- 1Department of Microbiology and Immunology, University of British Columbia, Vancouver, BC, Canada
- 2Department of Medical Genetics, University of British Columbia, Vancouver, BC, Canada
- 3BC Children's Hospital Research Institute, Vancouver, BC, Canada
- 4Department of Pediatrics, University of British Columbia, Vancouver, BC, Canada
- 5BC Children's Hospital, Vancouver, BC, Canada
- 6Department of Statistics and Actuarial Science, Simon Fraser University, Burnaby, BC, Canada
- 7Department of Pediatric Rheumatology and Immunology, University Hospital Muenster, Muenster, Germany
- 8Department of Pediatrics, Alberta Children's Hospital, Calgary, AB, Canada
- 9Faculty of Pharmaceutical Sciences, University of British Columbia, Vancouver, BC, Canada
- 10Nuffield Department of Orthopedics, Rheumatology and Musculoskeletal Sciences, University of Oxford, Oxford, United Kingdom
- 11Centre for Microbial Diseases and Immunity Research, University of British Columbia, Vancouver, BC, Canada
- 12Centre for Blood Research, University of British Columbia, Vancouver, BC, Canada
Objectives: Chronic primary vasculitis describes a group of complex and rare diseases that are characterized by blood vessel inflammation. Classification of vasculitis subtypes is based predominantly on the size of the involved vessels and clinical phenotype. There is a recognized need to improve classification, especially for small-to-medium sized vessel vasculitides, that, ideally, is based on the underlying biology with a view to informing treatment.
Methods: We performed RNA-Seq on blood samples from children (n = 41) and from adults (n = 11) with small-to-medium sized vessel vasculitis, and used unsupervised hierarchical clustering of gene expression patterns in combination with clinical metadata to define disease subtypes.
Results: Differential gene expression at the time of diagnosis separated patients into two primary endotypes that differed in the expression of ~3,800 genes in children, and ~1,600 genes in adults. These endotypes were also present during disease flares, and both adult and pediatric endotypes could be discriminated based on the expression of just 20 differentially expressed genes. Endotypes were associated with distinct biological processes, namely neutrophil degranulation and T cell receptor signaling.
Conclusions: Phenotypically similar subsets of small-to-medium sized vessel vasculitis may have different mechanistic drivers involving innate vs. adaptive immune processes. Discovery of these differentiating immune features provides a mechanistic-based alternative for subclassification of vasculitis.
Introduction
Vasculitis (1) is a group of complex rare diseases that are characterized by inflammation in the blood vessel walls. The disease can present in childhood and in adulthood, and can be life- and/or organ-threatening. The primary framework for classifying vasculitis syndromes is according to the predominant size of the involved vessels (small, medium, large), the clinical phenotype (pattern of organs affected), and histopathology of involved vessels (2–5). Recently, distinctive etiological/pathological processes have been incorporated in the classification framework; for example, an association with anti-neutrophil cytoplasmic antibodies (ANCA) against intracellular granule proteins proteinase-3 (PR3) and myeloperoxidase (MPO) enables classification of small-to-medium sized, ANCA-associated vasculitis (AAV) (3, 4, 6, 7).
AAV encompasses three specific diseases: microscopic polyangiitis (MPA), granulomatosis with polyangiitis (GPA) and eosinophilic granulomatosis with polyangiitis (EGPA). An absence of specific classification criteria for MPA and the considerable phenotypic overlap with GPA, however, makes it challenging to distinguish GPA and MPA (6–8). In adult clinical trials, they are frequently analyzed collectively for convenience (9), despite important clinical and biological differences that argue for tailored treatment. Specifically, some studies show that GPA has a more refractory and relapsing disease course than does MPA (10, 11), although in more recent studies the association with relapse seems to be stronger with the presence of PR3-ANCA. ANCA specificity (for PR3 or MPO) has been suggested as an alternative to the clinical phenotype classification, or as a “biomarker” in updated classification criteria for AAV. However, PR3-ANCA, despite being predominantly associated with GPA, is also present in one-quarter of patients with MPA. Similarly, MPO-ANCA is differentially, but not exclusively, associated with MPA (7, 12), and a proportion of patients with AAV do not have ANCA to either PR3 or MPO. It is also noteworthy that GPA and MPA have overlapping clinical features with polyarteritis nodosa (PAN) (a medium-sized vessel vasculitis), and other small-to-medium sized vessel vasculitides that remain “unclassifiable” according to existing classification criteria.
Here, we considered if classification of small-to-medium sized vessel vasculitides could be improved by a deeper understanding of the molecular events underlying the disease and distinct disease subsets. To explore this hypothesis, and despite the disease being especially rare in children compared to adults, we focused on a cohort of children and adolescents with small-to-medium sized vessel vasculitis for mechanistic discovery. The study of pediatric patients can be advantageous (13, 14); children have limited confounding disease comorbidities and may have more predominant genetic factors that lead to early disease manifestations, compared to adults that have multiple environmental factors contributing to the onset of disease, which, for vasculitis, typically occurs after 50 years of age. Using RNA-Seq on blood obtained from pediatric patients with different clinically defined subtypes of small-to-medium sized vasculitis, we were able to cluster patients into two groups with distinct transcriptomic profiles and associated immune processes. Individuals with adult-onset disease could also be categorized in a similar manner, together suggesting that both adult- and pediatric-onset small-to-medium sized vessel vasculitides within the same disease “category” due to overlapping clinical features, might have different endotypes (15, 16).
Materials and Methods
Participants
Patients described in this study were enrolled in the Pediatric Vasculitis Initiative (PedVas) (17, 18) and included children (18 yrs of age and younger) and adults with small-to-medium sized blood vessel vasculitis. Two pediatric cohorts were used for this study: Cohort 1 contained a total of 30 patients that contributed samples at diagnosis (n = 25) or relapse (n = 5); at the time of sample and data collection, disease activity was high (indicated by PVAS; see clinical data description) and this cohort was used for initial transcriptomic discovery. Pediatric Cohort 2 consisted exclusively of patients (n = 11) at relapse and was used to validate gene expression patterns observed in Cohort 1. Major relapse was defined as a new or recurrent appearance of life- or organ-threatening disease activity that occurs more than 18 months post diagnosis and requires a change in treatment. Adult participants with chronic primary vasculiltis were first enrolled in DCVAS, the Diagnostic and Classification Criteria for Vasculitis study.
