- 1Laboratory of Clinical and Experimental Immunology, Infant Center, School of Health Science, Pontifical Catholic University of Rio Grande do Sul (PUCRS), Porto Alegre, Brazil
- 2Kavraki Lab, Department of Computer Science, Rice University, Houston, TX, United States
- 3Antunes Lab, Department of Biology and Biochemistry, University of Houston, Houston, TX, United States
- 4School of Technology - Pontifical Catholic University of Rio Grande do Sul (PUCRS), Porto Alegre, Brazil
- 5Laboratório de alto desempenho – Centro de Apoio ao desenvolvimento cientifico e tecnológico da (IDEIA), Pontifical Catholic University of Rio Grande do Sul (PUCRS), Porto Alegre, Brazil
Although not being the first viral pandemic to affect humankind, we are now for the first time faced with a pandemic caused by a coronavirus. The Severe Acute Respiratory Syndrome Coronavirus 2 (SARS-CoV-2) has been responsible for the COVID-19 pandemic, which caused more than 4.5 million deaths worldwide. Despite unprecedented efforts, with vaccines being developed in a record time, SARS-CoV-2 continues to spread worldwide with new variants arising in different countries. Such persistent spread is in part enabled by public resistance to vaccination in some countries, and limited access to vaccines in other countries. The limited vaccination coverage, the continued risk for resistant variants, and the existence of natural reservoirs for coronaviruses, highlight the importance of developing additional therapeutic strategies against SARS-CoV-2 and other coronaviruses. At the beginning of the pandemic it was suggested that countries with Bacillus Calmette-Guérin (BCG) vaccination programs could be associated with a reduced number and/or severity of COVID-19 cases. Preliminary studies have provided evidence for this relationship and further investigation is being conducted in ongoing clinical trials. The protection against SARS-CoV-2 induced by BCG vaccination may be mediated by cross-reactive T cell lymphocytes, which recognize peptides displayed by class I Human Leukocyte Antigens (HLA-I) on the surface of infected cells. In order to identify potential targets of T cell cross-reactivity, we implemented an in silico strategy combining sequence-based and structure-based methods to screen over 13,5 million possible cross-reactive peptide pairs from BCG and SARS-CoV-2. Our study produced (i) a list of immunogenic BCG-derived peptides that may prime T cell cross-reactivity against SARS-CoV-2, (ii) a large dataset of modeled peptide-HLA structures for the screened targets, and (iii) new computational methods for structure-based screenings that can be used by others in future studies. Our study expands the list of BCG peptides potentially involved in T cell cross-reactivity with SARS-CoV-2-derived peptides, and identifies multiple high-density “neighborhoods” of cross-reactive peptides which could be driving heterologous immunity induced by BCG vaccination, therefore providing insights for future vaccine development efforts.
Introduction
Since March 2020, the coronavirus disease 2019 (COVID-19) pandemic caused by the Severe Acute Respiratory Syndrome Coronavirus 2 (SARS-CoV-2) has resulted in more than 5 million deaths globally (1). The virus is highly transmissible and infects mainly cells that express ACE2 receptors (2). The symptoms of COVID-19 vary widely, but typically include fever, dry cough, fatigue, and dyspnea (3). The eldelry and individuals with co-morbidities are at higher risk of severe disease, respiratory failure, and death. Several vaccines have been already approved, including vaccines based on messenger RNA, attenuated adenovirus, and inactivated virus (4). Despite the success of COVID-19 vaccines, mistrust and misinformation from segments of society, lack of resources in low-income countries, and impaired international coordination are all contributing to very limited vaccination coverage worldwide, a picture that will not change in the short-term. In addition, there is an increasing concern with new SARS-CoV-2 variants and the long-term effectiveness of the currently approved vaccines (5, 6).
The Bacillus Calmette-Guérin (BCG) is an old, live attenuated strain of Mycobacterium bovis. The BCG was originally formulated as a vaccine by Albert Calmette and Camille Guérin to prevent tuberculosis (7). This vaccine is safe, recommended for newborn children, and widely used in many countries (8). Interestingly, BCG vaccination has been reported to promote non-specific protection against other bacteria and viruses (9–12). Different mechanisms have been associated with this protection, also known as heterologous immunity (13), including epigenetics and trained immunity (14–16). Studies of heterologous immunity, as well as epidemiological data from vaccination studies, suggest that both infections and vaccines play a role in educating the immune system and that an optimal vaccination strategy can be beneficial to immune system maturation (17). In this context, several studies considered whether the BCG vaccine could provide partial protection against SARS-CoV-2 infection. Currently, there are 28 registered clinical trials testing the efficacy of BCG in attenuating COVID-19 (18). Several epidemiology and ecologic studies have been conducted to associate the vaccination of BCG with protection to COVID-19. However the data are controversial (11, 19–29). Studies comparing the mortality rates between different countries presented a drawback related to the inaccuracy of the reported mortality data in some countries. One epidemiologic study pointed out that BCG may offer some protection against COVID-19 (30). Still, the data should be interpreted with caution as it may depend on the time of pandemic and the age structure of the population vaccinated with BCG. A Phase III randomized clinical trial confirmed that recent vaccination with BCG protects the elderly against new respiratory infections (31). Also, booster BCG vaccination in high-risk healthcare workers was shown to prevent COVID-19 (32). Accordingly, in the mice model, BCG administration can protect from SARS-CoV-2 infection (33).
It is known that the individual repertoire of induced T cells (i.e., private specificity), shaped by previous infections and vaccinations, determines immunopathology and heterologous immunity (34). Therefore, the protection of BCG against COVID-19 might be mediated by cross-reactive T cells. T cells recognize peptides displayed by class I Human Leukocyte Antigen (HLA-I) receptors, and a recent study analyzing HLA-I-restricted peptides has shown sequence similarity between BCG and SARS-CoV-2 epitopes (35). Also, the study of Eggenhuizen et al., identified 8 BCG vaccine-derived peptides with sequence homology to SARS-CoV-2 NSP3-derived peptides (36). In a study with 20 individuals, they found that cells primed with BCG peptides developed enhanced T cell reactivity to 7 SARS-CoV-2 peptides (36). T cell cross-reactivity is not an uncommon event and was already described in the context of coronavirus infection (37). The hypothesis that cross-reactive T cells primed with BCG may be involved in the response against SARS-CoV-2 has already been discussed, and supported by in silico studies focused on peptide sequence similarity (38). However, T cell cross-reactivity is not determined only by sequence similarity. Recent studies have demonstrated that T cell cross-reactivity is also determined by structural similarities of unrelated peptide-HLA (pHLA) complexes (39–41).
In the present study we aimed at performing a structure-based screening for potential cross-reactive HLA-I-restricted peptides from BCG and SARS-CoV-2. We performed a large-scale proteome analysis and identified thousands of possible HLA-I binders. We modeled these peptides in the context of different HLAs, and used a large-scale image-based analysis to compute similarity between pHLA complexes, while accounting for biochemical and structural properties. This analysis produced a short list of immunogenic BCG-derived peptides that are the most likely primers for T cell cross-reactivity against SARS-CoV-2. It also produced a longer list of cross-reactivity clusters involving one SARS-CoV-2 peptide and multiple BCG-targets, in some cases binding multiple HLA-I alleles, which could represent interesting targets for vaccine development.
Material And Methods
Peptide and HLA Selection
We obtained the proteomes from BCG-Pasteur strain (ID: UP000032723, hereafter called BCG) and SARS-CoV-2 (ID: UP000464024, hereafter also called SARS) from the UniProtKB database. We applied a sliding window method on each protein aiming to generate all possible peptides with 9 amino acids in length (redundant peptides were removed). The HLAs were selected according to prevalence in the human population and information on literature about SARS-CoV-2 related HLAs (http://pypop.org/popdata/) (42). We end up with a total of 10 HLAs: HLA-A*01:01, HLA-A*02:01, HLA-A*02:02, HLA-A*11:01, HLA-A*24:02, HLA-B*07:02, HLA-B*15:03, HLA-B*35:01, HLA-B*40:01, and HLA-B*51:01.
Filtering by HLA Binding
We filtered peptides according to HLA-I binding affinity. This step defined the set of pHLA complexes that should be modeled in the next phase. The implementation of this phase uses the HLA-Arena tool (43). After setting up our environment, we implemented different processing pipelines, each one on a separate python script, using as reference the “virtual screening” notebook from HLA-Arena (i.e., 3_virtual_screening.ipynb). We integrated individual python scripts into a single script (i.e., run.sh) to parallelize the jobs. This script uses as input (i) 10 HLA-I alleles, (ii) 9,814 peptides from SARS-CoV-2, and (iii) 1,242,895 peptides from BCG as fasta/regular text files. After that, we first generate a list of all possible pHLA complexes and run the MHCflurry (44), generating a list of pHLAs with the respective peptide binding affinity. Secondly, we filter only the set of complexes that present a binding affinity equal to or lower than 500 nM. We set this threshold aiming to recover only strong and intermediate HLA-I binders. There is also evidence that there is a correlation between peptides below this threshold and immunogenicity (45). This set of complexes is selected to be modeled in the next phase. The result is stored in 28 files for each combination between the list of alleles and BCG or SARS-CoV-2 peptides (e.g., HLA-A0101_BCGpeps, HLA-A0101_SARSpeps; HLA-B0702_BCGpeps, HLA-B0702_SARSpeps). All scripts and files were made freely available in GitHub and more information can be found in Supplementary Data.
