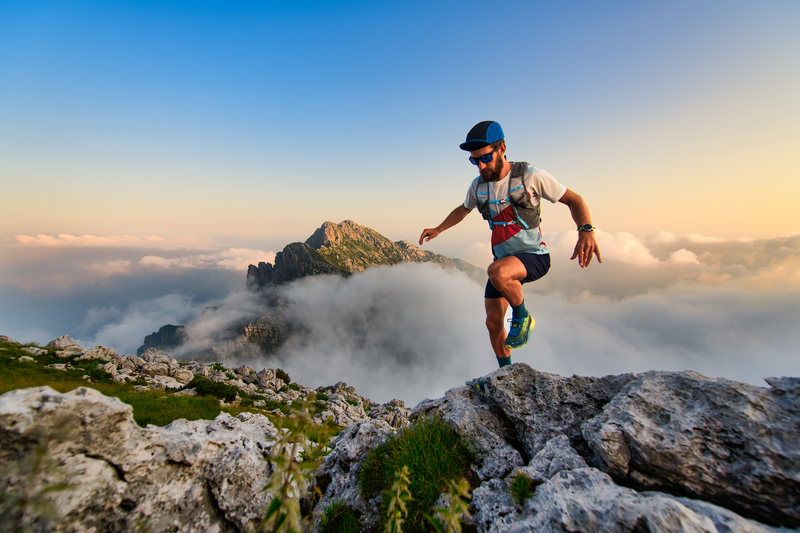
95% of researchers rate our articles as excellent or good
Learn more about the work of our research integrity team to safeguard the quality of each article we publish.
Find out more
ORIGINAL RESEARCH article
Front. Immunol. , 22 December 2022
Sec. Cancer Immunity and Immunotherapy
Volume 13 - 2022 | https://doi.org/10.3389/fimmu.2022.1083840
This article is part of the Research Topic Methods in Cancer Immunity and Immunotherapy: 2022 View all 17 articles
Background: Previous studies indicated the evidence that baseline levels of thyroid antibodies, thyroid status, and serum lactate dehydrogenase (LDH) and M stage may influence the prognosis of patients with advanced or metastatic melanoma treated with immune checkpoint inhibitors that targets programmed cell death-1 (PD-1) or programmed death ligand 1, which reported that dramatic improvements in survival rates were observed; however, the presence of controversy has prevented consensus from being reached. Study objectives were to develop a nomogram to identify several prognostic factors in Chinese patients with metastatic melanoma receiving immunotherapy.
Methods: This retrospective study included 231 patients from Sun Yat-sen University Cancer Center, and patients were split into internal cohort (n = 165) and external validation cohort (n = 66). We developed a nomogram for the prediction of response and prognosis on the basis of the levels of serum thyroid peroxidase antibody (A-TPO), free T3 (FT3), and LDH and M stage that were measured at the baseline of anti–PD-1 infusion. In addition, the follow-up lasted at least until 5 years after the treatment or mortality. RECIST v1.1 was used to classify treatment responses.
Results: Chi-square test showed that PD-1 antibody was more effective in patients with melanoma with high level baseline FT4 or earlier M stage. A multivariate Cox analysis showed that baseline FT3 (P = 0.009), baseline A-TPO (P = 0.016), and LDH (P = 0.013) levels and M stage (P < 0.001) independently predicted overall survival (OS) in patients with melanoma. The above factors are integrated, and a prediction model is established, i.e., nomogram. Survival probability area-under-the-curve values of 1, 2, and 3 years in the training, internal validation, and external validation cohorts showed the prognostic accuracy and clinical applicability of nomogram (training: 0.714, 0.757, and 0.764; internal validation: 0.7171963, 0.756549, and 0.7651486; external validation: 0.748, 0.710, and 0.856). In addition, the OS of low-risk (total score ≤ 142.65) versus high-risk (total score > 142.65) patients varied significantly in both training group (P < 0.0001) and external validation cohort (P = 0.0012).
Conclusions: According to this study, baseline biomarkers are associated with response to immunotherapy and prognosis among patients with metastatic melanoma. Treatment regimens can be tailor-made on the basis of these biomarkers.
