- 1Department of Respiratory Medicine and Critical Care, Beijing Hospital, National Center of Gerontology, Institute of Geriatric Medicine, Chinese Academy of Medical Sciences, Beijing, China
- 2Graduate School of Peking Union Medical College, Beijing, China
- 3Department of Respiratory and Critical Care Medicine, Shandong Provincial Hospital Affiliated to Shandong First Medical University, Jinan, Shandong, China
- 4Department of Respiratory & Critical Care Medicine, The Second Hospital, Cheeloo College of Medicine, Shandong University, Jinan, Shandong, China
Background: Idiopathic pulmonary fibrosis (IPF) is a chronic progressive interstitial lung disease. Many studies suggest that autophagy may be related to disease progression and prognosis in IPF. However, the mechanisms involved have not been fully elucidated.
Methods: We incorporated 232 autophagy-associated genes (AAGs) and two datasets, GSE28042 and GSE27957, from the GEO database. Univariate Cox analysis and least absolute shrinkage and selection operator (LASSO) regression were used to construct the autophagy-associated prognostic model. Gene Ontology (GO) and Kyoto Encyclopedia of Genes and Genomes (KEGG) analyses were performed to investigate the functions of these autophagy-associated genes. CIBERSORT algorithm was used to calculate the immune cell infiltration between patients in the high-risk score and low-risk score groups. Quantitative Real-Time Polymerase Chain Reaction (qRT-PCR) was performed to explore the mRNA expression of five genes in the autophagy-associated risk model.
Results: We constructed a 5-autophagy-associated genes signature based on Univariate Cox analysis and LASSO regression. In our autophagy-associated risk model, IPF patients in the high-risk group demonstrated a poor overall survival rate compared to patients in the low-risk group. For 1-, 2-, and 3-year survival rates, the AUC predictive value of the AAG signature was 0.670, 0.787, and 0.864, respectively. These results were validated in the GSE27957 cohort, confirming the good prognostic effect of our model. GO and KEGG pathway analyses enriched immune-related pathways between the high-risk and low-risk groups. And there was also a significant difference in immune cell infiltration between two groups. And the results of qRT-PCR showed that the expression levels of FOXO1, IRGM, MYC, and PRKCQ were significantly decreased in the Peripheral Blood Mononuclear Cell (PBMC) of IPF patient samples.
Conclusion: Our study constructed and validated an autophagy-associated risk model based on MYC, MAPK1, IRGM, PRKCQ, and FOXO1. And those five genes may influence the progression of IPF by regulating immune responses and immune cells.
Introduction
Idiopathic pulmonary fibrosis (IPF) is a chronic progressive interstitial lung disease (1). IPF occurs most often in middle-aged and elderly people and is associated with progressive cough and dyspnea and reduced quality of life (2). IPF patients have a very poor prognosis, with a median survival of approximately 2-3 years after diagnosis (3). Despite the growing research on IPF, the cause of IPF is unknown and the pathogenesis involves multiple factors, including aging, environmental exposure, smoking, and chronic viral infections (1, 4, 5). Lung transplantation is currently the only treatment for IPF that can reverse the disease process of IPF. However, the limited source of donors, the high cost of the procedure, and the high number of IPF patients with comorbid underlying diseases are factors that limit the use of lung transplantation in the treatment of IPF patients (6). Pirfenidone and nintedanib have been recommended by guidelines for the treatment of IPF (6). Although pirfenidone and nintedanib delayed the decline in lung function and improved progression-free survival in IPF patients, they did not significantly improve overall mortality and acute exacerbations in IPF patients (7–9). Therefore, it is important to explore new molecular mechanisms of IPF pathogenesis and to construct new predictive models related to IPF prognosis.
Autophagy is a cellular degradation and recycling process that is highly conserved in eukaryotes (10). In mammalian cells, there are three major types of autophagy: microautophagy, macroautophagy, and chaperone-mediated autophagy (CMA). Although each is morphologically distinct, all three ultimately transport cargo to lysosomes for degradation and recycling (11). Autophagy plays an important role in cell survival and maintenance by degrading organelles, proteins, and macromolecules in the cytoplasm, as well as recycling degradation products. However, uncontrolled autophagy can lead to apoptosis. Therefore, dysfunction of this process can lead to the development of a variety of diseases (12, 13). Autophagy also exhibits an important role in IPF. Autophagy can slow down the pathological progression of IPF by regulating apoptosis of fibroblasts and senescence of alveolar epithelial cells (14, 15). In lung epithelial cells and lung fibroblasts from IPF patients, autophagy-associated pathways (including macroautophagy and mitophagy) were attenuated in both IPF mouse models and fibroblasts activated with TGFβ1 (16).IL-37 can reduce the progression of IPF by inhibiting TGF-β1 signaling and enhancing IPF fibroblast autophagy to attenuate lung fibrosis in mice (17). However, excessive autophagy of macrophages can promote the proliferation and migration of fibroblasts and exacerbate the progression of silicotic fibrosis (18). Autophagy is involved in the generation of inflammation and fibrosis formation in lung tissue by regulating neutrophil extracellular traps (19). All these studies suggest that autophagy may be related to disease progression and prognosis in IPF. However, strong evidence for the correlation between autophagy and IPF prognosis is still lacking. Therefore, it is crucial to construct a prognostic model of IPF with autophagy-associated genes.
In this study, we constructed an autophagy-associated genetic prognostic model for IPF using univariate Cox analysis and least absolute shrinkage and selection operator (LASSO). Subsequently, we validated the model using a cohort of IPF patients from the GEO database and a cohort of collected IPF patients. Finally, we explored the relationship between the autophagy risk model and inflammatory cells. Our study contributes to the understanding of the link between autophagy and IPF prognosis. Autophagy-associated genes may be potential therapeutic targets for IPF patients.
Methods
Data acquisition and processing
We downloaded 232 autophagy-associated genes (AAGs) from the Human Autophagy Database website (http://www.autophagy.lu/index.html). We downloaded two datasets, GSE28042 and GSE27957, from the GEO database. The GSE28042 dataset contains PBMC of 75 IPF patients and 19 normal controls. The GSE27957 dataset contains PBMC of 45 IPF patients. The basic information of the patients in GSE28042 and GSE27957 is shown in Table 1. The GSE28042 dataset is used as the training set and the GSE27957 dataset is used as the validation set. The principal component analysis (PCA) is used to evaluate the repeatability of the GSE28042 dataset. We used the “limma” package by R to identify differentially expressed genes (DEGs) between the IPF patient group and the control group according to p-values< 0.05 and absolute log2-fold changes > 0.2 in the GSE28042 dataset.
PPI and correlation analyses of autophagy-associated DEGs
The STRING database (https://string-db.org/) and Cytoscape software (version 3.9.0) were used to analyze the protein-protein interaction (PPI) among autophagy-associated DEGs.
Autophagy-Associated prognostic model construction
First, we selected genes in which DEGs and AAGs overlapped. Subsequently, univariate Cox analysis was performed on the duplicated AAGs to select genes associated with prognosis. To further narrow the AAGs scope, we performed the least absolute shrinkage and selection operator (LASSO) regression algorithm in the training set, 10-fold cross-validation was used, and the “glmnet” package by R was used for the analysis. The risk score publicized to predict the prognosis of IPF patients was calculated as follows:
where N is the number of selected prognostic AAGs, βi is the regression coefficient derived from the LASSO regression model coefficients, and Expi represented the gene expression level for the identified AAGs. We divided IPF cases into high-risk score group and low-risk score group based on the median risk score. Kaplan-Meier survival analysis was used to assess the relationship between overall survival and the AAGs correlation model. We used the time-dependent receiver operating characteristic (ROC) curves and the corresponding areas under the curve (AUC) values for assessing the prognostic value of the AAGs-related risk model. The prognostic model was evaluated using the “survminer” and “timeROC” packages by R.
To predict the prognosis of IPF patients, we used the training set to create a nomogram predicting 2-year and 3-year survival rates for IPF patients using the “rms” package by R. Subsequently, we used the calibration curve to evaluate the predictive efficiency of the nomogram.
Functional enrichment analysis
To explore the molecular mechanisms and pathways that are different between patients with high and low-risk scores, we performed Gene Ontology (GO) and Kyoto Encyclopedia of Genes and Genomes (KEGG) analysis using the “clusterProfiler” package by R.
Immune infiltration analysis
We used the CIBERSORT algorithm to calculate the immune cell infiltration between patients in the high-risk score and low-risk score groups. CIBERSORT can calculate the composition and score of 22 immune cell subpopulations in clinical samples by gene expression profiling (20).
Validation of the prognostic model
We used data of 45 IPF patients from GSE27957 to validate the accuracy of the AAGs-related risk model’s predictive ability for IPF prognosis. We also used qRT-PCR to validate the expression levels of five genes in our prognostic model. We collected PBMC samples from IPF patients attending the Second Hospital of Shandong University from January 2022 to June 2022. All IPF patients were diagnosed by high-resolution computed tomography (HRCT) and met the official ATS/ERS/JRS/ALAT clinical practice guideline for 2022 (21). Eleven IPF patients and six healthy individuals of similar age were included in our validation cohort.
PBMC obtain, RNA extraction and quantitative real-time polymerase chain reaction
First, we added patient or control blood on the top of the lymphocyte separation solution slowly. After centrifugation at 700g for 30 min, the PBMC layer in the middle of the liquid was carefully aspirated. After resuspension of PBMC using PBS, we added Red Blood Cell (RBC) lysis buffer and leave at room temperature for 5 min. And we obtain the PBMC after the centrifugation at 300g for 5min.We extracted total RNA from PBMC using TRIzol (Invitrogen). subsequently, we performed cDNA reverse transcription and qRT-PCR using RT reagent Kit (Takara). The primer sequence is summarized in Supplementary Data.
MRC-5 cell culture and western blotting
The Human lung fibroblast MRC-5 cells were obtained from Peking Union Medical College. MRC-5 cells were maintained in Minimum Essential Medium with 10% serum, and 1% penicillin and streptomycin. Cells were prepared for the next western blotting analysis after incubation with 10 ng/ml TGF-β for 48 hours. The protein extraction and western blotting experiments were carried out using standard protocols.
Statistical analysis
We used R software (R x64 version 4.1.2) and GraphPad Prism 9 to analyze all the data. Statistical analysis for multiple group data was conducted using the Analysis of Variance (ANOVA) method followed by a Student t-test or Wilcoxon rank-sum test for the comparison between two groups. We considered the p-value<0.05 as statistically significant.
