- 1Department of Protein Engineering, Beijing Institute of Biotechnology, Beijing, China
- 2Division II of In Vitro Diagnostics for Infectious Diseases, Institute for In Vitro Diagnostics Control, National Institutes for Food and Drug Control, Beijing, China
- 3Division of Arboviral Vaccine, National Institutes for Food and Drug Control, Beijing, China
Vaccines are a key weapon against the COVID-19 pandemic caused by SARS-CoV-2. However, there are inter-individual differences in immune response to SARS-CoV-2 vaccines and genetic contributions to these differences have barely been investigated. Here, we performed genome-wide association study (GWAS) of antibody levels in 168 inactivated SARS-CoV-2 vaccine recipients. A total of 177 SNPs, corresponding to 41 independent loci, were identified to be associated with IgG, total antibodies or neutral antibodies. Specifically, the rs4543780, the intronic variant of FAM89A gene, was associated with total antibodies level and was annotated as a potential regulatory variant affecting gene expression of FAM89A, a biomarker differentiating bacterial from viral infections in febrile children. These findings might advance our knowledge of the molecular mechanisms driving immunity to SARS-CoV-2 vaccine.
Introduction
Coronavirus disease 2019 (COVID-19) is an infectious disease caused by Severe Acute Respiratory Syndrome-Coronavirus 2 (SARS-CoV-2). It was first discovered in 2019 (1–3) and has spread worldwide thereafter, resulting more than 610 million infections and six million deaths up to September 21, 2022 (https://covid19.who.int/). Therefore, the development of safe and effective vaccines against SARS-CoV-2 has been urgently needed.
An inactivated vaccine is one that uses a killed pathogen to stimulate the immune system to protect the body against infection, which has been successfully applied in preventing diseases such as polio, hepatitis A, influenza, Japanese encephalitis and rabies (4). Two kinds of inactivated SARS-CoV-2 vaccines, BBIBP-CorV and CoronaVac (5, 6), developed by Sinopharm-Bejing Institute of Biological Products Co. and Sinovac Life Sciences respectively, have shown safety and efficiency in clinical trials (7–10) and were granted for emergency use by the World Health Organization. Antibodies induced by vaccines play a key role in preventing disease, and researches indicate that neutralizing antibody levels are highly predictive of immune protection from symptomatic SARS-CoV-2 infection (11). However, differential antibody response to SARS-CoV-2 vaccines in healthy subjects is observed (12) while the related factors remain to be defined.
Human genetic background has been suggested to contribute to inter-individual difference in antibody response to many vaccines (13, 14). For example, multiple studies revealed HLA variants were associated with antibody response to hepatitis B vaccine (15–18). CD46 and IFI44L genetic variants were revealed to be associated with neutralizing antibody response to measles vaccine (19). Common SNPs in IL18R1 and IL18 genes were associated with variations in humoral immunity to smallpox vaccination in both Caucasians and African Americans (20). In addition, recent studies suggested that SNPs in the regulatory region of IGH gene were associated with antibody levels in response to SARS-CoV-2 vaccine (21). However, a genome-wide profiling of genetic variants associated with the antibody levels induced by the SARS-CoV-2 vaccine is still lacking.
Here, we performed genome-wide association study (GWAS) of genetic variants associated with antibody levels induced by inactivated SARS-CoV-2 vaccines. A total of 117 SNPs, corresponding to 41 independent loci, were identified to be associated with IgG, total antibodies (Ab) or neutral antibodies (NAbs) (P < 5e-7). Specifically, the rs4543780, residing in the intron of FAM89A (Family With Sequence Similarity 89 Member A) gene, which was associated with total antibodies level (P = 2.86e-7), was annotated as a potential regulatory variant affecting FAM89A gene expression. These findings might advance our knowledge of the precise mechanisms driving immunity to SARS-CoV-2 vaccine.
Materials and methods
Study participants
A total of 176 individuals who received two doses of SARS-CoV-2 inactivated vaccine was recruited at Beijing, China between February 24th and June 25th, 2021. The inactivated vaccine was either BBIBP-CorV (Sinopharm and Bejing Institute of Biological Products Co., Beijing, China) or CoronaVac (Sinovac Life Sciences, Beijing, China). After removing close related individuals and poor genotyped individuals based on genome-wide genotyping data, 168 individuals were kept for further analysis. Informed consent was obtained from all vaccinated volunteers enrolled in studies at the Beijing BGI Clinical Laboratories. The Institutional Review Board (IRB) of BGI-Shenzhen approved the serological and genomic polymorphism analyses of samples collected by the aforementioned institution under ethical clearance No. BGI-IRB 20158.
Antibody level assay
Serum samples were collected between day 12 and day 141 after the second dose of vaccine to measure antibody levels. IgG, IgA, IgM, total antibodies and neutralizing antibodies against SARS-CoV-2 were detected using magnetic chemiluminescence enzyme immunoassay kits (Bioscience), according to the manufacturer’s instructions. Antibody levels are presented as the measured chemiluminescence values divided by the cutoff (absorbance/cutoff, S/CO). The cutoff value of this test was defined by receiver operating characteristic curves. An S/CO value higher than 1 was regarded as positive.
Genotyping, imputation and quality control
Genomic DNAs were extracted from 200uL of peripheral whole blood, according to the manufacturer’s instructions (CWBIO, Magbead Blood DNA Kit). The DNA concentration was measured using Nanodrop, and the DNA degradation and contamination were monitored in 1% agarose gels.
Genotyping was performed using the CBT_PMRA Array, consisted of about 0.8 million SNPs, at CapitalBio Corporation (Beijing, China). Two of 176 samples had low dish quality control (DQC < 0.82) and were removed from further genotype calling. Genotype callings were performed using Axiom Analysis Suite 3.1.51 based on the default workflow. All the 174 individuals had genotype call rates > 90%. Seven individuals showed sex discrepancies and were changed of sex assignments to those imputed from X chromosome inbreeding coefficients. Six individuals were excluded as they were related with the other individuals based on pairwise identity-by-state by “PI_HAT” values in PLINK 1.9 (PI_HAT > 0.5 and between 0.25 and 0.5 indicates the first- and second-degree relatives, respectively), leaving 168 individuals for further analysis.
Imputation on the genotyping data of chromosomes 1-22 was performed using the ChinaMAP Imputation Server (http://www.mbiobank.com/imputation/) (22), a genotype imputation server utilizing the ChinaMAP reference panel constructed from the China Metabolic Analytics Project (ChinaMAP) WGS dataset. After imputation, the SNPs with R-squares (R2) below 0.6 or minor allele frequencies (MAF) < 0.01 were excluded. Further SNP quality controls filtered out SNPs that had call rates < 90%, deviated significantly from Hardy–Weinberg equilibrium (HWE) (P < 1e-6) or MAF < 0.05. Finally, a total of 5,089,908 SNPs passed quality controls.
