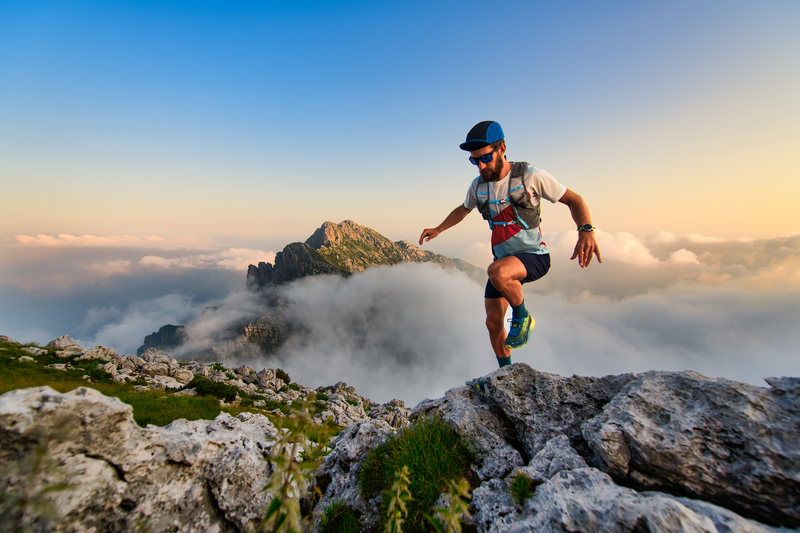
94% of researchers rate our articles as excellent or good
Learn more about the work of our research integrity team to safeguard the quality of each article we publish.
Find out more
ORIGINAL RESEARCH article
Front. Immunol. , 23 April 2021
Sec. Cancer Immunity and Immunotherapy
Volume 12 - 2021 | https://doi.org/10.3389/fimmu.2021.654660
Objective: Immune infiltration plays an important role in tumor development and progression and shows promising prognostic value in numerous tumors. In this study, we aimed to identify the role of immune infiltration in pancreatic neuroendocrine tumors (Pan-NETs) and to establish an Immunoscore system to improve the prediction of postsurgical recurrence-free survival.
Methods: To derive transcriptional signatures and deconvolute specific immune populations, two GEO datasets containing 158 Pan-NET patients were reanalyzed to summarize the immune infiltration landscape and identify immune-related signatures. Using real-time reverse transcription-polymerase chain reaction, immunofluorescence and immunochemistry methods, candidate signatures were further detected. The least absolute shrinkage and selection operator (LASSO) logistic regression model used statistically significant survival predicators in the training cohort (n=125) to build an Immunoscore system. The prognostic and predictive accuracy was validated in an external independent cohort of 77 patients.
Results: The immune infiltration profile in Pan-NETs showed significant heterogeneity, among which accumulated immune cells, T lymphocytes and macrophages were predominant. Fourteen statistically significant immune-related signatures were further identified in the screening cohort. The Immunoscore system for Pan-NETs (ISpnet) consisting of six immune features (CCL19, IL-16, CD163, IRF4, CD8PT and CD8IT) was constructed to classify patients as high and low risk in the training cohort (cutoff value = 2.14). Low-risk patients demonstrated longer 5-year recurrence-free survival (HR, 0.061; 95% CI, 0.026 to 0.14; p < 0.0001), with fewer recurrences and better prognoses. To predict the individual risk of recurrence, a nomogram incorporating both immune signatures and clinicopathological characteristics was developed.
Conclusion: Our model, ISpnet, captures immune feature-associated prognostic indicators in Pan-NETs and represents the first immune feature-based score for the postsurgical prognostic prediction. The nomogram based on the ISpnet and independent clinical risk factors might facilitate decision-making regarding early recurrence risk monitoring, identify high-risk patients in need of adjuvant therapy, and provide auxiliary guidance for patients with Pan-NETs that may benefit from immunotherapy in clinical trials.
Neuroendocrine tumors (NETs) represent heterogeneous malignancies originating from the secretory cells of the diffuse neuroendocrine system and are mainly characterized by indolent growth (1). Gastro-entero-pancreatic neuroendocrine tumors (GEP-NETs) account for most NETs. Specifically, the small intestine (30.8%), rectum (26.3%), colon (17.6%), pancreas (12.1%), and appendix (5.7%) are the most common primary sites in the digestive tract (2), and the incidence has steadily increased in the last 3 decades. Up to 90% of pancreatic neuroendocrine tumors (Pan-NETs) are hormonally silent, and nonfunctioning tumors seem to have a worse prognosis than functioning neoplasms probably because of a late diagnosis. Surgery represents the only curative approach and is recommended to remove all localized and limited metastatic disease. However, 12.3-42% of patients with Pan-NETs experience recurrence after curative resection, and the liver is the most common site (3–5). Currently, various prognostic prediction models have been established mainly based on clinicopathological characteristics, showing inconsistency, and novel molecular profiling markers have also been identified (6–11).
Extensive literature has investigated the host immune response against cancer and demonstrated the prognostic impact of immune infiltration in tumors. A methodology named the ‘Immunoscore’ has been defined to quantify in situ immune infiltration. In addition, current studies are consistent with the emerging concept that the neuroendocrine system can be regarded as a subsidiary extension of the innate immune system (12). Notably, the infiltration of immune cells appears to be higher in Pan-NETs than in midgut NETs possibly as a consequence of the higher mutational burden of Pan-NETs (13). A plethora of immune cells, including T cells (14, 15), natural killer (NK) cells (16), macrophages (10, 11, 17, 18), and mast cells (19, 20) have been reported to infiltrate Pan-NETs. Immunomodulatory factors were recently identified as master regulators of GEP-NET metastatic progression and may play a key role in promoting tumor immune escape (21).
