- 1School of Public Health, Southern Medical University, Guangzhou, China
- 2Guangdong Provincial Institute of Public Health, Guangdong Provincial Center for Disease Control and Prevention, Guangzhou, China
Background: Metabolic disorders caused by intestinal microbial dysregulation are considered to be important causes of gestational diabetes mellitus (GDM). Increasing evidence suggests that the diversity and composition of gut microbes are altered in disease states, yet the critical microbes and mechanisms of disease regulation remain unidentified.
Methods: PubMed® (National Library of Medicine, Bethesda, MD, USA), Embase® (Elsevier, Amsterdam, the Netherlands), the Web of Science™ (Clarivate™, Philadelphia, PA, USA), and the Cochrane Library databases were searched to identify articles published between 7 July 2012 and 7 July 2022 reporting on case–control and controlled studies that analyzed differences in enterobacteria between patients with GDM and healthy individuals. Information on the relative abundance of enterobacteria was collected for comparative diversity comparison, and enterobacterial differences were analyzed using random effects to calculate standardized mean differences at a p-value of 5%.
Results: A total of 22 studies were included in this review, involving a total of 965 GDM patients and 1,508 healthy control participants. Alpha diversity did not differ between the participant groups, but beta diversity was significantly different. Firmicutes, Bacteroidetes, Actinobacteria, and Proteobacteria were the dominant bacteria, but there was no significant difference between the two groups. Qualitative analysis showed differences between the groups in the Firmicutes/Bacteroidetes ratio, Blautia, and Collinsella, but these differences were not statistically different.
Conclusion: Enterobacterial profiles were significantly different between the GDM and non-GDM populations. Alpha diversity in patients with GDM is similar to that in healthy people, but beta diversity is significantly different. Firmicutes/Bacteroidetes ratios were significantly increased in GDM, and this, as well as changes in the abundance of species of Blautia and Collinsella, may be responsible for changes in microbiota diversity. Although the results of our meta-analysis are encouraging, more well-conducted studies are needed to clarify the role of the gut microbiome in GDM. The systematic review was registered with PROSPERO (https://www.crd.york.ac.uk/prospero/) as CRD42022357391.
1 Introduction
Gestational diabetes mellitus (GDM) is characterized by insufficient insulin secretion and impaired glucose intolerance during pregnancy (1). It has been estimated that over the past 20 years the worldwide prevalence of GDM has been up to 14% of pregnancies, though estimates may vary among regions depending on diagnostic criteria (2). GDM has been associated with adverse pregnancy outcomes among pregnant women including dystocia, macrosomia, neonatal hypoglycemia, and birth injuries (3). In addition, it has been reported that, in the long run, GDM is associated with type 2 diabetes mellitus (T2DM), metabolic syndrome, and cardiovascular disease, making it more potential of developing T2DM than ordinary pregnancies. It is generally believed that GDM develops when pancreatic beta cells fail to produce sufficient insulin to meet the demands of the relevant tissues for blood glucose regulation (4, 5). Therefore, GDM can be considered a manifestation of prediabetes in the form of impaired glucose tolerance in non-pregnant individuals (1). Undoubtedly, diagnosis and treatment can not only reduce the risk of perinatal complications but can also reduce the economic burden on both patients and countries. The human gut microbiota is considered to have a profound influence on host metabolism (6), and alteration of the homeostasis of the intestinal microbiota can have far-reaching consequences. For instance, a reduced number of bacteria such as bifidobacteria and Bacteroides affects lipid metabolism, whereas an increased number of enterobacteria can lead to insulin resistance (6–9). Although previous studies have explored different populations, most have focused on the components of and changes in the gut microbiota in GDM during different pregnancy periods. Few studies have explored differences in the microbiota in patients with GDM and healthy controls. In addition, the results have been inconsistent and findings cannot be reproduced. Specific and meticulous pathogenesis remains to be identified.
Various mechanisms explaining the link between the gut microbiota and GDM have been proposed. A lack of SCFAs (short-chain fatty acids) is one reason that has been suggested. This is particularly common among those consuming Western-style diets, which are known to be low in fiber and digestible carbohydrates, which may contribute to a reduction in microbial diversity and cause microbial dysbiosis. Such diets could also change the profile of gut microbiota, so as to impair the integrity of the wall of the intestine and cause gut permeability. Thus, one effect of insufficient SCFAs may be translocation of toxins from the gut lumen to the systemic circulation (10).
Another proposed mechanism is associated with some other foods, such as fish and red meat. The intestinal flora can produce trimethylamine N-oxide (TMAO) by metabolizing those meat, thus affecting the immune system. In addition, activation of the intracellular thioredoxin-interacting protein (TXNIP) leads to an in increase in the expression of the NLRP3 gene [NOD-like receptor (NLR) family pyrin domain containing 3] and increased inflammatory markers in blood, especially tumor necrosis factor-α (TNF-α), interleukin 6 (IL-6), interleukin 18 (IL-18), and interleukin-1β (IL-1β) (11–13). Dietary TMAO then further increases fasting insulin levels and homeostasis model assessment for insulin resistance (HOMA-IR) by inducing adipose tissue inflammation (14), exacerbating impaired glucose tolerance. The mechanisms affected by the gut microbiota may indicate possible treatments for diabetes mellitus.
The aim of this review was to collect evidence from cohort and case–control studies to provide a theoretical basis for the diagnosis, intervention, and treatment of diseases linked to the gut microbiota by analyzing differences in enterobacteria and exploring inflammation and possible immune mechanisms associated with disease pathophysiology.
2 Materials and methods
The scheme of the metareview has been registered with PROPSERO (as CRD42022357391) and searches were conducted in accordance with the updated 2008 Preferred Reporting Items for Systematic Reviews and Meta-Analyses (PRISMA) statement (15) and checklist.
2.1 Data sources and search strategy
A systematic literature search of four databases [PubMed® (National Library of Medicine, Bethesda, MD, USA), the Cochrane Library, Embase® (Elsevier, Amsterdam, the Netherlands), and Web of Science™ (Clarivate™, Philadelphia, PA, USA)] was conducted by combining medical subject headings (MeSH) words with free words. We selected articles in English published between 7 July 2012 and 7 July 2022 and focused on human studies.
The search strategy was built by combining the following MeSH words with free words: (((“Diet”[Mesh]) OR (“Life Style”[Mesh]) OR (“Exercise”[Mesh]) OR (“Motor Activity”[Mesh]) OR (“Probiotics”[Mesh]) AND ((“Cohort Studies”[Mesh]) OR (Cohort studies [Title/Abstract])) AND ((16S rRNA [Title/Abstract])) AND ((“Diabetes, Gestational”[Mesh])). Various free words were added to improve the search results and identify articles that might otherwise have been missed. The search strings are provided in Supplementary Table 1.
2.2 Eligibility criteria
Titles and abstracts were screened by two investigators. Any disagreements between the two researchers were resolved by a third one. Before the formal literature selection, the three investigators were trained to ensure that they had consistent screening standards. Studies were included if they met the following criteria (1): they were cohort studies or case studies among pregnant women with GDM (2); metagenomics sequencing or 16S rRNA sequence analysis was carried out (3); they reported maternal outcomes such as HbA1c, fasting blood glucose level, and gestational weight gain (4); they were published from 7 July 2012 to 7 July 2022 (5); they were published in English; and (6) the population studied was aged >18 years.
Articles were excluded if they met any of the following criteria (1): they reported on trials that were not carried out in humans (2); they were non-randomized controlled studies (3); they analyzed probiotics in conjunction with other GDM therapies in the same intervention group (4); they were abstracts, case reports, expert opinions, reviews, letters, or editorials; or (5) they lacked sufficient data or did not meet the inclusion criteria.
2.3 Data extraction and synthesis
Articles on pregnant women under 45 years old who had been diagnosed with GDM at a specific time point were chosen. The diagnosis of GDM was confirmed by a national or international standard. A further requirement was that the control groups should be healthy and the GDM group should not also have other metabolic diseases.
All articles were screened, and those deemed ineligible by the two researchers were removed; any disagreements were resolved by the third researcher. Detailed information was then recorded in an Excel spreadsheet. This included basic information such as authors, publication year, district, and study types, as well as maternal outcomes such as HbA1c and fasting blood glucose levels and details of the gut microbiome, including diversities in richness, evenness, and similarity between communities.
2.4 Quality assessment and risk of bias
We used the Newcastle–Ottawa Scale (NOS), which was developed for the assessment of cohort studies and case studies, to score the included studies (16). For the purpose of reaching accurate methodological quality, the NOS was evaluated through three dimensions (1): selection (2), comparability, and (3) outcome. A quality score ranging from 0 to 9 was obtained through a rating algorithm, with a score of 0–5 meaning poor quality, a score of 6–7 meaning moderate quality, and a score of 8–9 meaning high quality. The specific scores of each article according to the NOS are shown in Figure 1 and Supplementary Table 2.
