- 1Department of Pediatrics and Human Development, College of Human Medicine, Michigan State University, Grand Rapids, MI, United States
- 2HudsonAlpha Institute for Biotechnology, Huntsville, AL, United States
- 3Infectious Disease, Helen DeVos Children’s Hospital, Grand Rapids, MI, United States
- 4Department of Mathematics, University of North Alabama, Florence, AL, United States
- 5Department of Biology, Grand Valley State University, Allendale, MI, United States
- 6Department of Science, Davenport University, Grand Rapids, MI, United States
- 7Office of Research, Spectrum Health, Grand Rapids, MI, United States
- 8Department of Biology, Athens State University, Athens, AL, United States
- 9Allergy & Immunology, Spectrum Health, Grand Rapids, MI, United States
- 10Department of Pharmacology and Toxicology, Michigan State University, East Lansing, MI, United States
- 11Department of Philosophy, The University of Akron, Akron, OH, United States
- 12Department of Critical Care Medicine and Pediatrics, Children’s Hospital of Pittsburgh, University of Pittsburgh School of Medicine, Pittsburgh, PA, United States
- 13Pediatric Intensive Care Unit, Helen DeVos Children’s Hospital, Grand Rapids, MI, United States
- 14Medical Genetics, Spectrum Health, Grand Rapids, MI, United States
In the age of genomics, public understanding of complex scientific knowledge is critical. To combat reductionistic views, it is necessary to generate and organize educational material and data that keep pace with advances in genomics. The view that CCR5 is solely the receptor for HIV gave rise to demand to remove the gene in patients to create host HIV resistance, underestimating the broader roles and complex genetic inheritance of CCR5. A program aimed at providing research projects to undergraduates, known as CODE, has been expanded to build educational material for genes such as CCR5 in a rapid approach, exposing students and trainees to large bioinformatics databases and previous experiments for broader data to challenge commitment to biological reductionism. Our students organize expression databases, query environmental responses, assess genetic factors, generate protein models/dynamics, and profile evolutionary insights into a protein such as CCR5. The knowledgebase generated in the initiative opens the door for public educational information and tools (molecular videos, 3D printed models, and handouts), classroom materials, and strategy for future genetic ideas that can be distributed in formal, semiformal, and informal educational environments. This work highlights that many factors are missing from the reductionist view of CCR5, including the role of missense variants or expression of CCR5 with neurological phenotypes and the role of CCR5 and the delta32 variant in complex critical care patients with sepsis. When connected to genomic stories in the news, these tools offer critically needed Ethical, Legal, and Social Implication (ELSI) education to combat biological reductionism.
Introduction
Genetics and genomics are complex. Nearly every scientist is trained to integrate the scientific method into research design, formulating a hypothesis and testing it. However, this method of probing scientific insights was formulated in an age with limited data and resources in a simplified, often reductionistic, biological understanding. As the amount of data generated now often overcomes what a mind can comprehend, hypothesis-driven research becomes more and more challenging, especially when clinical, real-world decision-making occurs. Focused, hypothesis-driven research in genomics can often result in overly simplified views of genes that result in reductionism when not balanced with a full view of the biological complexity. To combat these reductionistic views in genomics, it is critical to look more broadly, often non-hypothesis driven and based on the larger data analysis. It is the responsibility of the genetics community to build tools that combat misunderstanding and reductionism (1), particularly when Ethical, Legal and Social Implications (ELSI) are involved. The big data community often is embraced to move beyond gene to single-function insights to broaden our view of how genetics contributes to biology.
Throughout our educational pipeline, genomic literacy has been a growing weakness, even in well-educated individuals, potentially elevating genetic essentialism (2, 3). We cannot utilize only scientific publications to combat genomic reductionism, as these are not accessible to most individuals. Studies in high school standards (4), undergraduate education (5), medical school training (6), specialized medical fields such as nursing (7, 8), practicing physicians (9), and general public education (10, 11) all suggest weaknesses in our genomics education pipelines. Many of our genomics classes, textbooks, and resources still focus on reductionistic genetics of Punnett squares and monogenic inheritance, missing the complexity of genomics (12). In a randomized control trial, it has been shown that students with more genomic literacy prevents essentialist views of genetics (13). The Public Understanding and Attitudes towards Genetics and Genomics (PUGGS) instrument applied to first-year university students suggested that the challenges of genetic reductionism also include social factors of age and religion (14). However, a more recent assessment of the PUGGS suggests the need for reform and further applications to educational assessments (15). Through a mixed-methods approach, others have shown the need to implement culturally and linguistically diverse backgrounds into our genomics education (16), which could be accomplished with more visual aids and interactive forums. The increasing education on gene regulation, epigenetics, and gene-by-environment regulation is critically needed at earlier levels to counter reductionistic views (17). Implementing more mechanistic reasoning abilities into genomic literacy is also critical (18). Here we lay out a strategy to engage students in complex research on genes, integrating large data resources into educational tools that others can be used to broaden genomics perspectives.
The fundamental insight of CCR5 as a receptor for HIV to infect cells, and that a human variant known as delta32 (hg38 3_46373452_TACAGTCAGTATCAATTCTGGAAGAATTTCCAG_T, rs333/rs775750898, CCR5 p.Ser185IlefsTer32) in the protein corresponds to HIV resistance (19, 20), gave rise to the potential to target CCR5 for HIV treatment and prophylaxis (21–23). What started as a potential to edit blood cells to give host HIV resistance (24, 25) created ambiguity and opportunities for scientists to perform the gene editing in human germline experiments. Yet, these germline experiments leave out many of the risks of the experiments ranging from off-target activity (26) to the role CCR5 plays in normal cell, tissue, and organ biology (27), which can be compensated by the complex multivariant inheritance of delta32. While the potential for CRISPR editing of CCR5 to create HIV resistance is intriguing (28), a more complex understanding of CCR5 biology is critical. HudsonAlpha Institute for Biotechnology and Michigan State University formulated the Characterizing Our DNA Exceptions (CODE) program to advance knowledge of genetic variation and provide insights into genetics through a research program for undergraduate and graduate students in performing gene-centric data surveillance and integration into knowledge. This program created an opportunity for students within our CODE program to build CCR5 tools for educational use to broaden understanding of the biology of CCR5. This work describes the tools and resources integrated for a richer, more complex view of CCR5, with tools and resources accessible outside of our traditional publication system that does not often reach those needing enhanced genomic literacy.
The C-C Chemokine Receptor Type 5 (CCR5) is a G-protein coupled receptor (GPCR) prominently known for its role as the co-receptor (with CD4 as the primary receptor) in HIV infection. However, this receptor has many roles outside of the infectious disease realm. CCR5 is predominantly located on the cell membrane of macrophages, T-cells, Hofbauer cells, and Kupffer cells with minor expression on epithelial cells, type 2 alveolar cells, fibroblasts, and B-cells (29). When comparing CCR5 expression among T cell subpopulations, it was found to be specific to TH1 T-cells (30) and CD8+ T-cells (31) as opposed to the TH2 subpopulation involved in allergy and parasitic responses, which were more specific for CCR3 (32). Ellwanger et al. have laid out many of the pros and cons of CCR5 removal, including a detailed literature review of the many experiments performed for CCR5 biology outside of HIV (33). In 2009, it was well laid out that the recent emergence of the HIV infection could not be a sole explanation for the emergence of delta32, instead suggesting a push-pull aspect of immune activation, where inhibition of immune overactivation due to infection or autoimmunity could be evolutionarily advantageous, but with consequences to immune system components (34). This is further defended by data in CCR5 knockout models, which suggest advantageous roles in decreased immune activation (35–37) while having neurological complications (38, 39) and, in some cases, blunted immune response to pathogens (40, 41). As CCR5 has also been extensively linked to autoimmunity and autoimmune liver diseases, targeting it with therapeutics has been suggested (42–44).
CCR5 is a receptor for several CC-chemokines, including CCL3 (MIP-1-alpha), CCL4 (MIP-1-beta), and CCL5 (RANTES), which induce intracellular signal amplification via activation of the AKT and NF-KB pathways (45, 46). Both CCL3 and CCL4 are predominantly produced and secreted by T-cells, Hofbauer cells, macrophages, and Kupffer cells, while CCL5 has higher expression and secretion by T-cells (29). When bound to CCR5, CCL3 plays a significant role in T-cell chemotaxis and transmigration with similar activities in macrophages and other immune cells (47, 48). CCL4 is a potent chemotactic factor for neutrophils (49), with knock-out studies demonstrating decreased neutrophil chemotaxis to sites of inflammation (50). CCL5 plays a role in the cellular migration of T-cells, NK cells, macrophages, eosinophils, and basophils (51). CCL5 production has also been shown to reduce HIV entry into host cells (52). Homology within the C-C Chemokine Receptor family may compensate for some of the CCR5 biology, but the extent to which these mechanisms can compensate for the broad phenotypes of CCR5 ligand activation within individuals carrying the delta32 or other CCR5 variants is not well understood. Therefore, we have integrated our CCR5 knowledgebase with that of the larger C-C Chemokine Receptor family and broader phenotypic knowledge, using publicly available data, to expand our understanding of CCR5, which is critical in establishing a broader biological context for understanding the consequences of genetic manipulation. This example demonstrates how public data needs to be better integrated before setting out on high-risk clinical experiments.
Methods
Amino Acid Knowledgebase and Human Genomic Variants
The human CCR5 protein sequence (UniProt P51681) was assessed on NCBI BLAST (53) against the Homo sapiens UniProtKB/Swiss-Prot database, and the top 100 hits were extracted for the canonical UniProt isoform. These 100 sequences were aligned using ClustalW (54), alignment available at https://doi.org/10.6084/m9.figshare.16619983, and a phylogenetic tree (https://doi.org/10.6084/m9.figshare.16619950) was constructed using MEGA (55) with 500 bootstrap calculations. Amino acids of the alignment were exported into Excel, where the conservation to all 100 GPCR sequences was calculated for each amino acid of human CCR5. The conservation was also calculated for the top 16 BLAST hits with an E-value less than 1E-50 (CCR5, CCR2, CCR1, CCR4, CCR3, CCR8, CCRL2, CCR9, CX3CR1, CCR6, XCR1, CCR7, CXCR4, ACKR2, ACKR4, CXCR6). Vertebrate orthologs of CCR5 were extracted from NCBI ortholog as RefSeq transcripts, which were parsed for open reading frames using TransDecoder-v5.5 (56) and aligned using ClustalW codon (alignment available at https://doi.org/10.6084/m9.figshare.16619986). The translated amino acid sequences were assessed for percent conservation relative to the human CCR5 sequence or were assessed for functional conservation based on hydrophobic (A, V, I, L, M, F, Y, W), aromatic (F, Y, W, H), polar basic (R, H, K), polar acidic (D, E), or Ser/Thr (S, T) amino acids. Codon selection and linear motif analysis of the open reading frame alignment were calculated as previously described (57, 58). Knowledge for human CCR5 topology, modifications, mutagenesis, and natural variants were extracted from the UniProt database (59) on 6/15/2021.
The human CCR5 (UniProt P51681) protein was modeled using homology modeling in YASARA (60), which merged PDB files 5UIW, 5T1A, 5LWE, and 4RWS. The single merged structure was energy minimized with a pH-based pka setting of 7.4 within a phosphatidyl-ethanolamine (PEA) lipid membrane and 0.997g/mL water equilibrated across the membrane using YASARA md_runmembrane macro. Molecular dynamic simulations (mds) were run for the membrane-embedded CCR5 with 14,206 explicit water molecules, 48 Cl, and 33 Na giving a compiled 67,402 atoms for 300 nanoseconds (ns) using the AMBER14 force field (61), and atomic positions collection every 100 picoseconds for analysis. The analysis was performed using YASARA macros md_analyze and md_analyzeres (yasara.org/macros.htm), using a correlation cutoff for each amino acid of >0.9 in dynamic cross-correlation matrix (DCCM) calculations. All the mds trajectory and analysis files can be found at https://doi.org/10.6084/m9.figshare.15134979, allowing for a full reanalysis as needed.
All CCR5 missense and loss-of-function (LoF) variants were extracted from gnomADv2.1 nonTOPmed (62), COSMIC (63), Bravo for TOPmed variants (64), and ClinVar (65) on 11/29/2018. CCR5 missense variants were extracted from Geno2MP (66) on 6/15/2021. All missense and LoF variants were compiled, and each unique change was assessed with PolyPhen2 (67), Provean (68), SIFT (69), and Align-GVGD (70), where the variant was scored 1 for damaging equivalent predictions of each tool. A variant impact score was calculated by adding the functional prediction scores with our codon selection score (max of 2) and multiplying that by the functional conservation score, our linear motif conservation score, and the total allele observations for the variant from gnomAD, TOPmed, ClinVar, COSMIC, and Geno2MP. The top five highest impact scores had the Geno2MP phenotypes extracted on 6/15/2021.
Public Dataset Generation
The 3D model of CCR5 was recorded for molecular videos using python scripted movement within the YASARA molecular modeling tools (60). The video files were uploaded into FigShare and YouTube, with links provided in the results section. The 3D coordinates were saved as a PDB file and loaded into PyMol (https://pymol.org/2/) to generate colored files for 3D printing, saving the files in VRML format and submitted to FigShare or Shapeways. Delta32 variant allele frequency was extracted from gnomADv2.1 (62). The CCR5 website was built using WordPress.
RNA Expression Analysis
The genome browser images and all GWAS variants near CCR5 were extracted from the UCSC genome browser (71) on 9/6/2021. CCR5 eQTLs were extracted from GTEx version 8 (72) on 9/6/2021. Open Targets Genetics (73) was used for the understanding of GWAS and pheWAS associations. Samples from our previous RNAseq work and details of methods used can be found in the three publications for MODS, RSV, or COVID-19 (74–76). All PAXgene tube blood RNAseq datasets within the NCBI SRA were downloaded with the SRA toolkit (https://trace.ncbi.nlm.nih.gov/Traces/sra/sra.cgi?view=software) and processed for abundance using Salmon_0.14.1 (77) and the Gencode38 transcriptome (78). Microglia datasets were extracted from BioProjects PRJNA649597, PRJNA662330, PRJNA665286, PRJNA667596, PRJNA688478, PRJNA689841, PRJNA387182, PRJNA483247 and the blood RNAseq datasets from BioProjects PRJEB14743, PRJEB20731, PRJEB23048, PRJEB27958, PRJEB27965, PRJEB33892, PRJEB36928, PRJEB41073, PRJEB44660, PRJNA201433, PRJNA230906, PRJNA232593, PRJNA251404, PRJNA277352, PRJNA305001, PRJNA315611, PRJNA327986, PRJNA329148, PRJNA352062, PRJNA354367, PRJNA357628, PRJNA358580, PRJNA369684, PRJNA378794, PRJNA380820, PRJNA384259, PRJNA390289, PRJNA397222, PRJNA398240, PRJNA401870, PRJNA427575, PRJNA437114, PRJNA454694, PRJNA476781, PRJNA493832, PRJNA494155, PRJNA504827, PRJNA511891, PRJNA526259, PRJNA526839, PRJNA533086, PRJNA552286, PRJNA562305, PRJNA588242, PRJNA591657, PRJNA600846, PRJNA601661, PRJNA607120, PRJNA630674, PRJNA632871, PRJNA634938, PRJNA638653, PRJNA639278, PRJNA647880, PRJNA664368, PRJNA679264, PRJNA679331, PRJNA680771, PRJNA683803, PRJNA686397, PRJNA702558, PRJNA728070 in addition to our groups studies on MODS, RSV, and COVID-19 (74–76). All Gencode38 mapped reads for these samples can be found at https://doi.org/10.6084/m9.figshare.16658449.v1. To calculate CCR5 delta32 read frequency we created a fasta file containing all isoforms of CCR5 and several additional paralog isoforms (https://doi.org/10.6084/m9.figshare.16649830.v1) that was indexed and assessed using Salmon, where the percent of reads containing delta32 were compared to the reads without the variant to calculate abundances for the variant from RNAseq datasets.
