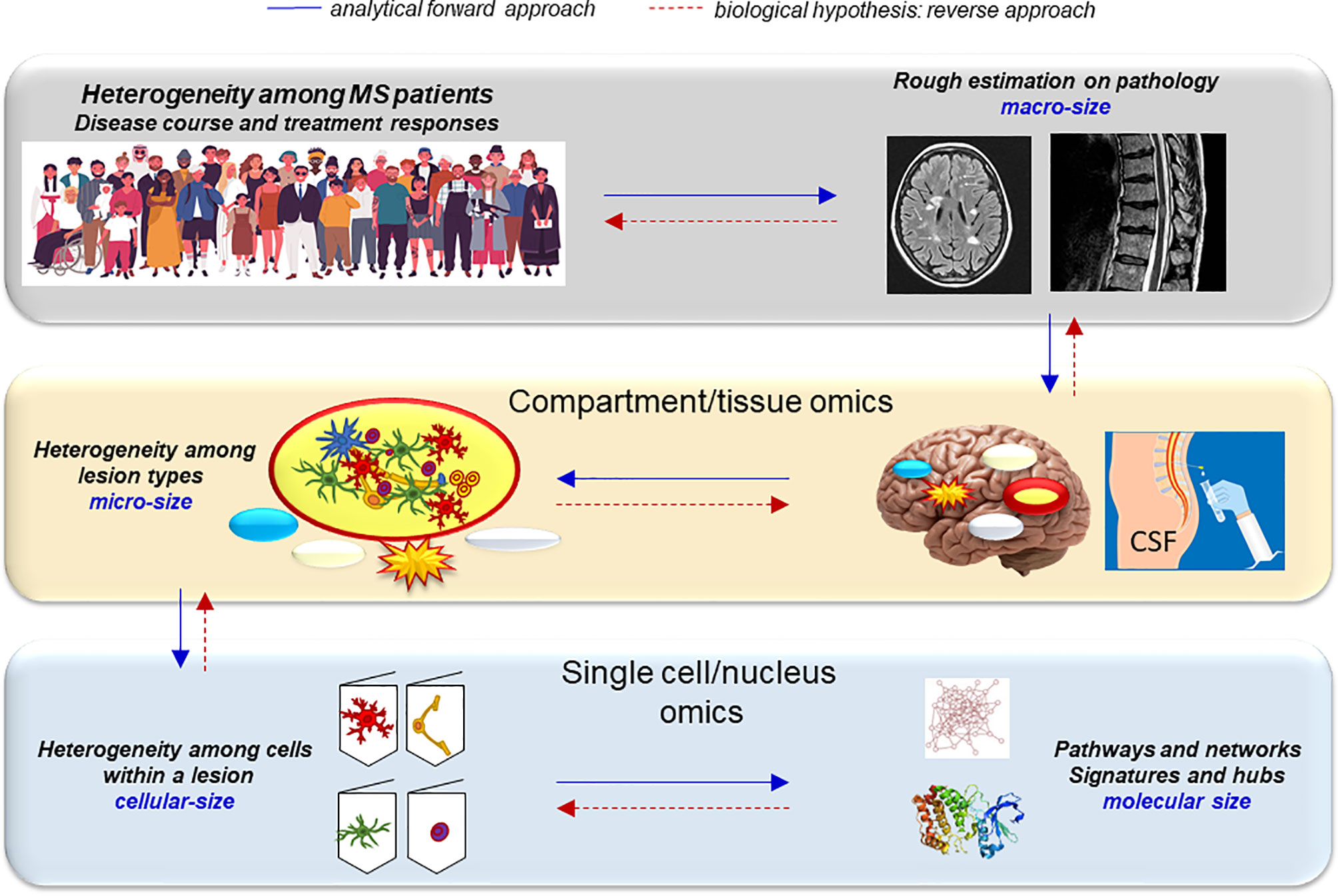
Figure 9 Decoding the heterogeneity of MS with a reverse genetics approach. Analytical forward approach (blue arrows): The heterogeneity of the MS population is reflected by the heterogeneous course of MS and treatment responses. The hallmark of MS, WM brain lesions look similar on conventional MRI scans, but their histopathology is very different: characterized as active, inactive, chronic active and remyelinating/repairing lesions. This heterogeneity is most likely caused by the different cell types present in the lesions that is controlled by the heterogeneity of different networks and pathways activated within the cells and determined by some major hubs and molecular signatures. Biological hypothesis, reverse approach (red arrows): To decode this complexity, a reversed biological approach can be an alternative strategy. It can start from genetic regulation and molecular changes within individual cells that contribute to their fate. This will determine the evolution of lesions, and such complexity of lesion types will determine the individual MS brain and clinical outcomes. MS fate thus ultimately may depend on the interaction of singular cells.