Results
Differential expression of autophagy-associated genes
GSE28042 and GSE27957 datasets were normalized (Figure 1A). The result of PCA showed that GSE28042 had a good data repeatability (Figure 1B). We identified 5368 differentially expressed genes in the cohort of GSE28042 in the GEO database, of which 2342 genes were up-regulated and 3026 genes were down-regulated (Figure 1C). Based on 232 autophagy-associated genes, we identified 208 autophagy-associated genes in the expression array of GSE28042. We obtained 71 genes by taking the intersection of 5368 differentially expressed genes with 208 autophagy-associated genes (Figure 1D). The expression of 71 autophagy-associated genes in IPF patients and controls is displayed in the heatmap (Figure 1E). The PPI analysis demonstrated the interrelationship between autophagy-associated DEGs. The PPI network contained 64 nodes and 367 edges (Figure 1F).
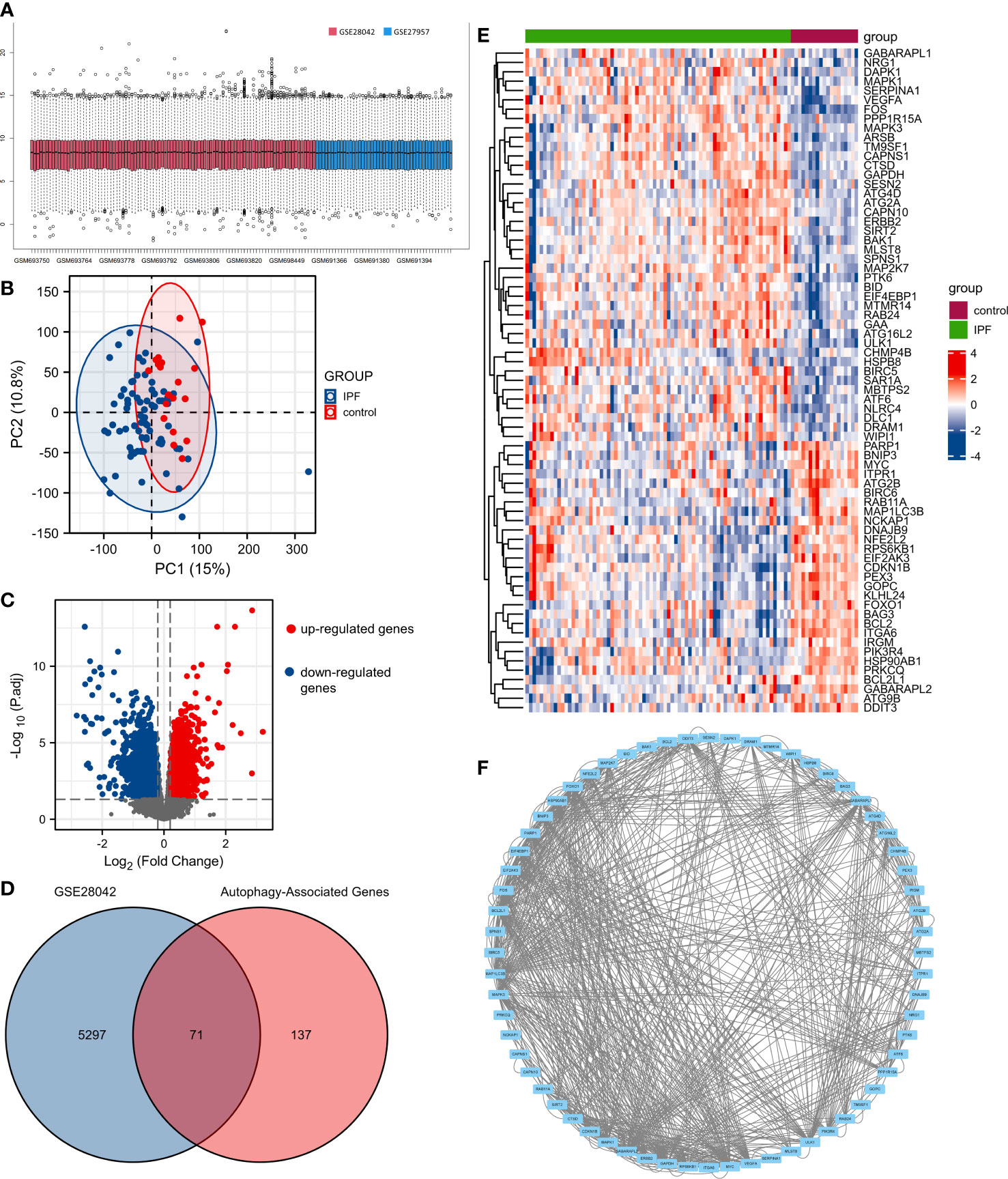
Figure 1 The differentially expressed autophagy-associated genes (AAGs) in idiopathic pulmonary fibrosis. (A) Data normalization of GSE28042 and GSE27957. (B) The principal component analysis (PCA) of GSE28042 dataset. (C) Volcano plot of differentially expressed genes in the cohort of GSE28042. (D) Intersection of 5368 differentially expressed genes with 208 autophagy-associated genes. (E) Heatmap of 71 autophagy-associated genes in IPF patients and controls. (F) The PPI network of autophagy-associated DEGs.
Identification of prognostic risk model of autophagy-associated genes in IPF
We identified 11 genes associated with IPF prognosis from 71 autophagy-associated differential genes using univariate Cox analysis. These 11 genes can be considered prognostic marker genes for IPF (BAG3, BCL2, BNIP3, EIF4EBP1, FOXO1, IRGM, ITGA6, MAPK1, MYC, PEX3, PRKCQ) (Figure 2A). We used LASSO regression analysis to further reduce the number of genes in the signature. We constructed a 5-autophagy-associated genes signature based on the optimal value of λ (Figures 2B, C).
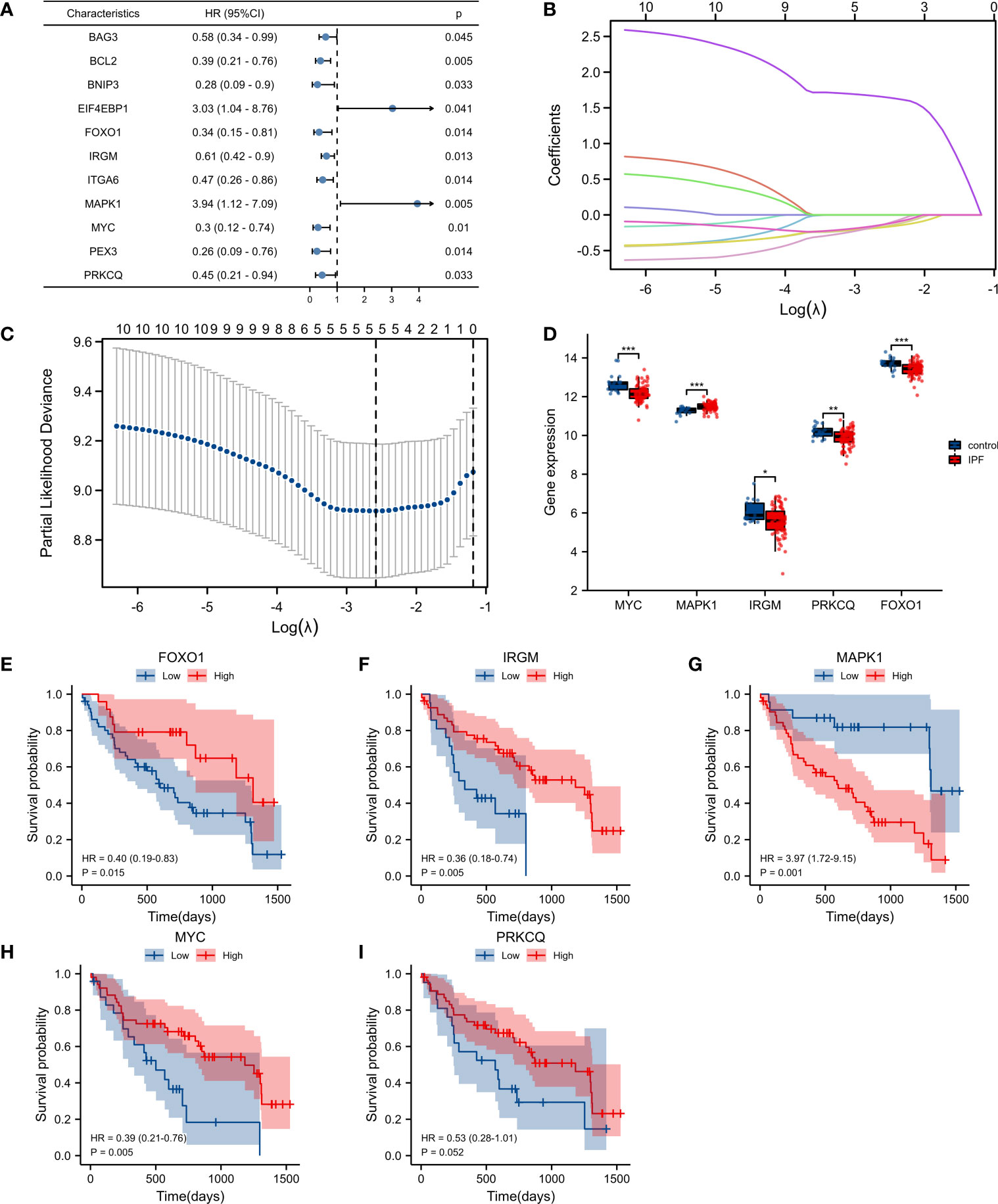
Figure 2 Construction of the autophagy-associated prognostic model. (A) Univariate Cox analysis identified genes related to the prognosis of IPF patients. (B, C) Screening of characteristic genes by LASSO regression analysis. (D) Differences of five genes in GSE28042. (E–I) Survival analysis of FOXO1, IRGM, MAPK1, MYC, and PRKCQ. *p< 0.05, **p< 0.01, and ***p< 0.001.
The risk score was calculated as follows: risk score =MAPK1×1.651141668−FOXO1×0.162139386−IRGM×0.173049268−MYC×0.132639078−PRKCQ×0.133252635. Among the 5-autophagy-associated genes signature, MAPK1 expression was higher in IPF patients than in controls, and FOXO1, IRGM, MYC, and PRKCQ expression was lower than in controls (Figure 2D). Survival analysis showed that the expression of FOXO1, IRGM, MYC, and MAPK1 were able to predict the prognosis of IPF patients (Figures 2E–H). And the expression of PRKCQ was not able to predict the prognosis of IPF patients (Figure 2I).
The K-M survival curve showed a significant difference in survival between the high-risk score group and the low-risk score group (p< 0.001), and the survival rate was lower in the high-risk group (Figure 3A). For 1-, 2-, and 3-year survival rates, the AUC predictive value of the AAG signature was 0.670, 0.787, and 0.864, respectively (Figure 3B). The risk map distribution and survival status of IPF patients showed that patients with high-risk scores had significantly lower survival times than patients with low-risk scores (Figure 3C). In addition, the heatmap of gene expression showed that MAPK1 was highly expressed in the high-risk score group, while FOXO1, IRGM, MYC, and PRKCQ were more highly expressed in the low-risk score group (Figure 3C). Univariate and multivariate cox analysis have shown that riskscore can be used as an independent and valid indicator to assess the prognosis of IPF patients (Figures 3D, E).