2.5 Statistical analysis
Standardized mean differences (SDMs) were used to summarize and study differences among the studies and relevant measures, and 95% confidence intervals (CI) were used to estimate the differences in gut microbiota diversity between the GDM and the non-GDM groups. Operational taxonomic unit (OTU) data for each study were analyzed using RevMan 5.3 software, provided by the Cochrane Collaboration Network. Meta-analysis was performed using a random-effects model or a fixed-effects model. Sensitivity analyses were performed using the Egger test. Forest plots were created to visualize differences in microbial community structure between samples using a random-effects (RE) model and a fixed-effects (FE) model according to the reported I2-value. An I2-value > 50% and a p-value > 0.05 were considered statistically significant (17).
Qualitative data, such as the relative abundance of specific genera, were recorded for analysis. Subgroup analyses for confounding factors, such as diet, were performed. Because qualitative beta diversity was not provided, descriptive analyses could not be performed. We therefore chose to perform semiquantitative analysis. If two or more articles reported consistent study results, it was considered that the results were related to the disease and were worthy of further exploration and explanation.
3 Results
3.1 Search results and study eligibility
A total of 632 articles identified from four databases (PubMed, 155; Embase, 34; the Cochrane Library, 48; and Web of Science, 395) were retrieved. Of these, 106 articles were duplicates and were removed, 354 articles were excluded because they reported on different study purposes, and 20 were excluded because they described other study designs. After excluding publications that did not meet the inclusion criteria, 22 (8, 18–27) studies remained and were subjected to full-text scanning for this systematic review. The flow chart illustrating the selection process is provided in Figure 1.
Among all the selected studies, 18 studies were conducted in Asia (16 from China, one from Malaysia, and one from Japan). Nine were cohort studies, while 13 were case studies. The age of the participants ranged from 28 to 45 years. The information on participants that was recorded included findings related to blood biochemistry, chronic inflammation, and biomarkers. Most of these studies used the International Association of Diabetes Pregnancy Group’s criteria; only one study, from Thailand, chose its own criteria (22). Owing to different methodologies of analysis, the storage temperature of the stool sample varied (–20°C in one study and –80°C in 19 studies). We focused on the differences emerging when comparing GDM patients with healthy people. One article divided participants into four groups according to their blood pressure and lipid measurement (20). Therefore, we took only the GDM group and the healthy group into consideration. One article did not exclude those who had probiotics or antibiotic treatment during pregnancy (23). Only two articles included the sample size calculation, which makes these studies more reliable than the others (18, 28) (Supplementary Table 4).
3.2 Quality of included studies
Quality was assessed using the NOS. Each study was evaluated based on three criteria, namely selection of the participants, comparability of groups (i.e., absence of confounder bias), and exposure (measurement of outcome influencing). Ten articles were assessed as being of fair quality and 12 as being of good quality, no studies were of poor quality The detailed scores for every study are shown in Figure 2.
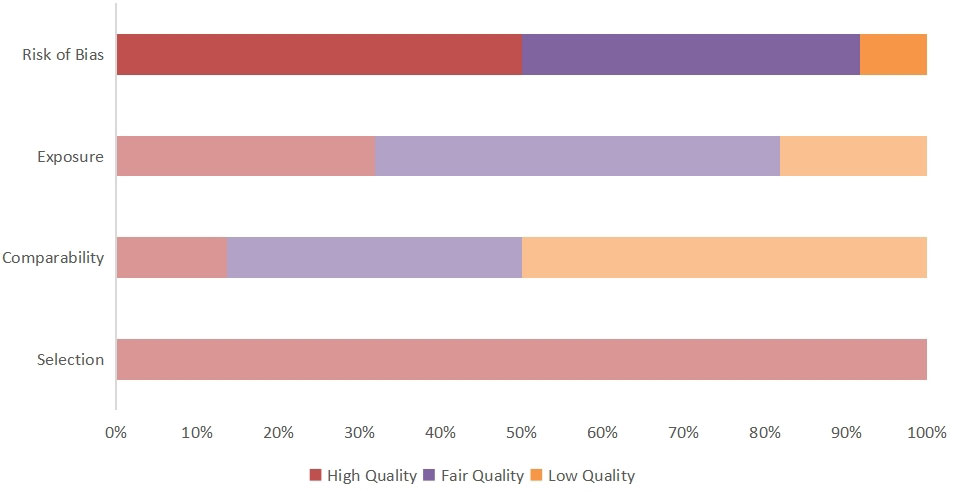
Figure 2 Quality score of included articles calculated using the NOS. The overall article quality score and risk of publication bias are obtained by calculating three indicators.
3.3 GDM detection and study criteria
In general, GDM is diagnosed at 24–28 gestational weeks, using established criteria from the International Association of Diabetes and Pregnancy Study Groups (IADPSG) based on the results of a standard 2-h, 75-g oral glucose tolerance test (OGTT) (29). Pregnant women are diagnosed with GDM if one or more glucose levels is elevated, as follows: fasting ≥ 5.1 mmol/L, 1 h ≥ 10.0 mmol/L, and 2 h ≥ 8.5 mmol/L.
The basic demographics and clinical characteristics of the participants are shown in Supplementary Table 3. The included studies involved 965 participants with GDM and 1,508 healthy people. Only four studies recorded and accounted for pre-study body mass index (BMI) in order to obtain more objective results (18, 19, 28, 30). Participants with other metabolic diseases or who had been treated with antibiotics or probiotics were excluded.
3.4 Methodological characteristics of selected articles about stool sample
Stool samples were generally stored at –80°C, except in one study, in which the samples were stored at –20°C (18). Studies used a wide range of DNA extraction kits. Only the QIAamp Fast DNA StoolMini Kit (Portsmouth, NH, USA) was used more than twice. Target DNA sequencing in the V3–V4 region was the most common technique, used in 15 studies, whereas four studies (20, 21, 31, 32) sequenced in the V4 region, one (23) sequenced in the V1–V2 region, and one (26) sequenced in the V6–V8 region. There were four criteria for defining clustering of OTUs, namely 95% OTUs (1/22), 97% OTUs (13/22), 99% OTUs (5/22), and 100% OTUs (1/22). Taxonomy annotation was conducted mostly with an RDP (Ribosomal Database Project) classifier trained on the SILVA (6/20), RDP (3/22), and Greengenes (5/22) databases. Specific information is shown in Supplementary Table 4.
3.5 Alpha diversity and beta diversity
Diversities are commonly known as alpha diversity and beta diversity. They are used to describe the species composition of the gut microbiota, which may be considered a significant factor influencing outcomes.
Alpha diversity can predict both the number of the species and individual distribution, known as richness and evenness, and can be measured by the ACE (abundance-based coverage estimator), Chao1, Simpson, and Shannon indexes. Among the included studies, four (18, 19, 33, 34) compared the ACE in GDM and non-GDM patients [SMD –0.28 (95% CI –1.98 to 1.42), p = 0.75, I2 = 98%]. Six studies (18, 19, 24, 33–35) reported the Chao1 index [SMD 0.48 (95% CI 0.23 to 0.73), p < 0.05, I2 = 67%] for quality assessment. The Shannon index [SMD –0.06 (95% CI –0.67, 0.94), p = 0.84, I2 = 88%] was provided in six studies (18, 19, 28, 33–35) and the Simpson index in seven studies (18, 19, 23, 24, 33–35) [SMD –0.44 (95% CI –1.30 to 0.41), p = 0.31, I2 = 95%]. The indexes were the same in each group (Figure 3).