4.7.4 Clinical/Pathological Classification vs Tissue Systems Biology
Finally, MS disease classification is only based on clinical phenotypes and not endophenotypes. Differential signatures in the CSF may reflect the presence of particular lesion types in the brain but also highlight the heterogeneity of lesion/pathogenesis subtypes (endophenotypes) in phenotypically similar patient groups. However, such heterogeneity may also arise from the timepoint of sampling. Avoiding this, repeated analyses of samples with large sample size are needed. While solving the “snap-shot problem”, and also adding the endophenotypic signatures for the patients may specify the pathological events, and thereby use more targeted therapies. A recent study found strong association between severe cortical pathology and a distinctive CSF inflammatory profile (91). Additionally, using positron-emission tomography (PET), potential future targets for biomarkers could be identified in different MS lesion types in vivo. By combining different sources of information, such as omics and structural/functional neuroimaging, it may be possible to obtain a new integrated picture of the pathophysiological process in MS that could span from molecular alterations to cognitive manifestations.
5 Conclusion
Systems biology approach on MS brain tissue may not yet have reached as far as hoped due to tissue availability including different tissue sampling, divergent methodologies, analytic obstacles, gaps of data, and integration of datasets from various sources. Therefore, despite omics studies in MS have been present for decades, it can still be difficult to present an economical summary. However, it clearly revealed that MS is a global brain disease, where all resident brain cells are altered in different degrees. It showed that MS is a more complex and heterogenous disease on molecular level compared to the clinical classification. Paradoxically, this is also reflected in the difficulties of finding validated biomarkers based on omics approaches. Defining endophenotypes may help to disentangle the observed heterogeneity and find common patterns and dysregulated pathways: overcoming the snapshot problem is necessary for such functional interpretations.
Some of the consistent and/or key findings achieved by the systems biology investigations are inflammation within the brain of progressive MS, high levels and multiple types of HLA expression, high neuronal changes in both WM and GM, where TNF signaling is important and that CUX-2-expressing neurons are the most vulnerable; marked oligodendrocyte heterogeneity in the different WM lesion types; pathological/molecular changes in microglia within the NAWM before lesion evolution and distinct functional subgroups during lesion evolution; different astrocyte and microglia polarizations even in slowly expanding lesion rim; and high expression of CXCL12, SCD, STAT6, CD163 and TGFβR2 in all types of WM lesions.
The main power of systems biology is the comprehensive and unbiased approach at a time when out-of-the-box hypotheses for the disease course and progression are needed. Omics-driven data in MS are exponentially growing and if solutions to the major limitations (e.g. sample size, snap-shot problem) are solved, novel hypothesis-driven data can emerge. Applying innovative integrative methods to tissue and single-cell multi-omics combined with extensive interdisciplinary and international collaboration is a logical step forward. This will help give direction for functional experiments and in-depth molecular biological studies.
Data Availability Statement
The original contributions presented in the study are included in the article. Further inquiries can be directed to the corresponding author.
Author Contributions
ME made the systematic article search and wrote the manuscript and made the figures. ZI provided critical feedback and helped shape the manuscript. JB and RR came with input and comments to the manuscript. All authors contributed to the article and approved the submitted version.
Conflict of Interest
The authors declare that the research was conducted in the absence of any commercial or financial relationships that could be construed as a potential conflict of interest.
Publisher’s Note
All claims expressed in this article are solely those of the authors and do not necessarily represent those of their affiliated organizations, or those of the publisher, the editors and the reviewers. Any product that may be evaluated in this article, or claim that may be made by its manufacturer, is not guaranteed or endorsed by the publisher.
Acknowledgments
ME is thankful for the Postdoc Fellowship supported by Lundbeckfonden (R347-2020-2454). ZI is thankful for Scleroseforeningen (R399-A289099, R458-A31829, R431-A29926, R487-A33600, R521-A35417, R561-A38748, R588-A39895) and Independent Reseach Fund Denmark (9039-00370A) and Direktør Ejnar Jonasson kaldet Johnsen og Hustrus Mindelegat (A3458).
