- 1Department of Biology, School of Life Sciences, Southern University of Science and Technology, Shenzhen, China
- 2Institute of Integrated Bioinfomedicine and Translational Science (IBTS), School of Chinese Medicine, Hong Kong Baptist University, Hong Kong, Hong Kong SAR, China
- 3Law Sau Fai Institute for Advancing Translational Medicine in Bone and Joint Diseases, School of Chinese Medicine, Hong Kong Baptist University, Hong Kong, Hong Kong SAR, China
- 4Institute of Arthritis Research in Integrative Medicine, Shanghai Academy of Traditional Chinese Medicine, Shanghai, China
- 5Department of Rheumatology, Shanghai Guanghua Hospital of Integrative Medicine, Shanghai, China
- 6Guangdong-Hong Kong-Macau Joint Lab on Chinese Medicine and Immune Disease Research, Guangzhou, China
Rheumatoid arthritis (RA) is an autoimmune disorder characterized by inflammation and bone erosion. The exact mechanism of RA is still unknown, but various immune cytokines, signaling pathways and effector cells are involved. Disease-modifying antirheumatic drugs (DMARDs) are commonly used in RA treatment and classified into different categories. Nevertheless, RA treatment is based on a “trial-and-error” approach, and a substantial proportion of patients show failed therapy for each DMARD. Over the past decades, great efforts have been made to overcome treatment failure, including identification of biomarkers, exploration of the reasons for loss of efficacy, development of sequential or combinational DMARDs strategies and approval of new DMARDs. Here, we summarize these efforts, which would provide valuable insights for accurate RA clinical medication. While gratifying, researchers realize that these efforts are still far from enough to recommend specific DMARDs for individual patients. Precision medicine is an emerging medical model that proposes a highly individualized and tailored approach for disease management. In this review, we also discuss the potential of precision medicine for overcoming RA treatment failure, with the introduction of various cutting-edge technologies and big data.
Introduction
Rheumatoid arthritis (RA), an autoimmune disorder that preferentially attacks the joints, affects approximately 1% of people worldwide (1). RA patients experience morning stiffness in the early stage, which manifests as facet joint pain, swelling, and synovitis. In the late stage, small focal necrosis and granulation tissue pannus formation appear, spreading to the cartilage surface, accompanied by symmetrical polyarticular swelling, bone erosion and pain mainly in the interphalangeal and metacarpophalangeal joints and limited mobility (2). Finally, granulation tissue and fibrous tissue adhesion appear on the articular surface, forming deformity symptoms such as ankylosis and joint subluxation. Most patients also present with extra-articular multisystem involvement in skin, blood, kidneys and lungs, further aggravating the condition (2).
The exact mechanism of RA development is unknown, but both genetic and environmental factors are contributory. Various proinflammatory cytokines and immune cells are involved in RA pathophysiology (3). In the progress of RA, the synovium is infiltrated by leukocytes and synovial fluid is inundated with pro-inflammatory cytokines, such as tumor necrosis factor (TNF), interleukin (IL)-6, IL-17 and IL-1β (4). These cytokines induce an inflammatory cascade characterized by interactions of fibroblast-like synoviocytes (FLS) with innate immune cells, including macrophages, monocytes, dendritic cells and mast cells, as well as adaptive immune cells such as B and T cells. TNF also promotes bone resorption and bone erosion. IL-1 indirectly stimulates osteoclast formation. IL-6 aggravates pathogenic effects in RA by enhancing the inflammatory effects of IL-1 and TNF (4). An imbalance between osteoblasts/osteoclasts and regulatory T (Treg)/T helper (Th)17 cells are typical characteristics of RA (5).
RA requires the combined effects of different signaling pathways, such as receptor activator of nuclear factor kappa B ligand (RANKL)/receptor activator of nuclear factor kappa B (RANK)/osteoprotegerin (OPG) and IL-6/glycoprotein 130 (gp130)/janus kinase (JAK)/signal transducer and activator of transcription (STAT). For RANKL/RANK/OPG signaling, binding of RANKL to RANK induces nuclear factor kappa B (NF-κB) activation, which upregulates levels of pro-inflammatory cytokines (IL-1, IL-6 and TNF) and mediates proliferation of T and B cells (6). OPG binds explicitly to RANKL and inhibits RANKL activity by preventing its binding to RANK. RANKL promotes the differentiation and production of osteoclasts. For IL-6/gp130/JAK/STAT signaling, IL-6 binds to IL-6 receptor (IL-6R). The IL-6/IL-6R complex interacts with gp130 to induce its dimerization and initiate intracellular signaling via JAK/STAT pathway, thus increasing T cell activity, inhibiting FLS apoptosis, allowing B cell maturation and stimulating differentiation of naive T cells into Th17 cells (7).
To assess disease activity of RA, the American College of Rheumatology (ACR), the European League Against Rheumatism (EULAR) and the World Health Organization/International League Against Rheumatism (WHO/ILAR) have established a core set of variables, which include swollen joint count (SJC), tender joint count (TJC), physician’s global assessment of disease activity (PhGA), patient’s global assessment of disease activity (PtGA), patient’s assessment of pain, patient’s assessment of physical function, and level of an acute phase reactant (APR, either C-reactive protein (CRP) or erythrocyte sedimentation rate (ESR)) (8). Based on these variables, composite measurement tools with strong clinimetric properties have been developed. Among these tools are dichotomous indices like the ACR response criteria (ACR20, 50 and 70) (9), and continuous scores like the Disease Activity Score for 28 joints (DAS28), the Clinical Disease Activity Index (CDAI) and the Simplified Disease Activity Index (SDAI) (10). ACR20, 50 and 70 are based on improvement of at least 20%, 50% and 70% in both TJC and SJC, and three of the five additional core set of variables listed above, respectively. DAS28 considers TJC and SJC of 28 joints, PtGA, plus level of an APR (either ESR or CRP) (11). CDAI is based on the simple summation of TJC and SJC of 28 joints, along with PhGA and PtGA (12). SDAI is the arithmetic sum of TJC and SJC of 28 joints, PhGA, PtGA and level of an APR (CRP) (12). These tools allow better standardization and interpretation of disease activity of RA and patient response to therapy.
The EULAR has updated its recommendations for the management of RA in 2019, which are regarded as the main guidelines worldwide. In this update, most of the recommendations remain unchanged when reviewing its first version one decade ago and the updates in 2013 and 2016. The target of treatment remains as sustained remission (according to the ACR-EULAR definition) or low disease activity, and the major focus continues to be pharmacological therapy with disease-modifying antirheumatic drugs (DMARDs) (13). The DMARDs are divided into conventional synthetic (cs) DMARDs (such as methotrexate, leflunomide and sulfasalazine), biological (b) DMARDs [TNF inhibitors (infliximab, etanercept, adalimumab, certolizumab pegol and golimumab), a T cell co-stimulation inhibitor (abatacept), a cluster of differentiation 20 (CD20) inhibitor (rituximab), IL-6R inhibitors (tocilizumab and sarilumab) and biosimilar (bs) DMARDs)] and targeted synthetic (ts) DMARDs [JAK inhibitors (such as tofacitinib, baricitinib and upadacitinib)] (13).
Over the past years, the management of RA has progressed remarkably, encompassing the development of the above measurement tools and approvals of various DMARDs. However, the response is not universal for any treatment option. A large number of clinical trials have demonstrated that substantial proportions of RA patients experience treatment failure after receiving csDMARDs and even bDMARDs and tsDMARDs (14–19). Treatment failure is defined as nonresponse or limited efficacy (16, 17, 20), including initial lack of response, responsiveness over time, and inadequate response (partial response). To date, continuous efforts have been made toward overcoming treatment failure in RA patients, such as identification of biomarkers for response or nonresponse to DMARDs, exploration of the reasons for loss of efficacy, development of sequential or combinational DMARDs strategies either within the same or different mechanistic class, and approval of new DMARDs (13). Some of them provide valuable insights that can help to improve the design of future clinical trials and enable accurate clinical medication (21).
Due to the striking heterogeneity of RA, people realize that the current efforts are far from enough to recommend specific DMARDs for individual patients, which is also highlighted by EULAR as an important issue to be addressed in the future (13). Precision medicine, also called personalized medicine, is an emerging medical model that proposes a highly individualized and tailored approach for patient management by accounting for individual variability in genes, environment, and lifestyle, instead of a one‐drug‐fits‐all model (22). It involves the ability to classify individuals into subpopulations that are susceptible or responsive to a specific treatment (23). Precision medicine is in its infancy and has not become a routine practice in RA. But it is anticipated that precision medicine would have tremendous potential to address the treatment failure for RA (24).
In this review, we summarize the current efforts in identifying biomarkers for DMARDs, exploring the reasons for loss of efficacy, developing sequential or combinational DMARDs strategies and approving of new DMARDs, toward overcoming treatment failure in RA. We also discuss the opportunities and advantages of precision medicine approaches to make a breakthrough in diagnosis, prognosis, and treatment selection for RA.
csDMARDs
Methotrexate: Mechanism, Biomarkers and Alternative Therapy
Despite the wealth of new agents, methotrexate approved by FDA in 1988 remains the primary starting therapy and anchor drug for the treatment of RA, owing to its inexpensive cost, extended safety record, and weekly treatment regimen (25). Mechanism of action in methotrexate is not fully understood. DMARD activity of methotrexate is thought to be due to its polyglutamated form and several mechanisms have been proposed to explain the clinical efficacy in RA, including generation of reactive oxygen species, antagonism of folate-dependent processes, inhibition of methyl-donor production, downregulation of adhesion-molecule, eicosanoids and matrix metalloproteinases (MMPs) expression, modification of cytokine profiles, stimulation of adenosine signaling, inhibition of RANKL/RANK/OPG and JAK/STAT pathways (26–28). Clinical trials with methotrexate monotherapy demonstrate that only 40% of patients with early RA obtain a good response based on ACR50 criteria (29).
Currently, adenosine signaling carries the most robust data for the action of methotrexate in RA (30). Both adenosine A2A receptor (ADORA2A) and A3 receptor (ADORA3) are required for the anti-inflammatory effects of methotrexate (31). The expression of ADORA2A and ADORA3 is increased on immune cells and inversely correlated with disease activity in RA patients (32). It is possible that RA patients with low expression of adenosine receptors will be less responsive to methotrexate. In a study with methotrexate monotherapy, RA patients were categorized into three groups, i.e. good, moderate and nonresponders. A low level of baseline ADORA3 mRNA expression in blood is associated with nonresponse to methotrexate and could serve as a potential biomarker for distinguishing response to methotrexate therapy in RA (33). Adenosine signaling through ADORA2A leads to the development of Tregs expressing both CD39 and CD73 that may decrease T cell activation (34, 35). A prospective study found that RA patients who did not respond to methotrexate had lower pretreatment CD39 expression on Tregs than methotrexate-responsive patients or healthy controls, suggesting that low expression of CD39 on Tregs could be a biomarker for identifying methotrexate-resistant RA patients (34). Clinical observations suggested that RA patients who had a high intake of adenosine receptor antagonists (such as caffeine) had impaired methotrexate responsiveness, which was consistent with data from animal models (36, 37). However, conflicting evidence manifested that methotrexate efficacy was not affected by adenosine receptor antagonists (38, 39).
In addition to the adenosine signaling, exploration of pharmacometabolic markers, expression and polymorphisms of genes linked to the action of methotrexate is underway to identify methotrexate-responsive or nonresponsive signatures. In a longitudinal study, an increase in methotrexate polyglutamates in erythrocytes was associated with lower disease activity of RA and thought to be a tool for monitoring methotrexate response (40). Changing from oral to subcutaneous methotrexate resulted in increased methotrexate polyglutamates and achieved a better improvement of disease activity of RA (41). A low baseline folate level was associated with a poor response to methotrexate and folate polyglutamate partially antagonized methotrexate efficacy (40, 42). Breast cancer resistance protein [BCRP, gene symbol ATP Binding Cassette Subfamily G Member 2 (ABCG2)] is an ATP-binding cassette efflux transporter that plays an important role in multidrug resistance (43). BCRP transported both methotrexate and polyglutamylated methotrexate and participated in methotrexate resistance (44). Good response to methotrexate was associated with a decrease in expression of BCRP in RA patients (45), while the association of BCRP polymorphisms with the effectiveness of methotrexate was not observed (46), suggesting that BCRP expression was not genetically determined, but might be associated with environmental factors (45). BCRP inhibition could be a strategy for overcoming nonresponse to methotrexate. Consistently, a six-month randomized, double-blind trial enrolling 148 RA patients showed that individuals with partial responses to methotrexate had clinical improvement after combination therapy with an FDA-approved BCRP inhibitor cyclosporine and methotrexate (47). Other studies found that baseline FcγRIIIa expression on CD14+ monocytes was negatively associated to methotrexate response in patients with early RA (48). Circulating miR-10a was upregulated in RA patients with good methotrexate response (49). Human leukocyte antigen (HLA)-DRB1 shared epitope alleles were linked to a lack of response to methotrexate at the genomic level (50). Stratification based on HLA-DRB4 allele expression revealed distinct innate and adaptive immune transcriptional patterns in early RA and response to methotrexate therapy could be suggested by a preponderance of innate but not adaptive immune activation (51). A number of single-nucleotide polymorphisms have been investigated for the prediction of methotrexate treatment response. Patients with solute carrier family 19 A (SLC19A) rs1051266, dihydrofolate reductase (DHFR) rs836788 and thymidylate synthetase (TYMS) rs2244500 showed response to methotrexate, while patients with 5-aminoimidazole-4-carboxamide ribonucleotide formyltransferase (ATIC) rs7563206, TYMS rs3786362 and rs2847153 showed reduced effectiveness of methotrexate (52). Patients with folylpolyglutamate synthetase (FPGS) rs1544105-AA or -AG and TYMS rs2853539-AA genotype were associated with poor response to methotrexate (53). An analysis of the -174 (rs1800795) -GC IL-6 gene promoter polymorphism in RA patients revealed that genotype -GG may be associated with a poorer response to methotrexate when compared to genotypes -GC and -CC (54), which was in disagreement with another study identifying no association between -GG genotype or G allele and risk of therapeutic failure using different measures for defining response to therapy (55) (Table 1).
In RA patients with treatment failure of methotrexate, combination therapy is an attractive alternative strategy. A series of clinical trials (such as an observational and descriptive CONAART study enrolling 106 RA patients, a 24-week, randomized, double-blind, controlled SLCTR study enrolling 40 patients and a 48-week, randomized, double-blind, placebo-controlled study enrolling 263 patients) showed that leflunomide in combination with methotrexate was effective for RA patients who did not respond to methotrexate (65, 66). In a 12-month, multicenter, randomized, double-blind, placebo-controlled, parallel-design, dose-finding phase II trial, 115, 105, and 119 RA patients with inadequate response to methotrexate were grouped to receive 2 mg/kg abatacept, 10 mg/kg abatacept, and placebo, in addition to continued methotrexate treatment, respectively. Results showed that 10 mg/kg abatacept presented better anti-inflammatory effects than either 2 mg/kg abatacept and placebo (67). In a double-blind, randomized, parallel-arm MUSICA trial enrolling 309 methotrexate nonresponders, patients were randomly assigned to receive either a high dose (20 mg/week) or a low dose (7.5 mg/week) of methotrexate and received open-label adalimumab for 24 weeks. Adalimumab treatment resulted in a rapid improvement in clinical indices in both groups, which is consistent with results from an OPTIMA study (a 78-week, randomized, double-blind, double-period phase 4 trial enrolling 348 methotrexate inadequate responders) (68) and a PREMIER study (a 2-year, randomized, double-blind, placebo-controlled phase 3 trial enrolling 177 methotrexate inadequate responders) (68). In a prospective, randomized, controlled SURPRISE study enrolling 223 RA patients, tocilizumab in combination with methotrexate more rapidly deceased inflammation than tocilizumab switched from methotrexate, resulting in greater clinical effectiveness and avoidance of joint damage (69).
Leflunomide: Mechanism, Biomarkers and Alternative Therapy
Leflunomide, approved by the FDA in 1998, is the first choice if methotrexate is contraindicated according to the latest EULAR recommendations (13). Leflunomide acts via its active metabolite A77 1726 after the metabolic opening of the isoxazole ring. Its primary target is thought to be dihydroorotate dehydrogenase (DHODH), an enzyme involved in de novo pyrimidine production (70). Leflunomide inhibits DHODH activity, resulting in nucleotide depletion, leading to cell cycle arrest and reproduction of rapidly dividing cells, particularly lymphocytes (71). Tyrosine kinases such as Lck and JAK3 in activated T and B cells are also targets of leflunomide (72). Clinical trials have shown that only 40-50% of RA patients taking leflunomide fulfilled the ACR response criteria for a 20% reduction in disease activity (59).
DHODH is located on the inner membrane of mitochondria (73). The human DHODH gene is relatively conserved, with only one common missense polymorphism (rs3213422) in the first exon (19C>A). This polymorphism led to Gln7Lys amino acid substitution in the cationic N-terminal region of the DHODH polypeptide, which was essential for transport and correct insertion into the mitochondrial inner membrane (57). A study reported that RA patients with A allele had a worse response to leflunomide than patients with the C allele (57). A proposed mechanism was that the amino acid substitution generated by the missense polymorphism in DHODH might block its import into mitochondria and subsequently affect the action of leflunomide (57). However, another study did not replicate the association between leflunomide response and rs3213422 in a smaller cohort of indviduals (74). Cytochromes P450 (CYP) enzymes, including CYP1A2 and CYP2C19, may be implicated in the conversion of leflunomide to A77 1726. Better response to leflunomide was accompanied by higher A77 1726 steady-state plasma concentration, which was influenced by CYP2C19*2 allele rather than CYP1A2 polymorphism (56). Evidence demonstrated that the efficacy of DMARDs is more effective in men than in women and estrogens play important roles in the immune response. A study found that the A/T haplotype of the estrogen receptor 1 (ESR1) rs9340799-rs2234693 was related with a better sensitivity to leflunomide, while the G/C haplotype was associated with a worse response (58). Researchers also evaluated the influence of the rs1800795-GC IL-6 gene promoter polymorphism on the therapeutic failure of leflunomide (54, 55, 75). RA patients with IL-6 rs1800795-GG genotype had a higher risk of failure in therapeutic response to leflunomide when compared to patients with -GC (55), which was contrary to other observations of noninfluence of the rs1800795-GC IL-6 gene polymorphism on response to leflunomide that used different measures for defining response to therapy (54, 75). Similar to that of methotrexate, drug efflux transporter BCRP was reported to interact with leflunomide and A771726, and an increased level of BCRP might contribute to inadequate response to leflunomide (76). In a 12-month open, prospective trial enrolling 106 RA patients, the combination of a BCRP inhibitor cyclosporine and leflunomide provided statistically significant benefit (77), suggesting that BCRP inhibition could be a potential approach for improving the nonresponse to leflunomide.
Recently, our group found that RA individuals with limited efficacy of leflunomide could be distinguished by higher serum baseline CRP level. Besides the immunomodulation via A77 1726, we revealed that leflunomide itself induced aryl hydrocarbon receptor (AHR)-AHR nuclear translocator (ARNT) interaction to inhibit hepatic CRP production and attenuate bone erosion in arthritic rat models. Nevertheless, enforced CRP expression upregulated hypoxia-inducible factor 1α (HIF1α), which competed with AHR for ARNT association and interfered leflunomide-AHR-CRP signaling, leading to nonresponse to leflunomide in arthritic rat models. Hepatocyte-specific HIF1α deletion or an FDA-approved HIF1α inhibitor Acriflavine re-activated leflunomide-AHR-CRP signaling to inhibit bone erosion in leflunomide-nonresponsive animals. This study presented a precision medicine-based therapeutic strategy for overcoming nonresponse to leflunomide in RA (59). In addition, we also performed a 48-week, randomized, controlled clinical trial enrolling 123 RA patients, and showed that leflunomide combined with ligustrazine extracted the Chinese herb Chuanxiong, which was an approved drug in China and had the capacity to inhibit HIF1α expression (78), could significantly reduce disease activity (79). Regarding other alternative treatment options, leflunomide plus infliximab present a general improvement in disease control compared with leflunomide alone in an open, multicenter, retrospective study (80). In a 24 week, double-blind phase of the multicenter, international RELIEF study enrolling 106 inadequate responders to leflunomide, the trend of benefit was indicated for combining leflunomide with sulfasalazine compared with switching to sulfasalazine alone (81) (Table 1).
Sulfasalazine: Mechanism, Biomarkers and Alternative Therapy
Based on the latest EULAR recommendation, sulfasalazine approved by FDA in 1996 is also considered as part of the (first) treatment strategy in RA patients with a contraindication to methotrexate, which is in parallel with leflunomide (13). Among the above agents, sulfasalazine has an acceptable safety profile during pregnancy (82, 83). The mechanism of sulfasalazine is not entirely understood. It is unknown if sulfasalazine or its metabolites such as sulfapyridine and 5-aminosalicylic acid have a role in its anti-inflammatory actions. It is suggested that sulfasalazine inhibits TNF expression by suppressing NF-κB and by inducing caspase 8-induced apoptosis in macrophages (84). Sulfasalazine inhibits osteoclast formation by suppression of RANKL and stimulation of osteoprotegerin (85). Sulfasalazine induces the conversion of adenine nucleotides to adenosine (86). Sulfapyridine and 5-aminosalicylic acid inhibit B cell function and suppress the production of IgM and IgG (87). Sulfapyridine inhibits chemokines IL-8, growth-related gene product-alpha (gro alpha), and monocyte chemotactic protein-1 (MCP-1/CCL2) (88). 5-aminosalicylic acid (5-ASA) inhibits NF-κB signaling by inducing phosphorylation and activation of adenosine monophosphate-activated protein kinase (89). Studies have confirmed that the ACR20 response in RA patients does not exceed 50% after 6 months of sulfasalazine treatment (90, 91).
Research showed that sulfasalazine also interacted primarily with the above-mentioned drug efflux transporter BCRP. BCRP knockout mice had a more than 100-folds increase in plasma concentration of sulfasalazine compared with wild-type (WT) mice (62). Sulfasalazine bioavailability in BCRP knockout mice was 97% compared to 3% in WT mice (92). Of note, treatment of WT mice with a BCRP inhibitor (gefitinib) resulted in a significant increase in plasma concentration and bioavailability of sulfasalazine (92). This suggests that BCRP could be a therapeutic target for eliminating nonresponse to sulfasalazine. Another study found a circulating intestine-derived exosomal miR-328 in plasma, which negatively regulated BCRP expression and resulted in a high plasma concentration of sulfasalazine (60), could be a biomarker of sulfasalazine responsiveness. P-glycoprotein (P-gp), like BCRP, is another drug efflux transporter. It was reported that serum P-gp level was higher in patients with active RA compared to inactive RA patients (93). Serum P-gp level was negatively correlated with sulfasalazine efficacy (61). Sulfasalazine oral bioavailability was markedly increased 2-3 folds in P-gp knockout rats (94). P-gp on Th1 cells participated in the drug resistance to sulfasalazine in RA (95). These results inspire a hypothesis that blockage of P-gp may mimic the effectiveness of BCRP inhibition in overcoming the nonresponse to sulfasalazine. However, it was discouraging to observe that a P-gp inhibitor verapamil could not reverse sulfasalazine nonresponse (96). We assumed that BCRP was mainly responsible for efflux transport of sulfasalazine because plasma concentration of sulfasalazine was more significantly increased in BCRP knockout animals (more than 100 folds) when compared to that in P-gp knockout animals (2-3 folds). It is possible that pharmacological inhibition of P-gp by verapamil could be compensated by the powerful BCRP function in the efflux of sulfasalazine, which should be verified in future studies. Regarding the gene polymorphism, an association between ABCG2 genotype and remission was found, and carriers of the loss of function alleles (that is, ABCG2 rs2231142 -AC or -AA genotype) had higher plasma sulfasalazine concentrations (62). Other reports indicated that a low interferon (IFN)/IL-4 ratio is associated with a better response to sulfasalazine (63). HLA-B27-positive patients presented a better response to sulfasalazine (64). A low level of soluble IL-2 receptor predicted remission in early RA patients treated with sulfasalazine (63). Sulfasalazine responders had lower serum MMP-3 values compared to partial responders or nonresponders (97) (Table 1).
In an 18-month, randomized, double-blind, placebo-controlled MASCOT study, 165 RA patients who were nonresponders to 6-month sulfasalazine therapy were grouped to receive methotrexate, sulfasalazine and a combination of sulfasalazine and methotrexate for additional 12 months, respectively. The combination significantly decreased DAS and improved the ACR scores when compared to either drug alone (98). This study, together with other randomized controlled trials, were included in a meta-analysis, which suggested that the addition of methotrexate to sulfasalazine is a therapeutic option in SSZ sulfasalazine failure (99). In a 2-year, double-blind, randomized study, 260 sulfasalazine nonresponders were randomly assigned to etanercept, sulfasalazine and etanercept plus sulfasalazine, respectively. A significant improvement was seen in the group treated with etanercept plus sulfasalazine when compared to the other two groups (100). In a 52-week, multicenter, double-blind, parallel-group trial, a total of 123 DMARDs (including sulfasalazine) nonresponders were randomized to receive tacrolimus (an inhibitor targeting calcineurin, which is involved in the production of IL-2) or placebo. Data showed that tacrolimus was helpful for achieving a better clinical response according to ACR20 and EULAR response criteria (101).
bDMARDs Targeting TNF
Infliximab: Mechanism, Biomarkers and Alternative Therapy
TNF has been identified its central role in RA at the end of the last century. At the time, little was known about the mechanisms of csDMARDs and people had no better choice for treating RA. This led to a question about whether blockade of TNF could serve as a treatment method. With the development of monoclonal antibodies, this question was firstly answered. In 1992, cA2, now known as infliximab, was produced to confirm that the inflammation driving RA could be suppressed by TNF blockade (102). Infliximab was approved by the FDA in 1999 for RA treatment and attracted an inordinate amount of attention over the past several decades (103). According to the most recent EULAR recommendation, if the treatment goal is not met with the initial csDMARD strategy and there are poor prognostic factors, a bDMARD should be added (13). TNF inhibitors are now the most frequently used bDMARDs (104) and infliximab serves as a first-in-class TNF inhibitor (103). Infliximab is an intravenous administrated, chimeric monoclonal IgG1κ antibody composed of human constant (75%) and murine variable (25%) regions (105). Infliximab binds to both soluble and transmembrane forms of TNF with high affinity, inducing the downregulation of local and systemic pro-inflammatory cytokines (e.g., IL-6), the reduction of lymphocyte and leukocyte migration to sites of inflammation, the induction of apoptosis in TNF-producing cells and the reduction of levels of endothelial adhesion molecules and APR (105). Only approximately 50% of RA patients showed ACR20 response after receiving infliximab treatment (106, 107).
Anti-drug antibodies (ADAs), generated by a T-cell dependent or independent B cell activation pathway, primarily contribute to a poor clinical outcome of biological treatment (108–110). In fact, there are two types of ADAs that can be produced: non-neutralizing antibodies that bind to the medication alongside TNF and neutralizing antibodies that compete with TNF for the antigen-binding site (paratope). Neutralizing antibodies can therefore immediately inhibit the working mechanism of the anti-TNF agents (111). Several clinical trials demonstrated that ADAs might be associated with treatment failure of infliximab (112, 113). Over 40% of patients treated with infliximab developed ADAs (114). Of interest, it was reported that concomitant administration of csDMARDs such as methotrexate might decrease ADAs and prolong therapeutic efficacy (115). Furthermore, the emergence of ADAs may be related with lower serum concentrations of (free) infliximab and a lower clinical response (116). Low infliximab serum concentrations, even 2 months after treatment commencement, were associated with the production of ADAs and predicted later treatment failure (117). However, another study found that lack of response could be due to a lack of infliximab, rather than the presence of ADAs (118), which in line with a hypothesis that drug tolerance was not directly related to the quantity of anti-drug antibodies, but rather depended on the size of the response in relation to the amount of drug that could be neutralized (115).
S100 calcium-binding protein A4 (S100A4) is a metastasis-inducing protein, which promotes the inflammatory response of mononuclear cells via the Toll-like receptors (TLR4) signaling in RA (119). It was reported that high S100A4 level was associated with inadequate response to infliximab, ADAs production and high levels of survivin and FMS-like tyrosine kinase 3 (Flt3) ligand (120). Flt3 ligand is a differentiation factor that has predictive value in the preclinical diagnosis of RA (121). Survivin is a downstream molecule of Flt3 signaling and high survivin level predicted poor clinical response to infliximab in RA patients (121, 122). It was proposed that S100A4, survivin and Flt3 ligand could form a new cluster of predictive biomarkers for infliximab nonresponders (120). Another study developed a customized low-density microarray for monitoring mRNA expression in peripheral blood cells, which was helpful for identifying a unique set of genes with differential expressions in infliximab responders and nonresponders. It was important to note that TNF-α itself did not differ significantly between responders and nonresponders, while a clear difference was observed in the kinetics of IFN-related genes during infliximab treatment between the two groups. Specifically, there was sustained inhibition of the IFN signature in responders and reappearance of the signature in nonresponders during infliximab treatment. The underlying mechanism remains to be clarified, and such knowledge will likely identify new therapeutic targets for RA (123). In addition, other data showed that AP-1-associated adaptor complex subunit responsible for protein transport between membrane compartments in receptor-mediated endocytosis was significantly downregulated in peripheral mononuclear cells of infliximab nonresponders (123). TNF receptor recycling was inhibited in nonresponders to infliximab (123). Human immunoglobulin allotypes in the IgG1 heavy chain (G1m1 and G1m17 allotypes) were associated with response to infliximab (124). A significantly decreased CRP level was a predictor of good response with infliximab treatment (125). Patients with low-affinity homozygotes, fragment crystallizable (Fc) fragment of IgG receptor (FCGR)2A and FCGR3A alleles showed better response to infliximab (126). The baseline level of IgG antibodies against centromere protein F was significantly increased in infliximab-responders (127). In patients with early RA, infliximab < 0.2 μg/mL, and ADAs development were associated with treatment failure and were more common in females (112). TNF level in the intimal lining layer and synovial sublining and number of macrophages, macrophage subsets and T cells were significantly higher in responders than in nonresponders (128). Infliximab responders had a higher number of CD4+CD25+ T cells than nonresponders at baseline (129).
