- 1Institute for Regenerative Medicine & Biotherapy (IRMB), INSERM, Univ Montpellier, CHU Montpellier, Montpellier, France
- 2Evotec ID (Lyon), Lyon, France
Mycobacterium tuberculosis (Mtb), the etiological agent of tuberculosis, kills 1.5 to 1.7 million people every year. Macrophages are Mtb’s main host cells and their inflammatory response is an essential component of the host defense against Mtb. However, Mtb is able to circumvent the macrophages’ defenses by triggering an inappropriate inflammatory response. The ability of Mtb to hinder phagolysosome maturation and acidification, and to escape the phagosome into the cytosol, is closely linked to its virulence. The modulation of the host inflammatory response relies on Mtb virulence factors, but remains poorly studied. Understanding macrophage interactions with Mtb is crucial to develop strategies to control tuberculosis. The present study aims to determine the inflammatory response transcriptome and miRNome of human macrophages infected with the virulent H37Rv Mtb strain, to identify macrophage genetic networks specifically modulated by Mtb virulence. Using human macrophages infected with two different live strains of mycobacteria (live or heat-inactivated Mtb H37Rv and M. marinum), we quantified and analyzed 184 inflammatory mRNAs and 765 micro(mi)RNAs. Transcripts and miRNAs differently modulated by H37Rv in comparison with the two other conditions were analyzed using in silico approaches. We identified 30 host inflammatory response genes and 37 miRNAs specific for H37Rv virulence, and highlight evidence suggesting that Mtb intracellular-linked virulence depends on the inhibition of IL-1β-dependent pro-inflammatory response, the repression of apoptosis and the delay of the recruitment and activation of adaptive immune cells. Our findings provide new potential targets for the development of macrophage-based therapeutic strategies against TB.
Introduction
Tuberculosis is one of the top 10 causes of death (1) and, right after COVID-19 (2), is the world’s deadliest infection caused by a single infectious agent: Mycobacterium tuberculosis (Mtb) (3). Mtb is responsible for 1.5 to 1.7 million deaths each year, but unlike COVID-19, is more prevalent in low and middle income countries (4). Active tuberculosis infection (ATBI) is characterized by replicating and metabolically active bacteria and causes severe lung lesions while latent tuberculosis infection (LTBI) remains asymptomatic. Seventy percent of newly infected individuals efficiently clear the bacteria. However, for the remaining 30%, the immune system is only able to contain, but not to eradicate the bacteria (5). These people develop LTBI that can persist for their entire lifetime (6). However, approximately 5 to 10% of LTBIs evolve into ATBIs when the immune system is no longer able to constrain Mtb (5). The ability of Mtb to persist for so long in the host is due to its ability to hijack host defense mechanisms by converting macrophages into a permissive cellular niche (7, 8).
Macrophages are first-line antimicrobial cells in our body. They play a key role during Mtb infection by triggering an arsenal of immune responses (7). Macrophages are the most abundant cell type within the site of infection and represent the primary host cells for Mtb (9). Depending on the polarization of their immune response, macrophages ability to fight Mtb differs. Classically activated (M1-like) macrophages trigger a Th1 pro-inflammatory response and help to eliminate the bacteria. Alternatively, activated (M2-like) macrophages trigger a Th2 anti-inflammatory response and become long term hosts (10–13). Many studies describe a strong pro-inflammatory response promoting M1-like polarization, immediately after Mtb infection, followed later by a shift to an anti-inflammatory response and M2-like polarization (14–17). However, pro-inflammatory mechanisms do not always kill the bacteria and anti-inflammatory mechanisms are not always protective for the host. Inability to orchestrate a protective inflammatory response leads to poor clinical outcome (9). This might be the reason why innate immunity is often related to genetic susceptibility to tuberculosis (18–20) and why cytokine signaling and inflammation pathways are found to be the most induced processes during the infection (21, 22). MicroRNAs (miRNAs) are critical regulators of the fine-tuning of cytokine signaling, macrophage polarization, and inflammation. They are small endogenous non-coding RNA molecules, acting mainly as post-transcriptional repressors by targeting mRNAs on their 3’UTR (23–27). Several studies have explored the roles of host miRNAs in tuberculosis and showed that Mtb infection modifies the miRNome of macrophages (28–31). Mtb might thus influence the macrophage inflammatory response by modulating their miRNAs expression.
Mtb encompasses a group of genetically related species (named the Mtb complex) that are highly contagious airborne mycobacterial strains and can cause tuberculosis in humans (32). Mycobacterium (M.) marinum is one of the most closely related mycobacterium species to the Mtb complex and a commonly used pertinent intracellular infection model. Indeed, both thrive in the same macrophage compartments (33) and their survival in the immature phagosome is made possible through pathogen-dependent inhibition of phagosome-lysosome fusion. Then, both Mtb and M. marinum use the ESX-1 secretion system to escape the phagosome into the cytosol and thus to circumvent macrophage defenses (34–39). Cutaneous lesions caused either by Mtb or M. marinum form similar granulomas in humans (40), suggesting that they trigger comparable immune responses. The M. marinum ESX-1 system however confers reduced virulence, compared to that of Mtb (41), and human granulomatous lesions are less severe than those caused by Mtb infection (42). This suggests that phagosome escape is not the only cause of mycobacterial virulence. After phagosomal escape, Mtb must be able to reprogram macrophages’ defenses in a way that favors its virulence in humans in a more effective way than M. marinum.
Recent studies have indicated that genetic diversity within the Mtb complex can influence host inflammatory response to infection and during tuberculosis disease (43). Because Mtb strains that diminish protective cytokine secretion show enhanced virulence (44, 45), the present study aims at identifying how the virulent Mtb strain H37Rv specifically reprograms the inflammatory transcriptome and related miRNAs of macrophages in comparison with less virulent Mtb strains. compared with M. marinum. We thus compared macrophages’ inflammatory transcriptomes following infection with either the virulent Mtb strain H37Rv, M. marinum or the heat killed avirulent H37Ra strain (HKMT). M. marinum infection was used to filter the response specific for H37Rv with a less, yet virulent mycobacterial strain, which is also able to escape the phagosome and to trigger a cytosolic immune response. We used HKMT infection as a way to focus on both post-phagocytosic changes and to identify changes related to human-specific pathogen recognition. Indeed, in contrast to H37Rv, macrophages successfully eliminate HKMT in their phagolysosomes (46). As a macrophage model, we used PMA-differentiated THP-1 cells since they display similar properties compared to human monocyte-derived macrophages during Mtb infection (47). We identified an H37Rv-specific signature of inflammatory genes. We also identified a miRNA-based signature following H37Rv infection and provide potential mRNA/miRNA circuits related to H37Rv virulence in the context of the macrophage inflammatory response.
Materials and Methods
Mycobacterial Strains and Cultures
We used fluorescent H37Rv and M. marinum strains that were both obtained as a kind gift from IPBS, Toulouse. H37Rv and M. marinum cultures were grown at 37°C or 33°C, respectively, and 5% CO2 in Middlebrook 7H9 broth supplemented with 10% Middlebrook Oleic Albumin Dextrose Catalase (Difco, Livonia, MI). Cultures were grown to a mid-log phase (optical density 600 [OD600] of 0.6), then frozen with 10% glycerol at −80°C in 1.5 ml aliquots prior to infection. Heat inactivated H37Ra (HKMT) was purchased from Invivogen.
Cell Cultures
The human monocytic cell line THP-1 cells (TIB-202) were purchased from ATCC. Cells were cultured in 24 well plates (Corning) and differentiated into THP-1 derived macrophages 24 hours prior to infection with 40 ng/mL phorbol 12-myristate 13-acetate (PMA) in RPMI 1640 Medium GlutaMAX™ Supplement (Gibco) at 37°C and 5% CO2. Experiments were realized within 15 passages and cell viability was measured before experiments by trypan blue exclusion and was greater than 97%. CD14+ primary human monocytes were extracted from peripheral blood of 5 healthy donors anonymously provided by the French Blood Establishment (EFS, Lyon). CD14+ monocytes were purified from whole blood using an autoMACS® Pro Separator, Whole Blood Column Kit and StraightFrom® Whole Blood CD14 MicroBeads (Mitenyi) according to the manufacturer’s instructions. Cell viability was measured before proceeding to macrophage differentiation, by trypan blue exclusion and was always greater than 80%. CD14+ monocytes were then plated in 24 well plates (Corning) at a density of 400 000 cells per well and differentiated into macrophages for 5 days prior to infection with 50 µg/ml Rh-GMCSF (Miltenyi) in RPMI 1640 Medium GlutaMAX™ Supplement (Gibco) with 10% FBS (Sigma-Aldrich) at 37°C, 5% CO2.
Infections
Macrophages were infected with H37Rv or M. marinum at a multiplicity of infection (MOI) of 1:1 for 1 hour and at an MOI of 4: 1 for 3 hours, respectively, in RPMI 1640 medium GlutaMAX™ Supplement with 10% HiFBS. Macrophages stimulation with HKMT was performed with 50 µg/ml of HKMT for 3 hours in RPMI 1640 medium GlutaMAX™ Supplement with 10% HiFBS. Cells were then rinsed with phosphate buffered saline (PBS) to remove extracellular mycobacteria and further cultured in RPMI 1640 medium GlutaMAX™ Supplement with 10% HiFBS for 48 hours before RNA extraction. Uninfected cells were handled in the same conditions as infected cells and served as controls.
Gene and miRNA Profiling
Total RNA including miRNAs was extracted 2 days post-infection. Briefly, to protect RNA from degradation, macrophages were rinsed with PBS, then scraped with Maxwell® RSC miRNA Tissue Kit homogenization solution/thioglycerol (50/1) (Promega). Followed 10 min of incubation with Maxwell® RSC miRNA Tissue Kit lysis buffer (Promega), cells and bacteria were lysed by bead beating into Matrix B tubes containing silica beads (MP Biomedical) with the Super-Fast Prep-1 instrument (MP Biomedical). Finally, samples were processed into a Maxwell® RSC instrument for RNA extraction. RNA concentration was measured with QIAxpert System (Qiagen) and RNA integrity was evaluated by automated electrophoresis with TapeStation Systems (Agilent). Reverse transcription of total mRNA or miRNAs were performed with 500 ng total RNA using SuperScript™ IV VILO™ Master Mix or a Taqman™ microRNA Reverse Transcription kit (Applied Biosystems), respectively. qPCR amplifications were run with a QuantStudio™ 12K Flex system (Applied Biosystems) and using a customized TaqMan® Array (Table S1 in Supplementary Material) for mRNAs and the TaqMan® Array Human MicroRNA Card Set v3.0 dispatched in two pools: highly characterized miRNAs (pool A) and more recently discovered miRNAs (pool B) (Applied Biosystems) for miRNAs, according to the manufacturer’s instructions.