References
1. Lublin FD, Reingold SC, Cohen JA, Cutter GR, Sørensen PS, Thompson AJ, et al. Defining the Clinical Course of Multiple Sclerosis: The 2013 Revisions. Neurology (2014) 83:278–86. doi: 10.1212/WNL.0000000000000560
2. Patsopoulos N, Baranzini S, Santaniello A, Shoostari P, Cotsapas C, Wong G, et al. The Multiple Sclerosis Genomic Map: Role of Peripheral Immune Cells and Resident Microglia in Susceptibility. bioRxiv (2017) 143933. doi: 10.1101/143933
3. Wallin MT, Culpepper WJ, Coffman P, Pulaski S, Maloni H, Mahan CM, et al. The Gulf War Era Multiple Sclerosis Cohort: Age and Incidence Rates by Race, Sex and Service. Brain (2012) 135:1778–85. doi: 10.1093/brain/aws099
4. Langer-Gould A, Brara SM, Beaber BE, Zhang JL. Incidence of Multiple Sclerosis in Multiple Racial and Ethnic Groups. Neurology (2013) 80:1734–9. doi: 10.1212/WNL.0b013e3182918cc2
5. Lassmann H. Targets of Therapy in Progressive MS. Mult Scler J (2017) 23:1593–9. doi: 10.1177/1352458517729455
6. Lassmann H. Pathology and Disease Mechanisms in Different Stages of Multiple Sclerosis. J Neurol Sci (2013) 333:1–4. doi: 10.1016/j.jns.2013.05.010
7. Reynolds R, Roncaroli F, Nicholas R, Radotra B, Gveric D, Howell O. The Neuropathological Basis of Clinical Progression in Multiple Sclerosis. Acta Neuropathol (2011) 122:155–70. doi: 10.1007/s00401-011-0840-0
8. Frischer JM, Weigand SD, Guo Y, Kale N, Parisi JE, Pirko I, et al. Clinical and Pathological Insights Into the Dynamic Nature of the White Matter Multiple Sclerosis Plaque. Ann Neurol (2015) 78:710–21. doi: 10.1002/ana.24497
9. Kuhlmann T, Lassmann H, Brück W. Diagnosis of Inflammatory Demyelination in Biopsy Specimens: A Practical Approach. Acta Neuropathol (2008) 115:275–87. doi: 10.1007/s00401-007-0320-8
10. Luchetti S, Fransen NL, van Eden CG, Ramaglia V, Mason M, Huitinga I. Progressive Multiple Sclerosis Patients Show Substantial Lesion Activity That Correlates With Clinical Disease Severity and Sex: A Retrospective Autopsy Cohort Analysis. Acta Neuropathol (2018) 135:511–28. doi: 10.1007/s00401-018-1818-y
11. Kutzelnigg A, Lucchinetti CF, Stadelmann C, Brück W, Rauschka H, Bergmann M, et al. Cortical Demyelination and Diffuse White Matter Injury in Multiple Sclerosis. Brain (2005) 128:2705–12. doi: 10.1093/brain/awh641
12. Milstein JL, Barbour CR, Jackson K, Kosa P, Bielekova B. Intrathecal, Not Systemic Inflammation Is Correlated With Multiple Sclerosis Severity, Especially in Progressive Multiple Sclerosis. Front Neurol (2019) 10:1232. doi: 10.3389/fneur.2019.01232
13. Machado-Santos J, Saji E, Tröscher AR, Paunovic M, Liblau R, Gabriely G, et al. The Compartmentalized Inflammatory Response in the Multiple Sclerosis Brain Is Composed of Tissue-Resident CD8+ T Lymphocytes and B Cells. Brain (2018) 141:2066–82. doi: 10.1093/brain/awy151
14. Rommer PS, Milo R, Han MH, Satyanarayan S, Sellner J, Hauer L, et al. Immunological Aspects of Approved MS Therapeutics. Front Immunol (2019) 10. doi: 10.3389/fimmu.2019.01564
15. Civelek M, Lusis AJ. Systems Genetics Approaches to Understand Complex Traits. Nat Rev Genet (2014) 15:34–48. doi: 10.1038/nrg3575
16. Gibson G, Marigorta UM, Ojagbeghru ER, Park S. PART of the WHOLE: A Case Study in Wellness-Oriented Personalized Medicine. Yale J Biol Med (2015) 88:397–406.