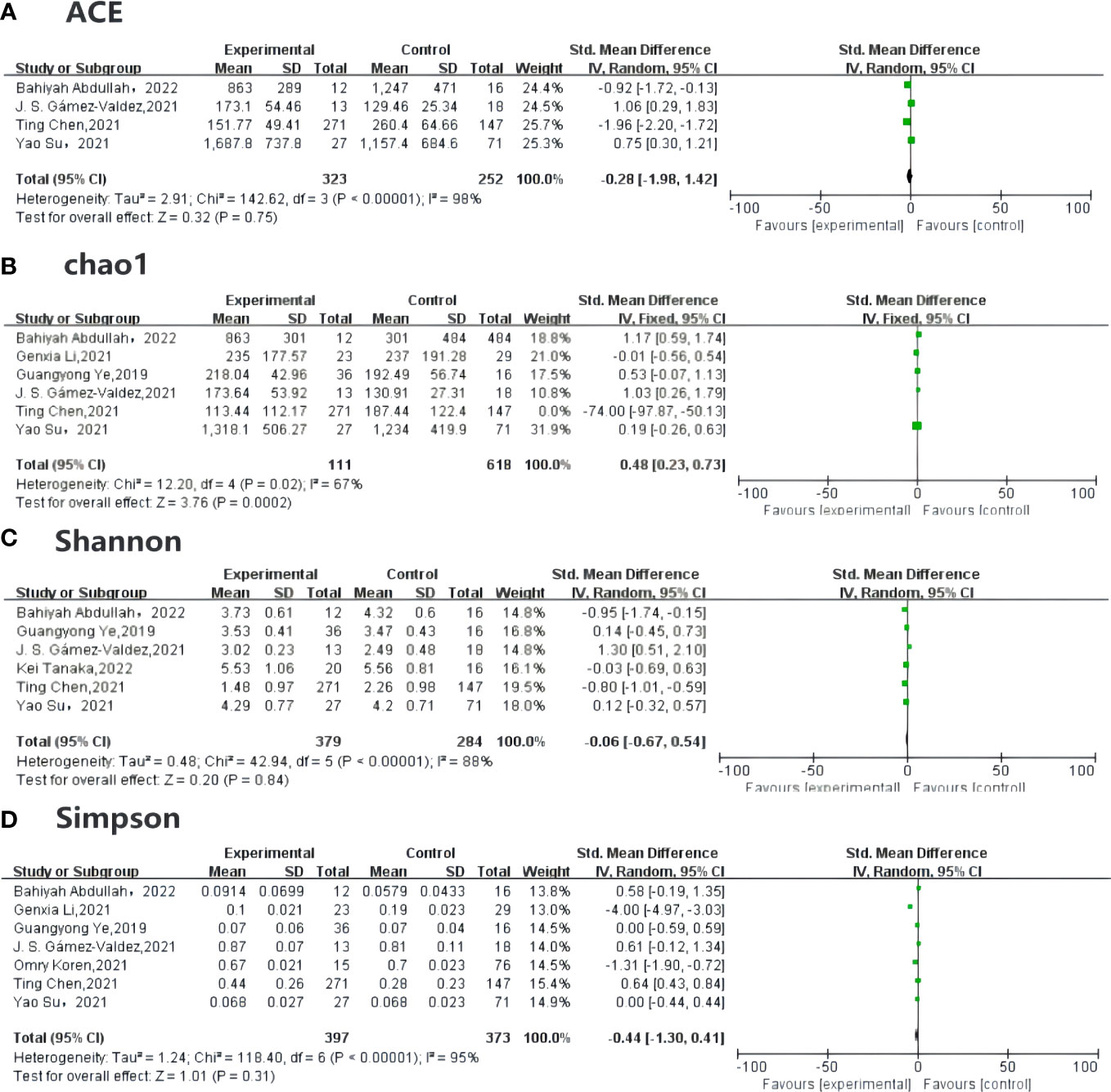
Figure 3 Forest plot of randomized controlled trials comparing the alpha diversity between GDM and NGDM. (A) plot means SDs of ACE, (B) plot described the Chao1between articles, and (C) plot indicates the result for Shannon index, (D) plot mentioned the Simpson index, which are all reported in studies. Differences between groups are presented as weights (percentages) and SMD (95% CI). CI, confidence interval; IV, inverse variance; SD, standard deviation; SMD, standardized mean difference.
The result for the heterogeneity assessment was not as good as we had anticipated. The I2-value was >50%, indicating strong heterogeneity. The source of this heterogeneity should be further explored. Therefore, we further performed sensitivity analyses, omitting each study in turn, to ensure accuracy and stability of the results. If, after removing an article from the indexes, both the I2-value and the p-value were stable, indicating that the article has stable sensitivity analysis results.
In addition, to test our hypothesis that the results were reliable, we assessed the risk of publication bias using Egger’s tests, based on the symmetry of a funnel chart. The results indicated no evidence of publication bias, since the p-value of each index was above 0.05, showing that the conclusions of the meta-analysis were relatively robust. These results can be seen in Supplementary Figure 1.
As for beta diversity,17 articles reported beta diversity, of which 11 used principal component analysis (PCA) and 10 reported results using principal coordinate analysis (PCoA). We were unable to conduct a robust analysis of beta diversity because results were mostly provided in graphical form, rather than as specific data.
3.6 Subgroup analysis
Owing to the significant statistical heterogeneity encountered in the analysis, several subgroup analyses were conducted separately. Analyses of classifications of gut microbiome and dietary intakes were carried out provided these were reported in at least two articles.
Subgroup analysis of the gut microbiome at genus level showed that the abundances of Blautia [SMD 0.36 (95% CI 0.02 to 0.71), p = 0.04, I2 = 0%] and Collinsella [SMD –4.18 (95% CI –8.73 to 0.38), p = 0.38, I2 = 78.9%] were significantly higher in the GDM group than in the control group. There were no differences in-between groups in the abundances of Clostridium [SMD –0.47 (95% CI –0.92 to –0.01), p = 0.187, I2 = 42.4%] and Faecalibacterium [SMD –0.19 (95% CI –0.42 to 0.04), p = 0.971, I2 = 0%].
Similarly, subgroup analysis showed that high fiber intake, compared with lower fiber intake, protects against GDM [SMD –0.96 (95% CI –0.95 to –0.43), p < 0.05, I2 = 92%], whereas there were no differences between patients and control participants in energy intake [SMD –0.46 (95% CI –1.84 to 0.93), p = 0.52, I2 = 97%], cereal intake [SMD –0.31 (95% CI –0.92 to 0.29), p = 0.31, I2 = 86%], meat intake [SMD –0.08 (95% CI –0.31 to 0.15), p = 0.15, I2 = 93%], or milk intake [SMD –0.66 (95% CI –1.55 to 0.24), p = 0.15, I2 = 93%], although there remained considerable heterogeneity in all analyses. Specific results and data can be viewed in Table 1.
3.7 Differences in taxa abundance in the gut microbiota during pregnancy
The current study compared the gut microbiota in patients with GDM and healthy participants. Nine (18, 19, 21, 23, 24, 27, 30, 34, 36) articles analyzed the taxa at the phylum level. Three (18, 19, 24) articles mentioned Firmicutes and concluded that they were less abundant in GDM patients, whereas two (30, 36) articles reported the opposite results. Bacteroidetes are more abundant in GDM patients than in participants without DGM. Two articles (23, 34) reported that the abundance of Actinobacteria was lower in GDM patients than in those without GDM. Four studies (24, 34, 36, 37) reported that the abundance of Proteobacteria was higher in GDM patients than in healthy controls. Only one study (23) reported the opposite. Three studies (24, 27, 30) compared Firmicutes/Bacteroides, two of which (24, 30) found that their abundance was higher in the GDM group.
At the class level, one (21) study mentioned that Rothia, Actinomyces, Bifidobacterium, Adlercreutzia, and Coriobacteriaceae from Actinobacteria were reduced in the GDM population.
At the family level, there were slight differences between studies. Both Veillonellaceae (21, 37) and Prevotella group 9 (30, 37) were increased in GDM patients, whereas Lachnospiraceae and Ruminococcaceae (24) were decreased. Some studies drew controversial conclusions as regard Streptococcaceae, Enterobacteriaceae, Lachnospira, Clostridiales, Clostridia, and Firmicutes. Two (24, 36) studies found that, at the family level, members of the family Clostridiales were increased in GDM patients.
Studies reporting findings at the genus level were the most common. We found enrichment of Ruminococcaceae, Lactococcus, Escherichia, Lachnospiraceae, Clostridia, Alistpes, Firmicutes, and Phascolarctobacterium. Results regarding Streptococcus and Bacteroidetes varied. We also found enrichment of Coprococcus, Staphylococcus, Oscillospira, Burkholderiales, Akkermansia, Prevotella group 9, and Faecalibacterium in participants without GDM. Abundances of Blautia, Staphylococcus, Sutterella, Oscillospira, Enterococcus, and Lactobacillus also varied, and this needs further study.
We performed further random forest analysis of four dominant bacteria. Proteobacteria are relatively abundant in GDM [SMD –0.44 (95% CI –1.3 to 0.41), p = 0.31, I2 = 95%], whereas the abundances of Actinobacteria [SMD –1.64 (95% CI –2.32 to –0.95), p < 0.05, I2 = 94%], Bacteroides [SMD 7.96 (95% CI –7.77 to 23.69), p = 0.32, I2 = 100%], Firmicutes [SMD 0.25 (95% CI –0.01 to 0.51), p = 0.06, I2 = 49%], and Proteobacteria [SMD 1.10 (95% CI –1.59 to 3.80), p = 0.42, I2 = 99%] did not differ significantly between the two groups.
3.8 Types of gut microbiota and their impacts on GDM
To further explore the potential correlations of key clinical indexes with altered gut microbiome in GDM, correlation analyses were performed using Spearman analysis. The results showed that phylum Bacteroidetes was positively associated with 1hPG, whereas Proteobacteria, Verrucomicrobiota, and Actinobacteria were all negatively associated with 1-hour plasma glucose level (1hPG) levels. Analysis at the genus level revealed a negative association between Ruminococcaceae UCG014 and 1hPG, but a positive association between Ruminococcaceae UCG014 and high-density lipoprotein (HDL) levels (36). The genus Akkermansia was negatively correlated with 1hPG and positive correlated with HDL levels. According to Chen et al., genera of Clostridiales, Ruminococcaceae and Lachnospiraceae, within the phylum Firmicutes, were significantly negatively correlated with at least one OGTT value (19). An unassigned genus of Enterococcaceae within the phylum Firmicutes, the genus Atopobium within the phylum Actinobacteria and the genus Sutterella within the phylum Proteobacteria were significantly positively associated with 1-h or 2-h OGTT values (Supplementary Table 5; Figure 4).