For the gene polymorphism, a series of studies showed that RA patients carrying TNF-α rs1800629 -GG genotype were better responders to infliximab, while the presence of A allele significantly decreases the response to infliximab (130–133). TNF receptor superfamily member 1B (TNFRSF1B, codes TNF receptors 2 (TNFR2)) rs1061622-GG or -TG was related to a lower responsiveness to infliximab (134, 135), while -TT genotype of the TNFRSF1B rs1061622 was a predictor of good response to infliximab (136, 137). A possible explanation was that the rs1061622 T>G induced an amino acid substitution at codon 196 (M196R), which located in the fourth cysteine-rich domain of the extracellular region of TNFR2. The R allele elicited a high inflammatory response via the TNF- pathway, which could explain the poor response to anti-TNF medication (134). Studies also confirmed that patients with TNFRSF1B rs3397-CC and TNFRSF1B rs1061631-AA genotypes had an increased risk for nonresponse to infliximab (135). TNF receptor superfamily member 1A (TNFRSF1A, codes TNF receptors 1 (TNFR1)) rs767455-AA genotype was associated with a worse EULAR response than -AG or -GG genotype (138). RA patients with homozygous rs396991 polymorphism (V158F) in FCGR3A had good response to infliximab (139) (Table 2).
In a cohort study, in the context of methotrexate, 95 consecutive patients with RA who were first treated with infliximab were switched to etanercept due to a lack of response (either primary, secondary, or with toxicity). Significant DAS28 reductions and ACR response were reported in the overall cohort and nonresponse subtype groups after 12 weeks of medication. Sixty-one percent of the group received a moderate or good EULAR score, confirming that etanercept was successful in patients who did not respond to infliximab (180). In a 12-week, multicenter, open-label clinical study involving 6610 difficult-to-treat patients using DMARDs (including 11% infliximab), results showed that adalimumab alone or in combination with standard DMARDs was effective to improving ACR20 response and EULAR response (181). In a 12-week, double-blind period of the phase IIIb trial, 1063 DMARDs nonresponders (37.6% had previous TNF inhibitor use including infliximab) were randomized to certolizumab pegol or placebo. Certolizumab pegol was linked to faster and more consistent clinical responses as well as increased physical function (182). In a prospective, 12-week, open label, single-arm, observational trial, 25 patients were enrolled, 18 of whom had stopped taking infliximab due to inefficacy, and 22 who had completed 12 weeks of switching etanercept medication. After 12 weeks, 64% of patients had an ACR20 response (183).
Etanercept: Mechanism, Biomarkers and Alternative Therapy
Etanercept was originally developed for treating sepsis but failed in clinical trials. It was then tested for treating RA (184). Etanercept was approved by FDA in 1998 (185), one year ahead of infliximab. Etanercept is a soluble fusion protein consisting of two human 75 kD TNFR 2, each linked to an Fc portion of human IgG1 (186). Functioning as a decoy receptor, etanercept binds to both TNF-α and TNF-β with much greater affinity than endogenous soluble TNFRs, which is unique from other TNF inhibitors that are variants of anti-TNF antibodies (187). TNF inhibition with etanercept modifies various physiologic responses caused or regulated by TNF, including the expression of adhesion molecules involved in leukocyte migration, serum levels of cytokines (e.g., IL-6), and serum levels of MMP-3 (188–190). Only approximately 40% patients achieved an ACR50 response when treated by etanercept monotherapy (191, 192).
ADAs against etanercept were not consistently detected (193, 194) and had no relationship with reduced clinical response (195, 196), while low etanercept level was associated with nonresponse (197).This could be explained by the following two reasons. Firstly, etanercept formed smaller immune complexes compared to infliximab when bound to TNF, which might reduce uptake by antigen-presenting cells (198). Secondly, only the fusion part of the etanercept protein contained foreign epitopes while the TNF binding area did not, which led to low immunogenicity (197). Same as infliximab, TNF-α rs1800629-GG was associated with better response to etanercept than -AA or -AG (140, 141). TNF-α rs1799724-TT or -CT were associated with better response than -CC (199). TNF-α rs1799724-TT showed better response than C allele carriers (138). TNFRSF1A rs767455-AA was associated with better response than -GG (138); TNFRSF1B rs1061622-TT was associated with better response to etanercept (136, 137). A combination of alleles (TNF-α rs1800629-GG and IL-10 rs1800896-GG) was associated with good response to etanercept (144). Other polymorphisms were also contributory. Several studies have confirmed that IL-6 rs1800795-GG was associated with better response than -GC or -CC (146–148). Patients with the combination of IL-6 rs1800795-GG and TNF-α rs1800629-GG genotype were more frequent among the responders compared to those with other combined genotypes. Patients with low TNF- and IL-6 production were the best responders to etanercept therapy (146). Nucleotide-binding protein-like (NUBPL) rs2378945 minor allele (A) had a significant association with poor response to etanercept (142). The IL-10 promoter microsatellite allele IL10.R3 and the haplotype R3-G9 were considerably more prevalent in patients who responded well to etanercept, whereas IL10.G13 was more common in patients who responded moderately or no response (143).
A combination of C allele in codon 25 of the transforming growth factor beta 1 (TGF-β1) gene and the A2 allele in intron 2 of the interleukin 1 receptor antagonist (IL-1RN, codes IL-1Ra) gene, were associated with nonresponse to etanercept (144). On one hand, TGF-1 has been shown to suppress T-cell functions such as proliferation and differentiation of cytotoxic T-cells and T-helper cells (200). The homozygous genotype CC, whether at codon 10 or codon 25, was highly related with reduced TGF-1 production (201). On the other hand, patients carrying the IL-1RN A2 allele had increased production of IL-1 and possibly decreased IL-1Ra (202–204). It was the above two reasons that contributed to nonresponse to etanercept. In addition, 4 CpG within exon 7 of LDL receptor related protein associated protein 1 (LRPAP1) were observed to be more methylated in nonresponders (145). LRPAP1 is a receptor for TGF-β1 (205). We speculated that LRPAP1 methylation blocked the function of TGF-β1 and then induced etanercept nonresponse. Thus, DNA methylation inhibitor might be helpful for such patients (206). Moreover, higher expression of CD84 was associated with better response to etanercept (149). Increased isoleucine, leucine, valine, alanine, glutamine, tyrosine, glucose and 3-hydroxybutyrate levels were associated with good response to etanercept (150). Higher baseline serum CRP, IL-1β and IL-17A were associated with better response to etanercept (151) (Table 2).
In an open-label, single-blind clinical trial, 28 patients with an inadequate response to etanercept were randomized 1:1 to receive infliximab, or to continue etanercept, with background methotrexate treatment. At week 16, 62% of infliximab-treated patients had ACR20 responses, compared to 29% of etanercept-treated patients (207). A multicenter, randomized, double-blind, placebo-controlled, phase III trial enrolled 461 patients with nonresponse to TNF inhibitors (including etanercept). Patients with continued background csDMARDs treatment were assigned in a 1:1:1 ratio to receive subcutaneous injections of placebo, 50 mg golimumab, or 100 mg golimumab. 140 patients achieved ACR20 at week 14, including 18% patients on placebo, 35% patients on 50 mg golimumab, and 38% patients on 100 mg golimumab, suggesting golimumab was a good choice for patients who had previously received one or more TNF-α inhibitors (208). These results were confirmed in a long-term extension, multicenter, randomized, double-blind, placebo-controlled, phase 3 GO-AFTER study with up to five years of therapy (209). Another trial included 18 RA patients who were first treated with etanercept and subsequently switched to infliximab due to inefficacy. The mean best DAS28 after switching to infliximab was considerably better than the previous result, indicating that a trial of infliximab was reasonable for such patients (210).
Adalimumab: Mechanism, Biomarkers and Alternative Therapy
Adalimumab, approved by FDA in 2002, is the first fully human, high-affinity, recombinant IgG1 anti-TNF monoclonal antibody (211). It has high selectivity and affinity for TNF, a low degree of immunogenicity, and a half-life comparable to that of IgG1, allowing every-other-week dosing for patients (211). Adalimumab exerts its therapeutic effects by blocking the interaction of TNF with the p55 and p75 cell surface TNFR (211). By blocking TNF signaling, MMP-1 and MMP-3 are downregulated and osteoclast maturation and activation are inhibited (190, 212). Only approximately 40% of RA patients showed ACR50 response after receiving adalimumab monotherapy (213).
ADAs rate of adalimumab was 28% and RA patients carrying the same IgG allotype as present on the adalimumab IgG had a high frequency of ADAs (153, 154), suggesting that these patients might not gain substantial clinical benefit from adalimumab treatment. In addition, a frequency of < 9.4% of signal regulatory protein (SIRP)α/β-expressing memory B cells predicted RA patients that would develop ADAs, and consequentially failed to respond to treatment (156). It was postulated that evaluating the percentage of SIRP/-expressing memory B cells in patients prior to adalimumab treatment could be a valuable biomarker for identifying a subset of active RA patients who will develop ADAs and develop nonresponse to adalimumab (156). Interestingly, there was no functional data showing the role of SIRP in B cells, while SIRPα was reported to be a critical regulator of myeloid cell activation via binding to CD47 and SIRPα/CD47 axis limited the efficacy of tumor-opsonising antibodies (214). Thus, it is necessary to explore the underlying mechanism involving SIRPα/β-expressing memory B cells in ADAs response of adalimumab. In addition to SIRPα/β-expressing memory B cells, it was suggested that the existence of other specific risk factors, genetic or environmental, predisposed some individuals to develop adalimumab ADAs (156). For example, smoking could predict ADAs development (215) and RA patients with over 1 year disease duration or with an initial DAS28 over 3.2 have a higher risk of ADAs positivity (215). In addition to ADAs, the status of anti-citrullinated protein/peptide antibody (ACPA) could affect the efficacy of adalimumab. Finer ACPA specificities in ACPA-negative might be predictive of response to treatment (adalimumab or methotrexate) (152) and adalimumab was more effective in patients who were ACPA positive than in those who were ACPA negative at baseline (155). However, another study reported a contradictory result that the presence of ACPA was associated with a reduced response to TNF inhibitors including adalimumab (158). Decreased CD68 and MMP-3 expression in the synovium was associated with a good response to adalimumab (157). RA patients with response to adalimumab had significantly lower chemokine receptor 6 (CCR6) expression and increased Th17 and Th1 (159). Elevated baseline levels of chemokine (C-X-C motif) ligand (CXCL)10 and CXCL13 were associated with favorable response to adalimumab (162). Increased expression of CD11c was correlated with a good response to adalimumab (163). Higher myeloid-related protein (MRP)8/14 levels predicted good adalimumab response (164). Elevated baseline neutrophil-to-lymphocyte ratio (NLR) and platelet-to-lymphocyte ratio (PLR) were associated with a higher risk of nonresponse to adalimumab (160). RA patients with high soluble intercellular adhesion molecule 1 (sICAM1) and low CXCL13 had a good clinical response to adalimumab (165) (Table 2).
Polymorphisms of TNF and TNFR also influenced response to adalimumab. A study showed that TNF-α rs1800629-GG was associated with a better response to adalimumab than -GA or -AA (166). In contrast, another study reported that TNF-α rs1800629 G haplotype in a homozygous form was associated with a lower response (161). Two meta-analyses failed to demonstrate that the rs1800629 G/A genotype, whether heterozygous or homozygous, is linked to a poor response to anti-TNF medication treatment (216, 217). In TNF-α rs1800629-GG patients, ACPA status did not affect the clinical response to adalimumab (218). TNF-α rs1799724-TT showed a better response than C allele carriers with adalimumab treatment (138). TNFR1 36 (rs767455) -AA was associated with a better response to adalimumab than -GG (138); RA patients with TNFR2 676 (rs1061622) -TT demonstrated a better response compared to those with -TG (137). Other polymorphisms were also studied. When RA patients treated with adalimumab, low-affinity Fc gamma receptors 2A (FCGR2A)-R(A)* allele shows a better EULAR good response (167), IL-6 rs1800795-GG was associated with a better response than -GC or -CC (168).
In a 52-week, double-blind, placebo-controlled, active-controlled phase III study, 1305 patients were randomized 3:3:2 to placebo, baricitinib (a JAK inhibitor) or adalimumab. At week 16, adalimumab nonresponders received rescue treatment with baricitinib. Results showed that switching from adalimumab to baricitinib was associated with improvements in disease management, physical function, and pain (219). In a 24-weeks Single-Arm study, 90 patients discontinued prior adalimumab treatment and continued methotrexate combined with etanercept for 24 weeks. ACR response data demonstrated that switching to etanercept was a therapeutic option in patients with RA who failed adalimumab treatment. ADAs response was examined to explain the treatment failure in this study. It was shown that patients with nonresponse to adalimumab produced higher anti-adalimumab antibodies, which did not cross-react with etanercept and provided additional support for switching to etanercept (220). In a 48-week, randomized, double-blind, SELECT-COMPARE study, 1629 patients were grouped 2:2:1 to upadacitinib (a JAK inhibitor), placebo or adalimumab, with stable background methotrexate. Upadacitinib in combination with methotrexate demonstrated superior clinical and functional responses versus adalimumab combined with methotrexate (221). Patients who did not respond well to adalimumab saw clinically significant improvements after switching to upadacitinib (221).
Certolizumab Pegol: Mechanism, Biomarkers and Alternative Therapy
Certolizumab pegol, approved by FDA in 2009, is an antigen-binding fragment (Fab) of a recombinant humanized monoclonal antibody conjugated to PEG. PEGylation enables the increase of the plasma half-life and solubility and reduces the immunogenicity and protease sensitivity (222). Certolizumab pegol binds to TNF with greater affinity and is more effective than adalimumab and infliximab at neutralizing soluble TNF-mediated signaling, but has equal or lesser potency than etanercept (223). Certolizumab pegol may be more effective in penetrating inflamed arthritic tissue than other anti-TNF medications and it cannot be actively transported through the placenta during pregnancy (222). ACR20 and ACR50 response in RA patients was only about 45% and 23% after 6-month treatment of certolizumab pegol, respectively (224).
Like other biologic agents, certolizumab pegol elicited immunogenic response, resulting in the formation of ADAs with a high incidence of about 65% (169). Further research showed that >97% of ADAs to certolizumab pegol was directed to the paratope of the drug and were thus neutralizing, indicating these patients with neutralizing ADAs had especially higher risk for drug nonresponse (225). However, a recent study advocated not to overvalue ADAs in a clinical setting, unless certolizumab pegol concentration was low, as they found that the drug concentration but not the presence of ADAs was highly correlated with the capacity to neutralize TNF (169). Clinimetric measurements were found to be associated with nonresponse to certolizumab pegol during course of treatment. Early response to certolizumab pegol predicted long-term outcomes (170). Failure to achieve improvement in DAS28 within the first 3 months of therapy was predictive of a low probability of achieving low disease activity at 12 months using certolizumab pegol (171). Failure to achieve improvements in DAS28(ESR), SJC or CDAI within the first 3 months of certolizumab pegol therapy was associated with a low chance of achieving low disease activity at 7 months (172). CDAI nonresponse at 3 months was a predictor of failure to achieve the therapeutic target of low disease activity at 12 months in patients with RA initiating treatment with certolizumab pegol (173). Studies observed that RA patients who had high serum pretreatment ratio of type I IFNβ/α (> 1.3) or undetectable type I IFN were likely to have EULAR nonresponse to TNF inhibitors (including certolizumab pegol) (174, 175). Mechanically, the pattern of gene expression that differed between the response and nonresponse groups suggested that canonical type I IFN pathway signaling via JAK/STAT was increased in peripheral blood classical monocytes of RA patients who were likely to respond to TNF inhibition, whereas JAK/STAT-independent non-canonical IFNβ-IFNAR1 signaling was increased in nonclassical monocytes of those who were not likely to respond to TNF inhibition (175, 226). Notably, JAK1 expression was absent in both classical and nonclassical monocytes from the patients with undetectable IFN or IFNβ/α > 1.3, suggesting JAK1 could be a predictive factor for nonresponders to TNF inhibitors (175) (Table 2).
In a 104-week, randomized, single-blind (double-blind until week 12 and investigator blind thereafter), parallel-group, head-to-head superiority study, 457 RA patients were treated by certolizumab pegol plus methotrexate or adalimumab plus methotrexate. At week 12, 65 nonresponders to certolizumab pegol were switched to adalimumab and 57 non-responders to adalimumab were switched to certolizumab pegol. Certolizumab pegol plus methotrexate was not found to be superior to adalimumab plus methotrexate. For patients with primary therapy failure, clinical benefit could be observed after drug switching in both groups (227). In a 2-year, phase 2a, double-blind, proof-of-concept study, 52 RA patients with inadequate response to certolizumab pegol received certolizumab pegol plus bimekizumab (a monoclonal IgG1 antibody that selectively inhibits IL-17A and IL-17F). Data showed that reduction of DAS28 was greater in the group treated with bimekizumab in combination with certolizumab pegol compared with the group treated with certolizumab pegol plus placebo after 20-week treatment (228). This suggested that the add-on therapy of bimekizumab was of great clinical significance for nonresponsive patients to certolizumab pegol.
Golimumab: Mechanism, Biomarkers and Alternative Therapy
Golimumab, a fully human IgG1κ monoclonal antibody with directed against the soluble and membrane bound forms of TNF-α, was the latest TNF inhibitor approved by the FDA in 2009 (229, 230). As a newer, second-generation TNF inhibitor, the clinical experience of golimumab was less in comparison with the older ones such as infliximab, etanercept and adalimumab (230). Different with other TNF inhibitors, golimumab has a specific mode of action: it binds to a distinct epitope on TNF-α that does not overlap with the binding residues of TNFR2, but the complex sterically hinders TNFR2 as well as TNFR1 from associating with TNF-α (231). In combination with methotrexate, golimumab has a UK marketing authorization for RA therapy (232), which may in part be attributable to the concomitant use of methotrexate reduces the clearance of golimumab by approximately 35% (230). A GO-BEFORE trial enrolling 637 RA patients and a GO-FORWARD trial enrolling 444 RA patients demonstrated that golimumab achieved ACR50 response in approximately 40-50% of patients (233, 234).
Regarding immunogenicity of golimumab, complementarity determining region loop grafting was developed to reduce some of the immunogenic issues associated with chimeric antibodies (235). A study showed that only 6.5% of golimumab-treated patients developed ADAs (236). However, other studies detected 31.7% of ADAs for golimumab using a more sensitive method (237). Some patients showed good response to golimumab even with a presence of ADAs and the numbers of ADAs-positive patients were insufficient to determine whether these ADAs are associated with an impaired clinical response (196). Golimumab concentration ≥ 1.0 mg/L was associated with improved treatment response (176). Larger magnitudes of the decrease in serum amyloid A (SAA), E-selectin and MMP-9 were observed in responders to golimumab plus methotrexate relative to nonresponders (178). Greater likelihood of low disease activity and remission were associated with being male, younger age, lower health assessment questionnaire, ESR (or CRP) and TJC (or SJC) scores and absence of comorbidities in golimumab-treated RA patients (179). Sustained increase of markers including IL-6, CRP, IL-2 receptor alpha chain, and MMP-1, was presented in golimumab-inadequate responders (177) (Table 2).
Currently, alternative therapies for golimumab are poorly studied. In a latest study, according to real-life data extracted from 11 Austrian social health insurance funds covering 86% of the Austrian population, 7637 RA patients on bDMARD therapy were retrieved in total. Golimumab was prescribed in 15% RA patients. After golimumab failure, patients were often switched to an IL-6R antagonist tocilizumab and efficacy was waiting to be determined (238).
bDMARDs Blocking T Cells, CD20 and IL-6R
Abatacept: Mechanism, Biomarkers and Alternative Therapy
Beyond TNF, CD28 signaling play a key role in T cell process and RA development (239). According to ACR guideline, RA patients with their first TNF Inhibitor failure could switch to abatacept (240). Abatacept, which was approved by the FDA in 2005, is a soluble, recombinant, fully humanized fusion protein that consists of the extracellular domain of cytotoxic T-lymphocyte antigen 4 (CTLA-4) and the Fc portion of IgG1 that has been modified to reduce the Fc region’s capacity to induce antibody-dependent and complement-dependent cytotoxicity (241). Abatacept is the first biological drug to primarily target T-cell activation in RA. Abatacept works therapeutically by binding to the costimulatory molecules CD80 and CD86 on antigen-presenting cells (APCs), preventing them from interacting with CD28 on T cells (241). Abatacept also functions through regulating macrophages (242, 243), monocytes (244) and B cells (245–248). Abatacept significantly decreases expression of IFN-γ, IL-1β, MMP-1 and MMP-3 (247). There are two approved formulations for abatacept, intravenous and subcutaneous, which have similar efficacy and safety profile (249). Immunogenicity for abatacept is low and transient, and do not interfere with clinical response (250). Abatacept can be used in conjunction with csDMARDs or as a monotherapy. However, because of an increased risk of infections and malignancies without a significant improvement in efficacy, concurrent treatment with abatacept and other bDMARDs is not indicated (251). In two phase III trials, patients treated with abatacept achieve ACR20 at 66% (252) and 50% (253), respectively.
Rheumatoid factor (RF) was the first autoantibody to be discovered in RA patients (254). A pooled study of data from 9 observational RA registries in Europe found that RF positivity was related with improved abatacept medication efficacy (255). However, A meta-analysis of clinical trials found that no association was found between abatacept response and RF (256). Recently, RF seropositivity could predict increased abatacept retention and abatacept showed preferential efficacy in patients with high-titer RF (257, 258). ACPA/anti-cyclic citrullinated peptides (anti-CCP, a surrogate for ACPA) (259), added to the 2010 ACR/EULAR diagnostic criteria (260), is a hallmark of RA and plays a role in disease pathogenesis (261). A retrospective observational cohort study found there was significantly higher clinical response and drug retention rate in ACPA-positive patients treated with abatacept (262). In an AMPLE trial, abatacept was more effective in patients who were positive for anti-CCP than those who were negative for anti-CCP at baseline (155). Data from the AVERT trial showed that abatacept patients who were anti-CCP IgM positive at baseline had stronger clinical effectiveness than those who were anti-CCP IgM negative at baseline (263). Patients in clinical trials are often a selected population that may not reflect the diverse population observed in ordinary practice settings. As a result, more real-world data are needed to investigate the relationship between anti-CCP status and abatacept therapy effects. According to real-world data from US clinical practices, better clinical response was observed in anti-CCP positive patients (264), which was consistent with a real-world ACTION study reporting that anti-CCP positive status was associated with greater efficacy of abatacept than seronegative status (257). The real-world ACTION study also discovered that double ACPA/RF positive led in increased abatacept retention rates (265). A major limitation still exists in these studies, i.e., they categorized patients according to ACPA/anti-CCP status (e.g., positive vs. negative) rather than titers. Most recently, RA patients treated with abatacept were classified based on ACPA/anti-CCP titers. Results showed that clinical effect of abatacept was most pronounced in patients with high-titer ACPA (258). However, this seems to be contradicting with other two studies, which found that sustained response to abatacept was associated with an early reduction in ACPA titers (266), and abatacept was more effective in patients who showed decreasing anti-CCP antibody titers during treatment (267). Taken together, although ACPA/anti-CCP has been used as a biomarker of disease progression in RA patients for decades, its exact relationship with abatacept response still needs to be explored.
Furthermore, in RA, a large baseline number of circulating CD28 negative T cells may indicate nonresponse to abatacept (268). RNA elongation, apoptosis-related, and NK cell-specifically expressed genes were upregulated in abatacept nonresponders, while inflammasome genes were upregulated in infliximab nonresponders and B cell-specifically expressed genes were downregulated in tocilizumab nonresponders (269). When RA patients with CTLA-4 rs5742909 T or CTLA-4 rs231775 G polymorphisms received abatacept, they had a greater EULAR response and lower disease activity (270). By metabolomic analysis, low level of 3-aminobutyric acid and high levels of quinic acid and citrate were observed in responders to abatacept treatment (271). Serum CXCL10 level was associated with better response to abatacept (272). A higher level of CD24-high and CD27 positive regulatory B cells at baseline was associated with DAS28 remission and a good EULAR response in abatacept-treated patients (273). Reduced type I IFN score, and higher expression of dendritic cells-related genes (Basic Leucine Zipper ATF-Like Transcription Factor 2 (BATF), Lysosomal Associated Membrane Protein 3 (LAMP-3), CD83, C-type Lectin Domain Family 4 Member A (CLEC4A), Indoleamine 2, 3-dioxygenase 1 (IDO), interferon regulatory factor (IRF)7, STAT1, STAT2 and TNF Superfamily Member 10 (TNFSF10)) could be used as biomarkers to predict good response to abatacept (274). Increased dickkopf (Dkk)-1 serum level and sclerostin might indicate a poor prognosis and resistance to abatacept treatment in RA patients (275). Increased cartilage oligomeric matrix protein level served as a strong predictive biomarker for inadequate response to abatacept treatment for RA patients with a first TNF inhibitor failure (276) (Table 3).
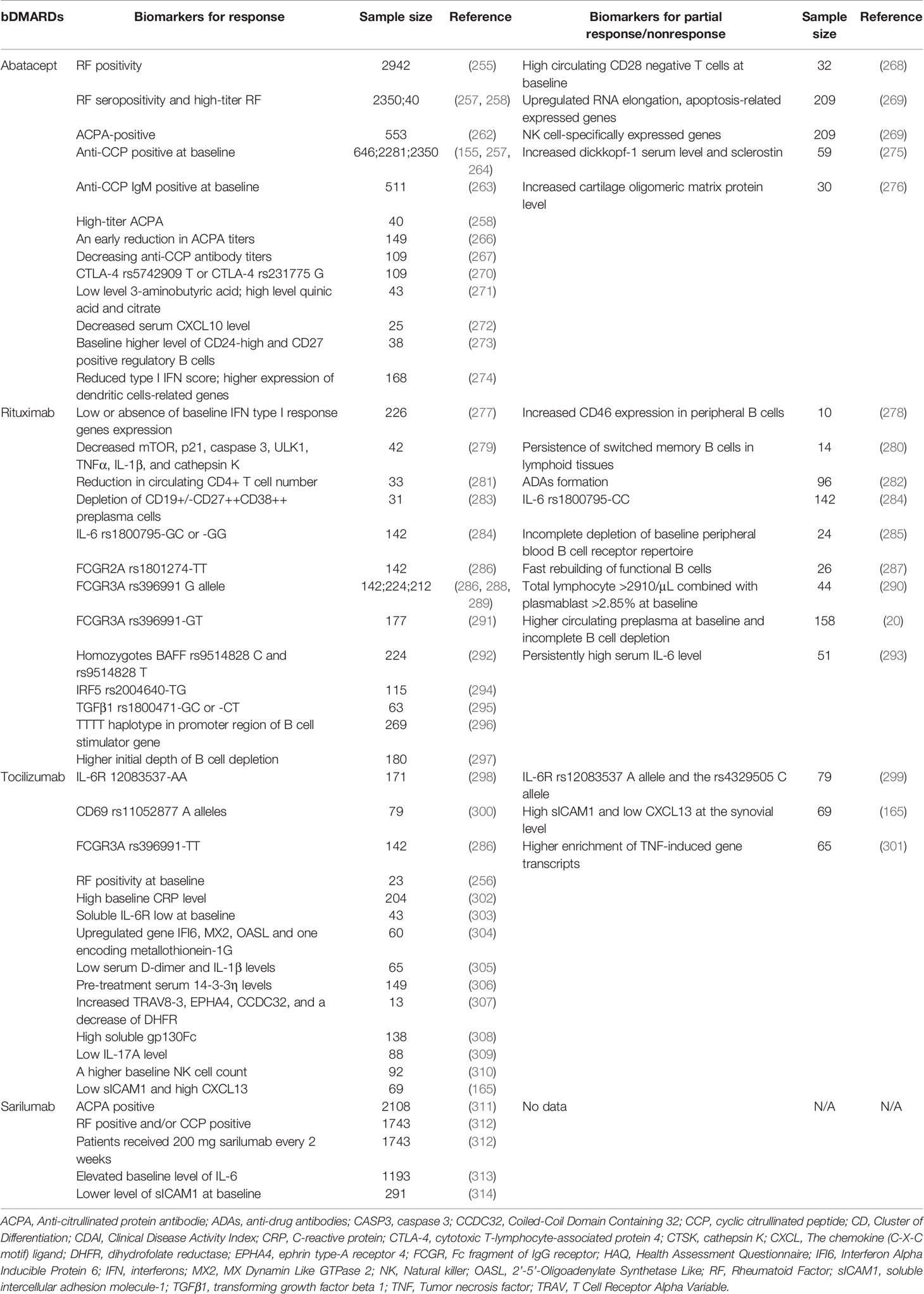
Table 3 Potential biomarkers for response or partial response/nonresponse to bDMARDs blocking T cells, CD20 and IL-6R.
In a multicenter, retrospective study, RA patients initially treated with abatacept (n = 76, most of them discontinued abatacept due to lack of effectiveness) were switched to either TNF inhibitors (adalimumab, certolizumab pegol, etanercept, golimumab, infliximab) or tocilizumab. Drug retention was estimated after 24 months. Switching to tocilizumab resulted in higher retention due to efficacy, although total retention was comparable when compared to TNF inhibitors (315). In a retrospective cohort study involving 550 RA patients treated with abatacept, 25 inadequate responders underwent an add-on macrolide calcineurin inhibitor tacrolimus therapy. At week 24, 40.0% of patients achieved low disease activity or remission, and the EULAR moderate or good response was 72.0% (316).
Rituximab: Mechanism, Biomarkers and Alternative Therapy
B cells play a critical role of in pathogenesis of RA (317). In 2001, a pilot trial evaluating B cell depletive therapy in RA patients was successfully performed (318). Rituximab, which was approved by the FDA in 2006, is a chimeric mouse/human monoclonal antibody that targets the transmembrane protein CD20 molecule on the surfaces of B cells, causing apoptosis through antibody- and complement-dependent cytotoxicity (319). Rituximab monotherapy and/or in combination with methotrexate is recommended as a treatment option for RA patients who have inadequate response to TNF inhibitors and thus serves as a second-line bDMARD (320, 321). Although no fetus damage has been reported in pregnancies exposed to rituximab within 6 months, it should be considered only when no other therapeutic option is available (322). Only approximately 50% of patients achieved ACR20 response after rituximab treatment (323).