17. Whitney LW, Becker KG, Tresser NJ, Caballero-Ramos CI, Munson PJ, Prabhu VV, et al. Analysis of Gene Expression in Mutiple Sclerosis Lesions Using cDNA Microarrays. Ann Neurol (1999) 46:425–8. doi: 10.1002/1531-8249(199909)46:3<425::AID-ANA22>3.0.CO;2-O
18. Baranzini SE, Elfstrom C, Chang SY, Butunoi C, Murray R, Higuchi R, et al. Transcriptional Analysis of Multiple Sclerosis Brain Lesions Reveals a Complex Pattern of Cytokine Expression. J Immunol (Baltimore Md 1950) (2000) 165:6576–82. doi: 10.4049/jimmunol.165.11.6576
19. Whitney LW, Ludwin SK, McFarland HF, Biddison WE. Microarray Analysis of Gene Expression in Multiple Sclerosis and EAE Identifies 5-Lipoxygenase as a Component of Inflammatory Lesions. J Neuroimmunol (2001) 121:40–8. doi: 10.1016/S0165-5728(01)00438-6
20. Lock C, Hermans G, Pedotti R, Brendolan A, Schadt E, Garren H, et al. Gene-Microarray Analysis of Multiple Sclerosis Lesions Yields New Targets Validated in Autoimmune Encephalomyelitis. Nat Med (2002) 8:500–8. doi: 10.1038/nm0502-500
21. Graumann U, Reynolds R, Steck AJ, Schaeren-Wiemers N. Molecular Changes in Normal Appearing White Matter in Multiple Sclerosis Are Characteristic of Neuroprotective Mechanisms Against Hypoxic Insult. Brain Pathol (2003) 13:554–73. doi: 10.1111/j.1750-3639.2003.tb00485.x
22. Mycko MP, Papoian R, Boschert U, Raine CS, Selmaj KW. cDNA Microarray Analysis in Multiple Sclerosis Lesions: Detection of Genes Associated With Disease Activity. Brain (2003) 126:1048–57. doi: 10.1093/brain/awg107
23. Tajouri L, Mellick AS, Ashton KJ, Tannenberg AEG, Nagra RM, Tourtellotte WW, et al. Quantitative and Qualitative Changes in Gene Expression Patterns Characterize the Activity of Plaques in Multiple Sclerosis. Mol Brain Res (2003) 119:170–83. doi: 10.1016/j.molbrainres.2003.09.008
24. Lindberg RLP, De Groot CJA, Certa U, Ravid R, Hoffmann F, Kappos L, et al. Multiple Sclerosis as a Generalized CNS Disease–Comparative Microarray Analysis of Normal Appearing White Matter and Lesions in Secondary Progressive MS. J Neuroimmunol (2004) 152:154–67. doi: 10.1016/j.jneuroim.2004.03.011
25. Mycko MP, Papoian R, Boschert U, Raine CS, Selmaj KW. Microarray Gene Expression Profiling of Chronic Active and Inactive Lesions in Multiple Sclerosis. Clin Neurol Neurosurg (2004) 106:223–9. doi: 10.1016/j.clineuro.2004.02.019
26. Zeis T, Graumann U, Reynolds R, Schaeren-Wiemers N. Normal-Appearing White Matter in Multiple Sclerosis Is in a Subtle Balance Between Inflammation and Neuroprotection. Brain (2008) 131:288–303. doi: 10.1093/brain/awm291
27. Cunnea P, McMahon J, O’Connell E, Mashayekhi K, Fitzgerald U, McQuaid S. Gene Expression Analysis of the Microvascular Compartment in Multiple Sclerosis Using Laser Microdissected Blood Vessels. Acta Neuropathol (2010) 119:601–15. doi: 10.1007/s00401-009-0618-9
28. Fischer MT, Sharma R, Lim JL, Haider L, Frischer JM, Drexhage J, et al. NADPH Oxidase Expression in Active Multiple Sclerosis Lesions in Relation to Oxidative Tissue Damage and Mitochondrial Injury. Brain (2012) 135:886–99. doi: 10.1093/brain/aws012
29. Mycko MP, Brosnan CF, Raine CS, Fendler W, Selmaj KW. Transcriptional Profiling of Microdissected Areas of Active Multiple Sclerosis Lesions Reveals Activation of Heat Shock Protein Genes. J Neurosci Res (2012) 90:1941–8. doi: 10.1002/jnr.23079
30. Mohan H, Friese A, Albrecht S, Krumbholz M, Elliott CL, Arthur A, et al. Transcript Profiling of Different Types of Multiple Sclerosis Lesions Yields FGF1 as a Promoter of Remyelination. Acta Neuropathol Commun (2014) 2:168. doi: 10.1186/s40478-014-0168-9
31. Licht-Mayer S, Wimmer I, Traffehn S, Metz I, Brück W, Bauer J, et al. Cell Type-Specific Nrf2 Expression in Multiple Sclerosis Lesions. Acta Neuropathol (2015) 130:263–77. doi: 10.1007/s00401-015-1452-x