Genome-wide association tests
Genetic association analysis was conducted using PLINK 1.9 software (23). As for IgG, Ab or NAbs levels, we carried out genome-wide association tests in linear regression models. As for IgA or IgM level, we categorized the antibody level as two group: S/CO ≥ 1 as positive group and S/CO < 1 as negative group, and carried out genome-wide association tests in logistic regression models. Either linear regression or logistic regression analysis adjusted for covariates including: age, gender, vaccine type, time from the 2nd dose of immunization to blood draw, and the top six principal components from PCA to correct for population stratification. To display the association results, Manhattan plots were constructed using the R-package qqman (24). Quantile–quantile (Q-Q) plots of the observed -log10 (P) against values predicted from the reference distribution under the null hypothesis were constructed and values of lambda (λ) inflation factor were calculated to assess any inflation in the levels of significance (25).
Annotation of significant SNPs
The position of significant SNPs relative to genes was annotated by Ensembl Variant Effect Predictor (VEP) (26). Regulatory potential of significant SNPs was prioritized using RegulomeDB (27), a database that annotates SNPs based on chromatin immunoprecipitation (ChIP)-seq, formaldehyde-assisted isolation of regulatory elements (FAIRE), and DNase I hypersensitive site data sets from the Encyclopedia of DNA Elements (ENCODE) project (28). Regional plot of significant SNPs were performed by LocusZoom (29).
Statistical analysis
The antibody levels in different vaccine type groups were compared using Kruskal-Wallis rank sum test. Comparing antibody levels between males and females was conducted using Wilcoxon rank sum test with continuity correction. Pearson correlation test was used to examine correlation of levels of different antibodies, as well as correlation of antibody levels with age and the interval from the 2nd dose of vaccine to blood draw. P < 0.05 was considered as statistically significant.
The currently accepted P-value threshold to declare a SNP to be genome-wide significant is < 5e-8. Because our current study is comprised of limited number of subjects, and because we plan to identify more candidate SNPs to be further validated and studied, we have chosen the less stringent, but still conservative significance threshold of 5e-7 and considered variants with P > 5e-8 but < 5e-7 as suggestive evidence of association to be highlighted in this report as well. Power analysis by Genetic Power Calculator (30) indicated that for a variant with a MAF of 0.2 and a heritability of 2% and given type I error rate of 5e-7, the sample size to achieve 80% power is at least 155.
Results
Demographic characteristics and antibody levels
A total of 176 individuals who had received two doses of inactivated SARS-CoV-2 vaccine participated in our study. After removing close related individuals and poor genotyped individuals based on genome-wide genotyping data, 168 individuals were kept for further analysis. Of these, 116 were vaccinated two doses of CoronaVac vaccine, 50 were vaccinated two doses of BBIBP-CorV vaccine and two were vaccinated one dose of CoronaVac vaccine and one dose of BBIBP-CorV vaccine (Figure 1A). Female individuals were slightly more than male (58% vs. 42%) (Figure 1B). The majority of individuals were young, with a median age of 31.5 years (Figure 1C). The time from the second SARS-CoV-2 vaccination to blood draw was between 12 and 141 days, with nearly half of individuals (41%) being 126 days (Figure 1D).
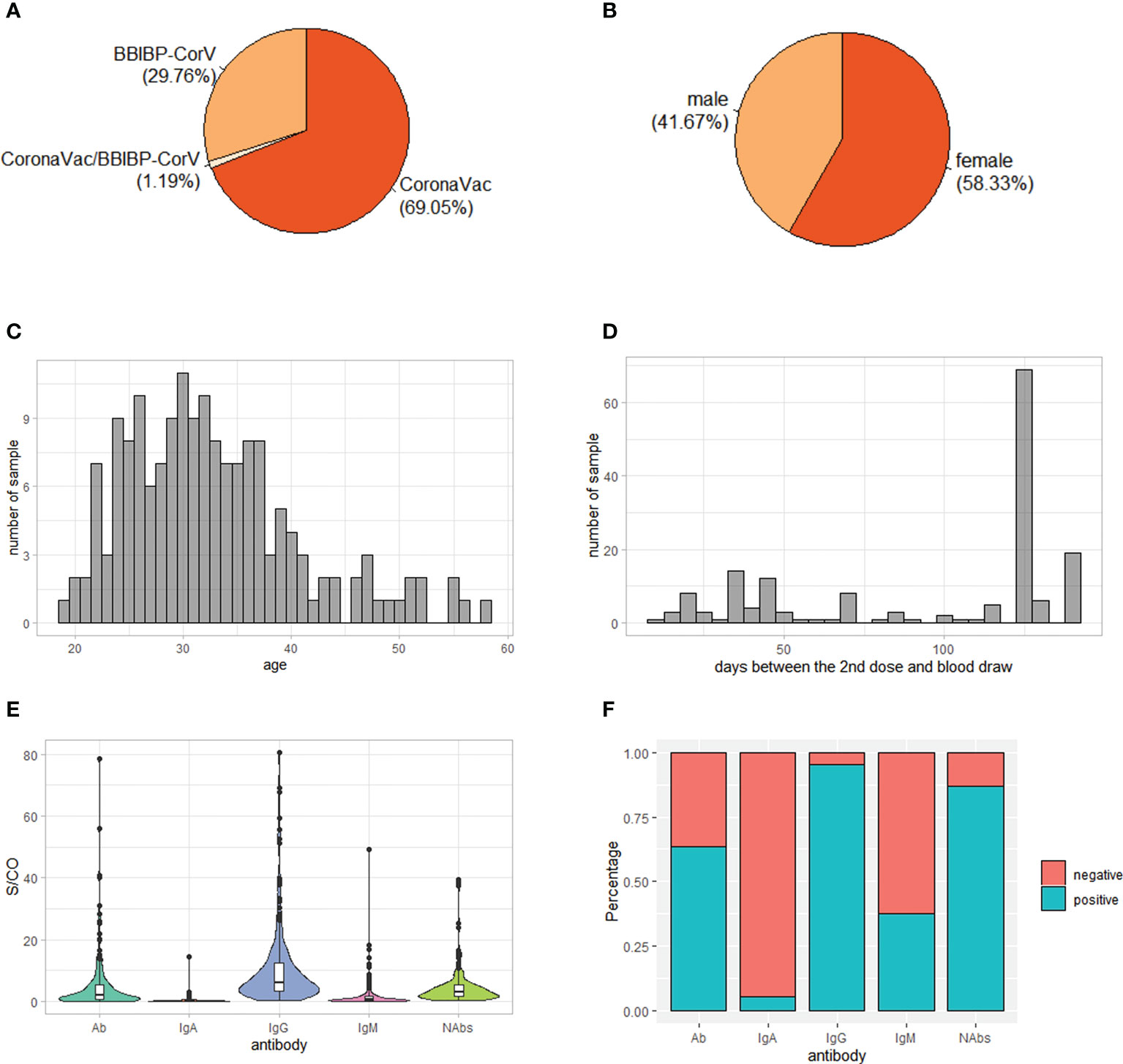
Figure 1 Demographic characteristics and antibody levels of the 168 study subjects. (A) Vaccine type distribution; (B) Sex ratio; (C) Age distribution; (D) Distribution of days between the 2nd dose and blood draw; (E) Level of IgG, IgM, IgA, total antibodies (Ab) and neutralizing antibodies (NAbs) S/CO: sample/cutoff. (F) Percentage of positive individuals for IgG, IgM, IgA, Ab and NAbs.