Clinical Data
Physicians collected data from pediatric participants (see Table 1 for Cohort 1 and Supplementary Table 1 for Cohort 2) (12, 17, 18) and entered it into A Registry of Childhood Vasculitis (ARChiVe), the RedCap data collection platform for PedVas. Generation of a pediatric vasculitis activity score (PVAS) (19) was a component of data entry; active and inactive disease was defined as a PVAS of > 2 and ≤ 2, respectively. The subtype of vasculitis was determined by a pediatric modified algorithm of the European Medicines Agency (EMA). ANCA status was reported by the participating site and validated in serum samples using a standard ELISA for anti-PR3 antibody (ORG518, Orgentec) and anti-MPO antibody (425-2380, BioRad). For adult patients, clinical data (Table 2) were collected through DCVAS. All DCVAS clinical data was reviewed independently by a panel of experts 6 months after the baseline assessment to provide a definitive, agreed diagnosis in accordance with the DCVAS protocol. For patients with GPA, MPA, and EGPA, there was ~25% rejection of the submitting physicians original diagnosis following the review.
RNA Sequencing and Analysis
Blood (2.5 ml) was collected from study participants in Tempus Blood RNA tubes (Applied BiosystemsTM, CA, USA) at the time of diagnosis or flare (flare; ≥18 months post diagnosis with a major change in the PVAS and sustained escalation of treatment) when disease activity was high (pediatric vasculitis activity score [PVAS] range 5–33). Extracted RNA (Tempus Spin RNA Isolation Kit, Thermo Fisher) underwent PolyA enrichment (NEBNEXT Poly(A) mRNA magnetic isolation kit, New England BioSciences), RNA-Seq library preparation (75bp or 100bp single end, KAPA Stranded total RNA kit, Roche) and was sequenced on an Illumina Genome Analyzer IIx or an Illumina HiSeq 2500. Fastq files were checked for quality (FastQC v0.11.8 and MultiQC v0.8) and aligned to the human genome (Ensembl GRCh38.93) using STAR v2.6 (20). HTSeq-count (HTSeq 0.61p1) was used to generate read count tables (21). Read counts from globin genes were removed bioinformatically and batch correction for sequencing date [shown to contribute to variance by the R package eigenR2 (22)] was performed using the ComBat function of the SVA package (23). Raw RNA-Seq counts were normalized for library size and heteroskedasticity by variance stabilizing transformation (vst). Differential gene expression analysis was performed using DESeq2 package v1.14.1 (24, 25). Pathway over-representation analysis was conducted in the ReactomePA v1.26.0 package for R (26) and network visualization was conducted using NetworkAnalyst (27). Differentially expressed genes identified by Grayson et al. (28) in nasal brushings from adults with GPA (compared to controls) were obtained and analyzed with ReactomePA. Entrez IDs and Ensembl IDs were then used to compare microarray probeset labels (28) to RNA-Seq data.
Statistics
Differential gene expression from DESeq2 analysis of RNA-Seq data was defined as a ≥ ± 1.5-fold change (FC) and ≤ 0.05 false discovery rate. Pathway enrichment analysis in ReactomePA used hypergeometric overlap and p-values were adjusted for multiple testing using false discovery rate. Significant enrichment was defined as a false discovery rate ≤ 0.05. Rotation gene set testing (ROAST) (29) and competitive gene set testing (CAMERA) (30) were used to determine whether the 20 gene signature, identified in pediatric patients at diagnosis, was also significantly different in pediatric patients in relapse and in adult patients. Clinical metadata (see Supplementary Table 2) were analyzed using the Description of Categories and Multiple Factor Analysis (MFA) functions from the FactoMineR package for R (31).
Data Availability
RNA-Seq data have been submitted to the Gene Expression Omnibus data sharing repository, and are accessible through GEO Series accession number: GSE129752.
Results
Whole Blood Gene Expression Patterns Delineated Distinct Endotypes of Pediatric Small-to-Medium Sized Vessel Vasculitis
To identify the underlying molecules and pathways associated with different pediatric small-to-medium vessel vasculitides, we sequenced the whole blood transcriptomes of the 30 children and adolescents in Cohort 1. Study samples were collected from the majority at first disease onset and included EMA-defined subtypes of GPA (n = 16), MPA (n = 4), PAN (n = 2), unclassified ANCA-associated vasculitis (unclassified AAV; n = 7), and unclassified (ANCA-negative) vasculitis (UCV; n = 1). Unsupervised hierarchical clustering of global gene expression (without consideration of EMA classification) placed the samples into two major and one minor cluster (Figure 1 and Table 1).
