- 1Department of Neurosurgery, Fuzong Clinical Medical College of Fujian Medical University, Fuzhou, Fujian, China
- 2Department of Neurosurgery, Affiliated Hospital of Guilin Medical University, Guilin, Guangxi, China
- 3Department of Geriatrics, Affiliated Huai’an No.2 People’s Hospital of Xuzhou Medical University, Huai’an, Jiangsu, China
- 4Department of Neurosurgery, 900th Hospital, Fuzong Clinical Medical College of Fujian Medical University, Fuzhou, China
- 5Department of Neurosurgery, Ganzhou People's Hospital, No.16 Meiguan Avenue, Zhanggong District, Ganzhou, Jiangxi, China
- 6Institute of Medical Genetics and Reproductive Immunity, School of Medical Science and Laboratory Medicine, Jiangsu College of Nursing, No.2 the Yellow River West Road Huai'an, Jiangsu, China
Background: Recent studies have shown that systemic inflammation responses and hyperventilation are associated with poor outcomes in patients with severe traumatic brain injury (TBI). The aim of this retrospective study was to investigate the relationships between the systemic immune inflammation index (SII = platelet × neutrophil/lymphocyte) and peripheral blood CO2 concentration at admission with the Glasgow Outcome Score (GOS) at 6 months after discharge in patients with severe TBI.
Methods: We retrospectively analyzed the clinical data for 1266 patients with severe TBI at three large medical centers from January 2016 to December 2021, and recorded the GOS 6 months after discharge. The receiver operating characteristic (ROC) curve was used to determine the best cutoff values for SII, CO2, neutrophil to lymphocyte ratio (NLR), platelet to lymphocyte ratio (PLR), and lymphocyte to monocyte ratio (LMR), and chi-square tests were used to evaluate the relationships among SII, CO2 and the basic clinical characteristics of patients with TBI. Multivariate logistic regression analysis was used to determine the independent prognostic factors for GOS in patients with severe TBI. Finally, ROC curve, nomogram, calibration curve and decision curve analyses were used to evaluate the value of SII and coSII-CO2 in predicting the prognosis of patients with severe TBI. And we used the multifactor regression analysis method to build the CRASH model and the IMPACT model. The CRASH model included age, GCS score (GCS, Glasgow Coma Scale) and Pupillary reflex to light: one, both, none. The IMPACT model includes age, motor score and Pupillary reflex to light: one, both, none.
Results: The ROC curves indicated that the best cutoff values of SII, CO2, PLR, NLR and LMR were 2651.43×109, 22.15mmol/L, 190.98×109, 9.66×109 and 1.5×109, respectively. The GOS at 6 months after discharge of patients with high SII and low CO2 were significantly poorer than those with low SII and high CO2. Multivariate logistic regression analysis revealed that age, systolic blood pressure (SBP), pupil size, subarachnoid hemorrhage (SAH), SII, PLR, serum potassium concentration [K+], serum calcium concentration [Ca2+], international normalized ratio (INR), C-reactive protein (CRP) and co-systemic immune inflammation index combined with carbon dioxide (coSII-CO2) (P < 0.001) were independent prognostic factors for GOS in patients with severe TBI. In the training group, the C-index was 0.837 with SII and 0.860 with coSII-CO2. In the external validation group, the C-index was 0.907 with SII and 0.916 with coSII-CO2. Decision curve analysis confirmed a superior net clinical benefit with coSII-CO2 rather than SII in most cases. Furthermore, the calibration curve for the probability of GOS 6 months after discharge showed better agreement with the observed results when based on the coSII-CO2 rather than the SII nomogram. According to machine learning, coSII-CO2 ranked first in importance and was followed by pupil size, then SII.
Conclusions: SII and CO2 have better predictive performance than NLR, PLR and LMR. SII and CO2 can be used as new, accurate and objective clinical predictors, and coSII-CO2, based on combining SII with CO2, can be used to improve the accuracy of GOS prediction in patients with TBI 6 months after discharge.
Introduction
Traumatic brain injury (TBI) refers to multiple commonly occurring physical brain injuries caused by mechanical forces (1–3). The incidence of TBI has gradually increased; by 2020, TBI became a major health problem and cause of disability (4). China has the largest number of patients with TBI worldwide, with a population-based TBI mortality rate of approximately 13/100,000, in agreement with the mortality rates reported in other countries. Acute primary TBI is characterized by destruction of brain tissue due to focal intracranial hemorrhage, epidural hematoma, subdural hematoma, cerebral contusion and diffuse axonal injury. This primary brain injury results in neuronal damage, excitatory toxicity, free radical production and inflammatory responses. These events can trigger secondary brain damage, which starts within minutes of the initial injury and often lasts for months or even years (5). Clinical treatment methods for patients with TBI are very limited, and traditional decompressive craniectomy is not adequate for all emergencies. The lack of treatment measures is due to the variety of injury types, and insufficient recognition and poor understanding of the mechanisms of secondary brain injury (6–8). TBI is a heterogeneous disease in terms of etiology, pathology, severity and prognosis, thus resulting in substantial uncertainty in the expected outcomes for individual patients (9). Therefore, methods to predict the prognosis of patients with TBI are urgently needed. Prognostic models can be used to predict outcomes according to the characteristics of individual patients. Reliable prediction results can provide information allowing physicians to perform clinical interventions more effectively and improve patient prognosis.
Clinical evidence and experiments have shown that TBI can lead to rapid inflammatory response and to secondary injury characterized by the activation of resident cells, migration and recruitment of peripheral neutrophils, and release of inflammatory mediators (10, 11). Neutrophils are the most abundant cells circulating after TBI, in both the acute phase and the post-injury period. The absolute number and frequency of circulating neutrophils is significantly higher in patients with TBI than healthy controls, and a doubling of neutrophils is observed 3 – 4.5 h after TBI (12). After neutrophils enter the brain, they release many inflammatory cytokines and factors, such as chemokines, reactive oxygen species, tumor necrosis factor (TNF-α), transforming growth factor-β (TGF-β) and interleukin-1 β (IL-1β), which in turn promote the downstream activation of intracellular signaling cascades, involving NF-Kb. Released cytokines in turn can recruit additional blood-borne neutrophils and monocytes into the injured tissue, propagating the inflammatory cascade (13). Recent clinical research has examined the ability of inflammatory biomarkers, such as the NLR, PLR and LMR, to predict outcomes after TBI. Inflammatory biomarkers after TBI have been demonstrated to have good predictive function (14–16). However, inflammation-based biomarkers are calculated on the basis of two immune cell types and do not fully indicate the role of inflammation in TBI. The SII, based on peripheral blood platelets, lymphocytes and neutrophils, more comprehensively reflects the immune state and the dynamic balance between host inflammatory process. Consequently, it has been found to provide better, more comprehensive predictions of prognosis in many types of cancer (17–19). However, the SII has not been validated in patients with TBI.
Previous studies have shown that patients with severe TBI may experience hyperventilation at early stages, thus decreasing peripheral blood CO2 levels. The decreases in peripheral blood CO2 concentrations and partial pressure of CO2 lead to intracranial vasoconstriction, decreased cerebral blood flow and decreased intracranial pressure (ICP), thus further aggravating the occurrence of brain metabolic disorders and inflammation after TBI (20–22). Peripheral blood CO2 concentrations have been found to influence the development of inflammation in mammalian innate immune and inflammatory experiments: elevated CO2 concentrations eliminate the NF-Kb activity induced by LPS in a manner independent of the IKKβ pathway. Hypercapnia inhibited the expression of TNF-α in macrophages stimulated by LPS and further attenuated macrophage phagocytosis. In addition, it inhibited IL-6 expression mediated by activation of multiple TLRS, while did not affect LPS-induced IL-10 or IFN-β expression. It also inhibited IL-6 expression by reducing luciferase activity driven by the IL-6 promoter. However, this process does not affect the stability of IL-6 Mrna. Hypercapnia significantly inhibits inflammation and decreases the concentration of CO2, thus enhancing NF-Kb activity and promoting inflammation. The use of humidified CO2 in laparoscopic tumor surgery decreases mesenchymal cell damage and inflammation, thereby diminishing the risk of peritoneal metastasis (23, 24). Induced hypocapnia has been used to treat elevated ICP, which is common in patients with TBI. Despite these findings, hypocapnia remains associated with adverse clinical outcomes of various forms of brain injury. Because the inhibition of cerebral blood flow may exacerbate ischemia and inflammation during acute brain injury and even lead to irreversible brain tissue infarction, chronic hypocapnia increases the risk of death and severe disability in patients with TBI (25, 26). To our knowledge, the relationship between changes in peripheral blood CO2 concentrations and the clinical prognosis of patients with severe TBI has not been studied. Peripheral blood CO2 concentration may be an important factor affecting inflammatory response in patients with TBI.
The objectives of this study were as follows. First, we aimed to collect data on systemic inflammatory indicators (SII, PLR, NLR and LMR), peripheral blood CO2 concentrations and other basic clinical characteristics of patients with severe TBI at admission. Second, we aimed to compare and analyze the predictive performance, best cutoff value and prognostic value of SII, PLR, NLR, LMR, CO2 and coSII-CO2. Third, we warranted to analyze the roles of SII and coSII-CO2 in predicting the prognosis of patients with TBI and to assess their order of importance by using nomograms and machine learning, to provide a valuable predictive model for physicians.