As oncology advances, immunotherapy targeting immune checkpoints are gaining popularity, such as cytotoxic T lymphocyte antigen 4 (CTLA-4) and programmed cell death-1 (PD-1). Because the immune checkpoint inhibitors (ICIs) have emerged, they have rapidly been integrated into many cancer treatment regimens in the past decade. As the leading cause of skin cancer–related mortality, melanoma has the capacity to develop distant metastases (1). Despite the absence of curative options for advanced melanoma, the advent of immunotherapy dramatically improves the prognoses (2). In spite of their impressive effects on malignancies, there were still a majority of patients who were not able to benefit from the anti–PD-1/programmed death ligand 1 (PD-L1) monotherapy, and these treatments also induce a variety of immune-related adverse events (irAEs), some of which can be fatal (3–7). According to randomized clinical trials, the occurrence of endocrine irAEs during anti–PD-1 monotherapy ranged from 3.8% to 20.8%, whereas anti–PD-1 + anti–CTLA-4 combination treatment regimen has been associated with 14.4% to 34% (8). During anti–PD-1 immunotherapy, thyroid toxicity accounts for 5% to 15% of all irAEs (9, 10). Most commonly, symptoms of thyroid toxicity include transient thyrotoxicosis and hypothyroidism, similar to classical thyroiditis, although its precise cause remains unknown (11, 12). Previously, thyroid toxicity during immunotherapy may be linked with improved overall survival (OS); however, with small sample sizes, these studies may be limited, including patients with renal cell carcinoma and non–small cell lung cancer (NSCLC), and progression-free survival (PFS) showed inconsistent effects (13–15). Lactate dehydrogenase (LDH) is a vital physiological enzyme involved in enhanced aerobic glycolysis, catalyzing pyruvate’s reversible transformation to lactate. In addition, lactate accumulation reduced CD8+ T cell and natural killer cell survival and cytolytic capacity, promoting tumor immune escape (16). Numerous studies suggested that patients with cancer with a high level of LDH may lead to poor prognosis in multiple cancer types due to the antagonism of anti–PD-1/PD-L1 antibodies, and LDH blockade improves the effectiveness of anti–PD-1 therapy (17–19). There is an ongoing debate regarding whether LDH can be used to assess the prognosis of patients with melanoma treated with anti–PD-1/PD-L1 antibodies, because some evidence suggest that OS/PFS and pretreatment LDH have no significant correlation (20–22).
Currently, oncologic outcome nomograms can be used to evaluate risk by considering important clinical and pathological factors (23, 24). The creation of predictive nomograms may help both patients and physicians in making better management decisions. In addition to this, several types of cancer have shown that nomograms are more accurate than traditional TNM classifications (25, 26). However, nomograms based on Chinese population cohort to predict prognosis and guide immunotherapy in metastatic melanoma are rare.
Therefore, we hypothesized that the baseline levels of thyroid antibodies, thyroid status, and serum LDH and M stage before immunotherapy might predict treatment outcomes in patients with metastatic melanoma. In present study, on the basis of a large prospective cohort of Chinese patients with metastatic melanoma, we explored the correlation between several baseline factors and prognosis. Furthermore, we constructed a risk score model and developed a nomogram in conjunction with clinical characteristics. Identifying effective biomarkers to predict response and prognosis after immunotherapy may benefit in guiding treatment regimen.
Study approval was granted by the Ethics Committee of Sun Yat-sen University Cancer Center. We retrospectively collected 165 patients diagnosed with metastatic melanoma receiving anti–PD-1 treatment between March 2015 and December 2019 at Sun Yat-sen University Cancer Center. The inclusion criteria are as follows: (a) patients diagnosed with advanced melanoma, (b) patients who underwent anti–PD-1 treatment, (c) measurability of tumor lesions/lymph nodes, and (d) availability of clinical data. The exclusion criteria are as follows: (a) preoperative therapy (neoadjuvant radiotherapy or chemotherapy), (b) patients with other types of malignancies, (c) missing essential histopathological results, (d) missing essential imaging data to evaluate response, and (e) incomplete information.
We enrolled another 66 patients with metastatic melanoma who underwent anti–PD-1 antibody treatment between March 2017 and December 2021 as an external validation cohort that is also from the Sun Yat-sen University Cancer Center.
Serum was collected at baseline to determine free T3 (FT3; reference range: 2.80–7.10 pmol/L), free T4 (FT4; reference range: 12.00–22.00 pmol/L), thyroid-stimulating hormone (TSH; reference range: 0.27–4.20 uIU/ml), thyroglobulin (TG; reference range: 3.5–77.00 ng/ml), thyroid peroxidase antibody (A-TPO; reference range: 0–35.00 U/ml), and LDH (reference range: 120.00–250.00 U/L) levels.
After anti–PD-1 treatment, we maintain a regular contact with patients through outpatient reexaminations, telephone calls, and hospital medical records at least until 5 years after the treatment or mortality. Patients with incomplete follow-up were censored. In this study, a follow-up is due by 28 February 2022. On the basis of the standard Response Evaluation Criteria In Solid Tumors (RECIST) version 1.1 (Supplementary Table 1) (27), OS is defined as the period between the start of therapy and death, whereas PFS is calculated until the tumor progresses or death. In melanoma, non-PD, composed of complete response (CR), partial response (PR), and simple disease (SD), which we always equate as “disease control rate”, predicts survival better than response alone.
All data analysis was performed using SPSS 25.0 software (IBM, USA), GraphPad Prism 9 (GraphPad Software, Inc., USA), and R software 4.1.0 (https://www.r-project.org/). It is worth noting that we use the median as the cutoff value of thyroid function grouping. Chi-square test was used to analyze the correlation between clinical baseline characteristics and short-term efficacy of the PD-1 antibody. Survival analysis was performed by the Kaplan-Meier (K-M) method and tested by the log rank test. Univariate and multivariate analyses were performed by Cox regression model to evaluate the correlations between prognostic factors and OS, and the hazard ratios (HRs) and corresponding 95% confidence intervals (CIs) were shown. All statistical tests were two-sided, and P < 0.05 was supposed to be statistically significant.
