- 1Department of Colorectal Surgery, State Key Laboratory of Oncology in South China, Collaborative Innovation Center for Cancer Medicine, Sun Yat-sen University Cancer Center, Sun Yat-sen University, Guangzhou, China
- 2Department of Medical Oncology (Key Laboratory of Cancer Prevention and Intervention, China National Ministry of Education, Key Laboratory of Molecular Biology in Medical Sciences), The Second Affiliated Hospital, Zhejiang University School of Medicine, Hangzhou, China
- 3Global Medical Affairs, MSD China, Shanghai, China
- 4Zhejiang Provincial Clinical Research Center for CANCER, Hangzhou, China
- 5Cancer Center of Zhejiang University, Hangzhou, China
Colorectal cancers (CRCs) with high microsatellite instability (MSI-H) and deficient mismatch repair (dMMR) show molecular and clinicopathological characteristics that differ from those of proficient mismatch repair/microsatellite stable CRCs. Despite the importance of MSI-H/dMMR status in clinical decision making, the testing rates for MSI and MMR in clinical practice remain low, even in high-risk populations. Additionally, the real-world prevalence of MSI-H/dMMR CRC may be lower than that reported in the literature. Insufficient MSI and MMR testing fails to identify patients with MSI-H/dMMR CRC, who could benefit from immunotherapy. In this article, we describe the current knowledge of the clinicopathological features, molecular landscape, and radiomic characteristics of MSI-H/dMMR CRCs. A better understanding of the importance of MMR/MSI status in the clinical characteristics and prognosis of CRC may help increase the rates of MMR/MSI testing and guide the development of more effective therapies based on the unique features of these tumors.
1 Introduction
Colorectal cancers (CRCs) with high microsatellite instability (MSI-H) and deficient mismatch repair (dMMR) are a unique subgroup of cancers of the colon and rectum. The molecular and clinicopathological characteristics of MSI-H/dMMR CRCs are distinct from those of proficient mismatch repair (pMMR)/microsatellite stable (MSS) CRCs (1).
Because of their unique etiology and clinicopathological characteristics, MSI-H/dMMR and pMMR/MSS CRCs respond differently to treatment (2). This is particularly true for immune checkpoint inhibition, as MSI-H/dMMR CRCs are more immunogenic and show a better response to immunotherapy than pMMR/MSS CRCs (3, 4). Recent clinical studies showed that, in patients with advanced or metastatic MSI-H/dMMR CRC, pembrolizumab treatment led to an objective response rate (ORR) of 40.0% (95% confidence interval [CI], 12.0–74.0) (5) and the ORR in patients treated with the combination of ipilimumab and nivolumab was 54.6% (95% CI, 45.2–63.8) (6). In addition, pembrolizumab led to a significantly longer progression-free survival (PFS) than chemotherapy when administered as first-line therapy for metastatic MSI-H/dMMR CRC (hazard ratio [HR] for disease progression or death, 0.10; P < 0.001), with fewer treatment-related adverse events (5). KEYNOTE-177, a phase 3 study of 307 previously untreated patients with metastatic MSI-H/dMMR CRC showed that first-line pembrolizumab was superior to chemotherapy in improving PFS (HR for progression, 0.60; 95% CI, 0.45–0.80; P = 0.0002) and ORR (43.8% [95% CI, 35.8–52.0] vs. 33.1% [95% CI, 25.8–41.1]) (7). The identification of MSI-H/dMMR as a potential biomarker for response to immunotherapy in patients with CRC has led to the initiation of various clinical trials evaluating the use of neoadjuvant immunotherapy in patients with early-stage disease. Preliminary findings from the exploratory NICHE study (NCT03026140) suggest that neoadjuvant immunotherapy with nivolumab plus ipilimumab may be a suitable regimen for patients with dMMR early-stage colon cancer (8). The ability of neoadjuvant treatment with nivolumab plus ipilimumab to improve outcomes was confirmed in patients with locally advanced dMMR colon cancer (9). Furthermore, neoadjuvant treatment with immunotherapy (nivolumab plus ipilimumab) in combination with the COX-2 inhibitor celecoxib in patients with non-metastatic dMMR CRC led to a major pathologic response in 97% of patients (95% CI, 91–100; 31 of 32) (10). The efficacy of immunotherapy in combination with other treatments (e.g., chemotherapy and radiotherapy) is also being investigated in multiple ongoing trials, including VOLTAGE-A (NCT02948348), AVANA (NCT03854799), NRG-GI002 (NCT02921256), and PANDORA (NCT04083365) (11–14). Neoadjuvant immunotherapy alone or in combination with other therapies may provide new treatment options for patients with early-stage CRC, especially in MSI-H/dMMR CRC.
Despite the importance of MSI-H/dMMR and pMMR/MSS status in clinical decision making, the rates of microsatellite instability (MSI) and mismatch repair (MMR) testing in clinical practice remain low, even in high-risk populations (15, 16). Consequently, the real-world prevalence of MSI-H/dMMR CRC may be higher than that reported in the literature. Insufficient MSI and MMR testing leads to failure to identify patients with MSI-H/dMMR CRC who could benefit from immunotherapy (5, 6). Additionally, because of differences in the epidemiological, molecular, anatomical, and histological characteristics of MSI-H/dMMR and pMMR/MSS CRCs, failure to distinguish between these subgroups may lead to discrepancies in CRC diagnostic and prognostic features (1, 17).
In this article, we comprehensively review the current knowledge of the clinicopathological characteristics, molecular landscape, and radiological findings of MSI-H/dMMR tumors among patients with CRC. This overview of the role of MMR and MSI status in CRCs could increase the understanding of MSI-H/dMMR CRCs, help clinicians identify this subgroup of patients using available approaches besides MSI/MMR testing, and guide the development of more effective therapies based on the unique molecular characteristics of these tumors.
2 Molecular mechanisms of MSI-H/dMMR in CRC
Inactivation of an MMR gene by mutation or transcriptional silencing results in deficient function of the MMR system, leading to the accumulation of errors during DNA replication (18). Multiple proteins that mediate DNA repair are involved in the MMR pathway, including the MutS family (MSH2, MSH3, and MSH6) and the MutL family (MLH1, MLH3, PMS1, and PMS2). Among these proteins, MLH1, MSH2, MSH6, and PMS2 are the most important regulators of MMR (18).
Studies have identified two distinct molecular pathways comprising germline or somatic mutations that contribute to the inactivation of MMR genes. Germline mutations in an MMR gene followed by a second hit to the wild-type copy due to point mutations, loss of heterozygosity (LOH), or methylation (18) can inactivate the gene. Inherited colorectal syndromes contribute to the development of approximately 5% of all CRCs, of which Lynch syndrome is the most common (Figure 1) (18). Mutations in MLH1 and MSH2 are found in approximately 70% of patients with Lynch syndrome, whereas mutations in MSH6 and PMS2 are less common and are found in only 15% of patients (19).
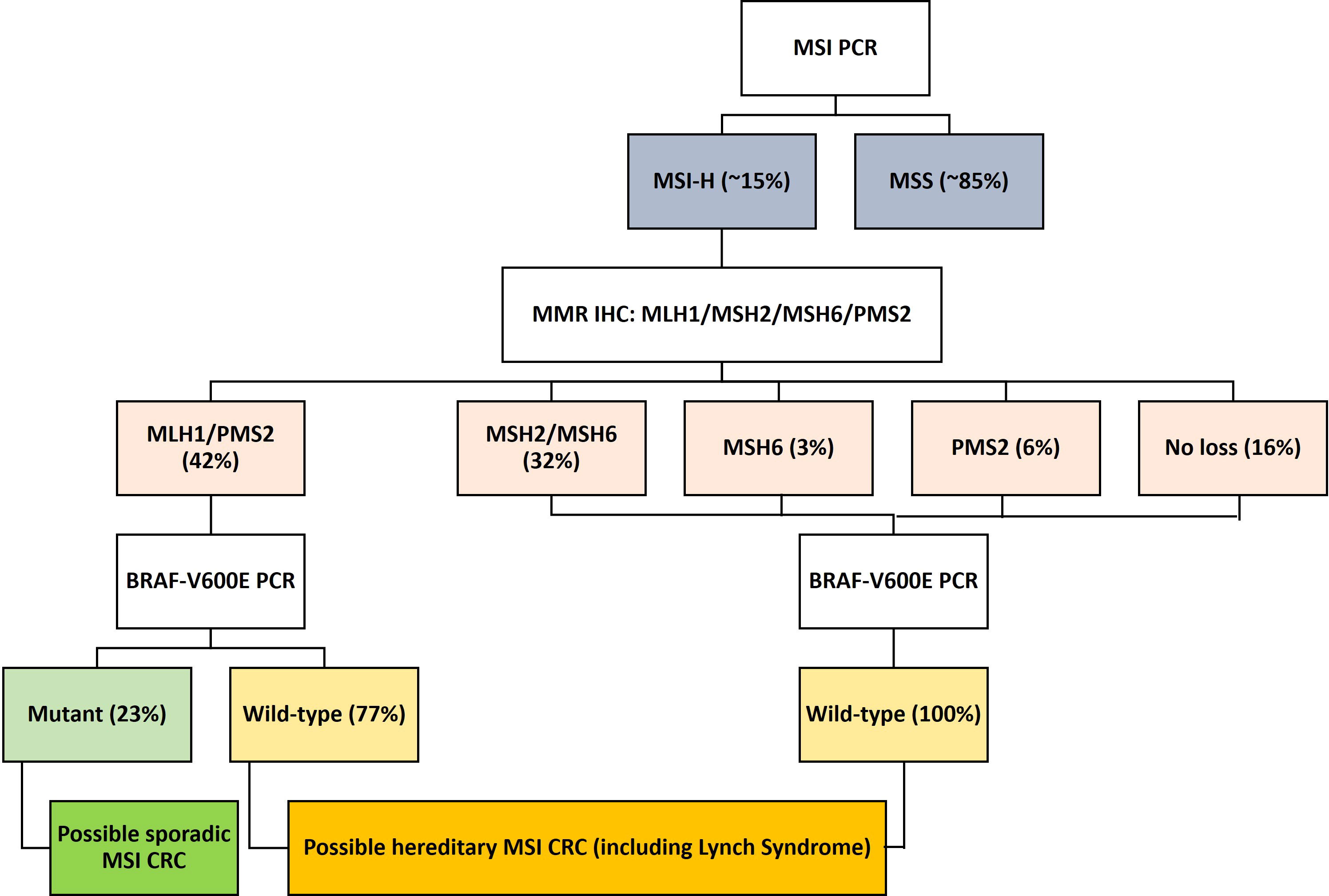
Figure 1 Flow diagram showing the molecular classification and frequency of sporadic and hereditary MSI-H/dMMR CRC. CRC, colorectal cancer; dMMR, deficient mismatch repair; MMR, mismatch repair; MSI-H, microsatellite instability-high.