Consistent with previous reports (12, 31), the serum antibody response to inactivated SARS-CoV-2 vaccines was mainly IgG, with the median level of IgG being 6.13 while the median levels of IgA and IgM being 0.20 and 0.56, respectively (Figure 1E). In addition, 95% of individuals was positive of IgG while only 5% and 38% of individuals was positive of IgA and IgM, respectively (Figure 1F). As expected, the level of Ab was positively correlated with levels of IgG, IgM and IgA (Figure S1A). On the other hand, the level of NAbs was positively correlated with level of IgG, suggesting that it was mainly IgG contributing to neutralizing effect of antibodies against SARS-CoV-2 (Figure S1A).
Differences of IgM and Ab levels were observed in different vaccine type groups (P = 6e-5 and P = 2e-4, respectively), with a higher level in BBIBP-CorV group than CoronaVac group, while IgG, IgA and NAbs levels had no significant differences among different vaccine type groups (P > 0.05) (Figure S1B). Furthermore, sex and age had no significant effect on antibody levels (Figures S1C, D). However, the time interval between the 2nd dose of immunization and blood draw was negatively correlated the levels of IgG, IgM, IgA and Ab, suggesting that the antibody levels might decline with time (Figure S1D).
Genetic associations with antibody responses
To characterize the genetic association with antibody responses after SARS-CoV-2 inactivated vaccine immunization, we performed genome-wide association study of IgG, Ab and NAbs levels in linear regression models adjusting for covariates including: age, gender and the top five principal components from PCA. As for IgM and IgA, because their levels were low and a large proportion of individuals were negative, we categorized the individuals into positive group and negative group based on the seropositivity, and carried out genome-wide association tests in logistic regression models. Q-Q plots of the observed vs. expected -log10(P) indicated that there was no severe inflation in these statistical tests (Figure S2).
Analysis of the genome-wide association data identified a total of 177 SNP associations with variations in antibody levels at P < 5e-7, corresponding 41 independent loci. Among these associations, 12 SNPs, corresponding eight independent loci were associated with IgG level (Figure 2A); 99 SNPs, corresponding 15 independent loci were associated with total antibodies level (Figure 2B); 66 SNPs, corresponding 18 independent loci were associated with neutralizing antibodies level (Figure 2C). The lead independent SNPs were listed in Tables 1–3. No SNPs were significantly associated with the seropositivity of IgM or IgA (Figure S3).
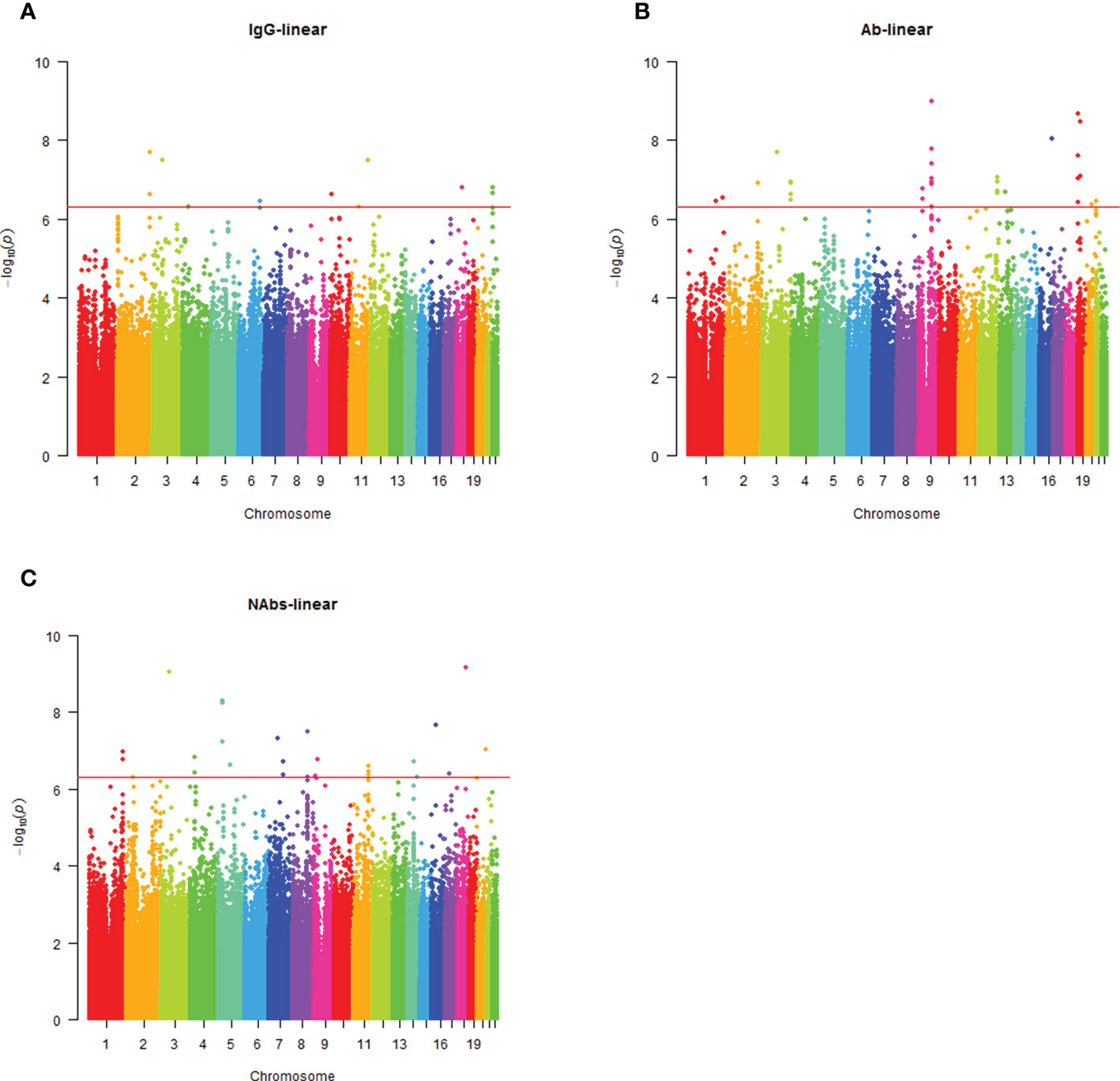
Figure 2 Manhattan plot summaries of GWAS results for antibody response. (A) Results for IgG level; (B) Results for Ab level; (C) Results for NAbs level.