32. Waller R, Woodroofe MN, Wharton SB, Ince PG, Francese S, Heath PR, et al. Gene Expression Profiling of the Astrocyte Transcriptome in Multiple Sclerosis Normal Appearing White Matter Reveals a Neuroprotective Role. J Neuroimmunol (2016) 299:139–46. doi: 10.1016/j.jneuroim.2016.09.010
33. Hendrickx DAEE, van Scheppingen J, van der Poel M, Bossers K, Schuurman KG, van Eden CG, et al. Gene Expression Profiling of Multiple Sclerosis Pathology Identifies Early Patterns of Demyelination Surrounding Chronic Active Lesions. Front Immunol (2017) 8:1810. doi: 10.3389/fimmu.2017.01810
34. Zeis T, Howell OW, Reynolds R, Schaeren-Wiemers N. Molecular Pathology of Multiple Sclerosis Lesions Reveals a Heterogeneous Expression Pattern of Genes Involved in Oligodendrogliogenesis. Exp Neurol (2018) 305:76–88. doi: 10.1016/j.expneurol.2018.03.012
35. Melief J, Orre M, Bossers K, van Eden CG, Schuurman KG, Mason MRJ, et al. Transcriptome Analysis of Normal-Appearing White Matter Reveals Cortisol- and Disease-Associated Gene Expression Profiles in Multiple Sclerosis. Acta Neuropathol. Commun (2019) 7:1–19. doi: 10.1186/S40478-019-0705-7
36. Magliozzi R, Howell OW, Durrenberger P, Aricò E, James R, Cruciani C, et al. Meningeal Inflammation Changes the Balance of TNF Signalling in Cortical Grey Matter in Multiple Sclerosis. J Neuroinflamm (2019) 16:1–16. doi: 10.1186/S12974-019-1650-X
37. Enz LS, Zeis T, Schmid D, Geier F, van der Meer F, Steiner G, et al. Increased HLA-DR Expression and Cortical Demyelination in MS Links With HLA-Dr15. Neurol Neuroimmunol Neuroinflamm (2020) 7. doi: 10.1212/NXI.0000000000000656
38. Jäckle K, Zeis T, Schaeren-Wiemers N, Junker A, van der Meer F, Kramann N, et al. Molecular Signature of Slowly Expanding Lesions in Progressive Multiple Sclerosis. Brain (2020) 143:2073–88. doi: 10.1093/brain/awaa158
39. Junker A, Krumbholz M, Eisele S, Mohan H, Augstein F, Bittner R, et al. MicroRNA Profiling of Multiple Sclerosis Lesions Identifies Modulators of the Regulatory Protein CD47. Brain (2009) 132:3342–52. doi: 10.1093/brain/awp300
40. Chomyk AM, Volsko C, Tripathi A, Deckard SA, Trapp BD, Fox RJ, et al. DNA Methylation in Demyelinated Multiple Sclerosis Hippocampus. Sci Rep (2017) 7:8696. doi: 10.1038/s41598-017-08623-5
41. Tripathi A, Volsko C, Datta U, Regev K, Dutta R. Expression of Disease-Related miRNAs in White-Matter Lesions of Progressive Multiple Sclerosis Brains. Ann Clin Transl Neurol (2019) 6:854–62. doi: 10.1002/acn3.750
42. Kular L, Needhamsen M, Adzemovic MZ, Kramarova T, Gomez-Cabrero D, Ewing E, et al. Neuronal Methylome Reveals CREB-Associated Neuro-Axonal Impairment in Multiple Sclerosis. Clin Epigenet (2019) 11. doi: 10.1186/s13148-019-0678-1
43. Becker KG, Mattson DH, Powers JM, Gado AM, Biddison WE. Analysis of a Sequenced cDNA Library From Multiple Sclerosis Lesions. J Neuroimmunol (1997) 77:27–38. doi: 10.1016/S0165-5728(97)00045-3
44. Chabas D. The Influence of the Proinflammatory Cytokine, Osteopontin, on Autoimmune Demyelinating Disease. Science (80-) (2001) 294:1731–5. doi: 10.1126/science.1062960
45. Schmitt K, Richter C, Backes C, Meese E, Ruprecht K, Mayer J. Comprehensive Analysis of Human Endogenous Retrovirus Group HERV-W Locus Transcription in Multiple Sclerosis Brain Lesions by High-Throughput Amplicon Sequencing. J Virol (2013) 87:13837–52. doi: 10.1128/JVI.02388-13
46. Huynh JL, Garg P, Thin TH, Yoo S, Dutta R, Trapp BD, et al. Epigenome-Wide Differences in Pathology-Free Regions of Multiple Sclerosis-Affected Brains. Nat Neurosci (2014) 17:121–30. doi: 10.1038/nn.3588
47. Kriesel J, Bhetariya P, Chan B, Wilson T, Fischer K. Enrichment of Retroviral Sequences in Brain Tissue From Patients With Severe Demyelinating Diseases. J Emerg Dis Virol (2017) 3. doi: 10.16966/2473-1846.132