DNA methylation, also referred to as CpG island methylator phenotype (CIMP), occurs in 20% of CRCs and results in a non-familial form of MSI (19). Such DNA hypermethylation results in gene silencing in most cases (19) or upregulation under certain circumstances (20, 21). Sporadic CRCs are mainly due to loss of MLH1 expression caused by hypermethylation of the MLH1 promoter in a CIMP background (19).
3 Clinical characteristics of MSI-H/dMMR CRCs
3.1 Demographic characteristics and MSI/MMR status in CRCs
Findings from multiple studies suggest that dMMR status is associated with early onset disease among patients with CRC, as dMMR CRCs are more frequent in younger patients than in older patients. A retrospective analysis of 133 patients with CRC showed that mutations in MLH1, MSH2, MSH6, and PMS2 were significantly associated with age (22). A subsequent retrospective study of 61 patients with stage I–III CRC confirmed a significant association between dMMR status and patient age (23). A recent real-world study revealed that, among patients with dMMR CRC, dMMR tumors were observed in both older (≥60 years) and younger (<50 years) patients. The frequency of MSH6/MSH2, MSH6, and PMS2 loss was higher in younger patients than in older patients. However, the statistical significance of this finding could not be determined because the expected expression values were low in >20% of the cells (24). Among patients with Lynch syndrome, the median age at CRC diagnosis was ten years higher for carriers of MSH6 mutations than for those carrying MLH1 and MSH2 mutations (25).
Similar associations have been reported for dMMR status and sex; in most studies, the percentage of women in the dMMR CRC group was higher than the percentage of men. For example, a large-scale study of 535 patients with CRC showed that tumors from women had a higher frequency of MLH1/PMS2 loss than tumors from men (26). Consistently, Viñal et al. (27) reported that the percentage of women was significantly higher among patients with dMMR CRC than among those with pMMR CRC (55% [n = 55/100] vs. 38% [n = 351/914]; P = 0.001).
3.2 Tumor characteristics and MSI/dMMR status in CRC
MSI-H/dMMR status has been associated with various CRC tumor characteristics, including the location of the primary tumor, tumor diameter, T stage, and distant metastasis. Several retrospective studies have shown a significant association between dMMR/MSI-H status and early onset disease, maximum tumor diameter, large tumor volume, primary tumor site, and advanced T stage in patients with stage (including tumor, node, metastasis [TNM] stage) I–III or I–IV CRC (23, 27–29).
A retrospective study of 245 patients with CRC showed that the incidence of MSI-H was higher in patients with right colon cancer and TNM stage I–II disease (30). Another retrospective analysis of 268 patients with CRC showed a high incidence of dMMR in patients with locally advanced (T4b) tumors without distant metastasis (31). Additionally, a recent analysis of 1,014 patients with CRC (100 [9.8%] with dMMR and 914 [90.2%] with pMMR tumors) indicated that advanced-stage tumors were significantly more common among patients with pMMR CRC than among those with dMMR CRC (stage IV: 21% vs. 3%; P < 0.001) (27). Similarly, Kang et al. (29) found a significant association between MSI-H and earlier-stage tumors in patients with CRC. These findings suggest that dMMR may play a protective role in CRC.
In a retrospective case series, Li et al. found that mutations in MLH1, MSH2, and MSH6 were significantly associated with primary tumor location among patients with dMMR CRC; hMLH1 or PMS2 loss was more common on the right side, whereas hMSH2 or hMSH6 loss was more common on the left side (22). Similarly, a retrospective analysis of 795 patients found that proximal lesions were a predictor for MSI, with a multivariate odds ratio (OR [95% CI]) of 0.419 (0.223–0.784; P = 0.007) (32).
However, Yan et al. found that larger tumor size was associated with MSI (OR [95% CI], 1.300 [1.076–1.572]; P = 0.007), as did Liang et al. (median diameters, 6.0 cm in the dMMR group compared with 4.5 cm in the pMMR group; P < 0.01) (23, 32).
3.3 Histopathological and pathomorphological characteristics of MSI-H/dMMR CRCs
MSI-H/dMMR CRCs and pMMR/MSS/MSI-L CRCs differ in their histopathological and pathomorphological characteristics. For instance, in a study of 312 patients with colorectal adenocarcinomas, mucinous adenocarcinomas were more common among patients with dMMR CRC than among those with pMMR CRC (33). Most dMMR CRCs show aggressive histological features, including an expansile growth pattern, a high degree of tumor cell infiltration, poor tumor differentiation, and a medullary pattern (34), as summarized in Figure 2. Consistently, Liang et al. (23) reported a significantly higher frequency of poorly differentiated tumors in patients with dMMR CRC than in those with pMMR CRC (41.0% [n = 25/61] vs. 10.9% [n = 20/183]; P < 0.05), although no significant differences in the rates of lymphovascular invasion and extranodal extension were observed. In contrast, localized disease at diagnosis (97% vs. 79%; P < 0.001) and histological grade 3 (20% vs. 8%; P < 0.001) were more frequent in patients with dMMR CRC than in those with pMMR CRC (27).
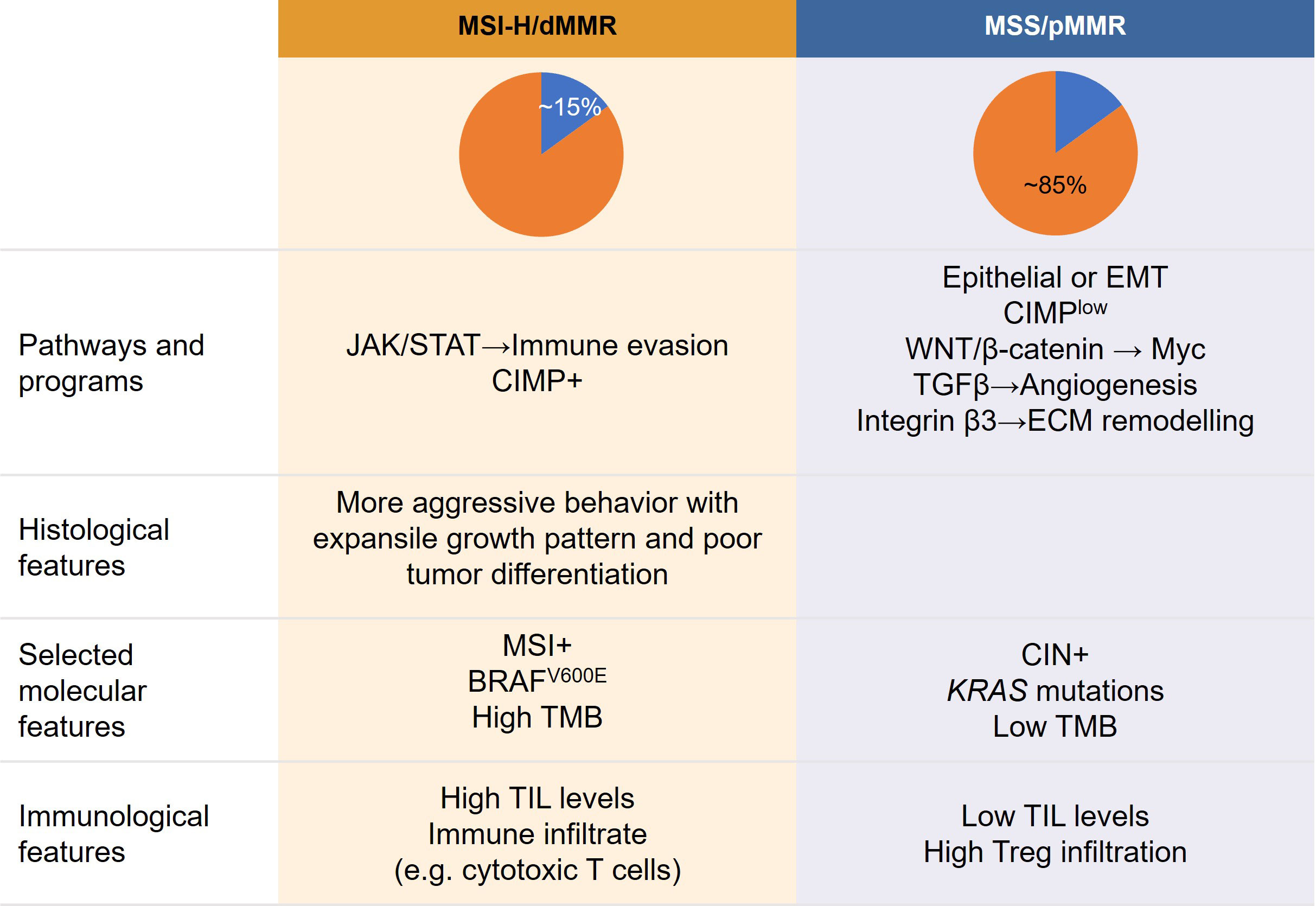
Figure 2 Proposed relationship between tumor features, molecular profiles, clinicopathological characteristics, and immunological features of colorectal cancer according to MSI subtype. CIMP, CpG island methylator phenotype; CIN, chromosomal instability; dMMR, mismatch repair deficient; ECM, extracellular matrix; EMT, epithelial–mesenchymal transition; JAK/STAT, Janus kinase/signal transducer and activator of transcription; MSI, microsatellite instability; MSS, microsatellite stable; pMMR, MMR proficient; TGF, transforming growth factor; TIL, tumor-infiltrating lymphocyte; TMB, tumor mutational burden; Tregs, T-regulatory cells; VEGF, vascular endothelial growth factor.