Structural Modeling
The structural modeling of the selected pHLAs also uses the HLA-I Arena tool and is based on the “virtual screening” notebook implemented in the tool. The phase is divided into three steps. The first step uses the MODELLER tool (46) to create an HLA-I structure for each of the 10 HLA-I alleles selected. The second step models the pHLA using the APE-Gen tool (47). The third step involves the management of big data, because we needed to parallelize the whole process in a limited number of machines. To manage the execution of all batches and handle file transfers between machines, we developed a scheduler in Python called ArenaDispatcher (see Supplementary Data). The ArenaDispatcher uses the Docker API to communicate with docker daemons on either local or remote machines and can schedule jobs in the form of containers to run on one of the connected docker daemons. The ArenaDispatcher also monitors the execution state of each job, schedules in accordance to a maximum (or unlimited) amount of available resources for each docker host, and retrieves data (files and directories) and execution logs (i.e., stdout and stderr). We also included problem circumvention functionalities such as rescheduling jobs of unavailable hosts, reconnecting to previously unavailable hosts, and a local log file for the dispatcher’s consistency (in case of a crash, it can reload the information of jobs completed, running, or not yet dispatched). By doing this, associated with cloud resources (i.e., Amazon Web Services acquired utilizing a grant from Brazilian National Research and Education Network), we reduced the time of simulation from 7 years to approximately 60 days.
Generation and Extraction of Surface Data From pHLA Complexes
The PyMOL tool (https://pymol.org) was used to generate a visual representation of the TCR-interacting surface electrostatic potential of each pHLA complex. A script was developed to automate this process for each PDB file, exporting the result in the format of PNG images after the alignment of the complex from a pivot point, assuring all pHLA complexes would be in the same orientation (i.e., same x, y, z coordinates). The ImageJ tool (https://imagej.nih.gov/ij/) was used to obtain the histogram containing RGB values (i.e., mean and standard deviation) of 46 regions of interest (Figure 2A) in each of these images. Given the large number of images and the ImageJ limitation in obtaining the histogram of one region at a time, we developed a plugin in Java (see histogram2csv in Supplementary Material). The plugin receives an image and a Region of Interest (ROI) Set, and generates the histogram for each region of interest through command line. The result is exported in a CSV format file, where each line corresponds to a region of interest and each column corresponds to information from the histogram of that region.
Hierarchical Clustering Analysis
To evaluate the potential of cross-reactivity between pHLA complexes, the electrostatic potential of the TCR-interacting surface was submitted to a hierarchical clustering analysis, similar to what has been done in (48). Briefly, after modeling the pHLA with PyMOL and extracting the RGB values of each complex, we used pvclust (version 2.2) (49) and fastcluster (version 1.1.25) packages (50) from R software (version 3.6.1) (https://www.r-project.org/) (51) to perform a hierarchical clustering analysis. To perform the clustering we used the pvclust parameters method.hclust = “single” and method.dist = “correlation”. The single linkage method was used to reduce memory usage and computational time. The procedure was replicated 1,000 (default nboot value for pvclust) times per HLA-I allele.
Biochemical Properties Analysis
Peptide features can be grouped upon residue physical and chemical similarities. To sample the most similar SARS-CoV-2 and BCG peptides in our analysis, we filtered candidates based on amino acid size, hydrogen bond donors and acceptors, charge, aliphaticity, and aromaticity. In short, our strategy compares a weight vector related to biochemical properties for SARS (query) and BCG (subject) peptides. As a result, a peptide similarity ranking is produced spanning the whole dataset of SARS-CoV-2 and BCG pairs for each HLA-I allele. Finally, the peptide ranking per allele was filtered and we considered 10,000 pairs for downstream analysis.
Filtering Putative Cross-Reactive Clusters
Dendrograms are broad structures that report several clusters in distinct levels. The closest levels are related to clusters with higher similarity, i.e., in our case indicate putative cross-reactions. To filter closest cross-reactive clusters, we adopt a depth-first search (DFS) approach to explore the adjacent branches in each HLA-I allele data. Firstly, the dendrograms were converted to a graph-based structure. Next, we develop a DFS-based algorithm that identifies the closest clusters using the SARS-CoV-2 peptides as a seed node. The search depth was arbitrarily limited to five branches or bipartitions (d <= 5). The DFS algorithm was developed in R using functions derived from igraph version 1.2.6 (52) and ape packages version 5.5 (53).
Cluster Prioritization and Experimental Evidence
To provide additional information, experimental data on SARS-CoV-2 peptides were integrated into cross-reactive clusters. The data was retrieved from IEDB (iedb.org) and we used filters to recover only HLA-I peptides that were recognized by T cells (i.e., immunogenic peptides) in humans. Peptide immunogenicity data were used to select the interaction pairs among all HLA-I alleles.
Computational Resources and Data Availability
The HLA-I filtering and modeling processes were executed in a single machine composed of 24 cores and 192 GBytes of memory. Each core uses hyper-threading, which provides two virtual (logical) cores (or execution threads). Therefore, the cluster has in total 192 virtual cores for processing. Analyzing the resource utilization of APE-Gen and aiming for the best parallelization, we used containers configured with 6 virtual cores, resulting in 32 containers in the cluster and 100 complexes per execution. To accelerate the performance, we used virtual machines from Amazon Web Services acquired utilizing a grant from RNP (Brazilian National Research and Education Network) dedicated for COVID-19 research. Each virtual machine was configured with 8 cores and 15 GB of memory (c4.2xlarge instance). We used 8 virtual machines. In the end, using local and cloud resources, we concluded the modeling of all complexes in approximately 60 days. The final result was 358,386 pdb files representing the modeled complexes. Each pdb file has approximately 480Kbytes, giving a total of 160 GBytes. For the image analysis we generated 358,383 png files (three pHLAs caused PyMol to fail and crash) occupying 257 GBytes of storage space. After concluding the surface generation with PyMol, ImageJ plugin processed sequentially every png file in approximately 12 hours, storing the extracted information on CSV (comma-separated values) files (each one with 12 KBytes) requiring 4,3 GBytes of storage space. The complete list of modelled pHLAs, fasta files, png files, plugins, and computational scripts are available at https://github.com/LAD-PUCRS/Arena_SARS-BCG. The structural data is also available upon request.
Results
We have implemented a new computational strategy to select putative cross-reactive peptides between BCG and SARS-CoV-2. Starting from the entire proteome of both viruses, our methodology allowed for the identification of 40 pairs of peptides with higher probability to trigger cross-reactive T cell responses. These sequences were selected through a series of filters considering affinity to multiple prevalent class I HLA-I alleles, and pHLA similarity in terms of biochemical and structural features. The entire workflow is summarized in Figure 1.