Univariate and multivariate Cox professional hazard models were used to determine the potential important prognostic factors of the entire cohort. Multivariate Cox regression analysis includes variables with P < 0.05 in univariate analysis. If significant effects were observed in Cox model (P < 0.05), then they were determined as prognostic factors independently. Finally, serum A-TPO, FT3, and LDH levels and M stage were confirmed as the independent prognostic factors. In addition, on the basis of Cox professional hazards models, nomogram models were constructed in R using the “rms” package and were shown by the “ggplot2” package.
The nomogram was internally and externally validated, and the model discrimination and calibration were evaluated. The discrimination accuracy of the nomogram was estimated using the area under the curve (AUC). The AUC values range from 0.5 to 1. In case the AUC value is equal to 0.5, the nomogram has no ability to discriminate. On the contrary, if the AUC value is 1, then the model’s ability of stratifying patients into different prognosis groups is perfect. The calibration was evaluated through the calibration curve, which is a chart showing the correlation between the prediction probability and the observed result frequency. Standard curves are straight lines with slope 1 that pass through the origin of the coordinate axis. Nomograms are more accurate if the calibration curve is closer to the standard curve.
On the basis of the nomogram, we calculated each patient’s sum score by the “nomogramFormula” R package. Subsequently, the “surv_cutpoint” function in the “survminer” R package generated the cutoff points for stratifying the risk into low and high, and then, we carried out the survival analysis of the subgroup by the “survival” R package.
Baseline characteristics of patients (n = 165) are shown in Table 1. In total, 165 patients with diagnosed melanoma were included in the study. In addition, 80 female patients (48.5%) and 85 male patients (51.5%) were included in the sample. One-hundred eleven of the 165 patients (67.2%) were younger than 60 years, and 54 (32.8%) were older than 60 years. According to the baseline LDH level, patients were stratified into two groups: 103 patients (62.4%) had normal LDH (LDH = 0), and 62 patients (37.6%) had increased LDH (LDH = 1). In addition, there are 42 (25.5%) patients carried a V-raf murine sarcoma viral oncogene homolog B1 (BRAF) mutation, and 123 (74.5%) patients were wild type. In patients with distant metastases (M1A, n = 42; M1B, n = 30; M1C, n = 72; M1D, n = 21), we delineate the M1 stage into two M categories: The first is defined as [M1A + M1B] and the second is defined as [M1C + M1D]. According to the indices of the baseline thyroid functions, each subgroup was divided into the low (below the median value) and high (above or equal to the median value) groups (cutoff values of each subgroup: baseline FT3, 4.54 pmol/L; baseline FT4, 16.18 pmol/L; baseline TSH, 1.66 uIU/ml; baseline A-TPO: 13.09 U/ml; baseline TG, 9.19 ng/ml).
According to whether disease had progressed, patients were stratified into two groups: progression (PD) and non-progression (CR + PR + SD) groups. Clear correlation between baseline FT4 (P = 0.001) or M stage (P = 0.015) and short-term tumor response was evident, suggesting that blocking PD-1 with therapeutic antibodies would be useful if patients had a high-level baseline FT4 or earlier M stage. In addition, there was no obvious correlation between a short-term efficacy of PD-1 antibodies and age, sex, LDH levels, BRAF mutation, baseline FT3, baseline TSH, baseline A-TPO, or baseline TG (Table 2).
Table 2 The association of efficacy of PD-1 blockade therapy with several baseline predictor variables.
On the basis of the univariate analysis, baseline FT3 (HR, 0.519; CI, 0.339–0.796; P = 0.002), baseline FT4 (HR, 0.625; CI, 0.410–0.950; P = 0.026), baseline A-TPO (HR, 0.601; CI, 0.394–0.917; P = 0.017), and LDH (HR, 2.220; CI, 1.461–3.374; P = 0.0001) levels and M stage (HR, 2.170; CI, 1.395–3.375; P = 0.0004) were significantly associated with OS (Figure 1, Table 3). In contrast, age, sex, baseline TSH, baseline TG, and BRAF mutation did not influence OS (P > 0.05). A multivariate Cox analysis incorporating meaningful univariate analysis variables showed that baseline FT3 (HR, 0.554; 95% CI, 0.355–0.865; P = 0.009), baseline A-TPO (HR, 0.567; 95% CI, 0.381–0.904; P = 0.016), and LDH (HR, 1.738; 95% CI, 1.121–2.693; P = 0.013) levels and M stage (HR, 2.156; 95% CI, 1.361–3.414; P < 0.001) independently predicted OS in patients with melanoma. In addition, it was confirmed in this analysis that baseline FT4 does not have an independent prognostic value for melanoma. Tables 3, 4 show results for univariate and multivariate Cox regression models.
Figure 1 Kaplan–Meier estimates of overall survival (OS) of patients with metastatic melanoma in different groups. (A) Baseline FT3:OS curve of patients in the high group (n = 82) versus the low group (n = 83). (B) baseline A-TPO : OS curve of patients in the high group (n = 83) versus the low group (n = 82). (C) LDH level:OS curve of patients in the normal group (n = 103) versus the rise group (n = 62). (D) M stage:OS curve of patients in group 1 (n = 72) versus group 2 (n = 93).