Data and Statistical Analysis
The data was analyzed using the ThermoFisher Connect™ online application (ThermoFisher). The mRNA content relative to the secreted proteins was normalized to GAPDH and GUSB expression, that of the receptors was normalized to GAPDH and TBP expressions and miRNA content was normalized using the global normalization method. Then, relative expression and expression fold changes were calculated following the ΔCt and 2ΔΔCt methods, respectively. Briefly: ΔCt = Ctgene – Ctendogenous control and ΔΔCt = ΔCt treated sample – ΔCt untreated control. Heatmaps and principal components analysis (PCA) were generated with Clustvis online software (48). All miRNA and gene Taqman Low Density Array data are available from the ncbi database: https://www.ncbi.nlm.nih.gov/geo/query/acc.cgi?acc=GSE165327. Genes and miRNAs interactions were further explored with the Ingenuity Systems™ Pathways Analysis (IPA) tool (http://www.ingenuity.com). We used GraphPad Prism 8 for statistical analysis. Student’s t-test was used on ΔCt to compare treated samples to their controls and on ΔΔCt to compare H37Rv-infected PMA differentiated THP-1 to H37Rv-infected CD14+ (monocyte-derived macrophages) MDM responses. ANOVA with multiple comparisons were used on ΔΔCt to compare H37Rv-infected macrophages to M. marinum-infected macrophages and to HKMT-stimulated macrophages. PCA and hierarchical or unsupervised clustering were performed using Clustvis online software with the correlation distance method and average linkage. Relative gene expression or gene expression fold changes as indicated were centered and unit variance scaled. Imputation was used for missing value estimation.
Results
Infection With Virulent Mycobacterium tuberculosis Leads to a Specific Deregulation of Macrophage Host Response-Related Genes
To compare the host inflammatory response induced by macrophages upon infection with a virulent or non-virulent mycobacterial strain, we used PMA-differentiated THP-1 cells as a human macrophage model and infected them with either the virulent H37Rv strain, or the occasional human pathogen M. marinum and the avirulent heat killed Mtb (HKMT). In this first attempt to dissect the host response associated with specific Mtb virulence, we decided to use the classical laboratory strain H37Rv as a surrogate for Mtb strains. Using RT-qPCR arrays, the host inflammatory response was evaluated by quantifying the expression level of 184 genes designed to encompass the full landscape of host immune responses (Table S1 in Supplementary Material). Among the 184 mRNAs tested, 98 genes were detectable (Ct values < 32) in THP-1, including 14 interleukins, 11 interleukin receptors, 23 chemokines, 12 chemokine receptors, 6 interferons, 2 interferon receptors, 11 tumor necrosis factor (TNF) superfamily members, 12 TNF receptors and 7 toll-like receptors (Table S2 in Supplementary Material). We used principal component analysis (PCA) and unsupervised hierarchical clustering to visualize the relationships between the three sample groups, based on the expression of these genes. PCA analysis displayed 60.5% of the dataset on the two principal components (Figure 1A), which was representative of the sample distribution. Interestingly, PCA showed that expression profiles obtained with M. marinum infection and HKMT stimulation were similar, as their distribution overlapped, and that both were very different from H37Rv infection. This was confirmed by the unsupervised hierarchical clustering analysis (Figure 1B). H37Rv-infected samples were found further from controls compared to the other two conditions on the PCA and heatmap (Figures 1A, B), showing that infection with H37Rv induced more drastic changes than the other two experimental conditions.
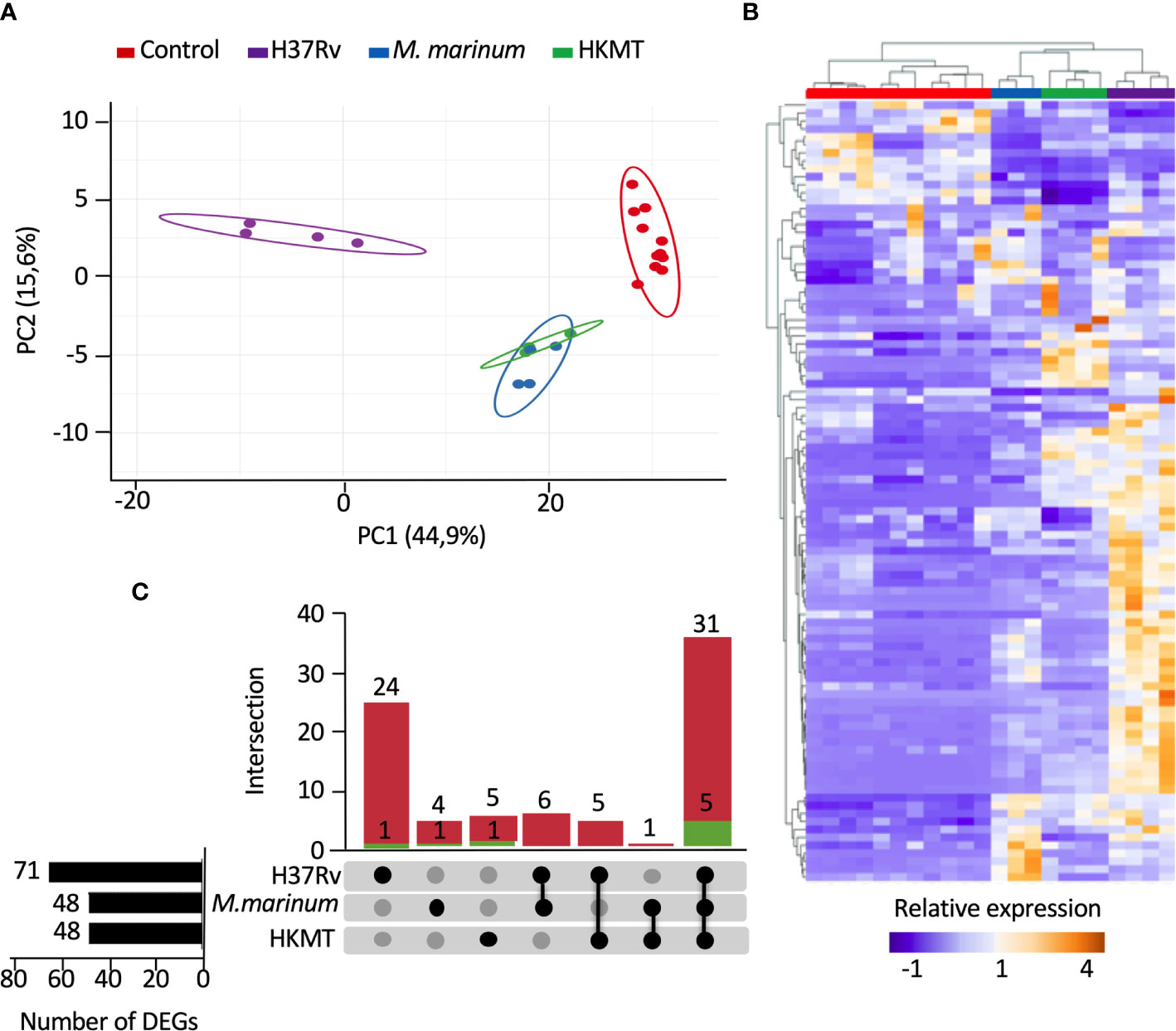
Figure 1 Infection with H37Rv led to a specific deregulation of macrophage inflammatory response-related genes. Macrophages were infected with H37Rv, M. Marinum or stimulated with HKMT for 48 hours, then total RNA was extracted and the expression levels of 184 host immune response-related genes were quantified using RT-PCR (n = 11 control, n = 3 M. Marinum, n=4 HKMT and H37Rv). For each experiment with the different mycobacterial strains, uninfected macrophages were used as control. Using Clustvis software, hierarchical clustering and principal component analysis were generated for genes, which after PCR had a CT value below 32 (98 genes). (A) Unsupervised PCA representation of the samples distribution depending on their overall relative gene expression following infection with H37Rv, M. marinum and stimulation with HKMT. Imputation was used for missing value estimation. (B) UpSet diagram representing the intersection of the DEGs relative to H37Rv or M. marinum infections or HKMT as compared with uninfected controls. In green: downregulated genes count, in red: upregulated genes count. (C) Unsupervised hierarchical clustering of the samples distribution depending on the overall relative expression of 93 genes following infection with H37Rv, M. marinum or stimulation with HKMT. Imputation was used for missing value estimation and samples were clustered using the correlation distance method and average linkage.
First, we assessed which genes are differentially expressed between uninfected and infected conditions. The UpSet diagram (49) represents the intersection between the three sets of differentially expressed genes (DEGs) from the three infections (Figure 1C). The data indicate that H37Rv infection significantly deregulated 71 DEGs, whereas both other conditions only modulated 48 DEGs. While 36 DEGs were shared between the three conditions, 25 DEGs were specific for H37Rv infection and only 5 and 6 DEGs for M. marinum and HKMT, respectively. Overall, our data showed that H37Rv infection induced greater changes to the host response, with more DEGs and higher fold changes.
Second, to identify how macrophages specifically respond to H37Rv, we compared the expression levels of all DEGs from the three infections (Table 1 and Figure 2A). For each DEG, we considered that the expression was specific for H37Rv infection if the fold change versus M. marinum or HKMT was greater than or equal to 2 and p-value < 0.05. Among the 25 DEGs specific for H37Rv, six genes [CCL17, CCL24, PPBP (CXCL7), CXCR5, CCR9 and TLR7] were significantly deregulated following H37Rv infection only and not in the other two conditions. On the other hand, IL24, IL31RA and TNFSF14 were not significantly deregulated by H37Rv, whereas they were deregulated by HKMT, M. marinum, and both, respectively (Table S2 in Supplementary Material).