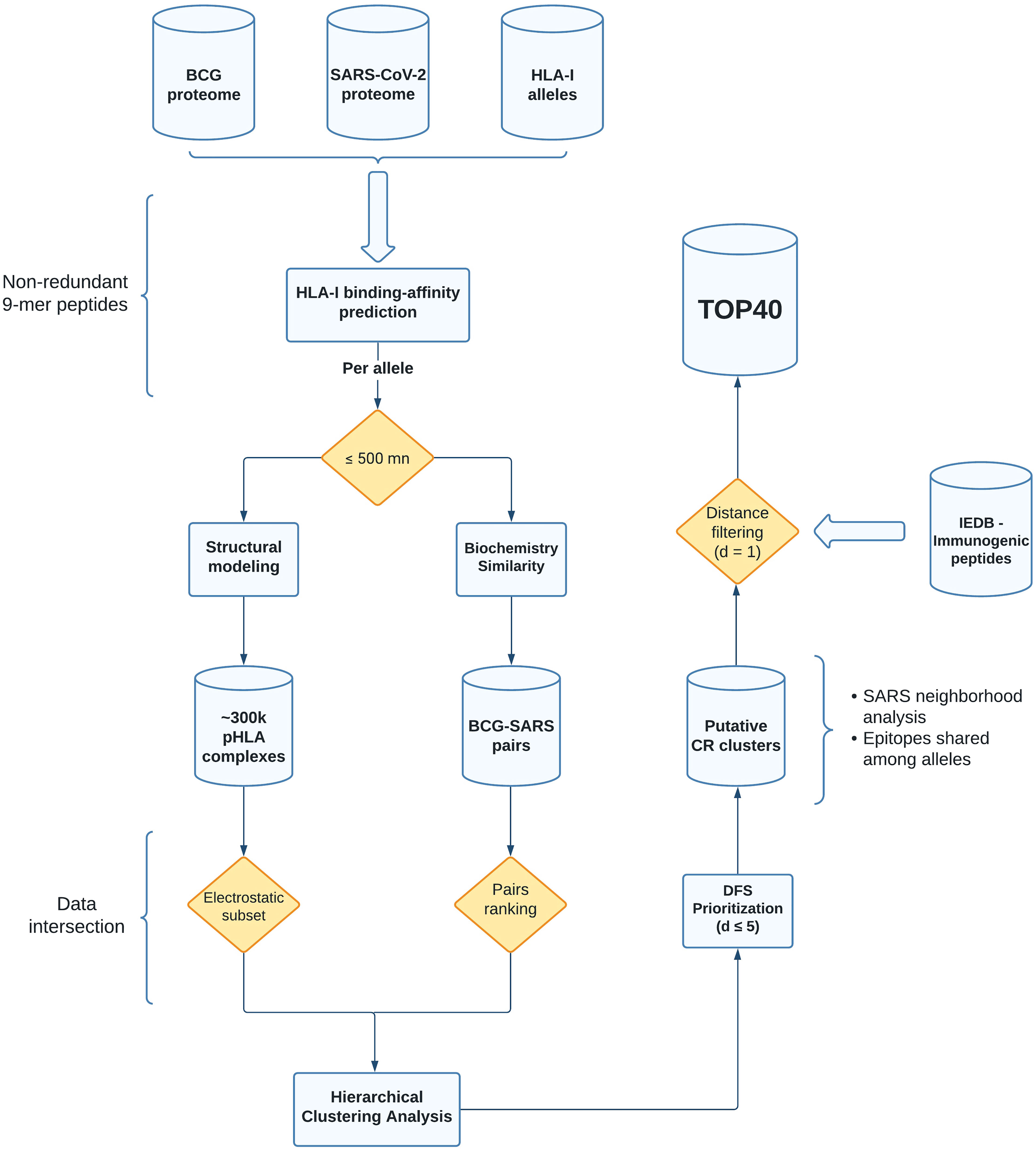
Figure 1 Flowchart with all the steps from data acquisition to processing and selection of the putative cross-reactive clusters. The shapes in the flowchart represent distinct aspects of our pipeline. Cylinders and rectangles are showing database (output storage) and computational analyses (including third-party tools, see Material and Methods), respectively. The yellow diamonds represent data filtering (pHLA binding affinity, electrostatic subset, pairs ranking, and distance filtering). The curly brackets are showing additional analysis or parameters chosen in our study. BCG, Bacillus Calmette-Guérin (Pasteur strain); SARS, SARS-CoV-2; HLA-I, Human Leukocyte Antigen of class I; pHLA, peptide-HLA; DFS, Depth-first search; CR, cross-reactive; IEDB, Immune Epitope Database.
Selection of Potential HLA-I Binders From BCG and SARS-CoV-2 Proteome
We hypothesize that partial heterologous immunity between BCG and SARS-CoV-2 can be mediated by T cell cross-reactivity between similar viral peptides displayed by HLA-I molecules. In order to identify candidate peptide-targets for these cross-reactive responses, we started by fetching the whole proteome of BCG and SARS-CoV-2 from UniprotKB. In total, we analyzed 3,891 proteins from BCG, and 14 proteins from SARS-CoV-2. We also searched the literature and public databases for HLAs with high population coverage, as well as those reported to be involved in SARS-CoV-2 immune responses. We restricted our analysis to a total of 10 HLAs (i.e., five HLA-A and five HLA-B alleles). Since HLA-I molecules most frequently present peptides of nine amino acids (9-mers) in length (54), we further recovered all possible 9-mers from BCG and SARS-CoV-2 proteome. This resulted in a total of 1,237,282 (BCG) and 9,814 (SARS-CoV-2) peptides.
Since not all 9-mers would be able to bind all HLA-I molecules, according to HLA-I binding restrictions, we further filtered these peptides using a sequence-based binding affinity predictor. For this step, we set a threshold of 500 nM aiming to recover only strong and intermediate HLA-I binders (45). As a result, we recovered a total of 296,651 and 2,918 peptides from BCG and SARS-CoV-2, respectively. Each peptide was modelled in the context of its specific HLA using APE-Gen (47) and HLA-Arena (43), generating a total of 358,386 pHLA structures. A small percentage of complexes could not be modeled by APE-Gen, and was removed from the analysis. The calculations were performed at PUCRS High Performance Computing Lab (LabLAD) and all the data is made freely available upon request. This represents one of the largest repositories for three-dimensional structures of pHLA complexes available.
Selection of Similar Peptides Based on Biochemical Properties
To further refine our analysis to consider only the most similar pairs of peptides (i.e., BCG-SARS pairs) we apply a filter based on biochemical properties (BP). For that, we represented each peptide as a biochemical properties (BP) vector reflecting its amino acid sequence, as previously described in (55). The L1 norm was used to score the overall differences between each SARS-CoV-2 and BCG peptides displayed by the same HLA-I allele, respectively. Next, we rank BCG-SARS pairs based on the BP score. The top 10,000 BCG-SARS pairs across all lists of the same HLA-I allele were selected for further analysis. Note that the number of recovered peptides varied across HLA-I alleles. For instance, most of the peptides selected are presented by the HLA-B*15:03 (13.5%), while HLA-B*51:01 is the HLA-I allele with the lowest number of peptides (2.1%).
After removing redundant peptides, we obtained 55,536 BCG-derived peptides that were biochemically similar to 2,113 peptides derived from SARS-CoV-2. The per-allele totals can be found on Supplementary Table 1.
Selection of Similar pHLAs Based on Structural Features
Although peptide similarity in terms of biochemical properties can suggest a higher probability for cross-reactivity (56–58), T cell cross-reactivity is also driven by structural features of the pHLA complex (e.g., topography and electrostatic potential over the TCR-interacting surface) (39). To account for those additional features, we included all the complexes selected in the previous step (i.e., a total of 57,649 pHLA complexes, divided into 10 HLA-I alleles) into an HLA-restricted pairwise structural comparison analysis. Briefly, we extracted the image of the TCR-interacting surface of each modeled pHLA complex, capturing its topography and electrostatic potential.
Following a similar protocol to that described in previous studies (48), we determined 46 gates over this image (Figure 2A). These gates were designed to cover known “hot-spots” for T cell interaction derived from crystal structures (i.e., curated interactions from IEDB) and previous studies (48). We then extracted summarizing statistics from each of these gates to create a vector representing each complex. Finally, these vectors were used as input for a Hierarchical Clustering Analysis (HCA). This strategy produced 10 dendrograms (i.e., one for each HLA-I allele) summarizing all structure-based pairwise comparisons of pHLA complexes (Figure 2B and Supplementary Figure 1).
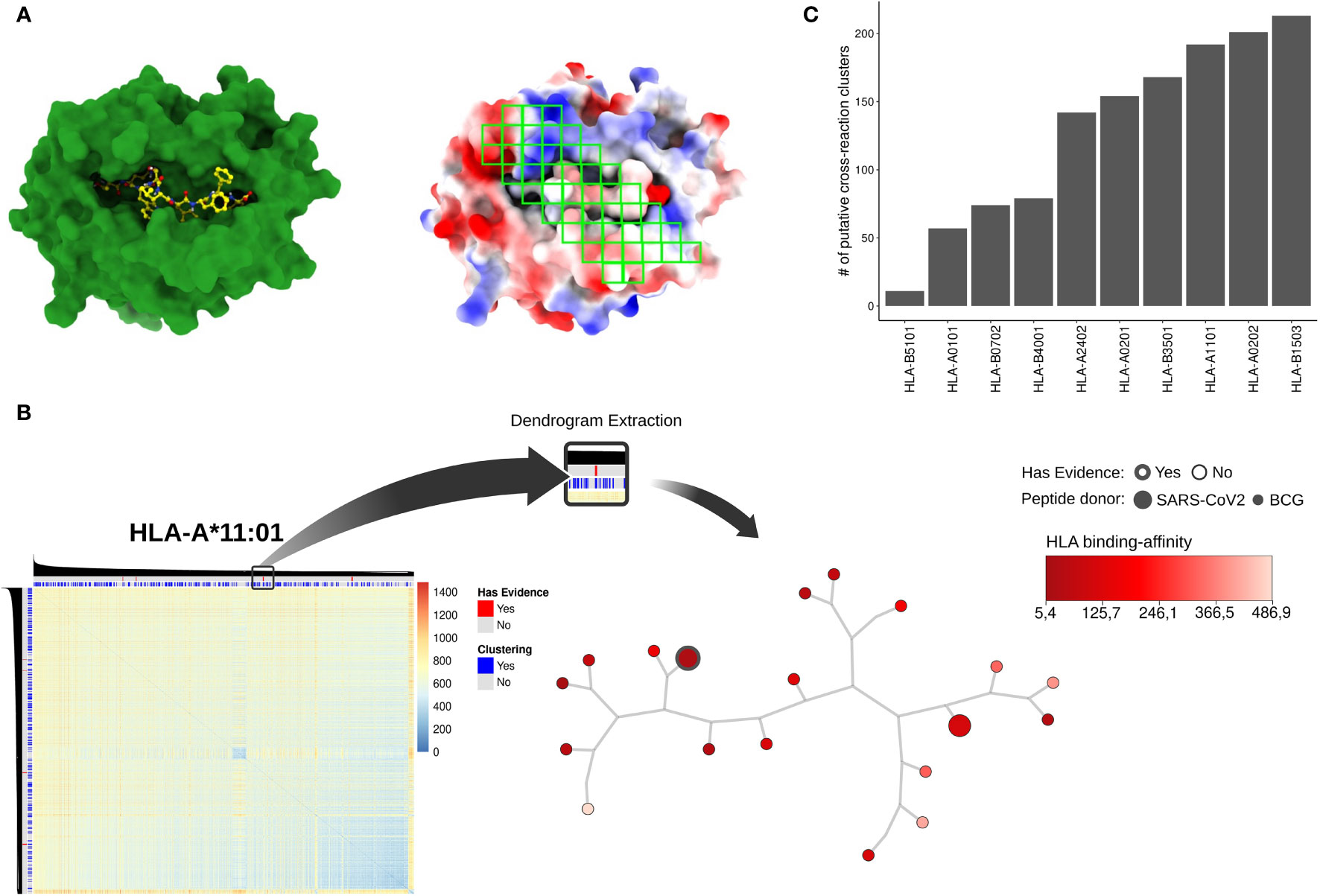
Figure 2 (A) pHLA models using HLA-A*02:01 and the SARS-derived peptide TQWSLFFFL as an example. On the left, the HLA-I alpha chain and the peptide are represented in green and yellow, respectively; on the right, forty-six regions (green squares) were sampled for electrostatic potential analysis through RGB mean and standard deviation values extraction. The red, white, and blue represent negative, neutral, and positive charges, respectively. The electrostatic potential range used was -3kT to +3kT. (B) An overview related to dendrogram extraction based on the DFS algorithm. As an example, the HLA-A*11:01 heatmap was obtained after the HCA procedure. The color key represents Euclidean distance applied as the similarity metric into dendrogram clustering. The color gradient blue to red indicates high to lower similarity among pHLA structures, respectively. On the superior bars the clustering (i.e., grouping pHLA by similarity using our approach) and experimental evidence status (i.e., data gathered from literature) are represented. SARS-CoV-2 peptides with experimental support are shown in red, and the DFS-based cross-reactive clusters are shown in blue. The tree-base structure in the right corner represents a filtered cluster in HLA-A*11:01 data. The predicted binding affinity (i.e., how well the peptide binds that specific HLA) is shown in the red color key. The node size represents the peptide-donor (i.e., SARS-CoV-2 or BCG) and the silhouette thickness indicates if the peptide is immunogenic, according to the IEDB database. In (C) we recover the total number of cross-reactivity clusters for each one of the HLAs present in our analysis.