In this study, we obtained two RNA-Seq datasets from Pan-NET patients and analyzed the immune characteristics to determine the immune profile of this heterogeneous tumor. Then, we quantified the expression of 10 immune markers (IL-16, IRF4, LRG1, MUC1, CXCL9, CCL19, CR2, PIR, CD79A and TCF21) via immunochemistry and CD4, CD8, CD163 distribution intratumorally and peritumorally based on immunofluorescence. The least absolute shrinkage and selection operator (LASSO) Cox regression model, a popular method for regression of high-dimensional predictors (22–24), was applied to establish a novel Immunoscore of Pan-NETs (ISpnet) for survival analysis of patients. Moreover, for clinical use, we constructed a nomogram-derived prognosis system that integrated the ISpnet index with clinicopathological risk factors for the early predictive identification of Pan-NET patients who might experience disease recurrence after surgery.
This study is intended to describe the immune infiltration profile of Pan-NETs. Therefore, two datasets (GSE98894 and GSE73338) from the Gene Expression Omnibus (GEO) were used. The gene expression profile data of primary tumors were used to quantify the infiltration of immune cells in tumor tissues by ssGSEA (single-sample gene set enrichment analysis). Differential gene expression analysis of high and low immune infiltration conditions was performed with the R package “DESeq2”. GSEA enrichment analysis and enrichment map analysis were performed using the ClusterProfiler package. The identification of 150 significant immune signatures was performed using GO:0002376 (immune system process, Gene Ontology Category), which contains 2,776 immune genes to screen candidate genes and verified by qPCR analysis in the Pan-NET patient cohort of our center with 60 patients. The details of the data processing are shown in the Supplementary Method.
The study workflow is shown in Figure 1. For the training cohort, we used a tissue microarray (TMA) of formalin-fixed, paraffin-embedded (FFPE) specimens from 125 consecutive patients who underwent surgery between March 2012 and May 2018 at the Department of Pancreatic Surgery, Fudan University Shanghai Cancer Center (FUSCC) and were pathologically diagnosed with Pan-NETs that were histologically confirmed, including patients diagnosed with Pan-NET G3 (well differentiated in histology, with Ki-67 over 20%). For each core (1.5 mm in diameter), at least two cores were used for every patient, including the tumor site and adjacent paratumor tissue with histologically normal pancreatic tissues. The validation cohort set comprised 77 patients with Pan-NETs who underwent pancreatectomy at an external hospital with pathology consultations performed at our institute. All clinical information was obtained from medical records, and patients were followed up regularly. Pathology records and site selection in TMA were reviewed and circled by the Department of Pathology at FUSCC. For the survival analysis, we used recurrence-free survival (RFS).
Based on the analysis of datasets, the expression of 150 genes was measured by quantitative PCR on sixty frozen Pan-NET tissue samples from patients who had undergone surgery between October 2018 and December 2019 in Shanghai Cancer Center as described in the Supplementary File. Furthermore, 10 candidate proteins for IHC staining were identified: IL-16, IRF4, LRG1, MUC1, CXCL9, CCL19, CR2, PIGR, CD79A and TCF21. IHC staining was performed and scored using TMA to determine the protein expression profiles. For calculation and evaluation, the expression levels were based on the score obtained by the intensity of the IHC staining. Briefly, a score was determined according to the intensity of the stain (negative=0, weak=1, intermediate=2, and strong=3). The distribution and numbers of tumor-infiltrating CD4+ and CD8+ T cells, as well as tumor-associated macrophages (TAMs) (CD163+), were determined by IF staining in the TMA and scored in five high-power fields (HPFs) (×400) of a maximal concentration of cells. CD4- and CD8-positive cells are green and red, respectively, according to the fluorescent labels used. The results were evaluated by 2 independent pathologists who were blinded to the clinical outcome. IHC and IF analysis using TMA were performed in the training and validation cohort. The primer sequences and antibodies used in qPCR, IHC and IF staining are listed in Supplementary Tables S1, S2.
Univariate analysis using Cox proportional hazards regression modeling was then used to test the significance of different immune markers in this original training group. The p-value for significant markers (< 0.05) remained for further validation within the training group. Specific features were preliminarily selected according to Cox regression in the Pan-NET training cohort of 125 samples from FUSCC, and LASSO regression was performed to confirm significant predictive features for predicting RFS using the R package glmnet. The optimal value of lambda (λ) was tuned via ten-fold cross-validation. A score was calculated for each sample via a linear combination of the selected features. To determine the optimal cutoff point for the ISpnet value, the survminer package was used, and the minimum group needed to account for at least 20% of the entire cohort. The potential association of the selected feature signature was assessed in the training cohort and then preliminarily validated in the validation cohort by using a calibration plot.
The ISpnet and patient-specific clinicopathological factors from 125 Pan-NET patients with survival information were subjected to subsequent analyses via univariate and multivariate Cox regression. For the multivariable Cox regression model, significant coefficients were used to construct nomograms. The calibration curves were made by plotting the observed rates against the predicted probabilities of the nomogram. A bootstrapping method was used to calculate the concordance index (C-index). The calibration consistency and predictive accuracy of the nomogram were indicated by the 3-, and 5-year RFS rates.
Data analysis was carried out using SPSS software version 20 (IBM Corporation, New York, USA), GraphPad Prism 8 (GraphPad Software, Inc., La Jolla, USA), R (version 3.6.0; R Foundation for Statistical Computing, Vienna, Austria; https://www.r-project.org/) and RStudio (Version 1.2.1335; RStudio, Inc., Boston, MA; https://www.rstudio.com/). Significance was determined using a one-tailed or two-tailed paired Student’s t test or the Mann-Whitney test as appropriate. The Kaplan-Meier method was used to estimate RFS, and comparisons between curves were performed using the log-rank test. The Cox proportional hazard regression model was used to estimate the hazard ratio (HR) with a 95% confidence interval (CI) for variables associated with RFS. Potential risk factors with a P value < 0.05 in the univariate Cox analysis were entered into a multivariate Cox regression model after considering collinearity among variables. The prognostic accuracy of the final model was estimated using time-dependent receiver operating characteristic (ROC) analysis. The area under the ROC curve (AUC) at different cutoff times was measured as prognostic accuracy. Calibration was assessed by visual examination of the calibration plot.