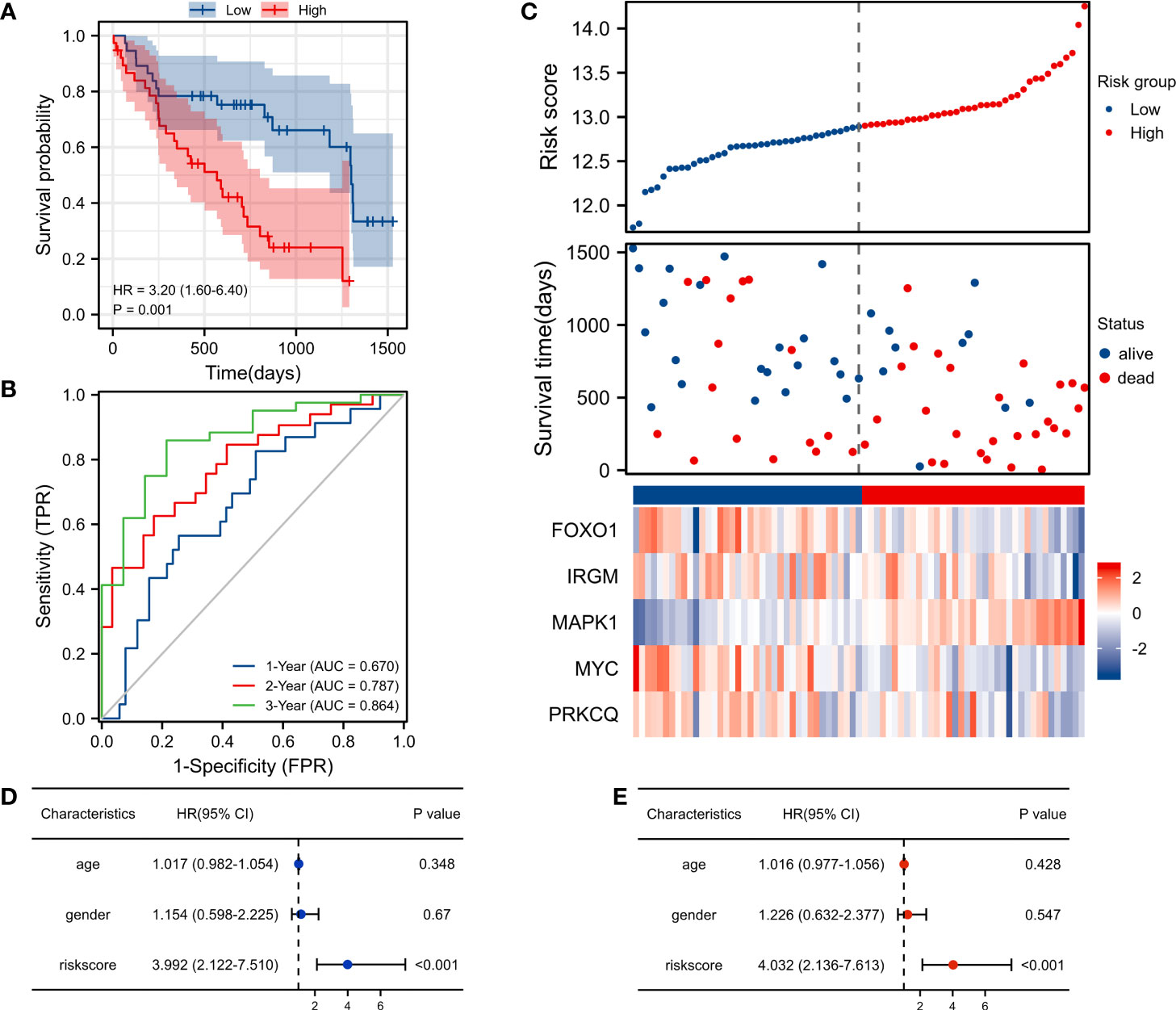
Figure 3 Risk score analysis of the autophagy-associated prognostic model in the GSE28042 training cohort. (A) Survival curve of high-risk score and low-risk score groups. (B) ROC curves evaluated the efficiency for predicting 1-, 2-, and 3-year survival. (C) Risk plot distribution, survival status, and heatmap of five genes expression. (D) Univariate cox analysis of riskscore in GSE28042. (E) Multivariate cox analysis of riskscore in GSE28042.
Validation of the autophagy-associated prognostic model
We included 45 patients with IPF from the GSE27957 database in the validation set cohort. In the validation cohort, Kaplan-Meier survival analysis showed that patients in the high-risk group had a shorter OS than those in the low-risk group(p<0.001) (Figure 4A). The AUC values for the 1-, 2-, and 3-year ROC curves in the validation cohort were 0.742, 0.729, and 0.634 respectively (Figure 4B). In the validation cohort, patients with IPF were also divided into the high-risk score group and the low-risk score group. Patients with high-risk scores had a worse survival status compared to patients with low-risk scores. In addition, we also plotted a heatmap showing the expression of the 5 AAGs (Figure 4C). In the validation dataset, univariate and multivariate cox analysis have shown that riskscore can be used as an independent and valid indicator (Figures 4D, E).
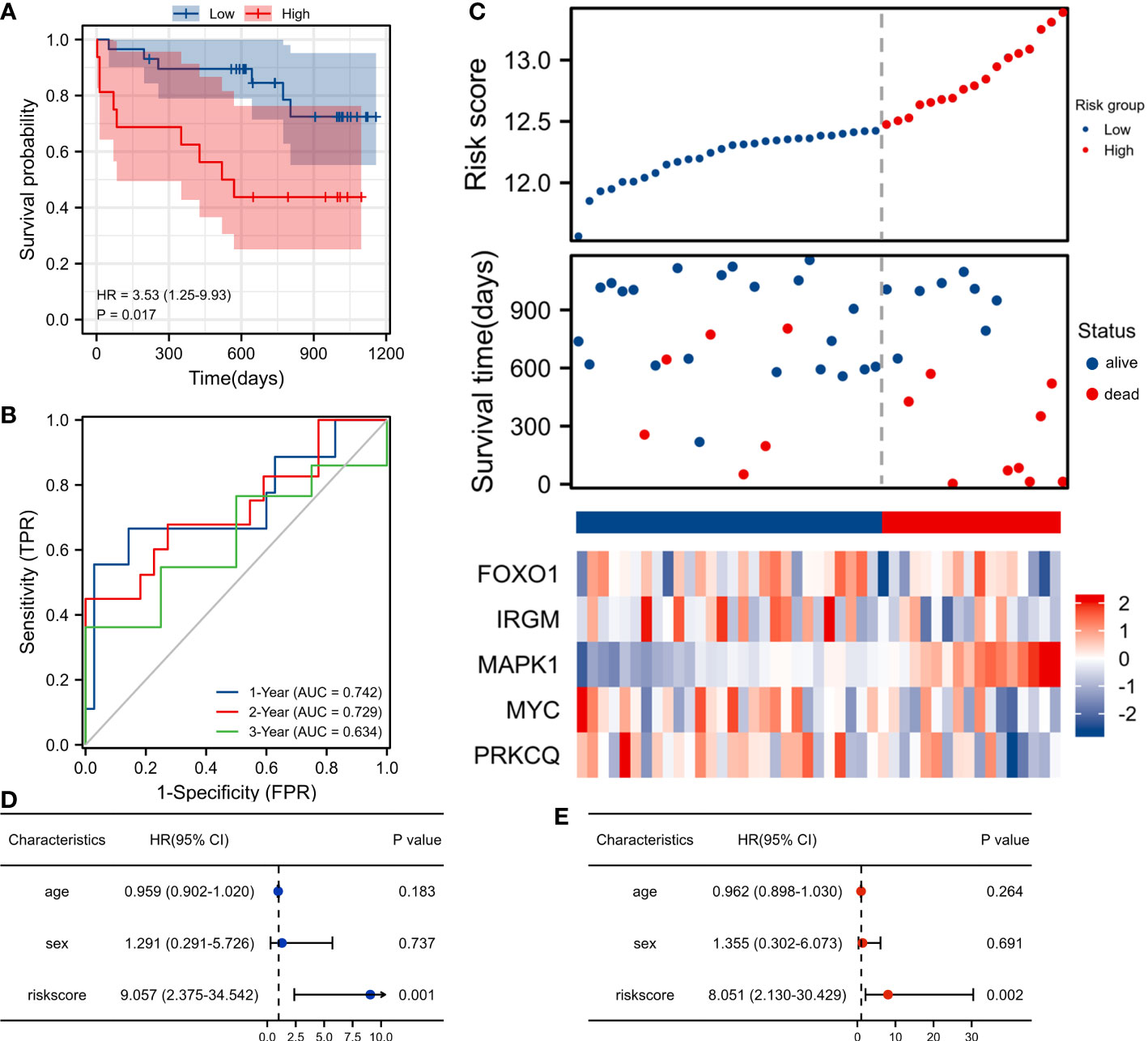
Figure 4 Validation of the autophagy-associated prognostic model in GSE27957. (A) Survival curve of high-risk score and low-risk score groups. (B) ROC curves evaluated the efficiency for predicting 1-, 2-, and 3-year survival. (C) Risk plot distribution, survival status, and heatmap of five genes expression. (D) Univariate cox analysis of riskscore in GSE27957. (E) Multivariate cox analysis of riskscore in GSE27957.
Construction of a nomogram based on five autophagy-associated genes
To establish a quantitative approach to the prognosis of IPF patients, we constructed a predictive nomogram based on age and five screened AAGs (Figure 5A). The calibration curve shows that the predicted values and true values are in good agreement (Figure 5B). Therefore, the nomogram based on age and five AAGs presented a good accuracy for predicting 2- and 3-year survival of IPF patients.