48. Elkjaer ML, Frisch T, Reynolds R, Kacprowski T, Burton M, Kruse TA, et al. Molecular Signature of Different Lesion Types in the Brain White Matter of Patients With Progressive Multiple Sclerosis. Acta Neuropathol Commun (2019) 7:205. doi: 10.1186/s40478-019-0855-7
49. Konjevic Sabolek M, Held K, Beltrán E, Niedl AG, Meinl E, Hohlfeld R, et al. Communication of CD 8 + T Cells With Mononuclear Phagocytes in Multiple Sclerosis. Ann Clin Transl Neurol (2019) acn3.783. doi: 10.1002/acn3.783
50. van der Poel M, Ulas T, Mizee MR, Hsiao CC, Miedema SSM, Adelia, et al. Transcriptional Profiling of Human Microglia Reveals Grey–White Matter Heterogeneity and Multiple Sclerosis-Associated Changes. Nat Commun (2019) 10:1139. doi: 10.1038/s41467-019-08976-7
51. Voskuhl RR, Itoh N, Tassoni A, Matsukawa MA, Ren E, Tse V, et al. Gene Expression in Oligodendrocytes During Remyelination Reveals Cholesterol Homeostasis as a Therapeutic Target in Multiple Sclerosis. Proc Natl Acad Sci USA (2019) 116:10130–9. doi: 10.1073/pnas.1821306116
52. Chiricosta L, Gugliandolo A, Bramanti P, Mazzon E. Could the Heat Shock Proteins 70 Family Members Exacerbate the Immune Response in Multiple Sclerosis? An in Silico Study. Genes (Basel) (2020) 11:1–12. doi: 10.3390/genes11060615
53. Frisch T, Elkjaer ML, Reynolds R, Michel TM, Kacprowski T, Burton M, et al. Multiple Sclerosis Atlas: A Molecular Map of Brain Lesion Stages in Progressive Multiple Sclerosis. Netw Syst Med (2020) 3:122–9. doi: 10.1089/nsm.2020.0006
54. Rodríguez-Lorenzo S, Ferreira Francisco DM, Vos R, Van Het Hof B, Rijnsburger M, Schroten H, et al. Altered Secretory and Neuroprotective Function of the Choroid Plexus in Progressive Multiple Sclerosis. Acta Neuropathol. Commun (2020) 8. doi: 10.1186/s40478-020-00903-y
55. Elkjaer ML, Nawrocki A, Kacprowski T, Lassen P, Simonsen AH, Marignier R, et al. CSF Proteome in Multiple Sclerosis Subtypes Related to Brain Lesion Transcriptomes. Sci Rep (2021) 11. doi: 10.1038/s41598-021-83591-5
56. Jäkel S, Agirre E, Mendanha Falcão A, van Bruggen D, Lee KW, Knuesel I, et al. Altered Huaman Oligodendrocyte Heterogeneity in Multiple Sclerosis. Nature (2019) 566:543–7. doi: 10.1038/s41586-019-0903-2
57. Masuda T, Sankowski R, Staszewski O, Böttcher C, Amann L, Sagar, et al. Spatial and Temporal Heterogeneity of Mouse and Human Microglia at Single-Cell Resolution. Nature (2019) 566:388–92. doi: 10.1038/s41586-019-0924-x
58. Schirmer L, Velmeshev D, Holmqvist S, Kaufmann M, Werneburg S, Jung D, et al. Neuronal Vulnerability and Multilineage Diversity in Multiple Sclerosis. Nature (2019) 573:75–82. doi: 10.1038/s41586-019-1404-z
59. Wheeler MA, Clark IC, Tjon EC, Li Z, Zandee SEJ, Couturier CP, et al. MAFG-Driven Astrocytes Promote CNS Inflammation. Nature (2020) 578:593–9. doi: 10.1038/s41586-020-1999-0
60. Absinta M, Maric D, Gharagozloo M, Garton T, Smith MD, Jin J, et al. A Lymphocyte–Microglia–Astrocyte Axis in Chronic Active Multiple Sclerosis. Nature (2021) 597:709–14. doi: 10.1038/s41586-021-03892-7
61. Zeis T, Probst A, Steck AJ, Stadelmann C, Brück W, Schaeren-Wiemers N. Molecular Changes in White Matter Adjacent to an Active Demyelinating Lesion in Early Multiple Sclerosis: Molecular Changes in MS Periplaque White Matter. Brain Pathol (2009) 19:459–66. doi: 10.1111/j.1750-3639.2008.00231.x
62. Liddelow SA, Guttenplan KA, Clarke LE, Bennett FC, Bohlen CJ, Schirmer L, et al. Neurotoxic Reactive Astrocytes Are Induced by Activated Microglia. Nature (2017) 541:481–7. doi: 10.1038/nature21029
63. Tripathi A, Pandit I, Perles A, Zhou Y, Cheng F, Dutta R. Identifying miRNAs in Multiple Sclerosis Gray Matter Lesions That Correlate With Atrophy Measures. Ann Clin Transl Neurol (2021) 8:1279–91. doi: 10.1002/acn3.51365