Annotation of significant associated SNPs
Annotation of significant associated SNPs using Ensembl Variant Effect Predictor (VEP) (26) revealed that the majority of SNPs (86.49%) resided in the intron of genes and intergenic region, and none of SNPs resided in the exon of genes (Figure S4), suggesting that these SNPs might function as regulatory variants affecting gene expression. Thus, we prioritized the regulatory potential of significant SNPs using RegulomeDB (27), a database that annotates SNPs based on chromatin immunoprecipitation (ChIP)-seq, formaldehyde-assisted isolation of regulatory elements (FAIRE), and DNase I hypersensitive site data sets from the Encyclopedia of DNA Elements (ENCODE) project (28).
Interestingly, rs4543780, an intronic SNP associated with total antibodies level (P = 2.86e-7, Figure 3A and Table 2), was ranked as 1f (Figure S5), which meant it was an eQTL and overlapped with transcription factor binding site or DNase peak. Indeed, it resided in DNase peak (Figure 3B) and GTEx data (32) showed that T allele of this variant was associated with lower expression of FAM89A gene in brain and muscle (Table 4), suggesting this variant might contribute to antibody response through regulating FAM89A gene expression. In addition, though the function of FAM89A gene has barely been investigated, this gene has been found to be upregulated in pathogen infections (33–35), indicating that it might be involved in immune response.
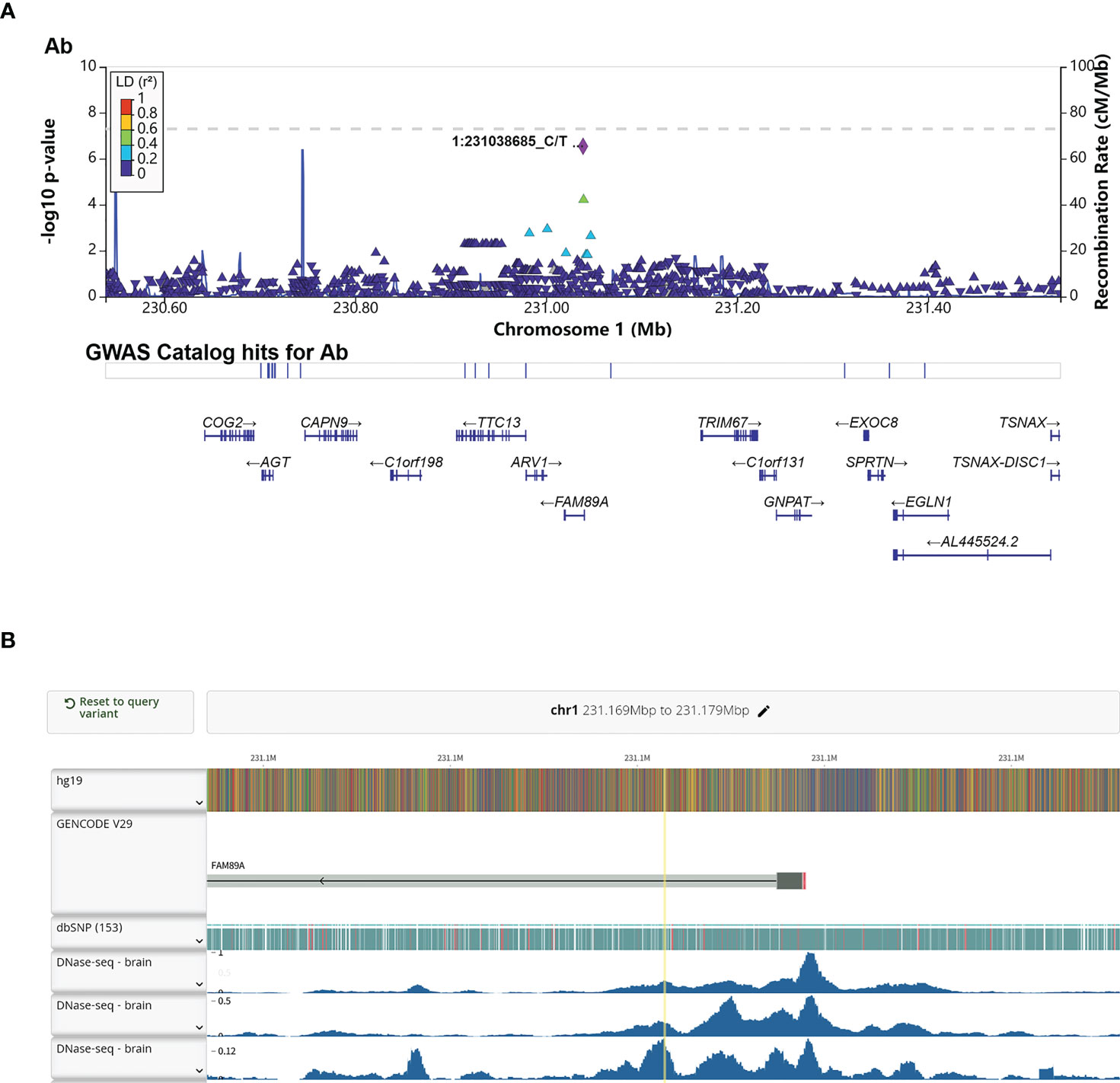
Figure 3 Annotation of the significant association SNP rs4543780. (A) LocusZoom plot showed the P value of the SNPs centering the lead SNP rs4543780, linkage disequilibrium degree, the recombination rate (top panel), SNP hits in GWAS catalogue (middle panel) and the genes in the region (bottom panel). 1:231038685_C/T indicated rs4543780 residing in chr1: 231038685 with reference allele of C and alternative allele of T; (B) DNase-seq peaks in the region of rs4543780 annotated by RegulomeDB. The yellow vertical line indicated the position of rs4543780.
Discussion
In this study, we identified 177 SNPs, corresponding to 41 independent loci, that were associated with IgG, total antibodies or neutral antibodies. Specifically, the intronic variant of FAM89A gene, rs4543780, which was associated with total antibodies level, was annotated as a potential regulatory variant affecting FAM89A gene expression.