Materials and methods
Patient section
The study was approved by the Fuzhou 900th Hospital of PLA ethics committee and conducted in accordance with the Declaration of Helsinki. As it was a retrospective study, the ethics committee approved the waiver of signed informed consent, in accordance with Chinese laws and institutional requirements. Data on patients with TBI at three large medical centers in different provinces of China from January 2016 to December 2021 were collected. The inclusion criteria were as follows: (1) types of injuries in patients with severe TBI: primary TBI caused by car accidents, high fall injury and external object strikes; (2) admission to the hospital within 12 h after injury, and completion of basic laboratory tests for basic blood indicators in the emergency department; (3) Glasgow Coma Scale score ≤ 8 at admission. The exclusion criteria were as follows: (1) death on admission; (2) clinical evidence of hematological malignancy, chronic inflammatory disease or acute infection; (3) admission to our hospital after emergency surgery in other hospitals.
Data collection and definition
Clinical data were collected by neurosurgeons from 3 different hospitals in their own hospitals. Patients with severe craniocerebral injury were first screened out through medical records, and relevant examination and blood routine results were collected. Subsequently, GOS scores of patients for 6 months were collected through telephone, outpatient and other following-up methods. Among them, 89 patients were lost to following-up and 14 patients refused to following-up. The examined patient characteristics included age, sex, systolic pressure (SBP), pupil size, subarachnoid hemorrhage (SAH), Glasgow Coma Scale (GCS) score at admission, C-reactive protein (CRP), platelet count (PLT), platelets × neutrophils/lymphocytes (SII), neutrophil/lymphocyte ratio (NLR), platelet/lymphocyte ratio (PLR), lymphocyte/monocyte ratio (LMR),International Normalized Ratio (INR), plasma D-dimer (D-dimer), blood glucose (Glu) level at admission, Albumin (Alb), venous blood carbon dioxide (CO2) concentration at admission, creatine kinase (CK), creatinine (Cr), blood electrolytes, routine blood examination and length of hospital stay (LOS).
Follow up
All patients were regularly followed up by professional staffs through outpatient service, SMS, and telephone, and discharge Glasgow Outcome Scale (GOS) assessment results were determined. Under this rating system, a GOS score of 1 indicates death, 2 indicates a persistent vegetative state, 3 indicated severe disability (conscious but disabled), 4 indicated moderate disability (disabled but independent), and 5 indicated excellent recovery with a return to baseline functional status. Dichotomized as favorable (GOS 4-5) vs. unfavorable (GOS 1-3) (27).
Statistical analysis
SPSS21.0 (SPSS Inc., Chicago, IL) was used for data analysis. R Studio (version 4.2.0) was used for nomograms, calibration curves, decision curve analysis (DCA) and C-index calculation. GraphPad Prism 8.0.2 was used to plot bar charts and the receiver operating characteristic (ROC) curves of six indicators. Kolmogorov-Smirnov tests were used to test the normality of the data. Mean ± standard deviation was used to represent the measurement data conforming to a normal distribution, and t-tests were used for inter-group comparisons. Median and quartiles were used to represent the measurement data conforming to a normal distribution, and rank-sum tests were used for inter-group comparisons. Statistical data are expressed as percentage N (%), and comparisons between groups were performed with chi-square or Fisher’s tests. The ROC curve was used to calculate the best cutoff values for SII, CO2, NLR, PLR and LMR, with end points based on the patients’ GOS scores 6 months after discharge. Binary logistic analysis was performed to evaluate independent predictors of poor outcome in patients with severe TBI for factors that were significant in univariate analysis. All tests were bidirectional, and P < 0.05 was considered to indicate significant differences. Factors with P < 0.05 in univariate analysis were included in multivariate analysis. Two-sided tests were used for all statistics, and P < 0.05 was considered statistically significant. For missing data, the direct deletion method is used.
Results
Patient characteristics
A total of 1266 patients with severe TBI in three large hospitals were collected from January 2016 to December 2021. Among them, 1009 patients were enrolled in the study (802 in the Fuzhou 900th Hospital of PLA, 104 in the Affiliated Hospital of Guilin Medical University and 103 in Ganzhou People’s Hospital of Jiangxi Province). A total of 802 patients from the Fuzhou 900th Hospital of PLA were included in the training group, and 207 patients from the two other centers were included in the external validation group. Figure 1 shows the exclusion process. 1009 patients met the analysis conditions, including 223 women (22.1%) and 786 men (77.1%). The age (and interquartile range [IQR]) of the cohort was 49 [34–62] years. All 1009 patients were followed up, and the median GOS [IQR] score was 3 [1–5] at 6 months after discharge; 368 deaths (36.4%) and 641 survivors (63.6%) were recorded.
Determination of the best cutoff values for SII, CO2, PLR, NLR and LMR at admission, and definition of coSII-CO2 grouping
The best cutoff values for SII, CO2, PLR, NLR and LMR in the study population were determined by ROC analysis, and GOS scores were predicted 6 months after discharge in patients with severe TBI. The GOS area under the curve (AUC) was as follows: SII=0.676 (95% CI: 0.644 - 0.710), CO2 = 0.648 (95% CI: 0.612 - 0.680), PLR=0.636 (95% CI: 0.6601 - 0.670), NLR=0.617 (95% CI: 0.583 - 0.653) and LMR=0.623 (95% CI: 0.586 - 0.655), corresponding to the best cutoff values of 2651.43, 22.15, 190.98, 9.66 and 1.5, respectively. According to the best cutoff values, patients were divided into two groups for analysis: a high SII group (SII ≥ 2651.43, n=336) and low SII group (SII < 2651.43, n=667); high CO2 group (CO2 ≥ 22.15, n=655) and low CO2 group (CO2 < 22.15, n=347); high PLR group (PLR ≥ 190.98, n=357) and low PLR group (PLR < 190.98, n=646); high NLR group (NLR ≥ 9.66, n=551) and low NLR group (NLR < 9.66, n=452); and high LMR group (LMR ≥ 1.5, n=629) and low LMR group (LMR < 1.5, n=374). coSII-CO2 groups were defined. Patients with high SII and low CO2 were assigned 3 points, patients with high SII and high CO2 were assigned 2 points, patients with low SII and low CO2 were assigned 1 point, and patients with low SII and high CO2 were assigned 0 points.
Relationships among clinical characteristics of patients with severe TBI and SII, CO2, PLR, NLR, LMR
SII correlated with SBP, pupil size, SAH, Glu, [K+], INR, CRP, NEU, LYM and MON (P < 0.05), but not with sex, age, LOS, Cr, Alb, CK, serum sodium concentration [Na+], [Ca2+], D-dimer and PLT (P > 0.05). CO2 was associated with LOS, pupil size, Alb, [Ca2+] and INR (P < 0.05). Sex, age, SBP, SAH, Cr, Glu, CK, [K+], [Na+], [Ca2+], D-dimer, CRP, NEU, PLT, LYM and MON were not correlated (P > 0.05). PLR correlated with SBP, pupil size, Glu, [Na+] and LYM (P < 0.05), but not sex, age, LOS, SAH, Cr, Alb, CK, [K+], [Ca2+], D-dimer, INR, CRP, NEU, PLT and MON (P > 0.05). NLR was associated with SBP, pupil size, Glu, CK, INR, NEU and LYM (P < 0.05), but not sex, age, LOS, SAH, Cr, Alb, [K+], [Na+], [Ca2+], D-dimer, CRP, PLT and MON (P > 0.05). LMR was correlated with sex, SBP, pupil size, Glu, CK, D-dimer, INR, CRP, NEU, LYM and MON (P < 0.05), but not with age, LOS, SAH, Cr, Alb, [K+], [Na+], [Ca2+] and PLT (P > 0.05; Table 1).