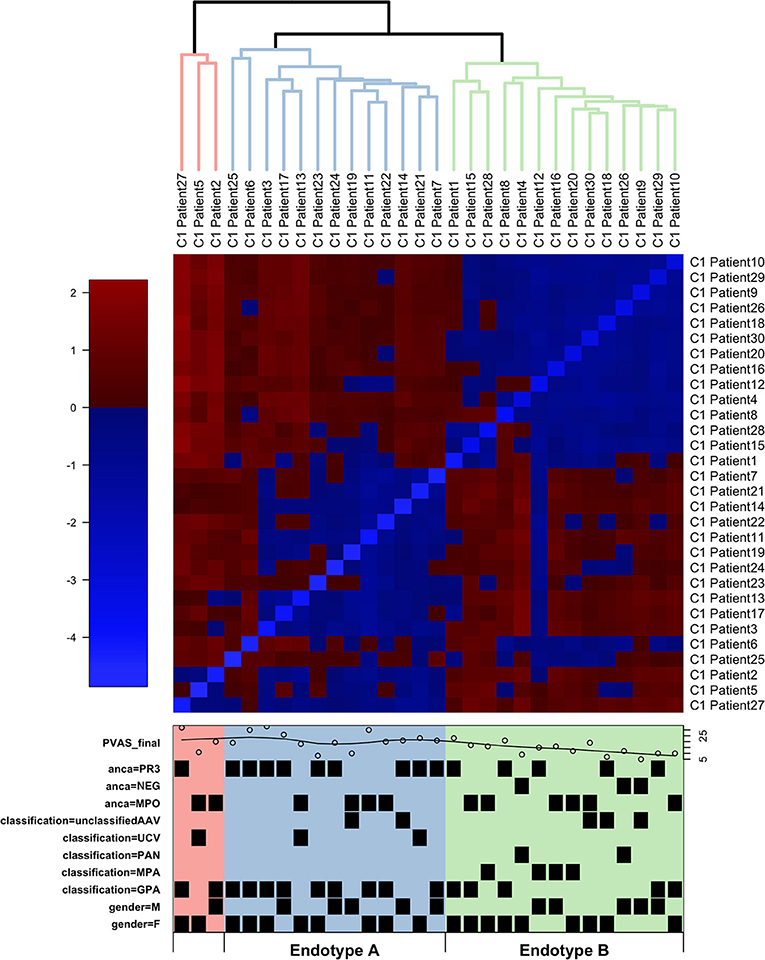
Figure 1. Hierarchical clustering of RNA-Seq data from children with small-to-medium sized vessel vasculitis. Hierarchical clustering (blue lines, Endotype A; green lines, Endotype B; red lines, “other”) and heatmap of normalized gene expression (variance stabilized counts) based on RNA sequencing of whole blood. Individual patient characteristics depicted in the lower box (also see Table 1): PVAS is the pediatric vasculitis activity score of disease activity at the time of sample collection; PR3 and MPO indicate positivity (or absence, NEG) for, respectively, anti-PR3 and anti-MPO antibodies; EMA classifications were uAAV, UCV, PAN, MPA, or GPA; M = male and F = female.
Distinct patterns of whole blood gene expression and a total of 3,809 genes were differentially expressed (± 1.5 FC, FDR ≤ 0.05) between the two major clusters. One major cluster (A) contained samples (n = 13) from 4 male and 9 female patients: 9 with GPA, and 4 with unclassified AAV (uAAV). PR3-ANCA were present in 9 of the 13 ANCA-positive individuals in this group. The other major cluster (B) contained samples (n = 14) from 5 male and 9 female patients: 5 with GPA, 4 with MPA, 2 with PAN, 2 with uAAV, and 1 with UCV. All patients with MPA and PAN were in this cluster and MPO-ANCA were present in 7 of the 13 ANCA-positive individuals in this group.
Patients in cluster A had greater overall disease activity (Supplementary Table 2: mean PVAS = 21, p-value = 0.013 compared to cluster B mean PVAS = 14, p-value = 0.006), and specifically, higher disease activity in the respiratory domain (mean chest PVAS = 4, p-value = 0.022). These children (cluster A) were also diagnosed at significantly older ages (mean age = 14, p-value = 0.015) and showed significantly greater neutrophil counts than patients in cluster B (mean neutrophil count in Endotype A = 10.4, p-value = 0.018) (Supplementary Table 2 and Supplementary Figure 1).
These clusters (A and B) were consistent with the predominant EMA subtype in each cluster, that is, GPA in A and MPA/PAN in B, although overlap was observed especially for patients with GPA (Figure 1 and Table 1). These data together with the distinct patterns of whole blood gene expression associated with each major cluster suggests that the four EMA-defined (phenotypically classified) subtypes of small-to-medium sized vessel vasculitis in our cohort may fall under two major endotypes, A and B. The final, minor cluster contained three samples; 2 from patients with GPA and one from a patient with uAAV, and they could conceivably represent a rarer endotype.
Neutrophil Degranulation and T Lymphocyte Activation Were Associated With Pediatric Vasculitis Endotype A and B, Respectively
Among the 3,809 differentially expressed (DE) genes between Endotype (cluster) A and Endotype (cluster) B, a total of 2,217 genes were expressed higher in Endotype A (relative to Endotype B) and 1,592 genes were expressed higher in Endotype B (relative to Endotype A). Genes with higher expression in Endotype A were involved in Toll-like receptor (TLR) signaling, interleukin signaling, Rho GTPase signaling, cellular senescence, and neutrophil degranulation (Supplementary Figure 2 and Supplementary Table 3A). Within the latter pathway, 178 of the 479 known neutrophil degranulation genes (~37%) were differentially expressed (FDR adjusted p-value for significance of association = 1.1 × 10−56). Functional interactions between the encoded proteins are shown in a protein:protein interaction network in Figure 2. Related, gene expression of biomolecules (including 16 histone genes, S100A8, S100A9, and PADI4) that are associated with neutrophil extracellular traps (NETs) (32) were higher (FDR <0.05) in Endotype A compared to Endotype B.
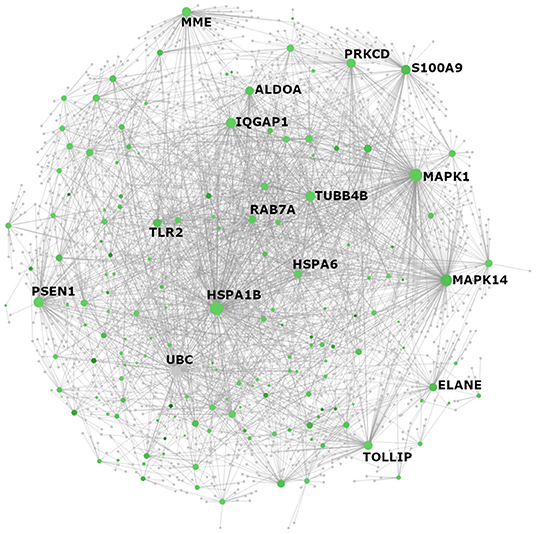
Figure 2. NetworkAnalyst visualization of Reactome pathway “neutrophil degranulation” genes with elevated expression in individuals from Endotype A. All genes with significantly higher expression in Endotype A that appeared in the Reactome PA category “neutrophil degranulation” (R-HSA-6798695) were visualized as a protein:protein interaction network using NetworkAnalyst. The color of each node reflects fold change between Endotypes A and B where green indicates higher expression in Endotype A. Gray nodes are genes that are not themselves differentially expressed (DE) but interact with other DE neutrophil degranulation genes. The size of each node (gene) reflects the number of documented interactions with other DE genes in the network. Thus, the largest circles have the greatest number of DE interacting partners and are predicted to be the most important regulators (“hub proteins”) of this network: inflammatory biomarker S100A8/A9, mitogen-activated protein kinases MAPK1 (ERK2) and MAPK14 (p38 MAPK), heat shock protein HSPA1B, the lysosome-associated glycoprotein LAMP2, tubulin TUBB4B, and Toll-like receptor protein TLR2 and its regulator/adaptor TOLLIP.