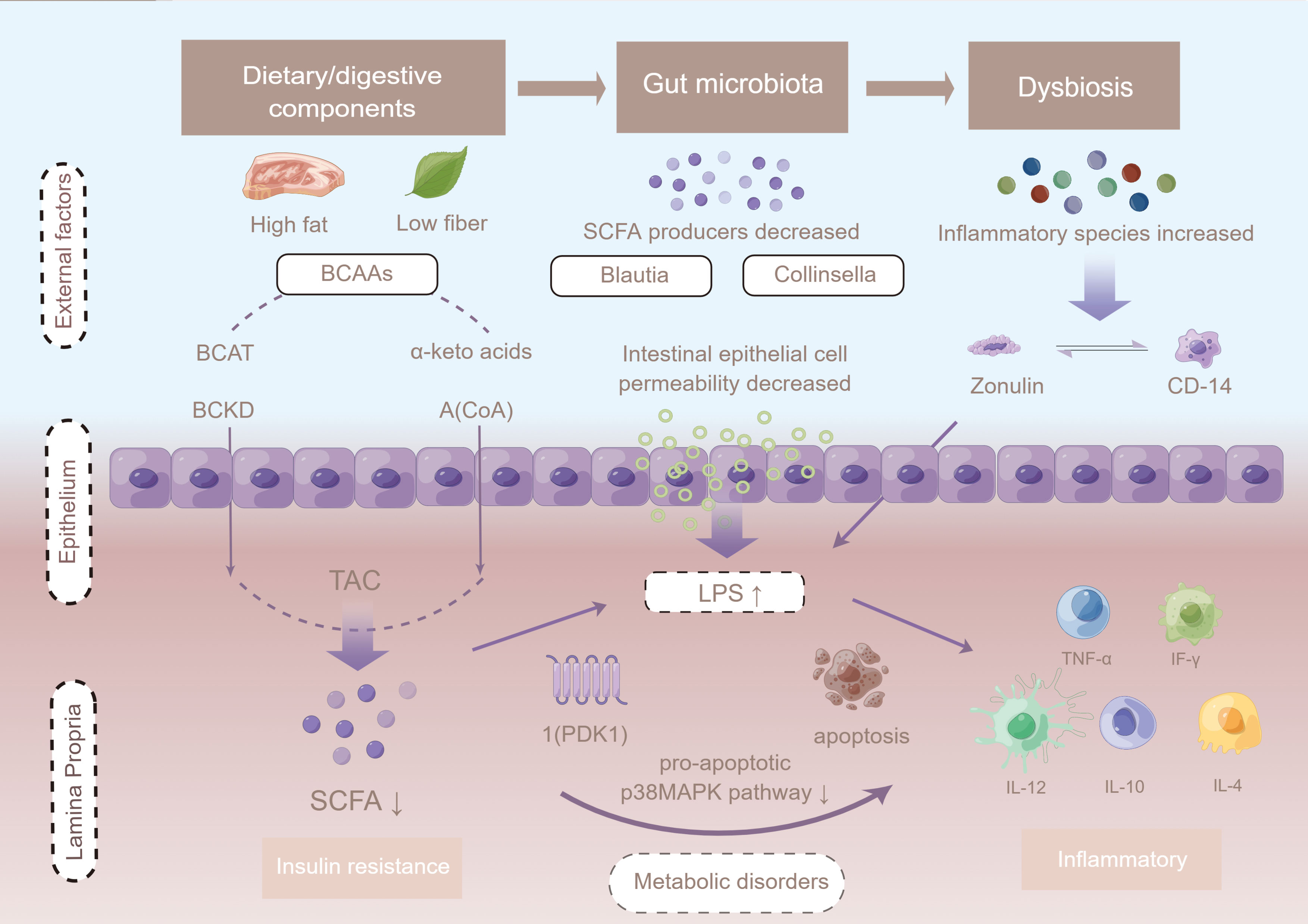
Figure 4 The potential mechanism linking gut dysbiosis and GDM. Intestinal permeability is regulated by dietary factors and the zonulin pathway. Cells in the basal layer of the intestinal epithelium secrete zonulin and bind to initiate complex intracellular signaling pathways, allowing phosphorylation of tight junctions. When LPS is ready to enter the advocate circulation, it increases absorption, and forms a complex with LBP that further binds CD14 released from monocytes, leading to the production of pro-inflammatory cytokines mediated by the MD2/TLR4 receptor complex, such as TNF-α, interleukin 1 (IL-1), and interleukin 6 (IL-6). LPS infiltrates peripheral adipose tissue and binds to TLRs, thus activating the adaptor proteins MyD-88, IRAK, TAK1, and TRAF6, and triggering macrophage infiltration and up-regulation of inflammatory pathways. Up-regulation of JNK/IKKβ/NF-κB may increase serine phosphorylation of IRS-1 + Ser307, resulting in PI3-K inhibition and Akt down-regulation of Ser473. Figure has been obtained for use of copyrighted material from Figuer (https://www.figdraw.com/, registered ID: 533420148).
Indexes of inflammation, fecal calprotectin (FCALP), lipopolysaccharide (LPS), lipopolysaccharide-binding protein (LBP), and fecal LPS (FLPS), were reported in two (20, 26) studies. Levels of zonulin, FCALP, LPS, LBP, and FLPS were higher in GDM patients than in those without GDM, and the results were statistically significant. Cui et al. reported that Enterococcus and Vagococcus are in direct proportion to FCALP and LPS, Streptococcus is inversely proportional to LBP, and Staphylococcus is directly proportional to FLPS (20). Women’s diet, including total energy and fiber intake, remained unchanged between sampling times.
Four articles reported Kyoto Encyclopedia of Genes and Genomes (KEGG) pathway analysis. The conclusions drawn in each article vary widely. Chen et al. identified several microbial gene pathways including the glycan biosynthesis and vitamin metabolic pathways (19). Li et al. showed that the species of gut microbes found in normoglycemic pregnant women (NOR) and GDM are involved in cell wall/membrane/envelope biogenesis, organic ion transport and metabolism, post-translational modifications, protein turnover, chaperones, transcription, unknown function, intracellular transport, secretion, and vesicular transport (24). Su et al. found a positive relationship between Bacteroides species enriched in patients with GDM, and amino sugar and nucleotide sugar metabolism (34). Wang et al. found that the predicted metagenome of women who developed GDM was enriched in organisms involved in starch and sucrose metabolism, whereas those implicated in lysine biosynthesis and nitrogen metabolism were reduced (37).
4 Discussion
To the best of our knowledge, this is the first article to systematically analyze how enterobacterial differences affect metabolic health in GDM patients and healthy individuals. Consistent evidence has shown that the composition of the gut microbiota is specifically altered in GDM. We found differences between groups in the abundance of microorganisms. These suggested that an increase in Blautia and a reduction in Clostridium may make a huge contribution, and thus may provide bacterial targets to prevent or treat GDM by reconstructing the homeostasis of the gut microbiota. Future studies in this area are warranted. The primary purpose of this study was to determine the validations between the participant groups, which may provide insights into possible mechanistic links between the gut microbiota and GDM and the pathway leading to disease development. The available results suggested an association between microbial composition and disease. Despite differences in lateral suction patterns, diversity and composition results were generally consistent between studies. Alpha diversity is widely used for measuring the richness and evenness of the gut microbiome. Our meta-analysis demonstrated a significant association between the presence of GDM and reductions in diversity indexes, suggesting that species richness was reduced in the affected individuals. Although alpha diversity has been shown to be a marker of chronic diseases, such as T2DM, colorectal cancer, and non-alcoholic fatty liver disease (NAFLD), multiple studies of the gut microbiome in patients withT2DM have shown no statistically difference in alpha diversity among well-matched participants (38–42).
Some studies found that alpha diversity was slightly lower, but not significantly reduced, in T2DM patients than in healthy participants (41). Occasionally, richness (as measured by the Shannon and Simpson indexes), which has been associated with elevated insulin resistance, shows an overlapping trend in GDM patients and healthy individuals. However, our meta-analysis results showed that there were no significant differences in diversity or richness between GDM patients and healthy individuals, indicating that alpha diversity may not be a hallmark indicator distinguishing between individuals with and without GDM. In contrast to alpha diversity, beta diversity differed in the GDM group and the control group participants, highlighting the fact that the profile of gut microbiota was altered in GDM. Further studies at the phylum level identified the taxa of the gut microbiota whose abundance was altered by GDM and suggested that an increase in Bacteroides and Proteobacteria and a reduction in Actinobacteria may contribute to GDM.