While both serrated and non-serrated sporadic colorectal adenocarcinomas can present MSI-H (35), studies have shown that MSI is more common in conventional carcinomas than in colorectal serrated adenocarcinomas (36). Other histological and morphological features of dMMR/MSI-H CRCs include high numbers of tumor-infiltrating lymphocytes, Crohn’s-like inflammatory reaction, mucinous/focal signet ring cell differentiation, and lack of dirty necrosis within the tumor lumen (37–39).
MSI-H/dMMR status in patients with CRC has also been correlated with high infiltration levels of immune cells, including T helper 1 (Th1) cells and cytotoxic T cells, which may explain the favorable response to immunotherapy among patients with MSI-H/dMMR tumors. The high degree of immune cell infiltration in dMMR CRCs may be attributed to the high mutational burden and neoantigen load of these tumors (3, 40), making dMMR/MSI-H CRCs amenable to immunotherapy. Despite the durable responses observed in some patients with MSI-H/dMMR CRC treated with immune checkpoint inhibitors, many CRCs are resistant to immunotherapy (41, 42). High intratumoral heterogeneity due to the high rate of mutations in MSI-H/dMMR CRCs may contribute to the generation of immune escape clones, leading to the development of immunotherapy resistance (41, 43). Although tumor mutational burden (TMB) and the expression levels of PD-1/PD-L1 have been proposed as determinants of differential responses to anti–PD-1/PD-L1 treatment among CRC patients with different MSI statuses (44), further studies are required to determine the roles of PD-1/PD-L1, BRAF/RAS mutations, TMB, and T-cell phenotype as biomarkers of response to immune checkpoint inhibitors in patients with MSI-H/dMMR CRC.
Despite the significant association between dMMR status and certain clinicopathological and tumor histologic characteristics as mentioned above, the role of MSI and MMR in clinicopathological features is complex and may be confounded by multiple factors. The higher prevalence of dMMR in earlier-stage tumors indicates a protective role of dMMR, while dMMR CRCs show aggressive histological features, including an expansile growth pattern, a high degree of tumor cell infiltration, poor tumor differentiation, and a medullary pattern.
Several factors may contribute to these paradoxical observations regarding the relationship between MSI and tumor characteristics in CRC. Most studies evaluating the relationship between MSI and tumor or clinicopathological features in patients with CRC included small cohorts because dMMR CRC is relatively rare. Additionally, there are no widely established criteria for the diagnosis of MSI-H/dMMR tumors. Variations in the evaluation of MMR status may contribute to contradictory findings regarding the predictive and prognostic roles of MSI-H/dMMR status in CRC. There is also evidence to suggest heterogeneous characteristics among the different subgroups of MSI-H/dMMR CRCs. For example, hereditary and sporadic MSI-H CRCs differ in their histological and morphological characteristics (45). Substantial racial differences in the tumor microenvironment of CRCs have also been reported (46). Moreover, because of defects in DNA repair pathways, dMMR CRCs have substantial genetic instability, which could lead to intertumoral molecular heterogeneity (17, 47). Accumulation of genetic mutations during the progression of MSI-H/dMMR CRCs can also lead to the acquisition of more aggressive features. These and other confounding factors must be accounted for in studies evaluating the role of MSI in the characteristics of CRC.
3.4 Pathomics and artificial-intelligence–assisted prediction of MSI-H/dMMR status in CRC
Advances in artificial intelligence (AI) have augmented the development of pathomics and AI-assisted methods for the characterization of tumors from patients with CRC. Most research efforts have focused on the development of models to automate the analysis and increase the accuracy of gland segmentation, tumor classification, tumor microenvironment characterization, and prognostication (48, 49).
Significant progress has also been made in the development of AI-assisted models to predict MSI status in CRC based on the distinct histomorphological features of MSI-H/dMMR tumors (Table 1) (61). For example, an open-source AI system that was trained using routine pathology slides from eight multicenter cohorts facilitated accurate and fully automated prediction of MSI status, yielding an area under the curve (AUC) value of 0.96 (95% CI, 0.94–0.98) (50). The system was successfully applied as a rule-out test to predict MSS/pMMR and identify patients with CRC for whom molecular MSI testing is not required. Similarly, Cao et al. (51) developed a pathomics-based deep-learning model trained using histological data from The Cancer Genome Atlas (TCGA) and an Asian CRC cohort. The model accurately predicted MSI status from histopathological images, with an AUC of 0.8848 (95% CI, 0.8185–0.9512) in the TCGA cohort and 0.8504 (95% CI, 0.7591–0.9323) in the Asian CRC cohort. The model accurately captured various characteristics of MSI-H tumors, including poor differentiation and high TMB (51). In an effort to improve the performance of AI algorithms in predicting MSI, Saillard et al. (52) developed a self-supervised deep-learning model that was trained using histology images from the TCGA dataset. The model predicted MSI status in CRC with high sensitivity and specificity, achieving an AUC of 0.92 (95% CI, 0.84–0.99) and outperforming previous supervised deep-learning models.
AI-assisted algorithms have also been developed to predict TMB-H status, which is strongly correlated with MSI-H/dMMR status. Shimada et al. (53) developed a convolutional neural network-based algorithm to predict TMB-H status (defined as MSI-H, high TMB, or both) from hematoxylin and eosin (H&E)-stained slides of CRC tissues. The model integrated various histomorphological features of MSI-H/dMMR CRCs, including increased lymphocytic infiltration, abundance of peritumoral lymphocytes, mucinous features, Crohn’s-like inflammatory reaction, and medullary features. The model accurately predicted TMB-H status, providing an AUC of 0.934 (range, 0.835–0.981) (53).
Current testing strategies for MSI-H/dMMR status include polymerase chain reaction (PCR), next-generation sequencing (NGS), and immunohistochemistry (IHC). High testing costs and limited resources are critical factors that hinder the wider application of MSI testing in patients with CRC. Kacew et al. (62) used a representative population-based sample of individuals receiving first-line treatment for metastatic CRC (N = 32,549) in the US to estimate the clinical and financial consequences of predicting MSI status in CRC using AI-assisted methods instead of conventional methods. Their model showed that, compared with current testing strategies, MSI testing using AI followed by confirmatory PCR or IHC testing for patients testing dMMR/MSI-H-positive by AI resulted in the lowest population-level diagnostic costs (including testing and first-line drug costs) in this cohort ($400 million [12.9%] lower than that of NGS alone). The method also maintained 91% diagnostic accuracy and facilitated timely diagnosis (62). However, a key limitation of this study was that population-based costs were estimated using a model, and no real-world validation of costs was conducted. Hence, it is possible that AI-related costs were underestimated, as additional costs of applying AI in real-world clinical settings (e.g., internal validation, maintenance of hardware and software, and scanning slides) were not taken into account. Further validation of AI-assisted MSI prediction algorithms in large datasets and real-world cohorts is required to support the clinical adoption of these models in routine practice.
3.5 Molecular characteristics and MSI/MMR status in CRCs
MSI-H tumors have high genetic instability, and dMMR/MSI-H CRCs exhibit extensive intratumoral and intertumoral molecular heterogeneity (47). A key feature of MSI-H CRCs is the lack of MMR proteins or deletions in MMR-related genes. Hence, MSI-H/dMMR CRCs can be distinguished from MSS/pMMR CRCs using IHC, PCR, or NGS. Epigenetic mechanisms (e.g., DNA methylation) also contribute to the loss of MMR proteins in dMMR/MSI-H CRC. Thus, numerous methods have been developed to detect epigenetic alterations in clinical samples from patients with CRC.
The frequencies of TP53 loss, MLH1 promoter methylation, and KRAS and BRAF mutations vary between MSI-H and MSS CRCs (Table 2) (1, 63). BRAF-V600E mutations are more common in MSI-H CRCs than in MSS CRCs. In contrast, KRAS mutations and TP53 loss are more frequent in MSS than in MSI-H CRCs (1, 63). Approximately 80% of dMMR CRCs exhibit MLH1 promoter methylation (67). Because most sporadic dMMR CRCs exhibit MLH1 promoter methylation and many also have BRAF mutations, MLH1 promoter methylation analysis can be used to discriminate sporadic tumors from Lynch syndrome in patients without BRAF mutations. Absence of BRAF mutations and MLH1 promoter methylation in tumors is associated with hereditary forms of CRC (67, 68). In contrast, the BRAF-V600E mutation in patients with dMMR CRC is strongly associated with sporadic tumors (69). Several clinicopathological characteristics, including age at diagnosis, tumor location, and patient survival, differ between patients with MLH1-deficient/BRAF-V600E–mutated dMMR CRC and those with MLH1-deficient/BRAF wild-type dMMR CRC (70, 71). Interestingly, the characteristics of BRAF-mutated MSS CRCs appear to be distinct from those of BRAF-mutated MSI-H CRCs and BRAF wild-type MSS CRCs. Landau et al. analyzed 205 CRCs and found that stage IV tumors at diagnosis were significantly more common among patients with BRAF-mutated MSS CRCs than among those with BRAF-mutated MSI-H CRCs and BRAF wild-type MSS CRCs (P < 0.001) (64). They also found that cytokeratin 7 (CK7) loss was significantly more common in BRAF-mutated MSI-H and BRAF wild-type MSS CRCs than in BRAF-mutated MSS CRCs (P = 0.0001). Furthermore, cytokeratin 20 (CK20) loss was more common in BRAF-mutated MSI-H CRCs than in BRAF-mutated MSS and BRAF wild-type MSS CRCs (P = 0.001) (64). BRAF mutations in patients with metastatic dMMR/MSI-H CRC have been associated with poor outcomes, including shorter overall survival (OS) (72).