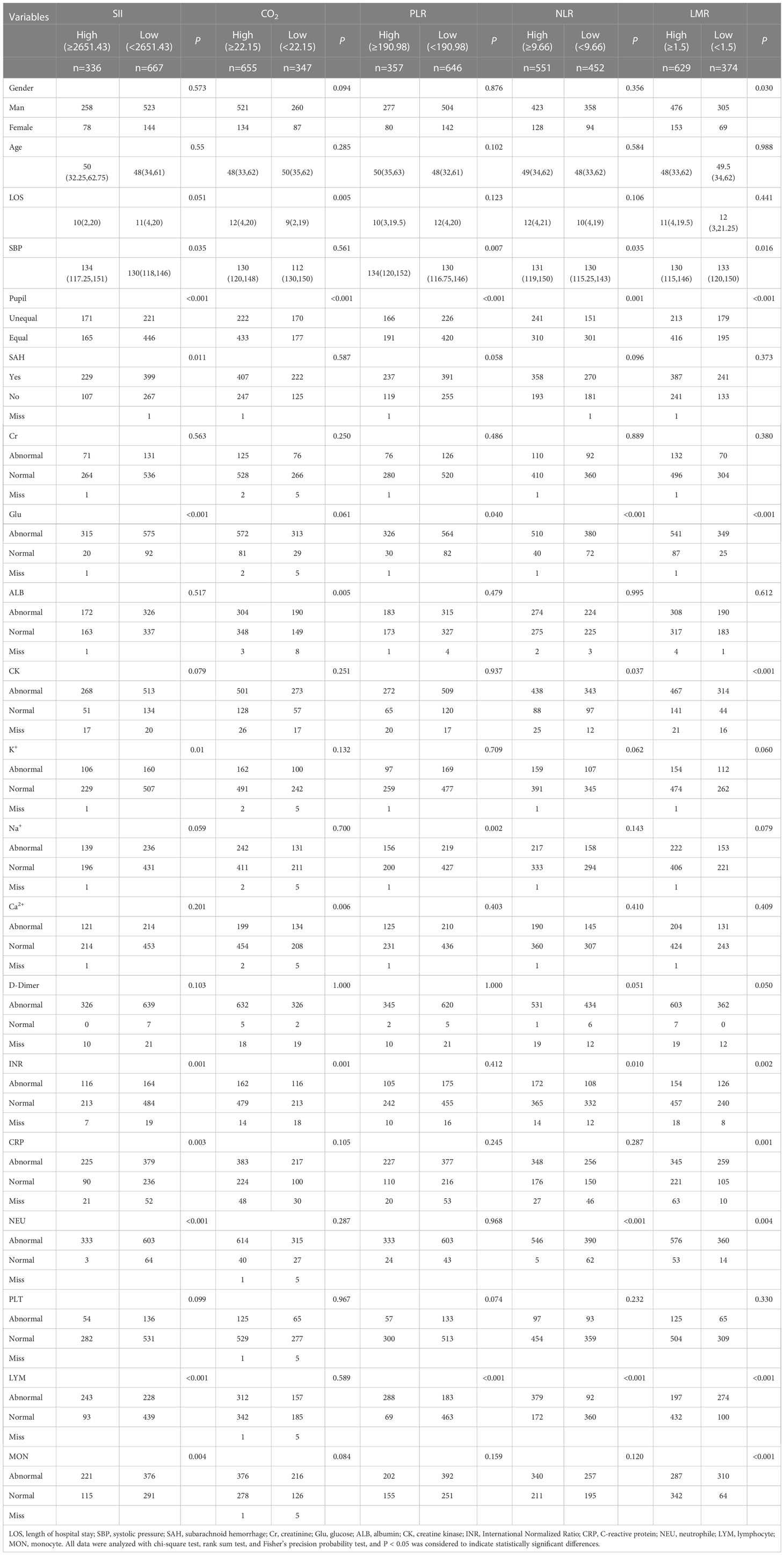
Table 1 Relationships among SII, CO2, PLR, NLR, and LMR, and basic clinical features in patients with severe TBI.
Relationships among SII, CO2, PLR, NLR, LMR, coSII-CO2 and GOS scores at 6 months after discharge in patients with severe TBI
The GOS significantly differed from SII, CO2, PLR, NLR, LMR and coSII-CO2 in patients with severe TBI (P < 0.001). Patients with GOS > 3 tended to have low SII (84%), high CO2 (80%), low PLR (77%), low NLR (55%), high LMR (74%) and coSII-CO2 scores of 0 (69%). Patients with GOS ≤ 3 showed opposite trends (Figure 2 and Table 2).
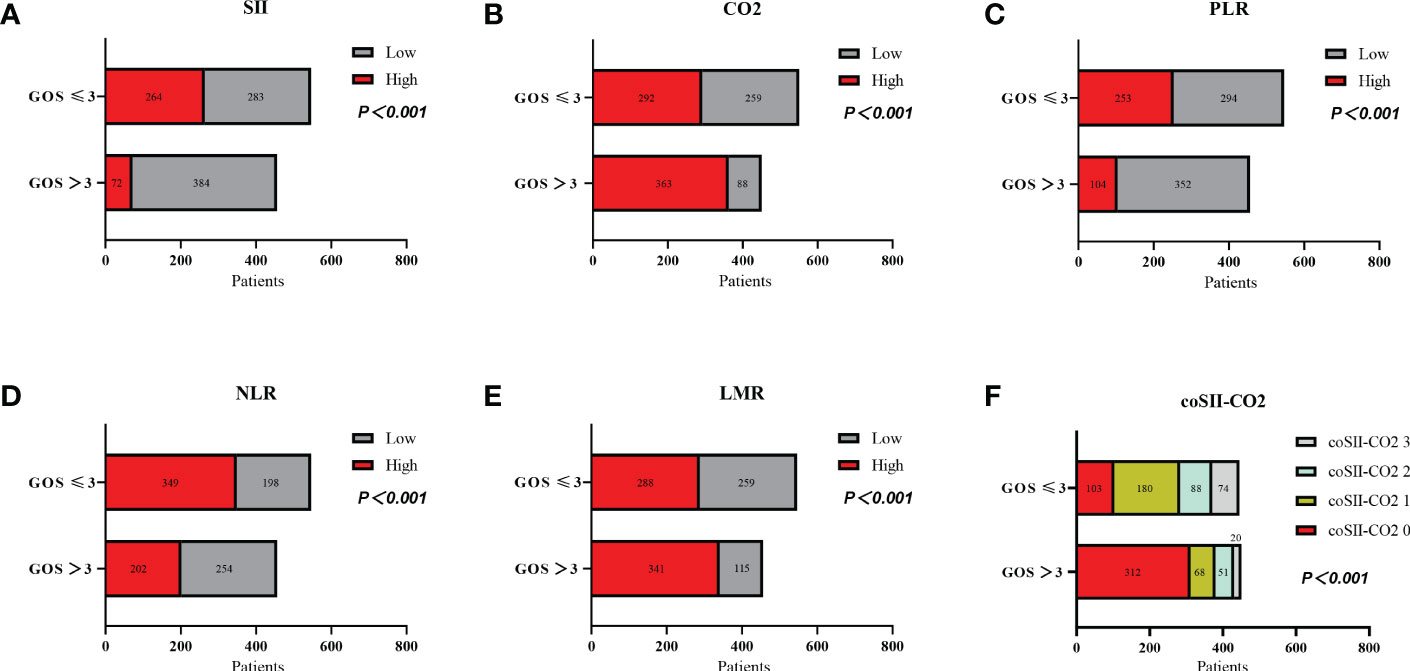
Figure 2 Relationships among levels of SII, CO2, PLR, NLR, and LMR, and coSII-CO2 and GOS in patients with severe TBI. (A) Patients with high SII ≥ 2651.43 had poorer GOS than patients with low SII<2651.43 (P < 0.001). (B) Patients with low CO2<22.15 had poorer GOS than patients with high CO2 ≥ 22.15 (P < 0.001). (C) Patients with high PLR ≥ 190.98 had poorer GOS than patients with low PLR<190.98 (P < 0.001). (D) Patients with high NLR ≥ 9.66 had poorer GOS than patients with low NLR<9.66 (P < 0.001). (E) Patients with low LMR<1.5 had poorer GOS than patients with high LMR ≥1.5 (P < 0.001). (F) GOS scores of patients: coSII-CO2 0 > coSII-CO2 1 > coSII-CO2 2 > coSII-CO2 3 (P < 0.001).

Table 2 Relationships among the levels of SII, CO2, PLR, NLR, and LMR, and the coSII-CO2 and GOS scores in patients with TBI.
Prognostic value of coSII-CO2 in patients with severe TBI
The predictive accuracy of SII, CO2, PLR, NLR, LMR and coSII-CO2 for GOS 6 months after discharge were compared by ROC curve analysis. The AUC of SII, CO2, PLR, NLR, LMR and coSII-CO2 were as follows: SII=0.676 (95%CI: 0.644–0.710), CO2 = 0.648 (95%CI: 0.612–0.680), PLR=0.636 (95%CI: 0.601–0.670), NLR=0.617 (95%CI: 0.583–0.653), LMR=0.623 (95%CI: 0.586–0.655) and coSII-CO2 = 0.751 (95%CI: 0.721–0.781). The coSII-CO2 had a higher AUC than SII or CO2 alone, thus suggesting that coSII-CO2 was the most reliable of these predictors for GOS 6 months after discharge and could be used as a predictive tool (Figure 3).