In contrast, genes with elevated expression in Endotype B were associated with T cell receptor (TCR) signaling, RNA processing, interferon (IFN) signaling and the differentiation and regulation of T cells and NK cells (Supplementary Figure 2 and Supplementary Table 3B). Dysregulated pathways in this cluster included: phosphorylation of CD3 and TCR zeta chains, PD-1 signaling, co-stimulation by the CD28 family, TCR signaling, and immunoregulatory interactions between lymphoid and non-lymphoid cells. Children in Endotype B had a moderate but significantly lower ratio of Th1:Th2 cells marker expression (TBX21:GATA3) (p-value = 0.038 by Wilcoxon test) compared to Endotype A (Figure 3 and Supplementary Figure 3), and a much overall higher ratio of expression of the Th2 marker GATA3 (p-value = 3.0 × 10−6). Individuals in Endotype B also had a higher expression ratio of the Treg marker FOXP3 (p-value = 0.014).
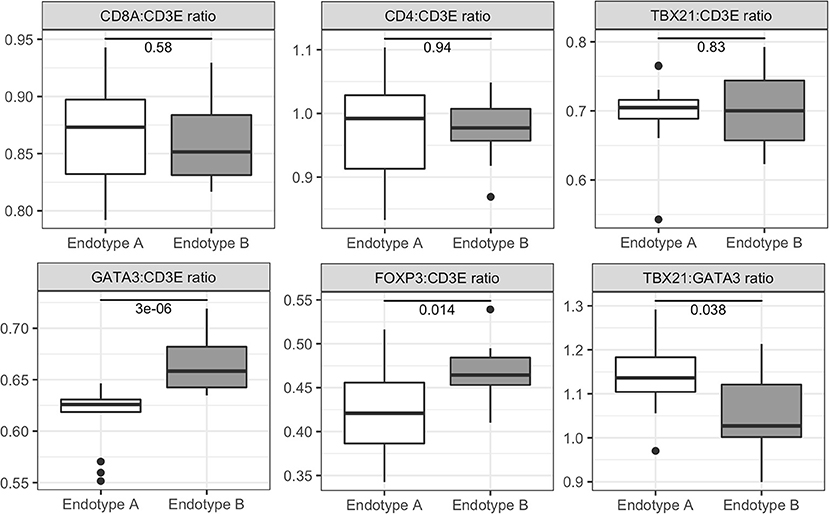
Figure 3. Expression ratios of T cell related genes for pediatric vasculitis Endotypes A and B. Relative abundance and ratios (y-axis) of the expression of genes for T cell markers in Endotype A and Endotype B patients (x-axis). T cell marker genes included CD3E (present in all T cells), CD8A (CD8+ cells), CD4 (CD4+ cells), TBX21 (Th1 cells), GATA3 (Th2 cells), FOXP3 (Tregs), and RORC (Th17 cells). Gene expression ratios were calculated from variance stabilized counts. Significance of the ratio between the clusters is reported within each boxplot and was determined by the Wilcoxon Rank Sum test. Additional data shown in Supplementary Figure 3.
Endotypes Present at Diagnosis Also Defined Inflammatory Mechanisms at Relapse
To determine if the endotypes (and immunologic pathways) identified in Cohort 1 were associated with subsequent relapses in disease post diagnosis, we performed RNASeq on samples collected from a smaller cohort of pediatric patients (Cohort 2, n = 11) during a major disease relapse. Using unsupervised hierarchical clustering of RNASeq data as was done with Cohort 1, patients in Cohort 2 also sorted into two major clusters, ‘Relapse cluster 1' and ‘Relapse cluster 2'. Two outlier samples did not fall into either cluster and did not cluster with each other (Supplementary Figure 4A).
Relapse cluster 1 contained 4 patients: 2 with GPA, 1 with uAAV, and 1 with UCV. ANCA status was unknown for 1 patient and the remaining 3 patients were all positive for PR3-ANCA. Relapse cluster 2 contained 5 patients: 3 with GPA and 2 with MPA. This cluster contained 4 ANCA-positive patients: 3 with MPO-ANCA and 1 patient with both MPO- and PR3-ANCA. We noted that all patients (4/4) in relapse cluster 1 were female, and suggest that this is a reflection of Cohort 2 being small, and predominantly female (7/11 patients); a separation of sexes between Endotypes A and B was not observed in Cohort 1, which was larger and balanced between male and female patients.
Using the more than 1,000 genes that were differentially expressed between relapse clusters 1 and 2, we performed Reactome analysis and compared pathway enrichment in the “relapse clusters”, (Supplementary Figure 4B and Supplementary Table 4) to those in the “diagnosis clusters” (i.e., Endotype A and Endotype B from Cohort 1). Our results revealed an overlap in enriched pathways between relapse cluster 1 and Endotype A, and between relapse cluster 2 and Endotype B, suggesting that the same driving mechanisms (behind the distinct Endotypes) are present at diagnosis and disease relapse.