Results
CCR5 Evolutionary Insights
CCR5 is a member of the GPCR superfamily. A BLAST analysis of the human CCR5 against other human protein sequences revealed the top 100 hits have E-values less than 5.23E-13, and percent identify greater than 22%. Phylogenetic reconstruction of these 100 GPCR human proteins shows that CCR5 clusters next to CCR2 and near CCR1, CCR3, CCRL2, CCR4, CCR8, CX3CR1, XCR1, and ACKR2 (Figure 1A). Using these 100 GPCR sequences, the percent of amino acids the same as CCR5 was calculated for each of the human CCR5 amino acids, where 12 amino acids (3.4%) are conserved >90%. In addition, the top 16 BLAST hits were also assessed for conservation with CCR5, where 26 amino acids (7.4%) are conserved >90%. A total of 98 vertebrate orthologs of CCR5 were assessed for codon selection, linear motifs, amino acid conservation, and functional amino acid conservation. The alligator CCR5 represents the most divergent sequence within CCR5 orthologs with 54% conservation of amino acids with human. A total of 186 amino acids (52.8%) are conserved >90% in CCR5 orthologs. These conserved amino acids at the GPCR, top 16, and CCR5 ortholog levels mapped onto a model of the CCR5 structure reveal a broad GPCR conservation in the core, 16 most similar conservation in several clusters, and broad CCR5 conservation of the transmembrane, intracellular, and extracellular residues (Figure 1B). The fact that other chemokine receptors show a lack of conservation at the ligand-binding interface challenges the notion that they could potentially compensate for CCR5 loss, in agreement with Ellwanger et al. (79).
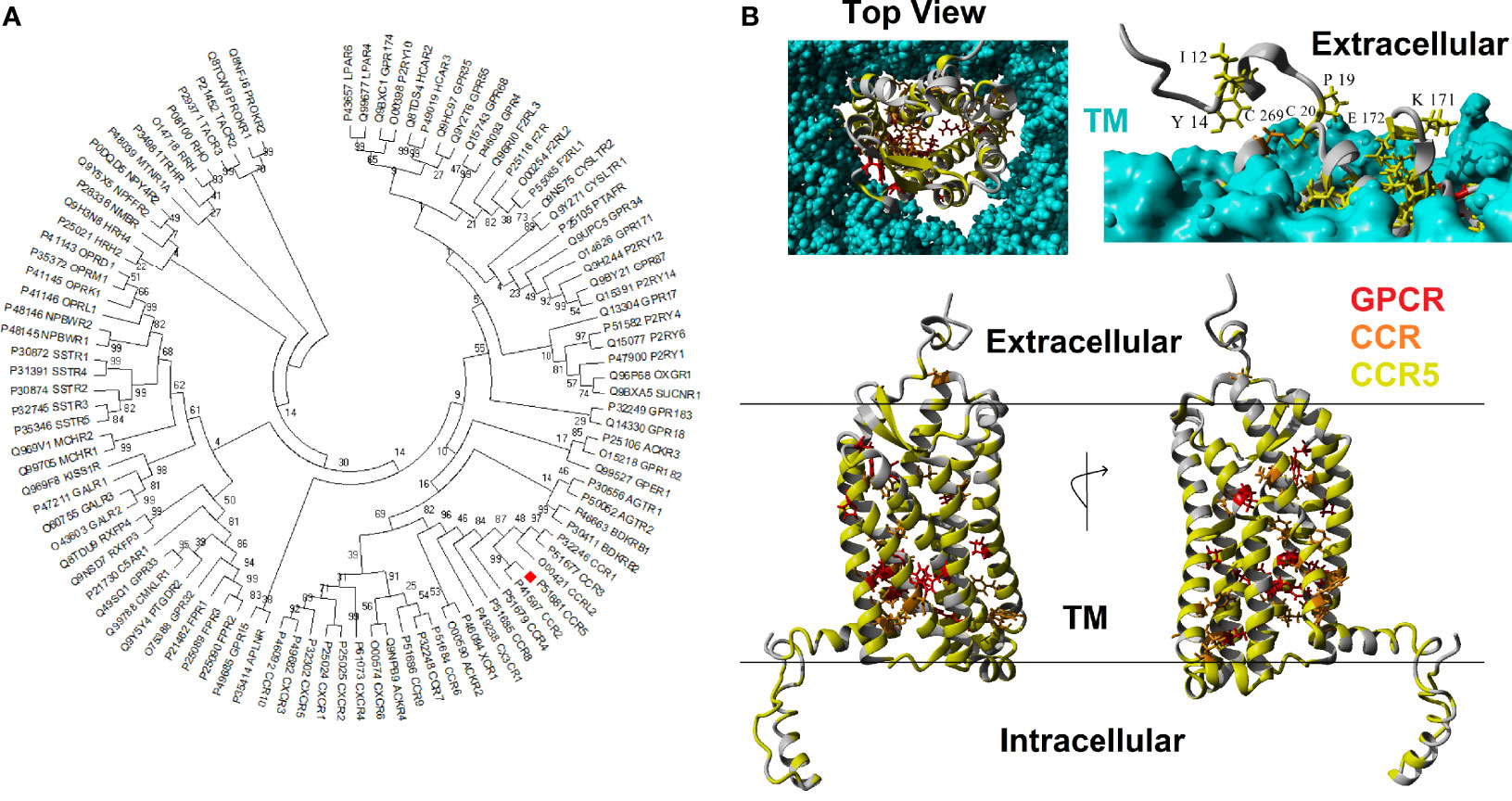
Figure 1 Human CCR5 paralogs. (A) Phylogenetic tree of the top 100 human paralogs for CCR5 protein. Values at each node represent the percent of trees that cluster out of 500 bootstrap analyses. CCR5 is marked with a red box.(B) Model of human CCR5 with conservation colored. The transmembrane is colored cyan, amino acids conserved >90% in 100 GPCRs in red, conserved >90% in 16 most related CCR5 paralogs in orange, and conserved >90% in CCR5 vertebrate orthologs in yellow. The top left shows the view of CCR5 from extracellular space looking into the GPCR. The top right shows the side view with the transmembrane to visualize exposed and conserved residues, which are labeled. The bottom shows a 180° rotation of side view of CCR5.
CCR5 Amino Acid Knowledgebase
The conservation data from above was compiled with molecular dynamic simulation (mds) data, UniProt insights, and known genomic variants for each of the human CCR5 amino acids to make a CCR5 amino acid knowledgebase (https://doi.org/10.6084/m9.figshare.16619974). The mds were generated by embedding the CCR5 protein of amino acids 6-352 into a PEA membrane, equilibrating water on the intra and extracellular portions, and simulating the protein movement for 300 nanoseconds. The mds tools use physics approximations of atomic movement at the femtosecond time scale, allowing users to determine the chemical environment around each amino acid of the modeled structure, providing information on the stability of movement, secondary structure, and how each amino acid correlates with every other amino acid. By recording the trajectory of amino acid movement using root-mean-squared deviation (RMSD) of the carbon alpha position, we know that the protein reaches an equilibrium of movement around ten nanoseconds of simulation, allowing for us to capture hundreds of nanoseconds of stabilized movement. The seven transmembrane helices of the GPCR structure all have a stable, low movement as reflected by a root-mean-square fluctuation (RMSF) below 4Å. The N- and C-termini both have high levels of RMSF, >10Å, reflective of decreased stability of the structure. A total of 15.0% of the amino acids are predicted in the initial structure to have coiled structure, 76.9% helical, 2.9% beta-sheet, and 5.2% as turns. A total of 70% of the amino acids have one or more amino acids that correlate with their movement greater than 0.9 based on dynamics cross-correlation matrix calculations. A total of eight amino acids have 10 or more amino acids in correlation >0.9 (10 = 54,55,69,149; 11 = 52,66,67; 13 = 70). These calculation values were included in the supplemental file’s main amino acid knowledgebase matrix (https://doi.org/10.6084/m9.figshare.16619974).
Genomic missense variant extraction from gnomAD (population variants useful for allele frequency insights), TOPmed (population variants), ClinVar (disease-associated variants), COSMIC (somatic cancer variants), and Geno2MP (disease-associated variants with correlated phenotype) identified 403 unique variants for CCR5. Based on gnomAD allele frequencies, the average variant was found in 0.0079% of the population, with only a single missense variant (L55Q) found in more than 1% of individuals. Of the 403 variants, they fell on amino acids with an average of 89.6% conservation in CCR5 orthologs with 39% of variants with a conservation >99%. A total of 27% of the variants were predicted probably damaging by PolyPhen2, 54% deleterious by Provean, 60% damaging by SIFT, and 12% as class C55/C65 by Align-GVGD. Only 9% of these variants were predicted bad outcomes in all four tools. To prioritize variant assessments, we used a combined variant impact calculation with an average score of 89,761. The top ten variants were L55Q, R223Q, A73V, V131F, S63C, T288A, L121R, G106R, V46M, R60S. Finally, we added into the amino acid knowledgebase the UniProt extracted data for topology (extracellular, transmembrane helices, or intracellular), posttranslational modification (PTMs: sulfotyrosine, O-linked GalNAc, disulfide bonds, S-palmitoyl, phosphorylation), and known experimental mutagenesis/natural variant insights on ligand binding and protein expression/size. The number of variants and the top impact score for variants were brought into the compiled amino acid matrix with all other datasets, allowing for multidimensional data insights for each variant, for example, the top ten variants and the PTMs (Table 1).
Launch of Public Tools for CCR5 Education
Our CODE students and faculty integrated our amino acid knowledgebase into additional tools and resources for the education of CCR5 protein structural insights, gene sequences throughout many species, and human variants in large databases (Figure 2). Many of the tools developed focus on the CCR5 delta32 variant (Ser185IlefsTer32). From the 3D models, we have generated a video of CCR5 and delta32 that brings to light the extreme mutation that results in 0% protein function from the allele. A long ~2 min video is available as a MPG file on FigShare (https://doi.org/10.6084/m9.figshare.16628905) and a video file on YouTube (https://youtu.be/74w2N51tSOg). The video shows the model of CCR5 embedded into a lipid membrane, rotating around all axes with the location of critical conserved amino acids and the delta32 variant. A shortened 14-second video of only delta32 is available as a MPG file (https://doi.org/10.6084/m9.figshare.16628956). A one-minute video of the movement of residues from the mds trajectory is available as an MPG file (https://doi.org/10.6084/m9.figshare.16628854) and a YouTube link (https://youtu.be/WaoPfQXA8Pg). To facilitate a hands-on interface with CCR5, we have created a 3D printing model of CCR5 and delta32 available as a VRML file (https://doi.org/10.6084/m9.figshare.16628962) and as large (https://www.shapeways.com/product/8XEK5N2EF/ccr5-large?optionId=147670860&li=shops), or small (https://www.shapeways.com/product/VNX7TE26B/ccr5-protein-model?optionId=127830241&li=shops) sized print that can be ordered from Shapeways for delivery. The small model provides a low-cost option, which our group has made into jewelry or keychains for distribution at genomic educational events. The large print works well for classrooms for students to hold and explore.
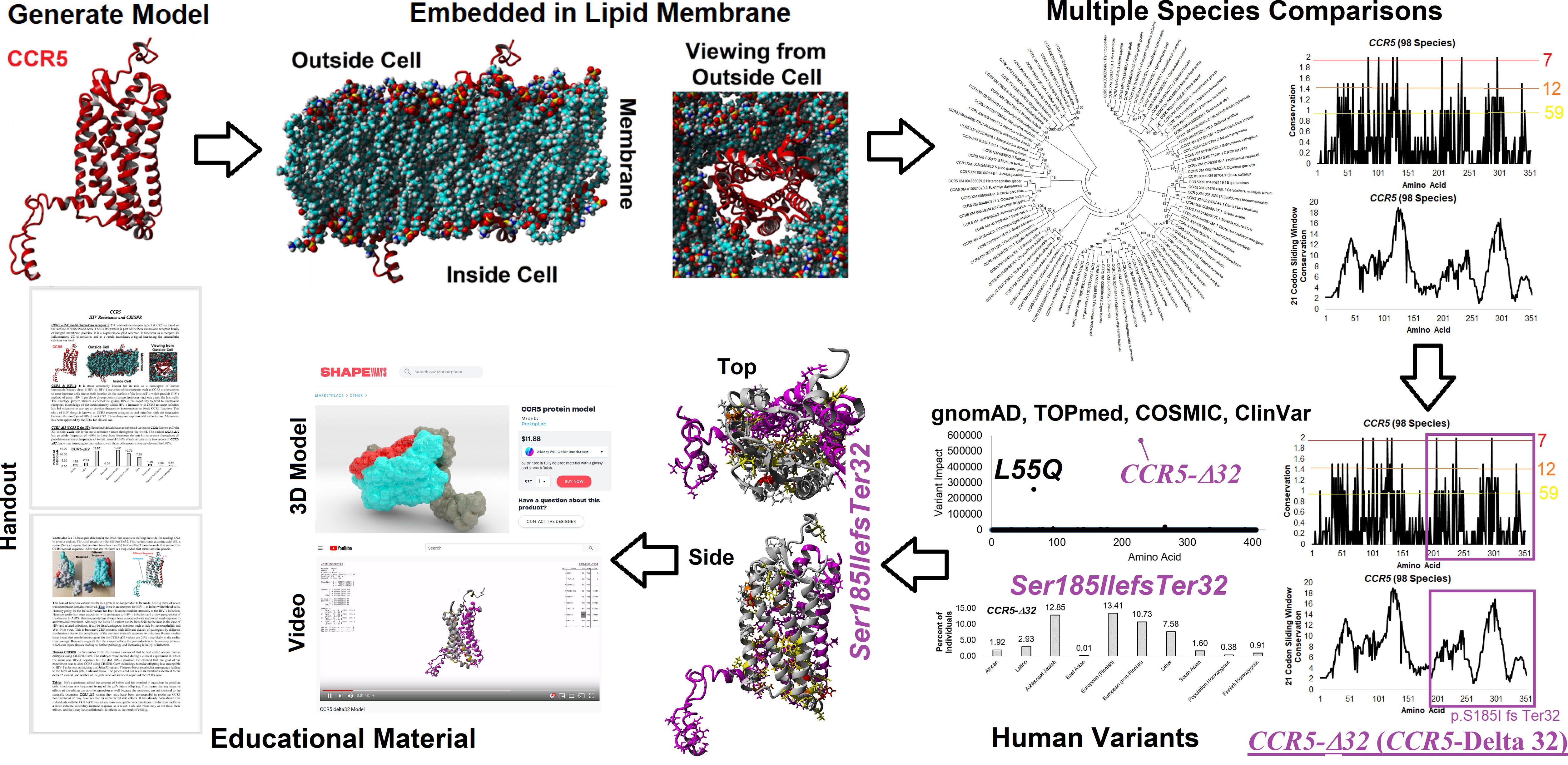
Figure 2 Amino acid knowledgebase of CCR5 used for educational insights. CODE students generated protein models, which were embedded into a lipid membrane and run for molecular dynamics simulations. These values were combined with multiple species analysis of CCR5 evolution and genomic variant extractions from gnomAD, TOPmed, COSMIC, and ClinVar. This amino acid knowledgebase was then used to assess the delta 32 variant and generate various educational handouts, videos, and 3D printed models.