The prediction model is presented in the form of nomogram (Figure 2). The variables included in the model were baseline FT3 (1 = low, 2 = high), baseline A-TPO (1 = low, 2 = high), M stage (1 = M1A or M1B, 2 = M1C or M1D), and LDH (normal = 0, elevated = 1) levels. A score is assigned to each independent predictor by writing a line pointing directly to the axis of score. The total score is obtained by adding up the scores of all related factors, and the corresponding predicted survival probability can be obtained by making a vertical line from the total score axis to intersect the survival probability axis of 1, 2, and 3 years. For example, a patient with stage M1C melanoma (100 points) with normal LDH level (0 points), whose baseline FT3 is 2.25 pmol/L (78 points) and baseline A-TPO is 17.24 U/ml (0 points), has a sum score equal to 178, which corresponds to the foreseen survival probability of 1, 2, and 3 years of 69%, 44%, and 33%, respectively (Supplementary Table 2).
Figure 2 Prognostic Nomograms of 1-, 2-, and 3-year OS for patients with metastatic melanoma. For every patient in the nomogram, four lines are drawn upward to calculate the points received from the four predictors. The sum of the points is located on the “Total Points” axis. In addition, the possibility of 1-, 2-, and 3-year OS is determined by drawing a downward line.
First, the calibration curve shows that, in the training cohort, the actual observations are in excellent agreement with the prediction results of the nomogram (Figure 3). Second, we carried out internal verification. The internal verification is carried out by randomly segmenting the data set at 7:3 and repeating it 500 times, calculating the AUC value, and counting its mean value, respectively. The 1-year survival probability AUC mean is 0.7171963, the 2-year survival probability mean value is 0.756549, and the 3-year survival probability mean value is 0.7651486 (Figure 4), which showed a good discrimination ability in the training cohort made up of 165 Chinese patients with metastatic melanoma. Last, prognostic accuracy and clinical applicability of nomogram were evaluated using ROC curves and AUC values of 1-, 2-, and 3-year survival probability in the training cohort and the external verification cohort. The AUC values in the ROC curve analysis showed a good accuracy (the AUC values of 1-, 2-, and 3-year survival probability in the training cohort were 0.714, 0.757, and 0.764 and in the external verification cohort were 0.748, 0.710, and 0.856, respectively) (Figure 5).
Figure 3 Calibration curves predicting the (A) 1-, (B) 2-, and (C) 3-year OS of patients in the training cohort. On the x-axis are the predicted survival probabilities, and on the y-axis are the actual survival probabilities. There is an agreement between the prediction and reality based on the 45° line (gray line).
Figure 5 The prognostic accuracy of the nomogram was estimated by using ROC curves and AUCs at 1, 2, and 3 years in the training cohort (A) and the external validation cohort (B).
On the basis of the nomogram, 142.65 was the cutoff value for the sum score (Supplementary Figure S1). We divided all patients into two risk groups based on the cutoff value (142.65): high-risk group (>142.65) and low-risk group (≤142.65). There was a remarkable difference in OS between individuals who were at low risk and those who were at high risk (P < 0.0001). When the unchanged cutoff value was applied to the external validation cohort, it was also possible to discriminate between high- and low-risk OS in Kaplan-Meier (K-M) curves. Observations show that the OS of low-risk group is overwhelmingly superior to that of the high-risk group OS (P = 0.0012) (Figure 6).
Figure 6 The Kaplan–Meier curve shows OS with risk stratification in the training cohort (A) and in the external validation cohort (B).
Melanoma is one of the most aggressive forms of skin cancer, accounting for 5% of all cases, but 80% of mortality is related to it (28). Therefore, immunotherapy is administered to patients with metastatic melanoma as an adjuvant treatment (29). Nevertheless, not all patients benefit from immunotherapy, so new methods that predict treatment response are needed. Several studies have shown that thyroid antibodies, thyroid status, serum LDH, and M stage are all predictors of immunotherapy response, but they each have their limitations (15, 30). Thus, further research into the association may be of value in developing a combined biomarker model that can better predict the response in advanced melanoma.
Our study explored the relationship between various baseline clinicopathological factors before treatment and the short-term efficacy of PD-1 antibody. Chi-square test showed that PD-1 antibody was more effective in patients with melanoma with higher level baseline FT4 or earlier M stage. Furthermore, in Table 2, we can see that lower baseline FT3 is associated with a worse efficacy [more patients with progressive disease (PD)], which is consistent with the results of Cox that lower baseline FT3 levels are correlated with worse prognosis (shorter OS in the low-level baseline FT3 group). The reason why the statistical value is meaningless (P > 0.05) should be that the sample size is not big enough.
It has been proven that the comprehensive analysis of baseline clinicopathological factors by nomogram can accurately predict the clinical results of metastatic melanoma checkpoint inhibitor immunotherapy (31). However, it is still an unsolved challenge to use clinical indicators to stratify the prognosis of metastatic melanoma treated with PD-1 antibody and predict its oncological outcome in the Chinese population. The research described here is the first to develop a clinically useful nomogram for accurately predicting the outcomes of checkpoint inhibitor immunotherapy in metastatic melanoma in Chinese patients, based on a comprehensive analysis of a variety of baseline clinicopathological variables. In this study, the patient cohort was originated from the Sun Yat-sen University Cancer Center (Guangzhou, China), one of the largest cancer hospitals in China. It appears that the sample enrolled in this cohort is both generalizable and representative of Chinese patients with melanoma. Our study explored the relationship between the baseline pretreatment clinicopathologic factors and the prognosis of PD-1 antibody therapy. It may assist clinicians to identify patients with poor prognosis in PD-1 antibody therapy, who may be in need of novel clinical trials options at the outset (i.e., first line).