Normal cells are resistant to the complement-mediated lysis through complement regulatory proteins (CRPs), including CD55, CD59, CD46 and CD35 (324). A study showed that increased CD46 expression in peripheral B cells, but not CD35, seemed to be able to predict nonresponders (278). CD46 reduced complement-mediated lysis, one of the mechanisms of action of rituximab, thus decreasing the effectiveness of rituximab (278). It is possible that CD46 inhibitor monotherapy or combined with rituximab could be an alternative strategy for nonresponders to rituximab. Another study demonstrated that depleting CD46 from the cell surface by Ad35K++ sensitized complement-dependent cytotoxicity triggered by rituximab in CD20-positive B-cell malignancies (325, 326). However, effects of CD46 inhibition have not been validated in RA patients who do not respond to rituximab. Roles of CD55 and CD59 were also investigated in RA patients. There was no correlation between the expression levels of CD55 or CD59 at baseline or after treatment and the frequencies of B cell subsets after rituximab treatment or the extent of B cell depletion (280). Apart from these studies, persistence of switched memory B cells in lymphoid tissues was related to rituximab nonresponse (280). Structurally like infliximab, about 11% of rituximab-treated patients developed ADAs, which might influence treatment efficacy and tolerability of rituximab (282). Low or absence of baseline IFN type I response genes expression was associated with good response to rituximab (277). Decreased in expression of mammalian target of rapamycin (mTOR), p21, caspase 3, unc-51 like autophagy activating kinase 1 (ULK1), TNFα, IL-1β, and cathepsin K was predictive of better rituximab response (279). A significant reduction in circulating CD4+ T cell number was observed in RA patients with good response to rituximab (281). Depletion of CD19+/-CD27++CD38++ preplasma cells could be a predictor of good response (283).
Polymorphisms related to rituximab therapy have been well studied. The IL-6 rs1800795-CC served as a predictor of nonresponse to rituximab in RA patients, while patients with -GC or -GG was more susceptible to rituximab (284). There was a significant correlation between this homozygosis polymorphism in the promoter region with a higher IL-6 expression level (327). It was rational that IL-6R inhibitor tocilizumab could be used as a companion to rituximab treatment in nonresponders (293, 328). FCGR2A polymorphism rs1801274-TT was associated with better response to rituximab (286). Several studies suggested that FCGR3A rs396991 genotypes, either in heterozygous or homozygous conditions, were associated with different response rates to rituximab. FCGR3A rs396991 G allele were associated with better response to rituximab (286, 288, 289). Paradoxically, patients with rs396991 -GT showed the highest response rate, when compared to patients with rs396991-TT or rs396991-GG (291). Homozygous carriers of the B-cell activating factor belonging to the TNF family (BAFF) rs9514828 C served as a better response marker to rituximab as well as the homozygotes BAFF rs9514828 T (292). IRF5 rs2004640-TG (294), TGFβ1 rs1800471-GC or -CT (295) was related to good response to rituximab. The TTTT haplotype in promoter region of B cell stimulator gene was associated with good response to rituximab therapy in RF and/or ACPA seropositive RA patients (296).
A series of studies focused on the relationships between rituximab efficacy and B cells. Incomplete depletion of baseline peripheral blood B cell receptor repertoire might predict clinical nonresponse (285). The fast rebuilding of functional B cells might be present in rituximab nonresponders (287). Total lymphocyte counts >2910/μL combined with plasmablast frequency >2.85% at baseline predicted rituximab nonresponse (290). Higher initial depth of B cell depletion was associated with good response to rituximab (297). Patients with RA who did not respond to an initial cycle of rituximab had larger circulating preplasma cell counts and incomplete B cell depletion, whereas an extra cycle of rituximab delivered prior to total B cell repopulation improved B cell depletion and clinical response (20). However, another study found that despite adequate B cell depletion, failed rituximab therapy still existed in some RA patients, and nonresponse to rituximab was associated with persistently high serum IL-6 level (293). Further, in a single-center, prospective, observational database, 51 RA patients who had discontinued rituximab therapy owing to inefficacy received either a T cell costimulation inhibitor abatacept or IL-6R inhibitor tocilizumab. After 6-month treatment, reduction of disease activity score (DAS28-ESR) and swollen joint count was more significant in tocilizumab-treated patients than in abatacept-treated patients, suggesting that IL-6-directed therapy might be a more logical and effective treatment choice than T cell costimulation blockade in RA patients with failed rituximab therapy (293). Consistently, in an investigator-led, industry-supported, prospective, longitudinal, multinational CERERRA database, 265 RA patients were analyzed and majority of them (78%) had stopped rituximab owing to ineffectiveness (328). 90 patients were prescribed abatacept, 86 were started on tocilizumab and the remaining 89 patients received TNF inhibitors (including etanercept, adalimumab, infliximab, certolizumab pegol and golimumab). After 6-month treatment, patients treated with tocilizumab had a greater decline of DAS28-ESR and better EULAR response than patients treated with TNF inhibitors or abatacept (328) (Table 3).
Tocilizumab: Mechanism, Biomarkers and Alternative Therapy
IL-6 is one of the most abundant pro-inflammatory cytokines in RA. It can signal through two distinct mechanisms. In the cis-signaling, IL-6 binds to its membrane IL-6R which is mainly expressed in hepatocytes and hematopoietic cells (T cells, monocytes/macrophages, B cells and neutrophils). In the trans-signaling, IL-6 binds to its soluble IL-6R. The complex consisting of IL-6 and membrane and soluble IL-6R associates with gp130, resulting in the activation of downstream signaling events via JAK/STAT (329). The option to target IL-6R rather than IL-6 itself was chosen after considering that receptor concentrations have less interpatient variability than IL-6 concentrations, potentially simplifying dose and regimen selection (330). Approved by FDA in 2010, tocilizumab is the first anti-IL-6Rα humanized IgG1/kappa monoclonal antibody, used for the treatment of moderate to severe RA (331). Tocilizumab targets and neutralizes both soluble and membrane-bound IL-6R, resulting in inhibition of IL-6-mediated inflammatory activities (332). Tocilizumab can be either applied in combination with methotrexate or used as a monotherapy (330, 333, 334). Compared with TNF inhibitors, tocilizumab monotherapy improves the healing of focal bone erosions in RA patients and outperforms methotrexate or other csDMARDs in terms of lowering RA symptoms (330). Tocilizumab treated patients achieved an approximately 50% ACR20 response rate (335, 336).
Tocilizumab-subcutaneous and -intravenous treatment had a low immunogenicity risk, whether used alone or in combination with csDMARDs (337). The development of ADAs in a small fraction of patients had no noticeable impact on the efficacy of tocilizumab (337). Several studies investigated whether polymorphisms of genes were associated with response to tocilizumab therapy. A study with 79 RA patients enrolled reported that the major allele (A) of rs12083537 and the minor allele (C) of rs4329505 with IL-6R were associated with poor tocilizumab response (299). But another study with 171 RA patients enrolled found that 12083537-AA could predict better EULAR response to tocilizumab (298). Further, a larger cohort of 927 patients demonstrated no association between them (338). A genome-wide association analysis implicated the involvement of 8 loci (CD69 rs11052877; GalNAc-T-Like Protein 4 (GALNTL4) rs4910008; Ecto-NOX Disulfide-Thiol Exchanger 1 (ENOX1) rs9594987, rs10108210 and rs703297; Potassium Voltage-Gated Channel Interacting Protein 1 (KCNIP1) rs703505; C-Type Lectin Domain Family 2 Member D (CLEC2D) rs1560011; Solute Carrier Family 9 Member A7 (SLC9A7) rs7055107) with response to tocilizumab (339). Relationship between CD69 rs11052877 A alleles and good tocilizumab response was further validated in a study with 79 RA patients enrolled (300). In contrast, another study concluded that CD69 rs11052877 A/G genetic polymorphism was not useful as a predictor of tocilizumab response in RA patients (340). Data from 87 patients suggested that FCGR3A rs396991-TT could be used to predict better EULAR response (286). However, no relationship between rs396991 and EULAR response was shown in a research enrolling 171 RA patients (298). Due to the small sample sizes and/or conflicting findings, larger studies are necessary to resolve whether the above genetic variations had real impact on therapeutic response to tocilizumab.
Features of pre-treatment disease activity had been demonstrated to be associated with response to tocilizumab in RA patients. A meta-analysis found that RF positivity at baseline predicted better response to tocilizumab (256). Several studies, however, found no link between RF positive and response (341, 342). A high baseline CRP level could serve as a predictor of better response to tocilizumab (302). Patients with a strong acute phase response, extra-articular symptoms, and a history of DMARDs and biological treatments may be more likely to respond quickly to tocilizumab. However, no parameter was likely to predict reaction if examined separately (343). A significantly higher proportion of patients in soluble IL-6R-low group achieved SDAI remission compared with those in soluble IL-6R-high group (303). Upregulation of gene Interferon Alpha Inducible Protein 6 (IFI6), MX Dynamin Like GTPase 2 (MX2), 2’-5’-Oligoadenylate Synthetase Like (OASL) and one encoding metallothionein-1G in peripheral blood mononuclear cells was observed in tocilizumab good/moderate responders (304). Low blood D-dimer and IL-1 levels at 4 weeks were found to predict favorable treatment response to tocilizumab at 52 weeks in a population of 65 patients (305). In patients treated with tocilizumab, pre-treatment blood 14-3-3 levels predicted 1-year DAS28 remission (306). Patients with increased T Cell Receptor Alpha Variable 8-3 (TRAV8-3), EPH Receptor A4 (EPHA4), Coiled-Coil Domain Containing 32 (CCDC32), and a decrease of DHFR presented good response to tocilizumab (307). High soluble gp130Fc strongly predicted good response to tocilizumab (308). Low IL-17A level was linked to higher response rate of tocilizumab (309). A higher baseline NK cell count was associated with better clinical remission after treatment with tocilizumab (310). A serological cytokine signature showed that patients with high sICAM1 and low CXCL13 at the synovial level was negatively associated with the ACR50 response to tocilizumab, whereas patients with low sICAM1 and high CXCL13 showed good tocilizumab response (165). The presence of more TNF-induced gene transcripts in synovial samples was linked to a poor response to tocilizumab (301) (Table 3).
In a multicenter, retrospective study, 145 RA patients initially treated with tocilizumab (most of them discontinued tocilizumab due to lack of effectiveness) were switched to TNF inhibitors (adalimumab, certolizumab pegol, etanercept, golimumab, infliximab), abatacept or JAK inhibitors. After 24 months, drug retention was estimated. Switching to abatacept in tocilizumab-treated patients led to higher retention (315). In an open-label, non-randomized phase 3 study, 519 RA patients with inadequate response to conventional synthetic DMARDs received tocilizumab. 213 partial responders continued tocilizumab treatment and 27 nonresponders were switched to an CD20 inhibitor rituximab. At week 32, half of early partial responders benefitted from continuing tocilizumab and switching non-responders to rituximab seemed feasible (344). In a retrospective, observational clinical study, 63 nonresponders from 527 RA patients treated with tocilizumab were switched to TNF inhibitors (infliximab, adalimumab, etanercept, golimumab, and certolizumab pegol) or abatacept. The proportion of patients achieving CDAI ≤ 10 at week 24 was significantly higher in patient treated with TNF inhibitors than those treated with abatacept, and the values of the CDAI at week 24 showed the same tendency (345).
Sarilumab: Mechanism, Biomarkers and Alternative Therapy
Sarilumab, a completely human IgG1 monoclonal antibody authorized by the FDA in 2017, specifically binds to soluble and membrane-bound IL-6R and blocks IL-6-mediated cis- and trans-signalling (346). Sarilumab presented good therapeutic efficacy when administered in combination with csDMARD in patients with inadequate response to methotrexate or at least one TNF inhibitor (346). It should be noted that sarilumab was developed in mice engineered to produce human antibodies with an affinity for human IL-6R 20-fold greater than tocilizumab (347). Preclinical findings showed that sarilumab inhibited IL-6 signaling in a dose-dependent manner at a lower concentration than tocilizumab, with no evidence of complement-dependent or antibody-dependent cytotoxicity (348). Sarilumab on the background of methotrexate significantly suppresses CRP level (349), biomarkers of bone resorption (RANKL and RANKL/OPG), bone and cartilage destruction and synovial inflammation (350). Approximately 60% of sarilumab-treated patients achieved an ACR20 response (351, 352).
Currently, only a few studies have been undertaken to identify potential biomarkers that can predict sarilumab response or nonresponse in RA patients, and alternative therapy in sarilumab nonresponders is not reported as sarilumab is approved very recently. In biomarker analysis of two phase III trials (MOBILITY involving RA patients with inadequate response to prior methotrexate and TARGET involving RA patients with inadequate response to prior TNF inhibitors), ADAs response rates were 5.6% (150 sarilumab) and 4.0% (200 mg sarilumab) and neutralizing antibodies were detected at 1.6% and 1.0% (346). Likewise, 7% of RA patients received sarilumab monotherapy in a MONARCH study exhibited an ADAs presentation but no detectable neutralizing antibodies (353). The development of ADAs was not connected with adverse effects or loss of efficacy, although it may have an impact on sarilumab pharmacokinetics (346). A phase III multicenter, randomized, controlled studies indicated that sarilumab might be more effective in RA patients who were ACPA positive (311). Also, better clinical response to sarilumab was consistently observed among patients who were RF positive and/or CCP positive in MOBILITY and TARGET studies (312). Regardless of autoantibody status, there was a more robust response in patients received 200 mg sarilumab every 2 weeks (312). Patients with elevated baseline IL-6 levels were found to have a better response to sarilumab compared to methotrexate or adalimumab than patients with normal IL-6 levels (313). Lower level of sICAM-1 at baseline was predictive of improved response to sarilumab (314) (Table 3).
tsDMARDs Targeting JAKs
Tofacitinib: Mechanism, Biomarkers and Alternative Therapy
Even through development of bDMARDs revolutionizes treatment of RA (103), these bDMARDs bring up new issues, e.g., formation of neutralizing antibodies, biologics-related toxicity and infusion-related adverse effects (354). With discovery more than 20 years ago, JAKs attract much attention since they are the most important signaling transducers (355). When triggered by cytokines such as TNF-α and IL-6, JAKs phosphorylate STATs, causing dimerization and translocation of STATs to the nucleus, where they control inflammation-related genes (7, 356). In other words, JAK inhibition blocks the action of all dependent cytokines (“many birds with one shot”) (357). The ACR and EULAR affirm that JAK inhibitors could be a viable option for RA patients who are refractory to methotrexate monotherapy and viewed on equal footing with TNF inhibitors and non-TNF biologics such as abatacept, tocilizumab and rituximab (13, 240). As the first JAK inhibitor approved by FDA in 2012, tofacitinib preferentially inhibits JAK1 and/or JAK3, and to a lesser extent of JAK2 (358, 359). Tofacitinib reduced JAK1/JAK3-mediated signaling of IL-2, IL-4, IL-6, IL-7, IL-15 and IL-21, as well as IFNα and IFNγ, resulting in the regulation of inflammatory response (360). Tofacitinib also reduced levels of CRP, C-C motif chemokine ligand (CCL)2, CXCL10, CXCL13, MMP-1 and MMP-3 (361). In the background methotrexate, either 5 mg or 10 mg tofacitinib achieved more than 50% ACR20 response in patients with methotrexate inadequate response (359).
High baseline musculoskeletal ultrasound (MSUS) and the multi-biomarker disease activity (MBDA) score could predict tofacitinib nonresponse at week 12 (362). miR-432-5p was significantly downregulated in RA patients who were responsive to tofacitinib therapy (363). Lower levels of IFN-γ, IL-6, IL-17 and higher levels of IL-35 were found in tofacitinib responders than in nonresponders (364). Lower age, CRP, ACPA, and DKK-1 indicated the good treatment effects of tofacitinib therapy on bone mineral density changes (365). MMP-3 had higher pre-treatment values correlating with improved tofacitinib response (361). Clinical improvement with tofacitinib therapy correlated with reductions in STAT1 and STAT3 phosphorylation (361). The available evidence is insufficient to support alternative therapy for tofacitinib because limited clinical trials have been conducted (13) (Table 4).
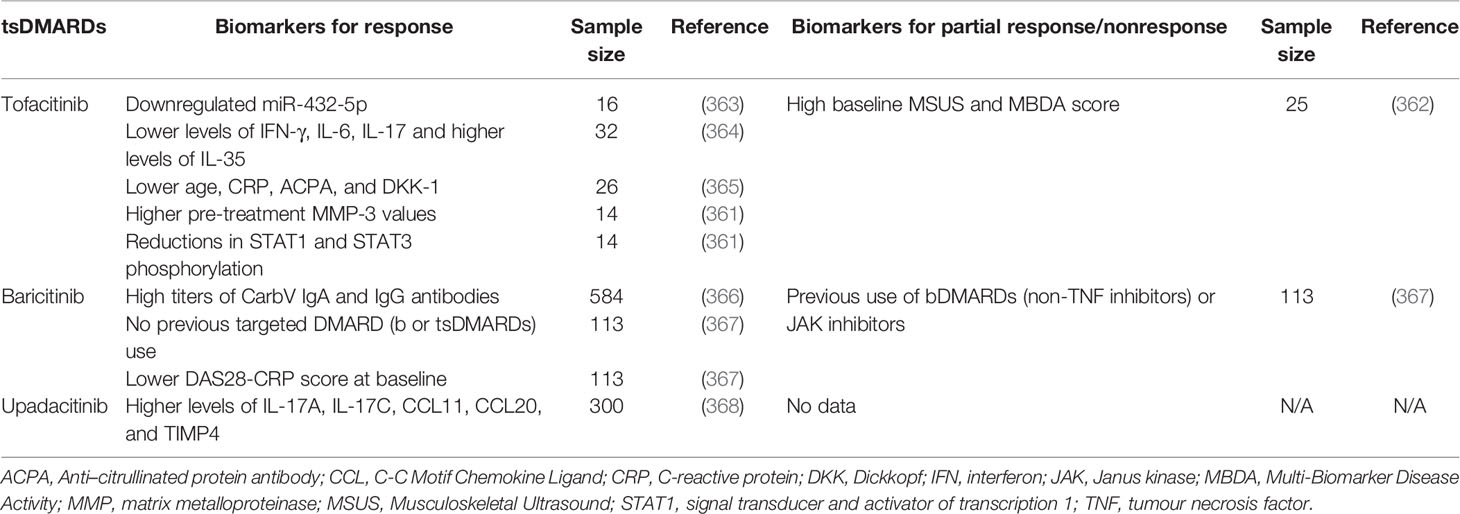
Table 4 Potential biomarkers for response or partial response/nonresponse to tsDMARDs targeting JAKs.
Baricitinib: Mechanism, Biomarkers and Alternative Therapy
Approved by FDA in 2018, baricitinib is the second JAK inhibitor for RA treatment that selectively and reversibly inhibits JAK1 and JAK2 and then modulates JAK-STATs intracellular signaling pathways (369, 370). Baricitinib also inhibits the effects of JAK3, TyK2, tyrosine-protein kinase Met (c-MET) and Checkpoint kinase 2 (Chk2) (371). Baricitinib could reduce pannus formation and bone damage in multiple murine models of arthritis (371), and also present an osteoprotective effect, increasing mineralization in bone-forming cells in phase III studies (372). After treatment, mean serum values of IgG, IgM and IgA were decreased and remained stable below baseline (373). Treatment with baricitinib did not result in increased autoantibody (RF and ACPA) titers (374). Caution is recommended when leflunomide or teriflunomide was co-administered with baricitinib (373). Baricitinib demonstrated a consistent, beneficial treatment effects in bDMARD-refractory patients (375). Baricitinib also ameliorated disease progression in RA patients who were naïve to DMARDs or respond inadequately to csDMARDs, and the beneficial effects were similar to those observed with adalimumab (376). In two clinical trials, RA patients treated with baricitinib achieved ACR20 for 77% and 55%, respectively (377, 378).
High titers of anti-carbamylated vimentin (CarbV) IgA and IgG antibodies were associated with a greater clinical response to baricitinib as measured by SDAI and DAS28-high-sensitivity CRP (366). Patients who had previously used bDMARDs (non-TNF inhibitors) or JAK inhibitors had decreased rates of DAS28-CRP improvement when treated with baricitinib (367). No previous targeted DMARD (b or tsDMARDs) use was associated with the achievement of low disease activity (367). However, this result was contradicted to another study in which baricitinib nonresponse was not related to prior use of one or more of bDMARDs (375). Besides, baseline characteristics (excluding DAS28-CRP score) did not substantially affect the clinical response to baricitinib in RA patients (379), but a lower DAS28-CRP score at baseline was associated with the achievement of low disease activity (367). Taken together, predicting response to baricitinib by previous treatment with DMARDs and baseline characteristics still needs further investigation (Table 4).
Upadacitinib: Mechanism, Biomarkers and Alternative Therapy
Unlike tofacitinib (359) and baricitinib (380), upadacitinib is engineered to be selective for JAK1 and serves as the second-generation JAK inhibitor approved by FDA in 2019 for RA (381). The rationale for selectively targeting JAK1 is that the anti-inflammatory effect should be maintained via inhibiting JAK1, but effects on undesired JAK2- and JAK3-dependent processes should be minimized (382, 383). Upadacitinib was shown to be >40-fold, 130-fold and 190-fold more selective for JAK1 versus JAK2, JAK3 and TYK2, respectively (383). Upadacitinib may be used as monotherapy or in combination with methotrexate in active RA patients with inadequate response to cs or bDMARDs (384). It has been demonstrated that upadacitinib was more effective than adalimumab in RA patients who were concomitantly receiving methotrexate (221). Upadacitinib could decrease circulating numbers of lymphocytes, neutrophils and NK cells with no significant changes in RF and ACPA levels (383, 385–387). In three studies (SELECT-NEXT, SELECT-BEYOND and SELECT-MONOTHERAPY), rates of ACR20 were 64%, 65% and 68% in RA patients treated with 15 mg upadacitinib, and 66%, 56% and 71% in patients treated with 30 mg upadacitinib, respectively (388–390).
Clinical response with upadacitinib treatment was mainly associated with slightly higher levels of the IL-17A, IL-17C, CCL11, CCL20, and Tissue inhibitor of metalloproteinases-4 (TIMP4) (368). A study showed that adalimumab appeared to affect M1 macrophages, while upadacitinib appeared to affect T cells preferentially (368), which was in line with another study (391). This modulatory pattern by upadacitinib was consistent with its wide cytokine receptor inhibition profile as compared to specific TNF inhibition and could account, at least in part, for superior efficacy of upadacitinib over adalimumab (368). In a randomized, double-blinded SELECT-COMPARE study enrolled 651 upadacitinib-treated patients, a total of 39% (252/651, including non- and incomplete-responders) patients were randomized to adalimumab. Low disease activity was achieved in 36% nonresponders and 45% incomplete-responders after switching for 6 months (392) (Table 4).
Precision Medicine and RA
Over the past decades, treatment of RA always depends on “trial-and-error” methods of finding a DMARD that works (393). Regarding the above-mentioned clinical trials enrolling RA patients who have failed initial DMARDs therapy, we can see that successive conventional or biologic switching, either within the same or different mechanistic class, is advocated as an alternative therapy by an experience-oriented principle. This leads to the apparent disadvantage of continuously exposing patients to multiple drugs that they do not respond to, with unnecessary side-effects, delaying the use of effective agents, and causing a serious economic burden to society (19). According to the current ACR and EULAR guidelines for managing RA, it is still a great challenge to choose the right treatment for the right patients.
The most recent EULAR recommendations provide an updated research agenda that highlights important issues to be addressed in the future, such as the safety and efficacy of various drug sequences and combinations, the discovery of biomarkers to stratify patients and predict therapeutic response, and the reasons for efficacy loss (13). It is worth noting that these issues fall into the category of the emerging precision medicine approach for disease treatment, which is a medical model that proposes the customization of healthcare, with diagnosis, prognosis and treatment being tailored to different subgroups of patients (394). Precision medicine in RA has been recently discussed regarding its great potential in allowing a better diagnosis (RA vs. non-RA), finding biomarkers for preferential treatment selection in patients (responders vs. nonresponders), as well as understanding the prognosis of the disease (progressor vs. non-progressor) (24). Precision medicine is believed to lead to the next revolution for overcoming treatment failure in RA, with the introduction of cutting-edge technologies and big data, especially the multi-omics, single-cell analysis, bioinformatics and biostatistics (Figure 1).
Multi-Omics in Precision Medicine of RA
Multi-omics is the integration of datasets generated from genomics, transcriptomics, epigenomics, proteomics and metabolomics (395). There has been a growing trend of studies, which utilize high-throughput multi-omics analyses to achieve personalized health care, especially through prediction of disease risk and early intervention for a potentially better outcome (396). Despite much hoopla based primarily on oncology data, the progress of multi-omics in autoimmunity is currently restricted (397). Previously, genomics, transcriptomics and epigenetics have been used separately to characterize the molecular basis of treatment efficacy in RA patients (145, 274, 398–400). However, the molecular effects of DMARDs from multi-omics perspectives are unknown. Recently, researchers reported longitudinal monitoring of the drug response at multi-omics levels in RA patients (401). They revealed that DMARDs treatments altered the molecular profile of RA patients closer to that of healthy individuals at the transcriptome, serum proteome, and immunophenotype levels. Effects of infliximab and tocilizumab on this molecular profile which defined stable clinical remission were greater than that of methotrexate. Tocilizumab normalized some specific molecular markers that methotrexate and infliximab did not modify, implying that tocilizumab was a more potent treatment for RA at the molecular level (401). Moreover, researchers also identified molecular signatures in transcripts and serum proteins that were resistant to DMARDs. These signals were linked to RA independently of known disease severity indices and were mostly explained by a neutrophil, monocyte, and lymphocyte imbalance. This knowledge will facilitate the identification of biomarkers and drug discovery and contribute to the development of precision therapy for RA (401). Besides, another study performed a sequential multi-omics analysis integrating transcriptomics and genomics to identify gene signatures associated with the response to anti-TNF therapy in RA patients (402). Using transcriptomic data from the RA synovium, thirteen gene co-expressed modules were found to be associated with anti-TNF response. At the genetic level, two of these modules were confirmed to be associated with the anti-TNF response using two independent GWAS cohorts of RA patients. Functional analysis suggested that nucleotide metabolism and Tregs could mediate the response to anti-TNF therapy. These findings demonstrated the existence of a drug-specific genetic foundation for an anti-TNF response, allowing for therapy stratification in the quest for response biomarkers in RA (402). In addition, the latest study conducted multi-omics and machine learning to predict response to anti-TNF therapies in RA patients. Transcription and/or DNA methylome signatures were found to be associated with response to different anti-TNF therapy in peripheral blood mononuclear cells (PBMCs), monocytes, and CD4+ T cells from RA patients (403). Further, transcription signatures in responders to adalimumab and etanercept were divergent in PBMCs, and this phenomenon was reproduced in monocytes and CD4+ T cells. Differentially methylated sites in etanercept responders but not in adalimumab responders were substantially hypermethylated (403). Finally, machine learning models based on these molecular signatures were built to reliably predict response prior to anti-TNF treatment, paving the way for tailored anti-TNF therapeutic treatment regimens (403).
Single-Cell Analysis in Precision Medicine of RA
Unlike traditional omics research, researchers have discovered that cells differ dramatically at the transcriptome, proteomic, and epigenomic levels among tissues, organs, organ systems, and organisms. Individual immune cell coordination is crucial in RA for the production of efficient immune responses to infections while immunological tolerance is maintained to protect the host. Furthermore, when immune responses are dysregulated, pathologically essential cells may constitute only a minor proportion of the immune system. Examining the roles of particular immune cells in etiology, disease progression, and medication failure should yield valuable insights into RA (404). Single-cell analysis investigates genomes, transcriptomics, proteomics, metabolomics, and cell-cell interactions in individual cells, leading to a higher resolution of cellular distinctions and a better understanding of an individual cell activity in the context of its microenvironment (405–407). It enables the high-dimensional dissection of single cells at multi-omics levels, which could facilitate the discovery of new biomarkers and stratified RA patients into more precise subgroups (408). As mentioned in a subsection of certolizumab pegol, the circulating T1IFN ratio linked to remarkably diverse gene expression patterns in monocytes of RA patients, and certain transcripts such as JAK1 were very informative and could indicate alternative treatment paths in individuals anticipated to be non-responders to anti-TNF therapy. This work was done mainly using a novel single-cell PCR approach, which was similar to single-cell sequencing (175). Another study described a robust statistical method to test for disease associations with single-cell data called MASC (Mixed-effects modeling of Associations of Single Cells). This approach enabled modeling of technical and inter-individual variance as random effects, allowing robust detection of disease-associated cellular populations. Using MASC to analyze CD4+ T cells from blood of RA patients, researchers discovered a population of memory CD4+ T cells known as CD27-HLA-DR+ that was enlarged in the circulation of RA patients. Further, CD27-HLA-DR+ T cells were enriched within inflamed RA joints, rapidly produced IFN-γ and cytolytic factors, and contracted with successful treatment of RA (409). Furthermore, the repertoires of B cell receptors, which may be collected using single cell-resolution sequencing technology, carry a personal history of antigen exposure for a donor (410). Single-cell sequencing of plasmablasts derived from RA patients revealed the presence of B cell receptors specific for CCP and other RA-associated autoantigens (411). Plasmablasts from ACPA-positive patients were sequenced, and both IgA-secreting and IgG-secreting clones were found to be responsive to common RA autoantigens (412). A longitudinal analysis of plasmablasts from individuals with clinically apparent RA revealed the presence of persistent IgA-producing cells that underwent ongoing affinity maturation and produced ACPAs (413). As the complex relationship between the response of DMARDs and the ACPA/anti-CCP has been discovered, single-cell functional B cell receptors sequencing is likely to provide new insights into precision medicine of RA.