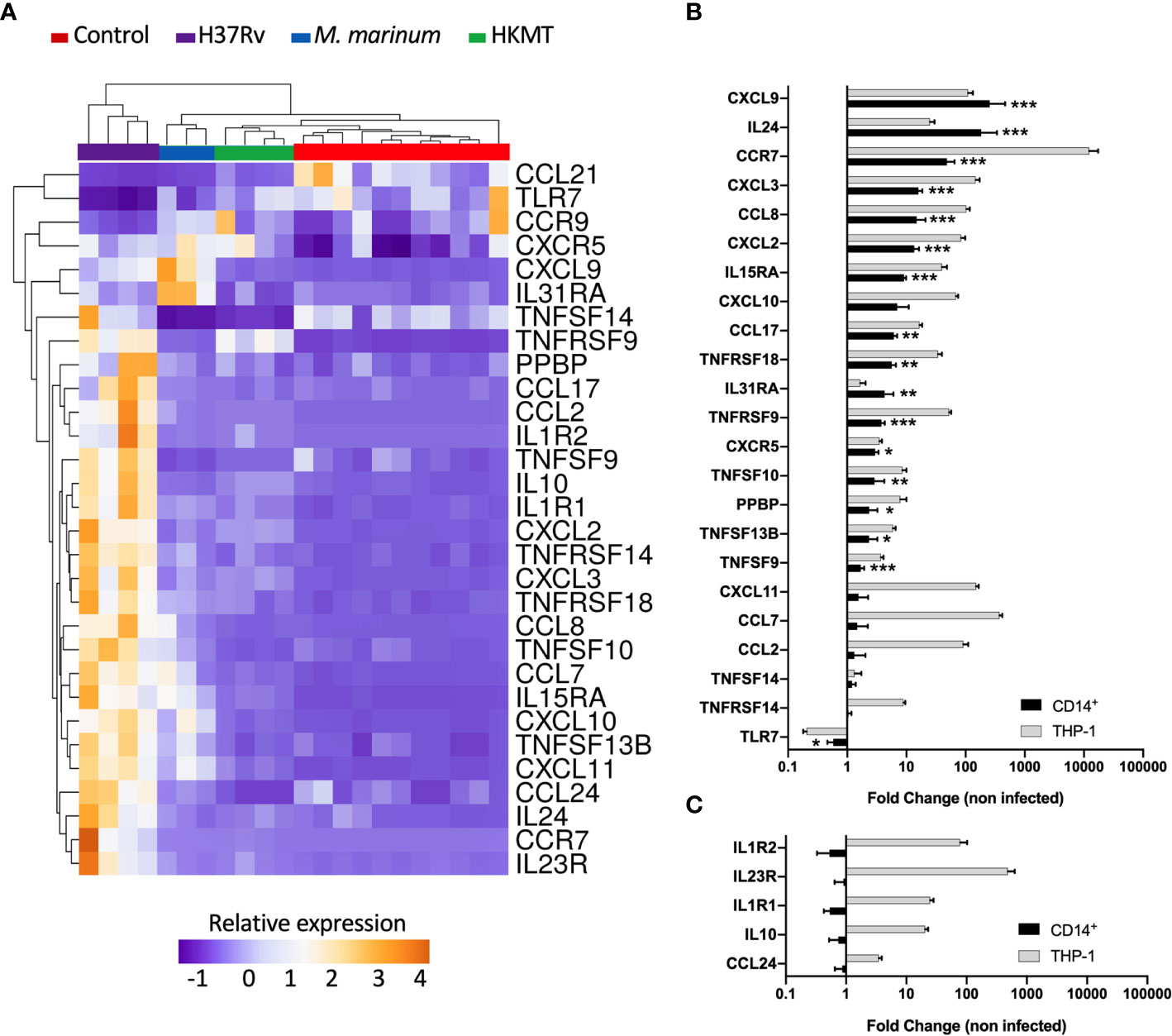
Figure 2 Infection with H37Rv led to a specific and significantly different host inflammatory response. (A) THP-1 differentiated into macrophages were infected with H37Rv, M. Marinum or stimulated with HKMT for 48 hours, then total RNA was extracted and the expression level of the 184 host immune response-related genes were quantified using RT-PCR (n = 11 control, n = 3 M.marinum, n=4 HKMT and H37Rv). For each experiment with the different mycobacterial strains, uninfected macrophages were used as control, independently. Using Clustvis software, a supervised hierarchical clustering was generated for the 98 genes with CT value below 32. Supervised hierarchical clustering of macrophage samples distribution depending on the relative expression of genes that were significantly deregulated by H37Rv infection as compared with both M. marinum infection and HKMT stimulation. Statistical analysis was performed on ΔΔCt using ANOVA and multiple comparisons. Imputation was used for missing value estimation. Samples were clustered using the correlation distance method and average linkage. (B, C) THP-1 and CD14+ MDM were infected with H37Rv for 48 hours, then total RNA was extracted and the expression level of the 30 H37Rv-specific host inflammatory response was quantified using RT-PCR (n = 4). Graphs represent gene expression fold change compared to uninfected cells. Statistical analysis was performed on ΔΔCt using Student’s t-test. *p value < 0.05, **p value < 0.01, ***p value < 0.001.
The majority of the genes that had an expression level specific for H37Rv infection belonged to the common DEGs induced by the three pathogens. Indeed, the H37Rv-specific host response led to a more drastic deregulation of 12 genes by either inducing upregulation of CCR7, IL1R1, TNFRSF9, TNFRSF18, IL1R2, CCL7, IL10, TNFRSF14, CXCL2, CXCL3, CCL2 and IL15RA or inducing downregulation of CCL21. CCR7 and IL23R both ranked as the first and second most upregulated genes, with fold changes equal to 19 and 115 for CCR7, and 50 and 123 for IL23R, respectively. TLR7 and CCL21 were the only two downregulated genes following H37Rv infection with respect to the uninfected controls. In addition, both genes were even more downregulated when comparing infection with H37Rv and with M. marinum or HKMT. CCL21 ranked as the most downregulated gene with a 16-fold downregulation in H37Rv infection as compared with the other two conditions. H37Rv-infection of macrophages did not always lead to extreme expression profiles. Indeed, CXCL11, TNFSF13B, TNFSF10, IL31RA, CXCL9, CXCL10, CCL8 expressions were intermediate, all downregulated as compared with M. marinum infection and upregulated as compared with HKMT stimulation. Overall, the H37Rv-specific host inflammatory response included 30 genes (Figure 2A and Table 1). Finally, we validated this finding in primary human macrophages. Using RT-qPCR, we quantified the expression level of the 30 H37Rv-specific genes in Mtb-infected macrophages differentiated from blood CD14+ monocytes of healthy donors. Results showed that expression levels were comparable between the human THP-1 macrophage cell line and primary macrophages for more than 80% of the genes (Figure 2B). Only 5 out of 30 genes did not show the same variation (Figure 2C). While IL1R2, IL23R, IL1R1, IL-10, and CCL24 were highly upregulated upon infection of THP-1 macrophages, their expression was barely affected upon infection of primary macrophages.
H37Rv Infection Leads to the Modulation of 37 miRNAs
To further decipher virulence-mediated changes in the host response, we performed a global miRNA profiling of H37Rv-infected macrophages (Figure 3, Table 2). Of the 765 human miRNAs analyzed, up to 54.8% were detectable with a CT value < 32. When considering a fold change > 2 and a p-value < 0.05, 37 miRNAs were significantly deregulated following H37Rv infection as compared to the uninfected controls. Quantification of human miRNA expression levels showed that 17 miRNAs were upregulated and 20 were downregulated. As miRNAs belonging to the same family or cluster suggests that they co-regulate the same biological pathway, it seems relevant to highlight that, among the 37 differentially expressed miRNAs, there were two miRNA families: miR-30 family (miR-30a and miR-30b) and miR-181 family (miR-181a-1-3p and miR-181a-2-3p). We also observed that the miR-132/212 cluster was significantly increased. Using a miRNome published dataset of CD14+ isolated from the blood of TB patients (GSE70425), we compared the expression levels of the 37 H37Rv-related miRNAs between Mtb-infected THP-1 and primary CD14+. Results showed that 80% of miRNAs displayed comparable expression levels between both conditions (Figure S1 in Supplementary Material).
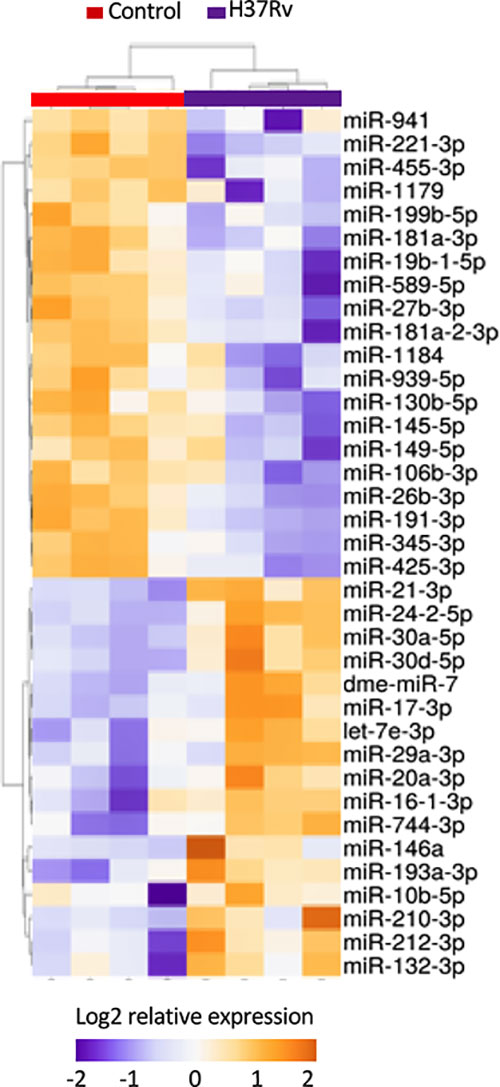
Figure 3 Expression levels of miRNAs significantly deregulated after H37Rv infection. THP-1 differentiated into macrophages were infected with H37Rv for 48 hours (n = 4). After total RNA including small RNA extraction, the expression level quantification of 762 human miRNAs was performed using TaqMan® Array Human MicroRNA Card. Using Clustvis software, a supervised hierarchical analysis of the distribution of macrophage samples based on relative log2 expression of 37 miRNAs significantly modified by H37Rv infection compared to uninfected controls. Statistical analysis was performed on ΔCt using Student’s t test to identify the differently expressed miRNAs compared with uninfected controls. Imputation was used for missing value estimation. Samples were clustered using the correlation distance method and average linkage.