To make access to these files easier, we have created a web resource page (https://prokoplab.com/ccr5-and-hiv/). From this site, anyone can obtain the video of CCR5, sequence data, insights on delta32, and a handout used in classrooms. In addition, visitors can order 3D printed models of CCR5 with the delta32 location marked in red. The two-sided handout (https://doi.org/10.6084/m9.figshare.16628815) walks students through the biological role of CCR5, how many sites on the protein are conserved throughout evolution, and details of delta32. This handout pairs well with the large and small 3D prints of the protein. All material is provided to the public for free except the 3D printed models, offered at production cost with no markup. The generation of this material by students and faculty from the CODE program represents an exciting new potential framework in the genomic era that can be expanded to many additional variants and proteins moving forward.
CCR5 Expression Insights
Additional insights about CCR5 are available through public datasets of expression and noncoding variants, broadening the insights and knowledge to challenge genomic reductionism. One effective way to combat genetic reductionism is to demonstrate that the expression of CCR5 is not only subject to variation in the gene but is also highly dependent on the molecular context, such as genes proximate to CCR5, epigenetic factors, as well as the type of cell in which expression occurs. The CCR5 gene is located on chromosome 3 from bases 46,370,854 to 46,376,206 (based on hg38 annotation). Near CCR5 are multiple cytokine receptor genes and many known genomic associations from genome-wide association studies (GWAS), including the strongest locus for severe COVID-19 (Figure 3A). This COVID-19 locus (80) has its highest association signal over the SLC6A20 gene and does not overlap the CCR5 gene body (Figure 3B). Located around 57 kilobases near CCR5 are associations for multiple immune system-connected phenotypes notable for lymphocytes, monocytes, and macrophages (Table 2). Of the variants in this region, several contribute to observed changes in CCR5 expression based on expression quantitative trait loci (eQTL), with the strongest associations seen in whole blood and lung, which contain large portions of monocytes and macrophages (Table 3). It should also be noted that multiple eQTLs were observed in brain tissue. The top SNP for blood CCR5 expression influence, rs76258812, is also an eQTL for the other paralogs of cytokine receptors near CCR5, including CCR1, CCR3, and CCR2 (https://genetics.opentargets.org/variant/3_46318831_T_C), making it difficult to determine if the monocyte associated GWAS are from CCR5 or these other genes. This variant is also found throughout multiple subpopulations with the highest known allele frequency in East Asian ancestry. Contrary, the top two variants for the brain CCR5 expression, rs9862021, and rs140177427, are rarer. rs9862021 is found highest in 10% of African ancestry and has no trait associations. rs140177427 is found highest in 10% of Finnish ancestry and is associated with various lymphocyte and macrophage phenotypes (https://genetics.opentargets.org/variant/3_47234712_G_A).
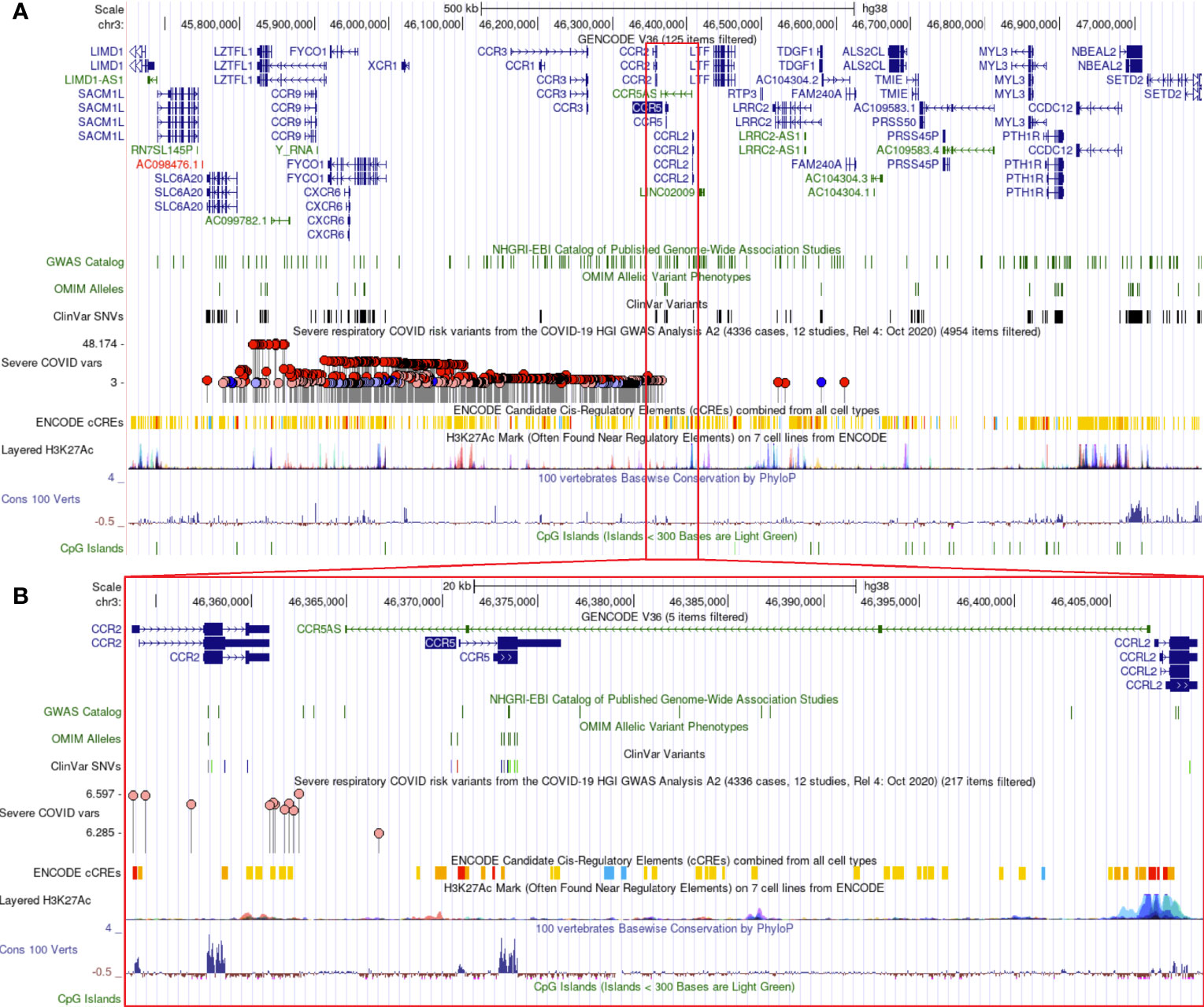
Figure 3 Genomic architecture around CCR5. The genome browser view (hg38) of around one million bases near CCR5 (A) or zoomed into around 40,000 bases (B). Tracks shown include the Gencode transcripts, known variants from GWAS/OMIM/ClinVar, the high-risk COVID-19 loci (higher dots are the strongest signal), various gene regulation insights (ENCODE cCTEs, H3K27Ac, CpG Islands), and evolutionary conservation (Cons 100 Verts).
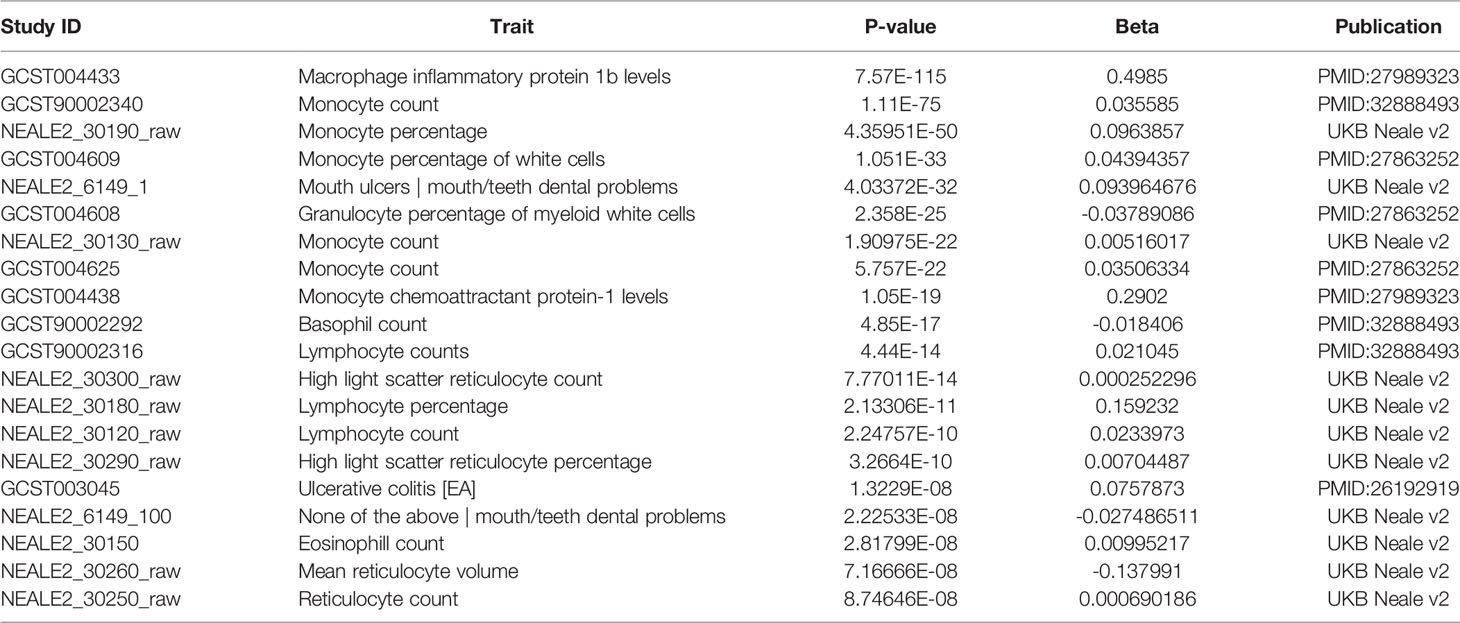
Table 2 Traits associated with variants within the CCR5 gene region (chr3:46,353,419-46,409,888, hg38) of Figure 3B.
Expression across broad tissues of the human protein atlas (HPA) (29) and GTEx (72) show higher levels in immune tissues such as spleen, tonsils, appendix, and lymph nodes and tissues associated with immune cells like blood, lung, and intestine. Further dissection of cell types within HPA shows high CCR5 expression in T-cell levels in blood and macrophages in peripheral tissues. Therefore, we utilized a large single-cell RNAseq repository, PanglaoDB (81), to identify further cell types of importance for CCR5 expression, identifying a large number of microglia and macrophage identified cell experiments (Figure 4A). The macrophage and monocyte annotations come from broad tissues, including the liver, vessels, lung, and heart (Figure 4B). The microglia annotations come from whole-brain isolates and specific brain regions (Figure 4C). It should be noted that all of the microglia insights come from isolates of mouse brains, where there are no human brain single-cell RNAseq datasets integrated into PanglaoDB. In the mouse, the knock-out of CCR5 is associated with multiple neurological phenotypes, including microglial alterations and abnormal spatial learning (Table 4).
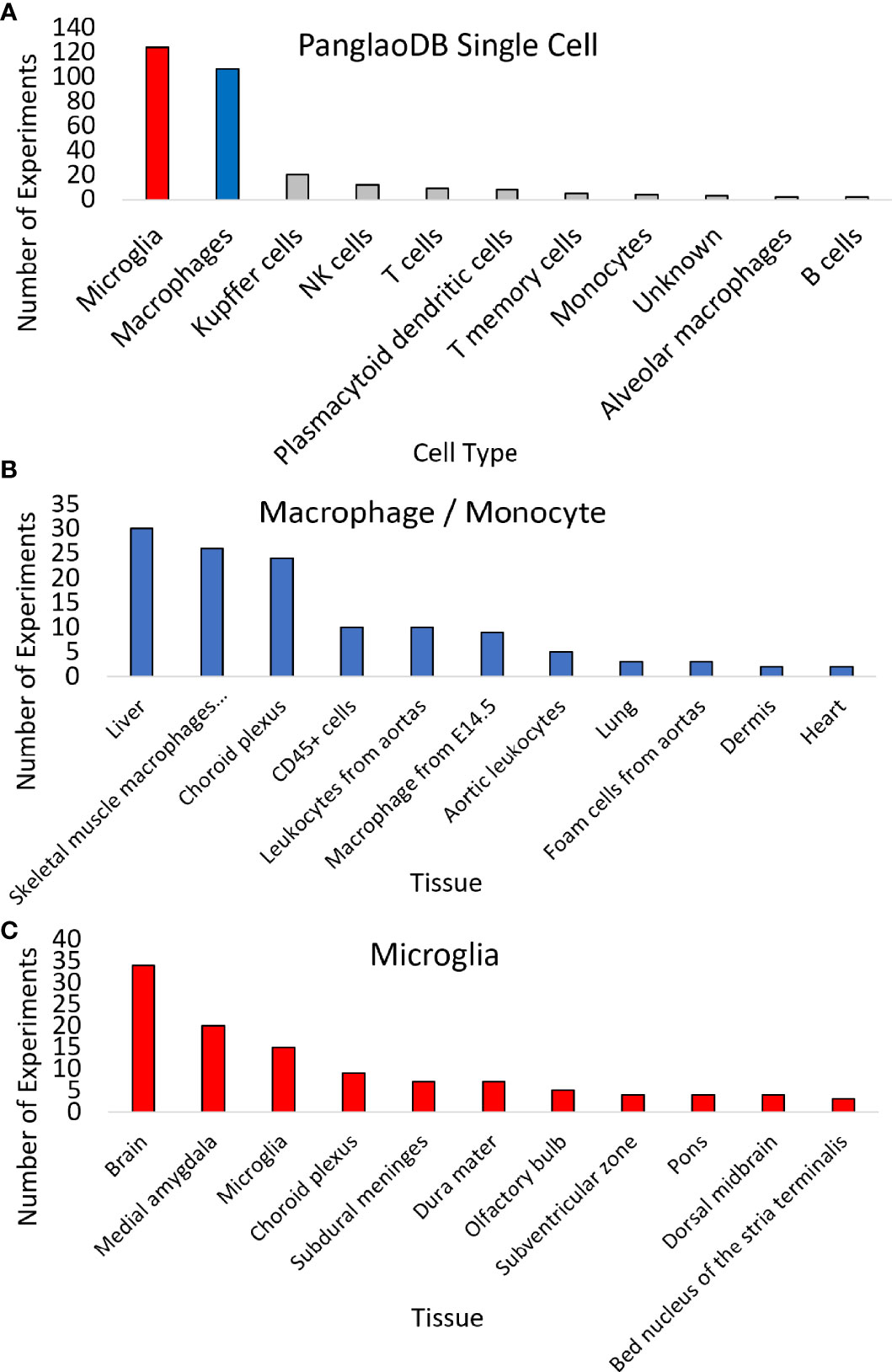
Figure 4 Expression of CCR5 in 1,063 mouse single-cell datasets. (A) PanglaoDB analysis of experiments that CCR5 was detected in various cells based on single-cell analysis. (B, C) The number of times sample types showed CCR5 expression for macrophages/monocytes (B) or microglia (C).