To demonstrate the immune profiles, we assessed the spectrum of immune cell infiltration in Pan-NETs, and the ssGSEA approach was utilized to deconvolve the relative abundance of each cell type based on expression profiling data retrieved from the GSE98894 datasets. Using unsupervised clustering, we categorized the cohort into two subgroups, high- and low-infiltration groups, as shown in Figure 2A. The immune cell profiles could be enriched from 24 immune cell types between these two populations with significant differences. The results of GSE98894 datasets were compared and showed that T lymphocytes, cytotoxic cells and macrophages had statistically significant differences (Figure 2B, Supplementary Table S3). Then, we conducted GSEA and confirmed that most of the biological processes of enriched immune signals were in the process of activation or response (Supplementary Figure S1). In Figure 2C, the enrichment map identified that T cell activation was the hub functional module, which was consistent with the enrichment of T lymphocytes in the high infiltration group.
Figure 2 Immune profiles and candidate immune signatures in Pan-NETs revealed by two GSE datasets (A) Heatmap for immune cell infiltration from the GSE98894 dataset. (B) Enriched T lymphocytes, cytotoxic cells and macrophages showed statistically significant differences between the high- (including high- and moderate-infiltration) and low-infiltration populations. (C) GSEA confirmed most of the biological processes of enriched immune signals. (D) The correlation of immune signature genes and immune cell types is shown. Red boxes indicate a positive correlation; blue boxes indicate a negative correlation. (E) Heatmap for clustering of the GSE73338 dataset with 150 immune signatures resulting in two subgroups with similar immune cell infiltration differences. (F) Enriched immune cell types were significantly different in the GSE73338 population. (*indicates 0.05, **indicates 0.01, ***indicates 0.001).
A total of 150 candidate immune signature genes were selected that met the following criteria: highly correlated with T cell infiltration, ranked according to fold change and an adjusted P value < 0.05 (Figure 2D, Supplementary Table S4). Unsupervised clustering of the GSE73338 dataset with 150 candidate genes also resulted in two subgroups with similar immune cell infiltration differences, as shown in Figure 2E. The results of GSE73338 datasets also showed that T lymphocytes, cytotoxic cells, and macrophages had statistically significant differences (Figure 2F). To further verify the reliability of the expression of 150 immune-related genes, we determined a group of Pan-NET specimens (n=60) with different T cell infiltration profiles and divided them into high and low groups (n=30) for PCR analysis of 150 genes. We found that IL-16, IRF4, LRG1, MUC1 and CXCL9 had the most differential expression changes (Supplementary Table S5, Figure 3A).
Figure 3 Quantitative Immunoscore establishment and validation in patients with Pan-NETs. (A) Ten immune signatures with statistically significant expression differences selected by qPCR methods in 60 Pan-NET patients for further analysis. (B) Feature selection using the LASSO regression model. Coefficient profile of the immune-related signatures associated with RFS of patients with Pan-NETs. (C) Time-dependent ROC curve describing the prognostic accuracy of the ISpnet and single immune features in the training cohort. (D) Kaplan-Meier curves for recurrence-free survival between the immune signature-defined high-risk and low-risk groups in the training and validation cohorts. (E) Scatter diagram illustrating the ISpnet of the training and validation cohorts. (F) Survival probability of Pan-NET patients with different AJCC/UICC staging system staging in low- and high-ISpnet patients. Ns, No significant.
The baseline characteristics of the resected patients with Pan-NETs in the training cohort (n=125) and validation cohort (n=77) are summarized in Table 1. The average age of the patients at the time of surgery was 51.8 years (range: 25-77); 92 (45.5%) patients were male. The majority (185, 91.6%) of Pan-NETs were nonfunctional. Eighty-three (41.1%) tumors were WHO grade 1, and the remaining 119 (58.9%) were grade 2 and grade 3. The median tumor size was 31 mm (range: 6 to 140 mm). Lymph node (LN) metastasis occurred in approximately one-third of patients. Twenty-eight patients (13.9%) had synchronous liver metastasis at the time of diagnosis and underwent simultaneous liver resection (25 of 28) or intraoperative curative radiofrequency ablation therapy (3 of 21). The median follow-up time was 41 months (IQR 27.00-59.75). Recurrence occurred in 26 (20.8%) patients, including 20 (16%) who developed liver metastasis and 6 (4.8%) with locoregional recurrence.
To identify the predictive value of immune-related signatures for prognosis, we then selected 10 candidate proteins with statistically significant differences in our FUSCC-PanNET cohort for IHC analysis: CCL19, CXCL9, IL-16, IRF4, MUC1, LRG1, PIGR, CD79A, TCF21, and CR2. Based on our analysis results and previous research (11), TAMs and CD4/8-positive T cells, which are regarded as significant indicators, were also reviewed. Based on the numeration of lymphocyte populations in both the tumor core (IT, intratumoral region) and peritumoral (PT) regions, the mean number of positive cells per HPF indicating the prevalence of immune infiltrates was calculated. Intratumoral TAMs were rarely detected in the Pan-NETs tested. As a result, the total number of TAMs (PT and IT) was used. The median number of peritumoral CD4+ T cells/HPF (13.8, range: 0.8-68.8) was significantly higher than that of intratumoral CD4+ T cells/HPF (4.8, range: 0-50.7; P< 0.0001). The median numbers of peri- and intratumoral CD8+ cells/HPF were 7.5 (range: 0.8-72.0) and 6.6 (range: 0.2-44.0), respectively, while there were no differences in CD8+ T cell infiltration distribution (P > 0.05). The median number of CD163+ cells/HPF was 6.6 (range: 0.1-12.0).