A popular way to visualize these pairwise comparisons is through the use of heatmaps, but they became harder to interpret for larger datasets. Here, we traverse the dendrogram using a DFS approach with a cut-off distance lower or equal to 5 (see Material and Methods). We arbitrarily choose this value aiming to recover clusters of possible cross-reactive targets between SARS-CoV-2 and BCG peptides. From this analysis, we retrieved a total of 16,606 putative BCG-SARS cross-reactions (Supplementary Table 2), distributed throughout a diverse number of clusters per-allele (Figure 2C). This database of putative cross-reactive clusters (Figure 1, see Putative CR clusters) was then used for two different analyses, presented in the following sessions. First, we conducted a qualitative analysis focused on available experimental data (i.e., identifying experimentally-determined immunogenic peptides from IEDB (59). Second, we performed a quantitative analysis driven by the data. Here we computed (i) the “density” of BCG pHLA-targets in close distance to SARS-CoV-2 pHLAs, and (ii) the overlap degree of SARS peptides within cross-reactive clusters displayed by distinct HLA-I alleles (Figure 1).
Identification of Cross-Reactive Clusters Including Immunogenic Peptides From BCG Vaccine
Immunogenicity, defined as the capacity of a peptide to trigger an immune response, is one of the most important features in epitope discovery. It is also a requirement for potential involvement in T cell cross-reactivity. Therefore, we initially focused on identifying SARS-CoV-2 peptides present in our putative cross-reactivity clusters, defining three conditions: i) a DFS distance equal to one, plus experimental evidence regarding ii) HLA-I binding-affinity matching to our prediction, and iii) T cell response assay reported by on IEDB. After this filtering, we produced a table of 40 possible cross-reactive BCG-SARS pairs (Table 1 and Supplementary Table 3). In summary, according to our approach, these immunogenic SARS-CoV-2 peptides are highly similar to BCG peptides, both in terms of biochemical properties as well as structural pHLA features (Supplementary Figure 4).
Cross-Reactivity Prediction Between BCG and SARS-CoV-2
Since there is currently no experimental support for the immunogenicity and cross-reactivity of most peptides in our predicted cross-reactivity clusters, we decided to also perform a quantitative analysis to explore other ways to prioritize potential targets of cross-reactivity in our data. In this context, we performed two different analyses: cluster density and HLA-I restriction.
In the first case, we focused on the “density” of BCG pHLA-targets in close distance to SARS-CoV-2 pHLAs (indicated in Figure 1 as SARS neighborhood analysis). The density was calculated based on a number of BCG peptides within the cross-reactivity cluster (i.e., SARS-BCG pairs inside d <= 5). As a result, several high-density SARS neighborhoods were identified across HLA-I alleles. The SARS pHLAs with high-density neighborhoods are an interesting resource due to the higher potential of triggering heterologous immunity with several BCG peptides. Finally, these findings can be used for further investigation of potential cross-reactivity. Supplementary Figures 2, 3 show 50 SARS neighborhoods for each HLA-I allele in this study.
In the second case, we examined whether SARS-CoV-2 epitopes in putative cross-reactive clusters can be presented by multiple HLA-I alleles using UpSetR to create visualizations of intersecting sets (Figure 3A) (60). Epitopes presented by multiple HLA-I alleles are promising resources for vaccine design and immunotherapy, since they maximize population coverage (61, 62). As expected, our results show a higher percentage of putative cross-reactive epitopes presented by a single HLA-I allele, and a lower percentage of epitopes shared between 2 or more than 3 HLA-I alleles (Figure 3B).
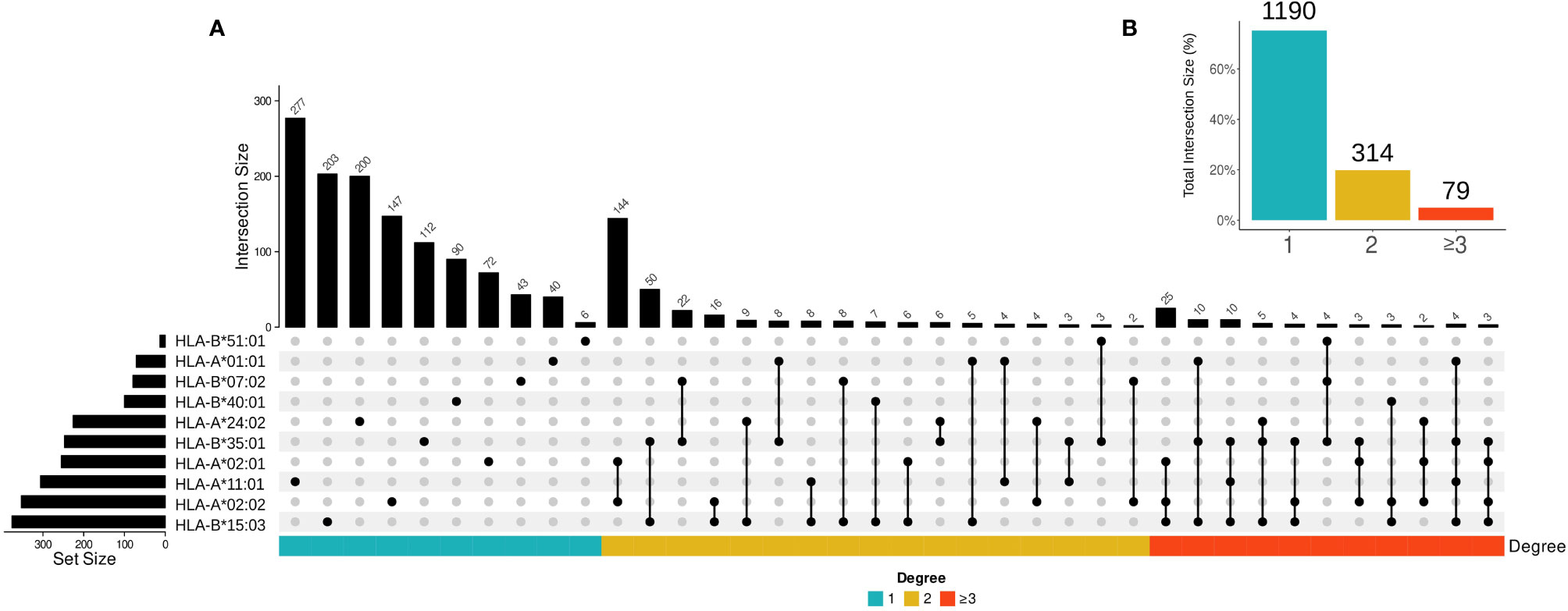
Figure 3 The overall profile of cross-reactive SARS-CoV-2 epitopes presented by different HLA-I alleles. (A) The intersection size represents the number of SARS-CoV-2 epitopes per cross-reactivity cluster when presented by one, two, and three or more HLAs. The edges connecting HLA-I alleles represent an intersection among SARS-CoV-2 epitopes (i.e., the number of epitopes presented by the same HLA molecule). Only the intersections higher or equal to two are shown. The set size represents the total number of SARS-CoV-2 epitopes in each HLA-I allele. (B) The percentage of shared SARS-CoV-2 epitopes per HLA-I allele bins is shown. The color bar represents intersection degree bins in each plot.