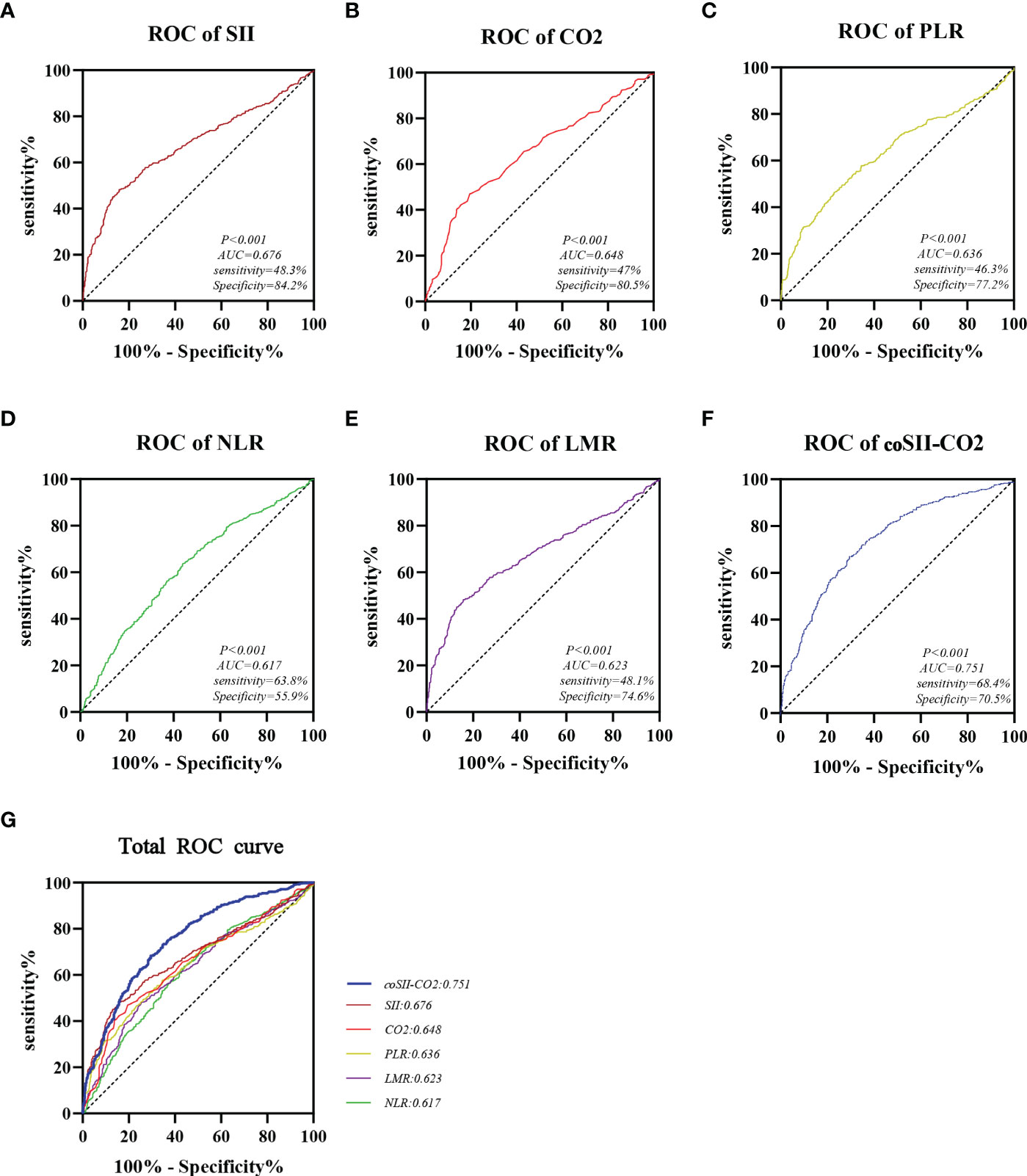
Figure 3 Areas under the curves of SII, CO2, PLR, NLR, LMR, and coSII-CO2. (A) The area under the curve of SII was 0.676. (B) The area under the curve of CO2 was 0.648. (C) The area under the curve of PLR was 0.636. (D) The area under the curve of NLR was 0.617. (E) The area under the curve of LMR was 0.623. (F) The area under the curve of coSII-CO2 was 0.751. (G) Compared with other independent predictive indicators, the area under the curve of coSII-CO2 in the combined group was largest, thus indicating that coSII-CO2 was the most accurate and reliable indicator for predicting the prognosis of patients with TBI.
Univariate and multivariate analysis of factors influencing GOS scores in patients with severe TBI
Univariate analysis indicated that age, SBP, pupil size, SAH, CO2, SII, PLR, NLR, LMR, Glu, Alb, Ck, [K+], [Na+], D-dimer, INR, CRP, LYM, MON and coSII-CO2 were important prognostic factors affecting GOS scores in patients with severe TBI. Multivariate logistic regression analysis showed that age (OR=1.576, 95% CI: 1.036 - 2.398, P=0.034), SBP (OR=1.925, 95%CI: 1.304 2.843, P=0.001), pupil size (OR=5.373, 95% CI: 3.614 7.988, P < 0.001), SAH (OR=1.472, 95% CI: 0.558 2.357, P=0.044), SII (OR=6.701, 95% CI: 3.821 11.752, P < 0.001), PLR (OR=1.671, 95% CI: 1.038 2.689, P=0.035), [K+] (OR=1.884, 95% CI: 1.222 2.904, P=0.004), [Ca2+] (OR=2.024, 95% CI: 1.321 3.100, P=0.001), INR (OR=3.138, 95% CI: 2.032 - 4.847, P < 0.001), CRP (OR=1.828, 95% CI: 1.242 - 2.694, P=0.002) and coSII-CO2 (OR=8.337, 95% CI: 3.579 - 19.606, P < 0.001) were independent prognostic factors affecting GOS in patients with severe TBI (Table 3).

Table 3 Univariate and multivariate logistic regression models used to analyze the factors influencing GOS in patients with TBI .
Comparison of basic clinical characteristic data between the training group and external validation group
The baseline characteristics of patients in the training group and the validation group were essentially the same, and no significant differences were observed between groups (P > 0.05), thus indirectly demonstrating that the clinical characteristic data in this study were not affected by the different hospitals (Table 4).
Comparison of nomogram predictive performance based on SII and coSII-CO2
To further predict the GOS of patients with severe TBI at 6 months after discharge, we used the logistic regression model (age, SBP, pupil size, SAH, SII, PLR, [K+], [Ca2+], INR, CRP and coSII-CO2) to construct nomograms (Figure 4). In the training group, the C-index (age, SBP, pupil size, SAH, SII, PLR, [K+], [Ca2+], INR and CRP) based on the SII nomogram was 0.837. The C index (age, SBP, pupil size, SAH, PLR, [K+], [Ca2+], INR, CRP and coSII-CO2) of the coSIi-CO2-based nomogram was 0.860. In the external validation group, the C-index (age, SBP, pupil size, SAH, SII, PLR, [K+], [Ca2+], INR and CRP) based on the SII nomogram was 0.907. The C index (age, SBP, pupil size, SAH, PLR, [K+], [Ca2+], INR, CRP and coSII-CO2) based on the coSII-CO2 nomogram was 0.916 (Figure 5). DCA also confirmed that the coSII-CO2-based nomogram had a higher clinical net benefit for GOS at 6 months after discharge in most cases than the SII-based nomogram, in both the training and external validation groups (Figure 6). In addition, the calibration curve based on the coSII-CO2 nomogram showed better agreement between the predicted GOS score and the actual observed results at 6 months after discharge than the calibration curve based on the SII nomogram (Figure 7).
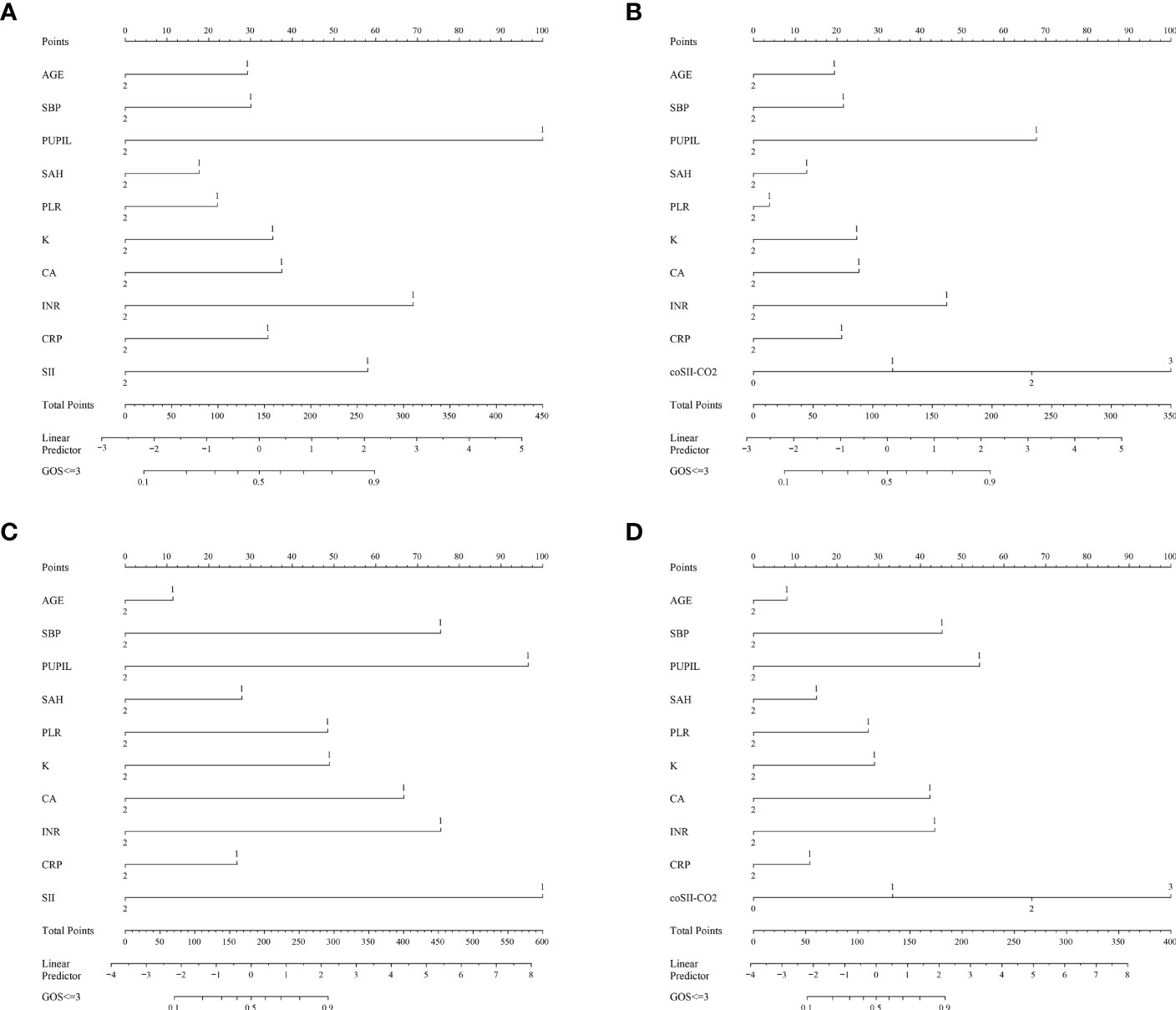
Figure 4 Establishment of the SII and coSII-CO2 nomograms in the training and validation groups. (A, B) Nomograms based on SII and coSII-CO2 in the training group. (C, D) Nomograms based on SII and coSII-CO2 in the validation group.