In the Cox regression analysis, the cohort was divided according to the number of CD4-, CD8- and CD163-positive cells: high versus medium and low group. For the 10 IHC immune markers, we divided negative and weak staining into the low expression group and intermediate and strong staining into the high expression group. The above nine significant immune markers (CXCL9, CCL19, MUC1, IL-16, IRF4, CD163, CD8IT, CD8PT, and CD4PT) were adopted for further verification using the training cohort of 125 patients according the Cox regression analysis (Table 2). The nine selected immune markers showed distinguishable and clear staining as shown in online Supplementary Figure S2.
The least absolute shrinkage and selection operator (LASSO) logistic regression model was used to establish the Immunoscore system, which involved six markers (percentage of CCL19, IL-16, CD163, IRF4, CD8IT, and CD8PT) identified by the training set (n=125) (Figure 3B, Supplementary Figure S3A). The immune signature of each patient was calculated based on their regression coefficients of the expression levels: ISpnet = (0.261 × the status of CCL19) + (0.490 × the status of IL-16) + (0.123 × the status of CD163) + (0.044 × the status of CD8PT) – (0.011× the status of CD8IT) – (0.493× the status of IRF4). The status of proteins, including CCL19, IL-16 and IRF4, is the IHC staining score. The status of CD8PT, CD8IT, and CD163 indicates the mean number of positive cells in five high-power fields (×400) of a maximal concentration of cells. In the training cohort, the patients were separated into low-risk and high-risk groups using an optimal cutoff value (ISpnet=2.14) generated by Survminer. The low-risk and high-risk groups comprised 77.6% (97/125) and 22.4% (28/125) of the patients, respectively. The 5-year RFS was 16.7% (95% CI, 5.29% to 52.6%) in the high-risk group and 91.7% (95% CI, 95.9% to 98.1%) in the low-risk group (HR, 0.061; 95% CI, 85,8% to 98.1% 0.026 to 0.14; p<0.0001). The ROC curve described the 5-year prognostic accuracy of the ISpnet and single immune features in the training cohort (Figure 3C, Supplementary Figure S3B).
Given the better accessibility and similar prognostic value of the immune signature ISpnet, it was selected to predict the prognosis of patients with Pan-NETs in the validation cohort. A scatter diagram illustrating the immune signature of the training and validation cohorts is shown in Figure 3D, with no significant difference in immune signature distribution observed between the two groups (p=0.998). In the validation cohort, ISpnet categorized 62 (80.5%) of the 77 patients into the low-risk group and 15 patients (19.5%) into the high-risk group. Patients in the high-risk group demonstrated far shorter RFS (HR, 0.15; 95% CI, 0.06 to 0.14; p<0.0001; Figure 3E) than patients in the low-risk group. We also performed stratified analyses of Pan-NET patients with stage I-II and stage III-IV disease in the whole cohort according to the 8th edition AJCC/UICC TNM staging system. Low-ISpnet patients had longer RFS than high-ISpnet patients (P< 0.0001, Figure 3F).
Univariate and multivariate Cox regression analyses were conducted to explore whether the prognostic value of the ISpnet was independent of conventional clinicopathological characteristics in our cohort with Pan-NETs (Table 3). After multivariable adjustment by clinicopathological risk factors, the results confirmed the robustness of ISpnet for independently predicting RFS in the training cohort (HR, 0.091; 95% CI, 0.035 to 0.23; p < 0.0001) and in the validation cohort (HR, 0.13; 95% CI, 0.04 to 0.38; p < 0.001). In addition, WHO grade (HR, 9.220; 95% CI, 1.120 to 75.908; p = 0.039), and liver metastasis (HR, 3.879; 95% CI, 1.581 to 9.519; p = 0.003) also remained significant for RFS after adjustment for various cofactors.
Table 3 Univariate and multivariate Cox regression analysis of prognostic factors in the training and validation cohorts.
Nomograms, with the ability to generate an individual probability of a clinical event by integrating diverse prognostic and determinant variables, are widely used as prognostic devices in oncology and medicine. Thus, a nomogram to predict the 3- and 5-year RFS was made that incorporated the ISpnet, synchronous liver metastasis, and grade (Figure 4A). In this nomogram, the recurrence score predicts the probability of developing recurrence after resection in patients with Pan-NETs. Among them, ISpnet had the highest C-index (0.796; 95% CI, 0.714 to 0.878; C-index, 0.714; 95% CI, 0.607 to 0.821, respectively) in both the training and validation cohorts. Liver metastasis and grade contributed to the most risk points after ISpnet. The calibration plots for the nomogram indicated good agreement in the training cohort (C-index, 0.917; 95% CI 0.884 to 0.950) and the validation cohort (C-index, 0.864; 95% CI, 0.798 to 0.930) (Figures 4B, C).
Figure 4 Nomogram for predicting the RFS of Pan-NET patients. (A) Nomogram scoring system based on the independent predictors from the multivariable Cox regression analysis to predict the risk of RFS in Pan-NETs. (B, C) Calibration plots of the nomogram to predict RFS at 5 years in the training cohort and the validation cohort, indicating good agreement between the prediction and the observation.
We also compared the predictive accuracy of this nomogram with individual predictors in the validation cohort; the nomogram performance (C-index, 0.864) was better than that of the ISpnet (C-index, 0.714), liver metastasis (C-index, 0.673), and grade (C-index, 0.676) (Supplementary Table S6). In summary, these findings suggest that the nomogram is a better model for predicting RFS in patients with Pan-NETs.
Since the early 1900s, immune infiltration of cancers has been believed to be a positive factor for patient outcome, and immunotherapy has recently modified cancer treatment (25, 26). Efforts are currently underway to explore the efficacy of immune checkpoint inhibitors in patients with Pan-NETs (27, 28). However, these insights have not had a major influence on cancer classification or clinical decision-making, and the immune cell landscape in patients with Pan-NETs has not yet been explored or summarized thoroughly.