Bioinformatics and Biostatistics in Precision Medicine of RA
Nowadays, there is an exponentially increasing number of databases integrating personal omics and volume of healthcare data (414, 415). However, due to the nature and complexity of such data, immediate interpretation or usage by healthcare practitioners is frequently out of the question. Biostatistics and bioinformatics pertain to the acquisition and interpretation of the quantitative data. No sharp delineation exists between them. Bioinformatics tends to deal with data in many dimensions, so-called “big data” (416), while biostatistics is a building block for the complex data analytics methods in bioinformatics (417). Integration of bioinformatics and biostatistics facilitate the establishment of sophisticated methods based on omics and advanced mathematics, such as artificial intelligence, machine learning and deep learning (415, 418–420). In a nutshell, artificial intelligence seeks to increase cognitive abilities and performance of computers in order to tackle complicated and massive data-oriented challenges by identifying interaction patterns among variables, learning from experiences, planning, and anticipating better directions. Machine learning is a subfield of artificial intelligence that employs and proposes various algorithms for learning from large amounts of data and revealing multifaceted relationships between data features in order to predict accuracy in various contexts and support decision-making processes, whereas deep learning is a dominant approach based on artificial neural networks (421). These methods have been widely utilized in oncology field to identify individuals at risk, to predict which prevention strategies work best on patients, to automatically screen different subtypes of diseases, or to perform drug repurposing (422). In recent years, application of these methods in RA is burgeoning. A study, for example, offered an automatic method for detecting RA disease activity in an electronic medical record. Different machine learning models were developed and tested using a training set of clinical notes and laboratory data. The models extracted terms such as synovitis, pain, or stiffness as input features by using a text analysis tool on clinical notes, and also used laboratory values of CRP or ESR. Disease activity of each RA patient was predicted by different DAS28 score. This study demonstrated that automatically discovering RA disease activity from electronic medical record data was, in principle, a learnable task, with results approximating human performance (423). Deep learning was also applied to forecast RA disease activity. Researchers classified disease activity into two categories: remission/low and moderate/high. Demographics, past CDAI score, ESR and CRP level, DMARDs, oral and injectable glucocorticoids, and autoantibodies (RF and/or ACPAs) were all taken into account. Results showed that CDAI was the most important feature for prediction of disease, followed by cortisone injections and CRP (424). Deep learning algorithms have been utilized in image processing to find patterns in images (so-called convolutional neural networks). This sort of neural network has been utilized to detect bone erosions (425) and differentiate RA patients from healthy participants from conventional hand radiographs (426). As discussed in the above subsection of multi-omics in precision medicine of RA, the combination of multi-omics and/or clinical data with machine learning could be used to predict response to DMARDs in RA patients (403). Transcription and/or DNA methylome signatures were found to be associated with response to different anti-TNF therapy in PBMCs, monocytes, and CD4+ T cells from RA patients (403). Machine learning models based on these molecular signatures were developed to accurately predict response before anti-TNF treatment. In another study, researchers used a regression model to predict the response to anti-TNF therapy after methotrexate failure, considering of demographic and clinical data in addition to genetic data (single nucleotide polymorphisms). The model classified the response to anti-TNF treatment with 78% accuracy (427).
Conclusion and Perspectives
Over the past few decades, researchers have delivered continuous efforts as above mentioned toward overcoming treatment failure in RA. In fact, these efforts have driven the diagnosis, treatment and prognosis of RA entering an early stage of precision medicine, which was commonly described as personalized medicine prior to the proposal of precision medicine. These efforts lead to a massive step when compared to the earlier era in which RA is seen as a devastating and stubborn disease. While gratifying, researchers have realized that even through sequential development of csDMARDs, bDMARDs and tsDMARDs has gradually improved treatment outcome of RA, response rates seem to reach ceiling (approximately 40-60%) in different clinical trials with DMARDs monotherapy or combination therapy in RA patients. Notably, this response ceiling is observed irrespective of the mode of action of the different types of DMARDs or their diverse specific cellular, molecular and signaling targets, such as CD20, TNF, IL-6, CD80-CD86, and the JAK-STAT pathways (18, 428). Although emerging data suggest that a higher response threshold could be reached, breaking through the response ceiling has been proven particularly difficult. This may be because that, on one hand, RA is a highly heterogeneous and complex disease with unclear understanding of mechanisms. On the other hand, small sample sizes and insufficient technologies lead to conflicting or uncertain conclusions as well as slow renewal of knowledge in basic research and translational studies of RA. Precision medicine refers more appropriately to the generation of criteria for advanced taxonomy of patients, producing models to identify and classify clinical decisions for each disease phenotype. This new perspective on patient evaluation can make use of both fundamental laboratory and clinical analyses as well as large data provided by cutting-edge technologies, as previously discussed. Precision medicine is exhibiting great potentials to be a more efficient way to overcome the treatment failure and has begun to emerge in RA studies. Undoubtedly, there are also some limitations of precision medicine, such as high cost of sequencing, existence of ethical issues, difficulty in collection and storage of large amount of data, and lack of easy and standardized approaches for data interpretation for doctors and other healthcare providers. It is believed that most of them will be solved with the rise of new technologies and algorithms. In the foreseeable future, RA patients will ultimately be precisely classified, receive their tailored therapy, and avoid wasting time during months or years of ineffective treatment. Precision medicine will also generate sufficient data to elucidate the molecular foundation underlying drug failure and push the development of next-generation DMARDs.
Author Contributions
CL, AL, and DH supervise and revise the manuscript. ZW writes and edits the manuscript. JH and DX provides their professional expertise. All authors contributed to the article and approved the submitted version.
Funding
This review is supported by the Natural Science Foundation Council of China (81700780, 81922081, 82172386, 81774114 and 82074234), The Science, Technology and Innovation Commission of Shenzhen (JCYJ20210324104201005), the Croucher Foundation (Gnt#CAS14BU/CAS14201), the 2020 Guangdong Provincial Science and Technology Innovation Strategy Special Fund (Guangdong-Hong Kong-Macau Joint Lab) (2020B1212030006) and the National administration of Traditional Chinese Medicine, regional Chinese medicine (Specialist) diagnosis and Treatment Center Construction project rheumatology.
Conflict of Interest
The authors declare that the research was conducted in the absence of any commercial or financial relationships that could be construed as a potential conflict of interest.
Publisher’s Note
All claims expressed in this article are solely those of the authors and do not necessarily represent those of their affiliated organizations, or those of the publisher, the editors and the reviewers. Any product that may be evaluated in this article, or claim that may be made by its manufacturer, is not guaranteed or endorsed by the publisher.
References
1. Gibofsky A. Epidemiology, Pathophysiology, and Diagnosis of Rheumatoid Arthritis: A Synopsis. Am J Manag Care (2014) 20(7 Suppl):S128–35.
2. Jeffery RC. Clinical Features of Rheumatoid Arthritis. Medicine (2014) 42(5):231–6. doi: 10.1016/j.mpmed.2014.02.011
3. Choy E. Understanding the Dynamics: Pathways Involved in the Pathogenesis of Rheumatoid Arthritis. Rheumatol (Oxford) (2012) 51(Suppl 5):v3–11. doi: 10.1093/rheumatology/kes113
4. Feldmann M, Maini SR. Role of Cytokines in Rheumatoid Arthritis: An Education in Pathophysiology and Therapeutics. Immunol Rev (2008) 223:7–19. doi: 10.1111/j.1600-065X.2008.00626.x
5. Smolen JS, Aletaha D, McInnes IB. Rheumatoid Arthritis. Lancet (2016) 388(10055):2023–38. doi: 10.1016/s0140-6736(16)30173-8
6. Walsh MC, Choi Y. Biology of the RANKL-RANK-OPG System in Immunity, Bone, and Beyond. Front Immunol (2014) 5:511. doi: 10.3389/fimmu.2014.00511
7. Malemud CJ. The Role of the JAK/STAT Signal Pathway in Rheumatoid Arthritis. Ther Adv Musculoskelet Dis (2018) 10(5-6):117–27. doi: 10.1177/1759720X18776224
8. Salaffi F, Ciapetti A. Clinical Disease Activity Assessments in Rheumatoid Arthritis Part of. in. Int J Clin Rheumatol (2013) 8(3):347–60. doi: 10.2217/ijr.13.24
9. Schoels M, Alasti F, Smolen JS, Aletaha D. Evaluation of Newly Proposed Remission Cut-Points for Disease Activity Score in 28 Joints (DAS28) in Rheumatoid Arthritis Patients Upon IL-6 Pathway Inhibition. Arthritis Res Ther (2017) 19(1):155. doi: 10.1186/s13075-017-1346-5
10. Heegaard C, Dreyer L, Egsmose C, Madsen OR. Test-Retest Reliability of the Disease Activity Score 28 CRP (DAS28-CRP), the Simplified Disease Activity Index (SDAI) and the Clinical Disease Activity Index (CDAI) in Rheumatoid Arthritis When Based on Patient Self-Assessment of Tender and Swollen Joints. Clin Rheumatol (2013) 32(10):1493–500. doi: 10.1007/s10067-013-2300-9
11. Carpenter L, Norton S, Nikiphorou E, Kiely P, Walsh DA, Dixey J, et al. Validation of Methods for Converting the Original Disease Activity Score (DAS) to the DAS28. Rheumatol Int (2018) 38(12):2297–305. doi: 10.1007/s00296-018-4184-0
12. Smolen JS, Aletaha D. Scores for All Seasons: SDAI and CDAI. Clin Exp Rheumatol (2014) 32(5 Suppl 85):S–75-9.
13. Smolen JS, Landewe RBM, Bijlsma JWJ, Burmester GR, Dougados M, Kerschbaumer A, et al. EULAR Recommendations for the Management of Rheumatoid Arthritis With Synthetic and Biological Disease-Modifying Antirheumatic Drugs: 2019 Update. Ann Rheum Dis (2020) 79(6):685–99. doi: 10.1136/annrheumdis-2019-216655
14. Hyrich KL, Lunt M, Dixon WG, Watson KD, Symmons DP, Register BSRB. Effects of Switching Between Anti-TNF Therapies on HAQ Response in Patients Who do Not Respond to Their First Anti-TNF Drug. Rheumatol (Oxford) (2008) 47(7):1000–5. doi: 10.1093/rheumatology/ken127
15. Rubbert-Roth A, Finckh A. Treatment Options in Patients With Rheumatoid Arthritis Failing Initial TNF Inhibitor Therapy: A Critical Review. Arthritis Res Ther (2009) 11 Suppl:1, S1. doi: 10.1186/ar2666
16. Mittal N, Mittal R, Sharma A, Jose V, Wanchu A, Singh S. Treatment Failure With Disease-Modifying Antirheumatic Drugs in Rheumatoid Arthritis Patients. Singapore Med J (2012) 53(8):532–6.
17. Gomes JL, Sepriano A, Eusebio M, Serra S, Fonseca JE, Saavedra MJ, et al. Predictors and Causes of First-Line Biologic Agent Discontinuation in Rheumatoid Arthritis: Data From Reuma.Pt. Acta Reumatol Port (2019) 44(1):57–64.
18. Favalli EG, Raimondo MG, Becciolini A, Crotti C, Biggioggero M, Caporali R. The Management of First-Line Biologic Therapy Failures in Rheumatoid Arthritis: Current Practice and Future Perspectives. Autoimmun Rev (2017) 16(12):1185–95. doi: 10.1016/j.autrev.2017.10.002
19. Astorri E, Nerviani A, Bombardieri M, Pitzalis C. Towards a Stratified Targeted Approach With Biologic Treatments in Rheumatoid Arthritis: Role of Synovial Pathobiology. Curr Pharm Des (2015) 21(17):2216–24. doi: 10.2174/1381612821666150310145758
20. Vital EM, Dass S, Rawstron AC, Buch MH, Goeb V, Henshaw K, et al. Management of Nonresponse to Rituximab in Rheumatoid Arthritis: Predictors and Outcome of Re-Treatment. Arthritis Rheum (2010) 62(5):1273–9. doi: 10.1002/art.27359
21. Mahler M, Martinez-Prat L, Sparks JA, Deane KD. Precision Medicine in the Care of Rheumatoid Arthritis: Focus on Prediction and Prevention of Future Clinically-Apparent Disease. Autoimmun Rev (2020) 19(5):102506. doi: 10.1016/j.autrev.2020.102506
22. Denny JC, Collins FS. Precision Medicine in 2030—Seven Ways to Transform Healthcare. Cell (2021) 184(6):1415–9. doi: 10.1016/j.cell.2021.01.015
23. Redekop WK, Mladsi D. The Faces of Personalized Medicine: A Framework for Understanding Its Meaning and Scope. Value Health (2013) 6(6, Supplement):S4–9. doi: 10.1016/j.jval.2013.06.005
24. Aletaha D. Precision Medicine and Management of Rheumatoid Arthritis. J Autoimmun (2020) 110:102405. doi: 10.1016/j.jaut.2020.102405
25. Salliot C, van der Heijde D. Long-Term Safety of Methotrexate Monotherapy in Patients With Rheumatoid Arthritis: A Systematic Literature Research. Ann Rheum Dis (2009) 68(7):1100–4. doi: 10.1136/ard.2008.093690
26. Thomas S, Fisher KH, Snowden JA, Danson SJ, Brown S, Zeidler MP. Methotrexate Is a JAK/STAT Pathway Inhibitor. PLoS One (2015) 10(7):e0130078. doi: 10.1371/journal.pone.0130078
27. Revu S, Neregard P, af Klint E, Korotkova M, Catrina AI. Synovial Membrane Immunohistology in Early-Untreated Rheumatoid Arthritis Reveals High Expression of Catabolic Bone Markers That is Modulated by Methotrexate. Arthritis Res Ther (2013) 15(6):R205. doi: 10.1186/ar4398
28. Brown PM, Pratt AG, Isaacs JD. Mechanism of Action of Methotrexate in Rheumatoid Arthritis, and the Search for Biomarkers. Nat Rev Rheumatol (2016) 12(12):731–42. doi: 10.1038/nrrheum.2016.175
29. Hazlewood GS, Barnabe C, Tomlinson G, Marshall D, Devoe D, Bombardier C. Methotrexate Monotherapy and Methotrexate Combination Therapy With Traditional and Biologic Disease Modifying Antirheumatic Drugs for Rheumatoid Arthritis: Abridged Cochrane Systematic Review and Network Meta-Analysis. BMJ (2016) 353:i1777. doi: 10.1136/bmj.i1777
30. Cronstein BN, Sitkovsky M. Adenosine and Adenosine Receptors in the Pathogenesis and Treatment of Rheumatic Diseases. Nat Rev Rheumatol (2017) 13(1):41–51. doi: 10.1038/nrrheum.2016.178
31. Montesinos MC, Desai A, Delano D, Chen JF, Fink JS, Jacobson MA, et al. Adenosine A2A or A3 Receptors are Required for Inhibition of Inflammation by Methotrexate and its Analog MX-68. Arthritis Rheum (2003) 48(1):240–7. doi: 10.1002/art.10712
32. Varani K, Padovan M, Vincenzi F, Targa M, Trotta F, Govoni M, et al. A2A and A3 Adenosine Receptor Expression in Rheumatoid Arthritis: Upregulation, Inverse Correlation With Disease Activity Score and Suppression of Inflammatory Cytokine and Metalloproteinase Release. Arthritis Res Ther (2011) 13(6):R197. doi: 10.1186/ar3527
33. Singh A, Misra R, Aggarwal A. Baseline Adenosine Receptor mRNA Expression in Blood as Predictor of Response to Methotrexate Therapy in Patients With Rheumatoid Arthritis. Rheumatol Int (2019) 39(8):1431–8. doi: 10.1007/s00296-019-04344-2
34. Peres RS, Liew FY, Talbot J, Carregaro V, Oliveira RD, Almeida SL, et al. Low Expression of CD39 on Regulatory T Cells as a Biomarker for Resistance to Methotrexate Therapy in Rheumatoid Arthritis. Proc Natl Acad Sci USA (2015) 112(8):2509–14. doi: 10.1073/pnas.1424792112
35. Vincenzi F, Padovan M, Targa M, Corciulo C, Giacuzzo S, Merighi S, et al. A(2A) Adenosine Receptors are Differentially Modulated by Pharmacological Treatments in Rheumatoid Arthritis Patients and Their Stimulation Ameliorates Adjuvant-Induced Arthritis in Rats. PloS One (2013) 8(1):e54195. doi: 10.1371/journal.pone.0054195
36. Nesher G, Mates M, Zevin S. Effect of Caffeine Consumption on Efficacy of Methotrexate in Rheumatoid Arthritis. Arthritis Rheum (2003) 48(2):571–2. doi: 10.1002/art.10766
37. Montesinos MC, Yap JS, Desai A, Posadas I, McCrary CT, Cronstein BN. Reversal of the Antiinflammatory Effects of Methotrexate by the Nonselective Adenosine Receptor Antagonists Theophylline and Caffeine: Evidence That the Antiinflammatory Effects of Methotrexate are Mediated via Multiple Adenosine Receptors in Rat Adjuvant Arthritis. Arthritis Rheum (2000) 43(3):656–63. doi: 10.1002/1529-0131(200003)43:3<656::Aid-anr23>3.0.Co;2-h
38. Andersson SE, Johansson LH, Lexmüller K, Ekström GM. Anti-Arthritic Effect of Methotrexate: Is it Really Mediated by Adenosine? Eur J Pharm Sci (2000) 9(4):333–43. doi: 10.1016/s0928-0987(99)00073-1
39. Benito-Garcia E, Heller JE, Chibnik LB, Maher NE, Matthews HM, Bilics JA, et al. Dietary Caffeine Intake Does Not Affect Methotrexate Efficacy in Patients With Rheumatoid Arthritis. J Rheumatol (2006) 33(7):1275–81.
40. de Rotte MCFJ, den Boer E, de Jong PHP, Pluijm SMF, Bulatović Ćalasan M, Weel AE, et al. Methotrexate Polyglutamates in Erythrocytes are Associated With Lower Disease Activity in Patients With Rheumatoid Arthritis. Ann Rheum Dis (2015) 74(2):408. doi: 10.1136/annrheumdis-2013-203725
41. Stamp LK, Barclay ML, O’Donnell JL, Zhang M, Drake J, Frampton C, et al. Effects of Changing From Oral to Subcutaneous Methotrexate on Red Blood Cell Methotrexate Polyglutamate Concentrations and Disease Activity in Patients With Rheumatoid Arthritis. J Rheumatol (2011) 38(12):2540–7. doi: 10.3899/jrheum.110481
42. Dervieux T, Furst D, Lein DO, Capps R, Smith K, Caldwell J, et al. Pharmacogenetic and Metabolite Measurements are Associated With Clinical Status in Patients With Rheumatoid Arthritis Treated With Methotrexate: Results of a Multicentred Cross Sectional Observational Study. Ann Rheum Dis (2005) 64(8):1180. doi: 10.1136/ard.2004.033399
43. Litman T, Druley TE, Stein WD, Bates SE. From MDR to MXR: New Understanding of Multidrug Resistance Systems, Their Properties and Clinical Significance. Cell Mol Life Sci (2001) 58(7):931–59. doi: 10.1007/pl00000912
44. Volk EL, Schneider E. Wild-Type Breast Cancer Resistance Protein (BCRP/ABCG2) is a Methotrexate Polyglutamate Transporter. Cancer Res (2003) 63(17):5538–43.
45. Muto S, Minamitani N, Ogura T, Nakajima A, Nakagawa K, Masaka T, et al. Good Response to Methotrexate Is Associated With a Decrease in the Gene Expression of ABCG2, a Drug Transporter, in Patients With Rheumatoid Arthritis. Mod Rheumatol (2021) 31(6):1079–86. doi: 10.1080/14397595.2021.1879429
46. Hakamata J, Kaneko Y, Shimizu M, Yamaoka K, Maruyama J, Takeuchi T, et al. Factors Predicting the Therapeutic Response to Methotrexate in Japanese Patients With Rheumatoid Arthritis: A Hospital-Based Cohort Study. Biol Pharm Bull (2018) 41(9):1414–22. doi: 10.1248/bpb.b18-00247
47. Tugwell P, Pincus T, Yocum D, Stein M, Gluck O, Kraag G, et al. Combination Therapy With Cyclosporine and Methotrexate in Severe Rheumatoid Arthritis. The Methotrexate-Cyclosporine Combination Study Group. N Engl J Med (1995) 333(3):137–41. doi: 10.1056/nejm199507203330301
48. Cooper DL, Martin SG, Robinson JI, Mackie SL, Charles CJ, Nam J, et al. Fcγriiia Expression on Monocytes in Rheumatoid Arthritis: Role in Immune-Complex Stimulated TNF Production and non-Response to Methotrexate Therapy. PloS One (2012) 7(1):e28918. doi: 10.1371/journal.pone.0028918
49. Hong H, Yang H, Xia Y. Circulating miR-10a as Predictor of Therapy Response in Rheumatoid Arthritis Patients Treated With Methotrexate. Curr Pharm Biotechnol (2018) 19(1):79–86. doi: 10.2174/1389201019666180417155140
50. O’Dell JR, Nepom BS, Haire C, Gersuk VH, Gaur L, Moore GF, et al. HLA-DRB1 Typing in Rheumatoid Arthritis: Predicting Response to Specific Treatments. Ann Rheum Dis (1998) 57(4):209–13. doi: 10.1136/ard.57.4.209
51. Stuhlmüller B, Mans K, Tandon N, Bonin MO, Smiljanovic B, Sörensen TA, et al. Genomic Stratification by Expression of HLA-DRB4 Alleles Identifies Differential Innate and Adaptive Immune Transcriptional Patterns - A Strategy to Detect Predictors of Methotrexate Response in Early Rheumatoid Arthritis. Clin Immunol (2016) 171:50–61. doi: 10.1016/j.clim.2016.08.013
52. Eektimmerman F, Swen JJ, Madhar MB, Allaart CF, Guchelaar HJ. Predictive Genetic Biomarkers for the Efficacy of Methotrexate in Rheumatoid Arthritis: A Systematic Review. Pharmacogenomics J (2020) 20(2):159–68. doi: 10.1038/s41397-019-0098-9
53. Sharma S, Das M, Kumar A, Marwaha V, Shankar S, Singh P, et al. Purine Biosynthetic Pathway Genes and Methotrexate Response in Rheumatoid Arthritis Patients Among North Indians. Pharmacogenet Genomics (2009) 19(10):823–8. doi: 10.1097/fpc.0b013e328331b53e
54. Pawlik A, Czerny B, Dabrowska-Zamojcin E, Górnik W, Poziomkowska I, Gawrońska-Szklarz B, et al. The Influence of IL-6 Polymorphism on Efficacy of Treatment of Rheumatoid Arthritis Patients With Methotrexate and Prednisone. Pol Arch Med Wewn (2005) 114(3):843–7.
55. Ruiz-Padilla AJ, Gamez-Nava JI, Saldaña-Cruz AM, Murillo-Vazquez JD, Vazquez-Villegas ML, Zavaleta-Muñiz SA, et al. The -174g/C Interleukin-6 Gene Promoter Polymorphism as a Genetic Marker of Differences in Therapeutic Response to Methotrexate and Leflunomide in Rheumatoid Arthritis. BioMed Res Int (2016) 2016:4193538. doi: 10.1155/2016/4193538
56. Bohanec Grabar P, Grabnar I, Rozman B, Logar D, Tomsic M, Suput D, et al. Investigation of the Influence of CYP1A2 and CYP2C19 Genetic Polymorphism on 2-Cyano-3-Hydroxy-N-[4-(Trifluoromethyl)Phenyl]-2-Butenamide (A77 1726) Pharmacokinetics in Leflunomide-Treated Patients With Rheumatoid Arthritis. Drug Metab Dispos (2009) 37(10):2061–8. doi: 10.1124/dmd.109.027482
57. Pawlik A, Herczynska M, Kurzawski M, Safranow K, Dziedziejko V, Drozdzik M. The Effect of Exon (19C>A) Dihydroorotate Dehydrogenase Gene Polymorphism on Rheumatoid Arthritis Treatment With Leflunomide. Pharmacogenomics (2009) 10(2):303–9. doi: 10.2217/14622416.10.2.303
58. Dziedziejko V, Kurzawski M, Safranow K, Chlubek D, Pawlik A. The Effect of ESR1 and ESR2 Gene Polymorphisms on the Outcome of Rheumatoid Arthritis Treatment With Leflunomide. Pharmacogenomics (2011) 12(1):41–7. doi: 10.2217/pgs.10.164
59. Liang C, Li J, Lu C, Xie D, Liu J, Zhong C, et al. Hif1α Inhibition Facilitates Leflunomide-AHR-CRP Signaling to Attenuate Bone Erosion in CRP-Aberrant Rheumatoid Arthritis. Nat Commun (2019) 10(1):4579. doi: 10.1038/s41467-019-12163-z
60. Gotanda K, Hirota T, Saito J, Fukae M, Egashira Y, Izumi N, et al. Circulating Intestine-Derived Exosomal miR-328 in Plasma, a Possible Biomarker for Estimating BCRP Function in the Human Intestines. Sci Rep (2016) 6:32299. doi: 10.1038/srep32299
61. Perez-Guerrero EE, Gonzalez-Lopez L, Munoz-Valle JF, Vasquez-Jimenez JC, Ramirez-Villafana M, Sanchez-Rodriguez EN, et al. Serum P-Glycoprotein Level: A Potential Biomarker of DMARD Failure in Patients With Rheumatoid Arthritis. Inflammopharmacology (2018) 26:1375–81. doi: 10.1007/s10787-018-0529-2
62. Wiese MD, Alotaibi N, O’Doherty C, Sorich MJ, Suppiah V, Cleland LG, et al. Pharmacogenomics of NAT2 and ABCG2 Influence the Toxicity and Efficacy of Sulphasalazine Containing DMARD Regimens in Early Rheumatoid Arthritis. Pharmacogenomics J (2014) 14(4):350–5. doi: 10.1038/tpj.2013.45
63. Romão VC, Canhão H, Fonseca JE. Old Drugs, Old Problems: Where do We Stand in Prediction of Rheumatoid Arthritis Responsiveness to Methotrexate and Other Synthetic DMARDs? BMC Med (2013) 11(1):17. doi: 10.1186/1741-7015-11-17
64. Varbanov B, Mircheva I. Efectiveness of Treatment With Sulfasalasine in Juvenile Idiopathic Arthritis. Pediatriya (2013) 53:59–61.
65. Wijesinghe H, Galappatthy P, de Silva R, Seneviratne SL, Saravanamuttu U, Udagama P, et al. Leflunomide is Equally Efficacious and Safe Compared to Low Dose Rituximab in Refractory Rheumatoid Arthritis Given in Combination With Methotrexate: Results From a Randomized Double Blind Controlled Clinical Trial. BMC Musculoskeletal Disord (2017) 18(1):310–0. doi: 10.1186/s12891-017-1673-3
66. Kremer J, Genovese M, Cannon GW, Caldwell J, Cush J, Furst DE, et al. Combination Leflunomide and Methotrexate (MTX) Therapy for Patients With Active Rheumatoid Arthritis Failing MTX Monotherapy: Open-Label Extension of a Randomized, Double-Blind, Placebo Controlled Trial. J Rheumatol (2004) 31(8):1521–31.
67. Weisman MH, Durez P, Hallegua D, Aranda R, Becker JC, Nuamah I, et al. Reduction of Inflammatory Biomarker Response by Abatacept in Treatment of Rheumatoid Arthritis. J Rheumatol (2006) 33(11):2162–6.