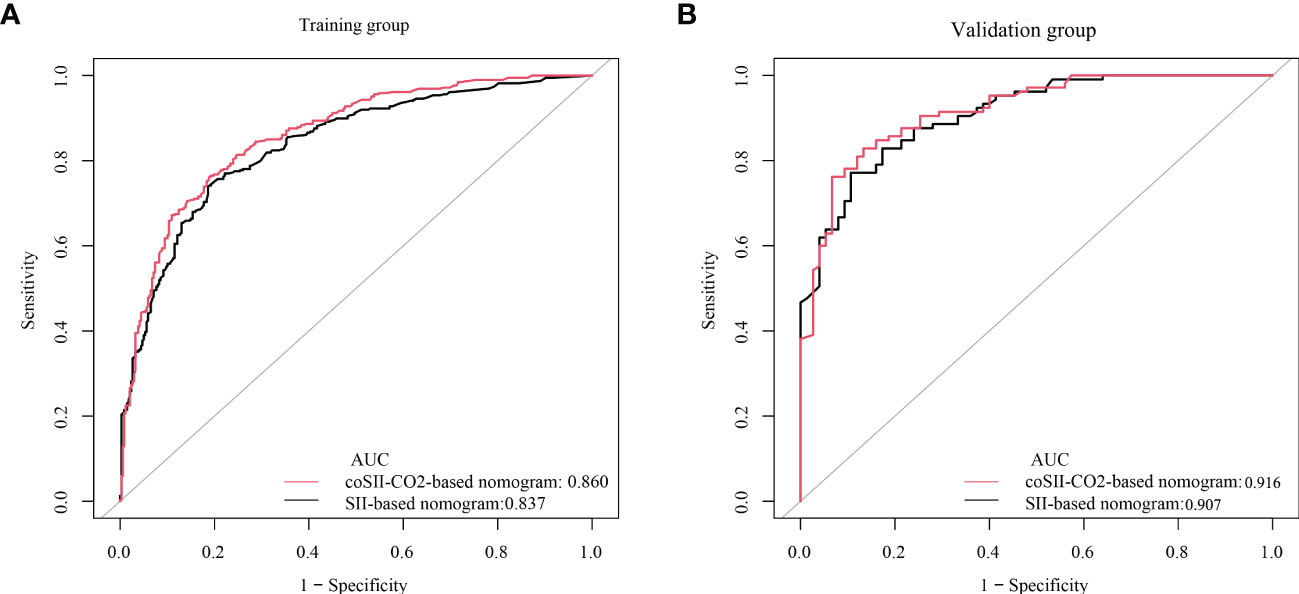
Figure 5 C-index based on SII and coSII-CO2 in the training and validation groups. (A) The C-index of SII and coSII-CO2 in the training group was 0.837 and 0.860, respectively. (B) The C-index of SII and coSII-CO2 in the validation group was 0.907 and 0.916, respectively.
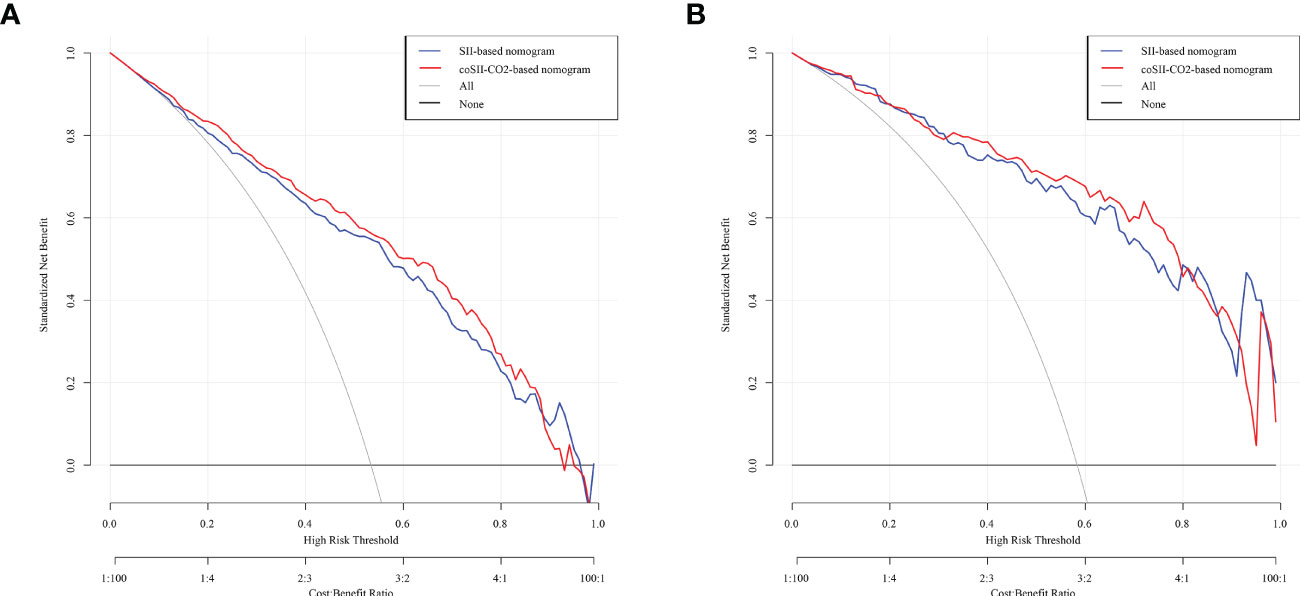
Figure 6 Decision curve analysis (DCA) based on SII and coSII-CO2 in the training and validation groups. (A) Clinical DCA of the training group. (B) Clinical DCA of the validation group.
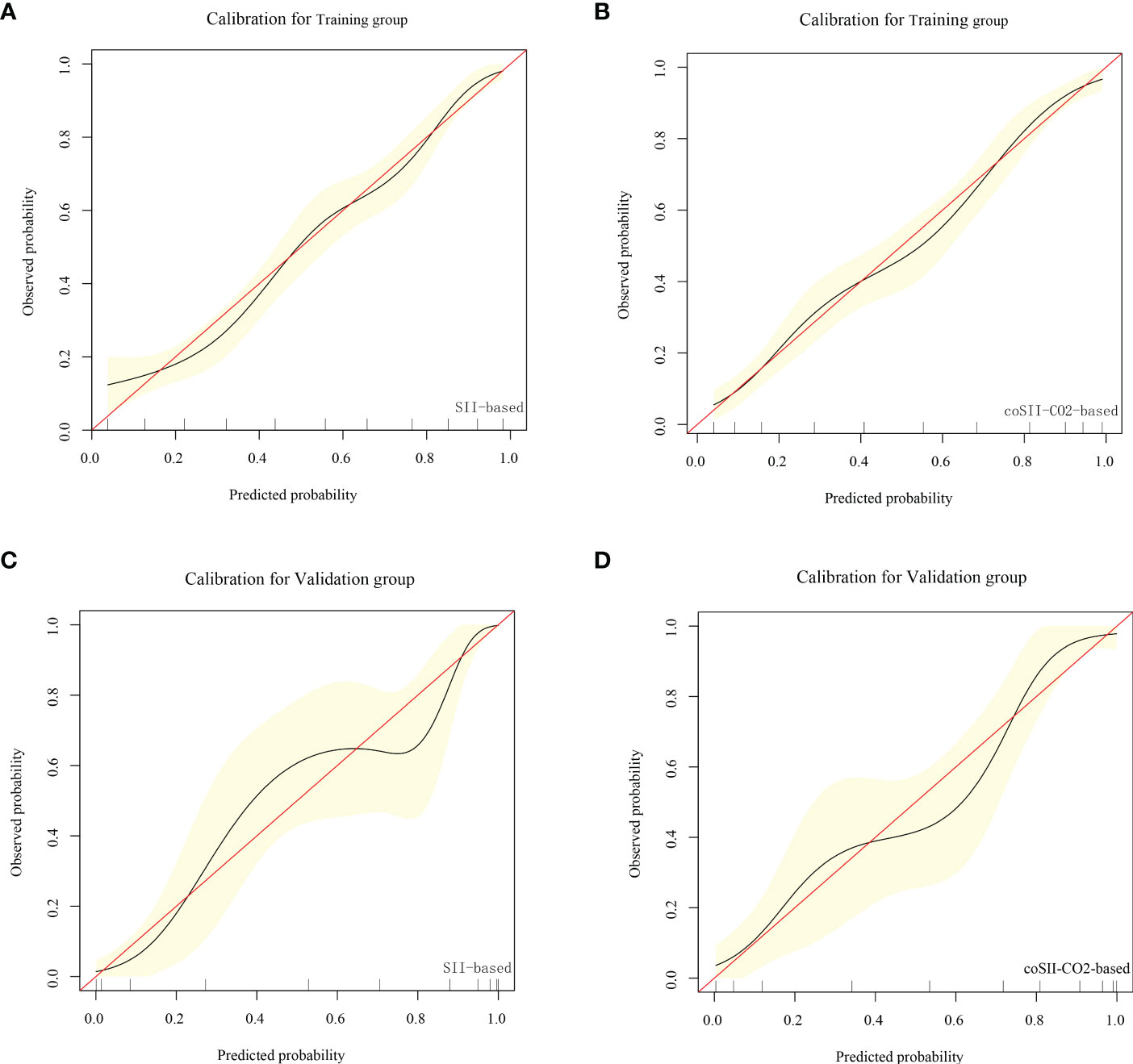
Figure 7 Calibration curve based on SII and coSII-CO2 in the training and validation groups. (A, B) Clinical calibration curve analysis of the training group. (C, D) Clinical calibration curve analysis of the validation group.