To the best of our knowledge, this is the first genome-wide association study of antibody response to SARS-CoV-2 vaccine. Previously there were two candidate gene studies. Ragone et al. found HLA did not impact on short-medium-term antibody response to Pfizer-BioNTech BNT162b2 vaccine, which was a SARS-CoV-2 mRNA vaccine (36), though HLA variants have been shown to be associated with antibody response to several other pathogen vaccines (15–18, 37–39). Consistent of this research, we did not found associations between HLA variants and antibody response either. Another study by Colucci et al. found associations between allelic variants of the human IgH 3’ regulatory region 1 and the immune response to BNT162b2 mRNA vaccine (21). However, these variants did not pass the statistical threshold in our analysis, probably due to different kinds of vaccines, as we only had inactivated COVID-19 vaccine recipients available. On the other hand, our genome-wide analysis newly identified 177 SNPs, corresponding to 41 independent loci, that were associated with IgG, total antibodies or neutral antibodies.
Annotation of these significant associated SNPs revealed that none of SNPs resided in the exon of genes, with the majority of SNPs (86.49%) residing in the intron of genes and intergenic region, suggesting that these SNPs might function as regulatory SNPs of gene expression. Further annotation by RegulomeDB (27) prioritized the intronic SNP of FAM89A gene, rs4543780, as a potential regulatory SNP. This variant was significantly associated with total antibodies level. Moreover, ENCODE (28) data showed it resided in the open chromatin region and GTEx (32) data showed it was associated with FAM89A gene expression, suggesting that it might affect antibody response by regulating FAM89A gene expression. Though the function of FAM89A gene is not well studied, researches indicated that FAM89A gene, together with IFI44L gene, was capable of differentiating between bacterial and viral infections with high sensibility and specificity (33–35), suggesting that FAM89A gene might be involved in immune response. However, FAM89A had elevated expression in the blood of febrile children with bacterial infection rather than viral infection, which seemed to conflict with FAM89A gene being associated with antibody response to virus vaccines. One possible reason for this discrepancy might be tissue-specific gene expression regulation, as GTEx data showed rs4543780 was associated with FAM89A gene expression in brain and muscle instead of blood.
In addition to FAM89A gene, a number of genes implicated in COVID-19 and immune process were identified to harbor polymorphisms associated with antibody response to COVID-19 vaccines, such as ADA2 (Adenosine Deaminase 2), COX6C (Cytochrome C Oxidase Subunit 6C), FUT8 (Fucosyltransferase 8) and ASIC2 (Acid Sensing Ion Channel Subunit 2).
ADA2 gene encodes a member of a subfamily of the adenosine deaminase protein family that contributes to the degradation of extracellular adenosine, a signaling molecule that controls a variety of cellular responses. Serum increases of ADA2 activity has been described in patients with bacterial and viral diseases (40, 41), including individuals with SARS-CoV-2 infection and who recovered from infection (42). The deficiency of adenosine deaminase 2 (DADA2) is an autosomal recessively inherited disease that undergoes immune dysregulation including hypogammaglobulinemia, absent to low class-switched memory B cells, and inadequate response to vaccination (43). In our study, rs5994195 in the intron of ADA2 was identified to be associated with IgG level of COVID-19 vaccine immunization.
COX6C gene encodes component of the cytochrome c oxidase, the last enzyme in the mitochondrial electron transport chain which drives oxidative phosphorylation. COX6C is differentially expressed in multiple myeloma (MM) and is associated with MM prognosis (44). Multiple myeloma (MM) is a malignant proliferation of plasma cells, with the coexistence of a monoclonal component (M-component) plus impairment of normal immunoglobulin production, which are associated with increased risk of viral and bacterial infections (45). MM patients with COVID-19 show a longer duration to clinical improvement (46) and a higher risk of inpatient mortality (47). Moreover, COX6C is downregulated in patients with mild COVID-19 infection compared with controls but is upregulated in patients with severe COVID-19 compared with patients with mild illness (44). In our study, rs12548840 in the intron of COX6C was identified to be associated with NAbs level of COVID-19 vaccine immunization.
FUT8 gene encodes an enzyme belonging to the family of fucosyltransferases. Core fucosylation of IgG B cell receptor by FUT8 is required for antigen recognition and antibody production (48, 49). In addition, genome-wide association study revealed multiple SNPs in FUT8 gene had strong influences on the IgG glycosylation patterns (50, 51). In our study, rs7146742 in the intron of FUT8 was identified to be associated with NAbs level of COVID-19 vaccine immunization.
ASIC2 gene, also known as ACCN1 (Amiloride-Sensitive Cation Channel Neuronal 1), encodes the cation channel with high affinity for sodium, which is gated by extracellular protons and inhibited by the diuretic amiloride. The SNP rs28936 located in the 3’ UTR of ASIC2 gene is significantly associated with Multiple Sclerosis (MS) (52), an autoimmune disease that your immune system mistakenly attacks cells in the myelin and interrupts nerve signals from your brain to other parts of your body. In addition, an increase of ASIC2 mRNA was observed in the human autoptic brain tissue of MS patients and knockout of Asic2 resulted in a significant reduction in the clinical score in experimental autoimmune encephalomyelitis (EAE) mice model (53), highlighting the involvement of ASIC2 in the immune progress. In our study, rs75953002 in the intron of ASIC2 was identified to be associated with NAbs level of COVID-19 vaccine immunization.
The primary limitation of this study is the relatively small sample size, which might result in limited statistical power and excess false positive results. Besides, population stratification, vaccine types and the time from immunization to antibody detection could potentially bias the results. As conditions like smoking, hypertension and type 2 diabetes are associated with COVID-19 outcomes (54–56), and diseases such as schizophrenia and Alzheimer’s disease tend to be post-COVID-19 sequelae (57, 58), they may also be confounding factors for an immune reaction to SARS-CoV-2 vaccination. Therefore, further validation of our findings in a larger cohort will be needed.
In summary, we have identified a list of associated genetic variants contributing to inter-individual variation in antibody response after SARS-CoV-2 vaccination, which might inspire further genetic association researches and contribute to biological insights into vaccine response and better vaccine development.
Data availability statement
The datasets presented in this study can be found in online repositories. The names of the repository/repositories and accession number(s) can be found in the article/Supplementary Material.
Ethics statement
The studies involving human participants were reviewed and approved by The Institutional Review Board (IRB) of BGI-Shenzhen. The patients/participants provided their written informed consent to participate in this study.
Author contributions
SX, YZ, and ZZ contributed to conception and design of the study. DS and SX were responsible for recruitment of vaccinated volunteers. PL and DS performed the experiments. PL analyzed the data. DS, WS, SS, XG, and JL helped to analyze and interpret the data. PL wrote the first draft of the manuscript. All authors contributed to the article and approved the submitted version.
Funding
This work was supported by grants from the National Key Research and Development Program of China (2018YFC1200704, 2018YFA0900801) and the National Natural Science Foundation of China (31801087).
Acknowledgments
We thank all the participants in this study.