64. Fritsche L, Teuber-Hanselmann S, Soub D, Harnisch K, Mairinger F, Junker A. MicroRNA Profiles of MS Gray Matter Lesions Identify Modulators of the Synaptic Protein Synaptotagmin-7. Brain Pathol (2020) 30:524–40. doi: 10.1111/bpa.12800
65. Elkjaer ML, Frisch T, Tonazzolli A, Röttger R, Reynolds R, Baumbach J, et al. Unbiased Examination of Genome-Wide Human Endogenous Retrovirus Transcripts in MS Brain Lesions. Mult Scler J (2021) 27:1829–37. doi: 10.1177/1352458520987269
66. Kriesel JD, Bhetariya P, Wang ZM, Renner D, Palmer C, Fischer KF. Spectrum of Microbial Sequences and a Bacterial Cell Wall Antigen in Primary Demyelination Brain Specimens Obtained From Living Patients. Sci Rep (2019) 9. doi: 10.1038/s41598-018-38198-8
67. Boscia F, Elkjaer ML, Illes Z, Kukley M. Altered Expression of Ion Channels in White Matter Lesions of Progressive Multiple Sclerosis: What Do We Know About Their Function? Front Cell Neurosci (2021) 15. doi: 10.3389/fncel.2021.685703
68. Qendro V, Bugos GA, Lundgren DH, Glynn J, Han MH, Han DK. Integrative Proteomics, Genomics, and Translational Immunology Approaches Reveal Mutated Forms of Proteolipid Protein 1 (PLP1) and Mutant-Specific Immune Response in Multiple Sclerosis. Proteomics (2017) 17:1600322. doi: 10.1002/pmic.201600322
69. Singh V, Stoop MP, Stingl C, Luitwieler RL, Dekker LJ, Van Duijn MM, et al. Cerebrospinal-Fluid-Derived Immunoglobulin G of Different Multiple Sclerosis Patients Shares Mutated Sequences in Complementarity Determining Regions. Mol Cell Proteomics (2013) 12:3924–34. doi: 10.1074/mcp.M113.030346
70. Newcombe J, Eriksson B, Ottervald J, Yang Y, Franzén B. Extraction and Proteomic Analysis of Proteins From Normal and Multiple Sclerosis Postmortem Brain. J Chromatogr B Anal Technol Biomed Life Sci (2005) 815:191–202. doi: 10.1016/j.jchromb.2004.10.073
71. Han MH, Hwang S-I, Roy DB, Lundgren DH, Price JV, Ousman SS, et al. Proteomic Analysis of Active Multiple Sclerosis Lesions Reveals Therapeutic Targets. Nature (2008) 451:1076–81. doi: 10.1038/nature06559
72. Fissolo N, Haag S, De Graaf KL, Drews O, Stevanovic S, Rammensee HG, et al. Naturally Presented Peptides on Major Histocompatibility Complex I and II Molecules Eluted From Central Nervous System of Multiple Sclerosis Patients. Mol Cell Proteomics (2009) 8:2090–101. doi: 10.1074/mcp.M900001-MCP200
73. Ly L, Barnett MH, Zheng YZ, Gulati T, Prineas JW, Crossett B. Comprehensive Tissue Processing Strategy for Quantitative Proteomics of Formalin-Fixed Multiple Sclerosis Lesions. J Proteome Res (2011) 10:4855–68. doi: 10.1021/pr200672n
74. Broadwater L, Pandit A, Clements R, Azzam S, Vadnal J, Sulak M, et al. Analysis of the Mitochondrial Proteome in Multiple Sclerosis Cortex. Biochim Biophys Acta (2011) 1812:630–41. doi: 10.1016/j.bbadis.2011.01.012
75. Brown N, Alkhayer K, Clements R, Singhal N, Gregory R, Azzam S, et al. Neuronal Hemoglobin Expression and Its Relevance to Multiple Sclerosis Neuropathology. J Mol Neurosci (2016) 59:1–17. doi: 10.1007/s12031-015-0711-6
76. Syed YA, Zhao C, Mahad D, Möbius W, Altmann F, Foss F, et al. Antibody-Mediated Neutralization of Myelin-Associated EphrinB3 Accelerates CNS Remyelination. Acta Neuropathol (2016) 131:281–98. doi: 10.1007/s00401-015-1521-1
77. Maccarrone G, Nischwitz S, Deininger SO, Hornung J, König FB, Stadelmann C, et al. MALDI Imaging Mass Spectrometry Analysis—A New Approach for Protein Mapping in Multiple Sclerosis Brain Lesions. J Chromatogr B Anal Technol Biomed Life Sci (2017) 1047:131–40. doi: 10.1016/j.jchromb.2016.07.001
78. Faigle W, Cruciani C, Wolski W, Roschitzki B, Puthenparampil M, Tomas-Ojer P, et al. Brain Citrullination Patterns and T Cell Reactivity of Cerebrospinal Fluid-Derived CD4+ T Cells in Multiple Sclerosis. Front Immunol (2019) 10. doi: 10.3389/fimmu.2019.00540