Pediatric Derived Molecular Pathways and Endotypes Defined Adult-Onset Small-to-Medium Sized Vessel Vasculitis
Unsupervised hierarchical clustering of RNASeq data from a small subset of adult vasculitis patients (n = 11, Table 2) also sorted into two distinct endotypes (Adult Endotype A and Adult Endotype B; Supplementary Figure 5A). Adult Endotype A contained 5 patients: 2 with MPA, 2 with GPA, and 1 with complex vasculitis (labeled COMP/GBM). Of these, 2 were positive for MPO-ANCA and 3 for PR3-ANCA. Adult Endotype B also included 5 patients: 1 with GPA, 1 with eosinophilic GPA (EGPA), 2 with MPA, and 1 with leucocytoclastic cutaneous vasculitis (labeled OSV). Three adult Endotype B patients were ANCA negative, 1 was positive for MPO-ANCA, and 1 was positive for PR3-ANCA. A total of 1,682 genes were differentially expressed between Adult Endotypes A and B. Similar to the pediatric endotypes, Adult Endotype A was enriched for neutrophil degranulation pathways, IL-4 and IL-13 signaling, and antimicrobial peptides, while Adult Endotype B was enriched for T cell receptor signaling, IL-2 signaling, CD28 dependent PI3K/AKT signaling, and translocation of ZAP-70 to the immunological synapse (Supplementary Figure 5B).
Due to the small cohort size, we also compared our results to a transcriptomic study by Grayson et al. (28) that identified 339 genes significantly DE in nasal brushings from adult GPA patients compared to healthy controls. Of these DE genes, 141 were highly expressed in pediatric Endotype A (Fisher's Exact test: p-value = 2.2 × 10−16, odds ratio = 7.6), and 62 were also highly expressed in Adult Endotype A (Fisher's Exact test: p-value = 2.2 × 10−16, odds ratio = 5.2). An overlap in Reactome pathways enriched in the nasal brushing dataset and Endotypes identified from our dataset were also observed (Supplementary Figure 5C).
Together, these findings indicate potentially common mechanisms between pediatric and adult vasculitis endotypes and suggest that gene signatures and disease processes in affected tissues may be evident in blood.
Differential 20-Gene Signature Defines Pediatric and Adult Vasculitis Subtypes
The differential gene expression patterns in Endotypes A and B, consistent in both the pediatric and adult cohorts, suggested fundamentally different disease mechanisms associated with each endotype. We therefore asked whether a small set of DE genes could reliably separate individual samples into the respective Endotypes (A and B). A 20 gene signature was identified from pediatric Cohort 1 (see Figure 4 and Materials and Methods) that, using hierarchical clustering, placed all samples within the same pediatric Endotypes as the full RNA-Seq dataset (Figure 4A and Supplementary Figure 6).
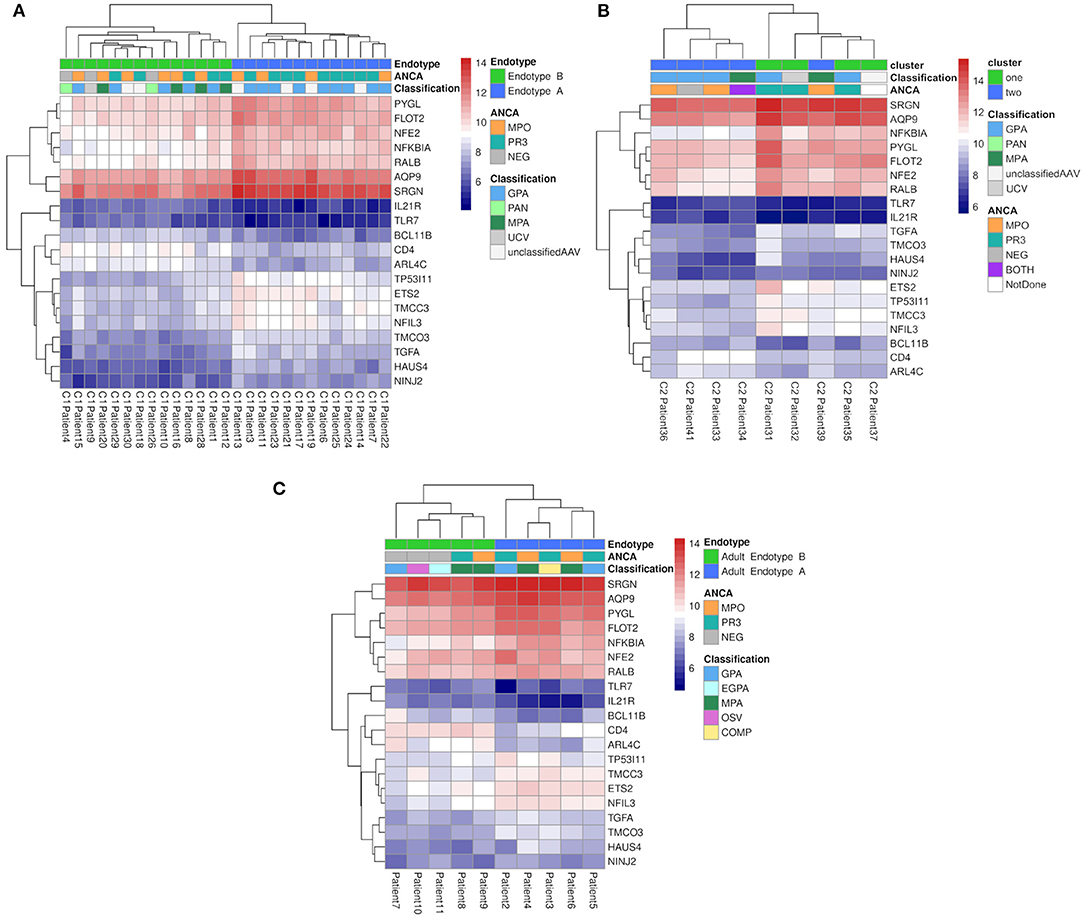
Figure 4. Hierarchical clustering of vasculitis patients based on expression data from 20 genes. Hierarchical clustering (hclust function of R) was performed using the average linkage method with Euclidean distances calculated from vst counts. The variance for all genes (as batch corrected vst counts) was calculated separately for major clusters. Genes with the lowest within-group variance, an absolute FC ≥ 2 between the clusters, the 50% least variable genes in both clusters, and an overall average count of at least 100 were selected and assessed for their ability to separate samples into the same clusters as the entire gene dataset. The heatmap represents expression (variance stabilized counts) of each of 20 genes (y-axis, right) that were (a) maximally divergent between Endotypes A and B (i.e.: significantly DE with a FDR adjusted p-value <0.05 and fold change >1.5) and (b) had low variance within each cluster to minimize the margin of error for testing individual samples. (A) Pediatric Cohort 1 patients (n = 27, x-axis) in Endotypes A (blue) and B (green). (B) Pediatric Cohort 2 patients (n = 9, x-axis) in relapse Endotypes A (blue) and B (green). (C) Adult patients (n = 10, x-axis) in Adult Endotypes A (blue) and B (green).