Here we utilized GSE98894 and GSE73338 combined with the immune cell list provided by Bindea et al. (29) to calculate the Immunoscore and divided 158 patients into high- and low-infiltration types, which demonstrated that Pan-NETs manifested with diverse immune infiltration, underlying the importance of immunologic biomarkers in predicting prognosis and the response to therapy. Through biological analysis, we found that T lymphocyte-oriented immune cells exhibit highly infiltrating characteristics in patients with Pan-NETs, yielding the same results proposed by Cai et al. (11). Low peritumoral CD4+ cell infiltration, high intratumoral CD8+ T cell infiltration, and low peritumoral CD8+ T cell infiltration were significantly associated with RFS in Pan-NET patients, partly in accordance with the conclusions reached by other researchers (11). It is well known that T lymphocytes are at the center of inducing an effective adaptive immune response and maintaining homeostasis and that the degree of T cell infiltration of tumors has been considered both a general prognostic factor and a specific predictor of the response to checkpoint inhibition (13). Inspired by this knowledge, our study further explored and revealed novel immune signatures in patients with Pan-NETs, including IL-16, CXCL9, CCL19, IRF4, and MUC1 expression profiles, which strengthen the evidence regarding the accumulation of tumor-induced cytokines, chemokines, immune-related regulatory factors, and glycoprotein antigens in Pan-NET tissues (30, 31).
International validation results support the implementation of a consensus Immunoscore as a new component of a TNM-Immune classification of cancer (32, 33). The Immunoscore could have several potential clinical applications, such as prognostic and theranostic applications (22, 34). In carcinoid tumors, especially in lung NETs, the Immunoscore may act as a further prognostic indicator (35). To our knowledge, there is no Immunoscore system for Pan-NETs reported in the literature, and we are the first to introduce this system. In this study, we constructed an immune classifier named the ISpnet comprising 6 immune-related signatures, CD8PT, CD8IT, CCL19, IL-16, IRF4 and CD163, as a prognostic tool independent of the AJCC/UICC staging or other clinicopathological factors to predict survival in Pan-NET patients with surgical resection. Specifically, CD8+ cytotoxic T lymphocytes are preferred immune cells for targeting cancer and are needed in a process called tumor immunity cycle for making durable and efficient antitumor immune responses (36). The cytokine CCL19 plays an important role in the active recruitment, trafficking and migration of T lymphocytes. IL-16 is associated with macrophages and Th cells (37). IRF4 plays a key role in the expression of T cell genes and the development of the T cell immune response (38). CD163 is a marker of tumor-associated macrophages with an M2 phenotype. Specific depletion of CD163+ macrophages results in massive infiltration of activated T cells and tumor regression (39). Pan-NET patients with a low ISpnet demonstrated fewer recurrences and better prognoses than patients with a high ISpnet, and most genes related to liver metastasis exhibited high expression in the high-infiltration population. Thus, intriguingly, progressive activation of the immune system during Pan-NET progression and mutation accumulation was envisaged.
The AJCC/UICC staging classification is crucial in assessing prognosis and establishing a treatment strategy in patients with Pan-NETs but provides limited prognostic information and does not predict the response to therapy. The established ISpnet classifier could indicate the bioimmunological characteristics of the Pan-NET population and contains significant signatures mainly related to T lymphocyte features. This classifier was constructed using LASSO Cox regression models and complements clinicopathological factors that could greatly improve its predictive accuracy. Currently, incorporating the Immunoscore as a prognostic factor and introducing immune parameters into cancer classification and as an integral part of management to guide therapeutic decisions is indicated (32, 35, 40). Thus, a nomogram that integrates the ISpnet with important risk factors indeed provides more comprehensive information than AJCC/UICC staging. More meaningfully, the multi-immune feature-based classifier may help optimize the adjuvant therapy protocol in patients with Pan-NETs. Beyond the results obtained in localized tumors, the relevance of the Immunoscore could extend to metastatic disease because the Immunoscore identifies tumors that are likely to metastasize and predicts the prognosis of patients with metastases from primary Pan-NETs.
A recent study demonstrated the immune microenvironment of Pan-NETs and identified the metastasis-like primary (MLP)-1 subtype as an immune-high phenotype featuring broad and robust activation of immune-related genes. The MLP-1 subtype was first identified by mRNA and miRNA transcriptome profiles and signature genes of PanNET tumors, which were characterized by poorly differentiated tumors associated with liver metastases, high proliferative activity and aggressive behavior (41). The existence of a human MLP-like Pan-NET cluster has also been confirmed by a whole-genome study (42). Based on multiple transcriptome profiling, Young K et al. (43) demonstrated that this subtype contains high levels of lymphocytes and macrophages, which yields similar results proposed by recent literature and our analysis. Both of the studies focused on the immune landscape of Pan-NETs, and an analysis conducted by Young K et al. emphasized the MLP subtypes. We aim to establish a postsurgical prognostic prediction system based on the immune signatures derived from transcriptome datasets, and the Young K et al. tried to identify potential therapeutic vulnerabilities in this disease and pave the way for future precision immunotherapy studies. There maybe a link between our signatures and these subgroups, which need for further investigation in clinical cases.
Our study has some limitations. This was a retrospective study on data obtained from a single institution. There was also a lack of external validation with limited generalizability, as all specimens were obtained from patients at our center. The adequacy of TMA cores compared to whole slides for such an analysis may bring the inevitable discrepancy. Another limitation of our ISpnet is that it was based on T lymphocyte-related signatures and did not include more features, such as CCR7 and CD56. In addition, this is the first version of a possible score that could be adapted with specific antibodies, or could be simplified using less markers with more adapted antibodies, the validation of suitable antibodies on signatures revealed by our result on multicenter patient populations should be necessary before applied it into standard use consideration. The current study had insufficient adjuvant therapy data, which may influence the prognosis and result in limitations to its application. Thus, a prospective study will be needed to further validate our findings.