Conflict of interest
The authors declare that the research was conducted in the absence of any commercial or financial relationships that could be construed as a potential conflict of interest.
Publisher’s note
All claims expressed in this article are solely those of the authors and do not necessarily represent those of their affiliated organizations, or those of the publisher, the editors and the reviewers. Any product that may be evaluated in this article, or claim that may be made by its manufacturer, is not guaranteed or endorsed by the publisher.
Supplementary material
The Supplementary Material for this article can be found online at: https://www.frontiersin.org/articles/10.3389/fimmu.2022.1054147/full#supplementary-material
Supplementary Figure 1 | Correlation between demographic characteristics and antibody levels. (A) Correlation between levels of different antibodies; (B) Antibody levels among different vaccine types; (C) Antibody levels between males and females. B: BBIBP-CorV, C: CoronaVac, BC: CoronaVac/BBIBP-CorV; (D) Correlation of antibody levels with age and interval from the 2nd dose to blood draw. * indicated P<0.05, ** indicated P<0.01, *** indicated P<0.001
Supplementary Figure 2 | Q-Q plot plots of the expected (x-axis) and observed (y-axis) -log10 (P) in SARS-CoC-2 vaccine response GWAS. (A) Results for IgG level; (B) Results for Ab level; (C) Results for NAbs level; (D) Results for IgA positivity; (E) Results for IgM positivity
Supplementary Figure 3 | Manhattan plot summaries of GWAS results for IgA and IgM. (A) Results for IgA positivity using logistic regression approach; (B) Results for IgM positivity using logistic regression approach.
Supplementary Figure 4 | Distribution of the relative position of significant SNPs to genes.
Supplementary Figure 5 | Distribution of the ranks of significant SNPs obtained from RegulomeDB. Red, green and blue indicated significant SNPs associated with Ab, IgG and NAbs level, respectively. The detail meaning of the rank represent could be found in the help page of RegulomeDB (https://regulome.stanford.edu/regulome-help/)
References
1. Huang C, Wang Y, Li X, Ren L, Zhao J, Hu Y, et al. Clinical features of patients infected with 2019 novel coronavirus in wuhan, China. Lancet (2020) 395(10223):497–506. doi: 10.1016/S0140-6736(20)30183-5
2. Wang C, Horby PW, Hayden FG, Gao GF. A novel coronavirus outbreak of global health concern. Lancet (2020) 395(10223):470–3. doi: 10.1016/S0140-6736(20)30185-9
3. Zhu N, Zhang D, Wang W, Li X, Yang B, Song J, et al. A novel coronavirus from patients with pneumonia in China, 2019. N Engl J Med (2020) 382(8):727–33. doi: 10.1056/NEJMoa2001017
4. Sanders B, Koldijk M, Schuitemaker H. Inactivated viral vaccines. Eds. Nunnally BK, Turula VE, Sitrin RD In: Vaccine Analys: Strategies, Principles, and Control (Berlin, Heidelberg: Springer Berlin Heidelberg) (2015), 45–80. doi: 10.1007/978-3-662-45024-6_2
5. Gao Q, Bao L, Mao H, Wang L, Xu K, Yang M, et al. Development of an inactivated vaccine candidate for SARS-CoV-2. Sci (New York NY) (2020) 369(6499):77–81. doi: 10.1126/science.abc1932
6. Wang H, Zhang Y, Huang B, Deng W, Quan Y, Wang W, et al. Development of an inactivated vaccine candidate, BBIBP-CorV, with potent protection against SARS-CoV-2. Cell (2020) 182(3): 713–21.e9 doi: 10.1016/j.cell.2020.06.008
7. Al Kaabi N, Zhang Y, Xia S, Yang Y, Al Qahtani MM, Abdulrazzaq N, et al. Effect of 2 inactivated SARS-CoV-2 vaccines on symptomatic COVID-19 infection in adults: A randomized clinical trial. JAMA (2021) 326(1):35–45. doi: 10.1001/jama.2021.8565
8. Tanriover MD, Doğanay HL, Akova M, Güner HR, Azap A, Akhan S, et al. Efficacy and safety of an inactivated whole-virion SARS-CoV-2 vaccine (CoronaVac): interim results of a double-blind, randomised, placebo-controlled, phase 3 trial in Turkey. Lancet (2021) 398(10296):213–22. doi: 10.1016/S0140-6736(21)01429-X
9. Xia S, Zhang Y, Wang Y, Wang H, Yang Y, Gao GF, et al. Safety and immunogenicity of an inactivated SARS-CoV-2 vaccine, BBIBP-CorV: a randomised, double-blind, placebo-controlled, phase 1/2 trial. Lancet Infect Dis (2021) 21(1):39–51. doi: 10.1016/S1473-3099(20)30831-8
10. Zhang Y, Zeng G, Pan H, Li C, Hu Y, Chu K, et al. Safety, tolerability, and immunogenicity of an inactivated SARS-CoV-2 vaccine in healthy adults aged 18-59 years: a randomised, double-blind, placebo-controlled, phase 1/2 clinical trial. Lancet Infect Dis (2021) 21(2):181–92. doi: 10.1016/S1473-3099(20)30843-4
11. Khoury DS, Cromer D, Reynaldi A, Schlub TE, Wheatley AK, Juno JA, et al. Neutralizing antibody levels are highly predictive of immune protection from symptomatic SARS-CoV-2 infection. Nat Med (2021) 27(7):1205–11. doi: 10.1038/s41591-021-01377-8
12. Zhang J, Xing S, Liang D, Hu W, Ke C, He J, et al. Differential antibody response to inactivated COVID-19 vaccines in healthy subjects. Front Cell infect Microbiol (2021) 11:791660. doi: 10.3389/fcimb.2021.791660
13. Tan PL, Jacobson RM, Poland GA, Jacobsen SJ, Pankratz VS. Twin studies of immunogenicity–determining the genetic contribution to vaccine failure. Vaccine (2001) 19(17-19):2434–9. doi: 10.1016/S0264-410X(00)00468-0
14. Newport MJ, Goetghebuer T, Weiss HA, Whittle H, Siegrist CA, Marchant A. Genetic regulation of immune responses to vaccines in early life. Genes Immun (2004) 5(2):122–9. doi: 10.1038/sj.gene.6364051
15. Png E, Thalamuthu A, Ong RTH, Snippe H, Boland GJ, Seielstad M. A genome-wide association study of hepatitis b vaccine response in an Indonesian population reveals multiple independent risk variants in the HLA region. Hum Mol Genet (2011) 20(19):3893–8. doi: 10.1093/hmg/ddr302