This 20 gene signature, when applied to pediatric Cohort 2 (relapse samples; Figure 4B) and the adult cohort (Figure 4C), also separated samples into the same Endotypes A and B as the entire RNA-Seq dataset with the exception of only 2 samples from the relapse cohort. The application of gene set significance testing, using both ROAST and CAMERA, showed that this 20 gene signature was also significantly differentially expressed between Endotypes A and B at relapse (ROAST p-value = 6.6 × 10−03, CAMERA p-value = 1.1 × 10−03). Similarly, the gene sets were also highly significantly different between endotypes (ROAST p-value = 4.10 × 10−03, CAMERA p-value = 7.72 × 10−04) in the adult cohort. Among the 20 gene signature identified in both pediatric and adult RNA-Seq data, 25% (5/20) of these genes were differentially expressed (28) in nasal brushings from adults with GPA.
Discussion
Despite step-wise improvements in classification criteria for small-to-medium sized vessel vasculitis, the ability to use current classification systems to accurately diagnose, prognosticate and tailor treatment remains limited due to overlapping clinical features, unclassifiable patients and variable disease trajectories/outcomes of patients classified as having the same disease. For pediatric patients, classification is even more challenging. Even relatively recent pediatric adaptations of the American College of Rheumatology (ACR) criteria (originally based on adult data) (3, 4) fail to uniquely classify 25% of pediatric patients (12).
In this study, we investigated a biological basis to differentiate small-to-medium sized vessel vasculitis in children. Our results demonstrate that the majority of patients fell into two primary groups with distinctive gene expression patterns and clinical phenotypes that were predominantly, but not exclusively, either GPA or MPA. The groups differed in the expression of approximately one-third of all expressed human genes and could be discriminated based on the differential expression of just 20 “biomarker” genes. These transcriptome-based signatures, which were elucidated from predominantly disease-onset (diagnosis) pediatric samples, were also found in a small cohort of pediatric patients experiencing a relapse in disease, and in a small cohort of adult patients at diagnosis of a variety of clinically-defined vasculitides affecting small-to-medium sized vessels.
The concept that mechanistic differences may enable separation of complex diseases into different “endotypes” has been demonstrated in other chronic inflammatory diseases, for example, asthma (15) and diabetes (16) that have a singular “disease category” yet intrinsic heterogeneity in symptoms and outcomes. In our cohort, the transcriptome-defined endotypes were not significantly associated with MPO/PR3 ANCA positivity or EMA classification for GPA, indicating differentiating biological factors between endotypes that are not apparent based on current clinical classification or ANCA status. Of interest, unbiased cluster analysis of clinical metadata, including data used in the EMA classification algorithm, organized patients in our cohort into two “new” clinically-defined groups (Supplementary Figure 7) that had substantial overlap with RNASeq data-defined endotypes. In one clinical cluster, 10/13 patients were associated with Endotype A (correlation analysis p-value = 0.0018), and in the other clinical cluster, 12/17 patients were associated with Endotype B (correlation analysis p-value = 0.0037). Although a much larger cohort is required for validation, the data suggest that these underlying biologic mechanisms (endotypes) might each associate with a unique clustering of patients according to clinical symptoms.
Endotype A was associated with pathways reflecting neutrophil degranulation while Endotype B demonstrated a gene expression pattern indicative of T cell activation. In AAV, both innate and adaptive immune processes are, as the name suggests, involved in disease pathogenesis (i.e., by the action of autoantibodies against neutrophil proteins). Our evidence however, suggests that these different arms of the immune system predominate in different subsets of patients, as opposed to operating in concert across all individuals regardless of subtype, and seemingly independent of ANCA specificity.
Neutrophil degranulation, a common feature of many inflammatory disorders, including severe asphyxia in asthma, acute lung injury, rheumatoid arthritis, and septic shock (32), was associated with Endotype A. The gene encoding a protein from the neutrophil degranulation pathway, glycogen phosphorylase L (PYGL), was one of the top 20 most significantly DE genes between the endotypes (p-value = 1.85 × 10−07, mean fold change of genes in pathway = 2.17). Consistent with the role of neutrophil degranulation in lung disease, Endotype A patients had higher component PVAS for respiratory involvement (chest score: Supplementary Table 2). In contrast, there was no significant association between either endotype and renal-specific PVAS despite a large majority of patients with GPA and MPA having renal involvement. Moreover, pulmonary disease, and specifically, the involvement of granulomatous inflammation, occurs more frequently (twice as often) in GPA compared to MPA. Neutrophil degranulation can lead to neutrophil extracellular traps (NETs) (32, 33) that play a role in the capture and killing of bacteria and have been described in adult AAV (34, 35). NETs contain a variety of biomolecules (36) including DNA, histone proteins, S100 proteins, MPO and PR3. Consistent with the role of NETs in bacterial infections, genes involved in the recognition and uptake of bacteria, including TLR- and NOD-like receptor signaling, and FcγR-mediated phagocytosis were enriched in Endotype A; notably FcγRIIA and the phagocytic pathway downstream of this receptor were significantly (p-value = 0.025) enriched in Endotype A.
Endotype B contained all MPA/PAN patients, which is noteworthy given that MPA and PAN, prior to the identification of ANCA, were considered manifestations of the same disease in different sized blood vessels. Patients within Endotype B differentially expressed many genes associated with T cell receptor (TCR) signaling and the differentiation and regulation of T cells and NK cells. T cell surface protein CD4 was one of the top 20 DE genes showing higher expression in Endotype B. The expression of genes in the MHC class II pathway responsible for CD4+ T helper cell activation were also elevated. The MHC class II pathway can be triggered either by endocytosed antigens or misfolded proteins (autoantigens) as is the case with certain types of arthritis (37, 38). These are interesting observations, since Th2 and Treg cells are involved in the prevention of autoimmune diseases (39), suggesting that the mechanism of pathogenesis in Endotype B might trigger immune regulatory responses to a greater extent. Given that specific Th2 populations can play a role in several inflammatory diseases [e.g., ulcerative colitis (40), chronic allergic inflammation (41) and eosinophilic gastrointestinal disease (42)], inflammation in patients associated with this Endotype could also be driven by a similar mechanism involving highly activated T cells, and an imbalance of Th2 cells that is pathogenic.