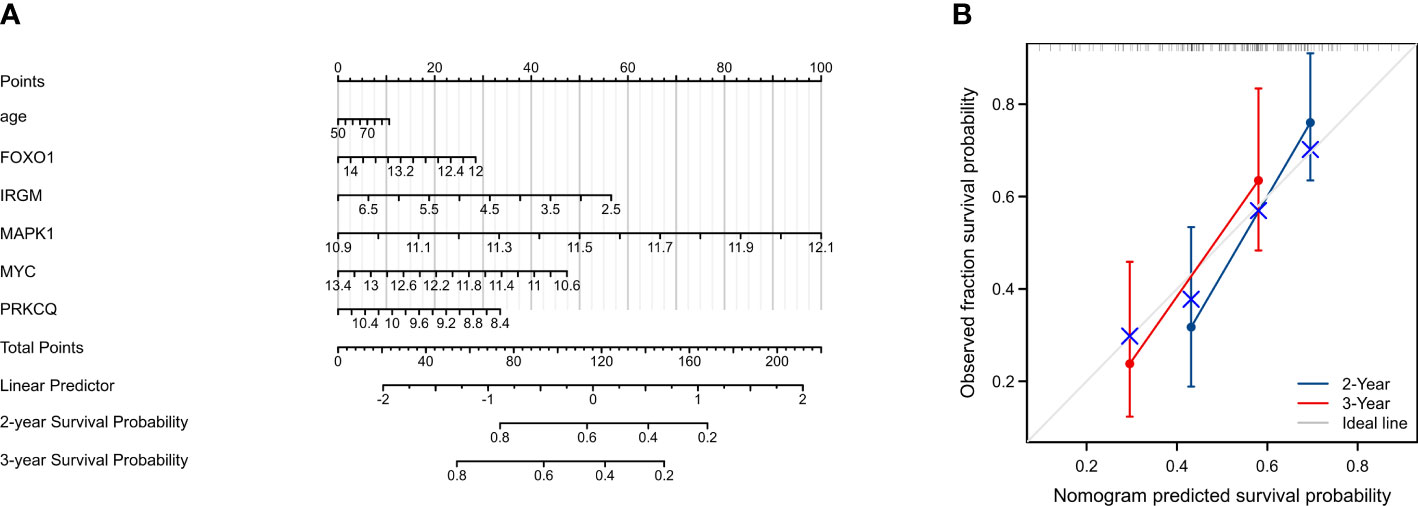
Figure 5 Construction and evaluation of a nomogram for predicting 2-, and 3-year overall survival rates of IPF patients. (A) Nomogram for predicting 2-, and 3-year overall survival of IPF patients. (B) Calibration curves showing the probability of 2-, and 3-year overall survival between the prediction and the observation.
Relationship between autophagy-associated prognostic model and immune cell infiltration in IPF
We calculated the difference in the level of infiltration of 22 immune cells between the high-risk score group and the low-risk score group using the CIBERSORT algorithm (Figure 6A). The IPF patients in the high-risk score group had lower T cells CD4 memory resting, T cells CD4 memory activated, T cells follicular helper, and T cells regulatory (Tregs), and had higher Monocytes and Mast cells resting than in the low-risk score group. Subsequently, we explored the relationship between the seven immune cells mentioned above and the five AAGs in the prognostic model. IPF patients with high FOXO1 expression had lower levels of Monocytes (Figure 6B). And IPF patients with high IRGM expression had higher levels of T cells CD4 memory resting (Figure 6C). IPF patients with high MAPK1 expression had lower levels of T cells CD4 memory resting and T cells CD4 memory activated, and higher Mast cells resting (Figure 6D). And IPF patients with high MYC expression had higher levels of T cells regulatory (Tregs) and lower Monocytes (Figure 6E). In addition, IPF patients with high PRKCQ expression had higher levels of T cells CD4 memory activated and T cells regulatory (Tregs), and lower Monocytes (Figure 6F). In addition, we provided the correlation analysis between autophagy-associated genes and specific immune cells. All correlations between five DEGs and specific immune cells were statistically significant, except for the correlation between PRKCQ and T cells regulatory (Tregs), which was not statistically significant (Figures 6G–P).
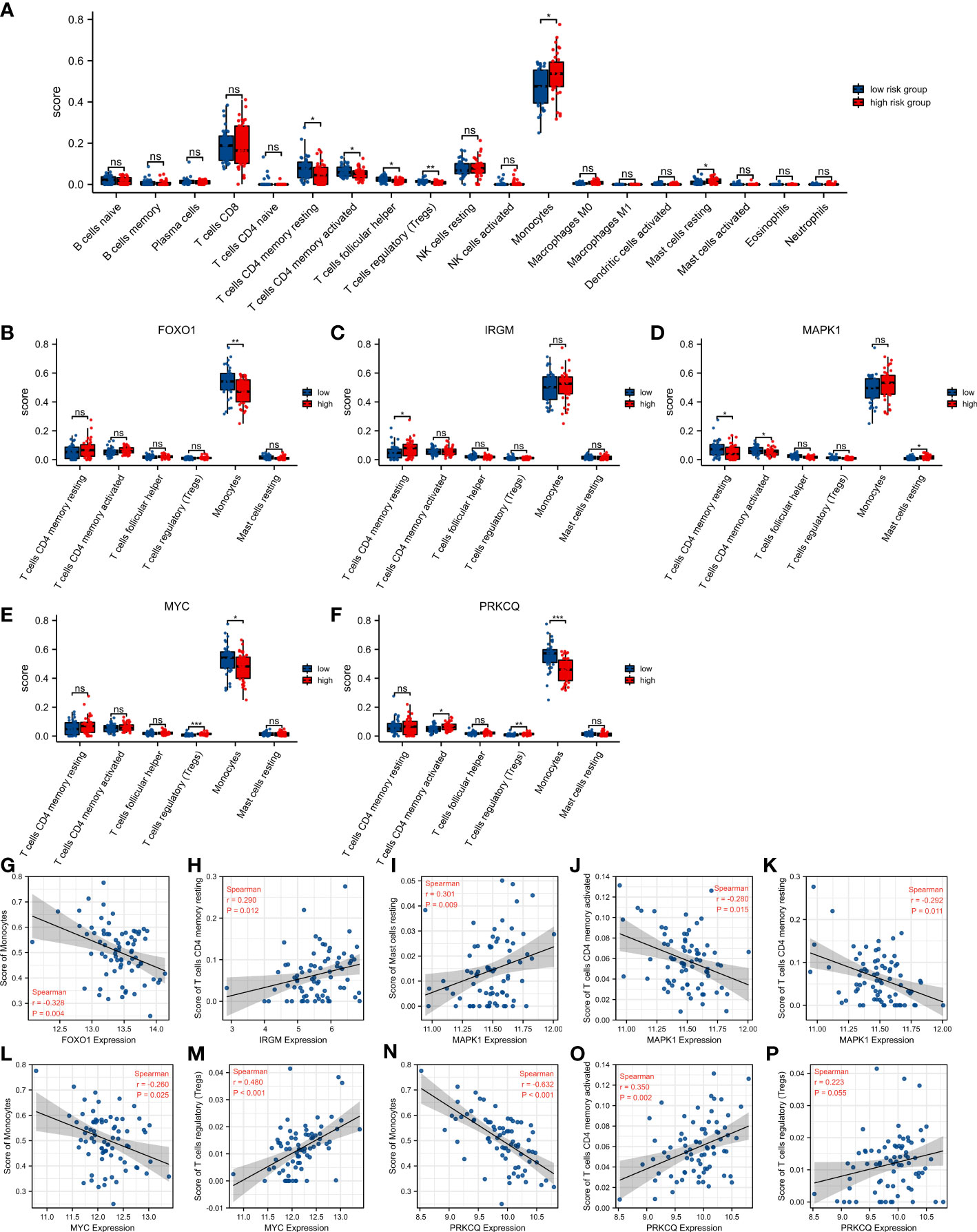
Figure 6 Relationship between autophagy-associated prognostic model and immune cell infiltration in IPF. (A) The difference of 22 immune cells between the high-risk score group and the low-risk score group. (B–F) Comparison of infiltration levels of 6 immune cells according to FOXO1 (B), IRGM (C), MAPK1 (D), MYC (E), and PRKCQ (F) expression levels. (G–P) The correlation analysis between autophagy-associated genes and specific immune cells. *p< 0.05, **p< 0.01, ***p< 0.001, and ns, no significance.
Functional enrichment analysis of autophagy-associated prognostic model
To reveal potential biological functions and pathways associated with the autophagy-associated prognostic model, we analyzed differential genes between high-risk and low-risk score groups using GO enrichment and the KEGG pathway analyses. GO enrichment revealed immune-related pathways, such as neutrophil activation, neutrophil mediated immunity, and T cell activation (Figure 7A). And KEGG pathway analyses were also enriched to inflammation-related pathways, such as T cell receptor signaling pathway, B cell receptor signaling pathway, and NF-kappa B signaling pathway (Figure 7B).
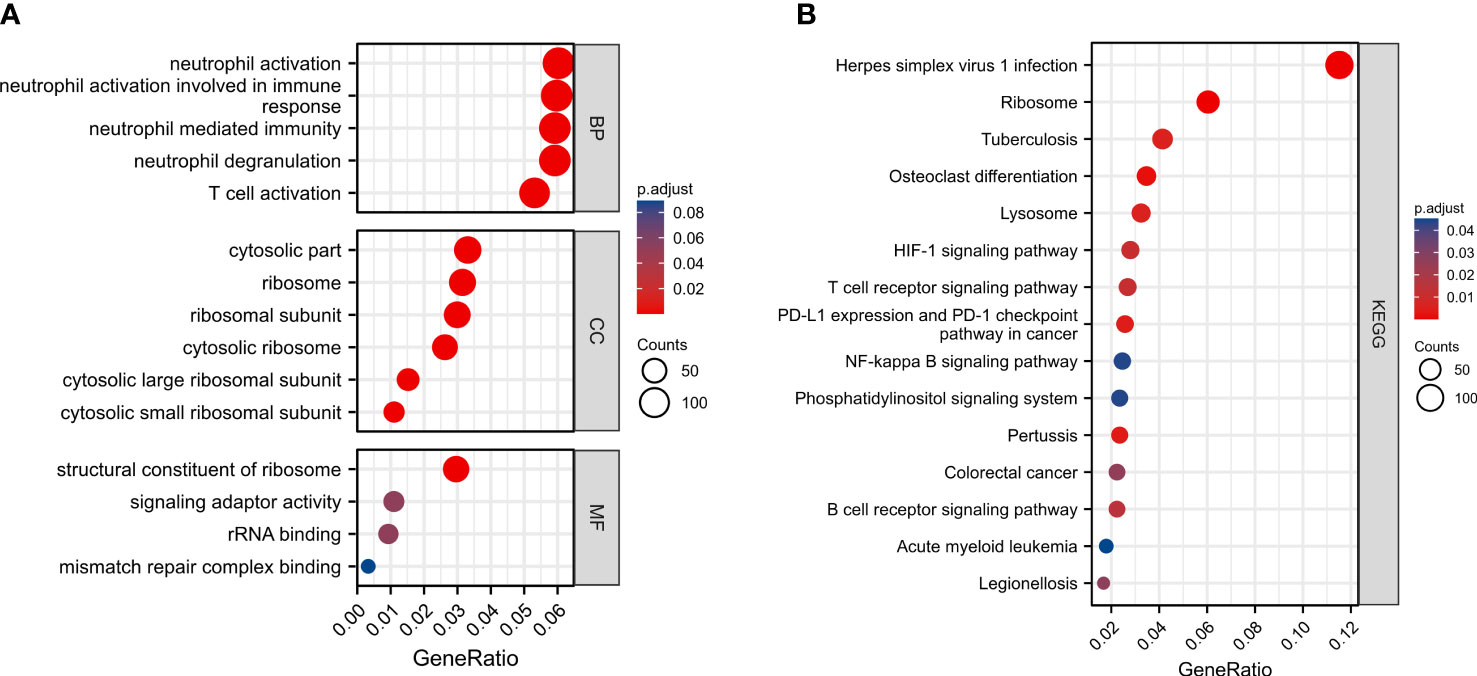
Figure 7 Functional enrichment analysis of autophagy-associated prognostic model between high-risk and low-risk score groups. (A) GO enrichment. (B) KEGG pathway analyses.