Because MSI-H tumors are more genetically unstable than MSS tumors, MSI-H CRCs tend to accumulate mutations in various oncogenes and tumor suppressor genes, including BRAF, CTNNB1, HNF1A, PTEN, BRCA1, and BRCA2 (65, 73). Consequently, MSI-H CRCs have a higher TMB and neoantigen load than MSS CRCs (66, 74). Advances in NGS have contributed to the identification of several mutations associated with dMMR/MSI-H status in CRC. NGS analysis of tissues from 430 patients with CRC showed that mutations in MAPK pathway genes (e.g., KRAS, NRAS, BRAF) and HER2 were significantly more frequent in MSI-H CRCs than in MSS tumors (83.6% vs. 58.4%, P = 0.0003) (66).
In line with the high TMB of dMMR/MSI-H CRCs, NGS analysis of tumor samples from 64 patients with CRC showed that MSI-H tumors harbored a total of 1756 alterations (mean, 125; range 63–302) across 447 genes, whereas MSS tumors had only 493 alterations (mean, 10; range 1–26) across 186 genes (75). Among the total of 633 mutated genes, only 165 were altered in both groups. Both MSI-H and MSS tumors harbored mutations in APC, TP53, and KRAS, which are among the most frequently mutated genes in CRC. The most commonly altered genes that were mutated only in MSI-H tumors were ANKRD11 (78.6%), ARID1A (71.4%), KMT2B (71.4%), BCORL1 (64.3%), IGF1R (50.0%), KDM5 (50.0%), POLD1 (50.0%), and TSC1 (50.0%). Additionally, mutations in microsatellite loci (mononucleotide repeats) were more frequent in MSI-H CRCs than in MSS tumors.
Interestingly, the serum levels of molecular tumor markers, including CEA, CA 19-9, and CA 72-4, have also been associated with MSI/MMR status in CRC (55, 63). A retrospective analysis of samples from 2279 patients with CRC indicated that dMMR status was associated with normal CEA serum levels and elevated CA 72-4 levels (54). The use of serum CEA levels to predict dMMR status yielded AUC scores of 0.546 in the entire cohort and 0.554 in the TNM II/III subgroup. Similarly, serum CA 72-4 levels showed a modest ability to predict dMMR status, with an AUC score of 0.583 (54). Although the ability of individual serum tumor markers to predict MSI/MMR status is limited, the combination of serum markers and clinicopathological features may help identify patients with MSI-H/dMMR CRC (55). The combination of serum levels of CA 72-4 and CEA with patient age, histology type, tumor size, tumor location, degree of differentiation, lymph node metastasis, and peripheral nerve invasion to predict dMMR status in patients with CRC provided an AUC score of 0.849, which was considerably higher than the AUC scores of individual markers (54). Another retrospective analysis of 3,274 patients with CRC confirmed that the addition of CEA and CA 72-4 to dMMR prediction models significantly improved the discriminative ability of a pathology-based model in the primary (AUC: 0.805 [95% CI, 0.774–0.835] vs. 0.756 [95% CI, 0.722–0.789]; P < 0.001) and validation cohorts (AUC: 0.796 [95% CI, 0.758–0.835] vs. 0.754 [95% CI, 0.715–0.793]; P < 0.001) (55).
3.6 Radiomic characteristics of MSI-H/dMMR CRCs
Using non-invasive methods to predict MSI status prior to treatment or surgery remains an unmet clinical need. The usefulness of traditional radiological evaluation of CRCs using computed tomography (CT) to predict MSI status is limited. Therefore, novel CT technologies and radiomic features to predict MSI/MMR status in CRC have been evaluated in several studies (Table 1).
In a recent radiomics analysis of iodine-based material decomposition images captured by dual-energy CT imaging, a nomogram based on a combination of clinical factors and radiomics scores predicted MSI status in pretreatment patients with CRC (76). Preliminary findings from a retrospective study indicated that a CT-based nomogram consisting of six radiomic features and 11 clinical characteristics could predict MSI status in patients with stage II CRC, yielding an AUC of 0.752 (sensitivity, 0.663; specificity, 0.842) (56). Consistently, a multicenter study demonstrated that preoperative triphasic enhanced CT radiomics signatures consisting of 32 features could predict MSI status in 502 patients with CRC (57). Delayed-phase models were superior to arterial- or venous-phase models in predicting MSI status. Although these studies demonstrated the feasibility of using radiomic features to predict MSI status in CRC, the nomograms were developed based on data from relatively small patient cohorts.
To develop an MSI-predictive nomogram based on radiomics data from a large cohort, Pei et al. (77) used texture analytical software to extract pelvic CT radiomic features from 762 patients with CRC. Patients with MSI-H tumors showed significantly higher radiomics nomogram scores, suggesting that pretreatment radiomic features can be used as a non-invasive method to predict MSI status in patients with CRC (77). In contrast, another study involving the development and validation of a model to predict MSI status by integrating clinical, pathological, and radiomics data from a large cohort of patients with rectal cancer (n = 788) showed that the nomogram provided a moderate ability to predict MSI status. However, the model provided a higher AUC than clinical, pathological, and radiomic features alone (AUCs: 0.757, 0.584, 0.585, and 0.737, respectively) (58). In contrast to previous efforts to develop MSI-prediction models based on tumoral CT-based radiomics, Ma et al. (59) developed a machine-learning model to predict MSI status using both tumoral and peritumoral radiomic signatures. The model predicted MSI status in rectal cancer, achieving AUCs of 0.817 (95% CI, 0.772–0.856) and 0.726 (95% CI, 0.648–0.796) in the training and validation sets, respectively.
Positron emission tomography (PET)/CT has also been used as a non-invasive method to predict MSI status in patients with CRC and analysis of quantitative imaging markers via statistical modelling may further reflect pathophysiology and allow objective evaluation of tumor heterogeneity, giving more information than a single IHC/PCR assay. Li et al. (78) conducted a radiomics analysis using preoperative 18FDG PET/CT images to predict MSI/MMR status in 173 patients with CRC. They identified one PET radiomic feature (wavelet-LHH_firstorder_Skewness_PET) and one CT radiomic feature (wavelet-HHL_firstorder_RootMeanSquared_CT) that were associated with MSI status (both P < 0.05), providing a quantitative and non-invasive approach to identify patients with MSI-H/dMMR CRC (78). Metabolic parameters derived from preoperative 18FDG PET/CT images have also been found to predict MSI status in 44 patients with CRC (79). Metabolic tumor volume (MTV)30%, MTV40%, MTV50%, MTV60%, total lesion glycolysis (TLG)50%, and TLG60% differed significantly between the MSI and MSS groups (all P < 0.05). Among these parameters, MTV50% was the strongest predictor of MSI (79). Although PET is expensive and may not be readily available in all clinics, predicting MSI status using PET/CT is non-invasive and does not require tissue biopsy. Therefore, it could be adopted for patients with insufficient biopsy tissues for IHC/PCR testing or without biopsy tissue. Even though IHC is inexpensive and widely available, variations in IHC fixation and staining protocols and objectivity in scoring may influence its accuracy in identifying dMMR/MSI-H tumors.
MRI-based radiomic features have also been used to develop non-invasive models to predict MSI status in CRC. For example, Zhang et al. (60) developed a model integrating six MRI-derived radiomic features and clinical characteristics to predict MSI preoperatively in 491 patients with rectal cancer. The combined model yielded an AUC of 0.895 (95% CI, 0.838–0.938), which was significantly higher than that obtained using clinical characteristics alone (AUC: 0.685 [95% CI, 0.608–0.755]; P = 0.015).
3.7 Racial disparities in MSI-H/dMMR CRC
Racial differences in the prevalence of MSI-H/dMMR CRC have been reported (Table 3) (102–104). For example, the incidence of MSI-H CRC is relatively high among Egyptians (37%), African Americans (12%–45%), Europeans (5%–24%), and Caucasian Americans of European descent (8%–20%) (73, 80, 86–88, 105). In contrast, the reported incidence of MSI-H CRC in Asian countries is relatively low, ranging from 3.8% to 20.0% in Japan (92, 93) and from 4.5% to 15.0% in China (94, 95). Furthermore, the reported frequency of dMMR in synchronous CRCs is lower in Japanese patients than in Western patients (106).
In line with racial differences in the prevalence of MSI-H/dMMR CRC, accumulating evidence suggests that racial/ethnic disparities also exist in the genetic profiles of CRCs (102, 107, 108). Zhang et al. sequenced tumors from 1,110 Chinese patients with CRC to identify oncogenic mutations. They found that 45.4%, 3.9%, 3.1%, and 3.5% of tumors harbored mutations in KRAS, NRAS, BRAF, and PIK3CA, respectively (109). Interestingly, the frequency of the BRAF V600E mutation was 3.1%, which is lower than that reported in studies conducted in Western countries. To identify racial differences in the tumor microenvironment of colon cancers, Paredes et al. analyzed gene expression in tumor tissues in a US cohort. They found that tumors from African American patients had higher expression levels of FOXP3, IL1B, and IL8 than tumors from Caucasian Americans (all P < 0.05) (46). In contrast, tumors from Caucasian Americans had higher expression levels of markers associated with antitumor immune responses, including GZMB, IFNG, CD274 (encoding PD-L1), and CTLA4 (all P < 0.05).
The combination of non-modifiable genetic factors (e.g., family history, genetic polymorphisms) and environmental factors (e.g., diet, body weight, sedentary lifestyle, and exercise) may contribute to racial and ethnic disparities in the incidence and mortality of CRC (109–111).
3.8 Disparities of MSI-H/dMMR CRCs in hereditary vs. sporadic CRCs
Family history, age at disease onset, and prognosis are among the clinical characteristics that differ between patients with Lynch syndrome and those with sporadic MSI-H CRC. In contrast to patients with Lynch syndrome, those with sporadic MSI-H CRC often have no family history of CRC (45). Consistent with the role of germline mutations in hereditary colorectal syndromes, Lynch syndrome typically presents earlier in life than sporadic MSI-H CRCs (45, 112). Additionally, patients with stage I–III MSI CRC have a lower OS rate than those with Lynch syndrome; however, no significant differences in recurrence-free survival rates have been reported (113).