There have been several clinical (e.g., presence/absence of liver and brain metastases) (30, 32) and hematologic factors (e.g., LDH) (33) that have been described to be linked to ICI response or resistance (34). In the majority of the cases, these factors, however, have not been included in multivariable models. In this study, we verified that baseline FT3, baseline A-TPO, LDH, and M stage (presence/absence of liver and brain metastases) were the independent prognostic factors of OS in patients with metastatic melanoma treated with PD-1 antibody, through the univariate and multivariate analyses. LDH, a factor of the melanoma American Joint Committee on Cancer (AJCC) staging, is a prognostic factor for other types of cancer. In addition, LDH has also proved to be a predictive marker, and a higher level of LDH is related with shorter PFS and OS in immunotherapy (35, 36). In addition, studies have shown that baseline FT3 levels are negatively correlated with cancer mortality (37, 38). Patients with baseline A-TPO level above the median had a higher OS (15), and the presence of liver or brain metastases is associated with shorter OS (31). The results of this study support these conclusions.
A nomogram was developed on the basis of the risk score model and clinical features to predict the survival possibility in metastatic melanoma. The nomogram was established according to four independent risk factors, and the patients were divided into high-risk group and low-risk group by the ROC curve analysis. It is encouraging to note that low-risk patients had a significantly better OS than high-risk patients. There is an excellent agreement between the actual observations and the predictions of the nomogram, as shown by the calibration curve. In China, we lack indicators for prognostic assessment and risk subgroup stratification of patients with metastatic melanoma treated with PD-1 antibody. Therefore, the line chart can help Chinese doctors in using this Chinese doctors’ scoring system to predict the individual survival of patients with metastatic melanoma. Aside from that, clinical studies can benefit from the scoring system because the system can provide stratified information for patients to decrease sample selection deviations.
It is important to note, however, that the current study still has some limitations that must be considered. Statistically valid risk score models and nomograms must be validated in larger clinical cohorts to determine the impact of thyroid antibodies, thyroid status, serum LDH, and M stage on response of immunotherapy and prognosis. In addition, it is necessary to validate our nomogram with a different experimental cohort. However, because of the limitation of data acquisition, we cannot do this for the time being. Moreover, more research studies are required to dynamically detect the level of serum thyroid hormone thyroid antibodies and LDH during the process of anti–PD-1 treatment and to verify the relationship between these factors and prognosis. Overt thyroid toxicity during treatment significantly prolonged OS and PFS in patients with metastatic melanoma, NSCLC, and renal cell carcinoma (RCC) receiving anti–PD-1. Previous studies in recent years assumed that these patients with higher levels of autoimmunity may benefit from anti-cancer treatment via autoimmune pathways because they are more susceptible to autoimmunity, which is supported by numerous studies indicating a positive correlation between irAEs and improved response and survival rates in patients treated with anti–PD-1 (39–44). There were, however, a number of limitations to most of these studies, including their retrospective design and/or small sample size; while comparing with published associations with other irAEs, we found that thyroid toxicity had much larger effect sizes on OS in our current retrospective study (39–44). In addition, the number of samples in this study is limited. Hence, to validate the model, additional external cohorts will be required. As a result, risk factors play an essential role in predicting clinical response and prognosis in patients with metastatic melanoma after anti–PD-1 treatment and are in need of further validation and updating in the future.
In conclusion, this study construct a nomogram based on the baseline levels of thyroid antibodies, thyroid status, serum LDH, and M stage before anti–PD-1, showing that the factors are strong predictive markers for response and prognosis to anti–PD-1 treatment in metastatic melanoma. This parameter may serve as a novel effective marker to predict response and prognosis, therefore assisting in treatment regimen selection.
The raw data supporting the conclusions of this article will be made available by the authors, without undue reservation.
The studies involving human participants were reviewed and approved by Ethics Committee of Sun Yat-sen University Cancer Center. The patients/participants provided their written informed consent to participate in this study. Written informed consent was obtained from the individual(s) for the publication of any potentially identifiable images or data included in this article.
All authors participated in the present study. YD, XZ, and YT contributed to the conception and design. JZ, DL, SX, XW, JL, XY, and ML conducted the collection of data. JZ, DL, and ZW analyzed and interpreted the data. SX and XD visualized the result. JZ and SX drafted the manuscript. All authors read and approved the manuscript.
This work was primarily supported by a grant from the National Natural Science Foundation of China (No. 81402560), the Guangdong Natural Science Foundation (Nos. 2021A1515010443, 2019A1515011263, and 2018A0303130344), the Guangdong Province Science and Technology Plan Project (No. 2017A020215029), and Guangdong Esophageal Cancer Institute Science and Technology Program (No. Q201802).
The authors thank the National Natural Science Foundation of China (No. 81402560), the Guangdong Province Science and Technology Plan Project (No. 2017A020215029), the Guangdong Esophageal Cancer Institute Science and Technology Program (No. Q201802), and the Guangdong Natural Science Foundation (Nos. 2021A1515010443 and 2018A0303130344).