The phyla identified after in vitro fecal fermentation were mainly Firmicutes, Bacteroides, Actinobacteria, and Proteobacteria. In addition, this review found that the Firmicutes/Bacteroidetes ratio was significantly higher in GDM patients than in healthy control participants. Firmicutes and Bacteroides are the two dominant bacterial groups in the gut. They can maintain energy balance in the host by participating in the metabolism of fats and bile acids. The Firmicutes/Bacteroides ratio is commonly used as a marker of low-grade systemic inflammation in obesity and insulin resistance and as an indicator of gut microbiota composition in different individuals (43–46). Similar to other findings (47, 48),the Firmicutes/Bacteroides ratio was higher in GDM patients, indicating that it is a sensitive indicator enabling patients with GDM to be distinguished from those who do not have GDM. Animal experiments have shown that colonization of the normal gastrointestinal tract, as shown in the cultivate experiments of Bacteroides species and meditated through toll-like receptors (TLRs) and other specific host–microbe interactions is a result of recognition and selection by the host immune system (49). In general, insulin resistance is associated with a higher Firmicutes/Bacteroides ratio and a reduction in the number of butyrate-producing bacteria, while Bacteroidetes, as the most stable component of the gastrointestinal microbiota in healthy adults, contains most genera that produce butyrate (50). Butyrate is considered a health-promoting molecule because it can increase insulin sensitivity, exert anti-inflammatory activity, regulate energy metabolism, and increase leptin gene expression (51–54). Propionate in the colon stimulates the release of glucagon-like peptide 1 (GLP-1) and peptide YY (PYY) from L-enteroendocrine cells, thereby suppressing appetite (55). It may also reach the portal circulation and get captured by the liver tissue, where it participates in hepatic gluconeogenesis and reduces the expression of enzymes involved in fatty acid and cholesterol synthesis (56).
Blautia has shown a significant negative correlation with many diseases, including type 1 diabetes mellitus (T1DM), obesity, and Crohn’s disease (57, 58). As a risk marker of adiposity and of cardiovascular and metabolic disease, Blautia abundance has been shown to be inversely associated with visceral fat tissue (58). This may be due to a potential anti-inflammatory effect that can reduce the ratio of TNF-α to IL-4 in T2DM patients, balance immunity with anti-inflammation, and help maintain glucose homeostasis, so as to regulate the transduction of insulin signaling, thereby regulating the conduction of insulin signals such as fasting blood glucose (FBG) level (59–61). The abundance of Blautia in patients with T1DM is consistent with HbA1c and FBG results (57). This study did in fact find that Blautia abundance was slightly increased in GDM patients, which may be because disease was most often diagnosed in the first trimester (62). From the first to the third trimesters, Blautia levels gradually declined, resulting in a decrease in butyrate production, stimulating neutrophils and macrophages to release inflammatory factors (63). An increase in inflammatory factors is associated with low levels of fiber intake, which may lead to the metabolism, and thereby cause dysbiosis and aggravated inflammation (64).
The genus Collinsella from the family Lachnospiraceae is often described as a strictly anaerobic pathobiont that produces lactate, which is often correlated with SCFAs (65). The decreased abundance of this taxon is associated with the health status of patients suffering from T1DM and T2DM (41). This genus of bacteria seems to be stimulated by a low-fiber diet, which could be observed in GDM patients. Both in vitro and animal experiments have concluded that SCFAs modulate intestinal inflammation by improving transepithelial resistance, altering various signaling pathways, and inhibiting pro-inflammatory cytokines, while up-regulating anti-inflammatory cytokines (66, 67). SCFAs stimulate glucagon production and signal the hypothalamus as a mechanism of diabetes. Butyrate suppresses the production of pro-inflammatory cytokines, for example TNF-α, IL-12, and interferon γ (IF-γ), and up-regulates the production of anti-inflammatory IL-10 by monocytes, thereby producing anti-inflammatory effects (53). Butyrate has been shown to attenuate LPS-stimulated pro-inflammatory effects (68, 69). Intake of a high-fat and low-fiber diet may alter the normal composition of gut microbiota and dietary fermentation. Alterations in dietary fermentation may lead to excessive production of SCFAs and absorption of energy from the diet. The relative abundance of Firmicutes was elevated, while the abundances of Actinobacteria and Bacteroidetes were lower, in women with GDM (70). This “gut microbiota signature” is similar to the phenotype of metabolic disorder, which is mainly due to the obese phenotype (71). Moreover, metabolic pathways mentioned in research are those linked with carbohydrate metabolism, such as glycolysis/gluconeogenesis, starch and sucrose metabolism, and galactose metabolism, which can be enriched in women with GDM (64). The results of our investigation of dietary status showed no difference in energy intake between the two groups of individuals (i.e., those with and without GDM), but that dietary fiber intake was lower in the GDM group in the non-GDM population, suggesting a possible mechanism of GDM. Butyrate-producing bacteria, such as Faecalibacterium and Akkermansia responses the same trend as on fiber intake in the context of the entire diet (72, 73). Thus, it is clear that dietary fiber components are enhanced with the microbiota.
Intestinal microbes also regulate the process of absorption of metabolites and endotoxins by affecting intestinal permeability. It has been shown that the development of GDM is associated with an increase in LPS in the intestine during late pregnancy as well as intestinal mucosal injury characterized by elevated levels of serum LPS and streptoglobulin. In this study, the level of inflammatory factors was significantly higher in GDM patients than in those without GDM, which is consistent with specific physiological changes. Intestinal permeability is regulated by dietary factors and the zonulin pathway. Cells in the basal layer of the intestinal epithelium secrete zonulin and bind to initiate complex intracellular signaling pathways, allowing phosphorylation of tight junctions, which in turn leads to an increased permeability (74). When LPS is ready to enter the advocate circulation, it would increase absorption, and form a complex with LBP that further binds CD14 from monocytes (75). This may lead to the production of pro-inflammatory cytokines mediated by the MD2/TLR4 receptor complex, such as TNF-α, IL-1, and IL-6 and LPS, which infiltrate peripheral adipose tissue and bind to TLRs, activating the adaptor proteins MyD-88, IRAK, TAK1, and TRAF6, and as a result triggering macrophage infiltration and up-regulation of inflammatory pathways. Up-regulation of JNK/IKKβ/NF-κB may increase serine phosphorylation of IRS-1 + Ser307, resulting in PI3-K inhibition and Akt down-regulation of Ser473. Reducing acetate Ser473 phosphorylation may impair insulin signaling and reduce glucose uptake in peripheral tissues, leading to hyperglycemia in women with GDM (74, 76).
Previous studies have shown that the efficiency of energy extraction from the diet is correlated with the enrichment of specific metabolic pathways, particularly those involved in carbohydrate transport and utilization (77). Amino acids are also insensitive to insulin action. Isoenzyme branched-chain amino acid aminotransferase (mitochondrial BCAT and cytosolic BCAT) catalyze the first reversible transamination/deamination of branched-chain amino acids (BCAAs) to their corresponding α-ketoacids. They are then combined in order to convert α-ketoglutarate into glutamate; leucine, isoleucine, and valine yield α-ketoisocaproate (KIC), α-keto-β-methylglutarate (KIM), and α-ketoisovalerate (KIV), respectively (78). The second step of BCAA catabolism is mainly regulated by the catalytic activity of the branched-chain ketoacid dehydrogenase (BCKD) complex (79). Branched-chain acyl-coenzyme A (CoA) species are produced from their cognate α-ketoacids (80). Complete metabolism of BCCA species generates cataplerotic metabolites that are subsequently used in the tricarboxylic acid (TCA) cycle for the generation of fuel (i.e., ATP), using lipogenic, ketogenic, or glucogenic substrates (81–83). In addition, down-regulated BCAA catabolic genes prominent in the adipose tissue of participants with elevated BCAA serum concentrations were correlated with high HOMA-IR values (p < 0.05) (84). It has been shown that bacteria of the genus Lactobacillus help maintain metabolic homeostasis by improving amino acid metabolic pathways in metabolically impaired mice to better compensate for impaired aryl hydrocarbon receptor (AhR) signaling by increasing the availability of intestinal metabolites capable of signaling through the AhR (85). It has been reported from experimental studies that the gut microbiota can directly utilize tryptophan and produce bacteria-derived indole (86, 87). Examples include indolyl sulfate and p-cresol sulfate, which stimulate GLP-1 and increase secretion insulin from pancreatic beta cells (83, 88, 89). Similarly, results from the KEGG enrichment analysis showed that by far the most-downregulated pathways were BCAA degradation pathways (74).
This review has several deficiencies. First, the small sample size means that the accuracy of the study is low. Second, most articles did not provide sufficient specific indicators of gut microbial diversity and composition, making accurate quantitative analysis impossible. As a result, the evidence provided by these studies is insufficient. Third, as a result of limited data, we were unable to stratify patients by sampling time, diet, obesity, Asian-European factors, etc. Mechanistic insight into the gut microbiota is still limited, so further study is needed to identify specific biomarkers and the mechanisms by which they cause disease.
Data availability statement
The original contributions presented in the study are included in the article/Supplementary Material. Further inquiries can be directed to the corresponding authors.
Author contributions
MY, XG and DH wrote the manuscript and researched the data. MY and RH researched data, contributed to discussion, and reviewed the manuscript. SC, ZL, RH, and DZ contributed to the discussion and reviewed the manuscript. All authors read and approved the final version of the manuscript, and take responsibility for the integrity of the data and the accuracy of the data analysis.