Histological and morphological differences also exist between sporadic MSI-H CRCs and Lynch syndrome. Sporadic MSI-H CRCs typically have cytoplasmic eosinophilia, and large, round, vesicular nuclei with a prominent nucleolus. In contrast, the cytological features of Lynch syndrome are similar to those of conventional adenomas (45). Furthermore, lymphocytic infiltration, tumor-cell de-differentiation, and presence of adenomas are more common in Lynch syndrome than in sporadic MSI-H CRCs. In contrast, mucin secretion, poor tumor differentiation, high intratumoral heterogeneity, Crohn’s-like reaction, glandular serration, and the presence of serrated polyps are more frequent in sporadic MSI-H CRCs than in Lynch syndrome (45, 113, 114).
Genetic factors predisposing individuals to DNA methylation may contribute to differences in clinicopathological characteristics between hereditary and sporadic MSI-H CRCs (45). Methylation of the MLH1 promoter and BRAF V600E mutations are frequently detected in sporadic MSI-H CRCs but not in patients with Lynch syndrome, suggesting that testing for BRAF mutations and MLH1 promoter methylation may help differentiate Lynch syndrome from sporadic MSI-H/dMMR CRC (115–117).
4 Conclusions
MSI CRCs possess distinct clinicopathological and molecular characteristics compared to MSS CRCs. In recent years, the development and exploration of novel testing technologies and methods, including NGS, AI-based histological algorithms, and image-based radiomic analysis, have shown promise for further defining and identifying this unique subgroup of CRCs. Combining multiple parameters with machine learning is a promising strategy to improve the performance of predictive models. The integration of histopathological and clinicopathological characteristics may improve the identification of patients with MSI-H/dMMR CRC. Progress in testing methods and predictive models has led to a deeper understanding of the disease and has important implications for patient management. The future development and utilization of these methods may hold promise for improving patient outcomes and for the development of novel therapeutics for patients with MSI-H/dMMR CRC.
Author contributions
All authors substantially contributed to planning, gathering, and interpreting the information or ideas used in the paper. During the process, they substantially contributed to providing suggestions for revision or critically reviewing subsequent iterations of the manuscript and ensured that questions related to the accuracy or integrity of any part of the work were appropriately investigated and resolved. Finally, they all reviewed and approved the final version of the paper.
Acknowledgments
Editorial assistance for this review article was provided by Christos Evangelou PhD and Jake Burrell PhD (Rude Health Consulting). This assistance was funded by MSD China.
Conflict of interest
Authors JQ and NX are employed by MSD China.
The remaining authors declare that the research was conducted in the absence of any commercial or financial relationships that could be construed as a potential conflict of interest.
Publisher’s note
All claims expressed in this article are solely those of the authors and do not necessarily represent those of their affiliated organizations, or those of the publisher, the editors and the reviewers. Any product that may be evaluated in this article, or claim that may be made by its manufacturer, is not guaranteed or endorsed by the publisher.
References
1. Kawakami H, Zaanan A, Sinicrope FA. Microsatellite instability testing and its role in the management of colorectal cancer. Curr Treat Options Oncol (2015) 16:30. doi: 10.1007/s11864-015-0348-2
2. Jin Z, Sinicrope FA. Prognostic and predictive values of mismatch repair deficiency in non-metastatic colorectal cancer. Cancers (Basel) (2021) 13;300. doi: 10.3390/cancers13020300
3. Ooki A, Shinozaki E, Yamaguchi K. Immunotherapy in colorectal cancer: Current and future strategies. J Anus Rectum Colon (2021) 5:11–24. doi: 10.23922/jarc.2020-064
4. Manz SM, Losa M, Fritsch R, Scharl M. Efficacy and side effects of immune checkpoint inhibitors in the treatment of colorectal cancer. Therap Adv Gastroenterol (2021) 14:17562848211002018. doi: 10.1177/17562848211002018
5. Le DT, Uram JN, Wang H, Bartlett BR, Kemberling H, Eyring AD, et al. PD-1 blockade in tumors with mismatch-repair deficiency. N Engl J Med (2015) 372:2509–20. doi: 10.1056/NEJMoa1500596
6. Overman MJ, Lonardi S, Wong KYM, Lenz HJ, Gelsomino F, Aglietta M, et al. Durable clinical benefit with nivolumab plus ipilimumab in DNA mismatch repair-Deficient/Microsatellite instability-high metastatic colorectal cancer. J Clin Oncol (2018) 36:773–9. doi: 10.1200/JCO.2017.76.9901
7. Andre T, Shiu KK, Kim TW, Jensen BV, Jensen LH, Punt C, et al. Pembrolizumab in microsatellite-Instability-High advanced colorectal cancer. N Engl J Med (2020) 383:2207–18. doi: 10.1056/NEJMoa2017699
8. Chalabi M, Fanchi LF, Dijkstra KK, Van den Berg JG, Aalbers AG, Sikorska K, et al. Neoadjuvant immunotherapy leads to pathological responses in MMR-proficient and MMR-deficient early-stage colon cancers. Nat Med (2020) 26:566–76. doi: 10.1038/s41591-020-0805-8
9. Chalabi M, Verschoor YL, van den Berg J. Neoadjuvant immune checkpoint inhibition in locally advanced MMR-deficient colon cancer: The NICHE-2 study. Ann Oncol (2022) 33:S1389. doi: 10.1016/j.annonc.2022.08.016
10. Verschoor YL, Berg Jvd, Beets G, Sikorska K, Aalbers A, Lent Av, et al. Neoadjuvant nivolumab, ipilimumab, and celecoxib in MMR-proficient and MMR-deficient colon cancers: Final clinical analysis of the NICHE study. J Clin Oncol (2022) 40:3511–1. doi: 10.1200/JCO.2022.40.16_suppl.3511
11. Inamori K, Togashi Y, Bando H, Tsukada Y, Fukuoka S, Suzuki A, et al. Translational research of VOLTAGE-a: Efficacy predictors of preoperative chemoradiotherapy and consolidation nivolumab in patients with both microsatellite stable and microsatellite instability-high locally advanced rectal cancer. J Clin Oncol (2021) 39:100–0. doi: 10.1200/JCO.2021.39.3_suppl.100
12. Salvatore L, Bensi M, Corallo S, Bergamo F, Pellegrini I, Rasola C, et al. Phase II study of preoperative (PREOP) chemoradiotherapy (CTRT) plus avelumab (AVE) in patients (PTS) with locally advanced rectal cancer (LARC): The AVANA study. J Clin Oncol (2021) 39:3511–1. doi: 10.1200/JCO.2021.39.15_suppl.3511
13. George TJ, Yothers G, Hong TS, Russell MM, You YN, Parker W, et al. NRG-GI002: A phase II clinical trial platform using total neoadjuvant therapy (TNT) in locally advanced rectal cancer (LARC)–first experimental arm (EA) initial results. J Clin Oncol (2019) 37:3505–5. doi: 10.1200/JCO.2019.37.15_suppl.3505
14. Tamberi S, Grassi E, Corbelli J, Papiani G, Barbera M. A., Zingaretti C, et al. A phase II study of capecitabine plus concomitant radiation therapy followed by durvalumab (MEDI4736) as preoperative treatment in rectal cancer: PANDORA study first-stage. J Clin Oncol (2021) 39:3607–7. doi: 10.1200/JCO.2021.39.15_suppl.3607
15. Shaikh T, Handorf EA, Meyer JE, Hall MJ, Esnaola NF. Mismatch repair deficiency testing in patients with colorectal cancer and nonadherence to testing guidelines in young adults. JAMA Oncol (2018) 4:e173580. doi: 10.1001/jamaoncol.2017.3580
16. Thiebault Q, Defossez G, Karayan-Tapon L, Ingrand P, Silvain C, Tougeron D. Analysis of factors influencing molecular testing at diagnostic of colorectal cancer. BMC Cancer (2017) 17:765. doi: 10.1186/s12885-017-3759-6
17. Evrard C, Tachon G, Randrian V, Karayan-Tapon L, Tougeron D. Microsatellite instability: Diagnosis, heterogeneity, discordance, and clinical impact in colorectal cancer. Cancers (Basel) (2019) 11:1567. doi: 10.3390/cancers11101567
18. Kanth P, Grimmett J, Champine M, Burt R, Samadder NJ. Hereditary colorectal polyposis and cancer syndromes: A primer on diagnosis and management. Am J Gastroenterol (2017) 112:1509–25. doi: 10.1038/ajg.2017.212
19. McCabe M, Perner Y, Magobo R, Magangane P, Mirza S, Penny C. Microsatellite instability assessment in black south African colorectal cancer patients reveal an increased incidence of suspected lynch syndrome. Sci Rep (2019) 9:15019. doi: 10.1038/s41598-019-51316-4