The authors declare that the research was conducted in the absence of any commercial or financial relationships that could be construed as a potential conflict of interest.
All claims expressed in this article are solely those of the authors and do not necessarily represent those of their affiliated organizations, or those of the publisher, the editors and the reviewers. Any product that may be evaluated in this article, or claim that may be made by its manufacturer, is not guaranteed or endorsed by the publisher.
The Supplementary Material for this article can be found online at: https://www.frontiersin.org/articles/10.3389/fimmu.2022.1083840/full#supplementary-material
1. Schadendorf D, van Akkooi ACJ, Berking C, Griewank KG, Gutzmer R, Hauschild A, et al. Melanoma. Lancet (2018) 392(10151):971–84. doi: 10.1016/S0140-6736(18)31559-9
2. Luke JJ, Flaherty KT, Ribas A, Long GV. Targeted agents and immunotherapies: optimizing outcomes in melanoma. Nat Rev Clin Oncol (2017) 14(8):463–82. doi: 10.1038/nrclinonc.2017.43
3. Keilholz U, Mehnert JM, Bauer S, Bourgeois H, Patel MR, Gravenor D, et al. Avelumab in patients with previously treated metastatic melanoma: phase 1b results from the JAVELIN solid tumor trial. J Immunother Cancer (2019) 7(1):12. doi: 10.1186/s40425-018-0459-y
4. Champiat S, Lambotte O, Barreau E, Belkhir R, Berdelou A, Carbonnel F, et al. Management of immune checkpoint blockade dysimmune toxicities: a collaborative position paper. Ann Oncol (2016) 27(4):559–74. doi: 10.1093/annonc/mdv623
5. Postow MA, Sidlow R, Hellmann MD. Immune-related adverse events associated with immune checkpoint blockade. N Engl J Med (2018) 378(2):158–68. doi: 10.1056/NEJMra1703481
6. Johnson DB, Chandra S, Sosman JA. Immune checkpoint inhibitor toxicity in 2018. JAMA (2018) 320(16):1702–3. doi: 10.1001/jama.2018.13995
7. Zhao JJ, Wen XZ, Ding Y, Li DD, Zhu BY, Li JJ, et al. Association between immune-related adverse events and efficacy of PD-1 inhibitors in Chinese patients with advanced melanoma. Aging (Albany NY) (2020) 12(11):10663–75. doi: 10.18632/aging.103285
8. Su Q, Zhang XC, Wang DY, Zhang HR, Zhu C, Hou YL, et al. The risk of immune-related endocrine disorders associated with anti-PD-1 inhibitors therapy for solid tumors: A systematic review and meta-analysis. Int Immunopharmacol (2018) 59:328–38. doi: 10.1016/j.intimp.2018.04.021
9. Wright JJ, Powers AC, Johnson DB. Endocrine toxicities of immune checkpoint inhibitors. Nat Rev Endocrinol (2021) 17(7):389–99. doi: 10.1038/s41574-021-00484-3
10. Chang LS, Barroso-Sousa R, Tolaney SM, Hodi FS, Kaiser UB, Min L. Endocrine toxicity of cancer immunotherapy targeting immune checkpoints. Endocr Rev (2019) 40(1):17–65. doi: 10.1210/er.2018-00006
11. Chalan P, Di Dalmazi G, Pani F, De Remigis A, Corsello A, Caturegli P. Thyroid dysfunctions secondary to cancer immunotherapy. J Endocrinol Invest (2018) 41(6):625–38. doi: 10.1007/s40618-017-0778-8
12. Byun DJ, Wolchok JD, Rosenberg LM, Girotra M. Cancer immunotherapy - immune checkpoint blockade and associated endocrinopathies. Nat Rev Endocrinol (2017) 13(4):195–207. doi: 10.1038/nrendo.2016.205
13. Osorio JC, Ni A, Chaft JE, Pollina R, Kasler MK, Stephens D, et al. Antibody-mediated thyroid dysfunction during T-cell checkpoint blockade in patients with non-small-cell lung cancer. Ann Oncol (2017) 28(3):583–9. doi: 10.1093/annonc/mdw640
14. Kim HI, Kim M, Lee SH, Park SY, Kim YN, Kim H, et al. Development of thyroid dysfunction is associated with clinical response to PD-1 blockade treatment in patients with advanced non-small cell lung cancer. Oncoimmunology (2017) 7(1):e1375642. doi: 10.1080/2162402X.2017.1375642
15. Basak EA, van der Meer JWM, Hurkmans DP, Schreurs MWJ, Oomen-de Hoop E, van der Veldt AAM, et al. Overt thyroid dysfunction and anti-thyroid antibodies predict response to anti-PD-1 immunotherapy in cancer patients. Thyroid (2020) 30(7):966–73. doi: 10.1089/thy.2019.0726
16. Brand A, Singer K, Koehl GE, Kolitzus M, Schoenhammer G, Thiel A, et al. LDHA-associated lactic acid production blunts tumor immunosurveillance by T and NK cells. Cell Metab (2016) 24(5):657–71. doi: 10.1016/j.cmet.2016.08.011
17. Blank C, Mackensen A. Contribution of the PD-L1/PD-1 pathway to T-cell exhaustion: an update on implications for chronic infections and tumor evasion. Cancer Immunol Immunother (2007) 56(5):739–45. doi: 10.1007/s00262-006-0272-1