Our results also demonstrated that pediatric gene expression patterns involving neutrophil degranulation and T cell activation were present in blood samples from adults with vasculitis and showed significant overlap with findings from a published study of gene expression in nasal tissue from adults with GPA (28). The latter observation is consistent with the notion that inflammatory mechanisms in AAV are independent of the organ systems involved (43).
It is important to note that therapy was initiated in the majority of patients prior to sample collection and many treatments, notably corticosteroids, can influence gene expression. The nature of treatment, dose and route of administration varied considerably among patients and was consistent with our previous report of treatment variability in clinical practice (44). Despite this, it should be noted that no association was observed between endotype and receipt of corticosteroids by multi-factor analysis (31) (Supplementary Table 2). In contrast, we found a significant correlation between patients in Endotype B and the receipt of non-biologic immunosuppressive treatment. However, it would be difficult to conclude that these treatments are driving differences in gene expression between groups given that factors other than the nature of the disease, including cost, accessibility of drugs, and physician experience influence treatment choice (44). It is also equally likely that inflammatory mechanisms, as observed by differences in gene expression, respond to different pharmacological agents, leading to improved patient outcomes and preferred use by physicians.
In summary, we have classified pediatric and adult patients with small-to-medium sized vessel vasculitis (AAV, PAN and unclassifiable disease) into two distinct endotypes based on whole blood gene expression profiling. These data may argue for categorization of vasculitides based on biologic mechanism, however it remains to be proven if transcriptome-defined groups have clinical utility. Exploration of links between treatment outcomes particularly relapsing or refractory disease, and the pathogenic mechanisms identified by transcriptomics, will be the subject of future analyses of pediatric and adult vasculitis patients.
Data Availability Statement
The datasets presented in this study can be found in online repositories. The names of the repository/repositories and accession number(s) can be found in the article.
Ethics Statement
The study protocol was approved by the Children's and Women's Research Ethics Board of the University of British Columbia [H12-00894] and the respective ethical committees or IRBs at participating PedVas sites. Written informed consent and assent/consent, respectively, was obtained from parents and from pediatric patients. Adults with chronic primary vasculitis were first enrolled in DCVAS, the Diagnostic and Classification Criteria for Vasculitis study. The DCVAS study consent form explicitly included an invitation for patients to participate in other research studies, subject to the patients consenting to those other studies, as was the case with inclusion of any adult samples in the pediatric vasculitis study.
Author Contributions
EG and MS: bioinformatics analysis and manuscript preparation. KG and AL: data analysis and final revision of manuscript. RF: RNA sequencing. CR and JG: experimental design and final revision of manuscript. DF, SB, and KM: clinical care of patients, clinical data collection and entry, and final revision of the manuscript. PedVas Initiative Investigators: clinical care of patients and clinical data collection and entry. RL: clinical care of patients, clinical data collection and interpretation, and final revision of the manuscript. DC: study design, clinical care of patients, clinical data collection and interpretation, and final revision of the manuscript. RH: study design and bioinformatics data oversight and interpretation and manuscript preparation. KB: study design, sample collection, and clinical and bioinformatic data interpretation and manuscript preparation. All authors read and approved the final manuscript.
Funding
The authors gratefully acknowledge funding from the Canadian Institutes for Health Research Rare Diseases grant TR2-119188. The content is solely the responsibility of the authors and does not necessarily represent the official views of the Canadian Institutes for Health Research. RH holds a Canada Research Chair in Health and Genomics and a UBC Killam Professorship. KB and CR are supported by Michael Smith Foundation for Health Research Awards. KG is supported by a UBC 4-Year Fellowship. DC is supported by The Arthritis Society (TAS) Canada through the Ross Petty Arthritis Society Chair.
Conflict of Interest
The authors declare that the research was conducted in the absence of any commercial or financial relationships that could be construed as a potential conflict of interest.
Acknowledgments
The authors thank Stephanie Hughes, Lauren Mak, and Jordan Chiu for outstanding technical assistance. The authors would like to acknowledge all participating patients and their families, without whom this study would not be possible. We thank the PedVas and DCVAS site investigators and clinical coordinators for their dedicated work: Angelyne Rivera, BC Children's Hospital, University of British Columbia, Vancouver BC Canada; Dr. Alan Rosenberg, Joan Dietz, Royal University Hospital and University of Saskatchewan, SK, Canada; Dr. Susanne Benseler, Ana Sepulveda, Alberta Children's Hospital, Calgary, AB, Canada; Dr. Rae Yeung, Shehla Sheikh, Hospital for Sick Children, Toronto ON Canada; Dr. Lillian Barra, Dr. Manoosh Moradizadeh, Sallie Elhayek, Lawson Health Research Institute, London Ontario; Dr. Adam Huber, Cindy Campbell, IWK Health Centre and Dalhousie University, Halifax, NS, Canada; Dr. Suzanne Li, Justine Zasa, Joseph M. Sanzari Children's Hospital, Hackensack University Medical Center, Hackensack, NJ, USA; Dr. Sirirat Charuvanij, Siriraj Hospital, Mahidol University, Bangkok, Thailand; Dr. Goran Ristic, Mother and Child Health Care Institute of Serbia, Belgrade, Serbia; Dr. Melissa Elder, Heather Bell-Brunson, University of Florida, Gainesville, FL, USA; Antje Hellige, University Hospital Muenster, Muenster, Germany; Dr. Aimee Hersh, Suzy Jones, University of Utah's Primary Children's Medical Center, Salt Lake City, UT, USA; Dr. Janet McDonagh, Ann McGovern, Royal Manchester Children's Hospital, Manchester, UK; Dr. Flora McErlane, Claire Duong, Amanda Wood, Great North Children's Hospital, Newcastle Upon Tyne, UK; Dr. Kathleen O'Neil, Katie Gannon, Brooke McCallum, Amy Rakestraw, Riley Children's Hospital, Indianapolis, IN, USA; Dr. Edmond O'Riordan, Salford Royal Foundatio Trust, Salford, UK; Dr. Joanna Robson, Anthea Craven, Jennifer O'Donoghue, Marian Montgomery, David Gray, Ann-Marie Morgan, Oxford University Hospitals NHS Foundation Trust, Oxford, UK; Dr. Marek Bohm, Heather Rostron, Charlotte Wilson, Leeds Children's Hospital, Leeds, UK; Dr. Vidya Sivaraman, Joanne Drew, Nationwide Children's Hopital, Columbus, OH, USA.