mRNA/miRNA Inflammatory Pathways Specific for H37Rv
To identify predicted target genes and related pathways and to provide experimentally verified information on miRNA-target interactions, we performed an automated text-mining search analysis. The thirty seven miRNAs identified as dysregulated in H37Rv-infected macrophages were examined using miRWalk database (50). We found between 11 and 699 putative target genes for each of the 37 miRNAs. We applied a filter consisting in identifying genes targeted by at least 3 miRNAs and found 320 genes (Table S3 in Supplementary Material). Using the Enrich database (51), gene ontology analysis revealed significant pathways with high Enrich combined score level such as cell cycle, FoxO signaling and P53 pathway with adjusted p-values 3.710-7, 1.110-5 and 6.210-5, respectively (Table S4 in Supplementary Material). Interestingly, we found that 28 genes were targeted by at least five miRNAs (Table S3 in grey color) and almost 70% of them have already been described as involved in Mtb infection (Table S3 in Supplementary Material). The HSPA1B gene, also called HSP72, is targeted by 9 miRNAs, and the two genes LDLR and NUFIP2 are targeted by 7 miRNAs. The integrative analysis of the 30 and 37 H37Rv-specific host inflammatory response genes and miRNAs, respectively, has been carried out to find possible relationships. In this attempt, we used the Ingenuity Pathway Analysis software program, associated with a miRNA target filter analysis, to identify miRNA-target genes and mRNA-mRNA interactions (Figure 4). miR-939-5p, miR-1184, miR-20a-3p, miR-193a-3p, miR-345-p, miR-26b-3p, miR146a-5p, miR-941, miR589-5p, miR-130b-5p, miR-27b-3p, miR-221-3p, miR-744-3p, miR-425-3p, miR-132-3p, miR-181a-1-3p, miR-181a-2-3p, miR-19b-1-5p, miR-30a-5p, miR-1179, miR-455-3p, miR-199b-5p and miR-21-3p, all targeted at least one inflammatory response genes related to Mtb virulence. Together, these 23 miRNAs might regulate 21 of the 34 H37Rv-specific genes: CCL21, CXCL2, CXCL3, CCL8, IL10, PPBP, TNFSF10, CXCL11, TNFSF13B, CXCL9, IL24, CCL17, CXCL10, TNFRSF18, TNFRSF14, TNFRSF9, IL15RA, IL1R1, CCR9, CXCR5 and IL23R. Some miRNAs targeted more than 3 genes and thus might be more involved in the H37Rv-specific host inflammatory response than others. MiR-939-5p, miR-345-5p, miR-130b-5p, miR-425-5p, miR-26b-3p targeted 3 to 4 H37Rv-specific host inflammatory response genes and miR-146a targeted 6 genes. The most targeted H37Rv-specific host inflammatory response genes were CXCL2, CXCL11, CCR9, TNFSF13B, CXCL9 and IL23R. miR-939-5p, miR-1184, miR-345-5p, miR-26b-3p, miR-941, miR-589-5p, miR-130-5p, miR-27b-3p, miR-221-3p, miR-425-3p, miR-181a-1-3p, miR-19b-1-5p, miR-1179, miR-455-3p, miR-119b-5p and miR-181a-2-3p targeted TNFRSF18/TNFRSF14, CXCL2, CCL8/TNFSF13B/CXCR5, CXCL2/CXCL3/TNFRSF9, IL15RA, PPBP, CXCL2/TNFSF10/CCR9, CXCL11/CCR9, CXCL11, CXCL9/CXCR5/IL23R, CXCR5, IL23R, TNFSF13B/CXCL10, CXCL9, IL23R/IL24 and CXCL10 with negative correlations (i.e. downregulated miRNAs correlated to upregulated genes), respectively. Altogether, 61% of the H37Rv-specific host inflammatory response gene set was targeted by 62% of the H37Rv deregulated miRNAs.
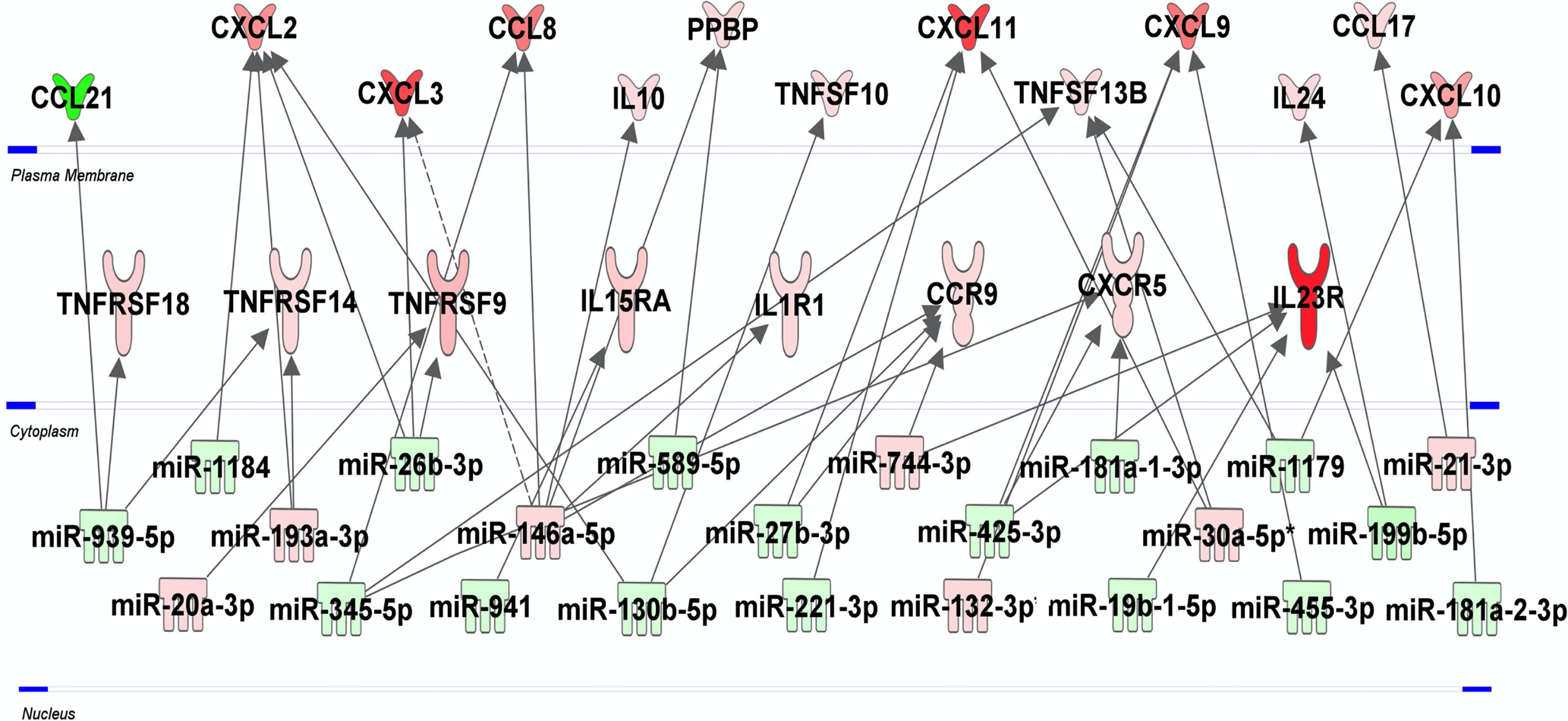
Figure 4 miRNA-mRNA networks generated with Ingenuity Pathway Analysis (IPA) for H37Rv-infected human macrophages. IPA analysis was performed to investigate the connection between the 30 and 37 H37Rv-specific host inflammatory response gene set and miRNAs, respectively. Filters were set to only include experimentally observed or high-confidence-predicted miRNA-mRNA interaction partners. Genes and miRNAs were colored in red or green depending on whether they were upregulated or downregulated (respectively) with respect to their uninfected controls and the shading intensity shows to what degree each miRNA or gene was deregulated. Direct and indirect miRNA-gene interactions are represented with a solid and dotted lines, respectively.
Discussion
Macrophages are the primary host for Mtb and their inflammatory response is critical for the infection outcome (9). Although Mtb and M. marinum can both circumvent the phagolysosome fusion and escape to the cytosol, Mtb remains more virulent than M. marinum in humans. We hypothesized that Mtb virulence is enhanced by its capacity to alter the host inflammatory response. We sought to identify H37Rv-specific mRNA and miRNA genes, to identify pathways of the macrophage inflammatory response that might be related to Mtb virulence. As a model for human macrophages, we used the PMA-differentiated THP-1 cell line (47) to compare the transcriptomic changes induced in the inflammatory response by either H37Rv, M. marinum or HKMT. We identified 30 genes and 37 miRNAs that were differently expressed upon H37Rv infection and studied their relationships and associated cellular signaling pathways. Importantly, at least 80% of the H37Rv-specific genes and miRNAs identified with the THP1 model are confirmed in primary macrophage cells, validating our experimental approach.
Our unbiased analysis of the H37Rv–related miRNAs with their target genes experimentally validated, revealed their involvement in Mtb infection. HSP72 and LDLR were the most targeted genes by the H37Rv-specific miRNAs. These genes are involved in the interaction between neutrophils and macrophages during the early phase of the innate immune response to Mtb infection (52) as well as in the control of infectious load and infection-induced changes in lipid metabolism (53), respectively.
Most of the 30 H37Rv-specific host inflammatory response genes identified in the present study are upregulated to affect positively the host-response. These genes lie into the categories of receptors and molecules involved in pro-inflammatory macrophage differentiation (54), recruitment and activation of innate and adaptive immune cells (54–68), type 1 interferon and IFN-γ response (69, 70, 71), and apoptosis (72). Almost 30% of those genes are found deregulated in the blood of TB patients and have already been reported to be upregulated during Mtb infection, entailing positive effects on macrophage defense (58, 61, 66, 68, 70, 71, 73–81). Interestingly, miR-132-3p, miR-146a-5p, miR-345-5p and miR-939, which each target as many as 3 or 4 of the H37Rv-specific host inflammatory response genes, have been reported to be implicated in TB or other cellular processes that are or might be relevant to TB. Our IPA analysis of the H37Rv-specific host inflammatory response genes and miRNAs allowed us to identify strong links between Mtb virulence and these genes, as well as their associated pathways.
Focusing on genes that might benefit or alter macrophages defenses, and pathways in which genes are most targeted by identified miRNAs, we then tried to understand how H37Rv circumvents the macrophage inflammatory response (Figure 5). Among the strongest H37Rv-specific host genes/miRNAs displaying inverse expression levels, CXCL2 is over-expressed following H37Rv infection, and targeted by 3 miRNAs (miR-1184, miR-26b-3p and miR-130b-5p). It has been shown that blocking CXCL2 expression significantly reduces Mtb-induced, but not M. bovis BCG-induced, IL-1β secretion through the NLRP3-dependent inflammasome (76, 82), implying that its regulation is related to Mtb virulence. The pro-inflammatory cytokine IL-1β is essential for Mtb control, responsible for activation, for classical inflammatory polarization of macrophages, and takes part in many defense mechanisms against Mtb including ROS production (83, 84). Sustained upregulation of IL-1β leads to detrimental effects of exacerbated inflammation and is associated with TB severity and immunopathology (85–87). The downregulation of miR-1184, miR-26b-3p and miR-130b-5p expressions by H37Rv, might favor CXCL2 upregulation and the subsequent IL-1β production. In addition, the expression level of TNFRS18, which is expressed on macrophages, and functions as an inflammatory enhancer by promoting the activation of the NLRP3 inflammasome (88), is inversely correlated with that of miR-939-3p. Interestingly, miR-939-3p directly inhibits iNOS in mouse macrophages (89). iNOS exerts a strong bactericidal activity against Mtb, while preventing an excess of inflammation by inhibiting NLRP3-dependent IL-1β production and the NF-κB pathway (90–92). Moreover, miR-26b-3p, which is thought to participate in NLRP3-dependent IL-1β production through regulation of CXCL2, has been shown to participate in the inflammatory response of LPS-stimulated alveolar macrophages by modulating the NF-κB pathway by regulating PTEN (83). Finally, miR-939-3p and miR-26b-3p, found downregulated in H37Rv-infected macrophages, suggests that NF-κB plays an important role in H37Rv virulence probably through a dialog involving the NLRP3 inflammasome (84, 85). As for many regulatory mechanisms involved in inflammation, these H37Rv-specific gene/miRNA interactions controlling IL-1β might benefit both the macrophage and H37Rv, and highlight the crucial implication of IL-1β-related pathways and the control of pro-inflammatory activation in the virulence of Mtb.