Verification of the differentially expressed autophagy-associated genes in clinical samples and cellular models
To further validate the reliability of the autophagy-associated prognostic model, we used clinical samples to further analyze the expression of five autophagy-associated genes. The results of qRT-PCR showed that the expression levels of FOXO1, IRGM, MYC, and PRKCQ were significantly decreased in IPF PBMC samples than in normal PBMC samples. However, MAPK1 expression was not significantly different between the IPF and normal groups (Figure 8A). We used western blotting to assay the expression levels of 5 proteins in the autophagy-associated prognostic model. Elevated α-SMA expression demonstrates the success of the IPF cell model. The expression of FOXO1, IRGM, c-MYC, and PRKCQ was significantly lower in the TGF-β stimulated MRC-5 cell. And p38 MAPK was upregulated after stimulated by TGF-β (Figure 8B).
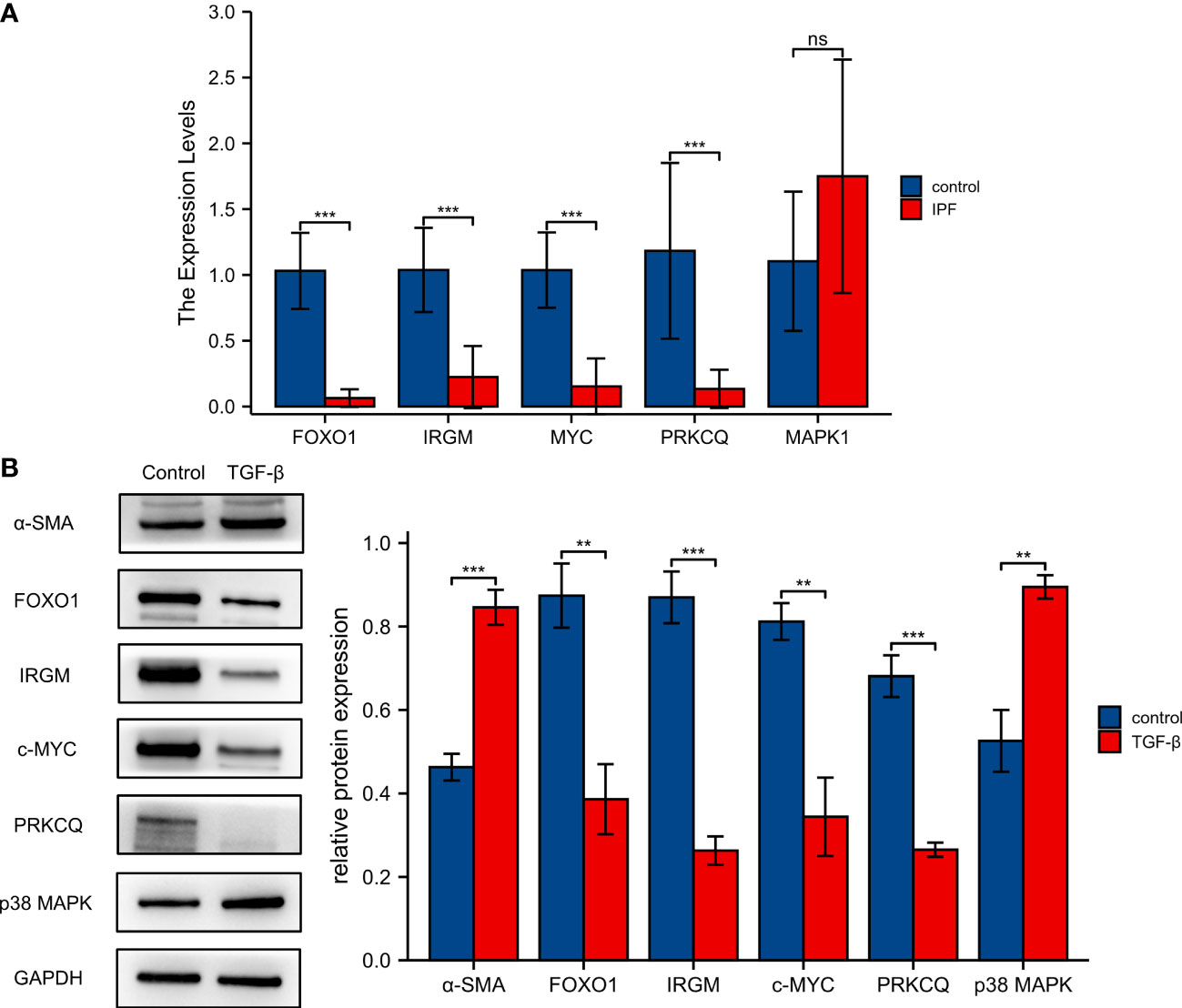
Figure 8 Validation of five screened autophagy-associated genes. (A)Validation of mRNA expression levels of FOXO1, IRGM, MYC, PRKCQ, and MAPK1 with qRT-PCR. (B) The protein expression of FOXO1, IRGM, MAPK1, MYC, and PRKCQ was detected by western blotting. **p< 0.01, ***p< 0.001, and ns, no significance.
Discussion
IPF is a highly heterogeneous disease, and the natural history of IPF patients is highly variable. Some patients with IPF deteriorate rapidly, while others have a slow progression (22, 23). It is difficult to predict the course and prognosis of individual patients (24). Over some time, several studies have been performed using clinical, imaging, and pathological indicators to predict progression and survival in IPF (25, 26). However, due to the retrospective nature of the studies and the uncertainty of the metrics, the accuracy of the predictors in these studies is various (24). Therefore, the development of appropriate and accurate prognostic models for IPF is urgent for clinical work.
In line with our study, the study by Huang et al. also constructed a prognostic model of autophagy-associated genes, which proved to be effective in predicting the prognosis of IPF patients (27). In our study, we used two GEO datasets to establish and validate our risk score prognostic model. And the samples were PMBC from IPF patients. In addition, we use the Human Autophagy Database website to determine the autophagy-associated genes. In our study, we first identified autophagy-associated genes associated with the prognosis of IPF patients. At first, we identified 11 autophagy-associated genes associated with IPF prognosis. Subsequently, we constructed an autophagy-associated prognostic model consisting of five genes using LASSO regression analysis. K-M survival curves and ROC curves demonstrated that the prediction model had good predictive power. Also, the K-M survival curves and ROC curves demonstrated that the model also showed excellent discrimination in the validation dataset. After that, we constructed a nomogram based on the expression of FOXO1, MAPK1, IRGM, MYC, and PRKCQ to predict the IPF patient prognosis directly.
MYC, also called c-Myc, is one of the major transcription factors involved in genomic regulation. MYC is involved in the regulation of fundamental biological processes such as cell proliferation, differentiation, metabolism, and apoptosis (28–30). MYC is involved in autophagosome formation in the early stages of autophagy, and MYC regulates autophagy through the JNK1-Bcl2 pathway and ROS. In contrast, MYC inhibition leads to defective autophagosome formation, which results in a decrease in autophagy (31). Aberrant activation of MYC in cancer has been extensively studied (32). In endothelial cells, downregulation of MYC activates a pro-inflammatory senescence phenotype and can act as a regulator of inflammation and endothelial dysfunction (33, 34). The expression of MYC in endothelial cells is protective in diet-induced liver inflammation and fibrosis (35). In a TGF-β-induced lung fibrosis model in rats, TGF-β inhibited cell proliferation by suppressing MYC expression (36). In addition, in a study on IPF patients, MYC was significantly lower in alveolar epithelial type II cells of IPF patients compared to controls (37). In our study, MYC expression was decreased in IPF patients and was seen as a protective factor in the risk model. Thus, the downregulation of MYC may play an important role in the development of IPF.
Mitogen-activated protein kinase 1(MAPK1), also known as extracellular signal-regulated kinases (ERKs), is an integration point for multiple signaling pathways (38). MAPK1 is involved in a variety of biological processes that including cell proliferation, cell adhesion, cell migration, cell differentiation, metabolism, and transcription (39–41).MAP kinases are activated after phosphorylation of upstream kinases, which translocate to the nucleus after phosphorylation to activate a series of transcription factors, synthesize effector proteins and achieve corresponding changes in cellular state (40). In a study of emphysema, ERK activity was significantly increased in the lung tissue of patients compared to control subjects (42). And the activation of ERKs was closely associated with emphysema-related airway inflammation (43). In the BLM-induced lung fibrosis model, the phosphorylation level of ERK1/2 protein was elevated (44). And inhibition of ERK1/2 signaling attenuated BLM pulmonary fibrosis by inhibiting EMT (45). The increase of p-ERK1/2 and downstream-related targets was also observed in the lung tissue of IPF patients (46). In the autophagy-associated prognostic model, elevated MAPK1 levels were associated with a worse prognosis in IPF. Thus, our study further confirms the role of MAPK1 in promoting IPF progression.
IRGM1 is a member of the interferon-inducible GTPases (IRGs) family, which is one of the strongest early resistance systems in cells (47). In inflammatory and infectious diseases, IRGM1 is a core protein that promotes autophagic clearance of microorganisms and anti-inflammation (48, 49). In IRGM1-depleted cells, the cGAS-STING and RIG-I-MAVS signaling pathways were activated, which in turn increased the level of IFN responses (50). In LPS-stimulated murine macrophages, IRGM1 was able to negatively regulate TLR4 signaling and prevent the overproduction of pro-inflammatory cytokines (51). In addition, IRGM1-/- mice exhibited lymphocytic infiltration in a variety of mucosal tissues, including lung tissue (52). In genome-wide association studies, mutations in IRGM were strongly associated with susceptibility to Crohn’s disease and tuberculosis (53, 54). Thus, decreased IRGM1 may play an important role in IPF by promoting autophagy and inflammatory responses.