Funding
The Guangdong Provincial Key Research and Development Program (2019B020210002), the National Key Research and Development Program of China (2018YFA0606200), the Guangdong Provincial Science and Technology Program (2018B020207006), the Guangdong Key Area R & D Plan Project (2019B020230001), and the National Key R & D Plan Project (2018YFC1314105) supported this study.
Acknowledgments
The author would like to acknowledge the Guangdong Institute of Public Health in Guangdong Provincial Center for Disease Control and Prevention.
Conflict of interest
The authors declare that the research was conducted in the absence of any commercial or financial relationships that could be construed as a potential conflict of interest.
Publisher’s note
All claims expressed in this article are solely those of the authors and do not necessarily represent those of their affiliated organizations, or those of the publisher, the editors and the reviewers. Any product that may be evaluated in this article, or claim that may be made by its manufacturer, is not guaranteed or endorsed by the publisher.
Supplementary material
The Supplementary Material for this article can be found online at: https://www.frontiersin.org/articles/10.3389/fimmu.2022.1097853/full#supplementary-material
References
1. Szmuilowicz ED, Josefson JL, Metzger BE. Gestational diabetes mellitus. Endocrinol Metab Clinics North America (2019) 48(3):479–93. doi: 10.1016/j.ecl.2019.05.001
2. Siregar DAS, Rianda D, Irwinda R, Dwi Utami A, Hanifa H, Shankar AH, et al. Associations between diet quality, blood pressure, and glucose levels among pregnant women in the Asian megacity of Jakarta. PloS One (2020) 15(11):e0242150. doi: 10.1371/journal.pone.0242150
3. Shen Y, Li W, Leng J, Zhang S, Liu H, Li W, et al. High risk of metabolic syndrome after delivery in pregnancies complicated by gestational diabetes. Diabetes Res Clin practice (2019) 150:219–26. doi: 10.1016/j.diabres.2019.03.030
4. Buchanan TA, Xiang AH, Page KA. Gestational diabetes mellitus: risks and management during and after pregnancy. Nat Rev Endocrinol (2012) 8(11):639–49. doi: 10.1038/nrendo.2012.96
5. Petersen JS, Dyrberg T, Damm P, Kühl C, Mølsted-Pedersen L, Buschard K. GAD65 autoantibodies in women with gestational or insulin dependent diabetes mellitus diagnosed during pregnancy. Diabetologia (1996) 39(11):1329–33. doi: 10.1007/s001250050578
6. Wang J, Zheng J, Shi W, Du N, Xu X, Zhang Y, et al. Dysbiosis of maternal and neonatal microbiota associated with gestational diabetes mellitus. Gut (2018) 67(9):1614–25. doi: 10.1136/gutjnl-2018-315988
7. Wickens K, Barthow C, Mitchell EA, Kang J, van Zyl N, Purdie G, et al. Effects of lactobacillus rhamnosus HN001 in early life on the cumulative prevalence of allergic disease to 11 years. Pediatr Allergy Immunol Off Publ Eur Soc Pediatr Allergy Immunol (2018) 29(8):808–14. doi: 10.1111/pai.12982
8. Kuang YS, Lu JH, Li SH, Li JH, Yuan MY, He JR, et al. Connections between the human gut microbiome and gestational diabetes mellitus. GigaScience (2017) 6(8):1–12. doi: 10.1093/gigascience/gix058
9. Sanmiguel C, Gupta A, Mayer EA. Gut microbiome and obesity: A plausible explanation for obesity. Curr Obes Rep (2015) 4(2):250–61. doi: 10.1007/s13679-015-0152-0
10. Woldeamlak B, Yirdaw K, Biadgo B. Role of gut microbiota in type 2 diabetes mellitus and its complications: Novel insights and potential intervention strategies. Korean J Gastroenterol = Taehan Sohwagi Hakhoe chi (2019) 74(6):314–20. doi: 10.4166/kjg.2019.74.6.314
11. Zhu W, Gregory JC, Org E, Buffa JA, Gupta N, Wang Z, et al. Gut microbial metabolite TMAO enhances platelet hyperreactivity and thrombosis risk. Cell (2016) 165(1):111–24. doi: 10.1016/j.cell.2016.02.011
12. Sun X, Jiao X, Ma Y, Liu Y, Zhang L, He Y, et al. Trimethylamine n-oxide induces inflammation and endothelial dysfunction in human umbilical vein endothelial cells via activating ROS-TXNIP-NLRP3 inflammasome. Biochem Biophys Res Commun (2016) 481(1-2):63–70. doi: 10.1016/j.bbrc.2016.11.017
13. Al-Obaide MAI, Singh R, Datta P, Rewers-Felkins KA, Salguero MV, Al-Obaidi I, et al. Gut microbiota-dependent trimethylamine-n-oxide and serum biomarkers in patients with T2DM and advanced CKD. J Clin Med (2017) 6(9):86. doi: 10.3390/jcm6090086
14. Gao X, Liu X, Xu J, Xue C, Xue Y, Wang Y. Dietary trimethylamine n-oxide exacerbates impaired glucose tolerance in mice fed a high fat diet. J biosci bioengineering. (2014) 118(4):476–81. doi: 10.1016/j.jbiosc.2014.03.001
15. Moher D, Liberati A, Tetzlaff J, Altman DG. Preferred reporting items for systematic reviews and meta-analyses: the PRISMA statement. PloS Med (2009) 6(7):e1000097. doi: 10.1371/journal.pmed.1000097
16. Stang A. Critical evaluation of the Newcastle-Ottawa scale for the assessment of the quality of nonrandomized studies in meta-analyses. Eur J Epidemiol (2010) 25(9):603–5. doi: 10.1007/s10654-010-9491-z
17. Higgins JP, Thompson SG, Deeks JJ, Altman DG. Measuring inconsistency in meta-analyses. BMJ (Clinical Res ed) (2003) 327(7414):557–60. doi: 10.1136/bmj.327.7414.557
18. Abdullah B, Daud S, Aazmi MS, Idorus MY, Mahamooth MIJ. Gut microbiota in pregnant Malaysian women: a comparison between trimesters, body mass index and gestational diabetes status. BMC pregnancy childbirth (2022) 22(1):152. doi: 10.1186/s12884-022-04472-x
19. Chen T, Zhang Y, Zhang Y, Shan C, Zhang Y, Fang K, et al. Relationships between gut microbiota, plasma glucose and gestational diabetes mellitus. J Diabetes Invest (2021) 12(4):641–50. doi: 10.1111/jdi.13373
20. Cui M, Qi C, Yang L, Zhang M, Wang H, She G, et al. A pregnancy complication-dependent change in SIgA-targeted microbiota during third trimester. Food Funct (2020) 11(2):1513–24. doi: 10.1039/C9FO02919B
21. Hu P, Chen X, Chu X, Fan M, Ye Y, Wang Y, et al. Association of gut microbiota during early pregnancy with risk of incident gestational diabetes mellitus. J Clin Endocrinol Metab (2021) 106(10):e4128–e41. doi: 10.1210/clinem/dgab346
22. Huang L, Sililas P, Thonusin C, Luewan S, Chattipakorn SC. Early gut dysbiosis could be an indicator of unsuccessful diet control in gestational diabetes mellitus. J diabetes (2021) 13(12):1054–8. doi: 10.1111/1753-0407.13225
23. Koren O, Goodrich JK, Cullender TC, Spor A, Laitinen K, Bäckhed HK, et al. Host remodeling of the gut microbiome and metabolic changes during pregnancy. Cell (2012) 150(3):470–80. doi: 10.1016/j.cell.2012.07.008
24. Li G, Yin P, Chu S, Gao W, Cui S, Guo S, et al. Correlation analysis between GDM and gut microbial composition in late pregnancy. J Diabetes Res (2021) 2021:8892849. doi: 10.1155/2021/8892849
25. Ma S, You Y, Huang L, Long S, Zhang J, Guo C, et al. Alterations in gut microbiota of gestational diabetes patients during the first trimester of pregnancy. Front Cell infect Microbiol (2020) 10:58. doi: 10.3389/fcimb.2020.00058
26. Mullins TP, Tomsett KI, Gallo LA, Callaway LK, McIntyre HD, Dekker Nitert M, et al. Maternal gut microbiota displays minor changes in overweight and obese women with GDM. Nutrition metabolism Cardiovasc Dis NMCD. (2021) 31(7):2131–9. doi: 10.1016/j.numecd.2021.03.029
27. Sililas P, Huang L, Thonusin C, Luewan S, Chattipakorn N, Chattipakorn S, et al. Association between gut microbiota and development of gestational diabetes mellitus. Microorganisms (2021) 9(8):1686. doi: 10.3390/microorganisms9081686
28. Tanaka K, Harata G, Miyazawa K, He F, Tanigaki S, Kobayashi Y. The gut microbiota of non-obese Japanese pregnant women with gestational diabetes mellitus. Biosci Microbiota Food Health (2022) 41(1):4–11. doi: 10.12938/bmfh.2021-025