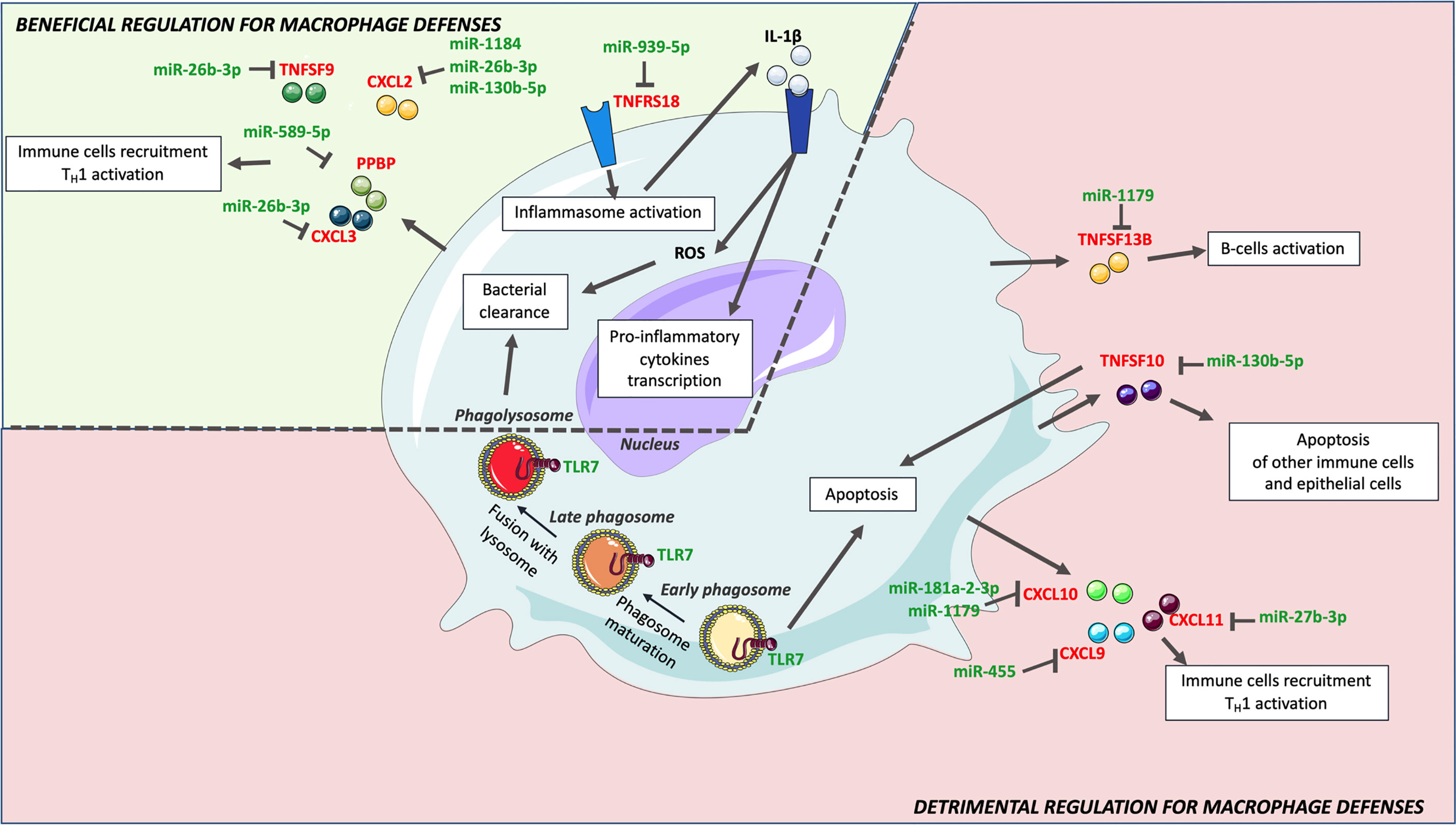
Figure 5 H37R-specific macrophage inflammatory response genes and associated miRNAs. Upon H37Rv infection, macrophages upregulated CXCL2, CXCL3, IL24, PPBP, TNFRSF18 and TNSF9, which have a beneficial effect on macrophages defense against Mtb. Downregulation of miR-19b-1-5p, miR-26b-3p, miR-130b-5p, miR-939-5p, miR-589-5p and miR-1184 might however limit the expression of these genes. Although H37Rv led to upregulation of CCL8, CCL17, CXCL9, CXCL10, CXCL11, TNFSF10 and TNFSF13B, their expression levels were significantly lower than that induced by Mm-infection. Hence, H37Rv mediated lower expression of these genes might rather be detrimental for macrophage defense. Their expression might be limited by miR-27b-3p, miR-130b-5p, miR-181a-2-3p, miR-425p, miR-455 and miR-1179.
Durable Mtb survival is only possible if their host-cells do not trigger cell death. In the early infection, apoptosis has been shown to be detrimental for Mtb to establish a long-term infection (86). TNFSF10, previously reported to be strongly induced by Mtb infection (87), prevents alveolar epithelial cell-mediated wound repair by inducing their apoptosis (93). These data suggest that TNFSF10 could promote fibrosis of the external layers of the granuloma and favor both the containment and the protection of the bacteria. TNFSF10 is putatively targeted by miR-130b-5p, which is downregulated in H37Rv-infected macrophages in the present study. In addition to the fact that this miRNA has already been shown to be downregulated in Mtb–infected primary human macrophages (94), P Ahluwalia et al. propose that miR-130b is involved in M1/M2 macrophages plasticity and Mtb survival (95). The expression of TLR7, an endosomal receptor expressed by macrophages to detect microbes single stranded RNA (96) and trigger apoptosis (97), is not modulated by M. marinum but significantly downregulated by H37Rv, which could also be beneficial for Mtb adaptation to the intracellular life survival process. Moreover, predicted miRNAs associated to the regulation of H37Rv-specific host inflammatory response genes were also involved in the regulation of apoptosis. miR-27b-3p, which expression is decreased upon H37Rv infection, favors apoptosis and decreases bacterial burden in Mtb-infected mouse macrophages (98). MiR-193a-3p and miR-1184, which expressions are decreased upon H37Rv infection, and both putatively target CXCL2, are also involved in apoptosis. miR-193a-3p upregulation triggers apoptosis through the direct repression of Mcl-1 expression (99), while miR-1184 promotes apoptosis via downregulation of its target CSNK2A1 (100). Reduced expression levels of TNFSF10, TLR7, miR-27b-3p, and miR-1184, in H37Rv-infected macrophages as compared with M. marinum -infected cells are concordant with apoptosis being reduced in alveolar macrophages infected with the virulent H37Rv strain as compared with attenuated strains such as M. bovis BCG and M. kansasii (101). The specific inverse regulation of these genes and miRNAs induced by H37Rv might inhibit apoptosis and enhance Mtb virulence.
Another factor that promotes Mtb long-term infection as compared to other pathogens, is the delay between the activation of innate immunity and the recruitment and activation of adaptive immunity (102). The CXCL9, CXCL10, CXCL11/CXCR3 axis and TNFSF13B play a role in both these recruitment and activation processes, responsible for attracting Th1 cells, cytotoxic lymphocytes and natural killer cells to the site of the infection, and related to B-cell activation, respectively (65). Although they were upregulated in H37Rv-infected macrophages, this upregulation was lower than that induced by M. marinum, suggesting that they are related to Mtb virulence. The fact that three of these genes are among the 6 most miRNA-targeted genes (i.e. CXCL9, CXCL11 and TNFRSF13B) emphasizes the importance of their role and might explain their lowered expression levels. Upregulation of miR-30a-5p and miR-30d-5p might lower the expression of CXCL11 and TNFSF13B, and upregulation of miR-132-3p that of CXCL9. Hence, modulating the expression of those genes and miRNAs might delay the adaptive immunity response and generate permissive conditions for Mtb survival (102). Additionally, miR-30a-5p upregulation is deleterious for Mtb-infected THP-1 cells, as it directly targets MyD88 (103), which is necessary for the establishment of a proper adaptive immune response in many pathologies (104–106). Furthermore, H37Rv-specific modulation of CCL8, CCL21 and CXCL2 might also contribute to impairing adaptive immunity. CCL8 is involved in T-cell activation (107–109) and CCL21 is a pro-inflammatory cytokine responsible for T-cells attraction to the lungs (110). Hence, as compared with M. marinum infection, lowered expression levels of CXCL9, CXCL11, TNFSF13B and CCL8 and CCL21, as well as upregulation of CXCL2 and miR-30a-5p, might enable H37Rv to expand its population before the burst of adaptive immunity. Consistently with our findings, Goenka et al. (111) also observed lower expression of CXCL9, CXCL10, CXCL11 and higher expression of CXCL2 in infant alveolar macrophage than in adult counterparts. With infant alveolar macrophages being more permissive to Mtb infection than adult macrophages (111), this is further evidence that CXCL2, CXCL9, CXCL10 and CXCL11 are likely involved in Mtb virulence.
Conclusion
The fragile balance between beneficial and harmful induction of inflammation might confer to genes and miRNAs paradoxical effects in macrophage defense and Mtb virulence. CXCL2 induction might both favor IL-1β-mediated protective immunity and impair the recruitment of adaptive immunity. In the broad spectrum of macrophage polarization and corresponding inflammatory responses, Mtb intracellular-linked virulence might depend mostly on: i) the inhibition of IL-1β-dependent pro-inflammatory polarization, ii) the repression of apoptosis, as well as iii) the delay of the adaptive immunity recruitment and activation (Figure 5). In this context, macrophage antimicrobial defenses remain low and, once activated, the adaptive immunity maintains this permissive environment.
These miRNA and gene signatures could be the hallmark of the host inflammatory response contribution to Mtb virulence and associated pathways could lead to new host directed therapies.
Data Availability Statement
The datasets presented in this study can be found in online repositories. The names of the repository/repositories and accession number(s) can be found in the article/Supplementary Material.
Author Contributions
ID-R, FA, and CR supervised the work and designed the experiments. Experimental work was performed by PB, FS, SS, PL, KK, NC and SL. PB, FA, CR, and ID-R analyzed and interpreted the data, and drafted the manuscript. All authors contributed to the article and approved the submitted version.
Funding
This research was initiated by Sanofi, funded by INSERM (Institut National de la Santé et Recherche Médicale), the University of Montpellier and EVOTEC ID, Lyon. PB received a fellowship from Association Nationale Recherche Technologie (ANRT, convention Cifre N°2017/1678).