CCR5 Role in Brain Development
To further dissect the brain and microglia insights into human biology, we integrated several additional datasets. The human brain microarray of the Allen Brain atlas suggests 16 genes that correlate >0.7 in expression with CCR5 in 500 human brain samples. Most are also highly expressed in the mouse single-cell datasets of PanglaoDB for microglia (Figure 5A). Most of these genes code for proteins that are known to interact and enrich synapse pruning and microglia phenotypes (Figure 5B). To further show the expression of CCR5 in isolated microglia, we pulled all human RNAseq paired-end datasets from the NCBI SRA mentioning “microglia.” We annotated them relative to the Gencode38 transcriptome using a quasi-based alignment strategy (Figure 5C). All Gencode38 mapping data for each sample can be found at https://doi.org/10.6084/m9.figshare.16649842. The HMC3 cell line (PRJNA649597) that is supposed to mimic microglia cells does not express any CCR5. Contrary iPS (induced pluripotent stem cell) derived microglia (PRJNA662330, PRJNA665286, PRJNA688478, PRJNA483247) and purified primary microglia (PRJNA387182) all show expression of CCR5.
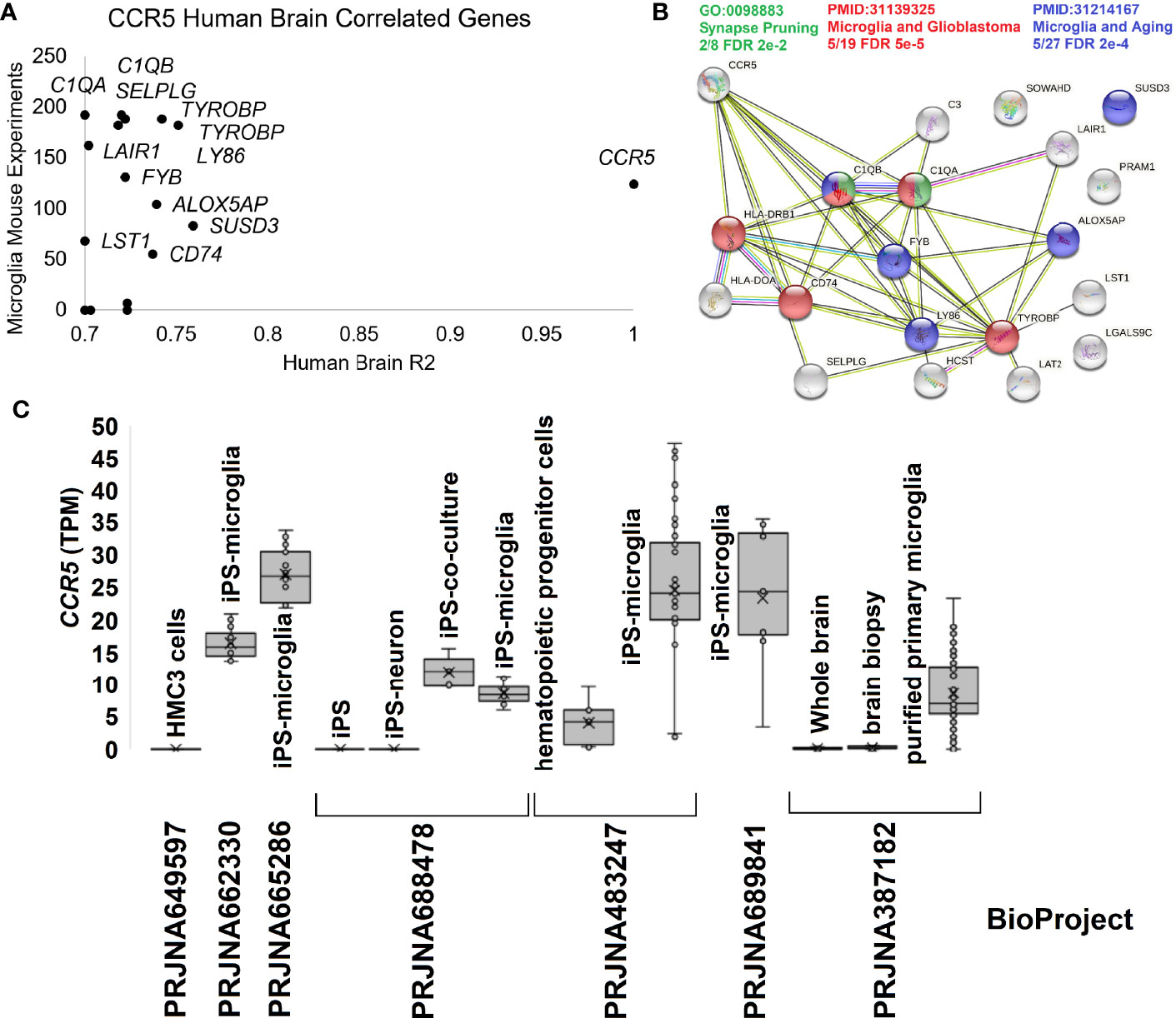
Figure 5 Human brain CCR5 and microglia. (A) The correlation of genes to CCR5 from the Allen Brain Atlas Human Brain microarray data for 500 samples relative to their expression in mouse microglia single-cell experiments. The x-axis shows the genes R2 from microarray relative to CCR5 expression, while the y-axis shows the number of experiments the gene is detected in mouse single-cell datasets for microglia. (B) STRING protein network for genes in panel (A) showing the enrichment of synapse pruning (green), microglia/glioblastoma (red), and microglia/aging (blue) genes. (C) Expression of CCR5 in transcripts per million (TPM) from seven BioProjects of human microglia cells or related experiments.
Broadening to the more extensive integrated STRING network for CCR5 shows interaction with many chemokines while identifying multiple genes connected to the central nervous system and microglia biology relative to HIV (Figure 6) (89, 90). Using our amino acid knowledgebase, we ranked the top five variants (L55Q, R223Q, A73V, V131F, S63C) followed by extracting human phenotypes associated with these changes in the Geno2MP database. A total of 273 affected individuals have a phenotype with one of these top five variants. Of these, 73 affected individuals (27%) are indicated as “Abnormality of the nervous system” in the broad term (Table 5). This is the top-ranked phenotype with “Abnormality of the cardiovascular system” at 21% and “Abnormality of the musculature” at 11%. The only neurological phenotype associated with homozygous CCR5 variants was L55Q associated with “Abnormality of brain morphology” with seven additional heterozygous individuals with the variant and individuals with R223Q, A73V, V131F, and S63C also having this annotation. Individuals with all five variants are also identified with “Intellectual disability”. Several variants are associated with “Epileptic encephalopathy”. The L55Q is found in ~1.5% of the population, and therefore association to phenotypes could be random; however, the 21 patients with neurological phenotypes with R223Q (AF=0.005) and 18 with the other three (A73V, S63C, V131F, AF average of 0.0006) suggest an enrichment from random probability. The Geno2MP database contains data for 19,344 individuals with phenotypes, where 458 have one of these five CCR5 variants. Based on allele frequencies, this number is expected to be 384, with an enrichment of 1.2. The V131F has an enrichment of 8.2 in observations relative to expect allele frequency, A73V of 4.3, and S63C of 2.2, suggesting these variants are hyper observed within Geno2MP. Moreover, with the enrichment of neurological phenotypes for individuals with these variants, it seems likely that there is an association with rare variants in CCR5 for neurological phenotypes.
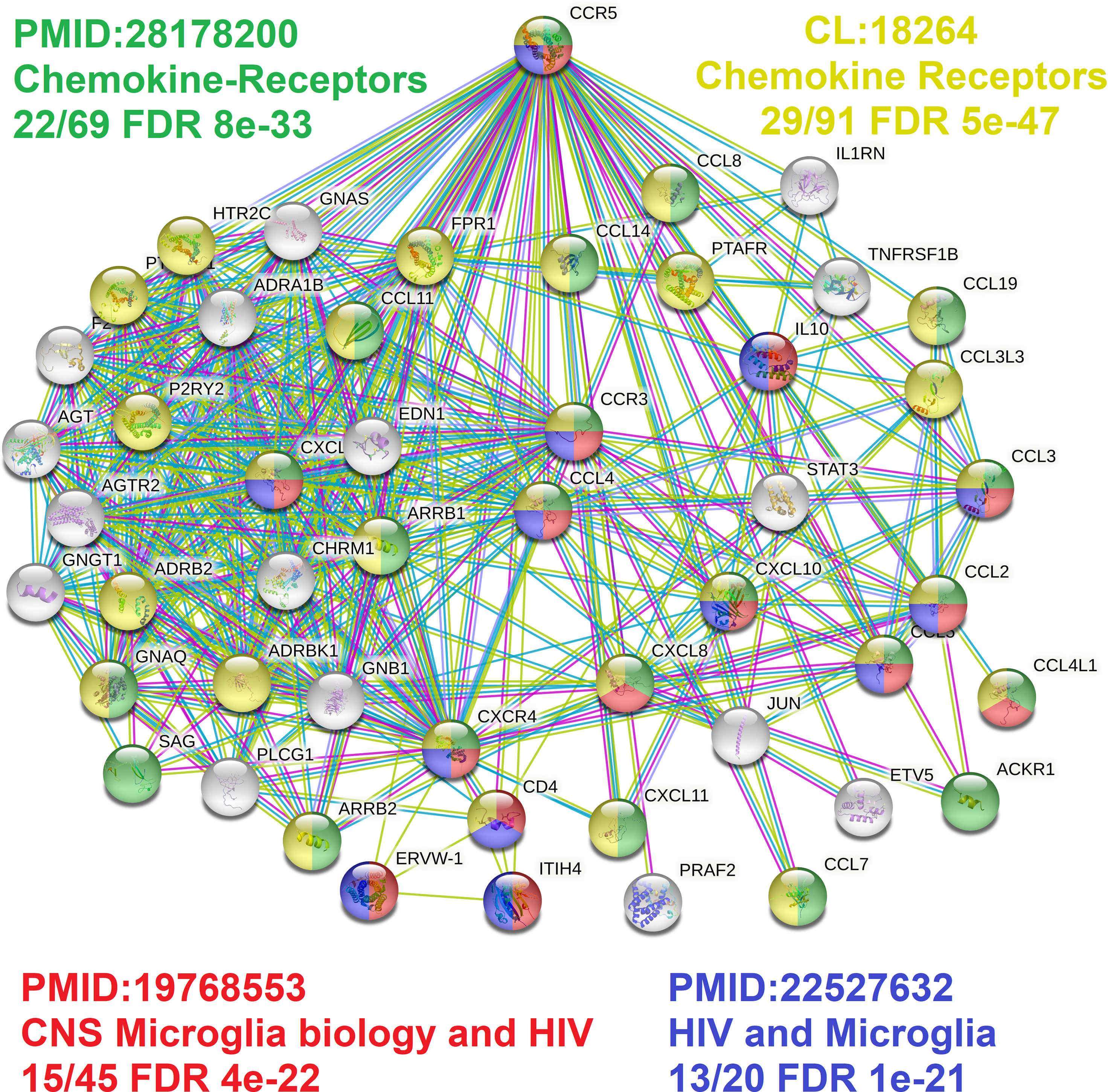
Figure 6 CCR5 protein network highlighting microglia factors. The STRING protein network for the top 50 proteins interacting with CCR5. In green/yellow are various genes annotated to chemokine biology, and in red/blue are genes connected to microglia biology.
CCR5 in Human Blood Samples
The phenotypes seen in the Geno2MP data suggested a broader analysis of CCR5 in disease pathologies. With the robust expression of CCR5 in blood and our history in studying the blood of immune challenged individuals, we selected to process CCR5 biology for blood-based RNAseq of 7,280 samples from 62 different BioProjects and three of our studies where we have patient-to-transcriptome insights (74–76) (Figure 7). All these samples were blood collected into RNA PAXgene tubes to standardize sample collection. Expression of CCR5 is highly variable across BioProjects (Figure 7A), suggesting that the TPM data is influenced by the RNA isolation or sequencing technique (polyA vs. total RNAseq). Therefore, we normalized the TPM data with a BioProject Z-score, identifying several samples with elevated CCR5 expression (Figure 7B). The highest Z-score was observed in SRR5225514 (12.4), a 17-year-old (yo) female control sample, yet very little is deposited into the SRA about this individual. This can also be said for SRR3236097 (8.8, 17 yo male control), SRR12291502 (8.3, male sepsis case), SRR3236039 (8.0, 17 yo male TB patient), SRR5902058 (7.4, male malaria vaccinated individual), SRR13224554 (6.1, 41 yo HIV-infected patient), and SRR3235984 (5.9, 14 yo female TB patient).
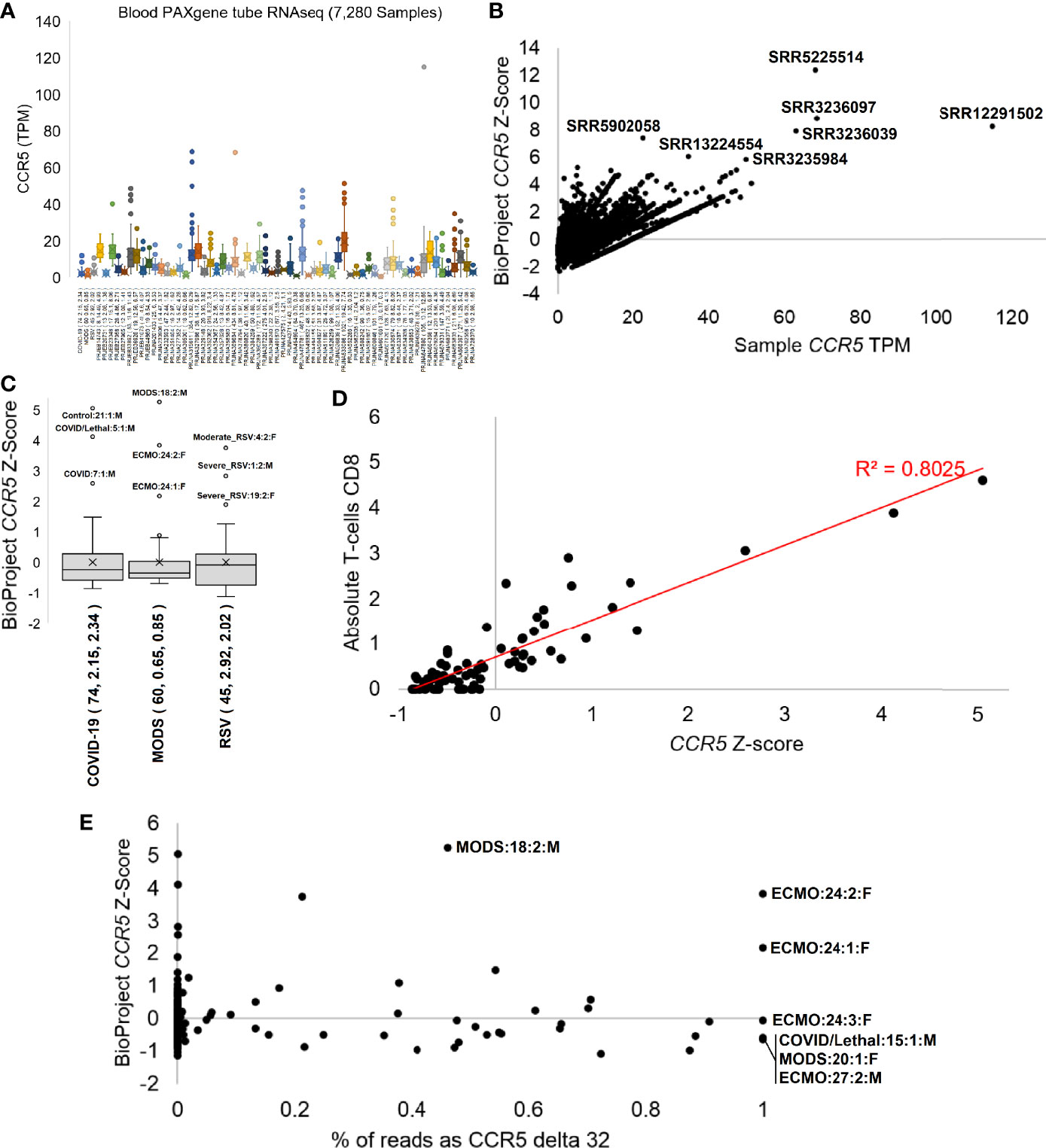
Figure 7 CCR5 expression in blood PAXgene tube RNAseq. (A) Box and whisker plots for the expression (TPM) of CCR5 from various NCBI BioProjects that were generated by Illumina paired-end RNA-Seq from human blood collected PAXgene tube samples. Listed next to each BioProject code is the number of samples, average CCR5 expression, and standard deviation of CCR5 expression. (B) The TPM expression for each sample (x-axis) of panel (A) relative to the BioProject normalized Z-score (y-axis). The top seven samples based on Z-score are labeled. (C) The Z-scores for our three pilot precision transcriptome datasets, with outlier samples labeled. (D) The COVID-19 study analysis of CCR5 normalized expression relative to CIBERSORTx absolute CD8 T-cell values. (E) The percent of transcripts containing the delta 32 variant relative to wild type (x-axis) for samples of panel (C) relative to the BioProject normalized Z-Score. All homozygous samples for delta 32 are labeled as is the heterozygous sample with the highest study Z-score.