Comparison of coSII-CO2 models with conventionally based CRASH and IMPACT models
CRASH (corticosteroid randomisation after significant head injury) model and IMPACT (international mission for prognosis and prognosis clinical trial (TBI) model is a commonly used and recognized prognostic model for traumatic brain injury. Based on our data study, we compared our coSII-CO2 model with the basic CRASH and IMPACT models. The AUC values of CRASH, IMPACT and coSII-CO2 models were 0.609, 0.786 and 0.882, respectively. The conclusion is that the accuracy of our prediction model in severe craniocerebral trauma patients is higher than that of the basic CRASH and IMPACT models (Figure 8).
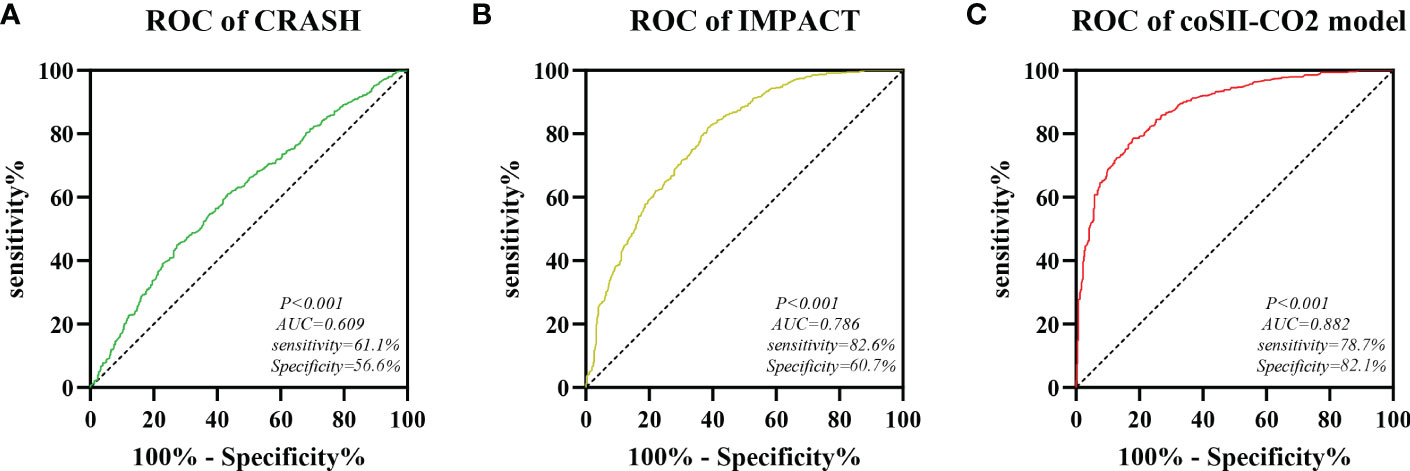
Figure 8 Comparison of predictive performance between CRASH, IMPACT and coSII-CO2 models. (A) The AUC value of CRASH is 0.609. (B) The AUC value of IMPACT is 0.786. (C) The AUC value of coSII-CO2 is 0.882.
Discussion
In this study, we evaluated the prognostic effects of inflammatory indicators at admission (SII, PLR, NLR and LMR) and the peripheral blood CO2 concentrations in patients with severe TBI, compared the prognostic accuracy of these indicators, established corresponding nomograms, and tested their predictive performance. These results may help clinicians intuitively assess individual survival outcomes in treatment and guide clinical practice. The accuracy ranking of the area under the ROC curves were as follows: coSII-CO2: 0.751 > SII: 0.676 > CO2: 0.648 > PLR: 0.636 > LMR: 0.632 > NLR: 0.617. Patients with severe TBI with high levels of SII and low levels of CO2 at admission had poorer outcomes. In addition, coSII-CO2 and SII have been shown to be independent prognostic factors for GOS in patients with severe TBI.
Neuroinflammation, which develops rapidly after TBI, has become a research hotspot in recent years. It is characterized by the activation of resident cells, migration and recruitment of peripheral inflammatory cells, and release of inflammatory mediators (10, 28). Damage to brain tissue results in the release of many endogenous factors, such as RNA, DNA, heat shock proteins and high mobility group protein B1 (HMGB1), which act as damage-associated molecular patterns, bind Toll-like receptors, and consequently activate the NF-κB and MAPK pathways. A variety of inflammatory factors are released, including cytokines (IL-1β and IL-6), chemokines and immune receptors (29, 30). Subsequently, the kinin–kallikrein system, oxidative stress response, excitatory toxicity and the innate immune system are activated through a cascade of signals, thus leading to recruitment of neutrophils, mitochondrial dysfunction and polarization of microglia. In turn, downstream pathways are triggered, thereby leading to inflammatory responses in brain tissue, oxidative stress damage and the disruption of tight junctions. The activation of matrix metallopeptidase-9 (MMP-9) during this process exacerbates the TBI-induced disruption of BBB permeability (31). Neutrophils migrate rapidly from the damaged vasculature to the corresponding cortical and hippocampal parenchyma after TBI. During this process, the migration of neutrophils is closely associated with activated endothelial cells, and the interaction between activated neutrophils and endothelial cells plays an important role in secondary injury after TBI (32). In fact, neutrophils and other immune cells secrete factors including platelet activator, leukotriene B4 and IL-18 in an autocrine manner, thus amplifying their activation. The process is difficult to stop after the danger signal is no longer present, consequently, neutrophils indiscriminately cause brain tissue damage (33, 34). Other studies have shown that the infiltration of T lymphocytes, monocytes, B cells and microglia after polarization plays a complex role in TBI, causing different effects, such as repair or aggravation of brain injury, at different periods (11, 12, 35, 36). Therefore, the infiltration of inflammatory cells after TBI and downstream reactions play indispensable roles in TBI. In our study, patients with GOS score less than or equal to 8 at admission were included, and the relationship among inflammatory factors, CO2 concentration and prognosis in patients with severe and extremely severe craniocerebral injury was emphatically studied. Few articles have conducted detailed studies on such patients.
Several recent clinical studies have shown that inflammatory indicators after TBI, such as NLR and PLR, play important roles in predicting the clinical outcomes of neurotraumatic diseases (37–41). For example, a study by Chen et al. based on 688 cases of severe head trauma showed that 508 cases (73.8%) of patients with poor prognosis 1 year after head trauma. The NLR value of the poor outcome group at admission was significantly higher than that of the good outcome group. Multivariate logistic analysis showed that the higher NLR was correlated with the adverse outcome. The NLR value of the patients at admission increased the poor prognosis and functional outcome and mortality of the patients with severe traumatic brain injury within 1 year. NLR may serve as an readily available clinical marker for preoperative prognosis in patients with severe TBI, and high NLR on admission is associated with poor prognosis in patients with neurological injury (16). Paradoxically, however a single-center study of 255 patients showed that routine blood tests including (NLR) measured at admission were not significant predictors of sTBI outcomes (42). In our study, NLR univariate analysis was significant, but after multivariate analysis, NLR was not an independent risk factor. It has been reported that SII is associated with the prognosis of patients with various tumors, such as gastric cancer, bladder cancer and rectal cancer (43–45). Some studies have also suggested that the higher the SII, the worse the prognosis of patients with cerebral hemorrhage and cerebral venous thrombosis (CVT) (46). A study of 95 patients with traumatic intracerebral hemorrhage (TICH) showed that NLR and SII were significantly correlated with GCS scores and were promising predictors of clinical outcomes in patients with TICH (47). But so far, there are no studies on the predictive effect of SII in patients with severe craniocerebral trauma. In addition, there are many emerging TBI biomarkers in the study of predicting the prognosis of TBI, for example, protein biomarkers for neuronal cell body injury (UCH-L1, NSE), astroglial injury (GFAP, S100B), neuronal cell death (alpha II-spectrin breakdown products), axonal injury (NF proteins), white matter injury (MBP), post-injury neurodegeneration (total Tau and phospho-Tau), The post-injury autoimmune response (brain antigen-targeting autoantibodies), however, these markers are still in the clinical trial stage, and not all hospitals have the ability to obtain these markers (48). In our study, SII, NLR, PLR, LMR and peripheral blood carbon dioxide concentration were used for the first time for analysis. The factors required by the study are indicators that can be detected in most hospitals, and the prediction model has good accuracy, which can be widely used in clinical practice. The prognosis of patients with severe craniocerebral injury is poor in clinic, so an accurate model is needed to predict the prognosis so as to guide our clinical work.