Conflict of Interest
Authors PB, FS, SS, PL, KK, NC, SL and CR were employed by the company Evotec ID.
The remaining authors declare that the research was conducted in the absence of any commercial or financial relationships that could be construed as a potential conflict of interest.
The authors declare that this study received funding from Evotec ID. The funder had the following involvement with the study: sharing H37rv related protocols, managing training and BSL3 space relative to this study, interpreting data and participating to project meetings.
Acknowledgments
We acknowledge support from A. Alam and J. Montegut for scientific advice and A. Upton for careful reading of the manuscript.
Supplementary Material
The Supplementary Material for this article can be found online at: https://www.frontiersin.org/articles/10.3389/fimmu.2021.668060/full#supplementary-material
References
1. World Health Organization. Global Tuberculosis Report 2019. World Health Organization (2019). Available at: https://apps.who.int/iris/handle/10665/329368
2. Diseases TLI. Tuberculosis and Malaria in the Age of COVID-19. Lancet Infect Dis (2021) 21:1. doi: 10.1016/S1473-3099(20)30946-4
3. WHO. Global Tuberculosis Report (2019). Available at: https://www.who.int/tb/publications/global_report/en/ (Accessed 2 July 2020).
4. Hogan AB, Jewell BL, Sherrard-Smith E, Vesga JF, Watson OJ, Whittaker C, et al. Potential Impact of the COVID-19 Pandemic on HIV, Tuberculosis, and Malaria in Low-Income and Middle-Income Countries: A Modelling Study. Lancet Global Health (2020) 8:e1132–41. doi: 10.1016/S2214-109X(20)30288-6
5. Bibbins-Domingo K, Grossman DC, Curry SJ, Bauman L, Davidson KW, Epling JW, et al. Screening for Latent Tuberculosis Infection in Adults: US Preventive Services Task Force Recommendation Statement. JAMA (2016) 316:962–9. doi: 10.1001/jama.2016.11046
6. Young D, Hussell T, Dougan G. Chronic Bacterial Infections: Living With Unwanted Guests. Nat Immunol (2002) 3:1026–32. doi: 10.1038/ni1102-1026
7. Flynn JL, Chan J, Lin PL. Macrophages and Control of Granulomatous Inflammation in Tuberculosis. Mucosal Immunol (2011) 4:271–8. doi: 10.1038/mi.2011.14
8. Schlossberg D. Acute Tuberculosis. Infect Dis Clinics North America (2010) 24:139–46. doi: 10.1016/j.idc.2009.10.009
9. Huynh KK, Joshi SA, Brown EJ. A Delicate Dance: Host Response to Mycobacteria. Curr Opin Immunol (2011) 23:464–72. doi: 10.1016/j.coi.2011.06.002
10. Paige C, Bishai WR. Penitentiary or Penthouse Condo: The Tuberculous Granuloma From the Microbe’s Point of View. Cell Microbiol (2010) 12:301–9. doi: 10.1111/j.1462-5822.2009.01424.x
11. Rubin EJ. The Granuloma in Tuberculosis — Friend or Foe? New Engl J Med (2009) 360:2471–3. doi: 10.1056/NEJMcibr0902539
12. Russell DG, VanderVen BC, Lee W, Abramovitch RB, Kim M, Homolka S, et al. Mycobacterium Tuberculosis Wears What It Eats. Cell Host Microbe (2010) 8:68–76. doi: 10.1016/j.chom.2010.06.002
13. Russell DG, Barry CE, Flynn JL. Tuberculosis: What We Don’t Know Can, and Does, Hurt Us. Science (2010) 328:852–6. doi: 10.1126/science.1184784
14. Benoit M, Desnues B, Mege J-L. Macrophage Polarization in Bacterial Infections. J Immunol (2008) 181:3733–9. doi: 10.4049/jimmunol.181.6.3733
15. Hernández-Pando R, Orozcoe H, Sampieri A, Pavón L, Velasquillo C, Larriva-Sahd J, et al. Correlation Between the Kinetics of Th1, Th2 Cells and Pathology in a Murine Model of Experimental Pulmonary Tuberculosis. Immunology (1996) 89:26–33.
16. Lugo-Villarino G, Hudrisier D, Benard A, Neyrolles O. Emerging Trends in the Formation and Function of Tuberculosis Granulomas. Front Immunol (2013) 3:405. doi: 10.3389/fimmu.2012.00405
17. Méndez-Samperio P. Expression and Regulation of Chemokines in Mycobacterial Infection. J Infect (2008) 57:374–84. doi: 10.1016/j.jinf.2008.08.010
18. Philips JA, Ernst JD. Tuberculosis Pathogenesis and Immunity. Annu Rev Pathol Mech Dis (2012) 7:353–84. doi: 10.1146/annurev-pathol-011811-132458
19. Low Penetrance, Broad Resistance, and Favorable Outcome of Interleukin 12 Receptor β1 Deficiency. (Accessed 2 July 2020). Rockefeller University Press.
20. Impairment of Mycobacterial Immunity in Human Interleukin-12 Receptor Deficiency. (Accessed 2 July 2020).
21. Wu K, Dong D, Fang H, Levillain F, Jin W, Mei J, et al. An Interferon-Related Signature in the Transcriptional Core Response of Human Macrophages to Mycobacterium Tuberculosis Infection. PloS One (2012) 7:e38367. doi: 10.1371/journal.pone.0038367
22. Jenner RG, Young RA. Insights Into Host Responses Against Pathogens From Transcriptional Profiling. Nat Rev Microbiol (2005) 3:281–94. doi: 10.1038/nrmicro1126
23. Eulalio A, Schulte L, Vogel J. The Mammalian MicroRNA Response to Bacterial Infections. RNA Biol (2012) 9:742–50. doi: 10.4161/rna.20018
24. Wei Y, Schober A. MicroRNA Regulation of Macrophages in Human Pathologies. Cell Mol Life Sci (2016) 73:3473–95. doi: 10.1007/s00018-016-2254-6
25. Xiao C, Rajewsky K. MicroRNA Control in the Immune System: Basic Principles. Cell (2009) 136:26–36. doi: 10.1016/j.cell.2008.12.027
26. Forster SC, Tate MD, Hertzog PJ. MicroRNA as Type I Interferon-Regulated Transcripts and Modulators of the Innate Immune Response. Front Immunol (2015) 6:334. doi: 10.3389/fimmu.2015.00334
27. Gantier MP, Sadler AJ, Williams BRG. Fine-Tuning of the Innate Immune Response by MicroRNAs. Immunol Cell Biol (2007) 85:458–62. doi: 10.1038/sj.icb.7100091
28. Rajaram MVS, Ni B, Dodd CE, Schlesinger LS. Macrophage Immunoregulatory Pathways in Tuberculosis. Semin Immunol (2014) 26:471–85. doi: 10.1016/j.smim.2014.09.010
29. Sabir N, Hussain T, Shah SZA, Peramo A, Zhao D, Zhou X. miRNAs in Tuberculosis: New Avenues for Diagnosis and Host-Directed Therapy. Front Microbiol (2018) 9:602. doi: 10.3389/fmicb.2018.00602
30. Behrouzi A, Alimohammadi M, Nafari AH, Yousefi MH, Riazi Rad F, Vaziri F, et al. The Role of Host Mirnas on Mycobacterium Tuberculosis. ExRNA (2019) 1:40. doi: 10.1186/s41544-019-0040-y
31. Kim JK, Kim TS, Basu J, Jo E-K. MicroRNA in Innate Immunity and Autophagy During Mycobacterial Infection. Cell Microbiol (2017) 19:e12687. doi: 10.1111/cmi.12687
32. Kaufmann SH. How Can Immunology Contribute to the Control of Tuberculosis? Nat Rev Immunol (2001) 1:20–30. doi: 10.1038/35095558
33. Clemens DL. Characterization of the Mycobacterium Tuberculosis Phagosome. Trends Microbiol (1996) 4:113–8. doi: 10.1016/0966-842X(96)81528-9
34. Bottai D, Di Luca M, Majlessi L, Frigui W, Simeone R, Sayes F, et al. Disruption of the ESX-5 System of Mycobacterium Tuberculosis Causes Loss of PPE Protein Secretion, Reduction of Cell Wall Integrity and Strong Attenuation. Mol Microbiol (2012) 83:1195–209. doi: 10.1111/j.1365-2958.2012.08001.x
35. Jamwal SV, Mehrotra P, Singh A, Siddiqui Z, Basu A, Rao KVS. Mycobacterial Escape From Macrophage Phagosomes to the Cytoplasm Represents an Alternate Adaptation Mechanism. Sci Rep (2016) 6:23089. doi: 10.1038/srep23089
36. Ehrt S, Schnappinger D. Mycobacterial Survival Strategies in the Phagosome: Defense Against Host Stresses. Cell Microbiol (2009) 11:1170–8. doi: 10.1111/j.1462-5822.2009.01335.x
37. Simeone R, Bobard A, Lippmann J, Bitter W, Majlessi L, Brosch R, et al. Phagosomal Rupture by Mycobacterium Tuberculosis Results in Toxicity and Host Cell Death. PloS Pathog (2012) 8:e1002507. doi: 10.1371/journal.ppat.1002507
38. Smith J, Manoranjan J, Pan M, Bohsali A, Xu J, Liu J, et al. Evidence for Pore Formation in Host Cell Membranes by ESX-1-Secreted ESAT-6 and Its Role in Mycobacterium Marinum Escape From the Vacuole. Infection Immun (2008) 76:5478–87. doi: 10.1128/IAI.00614-08
39. Conrad WH, Osman MM, Shanahan JK, Chu F, Takaki KK, Cameron J, et al. Mycobacterial ESX-1 Secretion System Mediates Host Cell Lysis Through Bacterium Contact-Dependent Gross Membrane Disruptions. Proc Natl Acad Sci USA (2017) 114:1371–6. doi: 10.1073/pnas.1620133114
40. Linell F, Norden A. Mycobacterium Balnei. A New Acid-Fast Bacillus Occurring in Swimming Pools and Capable of Producing Skin Lesions in Humans. Acta Tuberculosea Scandinavica (1954) 33:1–84.