16. Pan L, Zhang L, Zhang W, Wu X, Li Y, Yan B, et al. A genome-wide association study identifies polymorphisms in the HLA-DR region associated with non-response to hepatitis b vaccination in Chinese han populations. Hum Mol Genet (2014) 23(8):2210–9. doi: 10.1093/hmg/ddt586
17. Nishida N, Sugiyama M, Sawai H, Nishina S, Sakai A, Ohashi J, et al. Key HLA-DRB1-DQB1 haplotypes and role of the BTNL2 gene for response to a hepatitis b vaccine. Hepatology (2018) 68(3):848–58. doi: 10.1002/hep.29876
18. Chung S, Roh EY, Park B, Lee Y, Shin S, Yoon JH, et al. GWAS identifying HLA-DPB1 gene variants associated with responsiveness to hepatitis b virus vaccination in koreans: Independent association of HLA-DPB1*04:02 possessing rs1042169 G - rs9277355 c - rs9277356 a. J Viral Hepat (2019) 26(11):1318–29. doi: 10.1111/jvh.13168
19. Haralambieva IH, Ovsyannikova IG, Kennedy RB, Larrabee BR, Zimmermann MT, Grill DE, et al. Genome-wide associations of CD46 and IFI44L genetic variants with neutralizing antibody response to measles vaccine. Hum Genet (2017) 136(4):421–35. doi: 10.1007/s00439-017-1768-9
20. Haralambieva IH, Ovsyannikova IG, Dhiman N, Kennedy RB, O'Byrne M, Pankratz VS, et al. Common SNPs/haplotypes in IL18R1 and IL18 genes are associated with variations in humoral immunity to smallpox vaccination in caucasians and African americans. J Infect dis (2011) 204(3):433–41. doi: 10.1093/infdis/jir268
21. Colucci M, De Santis E, Totti B, Miroballo M, Tamiro F, Rossi G, et al. Associations between allelic variants of the human IgH 3' regulatory region 1 and the immune response to BNT162b2 mRNA vaccine. Vaccines (Basel) (2021) 9(10): 1207. doi: 10.3390/vaccines9101207
22. Li L, Huang P, Sun X, Wang S, Xu M, Liu S, et al. The ChinaMAP reference panel for the accurate genotype imputation in Chinese populations. Cell Res (2021) 31(12):1308–10. doi: 10.1038/s41422-021-00564-z
23. Chang CC, Chow CC, Tellier LC, Vattikuti S, Purcell SM, Lee JJ. Second-generation PLINK: rising to the challenge of larger and richer datasets. Gigascience (2015) 4:7. doi: 10.1186/s13742-015-0047-8
24. Turner SD. Qqman: an r package for visualizing GWAS results using q-q and manhattan plots. J Open Source Software (2018) 3(25):731. doi: 10.21105/joss.00731
25. Devlin B, Roeder K. Genomic control for association studies. Biometrics (1999) 55(4): 997–1004. doi: 10.1111/j.0006-341X.1999.00997.x
26. McLaren W, Gil L, Hunt SE, Riat HS, Ritchie GRS, Thormann A, et al. The ensembl variant effect predictor. Genome Biol (2016) 17(1):122. doi: 10.1186/s13059-016-0974-4
27. Boyle AP, Hong EL, Hariharan M, Cheng Y, Schaub MA, Kasowski M, et al. Annotation of functional variation in personal genomes using RegulomeDB. Genome Res (2012) 22(9):1790–7. doi: 10.1101/gr.137323.112
28. Consortium EP. An integrated encyclopedia of DNA elements in the human genome. Nature (2012) 489(7414):57–74. doi: 10.1038/nature11247
29. Boughton AP, Welch RP, Flickinger M, VandeHaar P, Taliun D, Abecasis GR, et al. LocusZoom.js: Interactive and embeddable visualization of genetic association study results. Bioinformatics (2021): 37(18): 3017–8. doi: 10.1101/2021.01.01.423803
30. Purcell S, Cherny SS, Sham PC. Genetic power calculator: design of linkage and association genetic mapping studies of complex traits. Bioinformatics (2003) 19(1):149–50. doi: 10.1093/bioinformatics/19.1.149
31. Ren C, Gao Y, Zhang C, Zhou C, Hong Y, Qu M, et al. Respiratory mucosal immunity: Kinetics of secretory immunoglobulin a in sputum and throat swabs from COVID-19 patients and vaccine recipients. Front Microbiol (2022) 13:782421. doi: 10.3389/fmicb.2022.782421
32. Consortium G. The GTEx consortium atlas of genetic regulatory effects across human tissues. Science (2020) 369(6509):1318–30. doi: 10.1126/science.aaz1776
33. Herberg JA, Kaforou M, Wright VJ, Shailes H, Eleftherohorinou H, Hoggart CJ, et al. Diagnostic test accuracy of a 2-transcript host RNA signature for discriminating bacterial vs viral infection in febrile children. JAMA (2016) 316(8):835–45. doi: 10.1001/jama.2016.11236
34. Kaforou M, Herberg JA, Wright VJ, Coin LJM, Levin M. Diagnosis of bacterial infection using a 2-transcript host RNA signature in febrile infants 60 days or younger. JAMA (2017) 317(15):1577–8. doi: 10.1001/jama.2017.1365
35. Barral-Arca R, Pardo-Seco J, Martinón-Torres F, Salas A. A 2-transcript host cell signature distinguishes viral from bacterial diarrhea and it is influenced by the severity of symptoms. Sci Rep (2018) 8(1):8043. doi: 10.1038/s41598-018-26239-1
36. Ragone C, Meola S, Fiorillo PC, Penta R, Auriemma L, Tornesello ML, et al. HLA does not impact on short-Medium-Term antibody response to preventive anti-SARS-Cov-2 vaccine. Front Immunol (2021) 12:734689. doi: 10.3389/fimmu.2021.734689
37. Ovsyannikova IG, Pankratz VS, Vierkant RA, Jacobson RM, Poland GA. Human leukocyte antigen haplotypes in the genetic control of immune response to measles-mumps-rubella vaccine. J Infect dis (2006) 193(5):655–63. doi: 10.1086/500144
38. Ovsyannikova IG, Vierkant RA, Pankratz VS, Jacobson RM, Poland GA. Human leukocyte antigen genotypes in the genetic control of adaptive immune responses to smallpox vaccine. J Infect dis (2011) 203(11):1546–55. doi: 10.1093/infdis/jir167
39. Liu Y, Guo T, Yu Q, Zhang H, Du J, Zhang Y, et al. Association of human leukocyte antigen alleles and supertypes with immunogenicity of oral rotavirus vaccine given to infants in China. Medicine (2018) 97(40):e12706. doi: 10.1097/MD.0000000000012706