79. Böttcher C, van der Poel M, Fernández-Zapata C, Schlickeiser S, Leman JKH, Hsiao CC, et al. Single-Cell Mass Cytometry Reveals Complex Myeloid Cell Composition in Active Lesions of Progressive Multiple Sclerosis. Acta Neuropathol Commun (2020) 8. doi: 10.1186/s40478-020-01010-8
80. De Luca C, Virtuoso A, Maggio N, Papa M. Neuro-Coagulopathy: Blood Coagulation Factors in Central Nervous System Diseases. Int J Mol Sci (2017) 18. doi: 10.3390/ijms18102128
81. Martin NANA, Nawrocki A, Molnar V, Elkjaer MLML, Thygesen EKEK, Palkovits M, et al. Orthologous Proteins of Experimental De- and Remyelination Are Differentially Regulated in the CSF Proteome of Multiple Sclerosis Subtypes. PloS One (2018) 13:e0202530. doi: 10.1371/journal.pone.0202530
82. Maccarrone G, Nischwitz S, Deininger S-O, Hornung J, König FB, Stadelmann C, et al. MALDI Imaging Mass Spectrometry Analysis-A New Approach for Protein Mapping in Multiple Sclerosis Brain Lesions. J Chromatogr B Analyt Technol Biomed Life Sci (2016) 1047:1–10. doi: 10.1016/j.jchromb.2016.07.001
83. Kim Y, Park J, Choi YK. The Role of Astrocytes in the Central Nervous System Focused on BK Channel and Heme Oxygenase Metabolites: A Review. Antioxidants (2019) 8. doi: 10.3390/antiox8050121
84. Picon C, Jayaraman A, James R, Beck C, Gallego P, Witte ME, et al. Neuron-Specific Activation of Necroptosis Signaling in Multiple Sclerosis Cortical Grey Matter. Acta Neuropathol (2021) 141:585–604. doi: 10.1007/s00401-021-02274-7
85. Absinta M, Sati P, Masuzzo F, Nair G, Sethi V, Kolb H, et al. Association of Chronic Active Multiple Sclerosis Lesions With Disability in Vivo. JAMA Neurol (2019) 76:1474–83. doi: 10.1001/jamaneurol.2019.2399
86. Stoop MP, Singh V, Dekker LJ, Titulaer MK, Stingl C, Burgers PC, et al. Proteomics Comparison of Cerebrospinal Fluid of Relapsing Remitting and Primary Progressive Multiple Sclerosis. PloS One (2010) 5:e12442. doi: 10.1371/journal.pone.0012442
87. Kroksveen AC, Guldbrandsen A, Vedeler C, Myhr KM, Opsahl JA, Berven FS. Cerebrospinal Fluid Proteome Comparison Between Multiple Sclerosis Patients and Controls. Acta Neurol Scand (2012) 126:90–6. doi: 10.1111/ane.12029
88. Mystery Solved Project | European Charcot Foundation. Available at: https://www.charcot-ms.org/initiatives/mystery-solved-project (Accessed: 19th October 2021).
89. Jahn O, Siems SB, Kusch K, Hesse D, Jung RB, Liepold T, et al. The CNS Myelin Proteome: Deep Profile and Persistence After Post-Mortem Delay. Front Cell Neurosci (2020) 14:239. doi: 10.3389/fncel.2020.00239
Keywords: multiple sclerosis, systems biology, transcriptome, proteome, single cell, brain lesions, NAWM, NAGM
Citation: Elkjaer ML, Röttger R, Baumbach J and Illes Z (2022) A Systematic Review of Tissue and Single Cell Transcriptome/Proteome Studies of the Brain in Multiple Sclerosis. Front. Immunol. 13:761225. doi: 10.3389/fimmu.2022.761225
Received: 19 August 2021; Accepted: 28 January 2022;
Published: 02 March 2022.
Edited by:
Takashi Yamamura, National Center of Neurology and Psychiatry, JapanReviewed by:
Allan G. Kermode, University of Western Australia, AustraliaAndre Ortlieb Guerreiro Cacais, Karolinska Institutet (KI), Sweden
Copyright © 2022 Elkjaer, Röttger, Baumbach and Illes. This is an open-access article distributed under the terms of the Creative Commons Attribution License (CC BY). The use, distribution or reproduction in other forums is permitted, provided the original author(s) and the copyright owner(s) are credited and that the original publication in this journal is cited, in accordance with accepted academic practice. No use, distribution or reproduction is permitted which does not comply with these terms.
*Correspondence: Maria L. Elkjaer, maria.louise.elkjaer@rsyd.dk