Therefore, we focused analysis on three cohorts our team collected blood PAXgene tube RNAseq in hospitalized patients with MODS, RSV, or COVID-19 in an age range from weeks of life to elderly. The adult hospitalized COVID-19 cohort shows highest CCR5 expression in the male Hispanic control patient 21 age 50-59 (Figure 7C), who had the highest interferon response, was noted to have a unique transcriptome, was the furthest outlier of the control samples, had markers of multiple organ damage, and generally seemed to be a highly divergent sample from the cohort (74). The second highest was a 50-59 yo European ancestry male who had a lethal case of COVID-19 marked by a SAPSII score of 61, suggesting multiple organ complications, had a robust interferon response, elevated cytokine expression profile, the highest cell markers of peripheral monocytes, and a weak clonal expansion of the immune repertoire. The third outlier of the study was a 40-49 yo European ancestry male with a SAPSII score of 30, a high activation of mitotic cell cycle control genes, an elevation of interleukin-7 and histone genes, and detectable reads in the blood related to Paraburkholderia and Streptomyces tsukubensis. None of the samples were identified as outliers of CCR5 expression on the low end, but samples with CCR5 expression <0.8 standard deviations were all noted in COVID-19 hospitalized patients and not controls. The depth of sequencing for this COVID-19 study allowed for various gene panels, immune repertoire, and foreign RNA mapping for all samples, which were correlated to the CCR5 values. The CIBERSORTx absolute values for CD8 T-cells were highly correlated (R2 = 0.8) to CCR5 expression (Figure 7D).
Further evaluation of our pediatric MODS and infant RSV cohorts (Figure 7C) reveals diseased samples as outliers of CCR5 expression. Sample 24, female 16 yo with European ancestry, within the MODS study had two separate measurements with high CCR5 and an Epstein Barr virus (EBV) infection that required ECMO and was also identified as having the highest levels of CIBERSORTx annotated CD8 T-cells (75). Sample 18, a male who required ventilation, had the highest levels of CCR5 within the MODS cohort and was noted to have elevated genes for interferon response, had a clinical Serratia marcescens infection, and was coronavirus positive. The lowest CCR5 levels in the MODS cohort were observed in sepsis patients, one of which (patient 27) was lethal. The RSV study’s top three outliers for CCR5 expression were all hospitalized RSV cases (patient 1, 4, 19) (76). Patient 1 had high levels of Agromyces aureus and Caulobacter vibrioides like reads in the blood in addition to clinically confirmed RSV, while also having multiple levels of elevated RNA associated with lung hyperinflammation. Patient 4 had elevated reads belonging to type-1 Alveolar cell and an elevation of viral defense response genes relative to the cohort. All three samples with a z-score below -1 were from hospitalized RSV samples. These three cohorts suggest that CCR5 levels, either high or low, often are found in sick patients and rarely seen in healthy controls within our cohorts.
To probe whether RNAseq samples contained the CCR5 delta32 variant, we developed a novel Salmon-based indexing file containing the wild-type CCR5 transcripts, the delta32 transcripts, and several of the top human paralog transcripts. Mapping samples with delta32 reads over our 179 in-house RNAseq samples reveals 75.4% of the samples to be homozygous wild type (<0.01% delta32 reads), 21.2% heterozygous, and 3.4% homozygous for delta32 (Figure 7E). The heterozygous samples were highly variable for the % of reads with delta32 and included the MODS sample 18, with the highest overall CCR5 z-score of the three studies. Surprisingly, all three samples of the ECMO patient 24 showed homozygous CCR5 delta32, where an additional two-year follow-up of the patient also showed homozygous CCR5 (data not shown as the z-score cannot be calculated as it was a single sample RNAseq). This patient was the focus of our 2020 MODS paper (75), where we discovered the 16 yo patient to have Hemophagocytic lymphohistiocytosis (HLH) likely driven by a dominant-negative splicing variant in RNASEH2B that is activated by the EBV suppression of nonsense-mediated decay. As this patient is an N=1 case, it is interesting to note the severe, nearly lethal phenotype of this patient and to be CCR5 delta 32 homozygous, suggesting that CCR5 complete inhibition does not remove HLH risks as others have proposed for COVID-19 (91). It is also interesting that as the EBV infection cleared in the patient, the CCR5 levels, even when delta32 homozygous, decreased in sample 3. The other three samples where we observed CCR5 homozygous delta32 were in severe outcomes, including patient 15 who had lethal COVID-19, the MODS patient 20 who had Alveolar rhabdomyosarcoma and human betaherpesvirus 7, and the MODS patient 27 who required ECMO and passed away. Our cohort of 98 unique individuals from these three studies had only 13 lethal cases, of which two were delta32 positive (15%). This trend demands further evaluation of delta32 status in sepsis and critical care patients.
Discussion
Creating modification in genomic backgrounds that are not commonly present with the variant yields unknown risks that need to be assessed before moving to human editing, particularly in utero. The delta32 variant is unique to subpopulations of humans, and there could be unknown variants co-evolved in these populations that modulate the deleterious effects of the delta32 variant. As CCR5 is found within a region of the genome containing multiple cytokine receptor paralogs and has a large and complex linkage disequilibrium block and eQTLs often overlap the different paralogs, genetic variants can be in linkage disequilibrium to compensate for deleterious outcomes. In other pathologies, these shared variants that regulate counter genes have been shown for cardiovascular biology and present on the Y-chromosome (92, 93). Viewed in the context of evolution by natural selection, selection on one trait has correlated effects and is often constrained if those correlated effects are themselves deleterious (94, 95). Editing genomes is independent of evolutionary selection over time and could result in unintended medical consequences due to genomic backgrounds used in human editing. Bioinformatic studies of susceptibility loci suggest that pleiotropy, the existence of multiple functions for a single gene, far from being the exception, appears to be the rule, not only in the case of CCR5 but also in the case of susceptibility loci for breast cancer, lung cancer, coronary artery disease and other severe diseases (96). Thus, understanding the effects of CCR5 removal in diverse genome backgrounds is critical before introducing the variants in diverse human backgrounds. These studies are very complex, requiring extensive data, experimentation, and a great deal of financial resources and time.
An additional set of ethical, legal, and social implications has been brought to light by the He Jiankui affair, in which CRISPR-Cas9 gene editing was used in an attempt to edit the CCR5 gene of two embryos to the Δ32 variant with the hope of conferring immunity to HIV. The germ line was crossed, and twin baby girls, Nana and Lulu, were born. This universally condemned ethical breach leaped over several ethical safeguards. Before translating germline gene-editing to the fertility clinic, it will be necessary to carefully assess the ethical and legal conditions for its permissibility and move toward finer line-drawing in determining these conditions (97). For example, there is a significant biological and ethical difference between a “correction” of a rare, disease-associated mutation to a widespread non-pathogenic allele as is being investigated for HBB and MYBPC3 in the case of β-thalassemia and hypertrophic cardiomyopathy respectively, and attempted edits to CCR5 with its complex, multiple phenotypic effects and potentially pathogenic off-target effects (97, 98). Germline gene-editing carries with it its own set of ethical issues, most notably extreme uncertainty about the effects on the edited individual, as the effects of edits—whether those intended, errors, or off-target—to the genome early in life ramify unpredictably throughout embryogenesis and later development, as well as concerns about long-term intergenerational effects that are difficult to study and whose risks cannot be easily assessed.
Genetic and genomic reductionism takes many forms, and the terms are variously used in the philosophy of biology (99). The particular form of genetic reductionism under criticism here is the mistaken belief that a given gene stands in a strict one-to-one relationship to a given character state, whereas a one-to-many relationship is the rule (99). With rare exceptions, causal pathways in biology are complex. Yet, in the case of genomics, there is good reason to believe that a reductionistic tendency has been inherited from a long-standing biomedical model (100). Reductionism has played an especially important role in the history of understanding pathogenicity, with the locus classicus being Koch’s postulates, whereby it can be experimentally demonstrated that a single species of microorganism is the cause of a particular disease state (100). Based on the prevalence of “gene-for-X” publications in the genomics literature, the reductionistic biomedical model that underlay Koch’s postulate throughout the expansion of the paradigm of the germ theory of disease appears to have been quite widely adopted in genomics research, despite acknowledgment of the complexity and context-dependence of gene expression, pleiotropy, and other effects that belie reductionism (96, 100). Genetic reductionism is best understood as a research strategy from which much is learned in its failures, which point to additional elements of the overall biological context necessary for fuller understanding (101, 102). In other words, methodological reductionism should be understood as a heuristic device for uncovering ontological complexity (101).
An explicit ethical framework, modeled on informed consent, is necessary to undergird a careful, thoughtful approach to assessing and considering the ethical, legal, and social implications of genomic research in which CODE can play a crucial role. In the same way that it has been urged that students of biology need a thorough understanding of the tools and principles of ethics (103), the public, as stakeholders in assessing the ethical, legal, and social implications of genomic research and genomic medicine, need an understanding of the complexities of molecular biology to the extent that this can be achieved. The importance of providing tools for a broad range of educational encounters to build a more complex and realistic understanding of genomics is necessary if the public is to be consulted on research oversight, policy, and clinical application. As research proceeds in the study of CCR5 and other potential intervention targets for germline gene editing, a complex conversation is unfolding in which scientists, when consulted on the need for ethical boundary policing and a cautious approach to moving from basic to translational research to potential application, are pointing to the need to include the public in deliberations over where to draw the line in gene therapy more broadly and more specifically in interventions and genomic edits that cross the germ line (104). An analogy can be drawn with informed consent, a pillar of biomedical ethics, whereby if the public’s voice is to play a role in providing ethical guidance for genomics research and genomic medicine, it is necessary for the public to be informed. CODE can serve as a platform for providing the sorts of educational tools to be deployed in various settings to improve understanding of the science of genomics.
Complicating research and public understanding, assessing the quality of large public databases has been challenging. During the middle of 2019, a Nature Medicine study brought to light the possibility that delta32 decreases life expectancies, yet the results were retracted due to errors in genotyping. This retraction has had consequences of potential public misinformation, suggesting that delta32 has no negative impact, even though the study only addressed a few associations, and public stories rarely discuss the known risks of delta32 (27), something to which our blood RNA-seq also lends support. The removal of CCR5 is not the only possible solution to developing HIV resistance, with the FDA fast-track work on compounds like Leronlimab to antagonize CCR5 binding by HIV. If these compounds are successful, then genomic modification may be unnecessary, with medications offering the safer approach for the patient. More importantly, all potential treatments need to be considered in the light of all available data, including their role in modulating neurodevelopment and sepsis outcomes. These discussions have diverged from the central question of what CCR5 does within cells, why it is important, and the severity of variants like delta32, which removes multiple transmembrane helices and thus prevents the correct proteins production and cellular localization. Further discussions are critically needed into potential gain-of-function roles of misfolded CCR5 delta32 (105) and our interesting observation of the broad distribution of blood CCR5 delta32 RNA-based allele frequencies in heterozygous individuals and accumulation of RNA in one individual that was homozygous (Figure 7E). Thus, the development of educational material for projects like CCR5 is critical for a more robust view of the protein, its variants, and its diverse physiological functions outside of what is emphasized in popular media.
Further complicating the role of CCR5 in human biology is the interplay of cytokine biology with CCL3, CCL4, and CCL5 (primary ligands for CCR5). The role of these chemokines in biology and disease have been well studied, and they are known to play a role in asthma, viral infections, dengue fever, acute kidney disease, and multiple sclerosis, with the oscillation of their role between benefit and harm depending on circumstance and insult (106–110). Although seen as inflammatory mediators, these chemokines are induced by inflammatory cytokine in multiple models, including neural inflammation. Typically treated as a site of immune privilege, resident macrophages, or glial cells within the brain, play an integral role in formulating a proper inflammatory response in a state of infection or injury. Microglia have been shown to secrete the inflammatory cytokines IL-1β and TNF-α, resulting in increased secretion of CCL3 and CCL4 (111), further supporting the role of CCR5 in microglia function and feedback.
Similarly, treatment of human brain endothelial cells with IL-1β, TNF-α, and IFN-γ resulted in a significant increase in the secretion of CCL3, CCL4, and CCL5, likely resulting in an increased capacity for leukocyte extravasation through integrin activation (110, 112). The interplay of cytokines in this paradigm is not monodirectional. CCL5 can skew the host’s CD4 T cell response to pathogen from a TH2 phenotype to a TH1 phenotype, altering cytokine secretion. This modulation results in the release of proinflammatory cytokines such as TNF-α and IFN-γ, further potentiating the skewing (108). The biological complexity of CCR5, its ligands, and the interplay of the immune response as a whole demonstrates the need for a more nuanced discussion of our understanding of the available data.