68. Smolen JS, van Vollenhoven RF, Florentinus S, Chen S, Suboticki JL, Kavanaugh A. Predictors of Disease Activity and Structural Progression After Treatment With Adalimumab Plus Methotrexate or Continued Methotrexate Monotherapy in Patients With Early Rheumatoid Arthritis and Suboptimal Response to Methotrexate. Ann Rheum Dis (2018) 77(11):1566–72. doi: 10.1136/annrheumdis-2018-213502
69. Kaneko Y, Atsumi T, Tanaka Y, Inoo M, Kobayashi-Haraoka H, Amano K, et al. Comparison of Adding Tocilizumab to Methotrexate With Switching to Tocilizumab in Patients With Rheumatoid Arthritis With Inadequate Response to Methotrexate: 52-Week Results From a Prospective, Randomised, Controlled Study (SURPRISE Study). Ann Rheum Dis (2016) 75(11):1917–23. doi: 10.1136/annrheumdis-2015-208426
70. Davis JP, Cain GA, Pitts WJ, Magolda RL, Copeland RA. The Immunosuppressive Metabolite of Leflunomide Is a Potent Inhibitor of Human Dihydroorotate Dehydrogenase. Biochemistry (1996) 35(4):1270–3. doi: 10.1021/bi952168g
71. Breedveld FC, Dayer J-M. Leflunomide: Mode of Action in the Treatment of Rheumatoid Arthritis. Ann Rheum Dis (2000) 59(11):841–9. doi: 10.1136/ard.59.11.841
72. Kirsch BM, Zeyda M, Stuhlmeier K, Grisar J, Smolen JS, Watschinger B, et al. The Active Metabolite of Leflunomide, A77 1726, Interferes With Dendritic Cell Function. Arthritis Res Ther (2005) 7(3):R694. doi: 10.1186/ar1727
73. Fang J, Uchiumi T, Yagi M, Matsumoto S, Amamoto R, Takazaki S, et al. Dihydro-Orotate Dehydrogenase is Physically Associated With the Respiratory Complex and its Loss Leads to Mitochondrial Dysfunction. Biosci Rep (2013) 33(2):e00021. doi: 10.1042/BSR20120097
74. O’Doherty C, Schnabl M, Spargo L, Cleland LG, James M, Proudman SM, et al. Association of DHODH Haplotype Variants and Response to Leflunomide Treatment in Rheumatoid Arthritis. Pharmacogenomics (2012) 13(12):1427–34. doi: 10.2217/pgs.12.118
75. Pawlik A, Herczyńska M, Kurzawski M, Safranow K, Dziedziejko V, Juzyszyn Z, et al. IL-1beta, IL-6, and TNF Gene Polymorphisms do Not Affect the Treatment Outcome of Rheumatoid Arthritis Patients With Leflunomide. Pharmacol Rep (2009) 61(2):281–7. doi: 10.1016/s1734-1140(09)70033-7
76. Kis E, Nagy T, Jani M, Molnar E, Janossy J, Ujhellyi O, et al. Leflunomide and its Metabolite A771726 are High Affinity Substrates of BCRP: Implications for Drug Resistance. Ann Rheum Dis (2009) 68(7):1201–7. doi: 10.1136/ard.2007.086264
77. Karanikolas G, Charalambopoulos D, Andrianakos A, Antoniades C, Katsilambros N. Combination of Cyclosporine and Leflunomide Versus Single Therapy in Severe Rheumatoid Arthritis. J Rheumatol (2006) 33(3):486–9. doi: 10.1136/ard.2007.086264
78. Lu C, Xu W, Shao J, Zhang F, Chen A, Zheng S. Nrf2 Activation Is Required for Ligustrazine to Inhibit Hepatic Steatosis in Alcohol-Preferring Mice and Hepatocytes. Toxicol Sci (2016) 155(2):432–43. doi: 10.1093/toxsci/kfw228
79. Zhang C, Guan D, Jiang M, Liang C, Li L, Zhao N, et al. Efficacy of Leflunomide Combined With Ligustrazine in the Treatment of Rheumatoid Arthritis: Prediction With Network Pharmacology and Validation in a Clinical Trial. Chin Med (2019) 14(1):26. doi: 10.1186/s13020-019-0247-8
80. Hansen KE, Cush J, Singhal A, Cooley DA, Cohen S, Patel SR, et al. The Safety and Efficacy of Leflunomide in Combination With Infliximab in Rheumatoid Arthritis. Arthritis Rheum (2004) 51(2):228–32. doi: 10.1002/art.20228
81. Dougados M, Emery P, Lemmel EM, Zerbini CA, Brin S, van Riel P. When a DMARD Fails, Should Patients Switch to Sulfasalazine or Add Sulfasalazine to Continuing Leflunomide? Ann Rheum Dis (2005) 64(1):44–51. doi: 10.1136/ard.2003.016709
82. Götestam Skorpen C, Hoeltzenbein M, Tincani A, Fischer-Betz R, Elefant E, Chambers C, et al. The EULAR Points to Consider for Use of Antirheumatic Drugs Before Pregnancy, and During Pregnancy and Lactation. Ann Rheum Dis (2016) 75(5):795–810. doi: 10.1136/annrheumdis-2015-208840
83. Kesharwani D, Paliwal R, Satapathy T, Das Paul S. Rheumatiod Arthritis: An Updated Overview of Latest Therapy and Drug Delivery. J Pharmacopuncture (2019) 22(4):210–24. doi: 10.3831/KPI.2019.22.029
84. Rodenburg R, Ganga A, Lent P, Putte L, Venrooij W. The Antiinflammatory Drug Sulfasalazine Inhibits Tumor Necrosis Factor α Expression in Macrophages by Inducing Apoptosis. Arthritis Rheum (2000) 43:1941–50. doi: 10.1002/1529-0131(200009)43:9<1941::AID-ANR4>3.0.CO;2-O
85. Plosker GL, Croom KF. Sulfasalazine: A Review of its Use in the Management of Rheumatoid Arthritis. Drugs (2005) 65(13):1825–49. doi: 10.2165/00003495-200565130-00008
86. Morabito L, Montesinos MC, Schreibman DM, Balter L, Thompson LF, Resta R, et al. Methotrexate and Sulfasalazine Promote Adenosine Release by a Mechanism That Requires Ecto-5’-Nucleotidase-Mediated Conversion of Adenine Nucleotides. J Clin Invest (1998) 101(2):295–300. doi: 10.1172/jci1554
87. MacDermott RP, Schloemann SR, Bertovich MJ, Nash GS, Peters M, Stenson WF. Inhibition of Antibody Secretion by 5-Aminosalicylic Acid. GastroenterolOGY (1989) 96(2 Pt 1):442–8. doi: 10.1016/0016-5085(89)91569-2
88. Volin M, Campbell P, Connors M, Woodruff D, Koch A. The Effect of Sulfasalazine on Rheumatoid Arthritic Synovial Tissue Chemokine Production. Exp Mol Pathol (2002) 73:84–92. doi: 10.1006/exmp.2002.2460
89. Choi J, Fenando A. Sulfasalazine. In: StatPearls. Treasure Island (FL: StatPearls Publishing Copyright © 2021, StatPearls Publishing LLC. (2021).
90. Huang R, Tang Y, Zeng J. Comparison of the Effects of Tripterygii Totorum and Sulfasalazine on Rheumatoid Arthritis: A Retrospective Cohort Study. Trop J Pharm Res (2020) 19:421–5. doi: 10.4314/tjpr.v19i2.26
91. Scott DL, Smolen JS, Kalden JR, van de Putte LB, Larsen A, Kvien TK, et al. Treatment of Active Rheumatoid Arthritis With Leflunomide: Two Year Follow Up of a Double Blind, Placebo Controlled Trial Versus Sulfasalazine. Ann Rheum Dis (2001) 60(10):913–23. doi: 10.1136/ard.60.10.913
92. Zaher H, Khan AA, Palandra J, Brayman TG, Yu L, Ware JA. Breast Cancer Resistance Protein (BCRP/ABCG2) is a Major Determinant of Sulfasalazine Absorption and Elimination in the Mouse. FASEB J (2006) 20(5):A1143–3. doi: 10.1096/fasebj.20.5.A1143-c
93. Pascual-Ramos V, Atisha-Fregoso Y, Lima G, Fragoso-Loyo H, Jákez-Ocampo J, Contreras-Yáñez I, et al. Rheumatoid Arthritis (RA) Disease Activity Predicts Function of ABCB1 (P-Gp) and ABCG2 (BCRP1) Drug-Efflux Transporters. Gac Med Mex (2016) 152(6):582–6.
94. Zamek-Gliszczynski MJ, Bedwell DW, Bao JQ, Higgins JW. Characterization of SAGE Mdr1a (P-Gp), Bcrp, and Mrp2 Knockout Rats Using Loperamide, Paclitaxel, Sulfasalazine, and Carboxydichlorofluorescein Pharmacokinetics. Drug Metab Dispos (2012) 40(9):1825–33. doi: 10.1124/dmd.112.046508
95. Yudoh K, Matsuno H, Nakazawa F, Yonezawa T, Kimura T. Increased Expression of Multidrug Resistance of P-Glycoprotein on Th1 Cells Correlates With Drug Resistance in Rheumatoid Arthritis. Arthritis Rheum (1999) 42(9):2014–5. doi: 10.1002/1529-0131(199909)42:9<2014::Aid-anr32>3.0.Co;2-r
96. van der Heijden J, de Jong MC, Dijkmans BAC, Lems WF, Oerlemans R, Kathmann I, et al. Development of Sulfasalazine Resistance in Human T Cells Induces Expression of the Multidrug Resistance Transporter ABCG2 (BCRP) and Augmented Production of TNFα. Ann Rheum Dis (2004) 63(2):138–43. doi: 10.1136/ard.2002.005249
97. Lee A, Park K, Choi SJ, Seo DH, Kim K, Kim HS, et al. Prediction of Antiarthritic Drug Efficacies by Monitoring Active Matrix Metalloproteinase-3 (MMP-3) Levels in Collagen-Induced Arthritic Mice Using the MMP-3 Probe. Mol Pharm (2014) 11(5):1450–8. doi: 10.1021/mp400622q
98. Capell HA, Madhok R, Porter DR, Munro RA, McInnes IB, Hunter JA, et al. Combination Therapy With Sulfasalazine and Methotrexate is More Effective Than Either Drug Alone in Patients With Rheumatoid Arthritis With a Suboptimal Response to Sulfasalazine: Results From the Double-Blind Placebo-Controlled MASCOT Study. Ann Rheum Dis (2007) 66(2):235–41. doi: 10.1136/ard.2006.057133
99. Schipper LG, Fransen J, Barrera P, Van Riel PLCM. Methotrexate in Combination With Sulfasalazine is More Effective in Rheumatoid Arthritis Patients Who Failed Sulfasalazine Than in Patients Naïve to Both Drugs. Rheumatology (2009) 48(7):828–33. doi: 10.1093/rheumatology/kep090
100. Combe B, Codreanu C, Fiocco U, Gaubitz M, Geusens PP, Kvien TK, et al. Efficacy, Safety and Patient-Reported Outcomes of Combination Etanercept and Sulfasalazine Versus Etanercept Alone in Patients With Rheumatoid Arthritis: A Double-Blind Randomised 2-Year Study. Ann Rheum Dis (2009) 68(7):1146–52. doi: 10.1136/ard.2007.087106
101. Kawai S, Takeuchi T, Yamamoto K, Tanaka Y, Miyasaka N. Efficacy and Safety of Additional Use of Tacrolimus in Patients With Early Rheumatoid Arthritis With Inadequate Response to DMARDs–a Multicenter, Double-Blind, Parallel-Group Trial. Mod Rheumatol (2011) 21(5):458–68. doi: 10.1007/s10165-011-0425-8
102. Elliott MJ, Maini RN, Feldmann M, Long-Fox A, Charles P, Katsikis P, et al. Treatment of Rheumatoid Arthritis With Chimeric Monoclonal Antibodies to Tumor Necrosis Factor Alpha. Arthritis Rheum (1993) 36(12):1681–90. doi: 10.1002/art.1780361206
103. Melsheimer R, Geldhof A, Apaolaza I, Schaible T. Remicade(®) (Infliximab): 20 Years of Contributions to Science and Medicine. Biologics: Targets Ther (2019) 13:139–78. doi: 10.2147/BTT.S207246
104. Chaabo K, Kirkham B. Rheumatoid Arthritis - Anti-TNF. Int Immunopharmacol (2015) 27(2):180–4. doi: 10.1016/j.intimp.2015.04.051
105. Klotz U, Teml A, Schwab M. Clinical Pharmacokinetics and Use of Infliximab. Clin Pharmacokinet (2007) 46(8):645–60. doi: 10.2165/00003088-200746080-00002
106. Harriman G, Harper LK, Schaible TF. Summary of Clinical Trials in Rheumatoid Arthritis Using Infliximab, an Anti-TNFα Treatment. Ann Rheum Dis (1999) 58(suppl 1):I61–4. doi: 10.1136/ard.58.2008.i61
107. Kim J, Ryu H, Yoo DH, Park SH, Song GG, Park W, et al. A Clinical Trial and Extension Study of Infliximab in Korean Patients With Active Rheumatoid Arthritis Despite Methotrexate Treatment. J Korean Med Sci (2013) 28(12):1716–22. doi: 10.3346/jkms.2013.28.12.1716
108. Talotta R, Rucci F, Canti G, Scaglione F. Pros and Cons of the Immunogenicity of Monoclonal Antibodies in Cancer Treatment: A Lesson From Autoimmune Diseases. Immunotherapy (2019) 11(3):241–54. doi: 10.2217/imt-2018-0081
109. Vos Q, Lees A, Wu ZQ, Snapper CM, Mond JJ. B-Cell Activation by T-Cell-Independent Type 2 Antigens as an Integral Part of the Humoral Immune Response to Pathogenic Microorganisms. Immunol Rev (2000) 176:154–70. doi: 10.1034/j.1600-065x.2000.00607.x
110. Atzeni F, Talotta R, Salaffi F, Cassinotti A, Varisco V, Battellino M, et al. Immunogenicity and Autoimmunity During Anti-TNF Therapy. Autoimmun Rev (2013) 12(7):703–8. doi: 10.1016/j.autrev.2012.10.021
111. van Schie KA, Hart MH, de Groot ER, Kruithof S, Aarden LA, Wolbink GJ, et al. The Antibody Response Against Human and Chimeric Anti-TNF Therapeutic Antibodies Primarily Targets the TNF Binding Region. Ann Rheum Dis (2015) 74(1):311–4. doi: 10.1136/annrheumdis-2014-206237
112. Hambardzumyan K, Hermanrud C, Marits P, Vivar N, Ernestam S, Wallman JK, et al. Association of Female Sex and Positive Rheumatoid Factor With Low Serum Infliximab and Anti-Drug Antibodies, Related to Treatment Failure in Early Rheumatoid Arthritis: Results From the SWEFOT Trial Population. Scand J Rheumatol (2019) 48(5):362–6. doi: 10.1080/03009742.2019.1602670
113. Eng GP, Bouchelouche P, Bartels EM, Bliddal H, Bendtzen K, Stoltenberg M. Anti-Drug Antibodies, Drug Levels, Interleukin-6 and Soluble TNF Receptors in Rheumatoid Arthritis Patients During the First 6 Months of Treatment With Adalimumab or Infliximab: A Descriptive Cohort Study. PloS One (2016) 11(9):e0162316. doi: 10.1371/journal.pone.0162316
114. Wolbink GJ, Vis M, Lems W, Voskuyl AE, de Groot E, Nurmohamed MT, et al. Development of Antiinfliximab Antibodies and Relationship to Clinical Response in Patients With Rheumatoid Arthritis. Arthritis Rheum (2006) 54(3):711–5. doi: 10.1002/art.21671
115. Murdaca G, Negrini S, Greco M, Schiavi C, Giusti F, Borro M, et al. Immunogenicity of Infliximab and Adalimumab. Expert Opin Drug Saf (2019) 18(5):343–5. doi: 10.1080/14740338.2019.1602117
116. Radstake TR, Svenson M, Eijsbouts AM, van den Hoogen FH, Enevold C, van Riel PL, et al. Formation of Antibodies Against Infliximab and Adalimumab Strongly Correlates With Functional Drug Levels and Clinical Responses in Rheumatoid Arthritis. Ann Rheum Dis (2009) 68(11):1739–45. doi: 10.1136/ard.2008.092833
117. Kalden JR, Schulze-Koops H. Immunogenicity and Loss of Response to TNF Inhibitors: Implications for Rheumatoid Arthritis Treatment. Nat Rev Rheumatol (2017) 13(12):707–18. doi: 10.1038/nrrheum.2017.187
118. Siljehult F, Ärlestig L, Eriksson C, Rantapää-Dahlqvist S. Concentrations of Infliximab and Anti-Drug Antibodies in Relation to Clinical Response in Patients With Rheumatoid Arthritis. Scand J Rheumatol (2018) 47(5):345–50. doi: 10.1080/03009742.2018.1433232
119. Cerezo LA, Remáková M, Tomčik M, Gay S, Neidhart M, Lukanidin E, et al. The Metastasis-Associated Protein S100A4 Promotes the Inflammatory Response of Mononuclear Cells via the TLR4 Signalling Pathway in Rheumatoid Arthritis. Rheumatology (2014) 53(8):1520–6. doi: 10.1093/rheumatology/keu031
120. Erlandsson MC, Forslind K, Andersson SEM, Lund A, Bokarewa MI. Metastasin S100A4 is Increased in Proportion to Radiographic Damage in Patients With RA. Rheumatology (2012) 51(5):932–40. doi: 10.1093/rheumatology/ker362
121. Andersson SEM, Svensson MND, Erlandsson MC, Dehlin M, Andersson KME, Bokarewa MI. Activation of Fms-Like Tyrosine Kinase 3 Signaling Enhances Survivin Expression in a Mouse Model of Rheumatoid Arthritis. PloS One (2012) 7(10):e47668–8. doi: 10.1371/journal.pone.0047668
122. Isgren A, Forslind K, Erlandsson M, Axelsson C, Andersson S, Lund A, et al. High Survivin Levels Predict Poor Clinical Response to Infliximab Treatment in Patients With Rheumatoid Arthritis. Semin Arthritis Rheum (2012) 41(5):652–7. doi: 10.1016/j.semarthrit.2011.08.005
123. Sekiguchi N, Kawauchi S, Furuya T, Inaba N, Matsuda K, Ando S, et al. Messenger Ribonucleic Acid Expression Profile in Peripheral Blood Cells From RA Patients Following Treatment With an Anti-TNF-Alpha Monoclonal Antibody, Infliximab. Rheumatol (Oxford) (2008) 47(6):780–8. doi: 10.1093/rheumatology/ken083
124. Montes A, Perez-Pampin E, Navarro-Sarabia F, Moreira V, de la Serna AR, Magallares B, et al. Genomics Study: Rheumatoid Arthritis Response to Treatment Across IgG1 Allotype – Anti-TNF Incompatibility: A Case-Only Study. Arthritis Res Ther (2015) 17(1):63. doi: 10.1186/s13075-015-0571-z
125. Buch MH, Seto Y, Bingham SJ, Bejarano V, Bryer D, White J, et al. C-Reactive Protein as a Predictor of Infliximab Treatment Outcome in Patients With Rheumatoid Arthritis: Defining Subtypes of Nonresponse and Subsequent Response to Etanercept. Arthritis Rheum (2005) 52(1):42–8. doi: 10.1002/art.20711
126. Canete JD, Suarez B, Hernandez MV, Sanmarti R, Rego I, Celis R, et al. Influence of Variants of Fc Gamma Receptors IIA and IIIA on the American College of Rheumatology and European League Against Rheumatism Responses to Anti-Tumour Necrosis Factor Alpha Therapy in Rheumatoid Arthritis. Ann Rheum Dis (2009) 68(10):1547–52. doi: 10.1136/ard.2008.096982
127. Lourido L, Ruiz-Romero C, Picchi F, Diz-Rosales N, Vilaboa-Galan S, Fernandez-Lopez C, et al. Association of Serum Anti-Centromere Protein F Antibodies With Clinical Response to Infliximab in Patients With Rheumatoid Arthritis: A Prospective Study. Semin Arthritis Rheum (2020) 50(5):1101–8. doi: 10.1016/j.semarthrit.2020.06.010
128. Wijbrandts CA, Dijkgraaf MG, Kraan MC, Vinkenoog M, Smeets TJ, Dinant H, et al. The Clinical Response to Infliximab in Rheumatoid Arthritis is in Part Dependent on Pretreatment Tumour Necrosis Factor Alpha Expression in the Synovium. Ann Rheum Dis (2008) 67(8):1139–44. doi: 10.1136/ard.2007.080440
129. Julia A, Erra A, Palacio C, Tomas C, Sans X, Barcelo P, et al. An Eight-Gene Blood Expression Profile Predicts the Response to Infliximab in Rheumatoid Arthritis. PloS One (2009) 4(10):e7556. doi: 10.1371/journal.pone.0007556
130. Mugnier B, Balandraud N, Darque A, Roudier C, Roudier J, Reviron D. Polymorphism at Position –308 of the Tumor Necrosis Factor α Gene Influences Outcome of Infliximab Therapy in Rheumatoid Arthritis. Arthritis Rheumatism (2003) 48(7):1849–52. doi: 10.1002/art.11168
131. Seitz M, Wirthmüller U, Möller B, Villiger PM. The -308 Tumour Necrosis Factor-Alpha Gene Polymorphism Predicts Therapeutic Response to TNFalpha-Blockers in Rheumatoid Arthritis and Spondyloarthritis Patients. Rheumatol (Oxford) (2007) 46(1):93–6. doi: 10.1093/rheumatology/kel175
132. Zeng Z, Duan Z, Zhang T, Wang S, Li G, Gao J, et al. Association Between Tumor Necrosis Factor-α (TNF-α) Promoter -308 G/A and Response to TNF-α Blockers in Rheumatoid Arthritis: A Meta-Analysis. Mod Rheumatol (2013) 23(3):489–95. doi: 10.1007/s10165-012-0699-5
133. O’Rielly DD, Roslin NM, Beyene J, Pope A, Rahman P. TNF-α –308 G/A Polymorphism and Responsiveness to TNF-α Blockade Therapy in Moderate to Severe Rheumatoid Arthritis: A Systematic Review and Meta-Analysis. Pharmacogenomics J (2009) 9(3):161–7. doi: 10.1038/tpj.2009.7
134. Rooryck C, Barnetche T, Richez C, Laleye A, Arveiler B, Schaeverbeke T. Influence of FCGR3A-V212F and TNFRSF1B-M196R Genotypes in Patients With Rheumatoid Arthritis Treated With Infliximab Therapy. Clin Exp Rheumatol (2008) 26(2):340–2.
135. Canet LM, Filipescu I, Cáliz R, Lupiañez CB, Canhão H, Escudero A, et al. Genetic Variants Within the TNFRSF1B Gene and Susceptibility to Rheumatoid Arthritis and Response to Anti-TNF Drugs: A Multicenter Study. Pharmacogenet Genomics (2015) 25(7):323–33. doi: 10.1097/fpc.0000000000000140
136. Fabris M, Tolusso B, Di Poi E, Assaloni R, Sinigaglia L, Ferraccioli G. Tumor Necrosis Factor-Alpha Receptor II Polymorphism in Patients From Southern Europe With Mild-Moderate and Severe Rheumatoid Arthritis. J Rheumatol (2002) 29(9):1847–50.
137. Ongaro A, De Mattei M, Pellati A, Caruso A, Ferretti S, Masieri FF, et al. Can Tumor Necrosis Factor Receptor II Gene 676T>G Polymorphism Predict the Response Grading to Anti-TNFalpha Therapy in Rheumatoid Arthritis? Rheumatol Int (2008) 28(9):901–8. doi: 10.1007/s00296-008-0552-5
138. Swierkot J, Bogunia-Kubik K, Nowak B, Bialowas K, Korman L, Gebura K, et al. Analysis of Associations Between Polymorphisms Within Genes Coding for Tumour Necrosis Factor (TNF)-Alpha and TNF Receptors and Responsiveness to TNF-Alpha Blockers in Patients With Rheumatoid Arthritis. Joint Bone Spine (2015) 82(2):94–9. doi: 10.1016/j.jbspin.2014.08.006
139. Morales-Lara MJ, Conesa-Zamora P, Garcia-Simon MS, Pedrero F, Santaclara V, Perez-Guillermo M, et al. Association Between the FCGR3A V158F Polymorphism and the Clinical Response to Infliximab in Rheumatoid Arthritis and Spondyloarthritis Patients. Scand J Rheumatol (2010) 39(6):518–20. doi: 10.3109/03009741003781969
140. Seitz M, Wirthmüller U, Möller B, Villiger PM. The –308 Tumour Necrosis Factor-α Gene Polymorphism Predicts Therapeutic Response to TNFα-Blockers in Rheumatoid Arthritis and Spondyloarthritis Patients. Rheumatology (2007) 46(1):93–6. doi: 10.1093/rheumatology/kel175
141. Guis S, Balandraud N, Bouvenot J, Auger I, Toussirot E, Wendling D, et al. Influence of –308 a/G Polymorphism in the Tumor Necrosis Factor α Gene on Etanercept Treatment in Rheumatoid Arthritis. Arthritis Care Res (2007) 57(8):1426–30. doi: 10.1002/art.23092
142. Ferreiro-Iglesias A, Montes A, Perez-Pampin E, Canete JD, Raya E, Magro-Checa C, et al. Evaluation of 12 GWAS-Drawn SNPs as Biomarkers of Rheumatoid Arthritis Response to TNF Inhibitors. A Potential SNP Association With Response to Etanercept. PloS One (2019) 14(2):e0213073. doi: 10.1371/journal.pone.0213073
143. Schotte H, Schluter B, Drynda S, Willeke P, Tidow N, Assmann G, et al. Interleukin 10 Promoter Microsatellite Polymorphisms are Associated With Response to Long Term Treatment With Etanercept in Patients With Rheumatoid Arthritis. Ann Rheum Dis (2005) 64(4):575–81. doi: 10.1136/ard.2004.027672
144. Padyukov L, Lampa J, Heimburger M, Ernestam S, Cederholm T, Lundkvist I, et al. Genetic Markers for the Efficacy of Tumour Necrosis Factor Blocking Therapy in Rheumatoid Arthritis. Ann Rheum Dis (2003) 62(6):526–9. doi: 10.1136/ard.62.6.526
145. Plant D, Webster A, Nair N, Oliver J, Smith SL, Eyre S, et al. Differential Methylation as a Biomarker of Response to Etanercept in Patients With Rheumatoid Arthritis. Arthritis Rheumatol (2016) 68(6):1353–60. doi: 10.1002/art.39590
146. Jančić I, Šefik-Bukilica M, Živojinović S, Damjanov N, Spasovski V, Kotur N, et al. Influence of Promoter Polymorphisms of the TNF-α (-308g/A) and IL-6 (-174g/C) Genes on Therapeutic Response to Etanercept in Rheumatoid Arthritis. J Med Biochem (2015) 34(4):414–21. doi: 10.2478/jomb-2014-0060
147. Jančić I, Arsenović-Ranin N, Šefik-Bukilica M, Živojinović S, Damjanov N, Spasovski V, et al. -174g/C Interleukin-6 Gene Promoter Polymorphism Predicts Therapeutic Response to Etanercept in Rheumatoid Arthritis. Rheumatol Int (2013) 33(6):1481–6. doi: 10.1007/s00296-012-2586-y
148. Dávila-Fajardo CL, Márquez A, Pascual-Salcedo D, Moreno Ramos MJ, García-Portales R, Magro C, et al. Confirmation of –174G/C Interleukin-6 Gene Promoter Polymorphism as a Genetic Marker Predicting Antitumor Necrosis Factor Treatment Outcome. Pharmacogenet Genomics (2014) 24(1):1–5. doi: 10.1097/FPC.0000000000000013
149. Cui J, Stahl EA, Saevarsdottir S, Miceli C, Diogo D, Trynka G, et al. Genome-Wide Association Study and Gene Expression Analysis Identifies CD84 as a Predictor of Response to Etanercept Therapy in Rheumatoid Arthritis. PloS Genet (2013) 9(3):e1003394. doi: 10.1371/journal.pgen.1003394
150. Priori R, Casadei L, Valerio M, Scrivo R, Valesini G, Manetti C. (1)H-NMR-Based Metabolomic Study for Identifying Serum Profiles Associated With the Response to Etanercept in Patients With Rheumatoid Arthritis. PloS One (2015) 10(11):e0138537. doi: 10.1371/journal.pone.0138537
151. Zhang B, Jiang W. IL-1β, IL-17a, CRP and Biologics History Might Serve as Potential Markers for Clinical Response to Etanercept in Rheumatoid Arthritis Patients. Inflammopharmacology (2019) 27(6):1123–30. doi: 10.1007/s10787-019-00624-2
152. Ling SF, Nair N, Verstappen SMM, Barton A, Zucht H-D, Budde P, et al. Proteomic Analysis to Define Predictors of Treatment Response to Adalimumab or Methotrexate in Rheumatoid Arthritis Patients. Pharmacogenomics J (2020) 20(3):516–23. doi: 10.1038/s41397-019-0139-4
153. Vaisman-Mentesh A, Gutierrez-Gonzalez M, DeKosky BJ, Wine Y. The Molecular Mechanisms That Underlie the Immune Biology of Anti-Drug Antibody Formation Following Treatment With Monoclonal Antibodies. Front Immunol (2020) 11:1951(1951). doi: 10.3389/fimmu.2020.01951
154. Bartelds GM, de Groot E, Nurmohamed MT, Hart MH, van Eede PH, Wijbrandts CA, et al. Surprising Negative Association Between IgG1 Allotype Disparity and Anti-Adalimumab Formation: A Cohort Study. Arthritis Res Ther (2010) 12(6):R221. doi: 10.1186/ar3208
155. Sokolove J, Schiff M, Fleischmann R, Weinblatt ME, Connolly SE, Johnsen A, et al. Impact of Baseline Anti-Cyclic Citrullinated Peptide-2 Antibody Concentration on Efficacy Outcomes Following Treatment With Subcutaneous Abatacept or Adalimumab: 2-Year Results From the AMPLE Trial. Ann Rheum Dis (2016) 75(4):709–14. doi: 10.1136/annrheumdis-2015-207942
156. Magill L, Adriani M, Berthou V, Chen K, Gleizes A, Hacein-Bey-Abina S, et al. Low Percentage of Signal Regulatory Protein Alpha/Beta(+) Memory B Cells in Blood Predicts Development of Anti-Drug Antibodies (ADA) in Adalimumab-Treated Rheumatoid Arthritis Patients. Front Immunol (2018) 9:2865. doi: 10.3389/fimmu.2018.02865
157. Kanbe K, Chiba J, Nakamura A. Decrease of CD68 and MMP-3 Expression in Synovium by Treatment of Adalimumab for Rheumatoid Arthritis. Int J Rheum Dis (2011) 14(3):261–6. doi: 10.1111/j.1756-185X.2011.01643.x
158. Potter C, Hyrich KL, Tracey A, Lunt M, Plant D, Symmons DP, et al. Association of Rheumatoid Factor and Anti-Cyclic Citrullinated Peptide Positivity, But Not Carriage of Shared Epitope or PTPN22 Susceptibility Variants, With Anti-Tumour Necrosis Factor Response in Rheumatoid Arthritis. Ann Rheum Dis (2009) 68(1):69–74. doi: 10.1136/ard.2007.084715
159. Aerts NE, De Knop KJ, Leysen J, Ebo DG, Bridts CH, Weyler JJ, et al. Increased IL-17 Production by Peripheral T Helper Cells After Tumour Necrosis Factor Blockade in Rheumatoid Arthritis is Accompanied by Inhibition of Migration-Associated Chemokine Receptor Expression. Rheumatol (Oxford) (2010) 49(12):2264–72. doi: 10.1093/rheumatology/keq224
160. Lee HN, Kim YK, Kim GT, Ahn E, So MW, Sohn DH, et al. Neutrophil-To-Lymphocyte and Platelet-to-Lymphocyte Ratio as Predictors of 12-Week Treatment Response and Drug Persistence of Anti-Tumor Necrosis Factor-Alpha Agents in Patients With Rheumatoid Arthritis: A Retrospective Chart Review Analysis. Rheumatol Int (2019) 39(5):859–68. doi: 10.1007/s00296-019-04276-x
161. Miceli-Richard C, Comets E, Verstuyft C, Tamouza R, Loiseau P, Ravaud P, et al. A Single Tumour Necrosis Factor Haplotype Influences the Response to Adalimumab in Rheumatoid Arthritis. Ann Rheum Dis (2008) 67(4):478–84. doi: 10.1136/ard.2007.074104
162. Han BK, Kuzin I, Gaughan JP, Olsen NJ, Bottaro A. Baseline CXCL10 and CXCL13 Levels are Predictive Biomarkers for Tumor Necrosis Factor Inhibitor Therapy in Patients With Moderate to Severe Rheumatoid Arthritis: A Pilot, Prospective Study. Arthritis Res Ther (2016) 18:93. doi: 10.1186/s13075-016-0995-0
163. Stuhlmuller B, Haupl T, Hernandez MM, Grutzkau A, Kuban RJ, Tandon N, et al. CD11c as a Transcriptional Biomarker to Predict Response to Anti-TNF Monotherapy With Adalimumab in Patients With Rheumatoid Arthritis. Clin Pharmacol Ther (2010) 87(3):311–21. doi: 10.1038/clpt.2009.244
164. Choi IY, Gerlag DM, Herenius MJ, Thurlings RM, Wijbrandts CA, Foell D, et al. MRP8/14 Serum Levels as a Strong Predictor of Response to Biological Treatments in Patients With Rheumatoid Arthritis. Ann Rheum Dis (2015) 74(3):499–505. doi: 10.1136/annrheumdis-2013-203923
165. Dennis G Jr., Holweg CT, Kummerfeld SK, Choy DF, Setiadi AF, Hackney JA, et al. Synovial Phenotypes in Rheumatoid Arthritis Correlate With Response to Biologic Therapeutics. Arthritis Res Ther (2014) 16(2):R90. doi: 10.1186/ar4555
166. Cuchacovich M, Soto L, Edwardes M, Gutierrez M, Llanos C, Pacheco D, et al. Tumour Necrosis Factor (TNF)alpha -308 G/G Promoter Polymorphism and TNFalpha Levels Correlate With a Better Response to Adalimumab in Patients With Rheumatoid Arthritis. Scand J Rheumatol (2006) 35(6):435–40. doi: 10.1080/03009740600904284
167. Dávila-Fajardo CL, van der Straaten T, Baak-Pablo R, Medarde Caballero C, Cabeza Barrera J, Huizinga TW, et al. FcGR Genetic Polymorphisms and the Response to Adalimumab in Patients With Rheumatoid Arthritis. Pharmacogenomics (2015) 16(4):373–81. doi: 10.2217/pgs.14.178
168. Dávila-Fajardo CL, Márquez A, Pascual-Salcedo D, Moreno Ramos MJ, García-Portales R, Magro C, et al. Confirmation of -174G/C Interleukin-6 Gene Promoter Polymorphism as a Genetic Marker Predicting Antitumor Necrosis Factor Treatment Outcome. Pharmacogenet Genomics (2014) 24(1):1–5. doi: 10.1097/fpc.0000000000000013
169. Berkhout LC, Vogelzang EH, Hart MM, Loeff FC, Dijk L, Derksen NIL, et al. The Effect of Certolizumab Drug Concentration and Anti-Drug Antibodies on TNF Neutralisation. Clin Exp Rheumatol (2020) 38(2):306–13. doi: 10.1136/annrheumdis-2018-eular.4399
170. Takeuchi T, Yamamoto K, Yamanaka H, Ishiguro N, Tanaka Y, Eguchi K, et al. Early Response to Certolizumab Pegol Predicts Long-Term Outcomes in Patients With Active Rheumatoid Arthritis: Results From the Japanese Studies. Mod Rheumatol (2015) 25(1):11–20. doi: 10.3109/14397595.2014.904475
171. van der Heijde D, Keystone EC, Curtis JR, Landewé RB, Schiff MH, Khanna D, et al. Timing and Magnitude of Initial Change in Disease Activity Score 28 Predicts the Likelihood of Achieving Low Disease Activity at 1 Year in Rheumatoid Arthritis Patients Treated With Certolizumab Pegol: A Post-Hoc Analysis of the RAPID 1 Trial. J Rheumatol (2012) 39(7):1326–33. doi: 10.3899/jrheum.111171
172. Weinblatt ME, Fleischmann R, van Vollenhoven RF, Emery P, Huizinga TW, Cutolo M, et al. Twenty-Eight-Week Results From the REALISTIC Phase IIIb Randomized Trial: Efficacy, Safety and Predictability of Response to Certolizumab Pegol in a Diverse Rheumatoid Arthritis Population. Arthritis Res Ther (2015) 17:325. doi: 10.1186/s13075-015-0841-9
173. Saraux A, Flipo R-M, Fagnani F, Massol J, Cukierman G, Joubert J-M, et al. Early non-Response to Certolizumab Pegol in Rheumatoid Arthritis Predicts Failure to Achieve Low Disease Activity at 1 Year: Data From a Prospective Observational Study. RMD Open (2020) 6(1):e000991. doi: 10.1136/rmdopen-2019-000991
174. Wampler Muskardin T, Vashisht P, Dorschner JM, Jensen MA, Chrabot BS, Kern M, et al. Increased Pretreatment Serum IFN-β/α Ratio Predicts non-Response to Tumour Necrosis Factor α Inhibition in Rheumatoid Arthritis. Ann Rheum Dis (2016) 75(10):1757–62. doi: 10.1136/annrheumdis-2015-208001
175. Wampler Muskardin TL, Fan W, Jin Z, Jensen MA, Dorschner JM, Ghodke-Puranik Y, et al. Distinct Single Cell Gene Expression in Peripheral Blood Monocytes Correlates With Tumor Necrosis Factor Inhibitor Treatment Response Groups Defined by Type I Interferon in Rheumatoid Arthritis. Front Immunol (2020) 11:1384(1384). doi: 10.3389/fimmu.2020.01384
176. Gehin JE, Warren DJ, Syversen SW, Lie E, Sexton J, Loli L, et al. Serum Golimumab Concentration and Anti-Drug Antibodies are Associated With Treatment Response and Drug Survival in Patients With Inflammatory Joint Diseases: Data From the NOR-DMARD Study. Scand J Rheumatol (2021) 50(6):445–54. doi: 10.1080/03009742.2021.1875040
177. Guo X, Wang S, Godwood A, Close D, Ryan PC, Roskos LK, et al. Pharmacodynamic Biomarkers and Differential Effects of TNF- and GM-CSF-Targeting Biologics in Rheumatoid Arthritis. Int J Rheum Dis (2019) 22(4):646–53. doi: 10.1111/1756-185X.13395
178. Visvanathan S, Wagner C, Rojas J, Kay J, Dasgupta B, Matteson EL, et al. E-Selectin, Interleukin 18, Serum Amyloid a, and Matrix Metalloproteinase 9 are Associated With Clinical Response to Golimumab Plus Methotrexate in Patients With Active Rheumatoid Arthritis Despite Methotrexate Therapy. J Rheumatol (2009) 36(7):1371–9. doi: 10.3899/jrheum.080755
179. Vastesaeger N, Kutzbach AG, Amital H, Pavelka K, Lazaro MA, Moots RJ, et al. Prediction of Remission and Low Disease Activity in Disease-Modifying Anti-Rheumatic Drug-Refractory Patients With Rheumatoid Arthritis Treated With Golimumab. Rheumatol (Oxford) (2016) 55(8):1466–76. doi: 10.1093/rheumatology/kew179
180. Buch MH, Bingham SJ, Bejarano V, Bryer D, White J, Emery P, et al. Therapy of Patients With Rheumatoid Arthritis: Outcome of Infliximab Failures Switched to Etanercept. Arthritis Care Res (2007) 57(3):448–53. doi: 10.1002/art.22617
181. Burmester GR, Mariette X, Montecucco C, Monteagudo-Sáez I, Malaise M, Tzioufas AG, et al. Adalimumab Alone and in Combination With Disease-Modifying Antirheumatic Drugs for the Treatment of Rheumatoid Arthritis in Clinical Practice: The Research in Active Rheumatoid Arthritis (ReAct) Trial. Ann Rheum Dis (2007) 66(6):732–9. doi: 10.1136/ard.2006.066761
182. Weinblatt ME, Fleischmann R, Huizinga TW, Emery P, Pope J, Massarotti EM, et al. Efficacy and Safety of Certolizumab Pegol in a Broad Population of Patients With Active Rheumatoid Arthritis: Results From the REALISTIC Phase IIIb Study. Rheumatol (Oxford) (2012) 51(12):2204–14. doi: 10.1093/rheumatology/kes150
183. Haraoui B, Keystone EC, Thorne JC, Pope JE, Chen I, Asare CG, et al. Clinical Outcomes of Patients With Rheumatoid Arthritis After Switching From Infliximab to Etanercept. J Rheumatol (2004) 31(12):2356–9. doi: 10.1093/rheumatology/kes115
184. Marshall JC. Such Stuff as Dreams are Made on: Mediator-Directed Therapy in Sepsis. Nat Rev Drug Discovery (2003) 2(5):391–405. doi: 10.1038/nrd1084
185. Azevedo VF, Galli N, Kleinfelder A, D’Ippolito J, Urbano PCM. Etanercept Biosimilars. Rheumatol Int (2015) 35(2):197–209. doi: 10.1007/s00296-014-3080-5
186. Moreland LW, Baumgartner SW, Schiff MH, Tindall EA, Fleischmann RM, Weaver AL, et al. Treatment of Rheumatoid Arthritis With a Recombinant Human Tumor Necrosis Factor Receptor (P75)–Fc Fusion Protein. New Engl J Med (1997) 337(3):141–7. doi: 10.1056/NEJM199707173370301
187. Haraoui B, Bykerk V. Etanercept in the Treatment of Rheumatoid Arthritis. Ther Clin Risk Manage (2007) 3(1):99–105. doi: 10.2147/tcrm.2007.3.1.99
188. Cao Z, Ding X, Huang Y, Liu S, Lin J, Lyu H, et al. Etanercept Inhibits Synovial Inflammation and Reduces the Expression of Adhesion Related Molecules in Synovial Tissues of Patients With Rheumatoid Arthritis. Xi Bao Yu Fen Zi Mian Yi Xue Za Zhi (2015) 31(4):511–5, 519.