20. Rauluseviciute I, Drablos F, Rye MB. DNA Hypermethylation associated with upregulated gene expression in prostate cancer demonstrates the diversity of epigenetic regulation. BMC Med Genomics (2020) 13:6. doi: 10.1186/s12920-020-0657-6
21. Yang X, Han H, De Carvalho DD, Lay FD, Jones PA, Liang G. Gene body methylation can alter gene expression and is a therapeutic target in cancer. Cancer Cell (2014) 26:577–90. doi: 10.1016/j.ccr.2014.07.028
22. Li J, Xu Q, Luo C, Chen L, Ying J. Clinicopathologic characteristics of resectable colorectal cancer with mismatch repair protein defects in Chinese population: Retrospective case series and literature review. Med (Baltimore) (2020) 99:e20554. doi: 10.1097/MD.0000000000020554
23. Liang Y, Cai X, Zheng X, Yin H. Analysis of the clinicopathological characteristics of stage I-III colorectal cancer patients deficient in mismatch repair proteins. Onco Targets Ther (2021) 14:2203–12. doi: 10.2147/OTT.S278029
24. Yamada A, Matsuoka Y, Minamiguchi S, Yamamoto Y, Kondo T, Sunami T, et al. Real-world outcome of universal screening for lynch syndrome in Japanese patients with colorectal cancer highlights the importance of targeting patients with young-onset disease. Mol Clin Oncol (2021) 15:247. doi: 10.3892/mco.2021.2409
25. Watson P, Vasen HFA, Mecklin JP, Bernstein I, Aarnio M, Jarvinen HJ, et al. The risk of extra-colonic, extra-endometrial cancer in the lynch syndrome. Int J Cancer (2008) 123:444–9. doi: 10.1002/ijc.23508
26. Ye JX, Liu Y, Qin Y, Zhong HH, Yi WN, Shi XY. KRAS and BRAF gene mutations and DNA mismatch repair status in Chinese colorectal carcinoma patients. World J Gastroenterol (2015) 21:1595–605. doi: 10.3748/wjg.v21.i5.1595
27. Viñal D, Martinez-Perez D, Martínez-Recio S, Ruiz I, Jiménez Bou D, Peña J, et al. Clinicopathological characteristics and outcomes of patients with deficient mismatch repair colorectal cancer. J Clin Oncol (2022) 40:181–1. doi: 10.1200/JCO.2022.40.4_suppl.181
28. Guo TA, Wu YC, Tan C, Jin YT, Sheng WQ, Cai SJ, et al. Clinicopathologic features and prognostic value of KRAS, NRAS and BRAF mutations and DNA mismatch repair status: A single-center retrospective study of 1,834 Chinese patients with stage I-IV colorectal cancer. Int J Cancer (2019) 145:1625–34. doi: 10.1002/ijc.32489
29. Kang S, Na Y, Joung SY, Lee SI, Oh SC, Min BW. The significance of microsatellite instability in colorectal cancer after controlling for clinicopathological factors. Med (Baltimore) (2018) 97:e0019. doi: 10.1097/MD.0000000000010019
30. Zheng J, Huang B, Nie X, Zhu Y, Han N, Li Y. The clinicopathological features and prognosis of tumor MSI in East Asian colorectal cancer patients using NCI panel. Future Oncol (2018) 14:1355–64. doi: 10.2217/fon-2017-0662
31. Han K, Tang JH, Liao LE, Jiang W, Sui QQ, Xiao BY, et al. Neoadjuvant immune checkpoint inhibition improves organ preservation in T4bm0 colorectal cancer with mismatch repair deficiency: A retrospective observational study. Dis Colon Rectum (2022). doi: 10.1097/DCR.0000000000002466
32. Yan WY, Hu J, Xie L, Cheng L, Yang M, Li L, et al. Prediction of biological behavior and prognosis of colorectal cancer patients by tumor MSI/MMR in the Chinese population. Onco Targets Ther (2016) 9:7415–24. doi: 10.2147/OTT.S117089
33. Chen J, Zhou L, Gao J, Lu T, Wang J, Wu H, et al. Clinicopathological characteristics and mutation spectrum of colorectal adenocarcinoma with mucinous component in a Chinese cohort: Comparison with classical adenocarcinoma. Front Oncol (2020) 10:917. doi: 10.3389/fonc.2020.00917
34. Sacdalan DL, Garcia RL, Diwa MH, Sacdalan DB. Clinicopathologic factors associated with mismatch repair status among Filipino patients with young-onset colorectal cancer. Cancer Manag Res (2021) 13:2105–15. doi: 10.2147/CMAR.S286618
35. Tuppurainen K, Makinen JM, Junttila O, Liakka A, Kyllonen AP, Tuominen H, et al. Morphology and microsatellite instability in sporadic serrated and non-serrated colorectal cancer. J Pathol (2005) 207:285–94. doi: 10.1002/path.1850
36. Garcia-Solano J, Conesa-Zamora P, Carbonell P, Trujillo-Santos J, Torres-Moreno DD, Pagan-Gomez I, et al. Colorectal serrated adenocarcinoma shows a different profile of oncogene mutations, MSI status and DNA repair protein expression compared to conventional and sporadic MSI-h carcinomas. Int J Cancer (2012) 131:1790–9. doi: 10.1002/ijc.27454
37. Echle A, Laleh NG, Schrammen PL, West NP, Trautwein C, Brinker TJ, et al. Deep learning for the detection of microsatellite instability from histology images in colorectal cancer: A systematic literature review. ImmunoInformatics (2021) 3-4:100008. doi: 10.1016/j.immuno.2021.100008
38. Shia J, Schultz N, Kuk D, Vakiani E, Middha S, Segal NH, et al. Morphological characterization of colorectal cancers in the cancer genome atlas reveals distinct morphology-molecular associations: clinical and biological implications. Mod Pathol (2017) 30:599–609. doi: 10.1038/modpathol.2016.198
39. Greenson JK, Huang SC, Herron C, Moreno V, Bonner JD, Tomsho LP, et al. Pathologic predictors of microsatellite instability in colorectal cancer. Am J Surg Pathol (2009) 33:126–33. doi: 10.1097/PAS.0b013e31817ec2b1
40. Lizardo DY, Kuang C, Hao S, Yu J, Huang Y, Zhang L. Immunotherapy efficacy on mismatch repair-deficient colorectal cancer: From bench to bedside. Biochim Biophys Acta Rev Cancer (2020) 1874:188447. doi: 10.1016/j.bbcan.2020.188447
41. Sahin IH, Akce M, Alese O, Shaib W, Lesinski GB, El-Rayes B, et al. Immune checkpoint inhibitors for the treatment of MSI-H/MMR-D colorectal cancer and a perspective on resistance mechanisms. Br J Cancer (2019) 121:809–18. doi: 10.1038/s41416-019-0599-y
42. Gallois C, Taieb J, Sabouret A, Broudin C, Karoui M, Garinet S, et al. Upfront progression under pembrolizumab followed by a complete response after encorafenib and cetuximab treatment in BRAF V600E-mutated and microsatellite unstable metastatic colorectal cancer patient: A case report. Genes Chromosomes Cancer (2022) 61:114–8. doi: 10.1002/gcc.23012
43. Wu W, Liu Y, Zeng S, Han Y, Shen H. Intratumor heterogeneity: The hidden barrier to immunotherapy against MSI tumors from the perspective of IFN-gamma signaling and tumor-infiltrating lymphocytes. J Hematol Oncol (2021) 14:160. doi: 10.1186/s13045-021-01166-3
44. Cui G. The mechanisms leading to distinct responses to PD-1/PD-L1 blockades in colorectal cancers with different MSI statuses. Front Oncol (2021) 11:573547. doi: 10.3389/fonc.2021.573547
45. Jass JR. HNPCC and sporadic MSI-h colorectal cancer: A review of the morphological similarities and differences. Fam Cancer (2004) 3:93–100. doi: 10.1023/B:FAME.0000039849.86008.b7
46. Paredes J, Zabaleta J, Garai J, Ji P, Imtiaz S, Spagnardi M, et al. Immune-related gene expression and cytokine secretion is reduced among African American colon cancer patients. Front Oncol (2020) 10:1498. doi: 10.3389/fonc.2020.01498
47. Kim JH, Kang GH. Molecular and prognostic heterogeneity of microsatellite-unstable colorectal cancer. World J Gastroenterol (2014) 20:4230–43. doi: 10.3748/wjg.v20.i15.4230
48. Thakur N, Yoon H, Chong Y. Current trends of artificial intelligence for colorectal cancer pathology image analysis: A systematic review. Cancers (Basel) (2020) 12:1884. doi: 10.3390/cancers12071884
49. Jiang W, Mei WJ, Xu SY, Ling YH, Li WR, Kuang JB, et al. Clinical actionability of triaging DNA mismatch repair deficient colorectal cancer from biopsy samples using deep learning. EBioMedicine (2022) 81:104120. doi: 10.1016/j.ebiom.2022.104120
50. Echle A, Ghaffari Laleh N, Quirke P, Grabsch HI, Muti HS, Saldanha OL, et al. Artificial intelligence for detection of microsatellite instability in colorectal cancer-a multicentric analysis of a pre-screening tool for clinical application. ESMO Open (2022) 7:100400. doi: 10.1016/j.esmoop.2022.100400
51. Cao R, Yang F, Ma SC, Liu L, Zhao Y, Li Y, et al. Development and interpretation of a pathomics-based model for the prediction of microsatellite instability in colorectal cancer. Theranostics (2020) 10:11080–91. doi: 10.7150/thno.49864
52. Saillard C, Dehaene O, Marchand T, Moindrot O, Kamoun A, Schmauch B, et al. Self supervised learning improves dMMR/MSI detection from histology slides across multiple cancers. MICCAI 2021 COMPAY (2021).