Note that there is not a clear correlation between the number of peptides presented by one allele and the number of putative cross-reactive clusters. For instance, most of the peptides are presented by HLA-A*11:01, while the largest number of cross-reactive clusters was observed for epitopes restricted to HLA-B*15:03. In terms of shared cross-reactive clusters, both HLA-B*15:03 and HLA-B*35:01 are the most represented alleles, as observed in Figure 3 by the number of connections to other HLA-I alleles (16 and 14 times, respectively).
Discussion
The development of functional memory T cell response is a complex process, which plays a fundamental role in vaccine-induced protection. Pre-existing memory T cells can be activated by cross-reactive peptide-targets (63), making response to immunization to be largely driven by individual immunological history (64). For instance, a study with yellow fever virus showed that T cell kinetics after vaccination depended on the pre-vaccination precursor frequencies and initial differentiation states (65). Their results indicate that vaccines re-prioritize the immune repertoire to more relevant T cells against the novel pathogen. In the context of the COVID-19 pandemic, cellular immune response mediated by CD8+ T cells specific to cross-reactive epitopes from common coronaviruses has been reported as one of the main determinants of immunological protection in SARS-CoV-2 infection (66). Taken together, these findings raise the possibility that individuals vaccinated with BCG present a pre-existing pool of cross-reactive CD8 T cells, which can be recruited during SARS-CoV-2 natural infection or COVID-19 vaccine, resulting in an enhanced cellular immunity.
Several studies have described a protective effect of BCG vaccination against viral infections (11, 15, 26, 67, 68). In fact, it has been suggested that the BCG vaccination program could be associated with a reduction in COVID-19 cases in some countries (69). In this context, the search for sequence similarity between SARS-CoV-2 peptides and peptides from the BCG vaccine has been the main tool available for the identification of potential targets for T cell cross-reactivity, which could be the basis for a BCG-induced heterologous immunity. In a recent study, Eggenhuizen and collaborators (36) used an in vitro co-culture system to identify human T cells specific to a BCG-derived peptide, which cross-react with a highly similar peptide from SARS-CoV-2.
Here we present a large-scale computational screening for potential targets of T cell cross-reactivity between SARS-CoV-2 and BCG that might help to explain the possible protective effect of BCG vaccination against COVID-19. We combined standard immunoinformatics methods (e.g., sequence-based HLA-binding prediction) with innovative structural approaches to better account for other aspects of biochemical and structural features that are potential drivers of T cell cross-reactivity. Some of the pHLA comparison methods used here have been proposed and applied in previous studies (39, 48), but have never been deployed to the scale of the analysis described here. The size of this analysis, encompassing the entire proteome of both pathogens of interest, created a series of technical challenges that could not be addressed with off-the-shelf solutions. For instance, our structural analysis included the modeling of 358,386 pHLA complexes with APE-Gen. This is the largest dataset of pHLA structures ever produced, and such large-scale modeling was enabled by the development of a dispatcher to optimize the use of APE-Gen in High Performance Computing clusters. As part of our analysis, we performed the TCR-interacting surface comparison of all possible pairs of pHLAs for a given HLA-I allele, considering 46 regions of interest in each surface, and multiple data points for each region. This was only possible through the implementation of a plugin for image processing with ImageJ. All APE-Gen models produced in this project, as well as the APE-Gen dispatcher and the ImageJ plugin will be made publicly available as part of this study.
Our analysis produced a large dataset of putative cross-reactive clusters involving BCG- and SARS-CoV-2-derived peptides. Then, we explored both qualitative and quantitative approaches to prioritize the most promising pairs of peptides in this dataset. Our initial focus on SARS-CoV-2 peptides with experimentally-determined immunogenicity led to the identification of 40 putative 9-mer peptide pairs with potential cross-reactivity with BCG peptides. This list included the HLA-B*40:01-restricted SARS-CoV-2-derived peptides GEAANFCAL, GEVITFDNL, and FIAGLIAIV which have been independently shown to induce T cell response, INF-γ production, and proliferation in COVID-19 patients (66, 70). In addition, multiple peptides from our top 40 list (Table 1) have been reported to induce T cell activation in recent studies analyzing aspects of cellular immunity in COVID-19 patients (71–74). Some of the peptides described by Eggenhuizen and colleagues as cross-reactive between BCG and SARS were found in the top 16,000 putative SARS-BCG cross-reactive peptide pairs (Supplementary Table 2) (36).
In our analysis, the number of putative clusters of cross-reactive peptides varied among HLA-I alleles. Although most of the peptides were predicted to bind to HLA-A*01:01, most of the cross-reactive clusters were restricted to HLA-B*15:03. Note that the importance of the HLA-B*15:03 allele for the presentation of SARS-CoV-2-derived peptides has already been described in previous work. For instance, molecular docking studies performed by Albagi et al. indicated higher affinity of Spike-derived peptide for the HLA-B*15:03 allele (75). In another study, Barquera and colleagues analyzed the binding affinities of peptides derived from complete proteomes of seven pandemic human viruses (including coronaviruses), against 438 Class I and Class II HLA proteins. In their study, statistical modeling indicated that HLA-B*15:03 binds to more than 200 peptides with strong affinity, with only a minimum number of peptides being predicted as weak/non-binders for this allele (76). Finally, a comprehensive in silico study by Nguyen and colleagues suggested that HLA-B*15:03 can generate cross-protective T cell dependent immunity, due to a greater ability to present highly conserved SARS-CoV-2 peptides (77). Our study complements these previous findings by incorporating large-scale structural modeling and structure-based comparison of pHLA complexes on the identification of potential targets of T cell cross-reactivity. Taken together, these findings further corroborate the notion that differences in HLA-I genotypes can alter the course of the COVID-19 disease and its transmission (78), and that HLA-B*15:03 might contribute to increased cellular immunity against SARS-CoV-2. On the other hand, the allele with the least number of predicted clusters in our analysis was HLA-B*51:01 (Figure 2C), which could suggest an increased susceptibility to COVID-19. In fact, a study on genetic association with susceptibility to SARS-CoV-2 infection and disease severity identified HLA-B*51:01, as well as HLA-A*11:01 and HLA-C*14:02, as the most prevalent in patients with severe disease (79).
The response mediated by CD4+ T cells is also important during SARS-CoV-2 infection and the vaccine immunity (80, 81). Although this was not the major goal of our study, we ran a sequence-based predictor on the selected 40 possible cross-reactive BCG-SARS pairs to analyze their capacity to bind HLA class II molecules. We have found only one epitope (YLASGGQPI) that can strongly bind to the human HLA-DRB1*07:01 (data not shown).
To further explore our dataset, going beyond available experimental data, we performed a quantitative analysis to identify both (i) clusters with higher density neighborhoods of potentially cross-reactive BCG pHLA-targets, and (ii) clusters with SARS epitopes that were shared across different HLAs (Figures 1 and 3). This effort identified a list of SARS peptides representing the 50 higher-density neighborhoods. These candidates are interesting because they could indicate instances in which T cell cross-reactivity with a single SARS-CoV-2 peptide could be primed by multiple BCG peptides, increasing its potential involvement in heterologous immunity. In addition, our data could help the analysis of results from several ongoing clinical trials on the protective effect of BCG vaccination for COVID-19. One limitation of our study is the lack of experimental validation of these peptides. These targets should be further investigated and confirmed in future studies.
Finally, we also identified clusters of potential cross-reactive targets that were shared across multiple HLA-I alleles. Considering the vast diversity of HLA-I alleles in the human population (i.e., over 19,000 alleles), HLA-I restriction is a concern for vaccine development. Even a highly immunogenic SARS-CoV-2 peptide will not constitute a useful target for vaccine development if its presentation to T cells is restricted to an HLA-I allele that is rare in the human population. On the other hand, peptides displayed by prevalent HLA-I alleles, or even multiple HLAs, would make very interesting targets for vaccine development due to broader population coverage. In fact, we have found two peptides - FSAVGNICY and QPGQTFSVL - that were (i) included in our top 50 high-density neighborhoods, (ii) can be presented by multiple alleles, and (iii) are immunogenic targets, with the capacity to elicitate CD8+ T cell response.
In the context of the current pandemic it is important to note that despite ongoing success with multiple COVID-19 vaccines, there are still open questions and concerns regarding the rise of different SARS-CoV-2 variants. In addition, other coronaviruses represent a continuous threat of new pandemics, considering the existence of large natural reservoirs (82). In this context, the development of peptide-based vaccines targeting conserved regions of coronaviruses, and presenting cross-reactivity with existing pools of memory T cells in the population, could be an interesting strategy to complement and extend the protection conferred by existing COVID-19 vaccines (83–85). We hope our work can contribute to these efforts by suggesting high priority targets for potential T cell cross-reactivity with BCG-derived peptides.
Data Availability Statement
The raw data supporting the conclusions of this article will be made available by the authors, without undue reservation.