189. Degboé Y, Rauwel B, Baron M, Boyer J-F, Ruyssen-Witrand A, Constantin A, et al. Polarization of Rheumatoid Macrophages by TNF Targeting Through an IL-10/STAT3 Mechanism. Front Immunol (2019) 10:3(3). doi: 10.3389/fimmu.2019.00003
190. Catrina AI, Lampa J, Ernestam S, af Klint E, Bratt J, Klareskog L, et al. Anti-Tumour Necrosis Factor (TNF)-Alpha Therapy (Etanercept) Down-Regulates Serum Matrix Metalloproteinase (MMP)-3 and MMP-1 in Rheumatoid Arthritis. Rheumatol (Oxford) (2002) 41(5):484–9. doi: 10.1093/rheumatology/41.5.484
191. Moreland LW, Schiff MH, Baumgartner SW, Tindall EA, Fleischmann RM, Bulpitt KJ, et al. Etanercept Therapy in Rheumatoid Arthritis. A Randomized, Controlled Trial. Ann Intern Med (1999) 130(6):478–86. doi: 10.7326/0003-4819-130-6-199903160-00004
192. Bathon JM, Martin RW, Fleischmann RM, Tesser JR, Schiff MH, Keystone EC, et al. A Comparison of Etanercept and Methotrexate in Patients With Early Rheumatoid Arthritis. N Engl J Med (2000) 343(22):1586–93. doi: 10.1056/nejm200011303432201
193. Moots RJ, Xavier RM, Mok CC, Rahman MU, Tsai WC, Al-Maini MH, et al. The Impact of Anti-Drug Antibodies on Drug Concentrations and Clinical Outcomes in Rheumatoid Arthritis Patients Treated With Adalimumab, Etanercept, or Infliximab: Results From a Multinational, Real-World Clinical Practice, non-Interventional Study. PloS One (2017) 12(4):e0175207. doi: 10.1371/journal.pone.0175207
194. Anderson PJ. Tumor Necrosis Factor Inhibitors: Clinical Implications of Their Different Immunogenicity Profiles. Semin Arthritis Rheum (2005) 34(5 Suppl1):19–22. doi: 10.1016/j.semarthrit.2005.01.005
195. Herszényi K, Jókai H, Rencz F, Brodszky V, Nagy E, Holló P. Antidrug Antibody Formation During Tumor Necrosis Factor α Inhibitor Treatment of Severe Psoriatic Patients in the Real-Life Practice. Postepy dermatologii i alergologii (2019) 36(5):589–94. doi: 10.5114/ada.2019.89507
196. van Schouwenburg PA, Rispens T, Wolbink GJ. Immunogenicity of Anti-TNF Biologic Therapies for Rheumatoid Arthritis. Nat Rev Rheumatol (2013) 9(3):164–72. doi: 10.1038/nrrheum.2013.4
197. Jamnitski A, Krieckaert CL, Nurmohamed MT, Hart MH, Dijkmans BA, Aarden L, et al. Patients Non-Responding to Etanercept Obtain Lower Etanercept Concentrations Compared With Responding Patients. Ann Rheum Dis (2012) 71(1):88–91. doi: 10.1136/annrheumdis-2011-200184
198. Kim MS, Lee SH, Song MY, Yoo TH, Lee BK, Kim YS. Comparative Analyses of Complex Formation and Binding Sites Between Human Tumor Necrosis Factor-Alpha and its Three Antagonists Elucidate Their Different Neutralizing Mechanisms. J Mol Biol (2007) 374(5):1374–88. doi: 10.1016/j.jmb.2007.10.034
199. Kang CP, Lee KW, Yoo DH, Kang C, Bae SC. The Influence of a Polymorphism at Position –857 of the Tumour Necrosis Factor α Gene on Clinical Response to Etanercept Therapy in Rheumatoid Arthritis. Rheumatology (2005) 44(4):547–52. doi: 10.1093/rheumatology/keh550
200. Kehrl JH, Wakefield LM, Roberts AB, Jakowlew S, Alvarez-Mon M, Derynck R, et al. Production of Transforming Growth Factor Beta by Human T Lymphocytes and its Potential Role in the Regulation of T Cell Growth. J Exp Med (1986) 163(5):1037–50. doi: 10.1084/jem.163.5.1037
201. Rafiei A, Hajilooi M, Shakib RJ, Alavi SA. Transforming Growth Factor-Beta1 Polymorphisms in Patients With Brucellosis: An Association Between Codon 10 and 25 Polymorphisms and Brucellosis. Clin Microbiol Infect (2007) 13(1):97–100. doi: 10.1111/j.1469-0691.2006.01575.x
202. Tountas NA, Casini-Raggi V, Yang H, Di Giovine FS, Vecchi M, Kam L, et al. Functional and Ethnic Association of Allele 2 of the Interleukin-1 Receptor Antagonist Gene in Ulcerative Colitis. Gastroenterology (1999) 117(4):806–13. doi: 10.1016/s0016-5085(99)70338-0
203. Schrijver HM, van As J, Crusius JB, Dijkstra CD, Uitdehaag BM. Interleukin (IL)-1 Gene Polymorphisms: Relevance of Disease Severity Associated Alleles With IL-1beta and IL-1ra Production in Multiple Sclerosis. Mediators Inflammation (2003) 12(2):89–94. doi: 10.1080/0962933031000097691
204. Huang CM, Wu MC, Wu JY, Tsai FJ. Interleukin-1 Receptor Antagonist Gene Polymorphism in Chinese Patients With Systemic Lupus Erythematosus. Clin Rheumatol (2002) 21(3):255–7. doi: 10.1007/s10067-002-8292-5
205. Boucher P, Li WP, Matz RL, Takayama Y, Auwerx J, Anderson RG, et al. LRP1 Functions as an Atheroprotective Integrator of TGFbeta and PDFG Signals in the Vascular Wall: Implications for Marfan Syndrome. PloS One (2007) 2(5):e448. doi: 10.1371/journal.pone.0000448
206. Ciechomska M, Roszkowski L, Maslinski W. DNA Methylation as a Future Therapeutic and Diagnostic Target in Rheumatoid Arthritis. Cells (2019) 8(9):953. doi: 10.3390/cells8090953
207. Furst DE, Gaylis N, Bray V, Olech E, Yocum D, Ritter J, et al. Open-Label, Pilot Protocol of Patients With Rheumatoid Arthritis Who Switch to Infliximab After an Incomplete Response to Etanercept: The Opposite Study. Ann Rheum Dis (2007) 66(7):893–9. doi: 10.1136/ard.2006.068304
208. Smolen JS, Kay J, Doyle MK, Landewé R, Matteson EL, Wollenhaupt J, et al. Golimumab in Patients With Active Rheumatoid Arthritis After Treatment With Tumour Necrosis Factor α Inhibitors (GO-AFTER Study): A Multicentre, Randomised, Double-Blind, Placebo-Controlled, Phase III Trial. Lancet (2009) 374(9685):210–21. doi: 10.1016/S0140-6736(09)60506-7
209. Smolen JS, Kay J, Doyle M, Landewé R, Matteson EL, Gaylis N, et al. Golimumab in Patients With Active Rheumatoid Arthritis After Treatment With Tumor Necrosis Factor α Inhibitors: Findings With Up to Five Years of Treatment in the Multicenter, Randomized, Double-Blind, Placebo-Controlled, Phase 3 GO-AFTER Study. Arthritis Res Ther (2015) 17(1):14–4. doi: 10.1186/s13075-015-0516-6
210. van Vollenhoven R, Harju A, Brannemark S, Klareskog L. Treatment With Infliximab (Remicade) When Etanercept (Enbrel) has Failed or Vice Versa: Data From the STURE Registry Showing That Switching Tumour Necrosis Factor Alpha Blockers can Make Sense. Ann Rheum Dis (2003) 62(12):1195–8. doi: 10.1136/ard.2003.009589
211. Mease PJ. Adalimumab in the Treatment of Arthritis. Ther Clin Risk Manage (2007) 3(1):133–48. doi: 10.2147/tcrm.2007.3.1.133
212. Catrina AI, af Klint E, Ernestam S, Catrina SB, Makrygiannakis D, Botusan IR, et al. Ulfgren: Anti-Tumor Necrosis Factor Therapy Increases Synovial Osteoprotegerin Expression in Rheumatoid Arthritis. Arthritis Rheum (2006) 54(1):76–81. doi: 10.1002/art.21528
213. Breedveld FC, Weisman MH, Kavanaugh AF, Cohen SB, Pavelka K, van Vollenhoven R, et al. The PREMIER Study: A Multicenter, Randomized, Double-Blind Clinical Trial of Combination Therapy With Adalimumab Plus Methotrexate Versus Methotrexate Alone or Adalimumab Alone in Patients With Early, Aggressive Rheumatoid Arthritis Who had Not had Previous Methotrexate Treatment. Arthritis Rheum (2006) 54(1):26–37. doi: 10.1002/art.21519
214. Zhang J, Jin S, Guo X, Qian W. Targeting the CD47-Sirpα Signaling Axis: Current Studies on B-Cell Lymphoma Immunotherapy. J Int Med Res (2018) 46(11):4418–26. doi: 10.1177/0300060518799612
215. Quistrebert J, Hässler S, Bachelet D, Mbogning C, Musters A, Tak PP, et al. Incidence and Risk Factors for Adalimumab and Infliximab Anti-Drug Antibodies in Rheumatoid Arthritis: A European Retrospective Multicohort Analysis. Semin Arthritis Rheum (2019) 48(6):967–75. doi: 10.1016/j.semarthrit.2018.10.006
216. Lee YH, Ji JD, Bae SC, Song GG. Associations Between Tumor Necrosis Factor-Alpha (TNF-Alpha) -308 and -238 G/A Polymorphisms and Shared Epitope Status and Responsiveness to TNF-Alpha Blockers in Rheumatoid Arthritis: A Metaanalysis Update. J Rheumatol (2010) 37(4):740–6. doi: 10.3899/jrheum.090707
217. Pavy S, Toonen EJ, Miceli-Richard C, Barrera P, van Riel PL, Criswell LA, et al. Tumour Necrosis Factor Alpha -308G->A Polymorphism is Not Associated With Response to TNFalpha Blockers in Caucasian Patients With Rheumatoid Arthritis: Systematic Review and Meta-Analysis. Ann Rheum Dis (2010) 69(6):1022–8. doi: 10.1136/ard.2009.117622
218. Soto L, Sabugo F, Catalan D, Wurmann P, Cermenatti T, Gatica H, et al. The Presence of Anti-Citrullinated Protein Antibodies (ACPA) Does Not Affect the Clinical Response to Adalimumab in a Group of RA Patients With the Tumor Necrosis Factor (TNF) α-308 G/G Promoter Polymorphism. Clin Rheumatol (2011) 30(3):391–5. doi: 10.1007/s10067-011-1679-4
219. Tanaka Y, Fautrel B, Keystone EC, Ortmann RA, Xie L, Zhu B, et al. Clinical Outcomes in Patients Switched From Adalimumab to Baricitinib Due to non-Response and/or Study Design: Phase III Data in Patients With Rheumatoid Arthritis. Ann Rheum Dis (2019) 78(7):890–8. doi: 10.1136/annrheumdis-2018-214529
220. Bessette L, Khraishi M, Kivitz AJ, Kaliyaperumal A, Grantab R, Poulin-Costello M, et al. Single-Arm Study of Etanercept in Adult Patients With Moderate to Severe Rheumatoid Arthritis Who Failed Adalimumab Treatment. Rheumatol Ther (2017) 4(2):391–404. doi: 10.1007/s40744-017-0079-x
221. Fleischmann R, Pangan AL, Song IH, Mysler E, Bessette L, Peterfy C, et al. Upadacitinib Versus Placebo or Adalimumab in Patients With Rheumatoid Arthritis and an Inadequate Response to Methotrexate: Results of a Phase III, Double-Blind, Randomized Controlled Trial. Arthritis Rheumatol (2019) 71(11):1788–800. doi: 10.1002/art.41032
222. Pasut G. Pegylation of Biological Molecules and Potential Benefits: Pharmacological Properties of Certolizumab Pegol. BioDrugs (2014) 28 Suppl:1, S15–23. doi: 10.1007/s40259-013-0064-z
223. Lim H, Lee SH, Lee HT, Lee JU, Son JY, Shin W, et al. Structural Biology of the TNFα Antagonists Used in the Treatment of Rheumatoid Arthritis. Int J Mol Sci (2018) 19(3):768. doi: 10.3390/ijms19030768
224. Fleischmann R, Vencovsky J, van Vollenhoven RF, Borenstein D, Box J, Coteur G, et al. Efficacy and Safety of Certolizumab Pegol Monotherapy Every 4 Weeks in Patients With Rheumatoid Arthritis Failing Previous Disease-Modifying Antirheumatic Therapy: The FAST4WARD Study. Ann Rheum Dis (2009) 68(6):805–11. doi: 10.1136/ard.2008.099291
225. van Schie K, Hart M, Groot E, Kruithof S, Aarden LA, Wolbink GJ, et al. Response to: ‘The Antibody Response Against Human and Chimeric Anti-TNF Therapeutic Antibodies Primarily Targets the TNF Binding Region’ by Rinaudo-Gaujous Et al. Ann Rheum Dis (2014) 74(8):e41. doi: 10.1136/annrheumdis-2014-206237
226. de Weerd NA, Vivian JP, Nguyen TK, Mangan NE, Gould JA, Braniff SJ, et al. Structural Basis of a Unique Interferon-β Signaling Axis Mediated via the Receptor IFNAR1. Nat Immunol (2013) 14(9):901–7. doi: 10.1038/ni.2667
227. Smolen JS, Burmester GR, Combe B, Curtis JR, Hall S, Haraoui B, et al. Head-To-Head Comparison of Certolizumab Pegol Versus Adalimumab in Rheumatoid Arthritis: 2-Year Efficacy and Safety Results From the Randomised EXXELERATE Study. Lancet (2016) 388(10061):2763–74. doi: 10.1016/S0140-6736(16)31651-8
228. Glatt S, Taylor PC, McInnes IB, Schett G, Landewe R, Baeten D, et al. Efficacy and Safety of Bimekizumab as Add-on Therapy for Rheumatoid Arthritis in Patients With Inadequate Response to Certolizumab Pegol: A Proof-of-Concept Study. Ann Rheum Dis (2019) 78(8):1033–40. doi: 10.1136/annrheumdis-2018-214943
229. Campbell J, Lowe D, Sleeman MA. Developing the Next Generation of Monoclonal Antibodies for the Treatment of Rheumatoid Arthritis. Br J Pharmacol (2011) 162(7):1470–84. doi: 10.1111/j.1476-5381.2010.01183.x
230. Pelechas E, Voulgari PV, Drosos AA. Golimumab for Rheumatoid Arthritis. J Clin Med (2019) 8(3):387. doi: 10.3390/jcm8030387
231. Ono M, Horita S, Sato Y, Nomura Y, Iwata S, Nomura N. Structural Basis for Tumor Necrosis Factor Blockade With the Therapeutic Antibody Golimumab. Protein Sci (2018) 27(6):1038–46. doi: 10.1002/pro.3407
232. Stevenson M, Archer R, Tosh J, Simpson E, Everson-Hock E, Stevens J, et al. Adalimumab, Etanercept, Infliximab, Certolizumab Pegol, Golimumab, Tocilizumab and Abatacept for the Treatment of Rheumatoid Arthritis Not Previously Treated With Disease-Modifying Antirheumatic Drugs and After the Failure of Conventional Disease-Modifying Antirheumatic Drugs Only: Systematic Review and Economic Evaluation. Health Technol Assess (2016) 20(35):1–610. doi: 10.3310/hta20350
233. Emery P, Fleischmann RM, Strusberg I, Durez P, Nash P, Amante EJ, et al. Efficacy and Safety of Subcutaneous Golimumab in Methotrexate-Naive Patients With Rheumatoid Arthritis: Five-Year Results of a Randomized Clinical Trial. Arthritis Care Res (Hoboken) (2016) 68(6):744–52. doi: 10.1002/acr.22759
234. Keystone EC, Genovese MC, Hall S, Bae SC, Han C, Gathany TA, et al. Safety and Efficacy of Subcutaneous Golimumab in Patients With Active Rheumatoid Arthritis Despite Methotrexate Therapy: Final 5-Year Results of the GO-FORWARD Trial. J Rheumatol (2016) 43(2):298–306. doi: 10.3899/jrheum.150712
235. Jones PT, Dear PH, Foote J, Neuberger MS, Winter G. Replacing the Complementarity-Determining Regions in a Human Antibody With Those From a Mouse. Nature (1986) 321(6069):522–5. doi: 10.1038/321522a0
236. Zidi I, Bouaziz A, Mnif W, Bartegi A, Al-Hizab FA, Amor NB. Golimumab Therapy of Rheumatoid Arthritis: An Overview. Scand J Immunol (2010) 72(2):75–85. doi: 10.1111/j.1365-3083.2010.02423.x
237. Leu JH, Adedokun OJ, Gargano C, Hsia EC, Xu Z, Shankar G. Immunogenicity of Golimumab and its Clinical Relevance in Patients With Rheumatoid Arthritis, Psoriatic Arthritis and Ankylosing Spondylitis. Rheumatol (Oxford) (2019) 58(3):441–6. doi: 10.1093/rheumatology/key309
238. Nell-Duxneuner V, Reichardt B, Stamm T. AB0312 Real-Life Data on the Use of Biological DMARDS in Rheumatoid Arthritis in Austria With Special Attention to Switching After First BDMARD Failure. Ann Rheum Dis (2020) 79(Suppl 1):1455–5. doi: 10.1136/annrheumdis-2020-eular.5723
239. Esensten JH, Helou YA, Chopra G, Weiss A, Bluestone JA. CD28 Costimulation: From Mechanism to Therapy. Immunity (2016) 44(5):973–88. doi: 10.1016/j.immuni.2016.04.020
240. Fantus S, Ruderman E. Updates in the Treatment of Rheumatoid Arthritis. Curr Treat Options Rheumatol (2021) 7. doi: 10.1007/s40674-021-00173-2
242. Bozec A, Zaiss MM, Kagwiria R, Voll R, Rauh M, Chen Z, et al. T Cell Costimulation Molecules CD80/86 Inhibit Osteoclast Differentiation by Inducing the IDO/tryptophan Pathway. Sci Transl Med (2014) 6(235):235ra60. doi: 10.1126/scitranslmed.3007764
243. Axmann R, Herman S, Zaiss M, Franz S, Polzer K, Zwerina J, et al. CTLA-4 Directly Inhibits Osteoclast Formation. Ann Rheum Dis (2008) 67(11):1603–9. doi: 10.1136/ard.2007.080713
244. Bonelli M, Ferner E, Göschl L, Blüml S, Hladik A, Karonitsch T, et al. Abatacept (CTLA-4IG) Treatment Reduces the Migratory Capacity of Monocytes in Patients With Rheumatoid Arthritis. Arthritis Rheum (2013) 65(3):599–607. doi: 10.1002/art.37787
245. O’Neill SK, Cao Y, Hamel KM, Doodes PD, Hutas G, Finnegan A. Expression of CD80/86 on B Cells is Essential for Autoreactive T Cell Activation and the Development of Arthritis. J Immunol (2007) 179(8):5109–16. doi: 10.4049/jimmunol.179.8.5109
246. Gazeau P, Alegria GC, Devauchelle-Pensec V, Jamin C, Lemerle J, Bendaoud B, et al. Memory B Cells and Response to Abatacept in Rheumatoid Arthritis. Clin Rev Allergy Immunol (2017) 53(2):166–76. doi: 10.1007/s12016-017-8603-x
247. Buch MH, Boyle DL, Rosengren S, Saleem B, Reece RJ, Rhodes LA, et al. Mode of Action of Abatacept in Rheumatoid Arthritis Patients Having Failed Tumour Necrosis Factor Blockade: A Histological, Gene Expression and Dynamic Magnetic Resonance Imaging Pilot Study. Ann Rheum Dis (2009) 68(7):1220–7. doi: 10.1136/ard.2008.091876
248. Scarsi M, Paolini L, Ricotta D, Pedrini A, Piantoni S, Caimi L, et al. Abatacept Reduces Levels of Switched Memory B Cells, Autoantibodies, and Immunoglobulins in Patients With Rheumatoid Arthritis. J Rheumatol (2014) 41(4):666–72. doi: 10.3899/jrheum.130905
249. Genovese MC, Covarrubias A, Leon G, Mysler E, Keiserman M, Valente R, et al. Subcutaneous Abatacept Versus Intravenous Abatacept: A Phase IIIb Noninferiority Study in Patients With an Inadequate Response to Methotrexate. Arthritis Rheum (2011) 63(10):2854–64. doi: 10.1002/art.30463
250. Weinblatt ME, Genovese MC, Schiff MH, Westhovens R, Alten R, Delaet I, et al. FRI0191 Immunogenicity is Low and Transient With Intravenous Abatacept Therapy. Ann Rheum Dis (2013) 71(Suppl 3):377–7. doi: 10.1136/annrheumdis-2012-eular.2648
251. Köller MD. Abatacept: The Evidence for its Place in the Treatment of Rheumatoid Arthritis. Core Evid (2008) 2(3):163–72.