Collectively, we established the first Immunoscore for Pan-NETs, the ISpnet, which might be a useful predictive tool to identify patients with different prognoses who might benefit from immunotherapy, especially high-ISpnet patients, who may show a response to T cell checkpoint inhibition. Moreover, the nomogram encompassing the ISpnet and patient-specific clinicopathological characteristics could integrate valuable patient-specific information and effectively predict RFS in Pan-NET patients. Thus, a nomogram based on the ISpnet might facilitate decision-making regarding early recurrence risk monitoring, identify high-risk patients in need of adjuvant therapy, and provide auxiliary guidance for patients with Pan-NETs who may benefit from immunotherapy in clinical trials.
The original contributions presented in the study are included in the article/Supplementary Material. Further inquiries can be directed to the corresponding authors.
The studies involving human participants were reviewed and approved by the Ethics Committee of the Fudan University Shanghai Cancer Center. The patients/participants provided their written informed consent to participate in this study. The animal study was reviewed and approved by the Ethics Committee of the Fudan University Shanghai Cancer Center.
MW, SS and XY conceptualized and designed the study. JH, QM, CL, JL and BZ were in charge of data acquisition, MW, JX and WW performed analysis. XY and SS interpreted the data. MW and JX drafted the manuscript. XY and SS revised the manuscript. All authors contributed to the article and approved the submitted version.
This study was jointly funded by the National Natural Science Foundation of China (No. 81772555, 81802352 and 81902428), the National Science Foundation for Distinguished Young Scholars of China (No. 81625016), the Shanghai Sailing Program (No. 19YF1409400 and 20YF1409000), the Shanghai Rising-Star Program (No. 20QA1402100), the Shanghai Anticancer Association Young Eagle Program (No.SACA-CY19A06), the Clinical and Scientific Innovation Project of Shanghai Hospital Development Center (No. SHDC12018109 and SHDC12019109) and the Scientific Innovation Project of Shanghai Education Committee (No. 2019-01-07-00-07-E00057).
The authors declare that the research was conducted in the absence of any commercial or financial relationships that could be construed as a potential conflict of interest.
The Supplementary Material for this article can be found online at: https://www.frontiersin.org/articles/10.3389/fimmu.2021.654660/full#supplementary-material
1. Cives M, Strosberg JR. Gastroenteropancreatic Neuroendocrine Tumors. CA Cancer J Clin (2018) 68(6):471–87. doi: 10.3322/caac.21493
2. Frilling A, Akerstrom G, Falconi M, Pavel M, Ramos J, Kidd M, et al. Neuroendocrine Tumor Disease: An Evolving Landscape. Endocr Relat Cancer (2012) 19(5):R163–85. doi: 10.1530/ERC-12-0024
3. Marchegiani G, Landoni L, Andrianello S, Masini G, Cingarlini S, D’Onofrio M, et al. Patterns of Recurrence After Resection for Pancreatic Neuroendocrine Tumors: Who, When, and Where? Neuroendocrinology (2019) 108(3):161–71. doi: 10.1159/000495774
4. Genc CG, Falconi M, Partelli S, Muffatti F, van Eeden S, Doglioni C, et al. Recurrence of Pancreatic Neuroendocrine Tumors and Survival Predicted by Ki67. Ann Surg Oncol (2018) 25(8):2467–74. doi: 10.1245/s10434-018-6518-2
5. Boninsegna L, Panzuto F, Partelli S, Capelli P, Delle Fave G, Bettini R, et al. Malignant Pancreatic Neuroendocrine Tumour: Lymph Node Ratio and Ki67 are Predictors of Recurrence After Curative Resections. Eur J Cancer (2012) 48(11):1608–15. doi: 10.1016/j.ejca.2011.10.030
6. Landoni L, Marchegiani G, Pollini T, Cingarlini S, D’Onofrio M, Capelli P, et al. The Evolution of Surgical Strategies for Pancreatic Neuroendocrine Tumors (Pan-Nens): Time-trend and Outcome Analysis From 587 Consecutive Resections At a High-volume Institution. Ann Surg (2019) 269(4):725–32. doi: 10.1016/j.pan.2018.05.377
7. Genc CG, Jilesen AP, Partelli S, Falconi M, Muffatti F, van Kemenade FJ, et al. A New Scoring System to Predict Recurrent Disease in Grade 1 and 2 Nonfunctional Pancreatic Neuroendocrine Tumors. Ann Surg (2018) 267(6):1148–54. doi: 10.1097/SLA.0000000000002123
8. Kudo A, Akahoshi K, Ito S, Akashi T, Shimada S, Ogura T, et al. Downregulated Pancreatic Beta Cell Genes Indicate Poor Prognosis in Patients With Pancreatic Neuroendocrine Neoplasms. Ann Surg (2020) 271(4):732–9. doi: 10.1097/SLA.0000000000002911
9. Miki M, Oono T, Fujimori N, Takaoka T, Kawabe K, Miyasaka Y, et al. Clec3a, MMP7, and LCN2 as Novel Markers for Predicting Recurrence in Resected G1 and G2 Pancreatic Neuroendocrine Tumors. Cancer Med (2019) 8(8):3748–60. doi: 10.1002/cam4.2232