To our knowledge, high concentration of peripheral blood CO2 will inhibit the process of inflammation through some ways, and inflammatory factors will also affect the prognosis of patients. However, there is no report on the combination of the two. This is the first report on the clinical and prognostic value of SII combined with peripheral blood CO2 concentration in patients with TBI. In the study, we had an independent external validation group and divided the different indicators into normal and outliers to account for possible baseline inconsistencies across hospitals. In addition, in the multiple regression analysis (nomogram), different scores occupied by SII alone and SII combined with CO2 were clearly listed, and coSII-CO2 was grouped in detail for clinical reference. The results also showed that the SII combined with CO2 model had better predictive performance and better clinical benefit. First, we used ROC analysis to calculate the predictive accuracy of SII, CO2, PLR, NLR, LMR and coSII-CO2 for GOS 6 months after discharge. The AUC values were as follows: SII=0.676, CO2 = 0.648, PLR=0.636, NLR=0.617, LMR=0.623 and coSII-CO2 = 0.751. Compared with SII or CO2 alone, coSII-CO2 had the highest AUC. Patients with high SII and low CO2 had significantly poorer GOS at 6 months after discharge than patients with low SII and high CO2. Multivariate logistic regression analysis revealed that age, SBP, pupil size, SAH, SII, PLR, [K+], [Ca2+], INR, CRP and coSII-CO2 (P < 0.001) were independent prognostic factors affecting GOS in patients with severe TBI. Subsequently, 802 patients in our hospital were selected as the training group, and 207 patients in two other centers were selected as the external validation group to construct the corresponding nomograms. In the training group, the C-index based on the SII nomogram was 0.837. The C-index of the coSII-CO2 based nomogram was 0.860. In the external validation group, the C-index based on the SII nomogram was 0.907. The C-index of the coSII-CO2 based nomogram was 0.916. In addition, our DCA confirmed that coSII-CO2-based nomograms had a net clinical benefit superior to that of SII-based nomograms at 6 months after discharge in most cases. Furthermore, the coSII-CO2-based calibration curve for the probability of GOS at 6 months after discharge showed better agreement with the actual observed results than the calibration curve based on the SII nomogram. These results supported our hypothesis that the combination of SII and CO2 improves prognostic accuracy for patients with TBI.
In summary, exploration of the mechanism of inflammatory response after TBI is important for applications in patient treatment and prognostication. The SII, which combines neutrophils, lymphocytes and platelets, and reflects inflammation and poor prognosis in patients with TBI, is more reliable and representative than NLR, LMR and PLR. In our study, SII and coSII-CO2 were independent predictors of 6-month adverse outcomes in patients with TBI.
Despite demonstrating the prognostic value of SII and coSII-CO2 in patients with TBI, this study has several limitations. First, this was a retrospective analysis; therefore, several factors might have influenced the results of the study. Second, although this was a multi-center study, the number of patients was not sufficient, and this study calculated only the SII and CO2 at admission, without dynamic monitoring, which might have provided more reliable results. Finally, prospective studies are needed to confirm our conclusions.
Conclusions
In conclusion, our study shows that SII and CO2 have better predictive performance than NLR, PLR and LMR. SII and CO2 can be used as new, accurate and objective clinical predictors, and coSII-CO2, based on combining SII with CO2, can be used to improve the accuracy of GOS prediction in patients with TBI 6 months after discharge.
Data availability statement
The original contributions presented in the study are included in the article/Supplementary Material. Further inquiries can be directed to the corresponding authors.
Ethics statement
The studies involving human participants were reviewed and approved by the ethics committee of the 900th Hospital. Written informed consent for participation was not required for this study in accordance with the national legislation and the institutional requirements.
Author contributions
Conceptualization, LC. Data curation, LC, TF, and SX. Formal analysis, LC, YZ and SX. Funding acquisition, XX and SW. Methodology, LC, YL and SW. Project administration, XX and SW. Resources, LC and SX. Writing original draft, LC, QC and XQ. Writing, review and editing, XX and SW. All authors have read and agreed to the published version of the manuscript.
Funding
The present study was funded by the Joint fund project for science and technology innovation (2019Y9045).
Acknowledgments
We thank International Science Editing (http://www.internationalscienceediting.com) for editing this manuscript.
Conflict of interest
The authors declare that the research was conducted in the absence of any commercial or financial relationships that could be construed as a potential conflict of interest.
Publisher’s note
All claims expressed in this article are solely those of the authors and do not necessarily represent those of their affiliated organizations, or those of the publisher, the editors and the reviewers. Any product that may be evaluated in this article, or claim that may be made by its manufacturer, is not guaranteed or endorsed by the publisher.
References
1. Maas AIR, Menon DK, Adelson PD, Andelic N, Bell MJ, Belli A, et al. Traumatic brain injury: Integrated approaches to improve prevention, clinical care, and research. Lancet Neurol (2017) 16(12):987–1048. doi: 10.1016/S1474-4422(17)30371-X
2. Van Horn JD, Bhattrai A, Irimia A. Multimodal imaging of neurometabolic pathology due to traumatic brain injury. Trends Neurosci (2017) 40(1):39–59. doi: 10.1016/j.tins.2016.10.007
3. Carrera E, Steiner LA, Castellani G, Smielewski P, Zweifel C, Haubrich C, et al. Changes in cerebral compartmental compliances during mild hypocapnia in patients with traumatic brain injury. J Neurotrauma (2011) 28(6):889–96. doi: 10.1089/neu.2010.1377
4. Dang B, Chen W, He W, Chen G. Rehabilitation treatment and progress of traumatic brain injury dysfunction. Neural Plast (2017) 2017:1582182. doi: 10.1155/2017/1582182
5. Shi K, Zhang J, Dong JF, Shi FD. Dissemination of brain inflammation in traumatic brain injury. Cell Mol Immunol (2019) 16(6):523–30. doi: 10.1038/s41423-019-0213-5
6. Roozenbeek B, Maas AI, Menon DK. Changing patterns in the epidemiology of traumatic brain injury. Nat Rev Neurol (2013) 9(4):231–6. doi: 10.1038/nrneurol.2013.22
7. Stocchetti N, Carbonara M, Citerio G, Ercole A, Skrifvars MB, Smielewski P, et al. Severe traumatic brain injury: Targeted management in the intensive care unit. Lancet Neurol (2017) 16(6):452–64. doi: 10.1016/S1474-4422(17)30118-7
8. Blennow K, Brody DL, Kochanek PM, Levin H, McKee A, Ribbers GM, et al. Traumatic brain injuries. Nat Rev Dis Primers (2016) 2:16084. doi: 10.1038/nrdp.2016.84
9. Roozenbeek B, Lingsma HF, Lecky FE, Lu J, Weir J, Butcher I, et al. Prediction of outcome after moderate and severe traumatic brain injury: External validation of the international mission on prognosis and analysis of clinical trials (Impact) and corticoid randomisation after significant head injury (Crash) prognostic models. Crit Care Med (2012) 40(5):1609–17. doi: 10.1097/CCM.0b013e31824519ce
10. Ziebell JM, Morganti-Kossmann MC. Involvement of pro- and anti-inflammatory cytokines and chemokines in the pathophysiology of traumatic brain injury. Neurotherapeutics (2010) 7(1):22–30. doi: 10.1016/j.nurt.2009.10.016
11. Corrigan F, Mander KA, Leonard AV, Vink R. Neurogenic inflammation after traumatic brain injury and its potentiation of classical inflammation. J Neuroinflamm (2016) 13(1):264. doi: 10.1186/s12974-016-0738-9
12. Alam A, Thelin EP, Tajsic T, Khan DZ, Khellaf A, Patani R, et al. Cellular infiltration in traumatic brain injury. J Neuroinflamm (2020) 17(1):328. doi: 10.1186/s12974-020-02005-x
13. Webster KM, Sun M, Crack P, O'Brien TJ, Shultz SR, Semple BD. Inflammation in epileptogenesis after traumatic brain injury. J Neuroinflamm (2017) 14(1):10. doi: 10.1186/s12974-016-0786-1
14. Sabouri E, Majdi A, Jangjui P, Rahigh Aghsan S, Naseri Alavi SA. Neutrophil-to-Lymphocyte ratio and traumatic brain injury: A review study. World Neurosurg (2020) 140:142–7. doi: 10.1016/j.wneu.2020.04.185
15. Chen Y, Tian J, Chi B, Zhang S, Wei L, Wang S. Factors associated with the development of coagulopathy after open traumatic brain injury. J Clin Med (2021) 11(1):185. doi: 10.3390/jcm11010185