252. Schiff M, Keiserman M, Codding C, Songcharoen S, Berman A, Nayiager S, et al. Efficacy and Safety of Abatacept or Infliximab vs Placebo in ATTEST: A Phase III, Multi-Centre, Randomised, Double-Blind, Placebo-Controlled Study in Patients With Rheumatoid Arthritis and an Inadequate Response to Methotrexate. Ann Rheum Dis (2008) 67(8):1096–103. doi: 10.1136/ard.2007.080002
253. Genovese MC, Schiff M, Luggen M, Becker JC, Aranda R, Teng J, et al. Efficacy and Safety of the Selective Co-Stimulation Modulator Abatacept Following 2 Years of Treatment in Patients With Rheumatoid Arthritis and an Inadequate Response to Anti-Tumour Necrosis Factor Therapy. Ann Rheum Dis (2008) 67(4):547–54. doi: 10.1136/ard.2007.074773
254. Ingegnoli F, Castelli R, Gualtierotti R. Rheumatoid Factors: Clinical Applications. Dis Markers (2013) 35:726598. doi: 10.1155/2013/726598
255. Gottenberg JE, Courvoisier DS, Hernandez MV, Iannone F, Lie E, Canhão H, et al. Brief Report: Association of Rheumatoid Factor and Anti-Citrullinated Protein Antibody Positivity With Better Effectiveness of Abatacept: Results From the Pan-European Registry Analysis. Arthritis Rheumatol (2016) 68(6):1346–52. doi: 10.1002/art.39595
256. Maneiro RJ, Salgado E, Carmona L, Gomez-Reino JJ. Rheumatoid Factor as Predictor of Response to Abatacept, Rituximab and Tocilizumab in Rheumatoid Arthritis: Systematic Review and Meta-Analysis. Semin Arthritis Rheum (2013) 43(1):9–17. doi: 10.1016/j.semarthrit.2012.11.007
257. Alten R, Mariette X, Lorenz HM, Nüßlein H, Galeazzi M, Navarro F, et al. Predictors of Abatacept Retention Over 2 Years in Patients With Rheumatoid Arthritis: Results From the Real-World ACTION Study. Clin Rheumatol (2019) 38(5):1413–24. doi: 10.1007/s10067-019-04449-w
258. Bozec A, Luo Y, Engdahl C, Figueiredo C, Bang H, Schett G. Abatacept Blocks Anti-Citrullinated Protein Antibody and Rheumatoid Factor Mediated Cytokine Production in Human Macrophages in IDO-Dependent Manner. Arthritis Res Ther (2018) 20(1):24. doi: 10.1186/s13075-018-1527-x
259. Ioan-Facsinay A, el-Bannoudi H, Scherer HU, van der Woude D, Ménard HA, Lora M, et al. Anti-CCP Antibodies are a Collection of ACPA That are Cross-Reactive to Multiple Citrullinated Antigens. Ann Rheum Dis (2010) 69(Suppl 2):A8–8. doi: 10.1136/ard.2010.129577s
260. Kay J, Upchurch KS. ACR/EULAR 2010 Rheumatoid Arthritis Classification Criteria. Rheumatology (2012) 51(suppl_6):vi5–9. doi: 10.1093/rheumatology/kes279
261. Puszczewicz M, Iwaszkiewicz C. Role of Anti-Citrullinated Protein Antibodies in Diagnosis and Prognosis of Rheumatoid Arthritis. Arch Med Sci: AMS (2011) 7(2):189–94. doi: 10.5114/aoms.2011.22067
262. Kida D, Takahashi N, Kaneko A, Hirano Y, Fujibayashi T, Kanayama Y, et al. A Retrospective Analysis of the Relationship Between Anti-Cyclic Citrullinated Peptide Antibody and the Effectiveness of Abatacept in Rheumatoid Arthritis Patients. Sci Rep (2020) 10(1):19717. doi: 10.1038/s41598-020-76842-4
263. Huizinga TWJ, Connolly SE, Johnsen A, Zhu J, Furst DE, Bykerk VP, et al. THU0114 Effect of Anti-Cyclic Citrullinated Peptide 2 Immunoglobulin M Serostatus on Efficacy Outcomes Following Treatment With Abatacept Plus Methotrexate in the Avert Trial. Ann Rheum Dis (2015) 74(Suppl 2):234–5. doi: 10.1136/annrheumdis-2015-eular.1983
264. Harrold LR, Litman HJ, Connolly SE, Kelly S, Hua W, Alemao E, et al. Effect of Anticitrullinated Protein Antibody Status on Response to Abatacept or Antitumor Necrosis Factor-α Therapy in Patients With Rheumatoid Arthritis: A US National Observational Study. J Rheumatol (2018) 45(1):32–9. doi: 10.3899/jrheum.170007
265. Alten R, Mariette X, Lorenz H-M, Galeazzi M, Cantagrel A, Nüßlein HG, et al. Real-World Predictors of 12–Month Intravenous Abatacept Retention in Patients With Rheumatoid Arthritis in the ACTION Observational Study. RMD Open (2017) 3(2):e000538. doi: 10.1136/rmdopen-2017-000538
266. Endo Y, Koga T, Kawashiri SY, Morimoto S, Nishino A, Okamoto M, et al. Anti-Citrullinated Protein Antibody Titre as a Predictor of Abatacept Treatment Persistence in Patients With Rheumatoid Arthritis: A Prospective Cohort Study in Japan. Scand J Rheumatol (2020) 49(1):13–7. doi: 10.1080/03009742.2019.1627411
267. Ogawa N, Ohashi H, Ota Y, Kobori K, Suzuki M, Tsuboi S, et al. Multicenter, Observational Clinical Study of Abatacept in Japanese Patients With Rheumatoid Arthritis. Immunol Med (2019) 42(1):29–38. doi: 10.1080/25785826.2019.1605036
268. Scarsi M, Ziglioli T, Airo P. Baseline Numbers of Circulating CD28-Negative T Cells may Predict Clinical Response to Abatacept in Patients With Rheumatoid Arthritis. J Rheumatol (2011) 38(10):2105–11. doi: 10.3899/jrheum.110386
269. Nakamura S, Suzuki K, Iijima H, Hata Y, Lim CR, Ishizawa Y, et al. Identification of Baseline Gene Expression Signatures Predicting Therapeutic Responses to Three Biologic Agents in Rheumatoid Arthritis: A Retrospective Observational Study. Arthritis Res Ther (2016) 18:159. doi: 10.1186/s13075-016-1052-8
270. Marquez Pete N, Maldonado Montoro MDM, Pérez Ramírez C, Sánchez Martín A, Martínez de la Plata JE, Martínez Martínez F, et al. Impact of Single-Nucleotide Polymorphisms of CTLA-4, CD80 and CD86 on the Effectiveness of Abatacept in Patients With Rheumatoid Arthritis. J Personalized Med (2020) 10(4):220. doi: 10.3390/jpm10040220
271. Takahashi S, Saegusa J, Onishi A, Morinobu A. Biomarkers Identified by Serum Metabolomic Analysis to Predict Biologic Treatment Response in Rheumatoid Arthritis Patients. Rheumatol (Oxford) (2019) 58(12):2153–61. doi: 10.1093/rheumatology/kez199
272. Yukawa K, Mokuda S, Kohno H, Oi K, Kuranobu T, Tokunaga T, et al. Serum CXCL10 Levels are Associated With Better Responses to Abatacept Treatment of Rheumatoid Arthritis. Clin Exp Rheumatol (2020) 38(5):956–63.
273. Salomon S, Guignant C, Morel P, Flahaut G, Brault C, Gourguechon C, et al. Th17 and CD24(hi)CD27(+) Regulatory B Lymphocytes are Biomarkers of Response to Biologics in Rheumatoid Arthritis. Arthritis Res Ther (2017) 19(1):33. doi: 10.1186/s13075-017-1244-x
274. Yokoyama-Kokuryo W, Yamazaki H, Takeuchi T, Amano K, Kikuchi J, Kondo T, et al. Identification of Molecules Associated With Response to Abatacept in Patients With Rheumatoid Arthritis. Arthritis Res Ther (2020) 22(1):46. doi: 10.1186/s13075-020-2137-y
275. Shin-Ya K, Yushiro E, Ayako N, Momoko O, Sosuke T, Ayuko T, et al. Association Between Serum Bone Biomarker Levels and Ultrasonographic Response in Abatacepttreated Rheumatoid Arthritis Patients. Res Square (2021) 22(1):506. doi: 10.21203/rs.3.rs-25419/v1
276. Nguyen MVC, Adrait A, Baillet A, Trocmé C, Gottenberg JE, Gaudin P. Identification of Cartilage Oligomeric Matrix Protein as Biomarker Predicting Abatacept Response in Rheumatoid Arthritis Patients With Insufficient Response to a First Anti-TNFα Treatment. Joint Bone Spine (2019) 86(3):401–3. doi: 10.1016/j.jbspin.2018.09.005
277. de Jong TD, Sellam J, Agca R, Vosslamber S, Witte BI, Tsang ASM, et al. A Multi-Parameter Response Prediction Model for Rituximab in Rheumatoid Arthritis. Joint Bone Spine (2018) 85(2):219–26. doi: 10.1016/j.jbspin.2017.02.015
278. Viecceli D, Garcia MP, Schneider L, Alegretti AP, Silva CK, Ribeiro AL, et al. Correlation Between Cellular Expression of Complement Regulatory Proteins With Depletion and Repopulation of B-Lymphocytes in Peripheral Blood of Patients With Rheumatoid Arthritis Treated With Rituximab. Rev Bras Reumatol Engl Ed (2017) 57(5):385–91. doi: 10.1016/j.rbre.2016.09.007
279. Tchetina EV, Pivanova AN, Markova GA, Lukina GV, Aleksandrova EN, Aleksankin AP, et al. Rituximab Downregulates Gene Expression Associated With Cell Proliferation, Survival, and Proteolysis in the Peripheral Blood From Rheumatoid Arthritis Patients: A Link Between High Baseline Autophagy-Related ULK1 Expression and Improved Pain Control. Arthritis (2016) 2016:4963950. doi: 10.1155/2016/4963950
280. Ramwadhdoebe TH, van Baarsen LGM, Boumans MJH, Bruijnen STG, Safy M, Berger FH, et al. Effect of Rituximab Treatment on T and B Cell Subsets in Lymph Node Biopsies of Patients With Rheumatoid Arthritis. Rheumatol (Oxford England) (2019) 58(6):1075–85. doi: 10.1093/rheumatology/key428
281. Piantoni S, Scarsi M, Tincani A, Airo P. Circulating CD4+ T-Cell Number Decreases in Rheumatoid Patients With Clinical Response to Rituximab. Rheumatol Int (2015) 35(9):1571–3. doi: 10.1007/s00296-015-3295-0
282. Einarsson JT, Evert M, Geborek P, Saxne T, Lundgren M, Kapetanovic MC. Rituximab in Clinical Practice: Dosage, Drug Adherence, Ig Levels, Infections, and Drug Antibodies. Clin Rheumatol (2017) 36(12):2743–50. doi: 10.1007/s10067-017-3848-6
283. Bergantini L, d’Alessandro M, Cameli P, Vietri L, Vagaggini C, Perrone A, et al. Effects of Rituximab Therapy on B Cell Differentiation and Depletion. Clin Rheumatol (2020) 39(5):1415–21. doi: 10.1007/s10067-020-04996-7
284. Fabris M, Quartuccio L, Lombardi S, Benucci M, Manfredi M, Saracco M, et al. Study on the Possible Role of the -174G>C IL-6 Promoter Polymorphism in Predicting Response to Rituximab in Rheumatoid Arthritis. Reumatismo (2010) 62(4):253–8. doi: 10.4081/reumatismo.2010.253
285. Pollastro S, Klarenbeek PL, Doorenspleet ME, van Schaik BDC, Esveldt REE, Thurlings RM, et al. Non-Response to Rituximab Therapy in Rheumatoid Arthritis is Associated With Incomplete Disruption of the B Cell Receptor Repertoire. Ann Rheum Dis (2019) 78(10):1339–45. doi: 10.1136/annrheumdis-2018-214898
286. Jimenez Morales A, Maldonado-Montoro M, Martinez de la Plata JE, Perez Ramirez C, Daddaoua A, Alarcon Payer C, et al. FCGR2A/FCGR3A Gene Polymorphisms and Clinical Variables as Predictors of Response to Tocilizumab and Rituximab in Patients With Rheumatoid Arthritis. J Clin Pharmacol (2019) 59(4):517–31. doi: 10.1002/jcph.1341
287. Konthur Z, Wiemkes MM, Haeupl T, Burmester GR, Skriner K. FRI0201 Rituximab Treated Non Responder Rheumatoid Arthritis Patients Are Generating A New Autoantibody Repertoire. In: BMJ Publishing Group Ltd (2016) 75:503. doi: 10.1136/annrheumdis-2017-eular.6978
288. Ruyssen-Witrand A, Rouanet S, Combe B, Dougados M, Le Loët X, Sibilia J, et al. Fcγ Receptor Type IIIA Polymorphism Influences Treatment Outcomes in Patients With Rheumatoid Arthritis Treated With Rituximab. Ann Rheum Dis (2012) 71(6):875–7. doi: 10.1136/annrheumdis-2011-200337
289. Quartuccio L, Fabris M, Pontarini E, Salvin S, Zabotti A, Benucci M, et al. The 158vv Fcgamma Receptor 3A Genotype is Associated With Response to Rituximab in Rheumatoid Arthritis: Results of an Italian Multicentre Study. Ann Rheum Dis (2014) 73(4):716–21. doi: 10.1136/annrheumdis-2012-202435
290. Stradner MH, Dejaco C, Brickmann K, Graninger WB, Brezinschek HP. A Combination of Cellular Biomarkers Predicts Failure to Respond to Rituximab in Rheumatoid Arthritis: A 24-Week Observational Study. Arthritis Res Ther (2016) 18:190. doi: 10.1186/s13075-016-1091-1
291. Kastbom A, Cöster L, Arlestig L, Chatzidionysiou A, van Vollenhoven RF, Padyukov L, et al. Influence of FCGR3A Genotype on the Therapeutic Response to Rituximab in Rheumatoid Arthritis: An Observational Cohort Study. BMJ Open (2012) 2(5):e001524. doi: 10.1136/bmjopen-2012-001524
292. Ruyssen-Witrand A, Rouanet S, Combe B, Dougados M, Le Loet X, Sibilia J, et al. Association Between -871C>T Promoter Polymorphism in the B-Cell Activating Factor Gene and the Response to Rituximab in Rheumatoid Arthritis Patients. Rheumatol (Oxford) (2013) 52(4):636–41. doi: 10.1093/rheumatology/kes344
293. Das S, Vital EM, Horton S, Bryer D, El-Sherbiny Y, Rawstron AC, et al. Abatacept or Tocilizumab After Rituximab in Rheumatoid Arthritis? An Exploratory Study Suggests non-Response to Rituximab is Associated With Persistently High IL-6 and Better Clinical Response to IL-6 Blocking Therapy. Ann Rheum Dis (2014) 73(5):909–12. doi: 10.1136/annrheumdis-2013-204417
294. Juge PA, Gazal S, Constantin A, Mariette X, Combe B, Tebib J, et al. Variants of Genes Implicated in Type 1 Interferon Pathway and B-Cell Activation Modulate the EULAR Response to Rituximab at 24 Weeks in Rheumatoid Arthritis. RMD Open (2017) 3(2):e000448. doi: 10.1136/rmdopen-2017-000448
295. Daien CI, Fabre S, Rittore C, Soler S, Daien V, Tejedor G, et al. TGF Beta1 Polymorphisms are Candidate Predictors of the Clinical Response to Rituximab in Rheumatoid Arthritis. Joint Bone Spine (2012) 79(5):471–5. doi: 10.1016/j.jbspin.2011.10.007
296. Fabris M, Quartuccio L, Vital E, Pontarini E, Salvin S, Fabro C, et al. The TTTT B Lymphocyte Stimulator Promoter Haplotype is Associated With Good Response to Rituximab Therapy in Seropositive Rheumatoid Arthritis Resistant to Tumor Necrosis Factor Blockers. Arthritis Rheum (2013) 65(1):88–97. doi: 10.1002/art.37707
297. Md Yusof MY, Candelier JB, Dass S, Rawstron A, Vital EM, Emery P. SAT0175 Validation of B Cell Depletion as A Predictor of Clinical Response and Efficacy of Retreatment of Non-Response in Rheumatoid Arthritis. Ann Rheum Dis (2016) 75(Suppl 2):731–1. doi: 10.1136/annrheumdis-2016-eular.6122
298. Luxembourger C, Ruyssen-Witrand A, Ladhari C, Rittore C, Degboe Y, Maillefert JF, et al. A Single Nucleotide Polymorphism of IL6-Receptor is Associated With Response to Tocilizumab in Rheumatoid Arthritis Patients. Pharmacogenomics J (2019) 19(4):368–74. doi: 10.1038/s41397-019-0072-6
299. Enevold C, Baslund B, Linde L, Josephsen NL, Tarp U, Lindegaard H, et al. Interleukin-6-Receptor Polymorphisms Rs12083537, Rs2228145, and Rs4329505 as Predictors of Response to Tocilizumab in Rheumatoid Arthritis. Pharmacogenet Genomics (2014) 24(8):401–5. doi: 10.1097/fpc.0000000000000071
300. Maldonado-Montoro M, Canadas-Garre M, Gonzalez-Utrilla A, Plaza-Plaza JC, Calleja-Hernandez MY. Genetic and Clinical Biomarkers of Tocilizumab Response in Patients With Rheumatoid Arthritis. Pharmacol Res (2016) 111:264–71. doi: 10.1016/j.phrs.2016.06.016
301. De Groof A, Ducreux J, Humby F, Nzeusseu Toukap A, Badot V, Pitzalis C, et al. Higher Expression of TNFalpha-Induced Genes in the Synovium of Patients With Early Rheumatoid Arthritis Correlates With Disease Activity, and Predicts Absence of Response to First Line Therapy. Arthritis Res Ther (2016) 18:19. doi: 10.1186/s13075-016-0919-z
302. Pers YM, Fortunet C, Constant E, Lambert J, Godfrin-Valnet M, De Jong A, et al. Predictors of Response and Remission in a Large Cohort of Rheumatoid Arthritis Patients Treated With Tocilizumab in Clinical Practice. Rheumatol (Oxford) (2014) 53(1):76–84. doi: 10.1093/rheumatology/ket301
303. Nishina N, Kikuchi J, Hashizume M, Yoshimoto K, Kameda H, Takeuchi T. Baseline Levels of Soluble Interleukin-6 Receptor Predict Clinical Remission in Patients With Rheumatoid Arthritis Treated With Tocilizumab: Implications for Molecular Targeted Therapy. Ann Rheum Dis (2014) 73(5):945–7. doi: 10.1136/annrheumdis-2013-204137
304. Sanayama Y, Ikeda K, Saito Y, Kagami S, Yamagata M, Furuta S, et al. Prediction of Therapeutic Responses to Tocilizumab in Patients With Rheumatoid Arthritis: Biomarkers Identified by Analysis of Gene Expression in Peripheral Blood Mononuclear Cells Using Genome-Wide DNA Microarray. Arthritis Rheumatol (2014) 66(6):1421–31. doi: 10.1002/art.38400
305. Okano T, Inui K, Tada M, Sugioka Y, Mamoto K, Wakitani S, et al. Levels of Interleukin-1 Beta can Predict Response to Tocilizumab Therapy in Rheumatoid Arthritis: The PETITE (Predictors of Effectiveness of Tocilizumab Therapy) Study. Rheumatol Int (2016) 36(3):349–57. doi: 10.1007/s00296-015-3379-x
306. Hirata S, Marotta A, Gui Y, Hanami K, Tanaka Y. Serum 14-3-3η Level is Associated With Severity and Clinical Outcomes of Rheumatoid Arthritis, and its Pretreatment Level is Predictive of DAS28 Remission With Tocilizumab. Arthritis Res Ther (2015) 17(1):280. doi: 10.1186/s13075-015-0799-7
307. Mesko B, Poliska S, Szamosi S, Szekanecz Z, Podani J, Varadi C, et al. Peripheral Blood Gene Expression and IgG Glycosylation Profiles as Markers of Tocilizumab Treatment in Rheumatoid Arthritis. J Rheumatol (2012) 39(5):916–28. doi: 10.3899/jrheum.110961
308. Uno K, Yoshizaki K, Iwahashi M, Yamana J, Yamana S, Tanigawa M, et al. Pretreatment Prediction of Individual Rheumatoid Arthritis Patients’ Response to Anti-Cytokine Therapy Using Serum Cytokine/Chemokine/Soluble Receptor Biomarkers. PloS One (2015) 10(7):e0132055. doi: 10.1371/journal.pone.0132055
309. Lee SJ, Park W, Park SH, Shim SC, Baek HJ, Yoo DH, et al. Low Baseline Interleukin-17A Levels are Associated With Better Treatment Response at 12 Weeks to Tocilizumab Therapy in Rheumatoid Arthritis Patients. J Immunol Res (2015) 2015:487230. doi: 10.1155/2015/487230
310. Daïen CI, Gailhac S, Audo R, Mura T, Hahne M, Combe B, et al. High Levels of Natural Killer Cells Are Associated With Response to Tocilizumab in Patients With Severe Rheumatoid Arthritis. Rheumatol (Oxford) (2015) 54(4):601–8. doi: 10.1093/rheumatology/keu363
311. Genovese MC, Fleischmann R, Kivitz A, Lee E-B, van Hoogstraten H, Kimura T, et al. Efficacy and Safety of Sarilumab in Combination With csDMARDs or as Monotherapy in Subpopulations of Patients With Moderately to Severely Active Rheumatoid Arthritis in Three Phase III Randomized, Controlled Studies. Arthritis Res Ther (2020) 22(1):139. doi: 10.1186/s13075-020-02194-z
312. Emery P, van Hoogstraten H, Mangan EK, van Adelsberg J, Fan C, Kivitz A, et al. SAT0178 Clinical Responses of Sarilumab Based on Rheumatoid Factor and Anti–Cyclic Citrullinated Peptide Antibody Status in Patients With Rheumatoid Arthritis. Ann Rheum Dis (2016) 75(Suppl 2):732–2. doi: 10.1136/annrheumdis-2016-eular.4415
313. Boyapati A, Msihid J, Schwartzman S, Choy E, Burmester G, Lam G, et al. 069 High Baseline Serum IL-6 Identifies a Subgroup of Rheumatoid Arthritis Patients With Rapid Joint Damage and Clinical Progression and Predicts Increased Sarilumab Treatment Response. Rheumatology (2018) 58(2019):L08. doi: 10.1093/rheumatology/kez106.068
314. Gabay C, Msihid J, Zilberstein M, Paccard C, Lin Y, Graham NMH, et al. Identification of Sarilumab Pharmacodynamic and Predictive Markers in Patients With Inadequate Response to TNF Inhibition: A Biomarker Substudy of the Phase 3 TARGET Study. RMD Open (2018) 4(1):e000607. doi: 10.1136/rmdopen-2017-000607
315. Ebina K, Hirano T, Maeda Y, Yamamoto W, Hashimoto M, Murata K, et al. Drug Retention of Secondary Biologics or JAK Inhibitors After Tocilizumab or Abatacept Failure as First Biologics in Patients With Rheumatoid Arthritis -the ANSWER Cohort Study. Clin Rheumatol (2020) 39(9):2563–72. doi: 10.1007/s10067-020-05015-5
316. Suzuki M, Takahashi N, Kida D, Hirano Y, Kato T, Yabe Y, et al. Clinical Effectiveness and Safety of Additional Administration of Tacrolimus in Rheumatoid Arthritis Patients With an Inadequate Response to Abatacept: A Retrospective Cohort Study. Int J Rheum Dis (2019) 22(12):2199–205. doi: 10.1111/1756-185X.13731
317. Silverman GJ, Carson DA. Roles of B Cells in Rheumatoid Arthritis. Arthritis Res Ther (2003) 5(4):S1. doi: 10.1186/ar1010
318. Boumans MJH, Tak PP. Rituximab Treatment in Rheumatoid Arthritis: How Does it Work? Arthritis Res Ther (2009) 11(6):134. doi: 10.1186/ar2852
319. Schioppo T, Ingegnoli F. Current Perspective on Rituximab in Rheumatic Diseases. Drug Des Devel Ther (2017) 11:2891–904. doi: 10.2147/dddt.S139248
320. Mian A, Ibrahim F, Scott DL. A Systematic Review of Guidelines for Managing Rheumatoid Arthritis. BMC Rheumatol (2019) 3(1):42. doi: 10.1186/s41927-019-0090-7
321. BMJ Group. Rituximab and ▼Abatacept for Rheumatoid Arthritis. Drug Ther Bull (2008) 46(8):57–61. doi: 10.1136/dtb.2008.07.0017
322. Das G, Damotte V, Gelfand JM, Bevan C, Cree BAC, Do L, et al. Rituximab Before and During Pregnancy: A Systematic Review, and a Case Series in MS and NMOSD. Neurology(R) Neuroimmunol Neuroinflamm (2018) 5(3):e453–3. doi: 10.1212/NXI.0000000000000453
323. Cohen SB, Emery P, Greenwald MW, Dougados M, Furie RA, Genovese MC, et al. Rituximab for Rheumatoid Arthritis Refractory to Anti–Tumor Necrosis Factor Therapy: Results of a Multicenter, Randomized, Double-Blind, Placebo-Controlled, Phase III Trial Evaluating Primary Efficacy and Safety at Twenty-Four Weeks. Arthritis Rheumatism (2006) 54(9):2793–806. doi: 10.1002/art.22025
324. Kim DD, Song W-C. Membrane Complement Regulatory Proteins. Clin Immunol (2006) 118(2):127–36. doi: 10.1016/j.clim.2005.10.014
325. Beyer I, Cao H, Persson J, Wang H, Liu Y, Yumul R, et al. Transient Removal of CD46 is Safe and Increases B-Cell Depletion by Rituximab in CD46 Transgenic Mice and Macaques. Mol Ther (2013) 21(2):291–9. doi: 10.1038/mt.2012.212
326. Wang H, Liu Y, Li Z, Hemminki A, Lieber A. 657. A CD46-Targeting Recombinant Protein That Increases the Therapeutic Efficacy of Monoclonal Antibodies for Cancer. Mol Ther (2010) 18:S256. doi: 10.1016/S1525-0016(16)38098-4
327. El-Azkalany G, Gheita P. A. B and R. B: Clinical Significance of Serum Interleukin-6 and �174 G/C Promoter Polymorphism in Rheumatoid Arthritis Patients. Egypt Rheumatol (2014) 35(2013):107–13. doi: 10.1016/j.ejr.2012.11.002
328. Walker UA, Jaeger VK, Chatzidionysiou K, Hetland ML, Hauge EM, Pavelka K, et al. Rituximab Done: What’s Next in Rheumatoid Arthritis? A European Observational Longitudinal Study Assessing the Effectiveness of Biologics After Rituximab Treatment in Rheumatoid Arthritis. Rheumatol (Oxford) (2016) 55(2):230–6. doi: 10.1093/rheumatology/kev297
329. Heinrich PC, Behrmann I, Müller-Newen G, Schaper F, Graeve L. Interleukin-6-Type Cytokine Signalling Through the Gp130/Jak/STAT Pathway. Biochem J (1998) 334(Pt 2)(Pt 2):297–314. doi: 10.1042/bj3340297
330. Choy EH, De Benedetti F, Takeuchi T, Hashizume M, John MR, Kishimoto T. Translating IL-6 Biology Into Effective Treatments. Nat Rev Rheumatol (2020) 16(6):335–45. doi: 10.1038/s41584-020-0419-z
331. Dayer JM, Choy E. Therapeutic Targets in Rheumatoid Arthritis: The Interleukin-6 Receptor. Rheumatol (Oxford) (2010) 49(1):15–24. doi: 10.1093/rheumatology/kep329
332. Rose-John S, Winthrop K, Calabrese L. The Role of IL-6 in Host Defence Against Infections: Immunobiology and Clinical Implications. Nat Rev Rheumatol (2017) 13(7):399–409. doi: 10.1038/nrrheum.2017.83
333. Cortellini G, Mascella F, Simoncelli M, Lippolis D, Focherini MC, Cortellini F, et al. Effective Desensitization to Tocilizumab in Delayed Hypersensitivity Reaction. Pharmacology (2018) 102(1–2):114–6. doi: 10.1159/000490419
334. Ogata A, Hirano T, Hishitani Y, Tanaka T. Safety and Efficacy of Tocilizumab for the Treatment of Rheumatoid Arthritis. Clin Med Insights Arthritis Musculoskeletal Disord (2012) 5:27–42. doi: 10.4137/CMAMD.S7371
335. Kremer JM, Blanco R, Brzosko M, Burgos-Vargas R, Halland AM, Vernon E, et al. Tocilizumab Inhibits Structural Joint Damage in Rheumatoid Arthritis Patients With Inadequate Responses to Methotrexate: Results From the Double-Blind Treatment Phase of a Randomized Placebo-Controlled Trial of Tocilizumab Safety and Prevention of Structural Joint Damage at One Year. Arthritis Rheum (2011) 63(3):609–21. doi: 10.1002/art.30158
336. Emery P, Keystone E, Tony HP, Cantagrel A, van Vollenhoven R, Sanchez A, et al. IL-6 Receptor Inhibition With Tocilizumab Improves Treatment Outcomes in Patients With Rheumatoid Arthritis Refractory to Anti-Tumour Necrosis Factor Biologicals: Results From a 24-Week Multicentre Randomised Placebo-Controlled Trial. Ann Rheum Dis (2008) 67(11):1516–23. doi: 10.1136/ard.2008.092932
337. Burmester GR, Choy E, Kivitz A, Ogata A, Bao M, Nomura A, et al. Low Immunogenicity of Tocilizumab in Patients With Rheumatoid Arthritis. Ann Rheum Dis (2017) 76(6):1078–85. doi: 10.1136/annrheumdis-2016-210297
338. Wang J, Platt A, Upmanyu R, Germer S, Lei G, Rabe C, et al. IL-6 Pathway-Driven Investigation of Response to IL-6 Receptor Inhibition in Rheumatoid Arthritis. BMJ Open (2013) 3(8):e003199. doi: 10.1136/bmjopen-2013-003199
339. Wang J, Bansal A, Martin M, Germer S, Benayed R, Essioux L, et al. Genome-Wide Association Analysis Implicates the Involvement of Eight Loci With Response to Tocilizumab for the Treatment of Rheumatoid Arthritis. Pharmacogenomics J (2012) 13:235–41. doi: 10.1038/tpj.2012.8
340. Díaz-Villamarín X, Dávila-Fajardo C, González-Medina M, Blánquez-Martínez D, Moreno-Raya P, Cabeza-Barrera J. CP-086 CD69 a<G (RS11052877) Genetic Polymorphism on the Response to Tocilizumab in Rheumatoid Arthritis Patients. Eur J Hosp Pharm (2017) 24(Suppl 1):A38–8. doi: 10.1136/ejhpharm-2017-000640.85
341. Burmester GR, Feist E, Kellner H, Braun J, Iking-Konert C, Rubbert-Roth A. Effectiveness and Safety of the Interleukin 6-Receptor Antagonist Tocilizumab After 4 and 24 Weeks in Patients With Active Rheumatoid Arthritis: The First Phase IIIb Real-Life Study (TAMARA). Ann Rheum Dis (2011) 70(5):755–9. doi: 10.1136/ard.2010.139725
342. Jones G, Ding C. Tocilizumab: A Review of its Safety and Efficacy in Rheumatoid Arthritis. Clin Med Insights Arthritis Musculoskelet Disord (2010) 3:81–9. doi: 10.4137/cmamd.S4864
343. Narváez J, Magallares B, Díaz Torné C, Hernández MV, Reina D, Corominas H, et al. Predictive Factors for Induction of Remission in Patients With Active Rheumatoid Arthritis Treated With Tocilizumab in Clinical Practice. Semin Arthritis Rheum (2016) 45(4):386–90. doi: 10.1016/j.semarthrit.2015.07.001
344. Dorner T, Schulze-Koops H, Burmester GR, Iking-Konert C, Schmalzing M, Engel A, et al. Early and Late Responses in Patients With Rheumatoid Arthritis Who Were Conventional Synthetic Disease-Modifying Anti-Rheumatic Drug Inadequate Responders and Were Treated With Tocilizumab or Switched to Rituximab: An Open-Label Phase 3 Trial (MIRAI). Clin Exp Rheumatol (2019) 37(6):937–45.