18. Mezquita L, Auclin E, Ferrara R, Charrier M, Remon J, Planchard D, et al. Association of the lung immune prognostic index with immune checkpoint inhibitor outcomes in patients with advanced non-small cell lung cancer. JAMA Oncol (2018) 4(3):351–7. doi: 10.1001/jamaoncol.2017.4771
19. Daneshmandi S, Wegiel B, Seth P. Blockade of lactate dehydrogenase-a (LDH-a) improves efficacy of anti-programmed cell death-1 (PD-1) therapy in melanoma. Cancers (Basel) (2019) 11(4):450. doi: 10.3390/cancers11040450
20. Gide TN, Silva IP, Quek C, Ahmed T, Menzies AM, Carlino MS, et al. Close proximity of immune and tumor cells underlies response to anti-PD-1 based therapies in metastatic melanoma patients. Oncoimmunology (2019) 9(1):1659093. doi: 10.1080/2162402X.2019.1659093
21. Namikawa K, Takahashi A, Mori T, Tsutsumida A, Suzuki S, Motoi N, et al. Nivolumab for patients with metastatic uveal melanoma previously untreated with ipilimumab: a single-institution retrospective study. Melanoma Res (2020) 30(1):76–84. doi: 10.1097/CMR.0000000000000617
22. Zou Y, Xie J, Zheng S, Liu W, Tang Y, Tian W, et al. Leveraging diverse cell-death patterns to predict the prognosis and drug sensitivity of triple-negative breast cancer patients after surgery. Int J Surg (2022) 107:106936. doi: 10.1016/j.ijsu.2022.106936
23. Valentini V, van Stiphout RG, Lammering G, Gambacorta MA, Barba MC, Bebenek M, et al. Nomograms for predicting local recurrence, distant metastases, and overall survival for patients with locally advanced rectal cancer on the basis of European randomized clinical trials. J Clin Oncol (2011) 29(23):3163–72. doi: 10.1200/JCO.2010.33.1595
24. Han DS, Suh YS, Kong SH, Lee HJ, Choi Y, Aikou S, et al. Nomogram predicting long-term survival after d2 gastrectomy for gastric cancer. J Clin Oncol (2012) 30(31):3834–40. doi: 10.1200/JCO.2012.41.8343
25. Wang Y, Li J, Xia Y, Gong R, Wang K, Yan Z, et al. Prognostic nomogram for intrahepatic cholangiocarcinoma after partial hepatectomy. J Clin Oncol (2013) 31(9):1188–95. doi: 10.1200/JCO.2012.41.5984
26. Zheng S, Zou Y, Xie X, Liang JY, Yang A, Yu K, et al. Development and validation of a stromal immune phenotype classifier for predicting immune activity and prognosis in triple-negative breast cancer. Int J Cancer (2020) 147(2):542–53. doi: 10.1002/ijc.33009
27. Eisenhauer EA, Therasse P, Bogaerts J, Schwartz LH, Sargent D, Ford R, et al. New response evaluation criteria in solid tumours: revised RECIST guideline (version 1.1). Eur J Cancer (2009) 45(2):228–47. doi: 10.1016/j.ejca.2008.10.026
28. León-Letelier RA, Bonifaz LC, Fuentes-Pananá EM. OMIC signatures to understand cancer immunosurveillance and immunoediting: Melanoma and immune cells interplay in immunotherapy. J Leukoc Biol (2019) 105(5):915–33. doi: 10.1002/JLB.MR0618-241RR
29. Herzberg B, Fisher DE. Metastatic melanoma and immunotherapy. Clin Immunol (2016) 172:105–10. doi: 10.1016/j.clim.2016.07.006
30. Xu J, Zhao J, Wang J, Sun C, Zhu X. Prognostic value of lactate dehydrogenase for melanoma patients receiving anti-PD-1/PD-L1 therapy: A meta-analysis. Med (Baltimore) (2021) 100(14):e25318. doi: 10.1097/MD.0000000000025318
31. Pires da Silva I, Ahmed T, McQuade JL, Nebhan CA, Park JJ, Versluis JM, et al. Clinical models to define response and survival with anti-PD-1 antibodies alone or combined with ipilimumab in metastatic melanoma. J Clin Oncol (2022) 40(10):1068–80. doi: 10.1200/JCO.21.01701
32. Manola J, Atkins M, Ibrahim J, Kirkwood J. Prognostic factors in metastatic melanoma: a pooled analysis of Eastern cooperative oncology group trials. J Clin Oncol (2000) 18(22):3782–93. doi: 10.1200/JCO.2000.18.22.3782
33. Petrelli F, Ardito R, Merelli B, Lonati V, Cabiddu M, Seghezzi S, et al. Prognostic and predictive role of elevated lactate dehydrogenase in patients with melanoma treated with immunotherapy and BRAF inhibitors: a systematic review and meta-analysis. Melanoma Res (2019) 29(1):1–12. doi: 10.1097/CMR.0000000000000520
34. Weide B, Martens A, Hassel JC, Berking C, Postow MA, Bisschop K, et al. Baseline biomarkers for outcome of melanoma patients treated with pembrolizumab. Clin Cancer Res (2016) 22(22):5487–96. doi: 10.1158/1078-0432.CCR-16-0127