10. Wei IH, Harmon CM, Arcerito M, Cheng DF, Minter RM, Simeone DM. Tumor-Associated Macrophages are a Useful Biomarker to Predict Recurrence After Surgical Resection of Nonfunctional Pancreatic Neuroendocrine Tumors. Ann Surg (2014) 260(6):1088–94. doi: 10.1097/SLA.0000000000000262
11. Cai L, Michelakos T, Deshpande V, Arora KS, Yamada T, Ting DT, et al. Role of Tumor-Associated Macrophages in the Clinical Course of Pancreatic Neuroendocrine Tumors (Pannets). Clin Cancer Res (2019) 25(8):2644–55. doi: 10.1158/1078-0432.CCR-18-1401
12. Cives M, Pelle E, Quaresmini D, Rizzo FM, Tucci M, Silvestris F. The Tumor Microenvironment in Neuroendocrine Tumors: Biology and Therapeutic Implications. Neuroendocrinology (2019) 109(2):83–99. doi: 10.1159/000497355
13. da Silva A, Bowden M, Zhang S, Masugi Y, Thorner AR, Herbert ZT, et al. Characterization of the Neuroendocrine Tumor Immune Microenvironment. Pancreas (2018) 47(9):1123–9. doi: 10.1097/MPA.0000000000001150
14. Katz SC, Donkor C, Glasgow K, Pillarisetty VG, Gonen M, Espat NJ, et al. T Cell Infiltrate and Outcome Following Resection of Intermediate-Grade Primary Neuroendocrine Tumours and Liver Metastases. HPB (Oxford) (2010) 12(10):674–83. doi: 10.1111/j.1477-2574.2010.00231.x
15. de Reuver PR, Mehta S, Gill P, Andrici J, D’Urso L, Clarkson A, et al. Immunoregulatory Forkhead Box Protein P3-Positive Lymphocytes are Associated With Overall Survival in Patients With Pancreatic Neuroendocrine Tumors. J Am Coll Surg (2016) 222(3):281–7. doi: 10.1016/j.jamcollsurg.2015.12.008
16. Aparicio-Pages MN, Verspaget HW, Pena AS, Jansen JB, Lamers CB. Natural Killer Cell Activity in Patients With Neuroendocrine Tumours of the Gastrointestinal Tract; Relation With Circulating Gastrointestinal Hormones. Neuropeptides (1991) 20(1):1–7. doi: 10.1016/0143-4179(91)90033-F
17. Krug S, Abbassi R, Griesmann H, Sipos B, Wiese D, Rexin P, et al. Therapeutic Targeting of Tumor-Associated Macrophages in Pancreatic Neuroendocrine Tumors. Int J Cancer (2018) 143(7):1806–16. doi: 10.1002/ijc.31562
18. Pyonteck SM, Gadea BB, Wang HW, Gocheva V, Hunter KE, Tang LH, et al. Deficiency of the Macrophage Growth Factor CSF-1 Disrupts Pancreatic Neuroendocrine Tumor Development. Oncogene (2012) 31(11):1459–67. doi: 10.1038/onc.2011.337
19. Soucek L, Lawlor ER, Soto D, Shchors K, Swigart LB, Evan GI. Mast Cells are Required for Angiogenesis and Macroscopic Expansion of Myc-induced Pancreatic Islet Tumors. Nat Med (2007) 13(10):1211–8. doi: 10.1038/nm1649
20. Soucek L, Buggy JJ, Kortlever R, Adimoolam S, Monclus HA, Allende MT, et al. Modeling Pharmacological Inhibition of Mast Cell Degranulation as a Therapy for Insulinoma. Neoplasia (2011) 13(11):1093–100. doi: 10.1593/neo.11980
21. Alvarez MJ, Subramaniam PS, Tang LH, Grunn A, Aburi M, Rieckhof G, et al. A Precision Oncology Approach to the Pharmacological Targeting of Mechanistic Dependencies in Neuroendocrine Tumors. Nat Genet (2018) 50(7):979–89. doi: 10.1038/s41588-018-0138-4
22. Jiang Y, Zhang Q, Hu Y, Li T, Yu J, Zhao L, et al. ImmunoScore Signature: A Prognostic and Predictive Tool in Gastric Cancer. Ann Surg (2018) 267(3):504–13. doi: 10.1097/SLA.0000000000002116
23. Qu L, Wang ZL, Chen Q, Li YM, He HW, Hsieh JJ, et al. Prognostic Value of a Long non-Coding RNA Signature in Localized Clear Cell Renal Cell Carcinoma. Eur Urol (2018) 74(6):756–63. doi: 10.1016/j.eururo.2018.07.032
24. Lee BP, Vittinghoff E, Hsu C, Han H, Therapondos G, Fix OK, et al. Predicting Low Risk for Sustained Alcohol Use After Early Liver Transplant for Acute Alcoholic Hepatitis: The Sustained Alcohol Use Post-Liver Transplant Score. Hepatology (2019) 69(4):1477–87. doi: 10.1002/hep.30478
25. Myint ZW, Goel G. Role of Modern Immunotherapy in Gastrointestinal Malignancies: A Review of Current Clinical Progress. J Hematol Oncol (2017) 10(1):86. doi: 10.1186/s13045-017-0454-7
26. Marin-Acevedo JA, Dholaria B, Soyano AE, Knutson KL, Chumsri S, Lou Y. Next Generation of Immune Checkpoint Therapy in Cancer: New Developments and Challenges. J Hematol Oncol (2018) 11(1):39. doi: 10.1186/s13045-018-0582-8
27. Ferrata M, Schad A, Zimmer S, Musholt TJ, Bahr K, Kuenzel J, et al. Pd-L1 Expression and Immune Cell Infiltration in Gastroenteropancreatic (GEP) and Non-GEP Neuroendocrine Neoplasms With High Proliferative Activity. Front Oncol (2019) 9:343. doi: 10.3389/fonc.2019.00343
28. Bosch F, Bruwer K, Altendorf-Hofmann A, Auernhammer CJ, Spitzweg C, Westphalen CB, et al. Immune Checkpoint Markers in Gastroenteropancreatic Neuroendocrine Neoplasia. Endocr Relat Cancer (2019) 26(3):293–301. doi: 10.1530/ERC-18-0494
29. Bindea G, Mlecnik B, Tosolini M, Kirilovsky A, Waldner M, Obenauf AC, et al. Spatiotemporal Dynamics of Intratumoral Immune Cells Reveal the Immune Landscape in Human Cancer. Immunity (2013) 39(4):782–95. doi: 10.1016/j.immuni.2013.10.003