Microglia, the resident macrophages of the central nervous system, express CCR5 as discussed. Microglia contribute to the blood-brain barrier (BBB) accompanied by a meshwork of endothelial cells, astrocytes, pericytes, and a basement membrane (113). In early development, microglia contribute to the pruning of neurons, with dysregulation of this process known to contribute to autism spectrum disorders (114). The ligands for CCR5 (CCL3, CCL4, and CCL5) display expression profiles from astrocytes, endothelial cells, microglia, and within specific subpopulations of neurons (112, 115). CCR5 expression and activation by its respective ligands promote CCR5+ leukocyte adhesion and transmigration through the blood-brain barrier (116). In response to CNS insults, CCR5 is upregulated, prompting an inflammatory response (117). In stroke, CCR5 knock-out is established to increase the severity of brain injury (118). However, this function of CCR5 in pathological states is either beneficial or detrimental depending on the inciting event. Studies with infectious agents such as Toxoplasma gondii (119), Herpes Simplex Virus type 1 (120), and Herpes simplex virus type 2 (121) have shown that knocking out CCR5 contributes to enhanced infection and increased disease severity, likely due to blunting of the immune response.
On the contrary, malarial infection with cerebral involvement in CCR5 knock-out mice resulted in neuroprotection by decreasing lymphocyte migration and destruction of the CNS (122). Much like our blood CCR5 expression analysis, this data suggests a balance of infection to outcomes based on CCR5 in the brain. Despite the prominent immunological role of CCR5, both peripherally and centrally, downregulation of CCR5 does not reduce immune microglial transmigration in the CNS during pathological insults (123), indicating that there are other alternative pathways able to compensate for a dampened immune response. Constitutive basal levels of CCR5 expression within the CNS in the absence of pathology indicate ancillary roles for this chemokine receptor outside of the known immune system component-with studies supporting its involvement in neural development and physiological functioning.
The complex role CCR5 plays in the central nervous system beyond the immune system has not been fully fleshed out; however, it has been demonstrated to play a role in fetal CNS development, neuronal differentiation, and neuronal survival. In a study by Westmoreland et al., it was found that CCR5 was expressed by primate fetal cells with increasing expression from birth to 9 months of age (124). Further studies have shown that CCR5 activation results in neuronal differentiation and neuronal survival during apoptotic states (125, 126). The expression of chemokine receptors, such as CCR5, plays a role in neural progenitor cell migration and neuronal connections (127). Collectively, it appears that CCR5 plays a role in neural cell migration, differentiation, and survival during the postnatal period of CNS development, correlating to the timing of microglial pruning. This conclusion is also supported by the fact that many CNS tumors upregulate CCR5 during their rapid growth, including glioblastoma multiforme (128) and primary CNS lymphoma (129). Further insight into CCR5’s role in the CNS is needed to determine the specific mechanisms at which it is involved outside of the known immune functions.
CODE projects lower the barriers to engaging in bioinformatics research by placing genomic analysis within the grasp of a broad and diverse audience. A typical project path mirrors the approach taken by clinical research analysts, beginning with database research, followed by modeling and simulations, and culminating in data analysis. Students first identify a genetic variant of interest identified through genomic research at HudsonAlpha, Michigan State University, or from a publicly available genome database like ClinVar (human). Students use publicly available databases and relevant software to learn more about the gene containing the variant and its functional product. Students compare the genomic sequence with similar segments from other organisms to study the evolutionary conservation of both DNA and protein. This information helps in making predictions regarding the functional consequence of the DNA variant.
Students next employ molecular modeling to study how variants may alter the protein. This type of molecular visualization provides essential support for reasoning on and formulating hypotheses related to molecular structure. Current software tools such as YASARA, PyMOL, and UCSF Chimera allow students to build a 3-D model of a protein, insert a variant, and visualize whether the variant changes protein folding and structure. Students can then study the DNA variant in a computationally-derived cellular environment, running simulations to predict how the variant-containing protein might behave inside a cell. Much like this study, that molecular level knowledge can be combined with analysis of multiple expression databases to allow the students to identify the cell types and tissues where the variants might alter function, known and genotype-to-phenotype insights. This type of analysis can facilitate understanding of the variant’s effect and even provide insight into interventions to offset potentially damaging impacts. Students document their work and findings of their genetic variant in written reports, poster presentations, and research talks at their schools and scientific conferences and contribute to published research in relevant journals. As a byproduct, the students often generate educational resources such as 3D models, videos, and worksheets that can inform clinical analysis and broader understanding of genetic variants, such as shown here for CCR5.
This report on the impact of studies fueled by academic resources shed light on the need for and potential impact of applying education resources to cases in genomics. In the growing era of precision medicine, the need for tools to define the effects of variants (90) and propagate the information to patients and the public has grown, especially to combat genomic reductionism. CODE provides undergraduate students at diverse institutions with an improved understanding of genetics and developing educational material. As knowledge continues to grow for CCR5, several of the CODE students can continue engaging in molecular-level research, such as simulating the recently solved CCR5 ligand-bound structures (130) and integrating the knowledge into our tools. We have expanded these tools to clinical variants from the HudsonAlpha genomic sequencing projects to help explain complex Variants of Uncertain Significance (VUS), including MED13 (131) and RALA (132) that can be found at prokoplab.com/educational-resources/. At the outbreak of the SARS-CoV-2 pandemic, we also used this same workflow to establish critical and rapid insights on the viral encoded proteins and their interactions with host proteins utilizing CODE as a distributed research network to gain faster insights of proteins (133, 134). Expanding these tools to additional projects and collaboration will open a door for educational information in the needed area of genomics and genomic medicine.
Data Availability Statement
The datasets presented in this study can be found in online repositories. The names of the repository/repositories and accession number(s) can be found in the article/supplementary material.
Author Contributions
JB, RS, RO, JM, AE, CS, SC, and JP contributed to the generation of CCR5 amino acid knowledgebase or expression analysis. MM, LB, CLS, DH, and JP contributed to the generation of CCR5 educational material. JB, RS, RO, JM, AE, JZ, NH, BC, SR, CB, and JP contributed to the analysis and interpretation of 3 house RNAseq studies. JH contributed philosophical oversight of genomic reductionism. MM, CLS, SC, BC, SR, CB, and JP supervised the completion of all work. JB, MM, JZ, and JP wrote the manuscript. All authors contributed to the article and approved the submitted version.
Funding
This work was supported by the Spectrum Health and MSU Alliance Corporation, Spectrum Health Foundation, National Institutes of Health (K01ES025435 to JP), Gerber Foundation, and Michigan State University.
Conflict of Interest
The authors declare that the research was conducted in the absence of any commercial or financial relationships that could be construed as a potential conflict of interest.
Publisher’s Note
All claims expressed in this article are solely those of the authors and do not necessarily represent those of their affiliated organizations, or those of the publisher, the editors and the reviewers. Any product that may be evaluated in this article, or claim that may be made by its manufacturer, is not guaranteed or endorsed by the publisher.
References
1. Campion M, Goldgar C, Hopkin RJ, Prows CA, Dasgupta S. Genomic Education for the Next Generation of Health-Care Providers. Genet Med (2019) 21:2422–30. doi: 10.1038/s41436-019-0548-4
2. Lea DH, Kaphingst KA, Bowen D, Lipkus I, Hadley DW. Communicating Genetic and Genomic Information: Health Literacy and Numeracy Considerations. PHG (2011) 14:279–89. doi: 10.1159/000294191
3. Chapman R, Likhanov M, Selita F, Zakharov I, Smith-Woolley E, Kovas Y. New Literacy Challenge for the Twenty-First Century: Genetic Knowledge Is Poor Even Among Well Educated. J Community Genet (2019) 10:73–84. doi: 10.1007/s12687-018-0363-7
4. Dougherty MJ, Pleasants C, Solow L, Wong A, Zhang H. A Comprehensive Analysis of High School Genetics Standards: Are States Keeping Pace With Modern Genetics? CBE Life Sci Educ (2011) 10:318–27. doi: 10.1187/cbe.10-09-0122
5. Wilcox RL, Adem PV, Afshinnekoo E, Atkinson JB, Burke LW, Cheung H, et al. The Undergraduate Training in Genomics (UTRIG) Initiative: Early & Active Training for Physicians in the Genomic Medicine Era. Per Med (2018) 15:199–208. doi: 10.2217/pme-2017-0077
6. Hyland K, Dasgupta S. Medical Genetics and Genomics Education and its Impact on Genomic Literacy of the Clinical Workforce. Genet Med (2019) 21:1259–60. doi: 10.1038/gim.2017.127
7. Anderson G, Alt-White AC, Schaa KL, Boyd AM, Kasper CE. Genomics for Nursing Education and Practice: Measuring Competency. Worldviews Evid Based Nurs (2015) 12:165–75. doi: 10.1111/wvn.12096
8. Camak DJ. Increasing Importance of Genetics in Nursing. Nurse Educ Today (2016) 44:86–91. doi: 10.1016/j.nedt.2016.05.018
9. Christensen KD, Vassy JL, Jamal L, Lehmann LS, Slashinski MJ, Perry DL, et al. Are Physicians Prepared for Whole Genome Sequencing? A Qualitative Analysis. Clin Genet (2016) 89:228–34. doi: 10.1111/cge.12626
10. Dougherty MJ, Lontok KS, Donigan K, McInerney JD. The Critical Challenge of Educating the Public About Genetics. Curr Genet Med Rep (2014) 2:48–55. doi: 10.1007/s40142-014-0037-7
11. Koehly LM, Persky S, Spotts E, Acca G. Social and Behavioral Science Priorities for Genomic Translation. Transl Behav Med (2018) 8:137–43. doi: 10.1093/tbm/ibx052
12. Stern F, Kampourakis K. Teaching for Genetics Literacy in the Post-Genomic Era. Stud Sci Educ (2017) 53:193–225. doi: 10.1080/03057267.2017.1392731
13. Donovan BM, Weindling M, Salazar B, Duncan A, Stuhlsatz M, Keck P. Genomics Literacy Matters: Supporting the Development of Genomics Literacy Through Genetics Education Could Reduce the Prevalence of Genetic Essentialism. J Res Sci Teach (2021) 58:520–50. doi: 10.1002/tea.21670
14. Gericke N, Carver R, Castéra J, Evangelista NAM, Marre CC, El-Hani CN. Exploring Relationships Among Belief in Genetic Determinism, Genetics Knowledge, and Social Factors. Sci Educ (2017) 26:1223–59. doi: 10.1007/s11191-017-9950-y
15. Tornabene RE, Sbeglia GC, Nehm RH. Measuring Belief in Genetic Determinism: A Psychometric Evaluation of the PUGGS Instrument. Sci Educ (2020) 29:1621–57. doi: 10.1007/s11191-020-00146-2
16. Uebergang E, Best S, de Silva MG, Finlay K. Understanding Genomic Health Information: How to Meet the Needs of the Culturally and Linguistically Diverse Community-a Mixed Methods Study. J Community Genet (2021) 12:549–57. doi: 10.1007/s12687-021-00537-0
17. Strohman RC. Genetic Determinism as a Failing Paradigm in Biology and Medicine. J Soc Work Educ (2003) 39:169–91. doi: 10.1080/10437797.2003.10779130
18. Newman DL, Coakley A, Link A, Mills K, Wright LK. Punnett Squares or Protein Production? The Expert-Novice Divide for Conceptions of Genes and Gene Expression. CBE Life Sci Educ (2021) 20:ar53. doi: 10.1187/cbe.21-01-0004
19. Liu R, Paxton WA, Choe S, Ceradini D, Martin SR, Horuk R, et al. Homozygous Defect in HIV-1 Coreceptor Accounts for Resistance of Some Multiply-Exposed Individuals to HIV-1 Infection. Cell (1996) 86:367–77. doi: 10.1016/s0092-8674(00)80110-5
20. Samson M, Libert F, Doranz BJ, Rucker J, Liesnard C, Farber CM, et al. Resistance to HIV-1 Infection in Caucasian Individuals Bearing Mutant Alleles of the CCR-5 Chemokine Receptor Gene. Nature (1996) 382:722–5. doi: 10.1038/382722a0
21. Hütter G, Nowak D, Mossner M, Ganepola S, Müssig A, Allers K, et al. Long-Term Control of HIV by CCR5 Delta32/Delta32 Stem-Cell Transplantation. N Engl J Med (2009) 360:692–8. doi: 10.1056/NEJMoa0802905
22. Huang Y, Paxton WA, Wolinsky SM, Neumann AU, Zhang L, He T, et al. The Role of a Mutant CCR5 Allele in HIV-1 Transmission and Disease Progression. Nat Med (1996) 2:1240–3. doi: 10.1038/nm1196-1240
23. He J, Chen Y, Farzan M, Choe H, Ohagen A, Gartner S, et al. CCR3 and CCR5 are Co-Receptors for HIV-1 Infection of Microglia. Nature (1997) 385:645–9. doi: 10.1038/385645a0
24. Kang H, Minder P, Park MA, Mesquitta W-T, Torbett BE, Slukvin II. CCR5 Disruption in Induced Pluripotent Stem Cells Using CRISPR/Cas9 Provides Selective Resistance of Immune Cells to CCR5-Tropic HIV-1 Virus. Mol Ther Nucleic Acids (2015) 4:e268. doi: 10.1038/mtna.2015.42
25. Xu L, Yang H, Gao Y, Chen Z, Xie L, Liu Y, et al. CRISPR/Cas9-Mediated CCR5 Ablation in Human Hematopoietic Stem/Progenitor Cells Confers HIV-1 Resistance In Vivo. Mol Ther (2017) 25:1782–9. doi: 10.1016/j.ymthe.2017.04.027
26. Cradick TJ, Fine EJ, Antico CJ, Bao G. CRISPR/Cas9 Systems Targeting β-Globin and CCR5 Genes Have Substantial Off-Target Activity. Nucleic Acids Res (2013) 41:9584–92. doi: 10.1093/nar/gkt714
27. Oppermann M. Chemokine Receptor CCR5: Insights Into Structure, Function, and Regulation. Cell Signal (2004) 16:1201–10. doi: 10.1016/j.cellsig.2004.04.007
28. Xu L, Wang J, Liu Y, Xie L, Su B, Mou D, et al. CRISPR-Edited Stem Cells in a Patient With HIV and Acute Lymphocytic Leukemia. N Engl J Med (2019) 381:1240–7. doi: 10.1056/NEJMoa1817426
29. Uhlen M, Oksvold P, Fagerberg L, Lundberg E, Jonasson K, Forsberg M, et al. Towards a Knowledge-Based Human Protein Atlas. Nat Biotechnol (2010) 28:1248–50. doi: 10.1038/nbt1210-1248
30. Loetscher P, Uguccioni M, Bordoli L, Baggiolini M, Moser B, Chizzolini C, et al. CCR5 is Characteristic of Th1 Lymphocytes. Nature (1998) 391:344–5. doi: 10.1038/34814
31. Ferguson AR, Engelhard VH. CD8 T Cells Activated in Distinct Lymphoid Organs Differentially Express Adhesion Proteins and Coexpress Multiple Chemokine Receptors. J Immunol (2010) 184:4079–86. doi: 10.4049/jimmunol.0901903
32. Watanabe S, Yamada Y, Murakami H. Expression of Th1/Th2 Cell-Related Chemokine Receptors on CD4+ Lymphocytes Under Physiological Conditions. Int J Lab Hematol (2020) 42:68–76. doi: 10.1111/ijlh.13141
33. Ellwanger JH, Kaminski V de L, Chies JAB. CCR5 Gene Editing - Revisiting Pros and Cons of CCR5 Absence. Infect Genet Evol (2019) 68:218–20. doi: 10.1016/j.meegid.2018.12.027
34. Vargas AE, Cechim G, Correa JF, Gomes PA, Macedo G de S, de Medeiros RM, et al. Pros and Cons of a Missing Chemokine Receptor–Comments on “Is the European Spatial Distribution of the HIV-1-Resistant CCR5-D32 Allele Formed by a Breakdown of the Pathocenosis Due to the Historical Roman Expansion?” by Eric Faure and Manuela Royer-Carenzi (2008). Infect Genet Evol (2009) 9:387–9. doi: 10.1016/j.meegid.2009.01.001
35. Maung R, Hoefer MM, Sanchez AB, Sejbuk NE, Medders KE, Desai MK, et al. CCR5 Knockout Prevents Neuronal Injury and Behavioral Impairment Induced in a Transgenic Mouse Model by a CXCR4-Using HIV-1 Glycoprotein 120. J Immunol (2014) 193:1895–910. doi: 10.4049/jimmunol.1302915
36. Gu SM, Park MH, Yun HM, Han SB, Oh KW, Son DJ, et al. CCR5 Knockout Suppresses Experimental Autoimmune Encephalomyelitis in C57BL/6 Mice. Oncotarget (2016) 7:15382–93. doi: 10.18632/oncotarget.8097
37. Choi D-Y, Ban J-O, Kim S-C, Hong JT. CCR5 Knockout Mice With C57BL6 Background are Resistant to Acetaminophen-Mediated Hepatotoxicity Due to Decreased Macrophages Migration Into the Liver. Arch Toxicol (2015) 89:211–20. doi: 10.1007/s00204-014-1253-3
38. Hwang CJ, Park MH, Hwang JY, Kim JH, Yun NY, Oh SY, et al. CCR5 Deficiency Accelerates Lipopolysaccharide-Induced Astrogliosis, Amyloid-Beta Deposit and Impaired Memory Function. Oncotarget (2016) 7:11984–99. doi: 10.18632/oncotarget.7453
39. Lee YK, Kwak DH, Oh KW, Nam S-Y, Lee BJ, Yun YW, et al. CCR5 Deficiency Induces Astrocyte Activation, Abeta Deposit and Impaired Memory Function. Neurobiol Learn Mem (2009) 92:356–63. doi: 10.1016/j.nlm.2009.04.003
40. Carr DJJ, Ash J, Lane TE, Kuziel WA. Abnormal Immune Response of CCR5-Deficient Mice to Ocular Infection With Herpes Simplex Virus Type 1. J Gen Virol (2006) 87:489–99. doi: 10.1099/vir.0.81339-0
41. Barr EL, Ouburg S, Igietseme JU, Morré SA, Okwandu E, Eko FO, et al. Host Inflammatory Response and Development of Complications of Chlamydia Trachomatis Genital Infection in CCR5-Deficient Mice and Subfertile Women With the CCR5delta32 Gene Deletion. J Microbiol Immunol Infect (2005) 38:244–54.