16. Chen W, Yang J, Li B, Peng G, Li T, Li L, et al. Neutrophil to lymphocyte ratio as a novel predictor of outcome in patients with severe traumatic brain injury. J Head Trauma Rehabil (2018) 33(1):E53–E9. doi: 10.1097/HTR.0000000000000320
17. Ke ZB, Chen H, Chen JY, Cai H, Lin YZ, Sun XL, et al. Preoperative abdominal fat distribution and systemic immune inflammation were associated with response to intravesical bacillus calmette-guerin immunotherapy in patients with non-muscle invasive bladder cancer. Clin Nutr (2021) 40(12):5792–801. doi: 10.1016/j.clnu.2021.10.019
18. Gao X, Pan Y, Han W, Hu C, Wang C, Chen L, et al. Association of systemic inflammation and body mass index with survival in patients with resectable gastric or gastroesophageal junction adenocarcinomas. Cancer Biol Med (2021) 18(1):283–97. doi: 10.20892/j.issn.2095-3941.2020.0246
19. Tong YS, Tan J, Zhou XL, Song YQ, Song YJ. Systemic immune-inflammation index predicting chemoradiation resistance and poor outcome in patients with stage iii non-small cell lung cancer. J Transl Med (2017) 15(1):221. doi: 10.1186/s12967-017-1326-1
20. Brandi G, Stocchetti N, Pagnamenta A, Stretti F, Steiger P, Klinzing S. Cerebral metabolism is not affected by moderate hyperventilation in patients with traumatic brain injury. Crit Care (2019) 23(1):45. doi: 10.1186/s13054-018-2304-6
21. Puppo C, Kasprowicz M, Steiner LA, Yelicich B, Lalou DA, Smielewski P, et al. Hypocapnia after traumatic brain injury: How does it affect the time constant of the cerebral circulation? J Clin Monit Comput (2020) 34(3):461–8. doi: 10.1007/s10877-019-00331-x
22. Gomez A, Froese L, Sainbhi AS, Batson C, Zeiler FA. Transcranial Doppler based cerebrovascular reactivity indices in adult traumatic brain injury: A scoping review of associations with patient oriented outcomes. Front Pharmacol (2021) 12:690921. doi: 10.3389/fphar.2021.690921
23. Carpinteri S, Sampurno S, Bernardi MP, Germann M, Malaterre J, Heriot A, et al. Peritoneal tumorigenesis and inflammation are ameliorated by humidified-warm carbon dioxide insufflation in the mouse. Ann Surg Oncol (2015) 22 Suppl 3:S1540–7. doi: 10.1245/s10434-015-4508-1
24. Wang N, Gates KL, Trejo H, Favoreto S Jr., Schleimer RP, Sznajder JI, et al. Elevated Co2 selectively inhibits interleukin-6 and tumor necrosis factor expression and decreases phagocytosis in the macrophage. FASEB J (2010) 24(7):2178–90. doi: 10.1096/fj.09-136895
25. Deng RM, Liu YC, Li JQ, Xu JG, Chen G. The role of carbon dioxide in acute brain injury. Med Gas Res (2020) 10(2):81–4. doi: 10.4103/2045-9912.285561
26. Beqiri E, Czosnyka M, Lalou AD, Zeiler FA, Fedriga M, Steiner LA, et al. Influence of mild-moderate hypocapnia on intracranial pressure slow waves activity in tbi. Acta Neurochir (Wien) (2020) 162(2):345–56. doi: 10.1007/s00701-019-04118-6
27. Oddo M, Levine JM, Kumar M, Iglesias K, Frangos S, Maloney-Wilensky E, et al. Anemia and brain oxygen after severe traumatic brain injury. Intensive Care Med (2012) 38(9):1497–504. doi: 10.1007/s00134-012-2593-1
28. Simon DW, McGeachy MJ, Bayir H, Clark RS, Loane DJ, Kochanek PM. The far-reaching scope of neuroinflammation after traumatic brain injury. Nat Rev Neurol (2017) 13(3):171–91. doi: 10.1038/nrneurol.2017.13
29. Manson J, Thiemermann C, Brohi K. Trauma alarmins as activators of damage-induced inflammation. Br J Surg (2012) 99 Suppl 1:12–20. doi: 10.1002/bjs.7717
30. Buchanan MM, Hutchinson M, Watkins LR, Yin H. Toll-like receptor 4 in cns pathologies. J Neurochem (2010) 114(1):13–27. doi: 10.1111/j.1471-4159.2010.06736.x
31. Pun PB, Lu J, Moochhala S. Involvement of ros in bbb dysfunction. Free Radic Res (2009) 43(4):348–64. doi: 10.1080/10715760902751902
32. Werner C, Engelhard K. Pathophysiology of traumatic brain injury. Br J Anaesth (2007) 99(1):4–9. doi: 10.1093/bja/aem131
33. Fortin CF, Ear T, McDonald PP. Autocrine role of endogenous interleukin-18 on inflammatory cytokine generation by human neutrophils. FASEB J (2009) 23(1):194–203. doi: 10.1096/fj.08-110213
34. Surette ME, Krump E, Picard S, Borgeat P. Activation of leukotriene synthesis in human neutrophils by exogenous arachidonic acid: Inhibition by adenosine a(2a) receptor agonists and crucial role of autocrine activation by leukotriene B(4). Mol Pharmacol (1999) 56(5):1055–62. doi: 10.1124/mol.56.5.1055
35. Zheng RZ, Lee KY, Qi ZX, Wang Z, Xu ZY, Wu XH, et al. Neuroinflammation following traumatic brain injury: Take it seriously or not. Front Immunol (2022) 13:855701. doi: 10.3389/fimmu.2022.855701
36. Das M, Mohapatra S, Mohapatra SS. New perspectives on central and peripheral immune responses to acute traumatic brain injury. J Neuroinflamm (2012) 9:236. doi: 10.1186/1742-2094-9-236
37. Zhao JL, Lai ST, Du ZY, Xu J, Sun YR, Yuan Q, et al. Circulating neutrophil-to-Lymphocyte ratio at admission predicts the long-term outcome in acute traumatic cervical spinal cord injury patients. BMC Musculoskelet Disord (2020) 21(1):548. doi: 10.1186/s12891-020-03556-z
38. Lattanzi S, Cagnetti C, Provinciali L, Silvestrini M. Neutrophil-to-Lymphocyte ratio predicts the outcome of acute intracerebral hemorrhage. Stroke (2016) 47(6):1654–7. doi: 10.1161/STROKEAHA.116.013627
39. Wang F, Wang L, Jiang TT, Xia JJ, Xu F, Shen LJ, et al. Neutrophil-to-Lymphocyte ratio is an independent predictor of 30-day mortality of intracerebral hemorrhage patients: A validation cohort study. Neurotox Res (2018) 34(3):347–52. doi: 10.1007/s12640-018-9890-6
40. Siwicka-Gieroba D, Malodobry K, Biernawska J, Robba C, Bohatyrewicz R, Rola R, et al. The Neutrophil/Lymphocyte count ratio predicts mortality in severe traumatic brain injury patients. J Clin Med (2019) 8(9):1453. doi: 10.3390/jcm8091453
41. Chen J, Qu X, Li Z, Zhang D, Hou L. Peak neutrophil-to-Lymphocyte ratio correlates with clinical outcomes in patients with severe traumatic brain injury. Neurocrit Care (2019) 30(2):334–9. doi: 10.1007/s12028-018-0622-9
42. Dolmans RGF, Hulsbergen AFC, Gormley WB, Broekman MLD. Routine blood tests for severe traumatic brain injury: Can they predict outcomes? World Neurosurg (2020) 136:e60–e7. doi: 10.1016/j.wneu.2019.10.086
43. Yatabe S, Eto K, Haruki K, Shiba H, Kosuge M, Ohkuma M, et al. Signification of systemic immune-inflammation index for prediction of prognosis after resecting in patients with colorectal cancer. Int J Colorectal Dis (2020) 35(8):1549–55. doi: 10.1007/s00384-020-03615-w
44. Li J, Cao D, Huang Y, Xiong Q, Tan D, Liu L, et al. The prognostic and clinicopathological significance of systemic immune-inflammation index in bladder cancer. Front Immunol (2022) 13:865643. doi: 10.3389/fimmu.2022.865643
45. Inoue H, Kosuga T, Kubota T, Konishi H, Shiozaki A, Okamoto K, et al. Significance of a preoperative systemic immune-inflammation index as a predictor of postoperative survival outcomes in gastric cancer. World J Surg Oncol (2021) 19(1):173. doi: 10.1186/s12957-021-02286-3
46. Trifan G, Testai FD. Systemic immune-inflammation (Sii) index predicts poor outcome after spontaneous supratentorial intracerebral hemorrhage. J Stroke Cerebrovasc Dis (2020) 29(9):105057. doi: 10.1016/j.jstrokecerebrovasdis.2020.105057
47. Defort P, Retkowska-Tomaszewska N, Kot M, Jarmuzek P, Tylutka A, Zembron-Lacny A. Inflammatory predictors of prognosis in patients with traumatic cerebral haemorrhage: Retrospective study. J Clin Med (2022) 11(3):705. doi: 10.3390/jcm11030705
Keywords: traumatic brain injury, system immune inflammation index, Glasgow Outcome Scale, prognosis, nomogram, carbon dioxide
Citation: Chen L, Xia S, Zuo Y, Lin Y, Qiu X, Chen Q, Feng T, Xia X, Shao Q and Wang S (2023) Systemic immune inflammation index and peripheral blood carbon dioxide concentration at admission predict poor prognosis in patients with severe traumatic brain injury. Front. Immunol. 13:1034916. doi: 10.3389/fimmu.2022.1034916
Received: 02 September 2022; Accepted: 22 December 2022;
Published: 09 January 2023.
Edited by:
Bing Zhao, Shanghai Jiao Tong University, ChinaReviewed by:
Weiqiang Chen, First Affiliated Hospital of Shantou University Medical College, ChinaAgnieszka Zembron-Lacny, University of Zielona Gora, Poland
Junji Wei, Peking Union Medical College Hospital (CAMS), China
Copyright © 2023 Chen, Xia, Zuo, Lin, Qiu, Chen, Feng, Xia, Shao and Wang. This is an open-access article distributed under the terms of the Creative Commons Attribution License (CC BY). The use, distribution or reproduction in other forums is permitted, provided the original author(s) and the copyright owner(s) are credited and that the original publication in this journal is cited, in accordance with accepted academic practice. No use, distribution or reproduction is permitted which does not comply with these terms.
*Correspondence: Xuewei Xia, xxw7456@163.com; Qixiang Shao, shao_qx@ujs.edu.cn; Shousen Wang, wshsen1965@126.com
†These authors have contributed equally to this work