Author Contributions
RT: Conceptualization, Software, Methodology, Validation, Investigation, Data Curation, Writing, Visualization. MM: Conceptualization, Software, Methodology, Validation, Investigation, Data Curation, Writing, Visualization. AF: Methodology, Software, Validation, Formal analysis, Investigation, Data Curation, Writing, Visualization. FR: Methodology, Software, Validation, Formal analysis, Data Curation. RB: Methodology, Software, Validation, Formal analysis, Data Curation. LK: Resources, Writing, Supervision, Project administration, Funding acquisition. TF: Methodology, Software, Validation, Formal analysis, Resources, Data Curation, Writing, Supervision, Project administration, Funding acquisition. DA: Conceptualization, Methodology, Resources, Writing, Supervision, Project administration, Funding acquisition. AS: Conceptualization, Methodology, Resources, Writing, Supervision, Project administration, Funding acquisition. All authors contributed to the article and approved the submitted version.
Funding
MM was supported by a CPRIT fellowship on RP170593. MM and LK were also supported in part by NCI 1U01CA258512-01 and NSF DBI 2033262. RT and AS were financed in part by the Coordenação de Aperfeiçoamento de Pessoal de Nivel Superior – Brasil (CAPES) – Finance Code 001. AF and DA were supported in part by funds from the University of Houston. AS was also supported by FAPERGS 20/2551-0000258-6.
Conflict of Interest
The authors declare that the research was conducted in the absence of any commercial or financial relationships that could be construed as a potential conflict of interest.
Publisher’s Note
All claims expressed in this article are solely those of the authors and do not necessarily represent those of their affiliated organizations, or those of the publisher, the editors and the reviewers. Any product that may be evaluated in this article, or claim that may be made by its manufacturer, is not guaranteed or endorsed by the publisher.
Acknowledgments
The authors acknowledge the High-Performance Computing Laboratory of the Pontifical Catholic University of Rio Grande do Sul (LAD-IDEIA/PUCRS, Brazil) for providing support and technological resources, which have contributed to the development of this project and to the results reported within this research. TF, FR, and RB received free computing time from Amazon Web Services acquired with a grant from RNP (Brazilian National Research and Education Network).
Supplementary Material
The Supplementary Material for this article can be found online at: https://www.frontiersin.org/articles/10.3389/fimmu.2021.812176/full#supplementary-material
References
2. Ziegler CGK, Allon SJ, Nyquist SK, Mbano IM, Miao VN, Tzouanas CN, et al. SARS-CoV-2 Receptor ACE2 Is an Interferon-Stimulated Gene in Human Airway Epithelial Cells and Is Detected in Specific Cell Subsets Across Tissues. Cell (2020) 181(5):1016–35. doi: 10.1016/j.cell.2020.04.035
3. Hu B, Guo H, Zhou P, Shi ZL. Characteristics of SARS-CoV-2 and COVID-19. Nat Rev Microbiol (2021) 19(3):141–54. doi: 10.1038/s41579-020-00459-7
4. Forni G, Mantovani A, Forni G, Mantovani A, Moretta L, Rappuoli R, et al. COVID-19 Vaccines: Where We Stand and Challenges Ahead. Cell Death Differ (2021) 28(2):626–39. doi: 10.1038/s41418-020-00720-9
5. Abu-Raddad LJ, Chemaitelly H, Butt AA. Effectiveness of the BNT162b2 Covid-19 Vaccine Against the B.1.1.7 and B.1.351 Variants. N Engl J Med (2021) 385(2):187–9. doi: 10.1056/nejmc2104974
6. Hacisuleyman E, Hale C, Saito Y, Blachere NE, Bergh M, Conlon EG, et al. Vaccine Breakthrough Infections With SARS-CoV-2 Variants. N Engl J Med (2021) 384(23):2212–8. doi: 10.1056/nejmoa2105000
7. Calmette A, Guérin C, Négre LL. Sur La Vaccination Préventive Des Enfants Nouveau-Nés Contre La Tuberculose Par Le BCG. Ann Inst Pasteur (Paris) (1927) 41:201–32.
8. Ahmed A, Rakshit S, Adiga V, Dias M, Dwarkanath P, D’Souza G. Vyakarnam A. A Century of BCG: Impact on Tuberculosis Control and Beyond. Immunol Rev (2021) 301(1):98–121. doi: 10.1111/imr.12968
9. Biering-Sørensen S, Aaby P, Napirna BM, Roth A, Ravn H, Rodrigues A, et al. Small Randomized Trial Among Low-Birth-Weight Children Receiving Bacillus Calmette-Guéerin Vaccination at First Health Center Contact. Pediatr Infect Dis J (2012) 31(3):306–8. doi: 10.1097/INF.0b013e3182458289
10. Hirve S, Bavdekar A, Juvekar S, Benn CS, Nielsen J, Aaby P. Non-Specific and Sex-Differential Effects of Vaccinations on Child Survival in Rural Western India. Vaccine (2012) 30(50):7300–8. doi: 10.1016/j.vaccine.2012.09.035
11. Moorlag SJCFM, Arts RJW, van Crevel R, Netea MG. Non-Specific Effects of BCG Vaccine on Viral Infections. Clin Microbiol Infect (2019) 25(12):1473–8. doi: 10.1016/j.cmi.2019.04.020
12. Butkeviciute E, Jones CE, Smith SG. Heterologous Effects of Infant BCG Vaccination: Potential Mechanisms of Immunity. Future Microbiol (2018) 13(10):1193–208. doi: 10.2217/fmb-2018-0026
13. Welsh RM, Che JW, Brehm MA, Selin LK. Heterologous Immunity Between Viruses. Immunol Rev (2010) 235(1):244–66. doi: 10.1111/j.0105-2896.2010.00897.x
14. Shet A, Ray D, Malavige N, Santosham M, Bar-Zeev N. Differential COVID-19-Attributable Mortality and BCG Vaccine Use in Countries. medRxiv (2020). doi: 10.1101/2020.04.01.20049478
15. Arts RJW, Moorlag SJCFM, Novakovic B, Li Y, Wang SY, Oosting M, et al. BCG Vaccination Protects Against Experimental Viral Infection in Humans Through the Induction of Cytokines Associated With Trained Immunity. Cell Host Microbe (2018) 23(1):89–100.e5. doi: 10.1016/j.chom.2017.12.010
16. Kleinnijenhuis J, Quintin J, Preijers F, Joosten LAB, Ifrim DC, Saeed S, et al. Bacille Calmette-Guérin Induces NOD2-Dependent Nonspecific Protection From Reinfection via Epigenetic Reprogramming of Monocytes. Proc Natl Acad Sci USA (2012) 109(43):17537–42. doi: 10.1073/pnas.1202870109
17. Gil A, Kenney LL, Mishra R, Watkin LB, Aslan N, Selin LK. Vaccination and Heterologous Immunity: Educating the Immune System. Trans R Soc Trop Med Hyg (2014) 109(1):62–9. doi: 10.1093/trstmh/tru198
19. Aksu K, Naziroğlu T, Özkan P. Factors Determining COVID-19 Pneumonia Severity in a Country With Routine BCG Vaccination. Clin Exp Immunol (2020) 202(2):220–5. doi: 10.1111/cei.13507
20. Brooks NA, Puri A, Garg S, Nag S, Corbo J, El Turabi A, et al. The Association of Coronavirus Disease-19 Mortality and Prior Bacille Calmette-Guerin Vaccination: A Robust Ecological Analysis Using Unsupervised Machine Learning. Sci Rep (2021) 11(1):774. doi: 10.1038/s41598-020-80787-z