40. Tsuboi I, Sagawa K, Shichijo S, Yokoyama MM, Ou DW, Wiederhold MD. Adenosine deaminase isoenzyme levels in patients with human T-cell lymphotropic virus type 1 and human immunodeficiency virus type 1 infections. Clin Diagn Lab Immunol (1995) 2(5):626–30. doi: 10.1128/cdli.2.5.626-630.1995
41. Kumabe A, Hatakeyama S, Kanda N, Yamamoto Y, Matsumura M. Utility of ascitic fluid adenosine deaminase levels in the diagnosis of tuberculous peritonitis in general medical practice. Can J Infect Dis Med Microbiol (2020) 2020:5792937. doi: 10.1155/2020/5792937
42. Franco-Martínez L, Tecles F, Torres-Cantero A, Bernal E, San Lázaro I, Alcaraz MJ, et al. Analytical validation of an automated assay for the measurement of adenosine deaminase (ADA) and its isoenzymes in saliva and a pilot evaluation of their changes in patients with SARS-CoV-2 infection. Clin Chem Lab Med (2021) 59(9):1592–9. doi: 10.1515/cclm-2021-0324
43. Barron KS, Aksentijevich I, Deuitch NT, Stone DL, Hoffmann P, Videgar-Laird R, et al. The spectrum of the deficiency of adenosine deaminase 2: An observational analysis of a 60 patient cohort. Front Immunol (2021) 12:811473. doi: 10.3389/fimmu.2021.811473
44. Wang F, Liu R, Yang J, Chen B. New insights into genetic characteristics between multiple myeloma and COVID-19: An integrative bioinformatics analysis of gene expression omnibus microarray and the cancer genome atlas data. Int J Lab Hematol (2021) 43(6):1325–33. doi: 10.1111/ijlh.13717
45. Blimark C, Holmberg E, Mellqvist U-H, Landgren O, Björkholm M, Hultcrantz M, et al. Multiple myeloma and infections: a population-based study on 9253 multiple myeloma patients. Haematologica (2015) 100(1):107–13. doi: 10.3324/haematol.2014.107714
46. Engelhardt M, Shoumariyeh K, Rösner A, Ihorst G, Biavasco F, Meckel K, et al. Clinical characteristics and outcome of multiple myeloma patients with concomitant COVID-19 at comprehensive cancer centers in Germany. Haematologica (2020) 105(12):2872–8. doi: 10.3324/haematol.2020.262758
47. Martínez-López J, Mateos M-V, Encinas C, Sureda A, Hernández-Rivas JÁ, Lopez de la Guía A, et al. Multiple myeloma and SARS-CoV-2 infection: clinical characteristics and prognostic factors of inpatient mortality. Blood Cancer J (2020) 10(10):103. doi: 10.1038/s41408-020-00372-5
48. Li W, Yu R, Ma B, Yang Y, Jiao X, Liu Y, et al. Core fucosylation of IgG b cell receptor is required for antigen recognition and antibody production. J Immunol (Baltimore Md 1950) (2015) 194(6):2596–606. doi: 10.4049/jimmunol.1402678
49. Zahid D, Zhang N, Fang H, Gu J, Li M, Li W. Loss of core fucosylation suppressed the humoral immune response in salmonella typhimurium infected mice. J Microbiol Immunol Infect (2021) 54(4):606–15. doi: 10.1016/j.jmii.2020.02.006
50. Wahl A, van den Akker E, Klaric L, Štambuk J, Benedetti E, Plomp R, et al. Genome-wide association study on immunoglobulin G glycosylation patterns. Front Immunol (2018) 9:277. doi: 10.3389/fimmu.2018.00277
51. Klarić L, Tsepilov YA, Stanton CM, Mangino M, Sikka TT, Esko T, et al. Glycosylation of immunoglobulin G is regulated by a large network of genes pleiotropic with inflammatory diseases. Sci Adv (2020) 6(8):eaax0301. doi: 10.1126/sciadv.aax0301
52. Bernardinelli L, Murgia SB, Bitti PP, Foco L, Ferrai R, Musu L, et al. Association between the ACCN1 gene and multiple sclerosis in central East Sardinia. PloS One (2007) 2(5):e480. doi: 10.1371/journal.pone.0000480
53. Fazia T, Pastorino R, Notartomaso S, Busceti C, Imbriglio T, Cannella M, et al. Acid sensing ion channel 2: A new potential player in the pathophysiology of multiple sclerosis. Eur J Neurosci (2019) 49(10):1233–43. doi: 10.1111/ejn.14302
54. Richardson S, Hirsch JS, Narasimhan M, Crawford JM, McGinn T, Davidson KW, et al. Presenting characteristics, comorbidities, and outcomes among 5700 patients hospitalized with COVID-19 in the new York city area. JAMA (2020) 323(20):2052–9. doi: 10.1001/jama.2020.6775
55. Rao S, Baranova A, Cao H, Chen J, Zhang X, Zhang F. Genetic mechanisms of COVID-19 and its association with smoking and alcohol consumption. Brief Bioinform (2021) 22(6): bbab284. doi: 10.1093/bib/bbab284
56. Cao H, Baranova A, Wei X, Wang C, Zhang F. Bidirectional causal associations between type 2 diabetes and COVID-19. J Med Virol (2022). doi: 10.1002/jmv.28100
57. Baranova A, Cao H, Zhang F. Causal effect of COVID-19 on alzheimer's disease: A mendelian randomization study. J Med virol (2022). doi: 10.1002/jmv.28107
Keywords: COVID-19, SARS-CoV-2, vaccine, antibody, SNP, GWAS
Citation: Li P, Shi D, Shen W, Shi S, Guo X, Li J, Xu S, Zhang Y and Zhao Z (2022) Pilot genome-wide association study of antibody response to inactivated SARS-CoV-2 vaccines. Front. Immunol. 13:1054147. doi: 10.3389/fimmu.2022.1054147
Received: 26 September 2022; Accepted: 26 October 2022;
Published: 14 November 2022.
Edited by:
Mahbuba Rahman, McMaster University, CanadaReviewed by:
Fuquan Zhang, Nanjing Medical University, ChinaFirdausi Qadri, International Centre for Diarrhoeal Disease Research (ICDDR), Bangladesh
Copyright © 2022 Li, Shi, Shen, Shi, Guo, Li, Xu, Zhang and Zhao. This is an open-access article distributed under the terms of the Creative Commons Attribution License (CC BY). The use, distribution or reproduction in other forums is permitted, provided the original author(s) and the copyright owner(s) are credited and that the original publication in this journal is cited, in accordance with accepted academic practice. No use, distribution or reproduction is permitted which does not comply with these terms.
*Correspondence: Sihong Xu, eHVzaG9uZ0BuaWZkYy5vcmcuY24=; Yan Zhang, emFueTE5ODNAMTYzLmNvbQ==; Zhihu Zhao, emhhb3poQGJtaS5hYy5jbg==
†These authors have contributed equally to this work and share first authorship