41. Gröschel MI, Sayes F, Shin SJ, Frigui W, Pawlik A, Orgeur M, et al. Recombinant BCG Expressing ESX-1 of Mycobacterium Marinum Combines Low Virulence With Cytosolic Immune Signaling and Improved TB Protection. Cell Rep (2017) 18:2752–65. doi: 10.1016/j.celrep.2017.02.057
42. Sato E, Imafuku S, Ishii K, Itoh R, Chou B, Soejima T, et al. Vitamin D-Dependent Cathelicidin Inhibits Mycobacterium Marinum Infection in Human Monocytic Cells. J Dermatol Sci (2013) 70:166–72. doi: 10.1016/j.jdermsci.2013.01.011
43. Coscolla M, Gagneux S. Consequences of Genomic Diversity in Mycobacterium Tuberculosis. Semin Immunol (2014) 26:431–44. doi: 10.1016/j.smim.2014.09.012
44. Gan H, Lee J, Ren F, Chen M, Kornfeld H, Remold HG. Mycobacterium Tuberculosis Blocks Annexin-1 Crosslinking and Thus Apoptotic Envelope Completion on Infected Cells to Maintain Virulence. Nat Immunol (2008) 9:1189–97. doi: 10.1038/ni.1654
45. Sohn H, Lee K-S, Kim S-Y, Shin D-M, Shin S-J, Jo E-K, et al. Induction of Cell Death in Human Macrophages by a Highly Virulent Korean Isolate of Mycobacterium Tuberculosis and the Virulent Strain H37Rv. Scandinavian J Immunol (2009) 69:43–50. doi: 10.1111/j.1365-3083.2008.02188.x
46. Mariotti S, Teloni R, Iona E, Fattorini L, Giannoni F, Romagnoli G, et al. Mycobacterium Tuberculosis Subverts the Differentiation of Human Monocytes Into Dendritic Cells. Eur J Immunol (2002) 32:3050–8. doi: 10.1002/1521-4141(200211)32:11<3050::AID-IMMU3050>3.0.CO;2-K
47. Madhvi A, Mishra H, Leisching G, Mahlobo P, Baker B. Comparison of Human Monocyte Derived Macrophages and THP1-Like Macrophages as In Vitro Models for M. Tuberculosis Infection. Comp Immunol Microbiol Infect Dis (2019) 67:101355. doi: 10.1016/j.cimid.2019.101355
48. Metsalu T, Vilo J. Clustvis: A Web Tool for Visualizing Clustering of Multivariate Data Using Principal Component Analysis and Heatmap. Nucleic Acids Res (2015) 43:W566–70. doi: 10.1093/nar/gkv468
49. Lex A, Gehlenborg N, Strobelt H, Vuillemot R, Pfister H. Upset: Visualization of Intersecting Sets. IEEE Trans Vis Comput Graph (2014) 20:1983–92. doi: 10.1109/TVCG.2014.2346248
50. Dweep H, Gretz N. Mirwalk2.0: A Comprehensive Atlas of MicroRNA-Target Interactions. Nat Methods (2015) 12:697. doi: 10.1038/nmeth.3485
51. Chen EY, Tan CM, Kou Y, Duan Q, Wang Z, Meirelles GV, et al. Enrichr: Interactive and Collaborative HTML5 Gene List Enrichment Analysis Tool. BMC Bioinf (2013) 14:128. doi: 10.1186/1471-2105-14-128
52. Braian C, Hogea V, Stendahl O. Mycobacterium Tuberculosis-Induced Neutrophil Extracellular Traps Activate Human Macrophages. J Innate Immun (2013) 5:591–602. doi: 10.1159/000348676
53. Johansen MD, Hortle E, Kasparian JA, Romero A, Novoa B, Figueras A, et al. Analysis of Mycobacterial Infection-Induced Changes to Host Lipid Metabolism in a Zebrafish Infection Model Reveals a Conserved Role for LDLR in Infection Susceptibility. Fish Shellfish Immunol (2018) 83:238–42. doi: 10.1016/j.fsi.2018.09.037
54. Xuan W, Qu Q, Zheng B, Xiong S, Fan G-H. The Chemotaxis of M1 and M2 Macrophages Is Regulated by Different Chemokines. J Leukocyte Biol (2015) 97:61–9. doi: 10.1189/jlb.1A0314-170R
55. Gonçalves AS, Appelberg R. The Involvement of the Chemokine Receptor CXCR2 in Neutrophil Recruitment in LPS-Induced Inflammation and in Mycobacterium Avium Infection. Scand J Immunol (2002) 55:585–91. doi: 10.1046/j.1365-3083.2002.01097.x
56. Schenk BI, Petersen F, Flad H-D, Brandt E. Platelet-Derived Chemokines CXC Chemokine Ligand (CXCL)7, Connective Tissue-Activating Peptide III, and CXCL4 Differentially Affect and Cross-Regulate Neutrophil Adhesion and Transendothelial Migration. J Immunol (2002) 169:2602–10. doi: 10.4049/jimmunol.169.5.2602
57. González-Cortés C, Diez-Tascón C, Guerra-Laso JM, González-Cocaño MC, Rivero-Lezcano OM. Non-Chemotactic Influence of CXCL7 on Human Phagocytes. Modulation of Antimicrobial Activity Against L. Pneumophila. Immunobiology (2012) 217:394–401. doi: 10.1016/j.imbio.2011.10.015
58. Boro M, Singh V, Balaji KN. Mycobacterium Tuberculosis-Triggered Hippo Pathway Orchestrates CXCL1/2 Expression to Modulate Host Immune Responses. Sci Rep (2016) 6:37695. doi: 10.1038/srep37695
59. Dorhoi A, Iannaccone M, Farinacci M, Faé KC, Schreiber J, Moura-Alves P, et al. MicroRNA-223 Controls Susceptibility to Tuberculosis by Regulating Lung Neutrophil Recruitment. J Clin Invest (2013) 123:4836–48. doi: 10.1172/JCI67604
60. Tokunaga R, Zhang W, Naseem M, Puccini A, Berger MD, Soni S, et al. CXCL9, CXCL10, CXCL11/CXCR3 Axis for Immune Activation - A Target for Novel Cancer Therapy. Cancer Treat Rev (2018) 63:40–7. doi: 10.1016/j.ctrv.2017.11.007
61. Saukkonen JJ, Bazydlo B, Thomas M, Strieter RM, Keane J, Kornfeld H. β-Chemokines are Induced by Mycobacterium Tuberculosis and Inhibit Its Growth. Infect Immun (2002) 70:1684–93. doi: 10.1128/IAI.70.4.1684-1693.2002
62. Sakala IG, Eickhoff CS, Blazevic A, Moheno P, Silver RF, Hoft DF. Dipterinyl Calcium Pentahydrate Inhibits Intracellular Mycobacterial Growth in Human Monocytes Via the C-C Chemokine MIP-1β and Nitric Oxide. Infect Immun (2013) 81:1974–83. doi: 10.1128/IAI.01393-12
63. Hasan Z, Cliff JM, Dockrell HM, Jamil B, Irfan M, Ashraf M, et al. CCL2 Responses to Mycobacterium Tuberculosis Are Associated With Disease Severity in Tuberculosis. PloS One (2009) 4(12):e8459. doi: 10.1371/journal.pone.0008459
64. Ben-Baruch A, Xu L, Young PR, Bengali K, Oppenheim JJ, Wang JM. Monocyte Chemotactic Protein-3 (MCP3) Interacts With Multiple Leukocyte Receptors. C-C CKR1, A Receptor for Macrophage Inflammatory Protein-1 Alpha/Rantes, Is Also a Functional Receptor for MCP3. J Biol Chem (1995) 270:22123–8. doi: 10.1074/jbc.270.38.22123
65. Ley K, Pramod AB, Croft M, Ravichandran KS, Ting JP. How Mouse Macrophages Sense What Is Going On. Front Immunol (2016) 7:204. doi: 10.3389/fimmu.2016.00204
66. Martínez Gómez JM, Koh VHQ, Yan B, Lin W, Ang MLT, Rahim SZZ, et al. Role of the CD137 Ligand (CD137L) Signaling Pathway During Mycobacterium Tuberculosis Infection. Immunobiology (2014) 219:78–86. doi: 10.1016/j.imbio.2013.08.009
67. Gordon S, Taylor PR. Monocyte and Macrophage Heterogeneity. Nat Rev Immunol (2005) 5:953–64. doi: 10.1038/nri1733
68. Slight SR, Khader SA. Chemokines Shape the Immune Responses to Tuberculosis. Cytokine Growth Factor Rev (2012) 24:105–13. doi: 10.1016/j.cytogfr.2012.10.002
69. Ottenhoff THM, Dass RH, Yang N, Zhang MM, Wong HEE, Sahiratmadja E, et al. Genome-Wide Expression Profiling Identifies Type 1 Interferon Response Pathways in Active Tuberculosis. PloS One (2012) 7:e45839. doi: 10.1371/journal.pone.0045839
70. Wu B, Huang C, Kato-Maeda M, Hopewell PC, Daley CL, Krensky AM, et al. IL-24 Modulates IFN-γ Expression in Patients With Tuberculosis. Immunol Lett (2008) 117:57–62. doi: 10.1016/j.imlet.2007.11.018
71. Fernández Do Porto DA, Jurado JO, Pasquinelli V, Alvarez IB, Aspera RH, Musella RM, et al. CD137 Differentially Regulates Innate and Adaptive Immunity Against Mycobacterium Tuberculosis. Immunol Cell Biol (2012) 90:449–56. doi: 10.1038/icb.2011.63
72. Walczak H. Death Receptor–Ligand Systems in Cancer, Cell Death, and Inflammation. Cold Spring Harb Perspect Biol (2013) 5(5):a008698. doi: 10.1101/cshperspect.a008698
73. Torraca V, Cui C, Boland R, Bebelman J-P, van der Sar AM, Smit MJ, et al. The CXCR3-CXCL11 Signaling Axis Mediates Macrophage Recruitment and Dissemination of Mycobacterial Infection. Dis Models Mech (2015) 8:253–69. doi: 10.1242/dmm.017756
74. Kipnis A, Basaraba R, Orme I, Cooper A. Role of Chemokine CCL2 in the Protective Response to Early Murine Pulmonary Tuberculosis. Immunology (2003) 109:547–51. doi: 10.1046/j.1365-2567.2003.01680.x
75. Kang DD, Lin Y, Moreno J-R, Randall TD, Khader SA. Profiling Early Lung Immune Responses in the Mouse Model of Tuberculosis. PloS One (2011) 6:e16161. doi: 10.1371/journal.pone.0016161
76. Boro M, Balaji KN. CXCL1 and CXCL2 Regulate NLRP3 Inflammasome Activation Via G-Protein-Coupled Receptor CXCR2. J Immunol (2017) 199:1660–71. doi: 10.4049/jimmunol.1700129
77. Bloom CI, Graham CM, Berry MPR, Rozakeas F, Redford PS, Wang Y, et al. Transcriptional Blood Signatures Distinguish Pulmonary Tuberculosis, Pulmonary Sarcoidosis, Pneumonias and Lung Cancers. PloS One (2013) 8:e70630. doi: 10.1371/journal.pone.0070630
78. Kaforou M, Wright VJ, Oni T, French N, Anderson ST, Bangani N, et al. Detection of Tuberculosis in HIV-Infected and -Uninfected African Adults Using Whole Blood RNA Expression Signatures: A Case-Control Study. PloS Med (2013) 10:e1001538. doi: 10.1371/journal.pmed.1001538
79. Gupta RK, Turner CT, Venturini C, Esmail H, Rangaka MX, Copas A, et al. Concise Whole Blood Transcriptional Signatures for Incipient Tuberculosis: A Systematic Review and Patient-Level Pooled Meta-Analysis. Lancet Respir Med (2020) 8:395–406. doi: 10.1016/S2213-2600(19)30282-6
80. Berry MPR, Graham CM, McNab FW, Xu Z, Bloch SAA, Oni T, et al. An Interferon-Inducible Neutrophil-Driven Blood Transcriptional Signature in Human Tuberculosis. Nature (2010) 466:973–7. doi: 10.1038/nature09247
81. Lavalett L, Rodriguez H, Ortega H, Sadee W, Schlesinger LS, Barrera LF. Alveolar Macrophages From Tuberculosis Patients Display An Altered Inflammatory Gene Expression Profile. Tuberculosis (2017) 107:156–67. doi: 10.1016/j.tube.2017.08.012
82. Bourigault M-L, Segueni N, Rose S, Court N, Vacher R, Vasseur V, et al. Relative Contribution of IL-1α, Il-1β and TNF to the Host Response to Mycobacterium Tuberculosis and Attenuated M. Bovis BCG. Immun Inflammation Dis (2013) 1:47–62. doi: 10.1002/iid3.9