35. Keung EZ, Gershenwald JE. The eighth edition American joint committee on cancer (AJCC) melanoma staging system: implications for melanoma treatment and care. Expert Rev Anticancer Ther (2018) 18(8):775–84. doi: 10.1080/14737140.2018.1489246
36. Nosrati A, Tsai KK, Goldinger SM, Tumeh P, Grimes B, Loo K, et al. Evaluation of clinicopathological factors in PD-1 response: derivation and validation of a prediction scale for response to PD-1 monotherapy. Br J Cancer (2017) 116(9):1141–7. doi: 10.1038/bjc.2017.70
37. Sohn W, Chang Y, Cho YK, Kim Y, Shin H, Ryu S. Abnormal and euthyroid ranges of thyroid hormones in serum and liver cancer mortality: A cohort study. Cancer Epidemiol Biomarkers Prev (2020) 29(10):2002–9. doi: 10.1158/1055-9965.EPI-20-0283
38. Krashin E, Silverman B, Steinberg DM, Yekutieli D, Giveon S, Fabian O, et al. Pre-diagnosis thyroid hormone dysfunction is associated with cancer mortality. Endocr Relat Cancer (2021) 28(11):705–13. doi: 10.1530/ERC-21-0187
39. Gibney GT, Kudchadkar RR, DeConti RC, Thebeau MS, Czupryn MP, Tetteh L, et al. Safety, correlative markers, and clinical results of adjuvant nivolumab in combination with vaccine in resected high-risk metastatic melanoma. Clin Cancer Res (2015) 21(4):712–20. doi: 10.1158/1078-0432.CCR-14-2468
40. Verzoni E, Cartenì G, Cortesi E, Giannarelli D, De Giglio A, Sabbatini R, et al. Real-world efficacy and safety of nivolumab in previously-treated metastatic renal cell carcinoma, and association between immune-related adverse events and survival: the Italian expanded access program. J Immunother Cancer (2019) 7(1):99. doi: 10.1186/s40425-019-0579-z
41. Ricciuti B, Genova C, De Giglio A, Bassanelli M, Dal Bello MG, Metro G, et al. Impact of immune-related adverse events on survival in patients with advanced non-small cell lung cancer treated with nivolumab: long-term outcomes from a multi-institutional analysis. J Cancer Res Clin Oncol (2019) 145(2):479–85. doi: 10.1007/s00432-018-2805-3
42. Shafqat H, Gourdin T, Sion A. Immune-related adverse events are linked with improved progression-free survival in patients receiving anti-PD-1/PD-L1 therapy. Semin Oncol (2018) 45(3):156–63. doi: 10.1053/j.seminoncol.2018.07.003
43. Judd J, Zibelman M, Handorf E, O'Neill J, Ramamurthy C, Bentota S, et al. Immune-related adverse events as a biomarker in non-melanoma patients treated with programmed cell death 1 inhibitors. Oncologist (2017) 22(10):1232–7. doi: 10.1634/theoncologist.2017-0133
Keywords: metastatic melanoma, immunotherapy, nomogram, anti-pd-1 treatment, baseline indicators
Citation: Zhao J, Li D, Xie S, Deng X, Wen X, Li J, Wu Z, Yang X, Li M, Tang Y, Zhang X and Ding Y (2022) Nomogram for predicting prognosis of patients with metastatic melanoma after immunotherapy: A Chinese population–based analysis. Front. Immunol. 13:1083840. doi: 10.3389/fimmu.2022.1083840
Received: 29 October 2022; Accepted: 06 December 2022;
Published: 22 December 2022.
Edited by:
Manel Juan, Hospital Clinic of Barcelona, SpainReviewed by:
Gatien Moriceau, University of California, Los Angeles, United StatesCopyright © 2022 Zhao, Li, Xie, Deng, Wen, Li, Wu, Yang, Li, Tang, Zhang and Ding. This is an open-access article distributed under the terms of the Creative Commons Attribution License (CC BY). The use, distribution or reproduction in other forums is permitted, provided the original author(s) and the copyright owner(s) are credited and that the original publication in this journal is cited, in accordance with accepted academic practice. No use, distribution or reproduction is permitted which does not comply with these terms.
*Correspondence: Ya Ding, ZGluZ3lhQHN5c3VjYy5vcmcuY24=; Xiaoshi Zhang, emhhbmd4c2hAc3lzdWNjLm9yZy5jbg==; Yan Tang, dGFuZ3lhbkBzeXN1Y2Mub3JnLmNu
†These authors have contributed equally to this work
Disclaimer: All claims expressed in this article are solely those of the authors and do not necessarily represent those of their affiliated organizations, or those of the publisher, the editors and the reviewers. Any product that may be evaluated in this article or claim that may be made by its manufacturer is not guaranteed or endorsed by the publisher.
Research integrity at Frontiers
Learn more about the work of our research integrity team to safeguard the quality of each article we publish.