21. Moorlag SJCFM, van Deuren RC, van Werkhoven CH, Jaeger M, Debisarun P, Taks E, et al. Safety and COVID-19 Symptoms in Individuals Recently Vaccinated With BCG: A Retrospective Cohort Study. Cell Rep Med (2020) 1(5):100073. doi: 10.1016/j.xcrm.2020.100073
22. Berg MK, Yu Q, Salvador CE, Melani I, Kitayama S. Mandated Bacillus Calmette-Guérin (BCG) Vaccination Predicts Flattened Curves for the Spread of COVID-19. Sci Adv (2020) 6(32):eabc1463. doi: 10.1126/sciadv.abc1463
23. Miller A, Reandelar MJ, Fasciglione K, Roumenova V, Li Y, Otazu GH. Correlation Between Universal BCG Vaccination Policy and Reduced Mortality for COVID-19. medRxiv (2020). doi: 10.1101/2020.03.24.20042937
24. Hamiel U, Kozer E, Youngster I. SARS-CoV-2 Rates in BCG-Vaccinated and Unvaccinated Young Adults. JAMA - J Am Med Assoc (2020) 323(22):2340–1. doi: 10.1001/jama.2020.8189
25. Curtis N, Sparrow A, Ghebreyesus TA, Netea MG. Considering BCG Vaccination to Reduce the Impact of COVID-19. Lancet (2020) 395(10236):1545–6. doi: 10.1016/S0140-6736(20)31025-4
26. Escobar LE, Molina-Cruz A, Barillas-Mury C. BCG Vaccine Protection From Severe Coronavirus Disease 2019 (COVID-19). Proc Natl Acad Sci USA (2020) 117(30):17720–6. doi: 10.1073/pnas.2008410117
27. Arlehamn CSL, Sette A, Peters B. Lack of Evidence for BCG Vaccine Protection From Severe COVID-19. Proc Natl Acad Sci USA (2020) 117(41):25203–4. doi: 10.1073/pnas.2016733117
28. Green CM, Fanucchi S, Dominguez-Andres J, Fok ET, Moorlag SJCFM, Negishi Y, et al. COVID-19: A Model Correlating BCG Vaccination to Protection From Mortality Implicates Trained Immunity. medRxiv (2020). doi: 10.1101/2020.04.10.20060905
29. Klinger D, Blass I, Rappoport N, Linial M. Significantly Improved COVID-19 Outcomes in Countries With Higher Bcg Vaccination Coverage: A Multivariable Analysis. Vaccines (2020) 8(3):378. doi: 10.3390/vaccines8030378
30. Fu W, Ho PC, Liu CL, Tzeng KT, Nayeem N, Moore JS, et al. Reconcile the Debate Over Protective Effects of BCG Vaccine Against COVID-19. Sci Rep (2021) 11(1):8356. doi: 10.1038/s41598-021-87731-9
31. Giamarellos-Bourboulis EJ, Tsilika M, Moorlag S, Antonakos N, Kotsaki A, Domínguez-Andrés J, et al. Activate: Randomized Clinical Trial of BCG Vaccination Against Infection in the Elderly. Cell (2020) 183(2):315–23.e9. doi: 10.1016/j.cell.2020.08.051
32. Amirlak l, Haddad R, Hardy JD, Khaled NS, Chung MH, Amirlak B. Effectiveness of Booster BCG Vaccination in Preventing Covid-19 Infection. Hum Vaccines Immunother (2021) 1–3. doi: 10.1080/21645515.2021.1956228
33. Hilligan KL, Namasivayam S, Clancy CS, O’Mard D, Oland SD, Robertson SJ, et al. Intravenous Administration of BCG Protects Mice Against Lethal SARS-CoV-2 Challenge. bioRxiv (2021). doi: 10.1101/2021.08.30.458273
34. Nie S, Lin SJ, Kim SK, Welsh RM, Selin LK. Pathological Features of Heterologous Immunity are Regulated by the Private Specificities of the Immune Repertoire. Am J Pathol (2010) 176(5):2107–12. doi: 10.2353/ajpath.2010.090656
35. Urbán S, Paragi G, Burián K, McLean GR, Virok DP. Identification of Similar Epitopes Between Severe Acute Respiratory Syndrome Coronavirus-2 and Bacillus Calmette–Guérin: Potential for Cross-Reactive Adaptive Immunity. Clin Transl Immunol (2020) 9(12):e1227. doi: 10.1002/cti2.1227
36. Eggenhuizen PJ, Ng BH, Chang J, Fell AL, Cheong RMY, Wong WY, et al. BCG Vaccine Derived Peptides Induce SARS-CoV-2 T Cell Cross-Reactivity. Front Immunol (2021) 12:692729. doi: 10.3389/fimmu.2021.692729
37. Weiskopf D, Schmitz KS, Raadsen MP, Grifoni A, Okba NMA, Endeman H, et al. Phenotype and Kinetics of SARS-CoV-2-Specific T Cells in COVID-19 Patients With Acute Respiratory Distress Syndrome. Sci Immunol (2020) 5(48):eabd2071. doi: 10.1126/SCIIMMUNOL.ABD2071
38. Tomita Y, Sato R, Ikeda T, Sakagami T. BCG Vaccine may Generate Cross-Reactive T Cells Against SARS-CoV-2: In Silico Analyses and a Hypothesis. Vaccine (2020) 38(41):6352–6. doi: 10.1016/j.vaccine.2020.08.045
39. Antunes DA, Rigo MM, Freitas MV, Mendes MFA, Sinigaglia M, Lizée G, et al. Interpreting T-Cell Cross-Reactivity Through Structure: Implications for TCR-Based Cancer Immunotherapy. Front Immunol (2017) 8:1210. doi: 10.3389/fimmu.2017.01210
40. Mandl JN, Germain RN. Focusing in on T Cell Cross-Reactivity. Cell (2014) 157(5):1006–8. doi: 10.1016/j.cell.2014.05.002
41. Birnbaum ME, Mendoza JL, Sethi DK, Dong S, Glanville J, Dobbins J, et al. Deconstructing the Peptide-MHC Specificity of T Cell Recognition. Cell (2014) 157(5):1073–87. doi: 10.1016/j.cell.2014.03.047
42. Solberg OD, Mack SJ, Lancaster AK, Single RM, Tsai Y, Sanchez-Mazas A, et al. Balancing Selection and Heterogeneity Across the Classical Human Leukocyte Antigen Loci: A Meta-Analytic Review of 497 Population Studies. Hum Immunol (2008) 69(7):443–64. doi: 10.1016/j.humimm.2008.05.001
43. Antunes DA, Abella JR, Hall-Swan S, Devaurs D, Conev A, Moll M, et al. HLA-Arena: A Customizable Environment for the Structural Modeling and Analysis of Peptide-HLA Complexes for Cancer Immunotherapy. JCO Clin Cancer Inf (2020) 4:623–36. doi: 10.1200/cci.19.00123
44. O’Donnell TJ, Rubinsteyn A, Bonsack M, Riemer AB, Laserson U, Hammerbacher J. MHCflurry: Open-Source Class I MHC Binding Affinity Prediction. Cell Syst (2018) 7(1):129–32.e4. doi: 10.1016/j.cels.2018.05.014
45. Sette A, Vitiello A, Reherman B, Fowler P, Nayersina R, Kast WM, et al. The Relationship Between Class I Binding Affinity and Immunogenicity of Potential Cytotoxic T Cell Epitopes. J Immunol (1994) 153(12):5586–92.
46. Webb B, Sali A. Comparative Protein Structure Modeling Using MODELLER. Curr Protoc Bioinf (2016) 54:5.6.1–.37. doi: 10.1002/cpbi.3
47. Abella JR, Antunes DA, Clementi C, Kavraki LE. Ape-Gen: A Fast Method for Generating Ensembles of Bound Peptide-MHC Conformations. Molecules (2019) 24(5):881. doi: 10.3390/molecules24050881
48. Mendes MFA, Antunes DA, Rigo MM, Sinigaglia M, Vieira GF. Improved Structural Method for T-Cell Cross-Reactivity Prediction. Mol Immunol (2015) 67(2 Pt B):303–10. doi: 10.1016/j.molimm.2015.06.017
49. Suzuki R, Shimodaira H. Pvclust: An R Package for Assessing the Uncertainty in Hierarchical Clustering. Bioinformatics (2006) 22(12):1540–2. doi: 10.1093/bioinformatics/btl117
50. Müllner D. Fastcluster: Fast Hierarchical, Agglomerative Clustering Routines for R and Python. J Stat Softw (2013) 53(9):1–18. doi: 10.18637/jss.v053.i09
51. Ihaka R, Gentleman R. R: A Language for Data Analysis and Graphics. J Comput Graph Stat (1996) 5:299. doi: 10.2307/1390807
52. Csardi G, Nepusz T. The Igraph Software Package for Complex Network Research. InterJournal Complex Syst (2006) 1695(5):1–9.