29. Metzger BE, Gabbe SG, Persson B, Buchanan TA, Catalano PA, Damm P, et al. International association of diabetes and pregnancy study groups recommendations on the diagnosis and classification of hyperglycemia in pregnancy. Diabetes Care (2010) 33(3):676–82. doi: 10.2337/dc10-0719
30. Zheng W, Xu Q, Huang W, Yan Q, Chen Y, Zhang L, et al. Gestational diabetes mellitus is associated with reduced dynamics of gut microbiota during the first half of pregnancy. mSystems (2020) 5(2):e00109-20. doi: 10.1128/mSystems.00109-20
31. Wei J, Qing Y, Zhou H, Liu J, Qi C, Gao J. 16S rRNA gene amplicon sequencing of gut microbiota in gestational diabetes mellitus and their correlation with disease risk factors. J endocrinol Invest (2022) 45(2):279–89. doi: 10.1007/s40618-021-01595-4
32. Wu Y, Bible PW, Long S, Ming WK, Ding W, Long Y, et al. Metagenomic analysis reveals gestational diabetes mellitus-related microbial regulators of glucose tolerance. Acta diabetol (2020) 57(5):569–81. doi: 10.1007/s00592-019-01434-2
33. Gámez-Valdez JS, García-Mazcorro JF, Montoya-Rincón AH, Rodríguez-Reyes DL, Jiménez-Blanco G, Rodríguez MTA, et al. Differential analysis of the bacterial community in colostrum samples from women with gestational diabetes mellitus and obesity. Sci Rep (2021) 11(1):24373. doi: 10.1038/s41598-021-03779-7
34. Su Y, Chen L, Zhang DY, Gan XP, Cao YN, Cheng DC, et al. The characteristics of intestinal flora in overweight pregnant women and the correlation with gestational diabetes mellitus. Endocr connections (2021) 10(11):1366–76. doi: 10.1530/EC-21-0433
35. Ye G, Zhang L, Wang M, Chen Y, Gu S, Wang K, et al. The gut microbiota in women suffering from gestational diabetes mellitus with the failure of glycemic control by lifestyle modification. J Diabetes Res (2019) 2019:6081248. doi: 10.1155/2019/6081248
36. Su Y, Wang HK, Gan XP, Chen L, Cao YN, Cheng DC, et al. Alterations of gut microbiota in gestational diabetes patients during the second trimester of pregnancy in the shanghai han population. J Trans Med (2021) 19(1):366. doi: 10.1186/s12967-021-03040-9
37. Wang X, Liu H, Li Y, Huang S, Zhang L, Cao C, et al. Altered gut bacterial and metabolic signatures and their interaction in gestational diabetes mellitus. Gut Microbes (2020) 12(1):1–13. doi: 10.1080/19490976.2020.1840765
38. Le Chatelier E, Nielsen T, Qin J, Prifti E, Hildebrand F, Falony G, et al. Richness of human gut microbiome correlates with metabolic markers. Nature (2013) 500(7464):541–6. doi: 10.1038/nature12506
39. Ahn J, Sinha R, Pei Z, Dominianni C, Wu J, Shi J, et al. Human gut microbiome and risk for colorectal cancer. J Natl Cancer Instit (2013) 105(24):1907–11. doi: 10.1093/jnci/djt300
40. Aron-Wisnewsky J, Vigliotti C, Witjes J, Le P, Holleboom AG, Verheij J, et al. Gut microbiota and human NAFLD: disentangling microbial signatures from metabolic disorders. Nat Rev Gastroenterol hepatol (2020) 17(5):279–97. doi: 10.1038/s41575-020-0269-9
41. Lambeth SM, Carson T, Lowe J, Ramaraj T, Leff JW, Luo L, et al. Composition, diversity and abundance of gut microbiome in prediabetes and type 2 diabetes. J Diabetes Obes (2015) 2(3):1–7. doi: 10.15436/2376-0949.15.031
42. Wu X, Ma C, Han L, Nawaz M, Gao F, Zhang X, et al. Molecular characterisation of the faecal microbiota in patients with type II diabetes. Curr Microbiol (2010) 61(1):69–78. doi: 10.1007/s00284-010-9582-9
43. Pascale A, Marchesi N, Govoni S, Coppola A, Gazzaruso C. The role of gut microbiota in obesity, diabetes mellitus, and effect of metformin: new insights into old diseases. Curr Opin Pharmacol (2019) 49:1–5. doi: 10.1016/j.coph.2019.03.011
44. DiGiulio DB, Callahan BJ, McMurdie PJ, Costello EK, Lyell DJ, Robaczewska A, et al. Temporal and spatial variation of the human microbiota during pregnancy. Proc Natl Acad Sci United States America (2015) 112(35):11060–5. doi: 10.1073/pnas.1502875112
45. Louis P, Flint HJ. Formation of propionate and butyrate by the human colonic microbiota. Environ Microbiol (2017) 19(1):29–41. doi: 10.1111/1462-2920.13589
46. Ma C, Han M, Heinrich B, Fu Q, Zhang Q, Sandhu M, et al. Gut microbiome-mediated bile acid metabolism regulates liver cancer via NKT cells. Sci (New York NY) (2018) 360(6391):eaan5931. doi: 10.1126/science.aan5931
47. Fei N, Zhao L. An opportunistic pathogen isolated from the gut of an obese human causes obesity in germfree mice. ISME J (2013) 7(4):880–4. doi: 10.1038/ismej.2012.153
48. Li J, Zhao F, Wang Y, Chen J, Tao J, Tian G, et al. Gut microbiota dysbiosis contributes to the development of hypertension. Microbiome (2017) 5(1):14. doi: 10.1186/s40168-016-0222-x
49. Rajilić-Stojanović M, de Vos WM. The first 1000 cultured species of the human gastrointestinal microbiota. FEMS Microbiol Rev (2014) 38(5):996–1047. doi: 10.1111/1574-6976.12075
50. Rakoff-Nahoum S, Paglino J, Eslami-Varzaneh F, Edberg S, Medzhitov R. Recognition of commensal microflora by toll-like receptors is required for intestinal homeostasis. Cell (2004) 118(2):229–41. doi: 10.1016/j.cell.2004.07.002
51. den Besten G, van Eunen K, Groen AK, Venema K, Reijngoud DJ, Bakker BM. The role of short-chain fatty acids in the interplay between diet, gut microbiota, and host energy metabolism. J Lipid Res (2013) 54(9):2325–40. doi: 10.1194/jlr.R036012
52. Gao Z, Yin J, Zhang J, Ward RE, Martin RJ, Lefevre M, et al. Butyrate improves insulin sensitivity and increases energy expenditure in mice. Diabetes (2009) 58(7):1509–17. doi: 10.2337/db08-1637
53. Säemann MD, Böhmig GA, Osterreicher CH, Burtscher H, Parolini O, Diakos C, et al. Anti-inflammatory effects of sodium butyrate on human monocytes: potent inhibition of IL-12 and up-regulation of IL-10 production. FASEB J Off Publ Fed Am Societies Exp Biol (2000) 14(15):2380–2. doi: 10.1096/fj.00-0359fje
54. Soliman MM, Ahmed MM, Salah-Eldin AE, Abdel-Aal AA. Butyrate regulates leptin expression through different signaling pathways in adipocytes. J vet sci (2011) 12(4):319–23. doi: 10.4142/jvs.2011.12.4.319
55. Chambers ES, Viardot A, Psichas A, Morrison DJ, Murphy KG, Zac-Varghese SE, et al. Effects of targeted delivery of propionate to the human colon on appetite regulation, body weight maintenance and adiposity in overweight adults. Gut (2015) 64(11):1744–54. doi: 10.1136/gutjnl-2014-307913
56. Demigné C, Morand C, Levrat MA, Besson C, Moundras C, Rémésy C. Effect of propionate on fatty acid and cholesterol synthesis and on acetate metabolism in isolated rat hepatocytes. Br J Nutr (1995) 74(2):209–19. doi: 10.1079/BJN19950124
57. Liu X, Cheng YW, Shao L, Sun SH, Wu J, Song QH, et al. Gut microbiota dysbiosis in Chinese children with type 1 diabetes mellitus: An observational study. World J gastroenterol (2021) 27(19):2394–414. doi: 10.3748/wjg.v27.i19.2394
58. Ozato N, Saito S, Yamaguchi T, Katashima M, Tokuda I, Sawada K, et al. Blautia genus associated with visceral fat accumulation in adults 20-76 years of age. NPJ biofilms microbiomes (2019) 5(1):28. doi: 10.1038/s41522-019-0101-x
59. Osaki H, Jodai Y, Koyama K, Omori T, Horiguchi N, Kamano T, et al. Clinical response and changes in the fecal microbiota and metabolite levels after fecal microbiota transplantation in patients with inflammatory bowel disease and recurrent clostridioides difficile infection. Fujita Med J (2021) 7(3):87–98. doi: 10.20407/fmj.2020-021