Protein kinase C theta (PRKCQ) is a serine/threonine kinase belonging to the PKC subfamily (55). Ca2+-dependent activation of PRKCQ is required in endoplasmic reticulum stress-induced autophagy (56). In the immune system, dysfunction of PRKCQ leads to the development of would autoimmune and inflammatory diseases (57). PRKCQ is highly expressed in T cells and is required for T cell-mediated responses (57, 58). PRKCQ expression is also decreased in lung adenocarcinoma and renal cell carcinoma compared to controls (59, 60). In the LPS-induced acute lung injury model in mice, inhibition of PRKCQ attenuated lung injury in mice by inhibiting Th17 cell responses through the Notch signaling pathway (61). And Th17 cells were reported to promote lung inflammation and fibrosis in a mouse lung fibrosis model (62, 63). However, studies of PRKCQ in IPF patients have not been reported. In our study, PRKCQ expression was found to be downregulated in IPF tissues and was associated with poor prognosis in IPF.
Forkhead transcription factors (FOXOs) family is considered to be key factors in cell fate regulation (64, 65). Activated FOXOs promote the expression of a variety of target genes that regulate various cellular processes including autophagy, cell cycle, and apoptosis (66, 67). Among them, FOXO1/3 are the two most widely studied isoforms and play an important role in a variety of fibrotic diseases (68). Upregulation of FOXO1 can attenuate renal tubular interstitial fibrosis and apoptosis in diabetic nephropathy by inhibiting STAT1 (69). In hepatic fibrosis, FOXO1 inhibits the activation, transdifferentiation, and proliferation of hepatic stellate cells (68). Ginsenoside attenuates hepatic fibrosis injury and inflammation by increasing the nuclear transport of FOXO1 (70). In the fibroblasts of IPF patients, low levels of FOXO3 favored the reduction of fibroblast autophagic activity and the maintenance of cell viability (71). However, the role of FOXO1 in IPF has not been reported in studies. In autophagy-associated prognostic model, FOXO1 is considered a protective factor in IPF. Decreased FOXO1 may play an important role in the disease progression of IPF.
Differential gene function enrichment analysis between the high-risk and low-risk groups showed that neutrophil activation, neutrophil mediated immunity, and T cell activation are significantly activated in the high-risk group. Similarly, KEGG pathway analyses enriched immune-related pathways. And there was also a significant difference in immune cell infiltration between the high-risk and low-risk groups (T cells CD4 memory resting, T cells CD4 memory activated, T cells follicular helper, T cells regulatory, Monocytes and Mast cells resting). CD4 T cells may regulate pulmonary fibrosis in multiple ways (63, 72). Secretion of IFN-γ, IL-12, and TNF-α by Th1 cells was associated with attenuation of fibrosis (73–75). IL-4, IL-5, and IL-13 secreted by Th2 cells are also associated with the development of fibrosis (76, 77). Therefore, CD4 T cells can be anti-fibrotic or pro-fibrotic, depending on the state of the inflammatory environment (72). In the early stages of pulmonary fibrosis, T cells regulatory (Tregs) play a pro-fibrotic role by increasing TGF-β1 release and collagen deposition. While in the late stages of IPF, Tregs can inhibit the progression of pulmonary fibrosis (78). In the mouse model, Tregs reduced fibroplasia due to acute lung injury by decreasing fibroblast recruitment (79). Furthermore, an increase in the proportion of activated Tregs in the peripheral blood of IPF patients was negatively correlated with the severity of the disease (80). Monocytes play a key role in fibrogenesis through the release of pro-fibrotic inflammatory cytokines (63). Increased numbers of monocytes have also been shown to be associated with poor prognosis in IPF in several studies (81, 82). In addition, monocytes are progenitors of pro-fibrotic macrophages and fibroblasts (83, 84). Mast cells have a large amount of pro-fibrotic cytokines in their granules (85, 86). The number of mast cells was increased in the lung tissue of IPF patients, and the products of mast cells were increased in BALF (87, 88). In addition, mast cells may promote the fibrotic process by stimulating fibroblasts in the lung (89). Therefore, we hypothesize that genes in autophagy-associated prognostic model may be involved in the development of IPF by regulating these immune cells.
In conclusion, our study constructed and validated an autophagy-associated prognostic model based on MYC, MAPK1, IRGM, PRKCQ, and FOXO1. We also found that MYC, MAPK1, IRGM, PRKCQ, and FOXO1 may influence the progression of IPF by regulating immune responses and immune cells. In addition, the results of qRT-PCR showed that the expression of FOXO1, IRGM, MYC, and PRKCQ was decreased in PBMC of IPF patients compared to normal samples. The expression of FOXO1, IRGM, c-MYC, and PRKCQ was significantly lower and p38 MAPK was upregulated in the TGF-β stimulated MRC-5 cell. However, our study also has some limitations. First, we need to follow up in a larger sample size population to validate the accuracy of the autophagy-associated prognostic model. The small sample size may make the difference of MAPK1 between the IPF patient and control groups insignificant. Our study also did not include drug use in IPF patients. As a recommended drug for the treatment of IPF, the use of pirfenidone may inhibit MAPK1 gene expression (90). In addition, we need further functional and mechanistic experiments to explore the potential mechanisms underlying the role of autophagy-associated genes in IPF progression.
Data availability statement
The datasets presented in this study can be found in online repositories. The names of the repository/repositories and accession number(s) can be found below: https://www.ncbi.nlm.nih.gov/geo/, GSE28042 https://www.ncbi.nlm.nih.gov/geo/, GSE27957.
Ethics statement
The studies involving human participants were reviewed and approved by The second hospital of Shandong University Ethics Committee. The patients/participants provided their written informed consent to participate in this study.
Author contributions
ZY, FGQ and LJJ designed the entire study. FGQ, and LJJ completed the experiments. ZY and FGQ performed the data analysis and wrote the article. FGQ, LJJ, WZ and LCY collected the data and revised the manuscript. FGQ and LJJ contributed equally to this work and should be considered the first authors. All authors approved the final manuscript. All authors contributed to the article and approved the submitted version.
Conflict of interest
The authors declare that the research was conducted in the absence of any commercial or financial relationships that could be construed as a potential conflict of interest.
Publisher’s note
All claims expressed in this article are solely those of the authors and do not necessarily represent those of their affiliated organizations, or those of the publisher, the editors and the reviewers. Any product that may be evaluated in this article, or claim that may be made by its manufacturer, is not guaranteed or endorsed by the publisher.
Supplementary material
The Supplementary Material for this article can be found online at: https://www.frontiersin.org/articles/10.3389/fimmu.2022.1049361/full#supplementary-material
References
1. Martinez FJ, Collard HR, Pardo A, Raghu G, Richeldi L, Selman M, et al. Idiopathic pulmonary fibrosis. Nat Rev Dis Primers (2017) 3:17074. doi: 10.1038/nrdp.2017.74
2. Richeldi L, Collard HR, Jones MG. Idiopathic pulmonary fibrosis. Lancet (London England) (2017) 389(10082):1941–52. doi: 10.1016/s0140-6736(17)30866-8
3. Lederer DJ, Martinez FJ. Idiopathic pulmonary fibrosis. New Engl J Med (2018) 378(19):1811–23. doi: 10.1056/NEJMra1705751
4. Pardo A, Selman M. Lung fibroblasts, aging, and idiopathic pulmonary fibrosis. Ann Am Thorac Soc (2016) 13 Suppl 5:S417–s21. doi: 10.1513/AnnalsATS.201605-341AW
5. Ryu JH, Moua T, Daniels CE, Hartman TE, Yi ES, Utz JP, et al. Idiopathic pulmonary fibrosis: Evolving concepts. Mayo Clinic Proc (2014) 89(8):1130–42. doi: 10.1016/j.mayocp.2014.03.016
6. Raghu G, Rochwerg B, Zhang Y, Garcia CA, Azuma A, Behr J, et al. An official Ats/Ers/Jrs/Alat clinical practice guideline: Treatment of idiopathic pulmonary fibrosis. an update of the 2011 clinical practice guideline. Am J Respir Crit Care Med (2015) 192(2):e3–19. doi: 10.1164/rccm.201506-1063ST
7. Richeldi L, du Bois RM, Raghu G, Azuma A, Brown KK, Costabel U, et al. Efficacy and safety of nintedanib in idiopathic pulmonary fibrosis. New Engl J Med (2014) 370(22):2071–82. doi: 10.1056/NEJMoa1402584
8. King TE Jr., Bradford WZ, Castro-Bernardini S, Fagan EA, Glaspole I, Glassberg MK, et al. A phase 3 trial of pirfenidone in patients with idiopathic pulmonary fibrosis. New Engl J Med (2014) 370(22):2083–92. doi: 10.1056/NEJMoa1402582
9. Noble PW, Albera C, Bradford WZ, Costabel U, Glassberg MK, Kardatzke D, et al. Pirfenidone in patients with idiopathic pulmonary fibrosis (Capacity): Two randomised trials. Lancet (London England) (2011) 377(9779):1760–9. doi: 10.1016/s0140-6736(11)60405-4
10. Parzych KR, Klionsky DJ. An overview of autophagy: Morphology, mechanism, and regulation. Antioxidants Redox Signaling (2014) 20(3):460–73. doi: 10.1089/ars.2013.5371
11. Yang Z, Klionsky DJ. Mammalian autophagy: Core molecular machinery and signaling regulation. Curr Opin Cell Biol (2010) 22(2):124–31. doi: 10.1016/j.ceb.2009.11.014
12. Yorimitsu T, Klionsky DJ. Autophagy: Molecular machinery for self-eating. Cell Death differentiation (2005) 12 Suppl 2(Suppl 2):1542–52. doi: 10.1038/sj.cdd.4401765
13. Racanelli AC, Kikkers SA, Choi AMK, Cloonan SM. Autophagy and inflammation in chronic respiratory disease. Autophagy (2018) 14(2):221–32. doi: 10.1080/15548627.2017.1389823