42. Turner JE, Steinmetz OM, Stahl RA, Panzer U. Targeting of Th1-Associated Chemokine Receptors CXCR3 and CCR5 as Therapeutic Strategy for Inflammatory Diseases. Mini Rev Med Chem (2007) 7:1089–96. doi: 10.2174/138955707782331768
43. Ajuebor MN, Carey JA, Swain MG. CCR5 in T Cell-Mediated Liver Diseases: What’s Going on? J Immunol (2006) 177:2039–45. doi: 10.4049/jimmunol.177.4.2039
44. Gong X, Feng H, Zhang S, Yu Y, Li J, Wang J, et al. Increased Expression of CCR5 in Experimental Autoimmune Myocarditis and Reduced Severity Induced by Anti-CCR5 Monoclonal Antibody. J Mol Cell Cardiol (2007) 42:781–91. doi: 10.1016/j.yjmcc.2007.02.003
45. Combadiere C, Ahuja SK, Tiffany HL, Murphy PM. Cloning and Functional Expression of CC CKR5, a Human Monocyte CC Chemokine Receptor Selective for MIP-1(Alpha), MIP-1(Beta), and RANTES. J Leukoc Biol (1996) 60:147–52. doi: 10.1002/jlb.60.1.147
46. Ridley AJ, Schwartz MA, Burridge K, Firtel RA, Ginsberg MH, Borisy G, et al. Cell Migration: Integrating Signals From Front to Back. Science (2003) 302:1704–9. doi: 10.1126/science.1092053
47. Tanaka Y, Adams DH, Hubscher S, Hirano H, Siebenlist U. Shaw S. T-Cell Adhesion Induced by Proteoglycan-Immobilized Cytokine MIP-1 Beta. Nature (1993) 361:79–82. doi: 10.1038/361079a0
48. Menten P, Wuyts A, Van Damme J. Macrophage Inflammatory Protein-1. Cytokine Growth Factor Rev (2002) 13:455–81. doi: 10.1016/s1359-6101(02)00045-x
49. Amanzada A, Moriconi F, Mansuroglu T, Cameron S, Ramadori G, Malik IA. Induction of Chemokines and Cytokines Before Neutrophils and Macrophage Recruitment in Different Regions of Rat Liver After TAA Administration. Lab Invest (2014) 94:235–47. doi: 10.1038/labinvest.2013.134
50. Feng L, Xia Y, Yoshimura T, Wilson CB. Modulation of Neutrophil Influx in Glomerulonephritis in the Rat With Anti-Macrophage Inflammatory Protein-2 (MIP-2) Antibody. J Clin Invest (1995) 95:1009–17. doi: 10.1172/JCI117745
51. da Silva JM, Moreira Dos Santos TP, Sobral LM, Queiroz-Junior CM, Rachid MA, Proudfoot AEI, et al. Relevance of CCL3/CCR5 Axis in Oral Carcinogenesis. Oncotarget (2017) 8:51024–36. doi: 10.18632/oncotarget.16882
52. Aldinucci D, Colombatti A. The Inflammatory Chemokine CCL5 and Cancer Progression. Mediators Inflamm (2014) 2014:292376. doi: 10.1155/2014/292376
53. Altschul SF, Gish W, Miller W, Myers EW, Lipman DJ. Basic Local Alignment Search Tool. J Mol Biol (1990) 215:403–10. doi: 10.1016/S0022-2836(05)80360-2
54. Thompson JD, Higgins DG, Gibson TJ. CLUSTAL W: Improving the Sensitivity of Progressive Multiple Sequence Alignment Through Sequence Weighting, Position-Specific Gap Penalties and Weight Matrix Choice. Nucleic Acids Res (1994) 22:4673–80. doi: 10.1093/nar/22.22.4673
55. Tamura K, Peterson D, Peterson N, Stecher G, Nei M, Kumar S. MEGA5: Molecular Evolutionary Genetics Analysis Using Maximum Likelihood, Evolutionary Distance, and Maximum Parsimony Methods. Mol Biol Evol (2011) 28:2731–9. doi: 10.1093/molbev/msr121
56. Haas BJ, Papanicolaou A, Yassour M, Grabherr M, Blood PD, Bowden J, et al. De Novo Transcript Sequence Reconstruction From RNA-Seq Using the Trinity Platform for Reference Generation and Analysis. Nat Protoc (2013) 8:1494–512. doi: 10.1038/nprot.2013.084
57. Prokop JW, Yeo NC, Ottmann C, Chhetri SB, Florus KL, Ross EJ, et al. Characterization of Coding/Noncoding Variants Forshroom3in Patients With CKD. J Am Soc Nephrol (2018) 29(5):1525–35. doi: 10.1681/ASN.2017080856
58. Prokop JW, Lazar J, Crapitto G, Smith DC, Worthey EA, Jacob HJ. Molecular Modeling in the Age of Clinical Genomics, the Enterprise of the Next Generation. J Mol Model (2017) 23:75. doi: 10.1007/s00894-017-3258-3
59. Apweiler R, Bairoch A, Wu CH, Barker WC, Boeckmann B, Ferro S, et al. UniProt: The Universal Protein Knowledgebase. Nucleic Acids Res (2004) 32:D115–9. doi: 10.1093/nar/gkh131
60. Krieger E, Joo K, Lee J, Lee J, Raman S, Thompson J, et al. Improving Physical Realism, Stereochemistry, and Side-Chain Accuracy in Homology Modeling: Four Approaches That Performed Well in CASP8. Proteins (2009) 77(Suppl 9):114–22. doi: 10.1002/prot.22570
61. Case DA, Cheatham TE, Darden T, Gohlke H, Luo R, Merz KM, et al. The Amber Biomolecular Simulation Programs. J Comput Chem (2005) 26:1668–88. doi: 10.1002/jcc.20290
62. Karczewski KJ, Francioli LC, Tiao G, Cummings BB, Alföldi J, Wang Q, et al. The Mutational Constraint Spectrum Quantified From Variation in 141,456 Humans. Nature (2020) 581:434–43. doi: 10.1038/s41586-020-2308-7
63. Forbes SA, Bindal N, Bamford S, Cole C, Kok CY, Beare D, et al. COSMIC: Mining Complete Cancer Genomes in the Catalogue of Somatic Mutations in Cancer. Nucleic Acids Res (2011) 39:D945–950. doi: 10.1093/nar/gkq929
64. Taliun D, Harris DN, Kessler MD, Carlson J, Szpiech ZA, Torres R, et al. Sequencing of 53,831 Diverse Genomes From the NHLBI TOPMed Program. Nature (2021) 590:290–9. doi: 10.1038/s41586-021-03205-y
65. Landrum MJ, Lee JM, Benson M, Brown G, Chao C, Chitipiralla S, et al. ClinVar: Public Archive of Interpretations of Clinically Relevant Variants. Nucleic Acids Res (2016) 44:D862–8. doi: 10.1093/nar/gkv1222
66. Wang J, Al-Ouran R, Hu Y, Kim S-Y, Wan Y-W, Wangler MF, et al. MARRVEL: Integration of Human and Model Organism Genetic Resources to Facilitate Functional Annotation of the Human Genome. Am J Hum Genet (2017) 100:843–53. doi: 10.1016/j.ajhg.2017.04.010
67. Adzhubei IA, Schmidt S, Peshkin L, Ramensky VE, Gerasimova A, Bork P, et al. A Method and Server for Predicting Damaging Missense Mutations. Nat Methods (2010) 7:248–9. doi: 10.1038/nmeth0410-248
68. Choi Y, Chan AP. PROVEAN Web Server: A Tool to Predict the Functional Effect of Amino Acid Substitutions and Indels. Bioinformatics (2015) 31:2745–7. doi: 10.1093/bioinformatics/btv195
69. Ng PC, Henikoff S. SIFT: Predicting Amino Acid Changes That Affect Protein Function. Nucleic Acids Res (2003) 31:3812–4. doi: 10.1093/nar/gkg509
70. Mathe E, Olivier M, Kato S, Ishioka C, Hainaut P, Tavtigian SV. Computational Approaches for Predicting the Biological Effect of P53 Missense Mutations: A Comparison of Three Sequence Analysis Based Methods. Nucleic Acids Res (2006) 34:1317–25. doi: 10.1093/nar/gkj518
71. Kent WJ, Sugnet CW, Furey TS, Roskin KM, Pringle TH, Zahler AM, et al. The Human Genome Browser at UCSC. Genome Res (2002) 12:996–1006. doi: 10.1101/gr.229102
72. GTEx Consortium. The GTEx Consortium Atlas of Genetic Regulatory Effects Across Human Tissues. Science (2020) 369:1318–30. doi: 10.1126/science.aaz1776
73. Ghoussaini M, Mountjoy E, Carmona M, Peat G, Schmidt EM, Hercules A, et al. Open Targets Genetics: Systematic Identification of Trait-Associated Genes Using Large-Scale Genetics and Functional Genomics. Nucleic Acids Res (2021) 49:D1311–20. doi: 10.1093/nar/gkaa840
74. Prokop JW, Hartog NL, Chesla D, Faber W, Love CP, Karam R, et al. High-Density Blood Transcriptomics Reveals Precision Immune Signatures of SARS-CoV-2 Infection in Hospitalized Individuals. Front Immunol (2021) 12:694243. doi: 10.3389/fimmu.2021.694243
75. Prokop JW, Shankar R, Gupta R, Leimanis ML, Nedveck D, Uhl K, et al. Virus-Induced Genetics Revealed by Multidimensional Precision Medicine Transcriptional Workflow Applicable to COVID-19. Physiol Genomics (2020) 52:255–68. doi: 10.1152/physiolgenomics.00045.2020
76. Gupta R, Leimanis ML, Adams M, Bachmann AS, Uhl KL, Bupp CP, et al. Balancing Precision Versus Cohort Transcriptomic Analysis of Acute and Recovery Phase of Viral Bronchiolitis. Am J Physiol Lung Cell Mol Physiol (2021) 320:L1147–57. doi: 10.1152/ajplung.00440.2020
77. Patro R, Duggal G, Love MI, Irizarry RA, Kingsford C. Salmon Provides Fast and Bias-Aware Quantification of Transcript Expression. Nat Methods (2017) 14:417–9. doi: 10.1038/nmeth.4197
78. Frankish A, Diekhans M, Ferreira A-M, Johnson R, Jungreis I, Loveland J, et al. GENCODE Reference Annotation for the Human and Mouse Genomes. Nucleic Acids Res (2019) 47:D766–73. doi: 10.1093/nar/gky955
79. Ellwanger JH, Kaminski V de L, Chies JA. What We Say and What We Mean When We Say Redundancy and Robustness of the Chemokine System - How CCR5 Challenges These Concepts. Immunol Cell Biol (2020) 98:22–7. doi: 10.1111/imcb.12291
80. COVID-19 Host Genetics Initiative. Mapping the Human Genetic Architecture of COVID-19. Nature (2021). doi: 10.1038/s41586-021-03767-x
81. Franzén O, Gan L-M, Björkegren JLM. PanglaoDB: A Web Server for Exploration of Mouse and Human Single-Cell RNA Sequencing Data. Database (Oxford) (2019) 2019:baz046. doi: 10.1093/database/baz046
82. Passos GF, Figueiredo CP, Prediger RDS, Pandolfo P, Duarte FS, Medeiros R, et al. Role of the Macrophage Inflammatory Protein-1alpha/CC Chemokine Receptor 5 Signaling Pathway in the Neuroinflammatory Response and Cognitive Deficits Induced by Beta-Amyloid Peptide. Am J Pathol (2009) 175:1586–97. doi: 10.2353/ajpath.2009.081113
83. Kaul M, Ma Q, Medders KE, Desai MK, Lipton SA. HIV-1 Coreceptors CCR5 and CXCR4 Both Mediate Neuronal Cell Death But CCR5 Paradoxically can Also Contribute to Protection. Cell Death Differ (2007) 14:296–305. doi: 10.1038/sj.cdd.4402006
84. Andres PG, Beck PL, Mizoguchi E, Mizoguchi A, Bhan AK, Dawson T, et al. Mice With a Selective Deletion of the CC Chemokine Receptors 5 or 2 are Protected From Dextran Sodium Sulfate-Mediated Colitis: Lack of CC Chemokine Receptor 5 Expression Results in a NK1.1+ Lymphocyte-Associated Th2-Type Immune Response in the Intestine. J Immunol (2000) 164:6303–12. doi: 10.4049/jimmunol.164.12.6303
85. Ajuebor MN, Aspinall AI, Zhou F, Le T, Yang Y, Urbanski SJ, et al. Lack of Chemokine Receptor CCR5 Promotes Murine Fulminant Liver Failure by Preventing the Apoptosis of Activated CD1d-Restricted NKT Cells. J Immunol (2005) 174:8027–37. doi: 10.4049/jimmunol.174.12.8027
86. Seki E, De Minicis S, Gwak G-Y, Kluwe J, Inokuchi S, Bursill CA, et al. CCR1 and CCR5 Promote Hepatic Fibrosis in Mice. J Clin Invest (2009) 119:1858–70. doi: 10.1172/jci37444
87. Liu C, Lou Y, Lizée G, Qin H, Liu S, Rabinovich B, et al. Plasmacytoid Dendritic Cells Induce NK Cell-Dependent, Tumor Antigen-Specific T Cell Cross-Priming and Tumor Regression in Mice. J Clin Invest (2008) 118:1165–75. doi: 10.1172/JCI33583
88. Huffnagle GB, McNeil LK, McDonald RA, Murphy JW, Toews GB, Maeda N, et al. Cutting Edge: Role of C-C Chemokine Receptor 5 in Organ-Specific and Innate Immunity to Cryptococcus Neoformans. J Immunol (1999) 163:4642–6.