53. Shimada Y, Okuda S, Watanabe Y, Tajima Y, Nagahashi M, Ichikawa H, et al. Histopathological characteristics and artificial intelligence for predicting tumor mutational burden-high colorectal cancer. J Gastroenterol (2021) 56:547–59. doi: 10.1007/s00535-021-01789-w
54. Zhao N, Cao Y, Yang J, Li H, Wu K, Wang J, et al. Serum tumor markers combined with clinicopathological characteristics for predicting MMR and KRAS status in 2279 Chinese colorectal cancer patients: A retrospective analysis. Front Oncol (2021) 11:582244. doi: 10.3389/fonc.2021.582244
55. Cao Y, Peng T, Li H, Yang M, Wu L, Zhou Z, et al. Development and validation of MMR prediction model based on simplified clinicopathological features and serum tumour markers. EBioMedicine (2020) 61:103060. doi: 10.1016/j.ebiom.2020.103060
56. Fan S, Li X, Cui X, Zheng L, Ren X, Ma W, et al. Computed tomography-based radiomic features could potentially predict microsatellite instability status in stage II colorectal cancer: A preliminary study. Acad Radiol (2019) 26:1633–40. doi: 10.1016/j.acra.2019.02.009
57. Cao Y, Zhang G, Zhang J, Yang Y, Ren J, Yan X, et al. Predicting microsatellite instability status in colorectal cancer based on triphasic enhanced computed tomography radiomics signatures: A multicenter study. Front Oncol (2021) 11:687771. doi: 10.3389/fonc.2021.687771
58. Yuan H, Yu P, Li J, Song N, Wan Z. A., Chen B, et al. An integrating of clinical, pathological, and radiomic approach to predict the microsatellite instability in rectal carcinoma. (2021). doi: 10.21203/rs.3.rs-957494/v1
59. Ma Y, Yuan H, Yu P, Xu X, Tu S, Wei Y. A tumoral and peritumoral CT-based radiomics and machine learning approach to predict the microsatellite instability of rectal carcinoma. Res Square (2022) 14:2409–18. doi: 10.21203/rs.3.rs-1305223/v1
60. Zhang W, Huang Z, Zhao J, He D, Li M, Yin H, et al. Development and validation of magnetic resonance imaging-based radiomics models for preoperative prediction of microsatellite instability in rectal cancer. Ann Transl Med (2021) 9:134. doi: 10.21037/atm-20-7673
61. Park JH, Kim EY, Luchini C, Eccher A, Tizaoui K, Shin JI, et al. Artificial intelligence for predicting microsatellite instability based on tumor histomorphology: A systematic review. Int J Mol Sci (2022) 23:2462. doi: 10.3390/ijms23052462
62. Kacew AJ, Strohbehn GW, Saulsberry L, Laiteerapong N, Cipriani NA, Kather JN, et al. Artificial intelligence can cut costs while maintaining accuracy in colorectal cancer genotyping. Front Oncol (2021) 11:630953. doi: 10.3389/fonc.2021.630953
63. Chang SC, Lin JK, Yang SH, Wang HS, Li AF, Chi CW. Relationship between genetic alterations and prognosis in sporadic colorectal cancer. Int J Cancer (2006) 118:1721–7. doi: 10.1002/ijc.21563
64. Landau MS, Kuan SF, Chiosea S, Pai RK. BRAF-mutated microsatellite stable colorectal carcinoma: An aggressive adenocarcinoma with reduced CDX2 and increased cytokeratin 7 immunohistochemical expression. Hum Pathol (2014) 45:1704–12. doi: 10.1016/j.humpath.2014.04.008
65. Gatalica Z, Vijayvergia N, Vranic S, Xiu J, Reddy SK, Snyder CL, et al. Therapeutic biomarker differences between MSI-h and MSS colorectal cancers. J Clin Oncol (2015) 33:3597–7. doi: 10.1200/jco.2015.33.15_suppl.3597
66. Xiao J, Li W, Huang Y, Huang M, Li S, Zhai X, et al. A next-generation sequencing-based strategy combining microsatellite instability and tumor mutation burden for comprehensive molecular diagnosis of advanced colorectal cancer. BMC Cancer (2021) 21:282. doi: 10.1186/s12885-021-07942-1
67. Maloberti T, De Leo A, Sanza V, Merlo L, Visani M, Acquaviva G, et al. BRAF and MLH1 analysis algorithm for the evaluation of lynch syndrome risk in colorectal carcinoma patients: Evidence-based data from the analysis of 100 consecutive cases. J Mol Pathol (2022) 3:115–24. doi: 10.3390/jmp3030011
68. Capper D, Voigt A, Bozukova G, Ahadova A, Kickingereder P, von Deimling A, et al. BRAF V600E-specific immunohistochemistry for the exclusion of lynch syndrome in MSI-h colorectal cancer. Int J Cancer (2013) 133:1624–30. doi: 10.1002/ijc.28183
69. Toon CW, Walsh MD, Chou A, Capper D, Clarkson A, Sioson L, et al. BRAFV600E immunohistochemistry facilitates universal screening of colorectal cancers for lynch syndrome. Am J Surg Pathol (2013) 37:1592–602. doi: 10.1097/PAS.0b013e31828f233d
70. Mahesh SA, Hanna E, Khan MS, Ravichandran P, Slezak F. Incidence and characteristics of BRAF V600E mutation in colorectal cancer (CRC) with mismatch repair (MMR) protein defect due to loss of MLH1: A prospective evaluation of 104 consecutive patients. J Clin Oncol (2011) 29:3539–9. doi: 10.1200/jco.2011.29.15_suppl.3539
71. Sahin IH, Goyal S, Pumpalova Y, Sonbol MB, Das S, Haraldsdottir S, et al. Mismatch repair (MMR) gene alteration and BRAF V600E mutation are potential predictive biomarkers of immune checkpoint inhibitors in MMR-deficient colorectal cancer. Oncologist (2021) 26:668–75. doi: 10.1002/onco.13741
72. Tan E, Whiting J, Xie H, Imanirad I, Carballido E, Felder S, et al. BRAF mutations are associated with poor survival outcomes in advanced-stage mismatch repair-deficient/Microsatellite high colorectal cancer. Oncologist (2022) 27:191–7. doi: 10.1093/oncolo/oyab055
73. Vilar E, Gruber SB. Microsatellite instability in colorectal cancer-the stable evidence. Nat Rev Clin Oncol (2010) 7:153–62. doi: 10.1038/nrclinonc.2009.237
74. Zhou Z, Li K, Wei Q, Chen L, Shuai Y, Wang Y, et al. Tumor mutation burden determined by a 645-cancer gene panel and compared with microsatellite instability and mismatch repair genes in colorectal cancer. J Gastrointest Oncol (2021) 12:2775–87. doi: 10.21037/jgo-21-572
75. Zheng K, Wan H, Zhang J, Shan G, Chai N, Li D, et al. A novel NGS-based microsatellite instability (MSI) status classifier with 9 loci for colorectal cancer patients. J Transl Med (2020) 18:215. doi: 10.1186/s12967-020-02373-1
76. Wu J, Zhang Q, Zhao Y, Liu Y, Chen A, Li X, et al. Radiomics analysis of iodine-based material decomposition images with dual-energy computed tomography imaging for preoperatively predicting microsatellite instability status in colorectal cancer. Front Oncol (2019) 9:1250. doi: 10.3389/fonc.2019.01250
77. Pei Q, Yi X, Chen C, Pang P, Fu Y, Lei G, et al. Pre-treatment CT-based radiomics nomogram for predicting microsatellite instability status in colorectal cancer. Eur Radiol (2022) 32:714–24. doi: 10.1007/s00330-021-08167-3
78. Li J, Yang Z, Xin B, Hao Y, Wang L, Song S, et al. Quantitative prediction of microsatellite instability in colorectal cancer with preoperative PET/CT-based radiomics. Front Oncol (2021) 11:702055. doi: 10.3389/fonc.2021.702055
79. Liu H, Ye Z, Yang T, Xie H, Duan T, Li M, et al. Predictive value of metabolic parameters derived from (18)F-FDG PET/CT for microsatellite instability in patients with colorectal carcinoma. Front Immunol (2021) 12:724464. doi: 10.3389/fimmu.2021.724464
80. Soliman AS, Bondy ML, El-Badawy SA, Mokhtar N, Eissa S, Bayoumy S, et al. Contrasting molecular pathology of colorectal carcinoma in Egyptian and Western patients. Br J Cancer (2001) 85:1037–46. doi: 10.1054/bjoc.2001.1838
81. Lungulescu C, Croitoru VM, Volovat SR, Cazacu IM, Turcu-Stiolica A, Gheonea DI, et al. An insight into deficient mismatch repair colorectal cancer screening in a Romanian population-a bi-institutional pilot study. Medicina (Kaunas) (2021) 57:847. doi: 10.3390/medicina57080847
82. Noepel-Duennebacke S, Juette H, Feder IS, Kluxen L, Basara N, Hiller W, et al. High microsatellite instability (MSI-h) is associated with distinct clinical and molecular characteristics and an improved survival in early colon cancer (CC); real world data from the AIO molecular registry colopredict plus. Z Gastroenterol (2020) 58:533–41. doi: 10.1055/a-1156-4433
83. Aasebo KO, Dragomir A, Sundstrom M, Mezheyeuski A, Edqvist PH, Eide GE, et al. Consequences of a high incidence of microsatellite instability and BRAF-mutated tumors: A population-based cohort of metastatic colorectal cancer patients. Cancer Med (2019) 8:3623–35. doi: 10.1002/cam4.2205
84. Koopman M, Kortman GA, Mekenkamp L, Ligtenberg MJ, Hoogerbrugge N, Antonini NF, et al. Deficient mismatch repair system in patients with sporadic advanced colorectal cancer. Br J Cancer (2009) 100:266–73. doi: 10.1038/sj.bjc.6604867
85. Loughrey MB, McGrath J, Coleman HG, Bankhead P, Maxwell P, McGready C, et al. Identifying mismatch repair-deficient colon cancer: near-perfect concordance between immunohistochemistry and microsatellite instability testing in a large, population-based series. Histopathology (2021) 78:401–13. doi: 10.1111/his.14233
86. Kumar K, Brim H, Giardiello F, Smoot DT, Nouraie M, Lee EL, et al. (V600E) and KRAS mutations in high microsatellite instability sporadic colorectal cancer in African americans. Clin Cancer Res (2009) 15:1155–61. doi: 10.1158/1078-0432.CCR-08-1029
87. Ashktorab H, Ahuja S, Kannan L, Llor X, Ellis NA, Xicola RM, et al. A meta-analysis of MSI frequency and race in colorectal cancer. Oncotarget (2016) 7:34546–57. doi: 10.18632/oncotarget.8945
88. Klaassen ZWA, Waller J, Gu L, De Hoedt AM, Wang T, Amonkar M, et al. DNA Mismatch repair and microsatellite instability in colorectal tumors: An observational study in the veterans affairs health care system. J Clin Oncol (2021) 39:e15562–2. doi: 10.1200/JCO.2021.39.15_suppl.e15562
89. Ricker CN, Hanna DL, Peng C, Nguyen NT, Stern MC, Schmit SL, et al. DNA Mismatch repair deficiency and hereditary syndromes in Latino patients with colorectal cancer. Cancer (2017) 123:3732–43. doi: 10.1002/cncr.30790
90. Rios-Valencia J, Cruz-Reyes C, Galindo-Garcia TA, Rosas-Camargo V, Gamboa-Dominguez A. Mismatch repair system in colorectal cancer. frequency, cancer phenotype, and follow-up. Rev Gastroenterol Mex (Engl Ed) (2022) 87:432–8. doi: 10.1016/j.rgmxen.2022.05.017
91. Lopez-Correa PE, Lino-Silva LS, Gamboa-Dominguez A, Zepeda-Najar C, Salcedo-Hernandez RA. Frequency of defective mismatch repair system in a series of consecutive cases of colorectal cancer in a national cancer center. J Gastrointest Cancer (2018) 49:379–84. doi: 10.1007/s12029-018-0132-1
92. Akagi K, Oki E, Taniguchi H, Nakatani K, Aoki D, Kuwata T, et al. Real-world data on microsatellite instability status in various unresectable or metastatic solid tumors. Cancer Sci (2021) 112:1105–13. doi: 10.1111/cas.14798
93. Lorenzi M, Amonkar M, Zhang J, Mehta S, Liaw K-L. Epidemiology of microsatellite instability high (MSI-h) and deficient mismatch repair (dMMR) in solid tumors: A structured literature review. J Oncol (2020) 2020:1807929. doi: 10.1155/2020/1807929
94. Zhang C, Ding H, Sun S, Luan Z, Liu G, Li Z. Incidence and detection of high microsatellite instability in colorectal cancer in a Chinese population: a meta-analysis. J Gastrointest Oncol (2020) 11:1155–63. doi: 10.21037/jgo-20-487
95. Jiang W, Sui QQ, Li WL, Ke CF, Ling YH, Liao LE, et al. Low prevalence of mismatch repair deficiency in Chinese colorectal cancers: a multicenter study. Gastroenterol Rep (Oxf) (2020) 8:399–403. doi: 10.1093/gastro/goaa006
96. Jiang W, Cai MY, Li SY, Bei JX, Wang F, Hampel H, et al. Universal screening for lynch syndrome in a large consecutive cohort of Chinese colorectal cancer patients: High prevalence and unique molecular features. Int J Cancer (2019) 144:2161–8. doi: 10.1002/ijc.32044
97. Choi YY. Genomic analysis for discovering genetic alterations in young Korean patients with double primary cancers of the stomach and colon, graduate school, yonsei university. (2019).