83. Microrna-26b Modulates the NF-κb Pathway in Alveolar Macrophages by Regulating PTEN (Accessed 3 May 2021).
84. Boaru SG, Borkham-Kamphorst E, Van de Leur E, Lehnen E, Liedtke C, Weiskirchen R. NLRP3 Inflammasome Expression Is Driven by NF-κb in Cultured Hepatocytes. Biochem Biophys Res Commun (2015) 458:700–6. doi: 10.1016/j.bbrc.2015.02.029
85. Kinoshita T, Imamura R, Kushiyama H, Suda T. Nlrp3 Mediates Nf-κb Activation and Cytokine Induction in Microbially Induced and Sterile Inflammation. PloS One (2015) 10:e0119179. doi: 10.1371/journal.pone.0119179
86. Dheda K, Booth H, Huggett JF, Johnson MA, Zumla A, Rook GAW. Lung Remodeling in Pulmonary Tuberculosis. J Infect Dis (2005) 192:1201–10. doi: 10.1086/444545
87. Badr MT, Häcker G. Gene Expression Profiling Meta-Analysis Reveals Novel Gene Signatures and Pathways Shared Between Tuberculosis and Rheumatoid Arthritis. PloS One (2019) 14:e0213470. doi: 10.1371/journal.pone.0213470
88. Liang S, Liu Y, Wu Y, Ming S, Wu M. GITR Expressed on Macrophages Functions as an Inflammatory Amplifier by Promoting NLRP3 Inflammasome Activation. J Immunol (2020) 204:68.11–1.
89. Li M, Wang J, Fang Y, Gong S, Li M, Wu M, et al. Microrna-146a Promotes Mycobacterial Survival in Macrophages Through Suppressing Nitric Oxide Production. Sci Rep (2016) 6:23351. doi: 10.1038/srep23351
90. Mishra BB, Rathinam VAK, Martens GW, Martinot AJ, Kornfeld H, Fitzgerald KA, et al. Nitric Oxide Controls the Immunopathology of Tuberculosis by Inhibiting NLRP3 Inflammasome-Dependent Processing of IL-1β. Nat Immunol (2013) 14:52–60. doi: 10.1038/ni.2474
91. Mishra BB, Lovewell RR, Olive AJ, Zhang G, Wang W, Eugenin E, et al. Nitric Oxide Prevents a Pathogen-Permissive Granulocytic Inflammation During Tuberculosis. Nat Microbiol (2017) 2:1–11. doi: 10.1038/nmicrobiol.2017.72
92. Braverman J, Stanley SA. Nitric Oxide Modulates Macrophage Responses to Mycobacterium Tuberculosis Infection Through Activation of HIF-1α and Repression of NF-κb. J Immunol (2017) 199:1805–16. doi: 10.4049/jimmunol.1700515
93. Akram KM, Lomas NJ, Spiteri MA, Forsyth NR. Club Cells Inhibit Alveolar Epithelial Wound Repair Via TRAIL-Dependent Apoptosis. Eur Respir J (2013) 41:683–94. doi: 10.1183/09031936.00213411
94. Ni B, Rajaram MVS, Lafuse WP, Landes MB, Schlesinger LS. Mycobacterium Tuberculosis Decreases Human Macrophage IFN-γ Responsiveness Through Mir-132 and Mir-26a. J Immunol (2014) 193:4537–47. doi: 10.4049/jimmunol.1400124
95. Ahluwalia PK, Pandey RK, Sehajpal PK, Prajapati VK. Perturbed Microrna Expression by Mycobacterium Tuberculosis Promotes Macrophage Polarization Leading to Pro-Survival Foam Cell. Front Immunol (2017) 8:107. doi: 10.3389/fimmu.2017.00107
96. Kim Y-M, Brinkmann MM, Paquet M-E, Ploegh HL. UNC93B1 Delivers Nucleotide-Sensing Toll-Like Receptors to Endolysosomes. Nature (2008) 452:234–8. doi: 10.1038/nature06726
97. Yu X, Wang Y, Zhao W, Zhou H, Yang W, Guan X. Toll-Like Receptor 7 Promotes the Apoptosis of THP-1-Derived Macrophages Through the CHOP-Dependent Pathway. Int J Mol Med (2014) 34:886–93. doi: 10.3892/ijmm.2014.1833
98. Liang S, Song Z, Wu Y, Gao Y, Gao M, Liu F, et al. MicroRNA-27b Modulates Inflammatory Response and Apoptosis During Mycobacterium Tuberculosis Infection. J Immunol (2018) 200:3506–18. doi: 10.4049/jimmunol.1701448
99. Kwon J-E, Kim B-Y, Kwak S-Y, Bae I-H, Han Y-H. Ionizing Radiation-Inducible MicroRNA Mir-193a-3p Induces Apoptosis by Directly Targeting Mcl-1. Apoptosis (2013) 18:896–909. doi: 10.1007/s10495-013-0841-7
100. Chen S, Wang Y, Xu M, Zhang L, Su Y, Wang B, et al. Mir-1184 Regulates the Proliferation and Apoptosis of Colon Cancer Cells Via Targeting CSNK2A1. Mol Cell Probes (2020) 53:101625. doi: 10.1016/j.mcp.2020.101625
101. Keane J, Remold HG, Kornfeld H. Virulent Mycobacterium Tuberculosis Strains Evade Apoptosis of Infected Alveolar Macrophages. J Immunol (2000) 164:2016–20. doi: 10.4049/jimmunol.164.4.2016
102. Wolf AJ, Desvignes L, Linas B, Banaiee N, Tamura T, Takatsu K, et al. Initiation of the Adaptive Immune Response to Mycobacterium Tuberculosis Depends on Antigen Production in the Local Lymph Node, Not the Lungs. J Exp Med (2008) 205:105–15. doi: 10.1084/jem.20071367
103. Wu Y, Sun Q, Dai L. Immune Regulation of Mir-30 on the Mycobacterium Tuberculosis-Induced TLR/Myd88 Signaling Pathway in THP-1 Cells. Exp Ther Med (2017) 14:3299–303. doi: 10.3892/etm.2017.4872
104. Nance SC, Yi A-K, Re FC, Fitzpatrick EA. Myd88 is Necessary for Neutrophil Recruitment in Hypersensitivity Pneumonitis. J leukocyte Biol (2008) 83:1207. doi: 10.1189/jlb.0607391
105. Babcock AA, Toft-Hansen H, Owens T. Signaling Through Myd88 Regulates Leukocyte Recruitment After Brain Injury. J Immunol (2008) 181:6481–90. doi: 10.4049/jimmunol.181.9.6481
106. Loures FV, Pina A, Felonato M, Feriotti C, de Araújo EF, Calich VLG. Myd88 Signaling Is Required for Efficient Innate and Adaptive Immune Responses to Paracoccidioides Brasiliensis Infection. Infection Immun (2011) 79:2470. doi: 10.1128/IAI.00375-10
107. Liu H, Liu Z, Chen J, Chen L, He X, Zheng R, et al. Induction of CCL8/MCP-2 by Mycobacteria Through the Activation of TLR2/PI3K/Akt Signaling Pathway. PloS One (2013) 8(2):e56815. doi: 10.1371/journal.pone.0056815
108. Uguccioni M, D’Apuzzo M, Loetscher M, Dewald B, Baggiolini M. Actions of the Chemotactic Cytokines MCP-1, MCP-2, MCP-3, RANTES, MIP-1 Alpha and MIP-1 Beta on Human Monocytes. Eur J Immunol (1995) 25:64–8. doi: 10.1002/eji.1830250113
109. Asano K, Takahashi N, Ushiki M, Monya M, Aihara F, Kuboki E, et al. Intestinal CD169 + Macrophages Initiate Mucosal Inflammation by Secreting CCL8 That Recruits Inflammatory Monocytes. Nat Commun (2015) 6:7802. doi: 10.1038/ncomms8802
110. Khader SA, Rangel-Moreno J, Fountain JJ, Martino CA, Reiley WW, Pearl JE, et al. In a Murine Tuberculosis Model, the Absence of Homeostatic Chemokines Delay Granuloma Formation and Protective Immunity. J Immunol (2009) 183:8004–14. doi: 10.4049/jimmunol.0901937
Keywords: miRNA, Mycobacterium tuberculosis, Mycobacterium marinum, macrophage, host response, inflammatory response, virulence
Citation: Bade P, Simonetti F, Sans S, Laboudie P, Kissane K, Chappat N, Lagrange S, Apparailly F, Roubert C and Duroux-Richard I (2021) Integrative Analysis of Human Macrophage Inflammatory Response Related to Mycobacterium tuberculosis Virulence. Front. Immunol. 12:668060. doi: 10.3389/fimmu.2021.668060
Received: 15 February 2021; Accepted: 07 June 2021;
Published: 28 June 2021.
Edited by:
Sangeeta Tiwari¸ The University of Texas at El Paso, United StatesReviewed by:
Anthony George Tsolaki, Brunel University London, United KingdomVinod Kumar, Radboud University Nijmegen Medical Centre, Netherlands
Copyright © 2021 Bade, Simonetti, Sans, Laboudie, Kissane, Chappat, Lagrange, Apparailly, Roubert and Duroux-Richard. This is an open-access article distributed under the terms of the Creative Commons Attribution License (CC BY). The use, distribution or reproduction in other forums is permitted, provided the original author(s) and the copyright owner(s) are credited and that the original publication in this journal is cited, in accordance with accepted academic practice. No use, distribution or reproduction is permitted which does not comply with these terms.
*Correspondence: Isabelle Duroux-Richard, aXNhYmVsbGUucmljaGFyZEBpbnNlcm0uZnI=
†These authors have contributed equally to this work