89. Yadav A, Collman RG. CNS Inflammation and Macrophage/Microglial Biology Associated With HIV-1 Infection. J Neuroimmune Pharmacol (2009) 4:430–47. doi: 10.1007/s11481-009-9174-2
90. Mocchetti I, Campbell LA, Harry GJ, Avdoshina V. When Human Immunodeficiency Virus Meets Chemokines and Microglia: Neuroprotection or Neurodegeneration? J Neuroimmune Pharmacol (2013) 8:118–31. doi: 10.1007/s11481-012-9353-4
91. Agresti N, Lalezari JP, Amodeo PP, Mody K, Mosher SF, Seethamraju H, et al. Disruption of CCR5 Signaling to Treat COVID-19-Associated Cytokine Storm: Case Series of Four Critically Ill Patients Treated With Leronlimab. J Transl Autoimmun (2021) 4:100083. doi: 10.1016/j.jtauto.2021.100083
92. Prokop JW, Tsaih S-W, Faber AB, Boehme S, Underwood AC, Troyer S, et al. The Phenotypic Impact of the Male-Specific Region of Chromosome-Y in Inbred Mating: The Role of Genetic Variants and Gene Duplications in Multiple Inbred Rat Strains. Biol Sex Differ (2016) 7:10. doi: 10.1186/s13293-016-0064-z
93. Flister MJ, Tsaih S-W, O’Meara CC, Endres B, Hoffman MJ, Geurts AM, et al. Identifying Multiple Causative Genes at a Single GWAS Locus. Genome Res (2013) 23:1996–2002. doi: 10.1101/gr.160283.113
94. Wagner GP, Kenney-Hunt JP, Pavlicev M, Peck JR, Waxman D, Cheverud JM. Pleiotropic Scaling of Gene Effects and the “Cost of Complexity.” Nature (2008) 452:470–2. doi: 10.1038/nature06756
95. Pavlicev M, Norgard EA, Fawcett GL, Cheverud JM. Evolution of Pleiotropy: Epistatic Interaction Pattern Supports a Mechanistic Model Underlying Variation in Genotype-Phenotype Map. J Exp Zool B Mol Dev Evol (2011) 316:371–85. doi: 10.1002/jez.b.21410
96. Li T, Shen X. Pleiotropy Complicates Human Gene Editing: Ccr5Δ32 and Beyond. Front Genet (2019) 10:669. doi: 10.3389/fgene.2019.00669
97. Cwik B. Revising, Correcting, and Transferring Genes. Am J Bioeth (2020) 20:7–18. doi: 10.1080/15265161.2020.1783024
98. Liang P, Xu Y, Zhang X, Ding C, Huang R, Zhang Z, et al. CRISPR/Cas9-Mediated Gene Editing in Human Tripronuclear Zygotes. Protein Cell (2015) 6:363–72. doi: 10.1007/s13238-015-0153-5
99. Brigandt I, Love A. Reductionism in BiologyThe Stanford Encyclopedia of Philosophy, in: . Metaphysics Research Lab, Stanford University. Available at: https://plato.stanford.edu/archives/spr2017/entries/reduction-biology/ (Accessed November 11, 2021).
100. Rocca E, Anjum RL. Complexity, Reductionism and the Biomedical Model. In: Anjum RL, Copeland S, Rocca E, editors. Rethinking Causality, Complexity and Evidence for the Unique Patient: A CauseHealth Resource for Healthcare Professionals and the Clinical Encounter. Cham: Springer International Publishing (2020) p. 75–94. doi: 10.1007/978-3-030-41239-5_5
101. Wimsatt WC. Reductionism and its Heuristics: Making Methodological Reductionism Honest. Synthese (2006) 151:445–75. doi: 10.1007/s11229-006-9017-0
102. Huss J. Philosophy of Biology: Genetic Reductionism and Behavioral Genetics. In: Bioethics. Farmington Hills, MI: Macmillan Reference (2014). p. 419–21.
103. Chan AH, Whitton BA, Chan GYS. The Need for Learning Bioethics and Law for Biology Students. J Biol Educ (2020) 0:1–7. doi: 10.1080/00219266.2020.1841667
104. Juengst ET. Crowdsourcing the Moral Limits of Human Gene Editing? Hastings Cent Rep (2017) 47:15–23. doi: 10.1002/hast.701
105. Agrawal L, Lu X, Qingwen J, VanHorn-Ali Z, Nicolescu IV, McDermott DH, et al. Role for CCR5Delta32 Protein in Resistance to R5, R5X4, and X4 Human Immunodeficiency Virus Type 1 in Primary CD4+ Cells. J Virol (2004) 78:2277–87. doi: 10.1128/jvi.78.5.2277-2287.2004
106. Krensky AM, Ahn Y-T. Mechanisms of Disease: Regulation of RANTES (CCL5) in Renal Disease. Nat Clin Pract Nephrol (2007) 3:164–70. doi: 10.1038/ncpneph0418
107. Singh A, Bisht P, Bhattacharya S, Guchhait P. Role of Platelet Cytokines in Dengue Virus Infection. Front Cell Infect Microbiol (2020) 10:561366. doi: 10.3389/fcimb.2020.561366
108. Chensue SW, Warmington KS, Allenspach EJ, Lu B, Gerard C, Kunkel SL, et al. Differential Expression and Cross-Regulatory Function of RANTES During Mycobacterial (Type 1) and Schistosomal (Type 2) Antigen-Elicited Granulomatous Inflammation. J Immunol (1999) 163:165–73.
109. Ammit AJ, Lazaar AL, Irani C, O’Neill GM, Gordon ND, Amrani Y, et al. Tumor Necrosis Factor-Alpha-Induced Secretion of RANTES and Interleukin-6 From Human Airway Smooth Muscle Cells: Modulation by Glucocorticoids and Beta-Agonists. Am J Respir Cell Mol Biol (2002) 26:465–74. doi: 10.1165/ajrcmb.26.4.4681
110. Chui R, Dorovini-Zis K. Regulation of CCL2 and CCL3 Expression in Human Brain Endothelial Cells by Cytokines and Lipopolysaccharide. J Neuroinflamm (2010) 7:1. doi: 10.1186/1742-2094-7-1
111. McManus CM, Brosnan CF, Berman JW. Cytokine Induction of MIP-1 Alpha and MIP-1 Beta in Human Fetal Microglia. J Immunol (1998) 160:1449–55.
112. Shukaliak JA, Dorovini-Zis K. Expression of the Beta-Chemokines RANTES and MIP-1 Beta by Human Brain Microvessel Endothelial Cells in Primary Culture. J Neuropathol Exp Neurol (2000) 59:339–52. doi: 10.1093/jnen/59.5.339
113. da Fonseca ACC, Matias D, Garcia C, Amaral R, Geraldo LH, Freitas C, et al. The Impact of Microglial Activation on Blood-Brain Barrier in Brain Diseases. Front Cell Neurosci (2014) 8:362. doi: 10.3389/fncel.2014.00362
114. Tetreault NA, Hakeem AY, Jiang S, Williams BA, Allman E, Wold BJ, et al. Microglia in the Cerebral Cortex in Autism. J Autism Dev Disord (2012) 42:2569–84. doi: 10.1007/s10803-012-1513-0
115. Subileau EA, Rezaie P, Davies HA, Colyer FM, Greenwood J, Male DK, et al. Expression of Chemokines and Their Receptors by Human Brain Endothelium: Implications for Multiple Sclerosis. J Neuropathol Exp Neurol (2009) 68:227–40. doi: 10.1097/NEN.0b013e318197eca7
116. Ubogu EE, Callahan MK, Tucky BH, Ransohoff RM. CCR5 Expression on Monocytes and T Cells: Modulation by Transmigration Across the Blood-Brain Barrier. vitro Cell Immunol (2006) 243:19–29. doi: 10.1016/j.cellimm.2006.12.001
117. Sorce S, Myburgh R, Krause K-H. The Chemokine Receptor CCR5 in the Central Nervous System. Prog Neurobiol (2011) 93:297–311. doi: 10.1016/j.pneurobio.2010.12.003
118. Sorce S, Bonnefont J, Julien S, Marq-Lin N, Rodriguez I, Dubois-Dauphin M, et al. Increased Brain Damage After Ischaemic Stroke in Mice Lacking the Chemokine Receptor CCR5. Br J Pharmacol (2010) 160:311–21. doi: 10.1111/j.1476-5381.2010.00697.x
119. Khan IA, Thomas SY, Moretto MM, Lee FS, Islam SA, Combe C, et al. CCR5 is Essential for NK Cell Trafficking and Host Survival Following Toxoplasma Gondii Infection. PloS Pathog (2006) 2:e49. doi: 10.1371/journal.ppat.0020049
120. Teixeira MM, Vilela MC, Soriani FM, Rodrigues DH, Teixeira AL. Using Intravital Microscopy to Study the Role of Chemokines During Infection and Inflammation in the Central Nervous System. J Neuroimmunol (2010) 224:62–5. doi: 10.1016/j.jneuroim.2010.05.018
121. Thapa M, Kuziel WA, Carr DJJ. Susceptibility of CCR5-Deficient Mice to Genital Herpes Simplex Virus Type 2 is Linked to NK Cell Mobilization. J Virol (2007) 81:3704–13. doi: 10.1128/JVI.02626-06
122. Belnoue E, Kayibanda M, Deschemin J-C, Viguier M, Mack M, Kuziel WA, et al. CCR5 Deficiency Decreases Susceptibility to Experimental Cerebral Malaria. Blood (2003) 101:4253–9. doi: 10.1182/blood-2002-05-1493
123. Babcock AA, Kuziel WA, Rivest S, Owens T. Chemokine Expression by Glial Cells Directs Leukocytes to Sites of Axonal Injury in the CNS. J Neurosci (2003) 23:7922–30. doi: 10.1523/JNEUROSCI.23-21-07922.2003
124. Westmoreland SV, Alvarez X, deBakker C, Aye P, Wilson ML, Williams KC, et al. Developmental Expression Patterns of CCR5 and CXCR4 in the Rhesus Macaque Brain. J Neuroimmunol (2002) 122:146–58. doi: 10.1016/s0165-5728(01)00457-x
125. Park MH, Lee YK, Lee YH, Kim Y-B, Yun YW, Nam SY, et al. Chemokines Released From Astrocytes Promote Chemokine Receptor 5-Mediated Neuronal Cell Differentiation. Exp Cell Res (2009) 315:2715–26. doi: 10.1016/j.yexcr.2009.06.017
126. Meucci O, Fatatis A, Simen AA, Bushell TJ, Gray PW, Miller RJ. Chemokines Regulate Hippocampal Neuronal Signaling and Gp120 Neurotoxicity. Proc Natl Acad Sci USA (1998) 95:14500–5. doi: 10.1073/pnas.95.24.14500
127. Tran PB, Banisadr G, Ren D, Chenn A, Miller RJ. Chemokine Receptor Expression by Neural Progenitor Cells in Neurogenic Regions of Mouse Brain. J Comp Neurol (2007) 500:1007–33. doi: 10.1002/cne.21229
128. Kranjc MK, Novak M, Pestell RG, Lah TT. Cytokine CCL5 and Receptor CCR5 Axis in Glioblastoma Multiforme. Radiol Oncol (2019) 53:397–406. doi: 10.2478/raon-2019-0057
129. Brunn A, Montesinos-Rongen M, Strack A, Reifenberger G, Mawrin C, Schaller C, et al. Expression Pattern and Cellular Sources of Chemokines in Primary Central Nervous System Lymphoma. Acta Neuropathol (2007) 114:271–6. doi: 10.1007/s00401-007-0258-x
130. Zhang H, Chen K, Tan Q, Shao Q, Han S, Zhang C, et al. Structural Basis for Chemokine Recognition and Receptor Activation of Chemokine Receptor CCR5. Nat Commun (2021) 12:4151. doi: 10.1038/s41467-021-24438-5
131. Snijders Blok L, Hiatt SM, Bowling KM, Prokop JW, Engel KL, Cochran JN, et al. De Novo Mutations in MED13, a Component of the Mediator Complex, are Associated With a Novel Neurodevelopmental Disorder. Hum Genet (2018) 137:375–88. doi: 10.1007/s00439-018-1887-y
132. Hiatt SM, Neu MB, Ramaker RC, Hardigan AA, Prokop JW, Hancarova M, et al. De Novo Mutations in the GTP/GDP-Binding Region of RALA, a RAS-Like Small GTPase, Cause Intellectual Disability and Developmental Delay. PloS Genet (2018) 14:e1007671. doi: 10.1371/journal.pgen.1007671
133. Sirpilla O, Bauss J, Gupta R, Underwood A, Qutob D, Freeland T, et al. SARS-CoV-2-Encoded Proteome and Human Genetics: From Interaction-Based to Ribosomal Biology Impact on Disease and Risk Processes. J Proteome Res (2020) 19:4275–90. doi: 10.1021/acs.jproteome.0c00421
Keywords: CCR5, viral infections, expression analysis, evolutionary profiling, molecular dynamic simulations, microglia, educational material generation
Citation: Bauss J, Morris M, Shankar R, Olivero R, Buck LN, Stenger CL, Hinds D, Mills J, Eby A, Zagorski JW, Smith C, Cline S, Hartog NL, Chen B, Huss J, Carcillo JA, Rajasekaran S, Bupp CP and Prokop JW (2021) CCR5 and Biological Complexity: The Need for Data Integration and Educational Materials to Address Genetic/Biological Reductionism at the Interface of Ethical, Legal, and Social Implications. Front. Immunol. 12:790041. doi: 10.3389/fimmu.2021.790041
Received: 06 October 2021; Accepted: 18 November 2021;
Published: 02 December 2021.
Edited by:
Joel Henrique Ellwanger, Federal University of Rio Grande do Sul, BrazilReviewed by:
Jose Artur Chies, Federal University of Rio Grande do Sul, BrazilLeonardo Augusto Luvison Araujo, University of São Paulo, Brazil
Copyright © 2021 Bauss, Morris, Shankar, Olivero, Buck, Stenger, Hinds, Mills, Eby, Zagorski, Smith, Cline, Hartog, Chen, Huss, Carcillo, Rajasekaran, Bupp and Prokop. This is an open-access article distributed under the terms of the Creative Commons Attribution License (CC BY). The use, distribution or reproduction in other forums is permitted, provided the original author(s) and the copyright owner(s) are credited and that the original publication in this journal is cited, in accordance with accepted academic practice. No use, distribution or reproduction is permitted which does not comply with these terms.
*Correspondence: Jeremy W. Prokop, jprokop54@gmail.com