98. Vg DR, Nayanar SK, Gopinath V, Philip KJ, Aryadan N, Nair V, et al. Testing for microsatellite instability in colorectal cancer - a comparative evaluation of immunohistochemical and molecular methods. Gulf J Oncolog (2022) 1:70–8.
99. Ostwal V, Pande NS, Engineer R, Saklani A, deSouza A, Ramadwar M, et al. Low prevalence of deficient mismatch repair (dMMR) protein in locally advanced rectal cancers (LARC) and treatment outcomes. J Gastrointest Oncol (2019) 10:19–29. doi: 10.21037/jgo.2018.10.01
100. Paulose RR, Ail DA, Biradar S, Vasudevan A, Sundaram KR. Prognostic and predictive significance of microsatellite instability in stage II colorectal carcinoma: An 8-year study from a tertiary center in south India. Indian J Cancer (2019) 56:302–8. doi: 10.4103/ijc.IJC_365_18
101. Rai PR, Shetty N, Rai PR, Shet D, Shetty A. A study on the frequency and clinicopathological correlates of mismatch repair-deficient colorectal cancer. J Cancer Res Ther (2020) 16:S183–8. doi: 10.4103/jcrt.JCRT_526_18
102. Sung H, Ferlay J, Siegel RL, Laversanne M, Soerjomataram I, Jemal A, et al. Global cancer statistics 2020: GLOBOCAN estimates of incidence and mortality worldwide for 36 cancers in 185 countries. CA Cancer J Clin (2021) 71:209–49. doi: 10.3322/caac.21660
103. Wong MCS, Huang J, Lok V, Wang J, Fung F, Ding H, et al. Differences in incidence and mortality trends of colorectal cancer worldwide based on sex, age, and anatomic location. Clin Gastroenterol Hepatol (2021) 19:955–66.e61. doi: 10.1016/j.cgh.2020.02.026
104. Wong MC, Ding H, Wang J, Chan PS, Huang J. Prevalence and risk factors of colorectal cancer in Asia. Intest Res (2019) 17:317–29. doi: 10.5217/ir.2019.00021
105. Sougklakos I, Athanasiadis E, Boukovinas I, Karamouzis M, Koutras A, Papakotoulas P, et al. Treatment pathways and associated costs of metastatic colorectal cancer in Greece. Cost Eff Resour Alloc (2022) 20:7. doi: 10.1186/s12962-022-00339-2
106. Nakano K, Yamamoto H, Fujiwara M, Koga Y, Tsuruta S, Ihara E, et al. Clinicopathologic and molecular characteristics of synchronous colorectal carcinoma with mismatch repair deficiency. Am J Surg Pathol (2018) 42:172–82. doi: 10.1097/PAS.0000000000000947
107. Jackson CS, Oman M, Patel AM, Vega KJ. Health disparities in colorectal cancer among racial and ethnic minorities in the united states. J Gastrointest Oncol (2016) 7:S32–43. doi: 10.3978/j.issn.2078-6891.2015.039
108. Petrick JL, Barber LE, Warren Andersen S, Florio AA, Palmer JR, Rosenberg L. Racial disparities and sex differences in early- and late-onset colorectal cancer incidence, 2001-2018. Front Oncol (2021) 11:734998. doi: 10.3389/fonc.2021.734998
109. Zhang J, Zheng J, Yang Y, Lu J, Gao J, Lu T, et al. Molecular spectrum of KRAS, NRAS, BRAF and PIK3CA mutations in Chinese colorectal cancer patients: analysis of 1,110 cases. Sci Rep (2015) 5:18678. doi: 10.1038/srep18678
110. Ollberding NJ, Nomura AM, Wilkens LR, Henderson BE, Kolonel LN. Racial/ethnic differences in colorectal cancer risk: The multiethnic cohort study. Int J Cancer (2011) 129:1899–906. doi: 10.1002/ijc.25822
111. Carethers JM, Doubeni CA. Causes of socioeconomic disparities in colorectal cancer and intervention framework and strategies. Gastroenterology (2020) 158:354–67. doi: 10.1053/j.gastro.2019.10.029
112. Son IT, Kim DW, Kim MH, Shin YK, Ku JL, Oh HK, et al. Comparison of oncologic outcomes between patients with lynch syndrome and sporadic microsatellite instability-high colorectal cancer. Ann Surg Treat Res (2021) 101:13–9. doi: 10.4174/astr.2021.101.1.13
113. Nakayama Y, Iijima T, Inokuchi T, Kojika E, Takao M, Takao A, et al. Clinicopathological features of sporadic MSI colorectal cancer and lynch syndrome: a single-center retrospective cohort study. Int J Clin Oncol (2021) 26:1881–9. doi: 10.1007/s10147-021-01968-y
114. Peng J, Xiao-ming M, Jian-qiu S, Zi-tao W, Lei F, He-juan A, et al. Clinicopathological features of non-familial colorectal cancer with high-frequency microsatellite instability. Chin Med Sci J (2010) 25:228–32. doi: 10.1016/S1001-9294(11)60007-2
115. Leclerc J, Vermaut C, Buisine MP. Diagnosis of lynch syndrome and strategies to distinguish lynch-related tumors from sporadic MSI/dMMR tumors. Cancers (Basel) (2021) 13:467. doi: 10.3390/cancers13030467
116. Sato K, Kawazu M, Yamamoto Y, Ueno T, Kojima S, Nagae G, et al. Fusion kinases identified by genomic analyses of sporadic microsatellite instability-high colorectal cancers. Clin Cancer Res (2019) 25:378–89. doi: 10.1158/1078-0432.CCR-18-1574
117. Cohen R, Buhard O, Cervera P, Hain E, Dumont S, Bardier A, et al. Clinical and molecular characterisation of hereditary and sporadic metastatic colorectal cancers harbouring microsatellite instability/DNA mismatch repair deficiency. Eur J Cancer (2017) 86:266–74. doi: 10.1016/j.ejca.2017.09.022
Keywords: colorectal cancer, high microsatellite instability, deficient mismatch repair, microsatellite instability, characteristics, prognosis, therapy development, review
Citation: Mei W-J, Mi M, Qian J, Xiao N, Yuan Y and Ding P-R (2022) Clinicopathological characteristics of high microsatellite instability/mismatch repair-deficient colorectal cancer: A narrative review. Front. Immunol. 13:1019582. doi: 10.3389/fimmu.2022.1019582
Received: 15 August 2022; Accepted: 28 November 2022;
Published: 23 December 2022.
Edited by:
Michele Ghidini, IRCCS Ca ‘Granda Foundation Maggiore Policlinico Hospital, ItalyReviewed by:
Mohd Farhan, King Faisal University, Saudi ArabiaYoutao Lu, University of Pennsylvania, United States
Daiwei Wan, The First Affiliated Hospital of Soochow University, China
Zheng Liu, National Cancer Center of China, China
Gabriel B. K. Sasa, Tianjin University, China
Copyright © 2022 Mei, Mi, Qian, Xiao, Yuan and Ding. This is an open-access article distributed under the terms of the Creative Commons Attribution License (CC BY). The use, distribution or reproduction in other forums is permitted, provided the original author(s) and the copyright owner(s) are credited and that the original publication in this journal is cited, in accordance with accepted academic practice. No use, distribution or reproduction is permitted which does not comply with these terms.
*Correspondence: Ying Yuan, yuanying1999@zju.edu.cn; Pei-Rong Ding, dingpr@sysucc.org.cn
†These authors have contributed equally to this work and share first authorship