60. Tong X, Xu J, Lian F, Yu X, Zhao Y, Xu L, et al. Structural alteration of gut microbiota during the amelioration of human type 2 diabetes with hyperlipidemia by metformin and a traditional Chinese herbal formula: a multicenter, randomized, open label clinical trial. mBio (2018) 9(3):e02392-17. doi: 10.1128/mBio.02392-17
61. Qi CJ, Zhang Q, Yu M, Xu JP, Zheng J, Wang T, et al. Imbalance of fecal microbiota at newly diagnosed type 1 diabetes in Chinese children. Chin Med J (2016) 129(11):1298–304. doi: 10.4103/0366-6999.182841
62. Borst SE. The role of TNF-alpha in insulin resistance. Endocrine (2004) 23(2-3):177–82. doi: 10.1385/ENDO:23:2-3:177
63. Tan J, McKenzie C, Potamitis M, Thorburn AN, Mackay CR, Macia L. The role of short-chain fatty acids in health and disease. Adv Immunol (2014) 121:91–119. doi: 10.1016/B978-0-12-800100-4.00003-9
64. Gomez-Arango LF, Barrett HL, Wilkinson SA, Callaway LK, McIntyre HD, Morrison M, et al. Low dietary fiber intake increases collinsella abundance in the gut microbiota of overweight and obese pregnant women. Gut Microbes (2018) 9(3):189–201. doi: 10.1080/19490976.2017.1406584
65. Rajilić-Stojanović M, Heilig HG, Tims S, Zoetendal EG, de Vos WM. Long-term monitoring of the human intestinal microbiota composition. Environ Microbiol (2012). doi: 10.1111/1462-2920.12023
66. Hasain Z, Mokhtar NM, Kamaruddin NA, Mohamed Ismail NA, Razalli NH, Gnanou JV, et al. Gut microbiota and gestational diabetes mellitus: A review of host-gut microbiota interactions and their therapeutic potential. Front Cell infect Microbiol (2020) 10:188. doi: 10.3389/fcimb.2020.00188
67. Mukherjee A, Lordan C, Ross RP, Cotter PD. Gut microbes from the phylogenetically diverse genus eubacterium and their various contributions to gut health. Gut Microbes (2020) 12(1):1802866. doi: 10.1080/19490976.2020.1802866
68. Han YH, Onufer EJ, Huang LH, Sprung RW, Davidson WS, Czepielewski RS, et al. Enterically derived high-density lipoprotein restrains liver injury through the portal vein. Sci (New York NY) (2021) 373(6553):eabe6729. doi: 10.1126/science.abe6729
69. Maa MC, Chang MY, Hsieh MY, Chen YJ, Yang CJ, Chen ZC, et al. Butyrate reduced lipopolysaccharide-mediated macrophage migration by suppression of src enhancement and focal adhesion kinase activity. J Nutr Biochem (2010) 21(12):1186–92. doi: 10.1016/j.jnutbio.2009.10.004
70. Ferrocino I, Ponzo V, Gambino R, Zarovska A, Leone F, Monzeglio C, et al. Changes in the gut microbiota composition during pregnancy in patients with gestational diabetes mellitus (GDM). Sci Rep (2018) 8(1):12216. doi: 10.1038/s41598-018-30735-9
71. Patterson E, Ryan PM, Cryan JF, Dinan TG, Ross RP, Fitzgerald GF, et al. Gut microbiota, obesity and diabetes. Postgraduate Med J (2016) 92(1087):286–300. doi: 10.1136/postgradmedj-2015-133285
72. Holscher HD, Bauer LL, Gourineni V, Pelkman CL, Fahey GC Jr., Swanson KS. Agave inulin supplementation affects the fecal microbiota of healthy adults participating in a randomized, double-blind, placebo-controlled, crossover trial. J Nutr (2015) 145(9):2025–32. doi: 10.3945/jn.115.217331
73. Wang W, Uzzau S, Goldblum SE, Fasano A. Human zonulin, a potential modulator of intestinal tight junctions. J Cell Sci (2000) 113 Pt 24:4435–40. doi: 10.1242/jcs.113.24.4435
74. Wiklund P, Zhang X, Pekkala S, Autio R, Kong L, Yang Y, et al. Insulin resistance is associated with altered amino acid metabolism and adipose tissue dysfunction in normoglycemic women. Sci Rep (2016) 6:24540. doi: 10.1038/srep24540
75. Tobias PS, Soldau K, Gegner JA, Mintz D, Ulevitch RJ. Lipopolysaccharide binding protein-mediated complexation of lipopolysaccharide with soluble CD14. J Biol Chem (1995) 270(18):10482–8. doi: 10.1074/jbc.270.18.10482
76. Lu YC, Yeh WC, Ohashi PS. LPS/TLR4 signal transduction pathway. Cytokine (2008) 42(2):145–51. doi: 10.1016/j.cyto.2008.01.006
77. Turnbaugh PJ, Hamady M, Yatsunenko T, Cantarel BL, Duncan A, Ley RE, et al. A core gut microbiome in obese and lean twins. Nature (2009) 457(7228):480–4. doi: 10.1038/nature07540
78. Zakaria NF, Hamid M, Khayat ME. Amino acid-induced impairment of insulin signaling and involvement of G-protein coupling receptor. Nutrients (2021) 13(7):2229. doi: 10.3390/nu13072229
79. Adeva MM, Calviño J, Souto G, Donapetry C. Insulin resistance and the metabolism of branched-chain amino acids in humans. Amino Acids (2012) 43(1):171–81. doi: 10.1007/s00726-011-1088-7
80. Haufe S, Engeli S, Kaminski J, Witt H, Rein D, Kamlage B, et al. Branched-chain amino acid catabolism rather than amino acids plasma concentrations is associated with diet-induced changes in insulin resistance in overweight to obese individuals. Nutrition metabolism Cardiovasc Dis NMCD. (2017) 27(10):858–64. doi: 10.1016/j.numecd.2017.07.001
81. Gerber PA, Rutter GA. The role of oxidative stress and hypoxia in pancreatic beta-cell dysfunction in diabetes mellitus. Antioxidants Redox Signaling (2017) 26(10):501–18. doi: 10.1089/ars.2016.6755
82. Schriever SC, Deutsch MJ, Adamski J, Roscher AA, Ensenauer R. Cellular signaling of amino acids towards mTORC1 activation in impaired human leucine catabolism. J Nutr Biochem (2013) 24(5):824–31. doi: 10.1016/j.jnutbio.2012.04.018
83. Mallmann NH, Lima ES, Lalwani P. Dysregulation of tryptophan catabolism in metabolic syndrome. Metab syndrome related Disord (2018) 16(3):135–42. doi: 10.1089/met.2017.0097
84. Natividad JM, Agus A, Planchais J, Lamas B, Jarry AC, Martin R, et al. Impaired aryl hydrocarbon receptor ligand production by the gut microbiota is a key factor in metabolic syndrome. Cell Metab (2018) 28(5):737–49.e4. doi: 10.1016/j.cmet.2018.07.001
85. Su X, Gao Y, Yang R. Gut microbiota-derived tryptophan metabolites maintain gut and systemic homeostasis. Cells (2022) 11(15):2296. doi: 10.3390/cells11152296
86. Agus A, Planchais J, Sokol H. Gut microbiota regulation of tryptophan metabolism in health and disease. Cell Host Microbe (2018) 23(6):716–24. doi: 10.1016/j.chom.2018.05.003
87. Gao H, Liu S. Role of uremic toxin indoxyl sulfate in the progression of cardiovascular disease. Life Sci (2017) 185:23–9. doi: 10.1016/j.lfs.2017.07.027
88. Chimerel C, Emery E, Summers DK, Keyser U, Gribble FM, Reimann F. Bacterial metabolite indole modulates incretin secretion from intestinal enteroendocrine l cells. Cell Rep (2014) 9(4):1202–8. doi: 10.1016/j.celrep.2014.10.032
Keywords: gestational diabetes mellitus, gut microbiota, meta-analysis, systematic review, insulin resistance
Citation: Yan M, Guo X, Ji G, Huang R, Huang D, Li Z, Zhang D, Chen S, Cao R, Yang X and Wu W (2023) Mechanismbased role of the intestinal microbiota in gestational diabetes mellitus: A systematic review and meta-analysis. Front. Immunol. 13:1097853. doi: 10.3389/fimmu.2022.1097853
Received: 14 November 2022; Accepted: 15 December 2022;
Published: 03 March 2023.
Edited by:
Barkat A. Khan, Gomal University, PakistanCopyright © 2023 Yan, Guo, Ji, Huang, Huang, Li, Zhang, Chen, Cao, Yang and Wu. This is an open-access article distributed under the terms of the Creative Commons Attribution License (CC BY). The use, distribution or reproduction in other forums is permitted, provided the original author(s) and the copyright owner(s) are credited and that the original publication in this journal is cited, in accordance with accepted academic practice. No use, distribution or reproduction is permitted which does not comply with these terms.
*Correspondence: Xingfen Yang, xfyang@vip.163.com; Wei Wu, cnnjwuwei@163.com
†These authors have contributed equally to this work and share first authorship