14. Araya J, Kojima J, Takasaka N, Ito S, Fujii S, Hara H, et al. Insufficient autophagy in idiopathic pulmonary fibrosis. Am J Physiol Lung Cell Mol Physiol (2013) 304(1):L56–69. doi: 10.1152/ajplung.00213.2012
15. Kuwano K, Araya J, Hara H, Minagawa S, Takasaka N, Ito S, et al. Cellular senescence and autophagy in the pathogenesis of chronic obstructive pulmonary disease (Copd) and idiopathic pulmonary fibrosis (Ipf). Respir Invest (2016) 54(6):397–406. doi: 10.1016/j.resinv.2016.03.010
16. Patel AS, Lin L, Geyer A, Haspel JA, An CH, Cao J, et al. Autophagy in idiopathic pulmonary fibrosis. PloS One (2012) 7(7):e41394. doi: 10.1371/journal.pone.0041394
17. Kim MS, Baek AR, Lee JH, Jang AS, Kim DJ, Chin SS, et al. Il-37 attenuates lung fibrosis by inducing autophagy and regulating tgf-B1 production in mice. J Immunol (Baltimore Md 1950) (2019) 203(8):2265–75. doi: 10.4049/jimmunol.1801515
18. Liu H, Fang S, Wang W, Cheng Y, Zhang Y, Liao H, et al. Macrophage-derived Mcpip1 mediates silica-induced pulmonary fibrosis Via autophagy. Particle fibre Toxicol (2016) 13(1):55. doi: 10.1186/s12989-016-0167-z
19. Chrysanthopoulou A, Mitroulis I, Apostolidou E, Arelaki S, Mikroulis D, Konstantinidis T, et al. Neutrophil extracellular traps promote differentiation and function of fibroblasts. J Pathol (2014) 233(3):294–307. doi: 10.1002/path.4359
20. Chen B, Khodadoust MS, Liu CL, Newman AM, Alizadeh AA. Profiling tumor infiltrating immune cells with cibersort. Methods Mol Biol (Clifton NJ) (2018) 1711:243–59. doi: 10.1007/978-1-4939-7493-1_12
21. Raghu G, Remy-Jardin M, Richeldi L, Thomson CC, Inoue Y, Johkoh T, et al. Idiopathic pulmonary fibrosis (an update) and progressive pulmonary fibrosis in adults: An official Ats/Ers/Jrs/Alat clinical practice guideline. Am J Respir Crit Care Med (2022) 205(9):e18–47. doi: 10.1164/rccm.202202-0399ST
22. Kim DS, Collard HR, King TE Jr. Classification and natural history of the idiopathic interstitial pneumonias. Proc Am Thorac Soc (2006) 3(4):285–92. doi: 10.1513/pats.200601-005TK
23. Raghu G, Collard HR, Egan JJ, Martinez FJ, Behr J, Brown KK, et al. An official Ats/Ers/Jrs/Alat statement: Idiopathic pulmonary fibrosis: Evidence-based guidelines for diagnosis and management. Am J Respir Crit Care Med (2011) 183(6):788–824. doi: 10.1164/rccm.2009-040GL
24. Kim HJ, Perlman D, Tomic R. Natural history of idiopathic pulmonary fibrosis. Respir Med (2015) 109(6):661–70. doi: 10.1016/j.rmed.2015.02.002
25. Ley B, Collard HR, King TE Jr. Clinical course and prediction of survival in idiopathic pulmonary fibrosis. Am J Respir Crit Care Med (2011) 183(4):431–40. doi: 10.1164/rccm.201006-0894CI
26. Mura M, Porretta MA, Bargagli E, Sergiacomi G, Zompatori M, Sverzellati N, et al. Predicting survival in newly diagnosed idiopathic pulmonary fibrosis: A 3-year prospective study. Eur Respir J (2012) 40(1):101–9. doi: 10.1183/09031936.00106011
27. Huang G, Xu X, Ju C, Zhong N, He J, Tang XX. Identification and validation of autophagy-related gene expression for predicting prognosis in patients with idiopathic pulmonary fibrosis. Front Immunol (2022) 13:997138. doi: 10.3389/fimmu.2022.997138
28. Pelengaris S, Khan M. The many faces of c-myc. Arch Biochem biophysics (2003) 416(2):129–36. doi: 10.1016/s0003-9861(03)00294-7
29. Dang CV, Resar LM, Emison E, Kim S, Li Q, Prescott JE, et al. Function of the c-myc oncogenic transcription factor. Exp Cell Res (1999) 253(1):63–77. doi: 10.1006/excr.1999.4686
30. Bretones G, Delgado MD, León J. Myc and cell cycle control. Biochim Biophys Acta (2015) 1849(5):506–16. doi: 10.1016/j.bbagrm.2014.03.013
31. Toh PP, Luo S, Menzies FM, Raskó T, Wanker EE, Rubinsztein DC. Myc inhibition impairs autophagosome formation. Hum Mol Genet (2013) 22(25):5237–48. doi: 10.1093/hmg/ddt381
32. Dhanasekaran R, Deutzmann A, Mahauad-Fernandez WD, Hansen AS, Gouw AM, Felsher DW. The myc oncogene - the grand orchestrator of cancer growth and immune evasion. Nat Rev Clin Oncol (2022) 19(1):23–36. doi: 10.1038/s41571-021-00549-2
33. Baudino TA, McKay C, Pendeville-Samain H, Nilsson JA, Maclean KH, White EL, et al. C-myc is essential for vasculogenesis and angiogenesis during development and tumor progression. Genes Dev (2002) 16(19):2530–43. doi: 10.1101/gad.1024602
34. Florea V, Bhagavatula N, Simovic G, Macedo FY, Fock RA, Rodrigues CO. C-myc is essential to prevent endothelial pro-inflammatory senescent phenotype. PloS One (2013) 8(9):e73146. doi: 10.1371/journal.pone.0073146
35. Qi Y, Qadir MMF, Hastreiter AA, Fock RA, Machi JF, Morales AA, et al. Endothelial c-myc knockout enhances diet-induced liver inflammation and fibrosis. FASEB J (2022) 36(1):e22077. doi: 10.1096/fj.202101086R
36. Khalil N, Xu YD, O'Connor R, Duronio V. Proliferation of pulmonary interstitial fibroblasts is mediated by transforming growth factor-Beta1-Induced release of extracellular fibroblast growth factor-2 and phosphorylation of P38 mapk and jnk. J Biol Chem (2005) 280(52):43000–9. doi: 10.1074/jbc.M510441200
37. Disayabutr S, Kim EK, Cha SI, Green G, Naikawadi RP, Jones KD, et al. Mir-34 mirnas regulate cellular senescence in type ii alveolar epithelial cells of patients with idiopathic pulmonary fibrosis. PloS One (2016) 11(6):e0158367. doi: 10.1371/journal.pone.0158367
38. Roux PP, Blenis J. Erk and P38 mapk-activated protein kinases: A family of protein kinases with diverse biological functions. Microbiol Mol Biol Rev MMBR (2004) 68(2):320–44. doi: 10.1128/mmbr.68.2.320-344.2004
39. Roskoski R Jr. Erk1/2 map kinases: Structure, function, and regulation. Pharmacol Res (2012) 66(2):105–43. doi: 10.1016/j.phrs.2012.04.005
40. Mebratu Y, Tesfaigzi Y. How Erk1/2 activation controls cell proliferation and cell death: Is subcellular localization the answer? Cell Cycle (Georgetown Tex) (2009) 8(8):1168–75. doi: 10.4161/cc.8.8.8147
41. Sun Y, Liu WZ, Liu T, Feng X, Yang N, Zhou HF. Signaling pathway of Mapk/Erk in cell proliferation, differentiation, migration, senescence and apoptosis. J receptor Signal transduction Res (2015) 35(6):600–4. doi: 10.3109/10799893.2015.1030412
42. Mercer BA, Kolesnikova N, Sonett J, D'Armiento J. Extracellular regulated Kinase/Mitogen activated protein kinase is up-regulated in pulmonary emphysema and mediates matrix metalloproteinase-1 induction by cigarette smoke. J Biol Chem (2004) 279(17):17690–6. doi: 10.1074/jbc.M313842200
43. Lee PJ, Zhang X, Shan P, Ma B, Lee CG, Homer RJ, et al. Erk1/2 mitogen-activated protein kinase selectively mediates il-13-Induced lung inflammation and remodeling in vivo. J Clin Invest (2006) 116(1):163–73. doi: 10.1172/jci25711
44. Chen L, Hou J, Fu X, Chen X, Wu J, Han X. Tpa promotes the proliferation of lung fibroblasts and activates the Wnt/B-catenin signaling pathway in idiopathic pulmonary fibrosis. Cell Cycle (Georgetown Tex) (2019) 18(22):3137–46. doi: 10.1080/15384101.2019.1669997
45. Zou M, Zhang G, Zou J, Liu Y, Liu B, Hu X, et al. Inhibition of the Erk1/2-ubiquitous calpains pathway attenuates experimental pulmonary fibrosis in vivo and in vitro. Exp Cell Res (2020) 391(1):111886. doi: 10.1016/j.yexcr.2020.111886
46. MacKenzie B, Korfei M, Henneke I, Sibinska Z, Tian X, Hezel S, et al. Increased Fgf1-fgfrc expression in idiopathic pulmonary fibrosis. Respir Res (2015) 16(1):83. doi: 10.1186/s12931-015-0242-2
47. Bekpen C, Xavier RJ, Eichler EE. Human irgm gene "to be or not to be". Semin immunopathol (2010) 32(4):437–44. doi: 10.1007/s00281-010-0224-x
48. Chauhan S, Mandell MA, Deretic V. Irgm governs the core autophagy machinery to conduct antimicrobial defense. Mol Cell (2015) 58(3):507–21. doi: 10.1016/j.molcel.2015.03.020
49. Mehto S, Jena KK, Nath P, Chauhan S, Kolapalli SP, Das SK, et al. The crohn's disease risk factor irgm limits Nlrp3 inflammasome activation by impeding its assembly and by mediating its selective autophagy. Mol Cell (2019) 73(3):429–45.e7. doi: 10.1016/j.molcel.2018.11.018
50. Jena KK, Mehto S, Nath P, Chauhan NR, Sahu R, Dhar K, et al. Autoimmunity gene irgm suppresses cgas-sting and rig-I-Mavs signaling to control interferon response. EMBO Rep (2020) 21(9):e50051. doi: 10.15252/embr.202050051
51. Bafica A, Feng CG, Santiago HC, Aliberti J, Cheever A, Thomas KE, et al. The ifn-inducible gtpase Lrg47 (Irgm1) negatively regulates Tlr4-triggered proinflammatory cytokine production and prevents endotoxemia. J Immunol (Baltimore Md 1950) (2007) 179(8):5514–22. doi: 10.4049/jimmunol.179.8.5514
52. Azzam KM, Madenspacher JH, Cain DW, Lai L, Gowdy KM, Rai P, et al. Irgm1 coordinately regulates autoimmunity and host defense at select mucosal surfaces. JCI Insight (2017) 2(16):e91914. doi: 10.1172/jci.insight.91914
53. Parkes M, Barrett JC, Prescott NJ, Tremelling M, Anderson CA, Fisher SA, et al. Sequence variants in the autophagy gene irgm and multiple other replicating loci contribute to crohn's disease susceptibility. Nat Genet (2007) 39(7):830–2. doi: 10.1038/ng2061
54. King KY, Lew JD, Ha NP, Lin JS, Ma X, Graviss EA, et al. Polymorphic allele of human Irgm1 is associated with susceptibility to tuberculosis in African americans. PloS One (2011) 6(1):e16317. doi: 10.1371/journal.pone.0016317
55. Steinberg SF. Structural basis of protein kinase c isoform function. Physiol Rev (2008) 88(4):1341–78. doi: 10.1152/physrev.00034.2007
56. Sakaki K, Wu J, Kaufman RJ. Protein kinase ctheta is required for autophagy in response to stress in the endoplasmic reticulum. J Biol Chem (2008) 283(22):15370–80. doi: 10.1074/jbc.M710209200
57. Zhang EY, Kong KF, Altman A. The yin and yang of protein kinase c-theta (Pkcθ): A novel drug target for selective immunosuppression. Adv Pharmacol (San Diego Calif) (2013) 66:267–312. doi: 10.1016/b978-0-12-404717-4.00006-8
58. Chaudhary D, Kasaian M. Pkctheta: A potential therapeutic target for T-Cell-Mediated diseases. Curr Opin investigational Drugs (London Engl 2000) (2006) 7(5):432–7.