345. Akiyama M, Kaneko Y, Kondo H, Takeuchi T. Comparison of the Clinical Effectiveness of Tumour Necrosis Factor Inhibitors and Abatacept After Insufficient Response to Tocilizumab in Patients With Rheumatoid Arthritis. Clin Rheumatol (2016) 35(11):2829–34. doi: 10.1007/s10067-016-3227-8
346. Lamb YN, Deeks ED. Sarilumab: A Review in Moderate to Severe Rheumatoid Arthritis. Drugs (2018) 78(9):929–40. doi: 10.1007/s40265-018-0929-z
347. Rafique A, Martin J, Blome M, Huang T, Ouyang A, Papadopoulos N. Evaluation of the Binding Kinetics and Functional Bioassay Activity of Sarilumab and Tocilizumab to the Human IL-6 Receptor (IL-6r) α. Ann Rheum Dis (2014) 72:A797–7. doi: 10.1136/annrheumdis-2013-eular.2360
348. Wang L-H, Xue Y, Liu X, Luo F, Kelly L, Huang T, et al. FRI0020 Preclinical Development of Sarilumab, the First Fully Human Monoclonal Antibody (MAB) Against IL-6r Alpha: Utilization and Value of Double Humanized Animal Model. Ann Rheum Dis (2013) 72(Suppl 3):A375–5. doi: 10.1136/annrheumdis-2013-eular.1148
349. Huizinga TW, Fleischmann RM, Jasson M, Radin AR, van Adelsberg J, Fiore S, et al. Sarilumab, a Fully Human Monoclonal Antibody Against IL-6rα in Patients With Rheumatoid Arthritis and an Inadequate Response to Methotrexate: Efficacy and Safety Results From the Randomised SARIL-RA-MOBILITY Part A Trial. Ann Rheum Dis (2014) 73(9):1626–34. doi: 10.1136/annrheumdis-2013-204405
350. Boyapati A, Msihid J, Fiore S, van Adelsberg J, Graham NM, Hamilton JD. Sarilumab Plus Methotrexate Suppresses Circulating Biomarkers of Bone Resorption and Synovial Damage in Patients With Rheumatoid Arthritis and Inadequate Response to Methotrexate: A Biomarker Study of MOBILITY. Arthritis Res Ther (2016) 18(1):225. doi: 10.1186/s13075-016-1132-9
351. Genovese MC, Fleischmann R, Kivitz AJ, Rell-Bakalarska M, Martincova R, Fiore S, et al. Sarilumab Plus Methotrexate in Patients With Active Rheumatoid Arthritis and Inadequate Response to Methotrexate: Results of a Phase III Study. Arthritis Rheumatol (2015) 67(6):1424–37. doi: 10.1002/art.39093
352. Fleischmann R, van Adelsberg J, Lin Y, Castelar-Pinheiro GD, Brzezicki J, Hrycaj P, et al. Sarilumab and Nonbiologic Disease-Modifying Antirheumatic Drugs in Patients With Active Rheumatoid Arthritis and Inadequate Response or Intolerance to Tumor Necrosis Factor Inhibitors. Arthritis Rheumatol (2017) 69(2):277–90. doi: 10.1002/art.39944
353. Burmester GR, Lin Y, Patel R, van Adelsberg J, Mangan EK, Graham NM, et al. Efficacy and Safety of Sarilumab Monotherapy Versus Adalimumab Monotherapy for the Treatment of Patients With Active Rheumatoid Arthritis (MONARCH): A Randomised, Double-Blind, Parallel-Group Phase III Trial. Ann Rheum Dis (2017) 76(5):840–7. doi: 10.1136/annrheumdis-2016-210310
354. Ebina K, Hashimoto M, Yamamoto W, Hirano T, Hara R, Katayama M, et al. Drug Tolerability and Reasons for Discontinuation of Seven Biologics in 4466 Treatment Courses of Rheumatoid Arthritis—the ANSWER Cohort Study. Arthritis Res Ther (2019) 21(1):91. doi: 10.1186/s13075-019-1880-4
355. Schwartz DM, Kanno Y, Villarino A, Ward M, Gadina M, O’Shea JJ. JAK Inhibition as a Therapeutic Strategy for Immune and Inflammatory Diseases. Nat Rev Drug Discov (2017) 17(1):78. doi: 10.1038/nrd.2017.267
356. Rosengren S, Corr M, Firestein GS, Boyle DL. The JAK Inhibitor CP-690,550 (Tofacitinib) Inhibits TNF-Induced Chemokine Expression in Fibroblast-Like Synoviocytes: Autocrine Role of Type I Interferon. Ann Rheum Dis (2012) 71(3):440–7. doi: 10.1136/ard.2011.150284
357. Kotyla PJ. Are Janus Kinase Inhibitors Superior Over Classic Biologic Agents in RA Patients? BioMed Res Int (2018) 2018:7492904–7492904. doi: 10.1155/2018/7492904
358. Ghoreschi K, Jesson MI, Li X, Lee JL, Ghosh S, Alsup JW, et al. Modulation of Innate and Adaptive Immune Responses by Tofacitinib (CP-690,550). J Immunol (2011) 186(7):4234–43. doi: 10.4049/jimmunol.1003668
359. Dhillon S. Tofacitinib: A Review in Rheumatoid Arthritis. Drugs (2017) 77(18):1987–2001. doi: 10.1007/s40265-017-0835-9
360. Meyer DM, Jesson MI, Li X, Elrick MM, Funckes-Shippy CL, Warner JD, et al. Anti-Inflammatory Activity and Neutrophil Reductions Mediated by the JAK1/JAK3 Inhibitor, CP-690,550, in Rat Adjuvant-Induced Arthritis. J Inflamm (Lond) (2010) 7:41. doi: 10.1186/1476-9255-7-41
361. Boyle DL, Soma K, Hodge J, Kavanaugh A, Mandel D, Mease P, et al. The JAK Inhibitor Tofacitinib Suppresses Synovial JAK1-STAT Signalling in Rheumatoid Arthritis. Ann Rheum Dis (2015) 74(6):1311–6. doi: 10.1136/annrheumdis-2014-206028
362. Razmjou AA, Brook J, Elashoff D, Kaeley G, Choi S, Kermani T, et al. Ultrasound and Multi-Biomarker Disease Activity Score for Assessing and Predicting Clinical Response to Tofacitinib Treatment in Patients With Rheumatoid Arthritis. BMC Rheumatol (2020) 4:55. doi: 10.1186/s41927-020-00153-4
363. Fernández-Ruiz JC, Ramos-Remus C, Sánchez-Corona J, Castillo-Ortiz JD, Castañeda-Sánchez JJ, Bastian Y, et al. Analysis of miRNA Expression in Patients With Rheumatoid Arthritis During Remission and Relapse After a 5-Year Trial of Tofacitinib Treatment. Int Immunopharmacol (2018) 63:35–42. doi: 10.1016/j.intimp.2018.07.028
364. Li Y, Yuan L, Yang J, Lei Y, Zhang H, Xia L, et al. Changes in Serum Cytokines May Predict Therapeutic Efficacy of Tofacitinib in Rheumatoid Arthritis. Mediators Inflamm (2019) 2019:5617431. doi: 10.1155/2019/5617431
365. Hamar A, Szekanecz Z, Pusztai A, Czókolyová M, Végh E, Pethő Z, et al. Effects of One-Year Tofacitinib Therapy on Bone Metabolism in Rheumatoid Arthritis. Osteoporosis Int (2021) 32(8):1621–9. doi: 10.1007/s00198-021-05871-0
366. López-Romero P, Martinez-Gamboa L, Bang H, de la Torre I, Holzkämper T, Feist E. Assessment of the Association of Baseline Anti-CarbV and Anti-MCV Antibodies With Response to Treatment and Radiographic Progression in an RA Population Treated With Either Methotrexate or Baricitinib: Post-Hoc Analyses From RA-BEGIN. Arthritis Res Ther (2020) 22(1):193. doi: 10.1186/s13075-020-02284-y
367. Takahashi N, Asai S, Kobayakawa T, Kaneko A, Watanabe T, Kato T, et al. Predictors for Clinical Effectiveness of Baricitinib in Rheumatoid Arthritis Patients in Routine Clinical Practice: Data From a Japanese Multicenter Registry. Sci Rep (2020) 10(1):21907. doi: 10.1038/s41598-020-78925-8
368. Sornasse T, Song IH, Radstake T, Mcinnes I. FRI0026 Proteomics Analysis Comparing the Mode OF Action of Upadacitinib and Adalimumab Head to Head in RA Identifies Novel, Discrete Early Immune Pathway Modulation in the Select-Compare Phase 3 Study. Ann Rheum Dis (2020) 79(Suppl 1):585–6. doi: 10.1136/annrheumdis-2020-eular.1908
369. Shi JG, Chen X, Lee F, Emm T, Scherle PA, Lo Y, et al. The Pharmacokinetics, Pharmacodynamics, and Safety of Baricitinib, an Oral JAK 1/2 Inhibitor, in Healthy Volunteers. J Clin Pharmacol (2014) 54(12):1354–61. doi: 10.1002/jcph.354
370. Two New Drugs for Rheumatoid Arthritis. Drug Ther Bull (2017) 55(9):102–5. doi: 10.1136/dtb.2017.8.0526
371. Fridman JS, Scherle PA, Collins R, Burn TC, Li Y, Li J, et al. Selective Inhibition of JAK1 and JAK2 is Efficacious in Rodent Models of Arthritis: Preclinical Characterization of INCB028050. J Immunol (2010) 184(9):5298–307. doi: 10.4049/jimmunol.0902819
372. Emery P, Durez P, Hueber AJ, de la Torre I, Larsson E, Holzkämper T, et al. Baricitinib Inhibits Structural Joint Damage Progression in Patients With Rheumatoid Arthritis-a Comprehensive Review. Arthritis Res Ther (2021) 23(1):3–3. doi: 10.1186/s13075-020-02379-6
373. Agency EM. Olumiant (Baricitinib) Film-Coated Tablets: EU Summary of Product Characteristics. In: European Medicines Agency. Amsterdam, The Netherlands: European Medicines Agency (2018).
374. Tanaka Y, McInnes IB, Taylor PC, Byers NL, Chen L, de Bono S, et al. Characterization and Changes of Lymphocyte Subsets in Baricitinib-Treated Patients With Rheumatoid Arthritis: An Integrated Analysis. Arthritis Rheumatol (2018) 70(12):1923–32. doi: 10.1002/art.40680
375. Genovese MC, Kremer JM, Kartman CE, Schlichting DE, Xie L, Carmack T, et al. Response to Baricitinib Based on Prior Biologic Use in Patients With Refractory Rheumatoid Arthritis. Rheumatol (Oxford) (2018) 57(5):900–8. doi: 10.1093/rheumatology/kex489
376. Emery P, Durez P, Hueber A, Torre I, Larsson E, Holzkaemper T, et al. Baricitinib Inhibits Structural Joint Damage Progression in Patients With Rheumatoid Arthritis – a Comprehensive Review. Arthritis Res Ther (2021) 23(1):3. doi: 10.1186/s13075-020-02379-6
377. Fleischmann R, Schiff M, van der Heijde D, Ramos-Remus C, Spindler A, Stanislav M, et al. Baricitinib, Methotrexate, or Combination in Patients With Rheumatoid Arthritis and No or Limited Prior Disease-Modifying Antirheumatic Drug Treatment. Arthritis Rheumatol (2017) 69(3):506–17. doi: 10.1002/art.39953
378. Taylor PC, Keystone EC, van der Heijde D, Weinblatt ME, Del Carmen Morales L, Reyes Gonzaga J, et al. Baricitinib Versus Placebo or Adalimumab in Rheumatoid Arthritis. N Engl J Med (2017) 376(7):652–62. doi: 10.1056/NEJMoa1608345
379. Kremer JM, Schiff M, Muram D, Zhong J, Alam J, Genovese MC. Response to Baricitinib Therapy in Patients With Rheumatoid Arthritis With Inadequate Response to csDMARDs as a Function of Baseline Characteristics. RMD Open (2018) 4(1):e000581. doi: 10.1136/rmdopen-2017-000581
380. Al-Salama ZT, Scott LJ. Baricitinib: A Review in Rheumatoid Arthritis. Drugs (2018) 78(7):761–72. doi: 10.1007/s40265-018-0908-4
381. Mohamed MF, Klunder B, Othman AA. Clinical Pharmacokinetics of Upadacitinib: Review of Data Relevant to the Rheumatoid Arthritis Indication. Clin Pharmacokinet (2020) 59(5):531–44. doi: 10.1007/s40262-019-00855-0
382. Biggioggero M, Becciolini A, Crotti C, Agape E, Favalli EG. Upadacitinib and Filgotinib: The Role of JAK1 Selective Inhibition in the Treatment of Rheumatoid Arthritis. Drugs Context (2019) 8:212595. doi: 10.7573/dic.212595
383. Parmentier JM, Voss J, Graff C, Schwartz A, Argiriadi M, Friedman M, et al. In Vitro and In Vivo Characterization of the JAK1 Selectivity of Upadacitinib (ABT-494). BMC Rheumatol (2018) 2(1):23. doi: 10.1186/s41927-018-0031-x
384. Conaghan PG, Mysler E, Tanaka Y, Da Silva-Tillmann B, Shaw T, Liu J, et al. Upadacitinib in Rheumatoid Arthritis: A Benefit–Risk Assessment Across a Phase III Program. Drug Saf (2021) 44(5):515–30. doi: 10.1007/s40264-020-01036-w
385. Kremer JM, Emery P, Camp HS, Friedman A, Wang L, Othman AA, et al. A Phase IIb Study of ABT-494, a Selective JAK-1 Inhibitor, in Patients With Rheumatoid Arthritis and an Inadequate Response to Anti–Tumor Necrosis Factor Therapy. Arthritis Rheumatol (2016) 68(12):2867–77. doi: 10.1002/art.39801
386. Genovese MC, Smolen JS, Weinblatt ME, Burmester GR, Meerwein S, Camp HS, et al. Efficacy and Safety of ABT-494, a Selective JAK-1 Inhibitor, in a Phase IIb Study in Patients With Rheumatoid Arthritis and an Inadequate Response to Methotrexate. Arthritis Rheumatol (2016) 68(12):2857–66. doi: 10.1002/art.39808
387. Strand V, Pope J, Tundia N, Friedman A, Camp HS, Pangan A, et al. Upadacitinib Improves Patient-Reported Outcomes in Patients With Rheumatoid Arthritis and Inadequate Response to Conventional Synthetic Disease-Modifying Antirheumatic Drugs: Results From SELECT-NEXT. Arthritis Res Ther (2019) 21(1):272. doi: 10.1186/s13075-019-2037-1
388. Burmester GR, Kremer JM, Van den Bosch F, Kivitz A, Bessette L, Li Y, et al. Safety and Efficacy of Upadacitinib in Patients With Rheumatoid Arthritis and Inadequate Response to Conventional Synthetic Disease-Modifying Anti-Rheumatic Drugs (SELECT-NEXT): A Randomised, Double-Blind, Placebo-Controlled Phase 3 Trial. Lancet (2018) 391(10139):2503–12. doi: 10.1016/s0140-6736(18)31115-2
389. Genovese MC, Fleischmann R, Combe B, Hall S, Rubbert-Roth A, Zhang Y, et al. Safety and Efficacy of Upadacitinib in Patients With Active Rheumatoid Arthritis Refractory to Biologic Disease-Modifying Anti-Rheumatic Drugs (SELECT-BEYOND): A Double-Blind, Randomised Controlled Phase 3 Trial. Lancet (2018) 391(10139):2513–24. doi: 10.1016/s0140-6736(18)31116-4
390. Smolen JS, Pangan AL, Emery P, Rigby W, Tanaka Y, Vargas JI, et al. Upadacitinib as Monotherapy in Patients With Active Rheumatoid Arthritis and Inadequate Response to Methotrexate (SELECT-MONOTHERAPY): A Randomised, Placebo-Controlled, Double-Blind Phase 3 Study. Lancet (2019) 393(10188):2303–11. doi: 10.1016/S0140-6736(19)30419-2
391. Sornasse T, Sokolove J, McInnes I. THU0181 Treatment With Upadacitinib Results in the Normalization of Key Pathobiologic Pathways in Patients With Rheumatoid Arthritis: Biomarker Results From the Phase 3 Select-Next and Select-Beyond Studies. Ann Rheum Dis (2019) 78(Suppl 2):365–6. doi: 10.1136/annrheumdis-2019-eular.2994
392. Fleischmann RM, Blanco R, Hall S, Thomson GTD, Van den Bosch FE, Zerbini C, et al. Switching Between Janus Kinase Inhibitor Upadacitinib and Adalimumab Following Insufficient Response: Efficacy and Safety in Patients With Rheumatoid Arthritis. Ann Rheum Dis (2021) 80(4):432–9. doi: 10.1136/annrheumdis-2020-218412
393. Bluett J, Barton A. Precision Medicine in Rheumatoid Arthritis. Rheum Dis Clin North Am (2017) 43(3):377–87. doi: 10.1016/j.rdc.2017.04.008
394. Yau TO. Precision Treatment in Colorectal Cancer: Now and the Future. JGH Open (2019) 3(5):361–9. doi: 10.1002/jgh3.12153
395. Olivier M, Asmis R, Hawkins GA, Howard TD, Cox LA. The Need for Multi-Omics Biomarker Signatures in Precision Medicine. Int J Mol Sci (2019) 20(19):4781. doi: 10.3390/ijms20194781
396. Subramanian I, Verma S, Kumar S, Jere A, Anamika K. Multi-Omics Data Integration, Interpretation, and Its Application. Bioinf Biol Insights (2020) 14:1177932219899051–1177932219899051. doi: 10.1177/1177932219899051
397. Putignani L, Gasbarrini A, Dallapiccola B. Potential of Multiomics Technology in Precision Medicine. Curr Opin Gastroenterol (2019) 35(6):491–8. doi: 10.1097/mog.0000000000000589
398. Oswald M, Curran ME, Lamberth SL, Townsend RM, Hamilton JD, Chernoff DN, et al. Modular Analysis of Peripheral Blood Gene Expression in Rheumatoid Arthritis Captures Reproducible Gene Expression Changes in Tumor Necrosis Factor Responders. Arthritis Rheumatol (2015) 67(2):344–51. doi: 10.1002/art.38947
399. Ducreux J, Durez P, Galant C, Nzeusseu Toukap A, Van den Eynde B, Houssiau FA, et al. Global Molecular Effects of Tocilizumab Therapy in Rheumatoid Arthritis Synovium. Arthritis Rheumatol (2014) 66(1):15–23. doi: 10.1002/art.38202
400. Cuppen BVJ, Rossato M, Fritsch-Stork RDE, Concepcion AN, Linn-Rasker SP, Bijlsma JWJ, et al. RNA Sequencing to Predict Response to TNF-α Inhibitors Reveals Possible Mechanism for Nonresponse in Smokers. Expert Rev Clin Immunol (2018) 14(7):623–33. doi: 10.1080/1744666x.2018.1480937
401. Tasaki S, Suzuki K, Kassai Y, Takeshita M, Murota A, Kondo Y, et al. Multi-Omics Monitoring of Drug Response in Rheumatoid Arthritis in Pursuit of Molecular Remission. Nat Commun (2018) 9(1):2755. doi: 10.1038/s41467-018-05044-4
402. Aterido A, Cañete JD, Tornero J, Blanco F, Fernández-Gutierrez B, Pérez C, et al. A Combined Transcriptomic and Genomic Analysis Identifies a Gene Signature Associated With the Response to Anti-TNF Therapy in Rheumatoid Arthritis. Front Immunol (2019) 10:1459(1459). doi: 10.3389/fimmu.2019.01459
403. Tao W, Concepcion AN, Vianen M, Marijnissen ACA, Lafeber FPGJ, Radstake TRDJ, et al. Multiomics and Machine Learning Accurately Predict Clinical Response to Adalimumab and Etanercept Therapy in Patients With Rheumatoid Arthritis. Arthritis Rheumatol (Hoboken NJ) (2021) 73(2):212–22. doi: 10.1002/art.41516
404. Cheung P, Khatri P, Utz PJ, Kuo AJ. Single-Cell Technologies — Studying Rheumatic Diseases One Cell at a Time. Nat Rev Rheumatol (2019) 15(6):340–54. doi: 10.1038/s41584-019-0220-z
405. Reyfman PA, Walter JM, Joshi N, Anekalla KR, McQuattie-Pimentel AC, Chiu S, et al. Single-Cell Transcriptomic Analysis of Human Lung Provides Insights Into the Pathobiology of Pulmonary Fibrosis. Am J Respir Crit Care Med (2019) 199(12):1517–36. doi: 10.1164/rccm.201712-2410OC
406. Hwang B, Lee JH, Bang D. Single-Cell RNA Sequencing Technologies and Bioinformaticspipelines. Exp Mol Med (2018) 50(8):1–14. doi: 10.1038/s12276-018-0071-8
407. Luecken MD, Theis FJ. Current Best Practices in Single-Cell RNA-Seq Analysis: A Tutorial. Mol Syst Biol (2019) 15(6):e8746. doi: 10.15252/msb.20188746
408. Lee J, Hyeon DY, Hwang D. Single-Cell Multiomics: Technologies and Data Analysis Methods. Exp Mol Med (2020) 52(9):1428–42. doi: 10.1038/s12276-020-0420-2
409. Fonseka CY, Rao DA, Teslovich NC, Korsunsky I, Hannes SK, Slowikowski K, et al. Mixed-Effects Association of Single Cells Identifies an Expanded Effector CD4(+) T Cell Subset in Rheumatoid Arthritis. Sci Transl Med (2018) 10(463):eaaq0305. doi: 10.1126/scitranslmed.aaq0305
410. Xu ZC, Li SL, Rozewicki J, Yamashita K, Teraguchi S, Inoue T, et al. Functional Clustering of B Cell Receptors Using Sequence and Structural Features. Mol Syst Design Eng (2019) 4(4):769–78. doi: 10.1039/c9me00021f
411. Tan YC, Kongpachith S, Blum LK, Ju CH, Lahey LJ, Lu DR, et al. Barcode-Enabled Sequencing of Plasmablast Antibody Repertoires in Rheumatoid Arthritis. Arthritis Rheumatol (2014) 66(10):2706–15. doi: 10.1002/art.38754
412. Kinslow JD, Blum LK, Deane KD, Demoruelle MK, Okamoto Y, Parish MC, et al. Elevated IgA Plasmablast Levels in Subjects at Risk of Developing Rheumatoid Arthritis. Arthritis Rheumatol (2016) 68(10):2372–83. doi: 10.1002/art.39771
413. Elliott SE, Kongpachith S, Lingampalli N, Adamska JZ, Cannon BJ, Mao R, et al. Affinity Maturation Drives Epitope Spreading and Generation of Proinflammatory Anti-Citrullinated Protein Antibodies in Rheumatoid Arthritis. Arthritis Rheumatol (2018) 70(12):1946–58. doi: 10.1002/art.40587
414. Chen R, Mias GI, Li-Pook-Than J, Jiang L, Lam HY, Chen R, et al. Personal Omics Profiling Reveals Dynamic Molecular and Medical Phenotypes. Cell (2012) 148(6):1293–307. doi: 10.1016/j.cell.2012.02.009
415. Gligorijević V, Malod-Dognin N, Pržulj N. Integrative Methods for Analyzing Big Data in Precision Medicine. Proteomics (2016) 16(5):741–58. doi: 10.1002/pmic.201500396
416. Hobbs BP, Berry DA, Coombes KR. 17 - Biostatistics and Bioinformatics in Clinical Trials. In: Niederhuber JE, Armitage JO, Kastan MB, Doroshow JH, Tepper JE, editors. Abeloff’s Clinical Oncology, Sixth Edition. Philadelphia: Elsevier (2020). doi: 10.1016/B978-0-323-47674-4.00017-7
417. Kashyap H, Ahmed HA, Hoque N, Roy S, Bhattacharyya DK. Big Data Analytics in Bioinformatics: Architectures, Techniques, Tools and Issues. Netw Model Anal Health Inf Bioinf (2016) 5(1):28. doi: 10.1007/s13721-016-0135-4
418. Bersanelli M, Mosca E, Remondini D, Giampieri E, Sala C, Castellani G, et al. Methods for the Integration of Multi-Omics Data: Mathematical Aspects. BMC Bioinf (2016) 17(2):S15. doi: 10.1186/s12859-015-0857-9
419. Ritchie MD, Holzinger ER, Li R, Pendergrass SA, Kim D. Methods of Integrating Data to Uncover Genotype–Phenotype Interactions. Nat Rev Genet (2015) 16(2):85–97. doi: 10.1038/nrg3868
420. Kristensen VN, Lingjærde OC, Russnes HG, Vollan HKM, Frigessi A, Børresen-Dale A-L. Principles and Methods of Integrative Genomic Analyses in Cancer. Nat Rev Cancer (2014) 14(5):299–313. doi: 10.1038/nrc3721
421. Ahmed Z, Mohamed K, Zeeshan S, Dong X. Artificial Intelligence With Multi-Functional Machine Learning Platform Development for Better Healthcare and Precision Medicine. Database: J Biol Database Curation (2020) 2020:baaa010. doi: 10.1093/database/baaa010
422. Nagy M, Radakovich N, Nazha A. Machine Learning in Oncology: What Should Clinicians Know? JCO Clin Cancer Inf (2020) 4):799–810. doi: 10.1200/CCI.20.00049
423. Lin C, Karlson EW, Canhao H, Miller TA, Dligach D, Chen PJ, et al. Automatic Prediction of Rheumatoid Arthritis Disease Activity From the Electronic Medical Records. PloS One (2013) 8(8):e69932–2. doi: 10.1371/journal.pone.0069932
424. Norgeot B, Glicksberg BS, Trupin L, Lituiev D, Gianfrancesco M, Oskotsky B, et al. Assessment of a Deep Learning Model Based on Electronic Health Record Data to Forecast Clinical Outcomes in Patients With Rheumatoid Arthritis. JAMA Netw Open (2019) 2(3):e190606. doi: 10.1001/jamanetworkopen.2019.0606
425. Murakami S, Hatano K, Tan J, Kim H, Aoki T. Automatic Identification of Bone Erosions in Rheumatoid Arthritis From Hand Radiographs Based on Deep Convolutional Neural Network. Multimedia Tools Appl (2018) 77(9):10921–37. doi: 10.1007/s11042-017-5449-4
426. Üreten K, Erbay H, Maraş HH. Detection of Rheumatoid Arthritis From Hand Radiographs Using a Convolutional Neural Network. Clin Rheumatol (2020) 39(4):969–74. doi: 10.1007/s10067-019-04487-4
427. Guan Y, Zhang H, Quang D, Wang Z, Parker SCJ, Pappas DA, et al. Machine Learning to Predict Anti-Tumor Necrosis Factor Drug Responses of Rheumatoid Arthritis Patients by Integrating Clinical and Genetic Markers. Arthritis Rheumatol (2019) 71(12):1987–96. doi: 10.1002/art.41056
Keywords: rheumatoid arthritis, DMARDs, biomarker, treatment failure, precision medicine
Citation: Wang Z, Huang J, Xie D, He D, Lu A and Liang C (2021) Toward Overcoming Treatment Failure in Rheumatoid Arthritis. Front. Immunol. 12:755844. doi: 10.3389/fimmu.2021.755844
Received: 09 August 2021; Accepted: 06 December 2021;
Published: 23 December 2021.
Edited by:
Zahava Vadasz, Technion Israel Institute of Technology, IsraelReviewed by:
Achilleas Floudas, Trinity College Dublin, IrelandXianghang Luo, Central South University, China
Copyright © 2021 Wang, Huang, Xie, He, Lu and Liang. This is an open-access article distributed under the terms of the Creative Commons Attribution License (CC BY). The use, distribution or reproduction in other forums is permitted, provided the original author(s) and the copyright owner(s) are credited and that the original publication in this journal is cited, in accordance with accepted academic practice. No use, distribution or reproduction is permitted which does not comply with these terms.
*Correspondence: Chao Liang, bGlhbmdjQHN1c3RlY2guZWR1LmNu; Aiping Lu, YWlwaW5nbHVAaGtidS5lZHUuaGs=; Dongyi He, aGVkb25neWkxOTY3QHNodXRjbS5lZHUuY24=