30. Pimenta EM, Barnes BJ. Role of Tertiary Lymphoid Structures (TLS) in Anti-Tumor Immunity: Potential Tumor-Induced Cytokines/Chemokines That Regulate Tls Formation in Epithelial-Derived Cancers. Cancers (Basel) (2014) 6(2):969–97. doi: 10.3390/cancers6020969
31. Colbeck EJ, Ager A, Gallimore A, Jones GW. Tertiary Lymphoid Structures in Cancer: Drivers of Antitumor Immunity, Immunosuppression, or Bystander Sentinels in Disease? Front Immunol (2017) 8:1830. doi: 10.3389/fimmu.2017.01830
32. Pages F, Mlecnik B, Marliot F, Bindea G, Ou FS, Bifulco C, et al. International Validation of the Consensus Immunoscore for the Classification of Colon Cancer: A Prognostic and Accuracy Study. Lancet (2018) 391(10135):2128–39. doi: 10.1016/S0140-6736(18)30789-X
33. Anitei MG, Zeitoun G, Mlecnik B, Marliot F, Haicheur N, Todosi AM, et al. Prognostic and Predictive Values of the Immunoscore in Patients With Rectal Cancer. Clin Cancer Res (2014) 20(7):1891–9. doi: 10.1158/1078-0432.CCR-13-2830
34. Hao D, Liu J, Chen M, Li J, Wang L, Li X, et al. Immunogenomic Analyses of Advanced Serous Ovarian Cancer Reveal Immune Score is a Strong Prognostic Factor and an Indicator of Chemosensitivity. Clin Cancer Res (2018) 24(15):3560–71. doi: 10.1158/1078-0432.CCR-17-3862
35. Roncati L, Manenti A, Piscioli F, Pusiol T, Barbolini G. The Immune Score as a Further Prognostic Indicator in Carcinoid Tumors. Chest (2017) 151(5):1186. doi: 10.1016/j.chest.2016.10.032
36. Farhood B, Najafi M, Mortezaee K. CD8(+) Cytotoxic T Lymphocytes in Cancer Immunotherapy: A Review. J Cell Physiol (2019) 234(6):8509–21. doi: 10.1002/jcp.27782
37. Richmond J, Tuzova M, Cruikshank W, Center D. Regulation of Cellular Processes by interleukin-16 in Homeostasis and Cancer. J Cell Physiol (2014) 229(2):139–47. doi: 10.1002/jcp.24441
38. Wu J, Zhang H, Shi X, Xiao X, Fan Y, Minze LJ, et al. Ablation of Transcription Factor IRF4 Promotes Transplant Acceptance by Driving Allogenic Cd4(+) T Cell Dysfunction. Immunity (2017) 47(6):1114–28. doi: 10.1016/j.immuni.2017.11.003
39. Etzerodt A, Tsalkitzi K, Maniecki M, Damsky W, Delfini M, Baudoin E, et al. Specific Targeting of CD163(+) Tams Mobilizes Inflammatory Monocytes and Promotes T Cell-Mediated Tumor Regression. J Exp Med (2019) 216(10):2394–411. doi: 10.1084/jem.20182124
40. Galon J, Mlecnik B, Bindea G, Angell HK, Berger A, Lagorce C, et al. Towards the Introduction of the ‘Immunoscore’ in the Classification of Malignant Tumours. J Pathol (2014) 232(2):199–209. doi: 10.1002/path.4287
41. Sadanandam A, Wullschleger S, Lyssiotis CA, Grotzinger C, Barbi S, Bersani S, et al. A Cross-Species Analysis in Pancreatic Neuroendocrine Tumors Reveals Molecular Subtypes With Distinctive Clinical, Metastatic, Developmental, and Metabolic Characteristics. Cancer Discovery (2015) 5(12):1296–313. doi: 10.1158/2159-8290.CD-15-0068
42. Scarpa A, Chang DK, Nones K, Corbo V, Patch AM, Bailey P, et al. Whole-Genome Landscape of Pancreatic Neuroendocrine Tumours. Nature (2017) 543(7643):65–71. doi: 10.1038/nature21063
Keywords: pancreatic neuroendocrine tumors, Immunoscore, recurrence-free survival, nomogram, prognosis
Citation: Wei M, Xu J, Hua J, Meng Q, Liang C, Liu J, Zhang B, Wang W, Yu X and Shi S (2021) From the Immune Profile to the Immunoscore: Signatures for Improving Postsurgical Prognostic Prediction of Pancreatic Neuroendocrine Tumors. Front. Immunol. 12:654660. doi: 10.3389/fimmu.2021.654660
Received: 17 January 2021; Accepted: 06 April 2021;
Published: 23 April 2021.
Edited by:
Franz Rodel, University Hospital Frankfurt, GermanyReviewed by:
Toshihiko Torigoe, Sapporo Medical University, JapanCopyright © 2021 Wei, Xu, Hua, Meng, Liang, Liu, Zhang, Wang, Yu and Shi. This is an open-access article distributed under the terms of the Creative Commons Attribution License (CC BY). The use, distribution or reproduction in other forums is permitted, provided the original author(s) and the copyright owner(s) are credited and that the original publication in this journal is cited, in accordance with accepted academic practice. No use, distribution or reproduction is permitted which does not comply with these terms.
*Correspondence: Xianjun Yu, eXV4aWFuanVuQGZ1ZGFucGNpLm9yZw==; Si Shi, c2hpc2lAZnVkYW5wY2kub3Jn
†These authors have contributed equally to this work
Disclaimer: All claims expressed in this article are solely those of the authors and do not necessarily represent those of their affiliated organizations, or those of the publisher, the editors and the reviewers. Any product that may be evaluated in this article or claim that may be made by its manufacturer is not guaranteed or endorsed by the publisher.
Research integrity at Frontiers
Learn more about the work of our research integrity team to safeguard the quality of each article we publish.