53. Paradis E, Schliep K. Ape 5.0: An Environment for Modern Phylogenetics and Evolutionary Analyses in R. Bioinformatics (2019) 35(3):526–8. doi: 10.1093/bioinformatics/bty633
54. Trolle T, McMurtrey CP, Sidney J, Bardet W, Osborn SC, Kaever T, et al. The Length Distribution of Class I–Restricted T Cell Epitopes Is Determined by Both Peptide Supply and MHC Allele–Specific Binding Preference. J Immunol (2016) 196(4):1480–7. doi: 10.4049/jimmunol.1501721
55. Bryant DH, Moll M, Chen BY, Fofanov VY, Kavraki LE. Analysis of Substructural Variation in Families of Enzymatic Proteins With Applications to Protein Function Prediction. BMC Bioinf (2010) 11:242. doi: 10.1186/1471-2105-11-242
56. Frankild S, de Boer RJ, Lund O, Nielsen M, Kesmir C. Amino Acid Similarity Accounts for T Cell Cross-Reactivity and for “Holes” in the T Cell Repertoire. PloS One (2008) 3(3):e1831. doi: 10.1371/journal.pone.0001831
57. Shrock E, Fujimura E, Kula T, Timms RT, Lee IH, Leng Y, et al. Viral Epitope Profiling of COVID-19 Patients Reveals Cross-Reactivity and Correlates of Severity. Sci (80- ) (2020) 370(6520):eabd4250. doi: 10.1126/science.abd4250
58. Kennedy PTF, Urbani S, Moses RA, Amadei B, Fisicaro P, Lloyd J, et al. The Influence of T Cell Cross-Reactivity on HCV-Peptide Specific Human T Cell Response. Hepatology (2006) 43(3):602–11. doi: 10.1002/hep.21081
59. Vita R, Mahajan S, Overton JA, Dhanda SK, Martini S, Cantrell JR, et al. The Immune Epitope Database (IEDB): 2018 Update. Nucleic Acids Res (2019) 47(D1):D339–43. doi: 10.1093/nar/gky1006
60. Conway JR, Lex A, Gehlenborg N. UpSetR: An R Package for the Visualization of Intersecting Sets and Their Properties. Bioinformatics (2017) 33(18):2938–40. doi: 10.1093/bioinformatics/btx364
61. De Groot AS, Moise L, McMurry JA, Martin W. Epitope-Based Immunome-Derived Vaccines: A Strategy for Improved Design and Safety. Clin Appl Immunomics (2008) 2:39–69. doi: 10.1007/978-0-387-79208-8_3
62. Zhao L, Zhang M, Cong H. Advances in the Study of HLA-Restricted Epitope Vaccines. Hum Vaccines Immunother (2013) 9(12):2566–77. doi: 10.4161/hv.26088
63. Su LF, Kidd BA, Han A, Kotzin JJ, Davis MM. Virus-Specific CD4+ Memory-Phenotype T Cells Are Abundant in Unexposed Adults. Immunity (2013) 38(2):373–83. doi: 10.1016/j.immuni.2012.10.021
64. Welsh RM, Selin LK. No One is Naive: The Significance of Heterologous T-Cell Immunity. Nat Rev Immunol (2002) 2(6):4177–26. doi: 10.1038/nri820
65. Pan YG, Aiamkitsumrit B, Bartolo L, Wang Y, Lavery C, Marc A, et al. Vaccination Reshapes the Virus-Specific T Cell Repertoire in Unexposed Adults. Immunity (2021) 54(6):1245–56.e5. doi: 10.1016/j.immuni.2021.04.023
66. Schulien I, Kemming J, Oberhardt V, Wild K, Seidel LM, Killmer S, et al. Characterization of Pre-Existing and Induced SARS-CoV-2-Specific CD8+ T Cells. Nat Med (2021) 27(1):78–85. doi: 10.1038/s41591-020-01143-2
67. Gupta PK. New Disease Old Vaccine: Is Recombinant BCG Vaccine an Answer for COVID-19? Cell Immunol (2020) 356:104187. doi: 10.1016/j.cellimm.2020.104187
68. Gonzalez-Perez M, Sanchez-Tarjuelo R, Shor B, Nistal-Villan E, Ochando J. The BCG Vaccine for COVID-19: First Verdict and Future Directions. Front Immunol (2021) 12:632478. doi: 10.3389/fimmu.2021.632478
69. Miyasaka M. Is BCG Vaccination Causally Related to Reduced COVID-19 Mortality? EMBO Mol Med (2020) 12(6):e12661. doi: 10.15252/emmm.202012661
70. Rha MS, Jeong HW, Ko JH, Choi SJ, Seo IH, Lee JS, et al. PD-1-Expressing SARS-CoV-2-Specific CD8+ T Cells Are Not Exhausted, But Functional in Patients With COVID-19. Immunity (2021) 54(1):44–52.e3. doi: 10.1016/j.immuni.2020.12.002
71. Tarke A, Sidney J, Kidd CK, Dan JM, Ramirez SI, Yu ED, et al. Comprehensive Analysis of {T} Cell Immunodominance and Immunoprevalence of SARS-CoV-2 Epitopes in COVID-19 Cases. Cell Rep Med (2021) 2:100204. doi: 10.1016/j.xcrm.2021.100204
72. Kared H, Redd AD, Bloch EM, Bonny TS, Sumatoh H, Kairi F, et al. SARS-CoV-2-Specific CD8+ T Cell Responses in Convalescent COVID-19 Individuals. J Clin Invest (2021) 131(5):e145476. doi: 10.1172/JCI145476
73. Prakash S, Srivastava R, Coulon P-G, Dhanushkodi NR, Chentoufi AA, Tifrea DF, et al. Genome-Wide Asymptomatic B-Cell, CD4+ and CD8+ T-Cell Epitopes, That are Highly Conserved Between Human and Animal Coronaviruses, Identified From SARS-CoV-2 as Immune Targets for Pre-Emptive Pan-Coronavirus Vaccines. SSRN Electron J (2020). doi: 10.2139/ssrn.3712675
74. Takagi A, Matsui M. Identification of HLA-A*02:01-Restricted Candidate Epitopes Derived From the Nonstructural Polyprotein 1a of SARS-CoV-2 That May Be Natural Targets of CD8+ T Cell Recognition. In Vivo J Virol (2021) 95(5):e01837–20. doi: 10.1128/JVI.01837-20
75. Abd Albagi SO, Al-Nour MY, Elhag M, Tageldein Idris Abdelihalim A, Musa Haroun E, Adam Essa ME, et al. A Multiple Peptides Vaccine Against COVID-19 Designed From the Nucleocapsid Phosphoprotein (N) and Spike Glycoprotein (S) via the Immunoinformatics Approach. Inf Med Unlocked (2020) 21. doi: 10.1016/j.imu.2020.100476
76. Barquera R, Collen E, Di D, Buhler S, Teixeira J, Llamas B, et al. Binding Affinities of 438 HLA Proteins to Complete Proteomes of Seven Pandemic Viruses and Distributions of Strongest and Weakest HLA Peptide Binders in Populations Worldwide. HLA (2020) 96. doi: 10.1111/tan.13956
77. Nguyen A, David JK, Maden SK, Wood MA, Weeder BR, Nellore A, et al. Human Leukocyte Antigen Susceptibility Map for Severe Acute Respiratory Syndrome Coronavirus 2. J Virol (2020) 94(13):e005107–20. doi: 10.1128/jvi.00510-20
78. Tomita Y, Ikeda T, Sato R, Sakagami T. Association Between HLA Gene Polymorphisms and Mortality of COVID-19: An in Silico Analysis. Immun Inflammation Dis (2020) 8. doi: 10.1002/iid3.358
79. Wang F, Huang S, Gao R, Zhou Y, Lai C, Li Z, et al. Initial Whole-Genome Sequencing and Analysis of the Host Genetic Contribution to COVID-19 Severity and Susceptibility. Cell Discovery (2020) 6(1):83. doi: 10.1038/s41421-020-00231-4
80. Mateus J, Grifoni A, Tarke A, Sidney J, Ramirez SI, Dan JM, et al. Selective and Cross-Reactive SARS-CoV-2 T Cell Epitopes in Unexposed Humans. Sci (80- ) (2020) 370(6512):89–94. doi: 10.1126/science.abd3871
81. Heitmann JS, Bilich T, Tandler C, Nelde A, Maringer Y, Marconato M, et al. A COVID-19 Peptide Vaccine for the Induction of SARS-CoV-2 T Cell Immunity. Nature (2021). doi: 10.1038/s41586-021-04232-5
82. Cui J, Li F, Shi ZL. Origin and Evolution of Pathogenic Coronaviruses. Nat Rev Microbiol (2019) 17(3):181–92. doi: 10.1038/s41579-018-0118-9
83. Tarke A, Sidney J, Methot N, Yu ED, Zhang Y, Dan JM, et al. Impact of SARS-CoV-2 Variants on the Total CD4+ and CD8+ T Cell Reactivity in Infected or Vaccinated Individuals. Cell Rep Med (2021) 2(7):100355. doi: 10.1016/j.xcrm.2021.100355
84. Geers D, Shamier MC, Bogers S, den Hartog G, Gommers L, Nieuwkoop NN, et al. SARS-CoV-2 Variants of Concern Partially Escape Humoral But Not T-Cell Responses in COVID-19 Convalescent Donors and Vaccinees. Sci Immunol (2021) 6(59):eabj1750. doi: 10.1126/sciimmunol.abj1750
Keywords: SARS-CoV-2, structural bioinformatics, BCG, vaccine, cross-reactivity, HLA, peptide
Citation: Tarabini RF, Rigo MM, Faustino Fonseca A, Rubin F, Bellé R, Kavraki LE, Ferreto TC, Amaral Antunes D and de Souza APD (2022) Large-Scale Structure-Based Screening of Potential T Cell Cross-Reactivities Involving Peptide-Targets From BCG Vaccine and SARS-CoV-2. Front. Immunol. 12:812176. doi: 10.3389/fimmu.2021.812176
Received: 09 November 2021; Accepted: 21 December 2021;
Published: 13 January 2022.
Edited by:
Christoph Wülfing, University of Bristol, United KingdomReviewed by:
Richard Kennedy, Mayo Clinic, United StatesHadida Yasmin, Cooch Behar Panchanan Barma University, India
Copyright © 2022 Tarabini, Rigo, Faustino Fonseca, Rubin, Bellé, Kavraki, Ferreto, Amaral Antunes and de Souza. This is an open-access article distributed under the terms of the Creative Commons Attribution License (CC BY). The use, distribution or reproduction in other forums is permitted, provided the original author(s) and the copyright owner(s) are credited and that the original publication in this journal is cited, in accordance with accepted academic practice. No use, distribution or reproduction is permitted which does not comply with these terms.
*Correspondence: Ana Paula Duarte de Souza, ana.duarte@pucrs.br; Dinler Amaral Antunes, dinler@uh.edu
†These authors have contributed equally to this work and share first authorship