59. Liu Z, Zhang K, Zhao Z, Qin Z, Tang H. Prognosis-related autophagy genes in female lung adenocarcinoma. Medicine (2022) 101(1):e28500. doi: 10.1097/md.0000000000028500
60. Wan B, Liu B, Yu G, Huang Y, Lv C. Differentially expressed autophagy-related genes are potential prognostic and diagnostic biomarkers in clear-cell renal cell carcinoma. Aging (2019) 11(20):9025–42. doi: 10.18632/aging.102368
61. Li M, Zhao Y, He J, Deng W, Cheng L, Jiang Z, et al. Protein kinase c theta inhibition attenuates lipopolysaccharide-induced acute lung injury through notch signaling pathway Via suppressing Th17 cell response in mice. Inflammation (2019) 42(6):1980–9. doi: 10.1007/s10753-019-01058-2
62. Lei L, Zhao C, Qin F, He ZY, Wang X, Zhong XN. Th17 cells and il-17 promote the skin and lung inflammation and fibrosis process in a bleomycin-induced murine model of systemic sclerosis. Clin Exp Rheumatol (2016) 34 Suppl 100(5):14–22.
63. Heukels P, Moor CC, von der Thüsen JH, Wijsenbeek MS, Kool M. Inflammation and immunity in ipf pathogenesis and treatment. Respir Med (2019) 147:79–91. doi: 10.1016/j.rmed.2018.12.015
64. Kaletsky R, Lakhina V, Arey R, Williams A, Landis J, Ashraf J, et al. The c. elegans adult neuronal Iis/Foxo transcriptome reveals adult phenotype regulators. Nature (2016) 529(7584):92–6. doi: 10.1038/nature16483
65. Wilhelm K, Happel K, Eelen G, Schoors S, Oellerich MF, Lim R, et al. Foxo1 couples metabolic activity and growth state in the vascular endothelium. Nature (2016) 529(7585):216–20. doi: 10.1038/nature16498
66. Maiese K, Hou J, Chong ZZ, Shang YC. A fork in the path: Developing therapeutic inroads with foxo proteins. Oxid Med Cell Longevity (2009) 2(3):119–29. doi: 10.4161/oxim.2.3.8916
67. Zhang J, Ng S, Wang J, Zhou J, Tan SH, Yang N, et al. Histone deacetylase inhibitors induce autophagy through Foxo1-dependent pathways. Autophagy (2015) 11(4):629–42. doi: 10.1080/15548627.2015.1023981
68. Xin Z, Ma Z, Hu W, Jiang S, Yang Z, Li T, et al. Foxo1/3: Potential suppressors of fibrosis. Ageing Res Rev (2018) 41:42–52. doi: 10.1016/j.arr.2017.11.002
69. Huang F, Wang Q, Guo F, Zhao Y, Ji L, An T, et al. Foxo1-mediated inhibition of Stat1 alleviates tubulointerstitial fibrosis and tubule apoptosis in diabetic kidney disease. EBioMedicine (2019) 48:491–504. doi: 10.1016/j.ebiom.2019.09.002
70. Xiang M, Liu T, Tian C, Ma K, Gou J, Huang R, et al. Kinsenoside attenuates liver fibro-inflammation by suppressing dendritic cells Via the Pi3k-Akt-Foxo1 pathway. Pharmacol Res (2022) 177:106092. doi: 10.1016/j.phrs.2022.106092
71. Im J, Hergert P, Nho RS. Reduced Foxo3a expression causes low autophagy in idiopathic pulmonary fibrosis fibroblasts on collagen matrices. Am J Physiol Lung Cell Mol Physiol (2015) 309(6):L552-61. doi: 10.1152/ajplung.00079.2015
72. Luzina IG, Todd NW, Iacono AT, Atamas SP. Roles of T lymphocytes in pulmonary fibrosis. J leukocyte Biol (2008) 83(2):237–44. doi: 10.1189/jlb.0707504
73. Saito A, Okazaki H, Sugawara I, Yamamoto K, Takizawa H. Potential action of il-4 and il-13 as fibrogenic factors on lung fibroblasts in vitro. Int Arch Allergy Immunol (2003) 132(2):168–76. doi: 10.1159/000073718
74. Atamas SP, White B. Cytokine regulation of pulmonary fibrosis in scleroderma. Cytokine Growth factor Rev (2003) 14(6):537–50. doi: 10.1016/s1359-6101(03)00060-1
75. Keane MP, Belperio JA, Burdick MD, Strieter RM. Il-12 attenuates bleomycin-induced pulmonary fibrosis. Am J Physiol Lung Cell Mol Physiol (2001) 281(1):L92–7. doi: 10.1152/ajplung.2001.281.1.L92
76. Kolahian S, Fernandez IE, Eickelberg O, Hartl D. Immune mechanisms in pulmonary fibrosis. Am J Respir Cell Mol Biol (2016) 55(3):309–22. doi: 10.1165/rcmb.2016-0121TR
77. Wynn TA. Fibrotic disease and the T(H)1/T(H)2 paradigm. Nat Rev Immunol (2004) 4(8):583–94. doi: 10.1038/nri1412
78. Boveda-Ruiz D, D'Alessandro-Gabazza CN, Toda M, Takagi T, Naito M, Matsushima Y, et al. Differential role of regulatory T cells in early and late stages of pulmonary fibrosis. Immunobiology (2013) 218(2):245–54. doi: 10.1016/j.imbio.2012.05.020
79. Garibaldi BT, D'Alessio FR, Mock JR, Files DC, Chau E, Eto Y, et al. Regulatory T cells reduce acute lung injury fibroproliferation by decreasing fibrocyte recruitment. Am J Respir Cell Mol Biol (2013) 48(1):35–43. doi: 10.1165/rcmb.2012-0198OC
80. Hou Z, Ye Q, Qiu M, Hao Y, Han J, Zeng H. Increased activated regulatory T cells proportion correlate with the severity of idiopathic pulmonary fibrosis. Respir Res (2017) 18(1):170. doi: 10.1186/s12931-017-0653-3
81. Kreuter M, Lee JS, Tzouvelekis A, Oldham JM, Molyneaux PL, Weycker D, et al. Monocyte count as a prognostic biomarker in patients with idiopathic pulmonary fibrosis. Am J Respir Crit Care Med (2021) 204(1):74–81. doi: 10.1164/rccm.202003-0669OC
82. Teoh AKY, Jo HE, Chambers DC, Symons K, Walters EH, Goh NS, et al. Blood monocyte counts as a potential prognostic marker for idiopathic pulmonary fibrosis: Analysis from the Australian ipf registry. Eur Respir J (2020) 55(4):1901855. doi: 10.1183/13993003.01855-2019
83. Moore BB, Fry C, Zhou Y, Murray S, Han MK, Martinez FJ, et al. Inflammatory leukocyte phenotypes correlate with disease progression in idiopathic pulmonary fibrosis. Front Med (2014) 1:56(56). doi: 10.3389/fmed.2014.00056
84. Lech M, Anders HJ. Macrophages and fibrosis: How resident and infiltrating mononuclear phagocytes orchestrate all phases of tissue injury and repair. Biochim Biophys Acta (2013) 1832(7):989–97. doi: 10.1016/j.bbadis.2012.12.001
85. Abraham SN, St John AL. Mast cell-orchestrated immunity to pathogens. Nat Rev Immunol (2010) 10(6):440–52. doi: 10.1038/nri2782
86. Veerappan A, O'Connor NJ, Brazin J, Reid AC, Jung A, McGee D, et al. Mast cells: A pivotal role in pulmonary fibrosis. DNA Cell Biol (2013) 32(4):206–18. doi: 10.1089/dna.2013.2005
87. Walls AF, Bennett AR, Godfrey RC, Holgate ST, Church MK. Mast cell tryptase and histamine concentrations in bronchoalveolar lavage fluid from patients with interstitial lung disease. Clin Sci (London Engl 1979) (1991) 81(2):183–8. doi: 10.1042/cs0810183
88. Wygrecka M, Kwapiszewska G, Jablonska E, von Gerlach S, Henneke I, Zakrzewicz D, et al. Role of protease-activated receptor-2 in idiopathic pulmonary fibrosis. Am J Respir Crit Care Med (2011) 183(12):1703–14. doi: 10.1164/rccm.201009-1479OC
89. Overed-Sayer C, Rapley L, Mustelin T, Clarke DL. Are mast cells instrumental for fibrotic diseases? Front Pharmacol (2013) 4:174. doi: 10.3389/fphar.2013.00174
Keywords: IPF, autophagy, prognosis, bioinformatics, immune infiltration
Citation: Fan G, Liu J, Wu Z, Li C and Zhang Y (2022) Development and validation of the prognostic model based on autophagy-associated genes in idiopathic pulmonary fibrosis. Front. Immunol. 13:1049361. doi: 10.3389/fimmu.2022.1049361
Received: 21 September 2022; Accepted: 28 November 2022;
Published: 12 December 2022.
Edited by:
Paola Maycotte, Instituto Mexicano del Seguro Social, MexicoReviewed by:
Cong Liu, First Affiliated Hospital of Nanchang University, ChinaXiao Xiao Tang, First Affiliated Hospital of Guangzhou Medical University, China
Xiaodong Song, Binzhou Medical University, China
Copyright © 2022 Fan, Liu, Wu, Li and Zhang. This is an open-access article distributed under the terms of the Creative Commons Attribution License (CC BY). The use, distribution or reproduction in other forums is permitted, provided the original author(s) and the copyright owner(s) are credited and that the original publication in this journal is cited, in accordance with accepted academic practice. No use, distribution or reproduction is permitted which does not comply with these terms.
*Correspondence: Ying Zhang, emhfZGp0QDE2My5jb20=
†These authors have contributed equally to this work and share first authorship