Supplementary Material
The Supplementary Material for this article can be found online at: https://www.frontiersin.org/articles/10.3389/fimmu.2021.638571/full#supplementary-material
Abbreviations
AAV, ANCA-associated vasculitis; ANCA, anti-neutrophil cytoplasmic antibody; ARChiVe, A registry for childhood vasculitis; BVAS, Birmingham vasculitis activity score; DCVAS, diagnostic and classification criteria for vasculitis; DE, differentially expressed; EMA, European medicines agency; FDR, false discovery rate; GPA, granulomatosis with polyangiitis; MPA, microscopic polyangiitis; MPO, myeloperoxidase; PAN, polyarteritis nodosa; PR3, proteinase 3; PVAS, pediatric vasculitis activity score; UCV, unclassified vasculitis.
References
1. Okazaki T, Shinagawa S, Mikage H. Vasculitis syndrome-diagnosis and therapy. J Gen Fam Med. (2017) 18:72–78. doi: 10.1002/jgf2.4
2. Hunder GG, Arend WP, Bloch DA, Calabrese LH, Fauci AS, Fries JF, et al. The American College of Rheumatology 1990 criteria for the classification of vasculitis. Introduction. Arthritis Rheum. (1990) 33:1065–7. doi: 10.1002/art.1780330802
3. Jennette JC, Falk RJ, Bacon PA, Basu N, Cid MC, Ferrario F, et al. 2012 revised International Chapel Hill Consensus Conference Nomenclature of Vasculitides. Arthritis Rheum. (2013) 65:1–11. doi: 10.1002/art.37715
4. Ozen S, Pistorio A, Iusan SM, Bakkaloglu A, Herlin T, Brik R, et al. Paediatric Rhematology International Trials Organisation (PRINTO), EULAR/PRINTO/PRES criteria for Henoch-Schonlein purpura, childhood polyarteritis nodosa, childhood Wegener granulomatosis and childhood Takayasu arteritis: Ankara 2008. Part II: Final classification criteria. Ann Rheum Dis. (2010) 69:798–806. doi: 10.1136/ard.2009.116657
5. Watts R, Lane S, Hanslik T, Hauser T, Hellmich B, Koldingsnes W, et al. Development and validation of a consensus methodology for the classification of the ANCA-associated vasculitides and polyarteritis nodosa for epidemiological studies. Ann Rheum Dis. (2007) 66:222–7. doi: 10.1136/ard.2006.054593
6. Yates M, Watts R. ANCA-associated vasculitis. Clin Med. (2017) 17:60–4. doi: 10.7861/clinmedicine.17-1-60
7. Cornec D, Cornec-Le Gall E, Fervenza FC, Specks U. ANCA-associated vasculitis—clinical utility of using ANCA specificity to classify patients. Nat Rev Rheumatol. (2016) 12:570–9. doi: 10.1038/nrrheum.2016.123
8. Uribe AG, Cabral DA, Morishita K. Relative performance of two validated classification systems for Wegener's granulomatosis among patients with ANCA-associated vasculitis in a registry of children with vasculitis (ARChiVe). Arthritis Rheum. (2010) 62(10 suppl):S705.
9. Stone JH, Merkel PA, Spiera R, Seo P, Langford CA, Hoffman GS, et al. Rituximab versus cyclophosphamide for ANCA-associated vasculitis. N Engl J Med. (2010) 363:221–32. doi: 10.1056/NEJMoa0909905
10. Schmitt WH, Birck R, Heinzel PA, Gobel U, Choi M, Warnatz K, et al. Prolonged treatment of refractory Wegener's granulomatosis with 15-deoxyspergualin: an open study in seven patients. Nephrol Dial Transplant. (2005) 20:1083–92. doi: 10.1093/ndt/gfh763
11. Jones RB, Ferraro AJ, Chaudry AN, Brogan P, Salama AD, Smith KG, et al. A multicenter survey of rituximab therapy for refractory antineutrophil cytoplasmic antibody-associated vasculitis. Arthritis Rheum. (2009) 60:2156–68. doi: 10.1002/art.24637
12. Cabral DA, Canter DL, Muscal E, Nanda K, Wahazi DM, Spalding SJ, et al. Comparing presenting clinical features in 48 children with microscopic polyangiitis to 183 children who have granulomatosis with polyangiitis (Wegener's): an archive cohort study. Arthritis Rheum. (2016) 68:2514–26. doi: 10.1002/art.39729
13. Goldman J, Becker ML, Jones B, Clements M, Leeder JS. Development of biomarkers to optimize pediatric patient management: what makes children different? Biomark Med. (2011) 5:781–94. doi: 10.2217/bmm.11.96
14. Shores DR, Everett AD. Children as biomarker orphans: progress in the field of pediatric biomarkers. J Pediatr. (2017) 193:14–20.e31. doi: 10.1016/j.jpeds.2017.08.077
15. Agache I, Akdis CA. Precision medicine and phenotypes, endotypes, genotypes, regiotypes, and theratypes of allergic diseases. J Clin Invest. (2019) 129:1493–503. doi: 10.1172/JCI124611
16. Battaglia M, Ahmed S, Anderson MS, Atkinson MA, Becker D, Becker PJ, et al. Introducing the endotype concept to address the challenge of disease heterogeneity in type 1 diabetes. Diabetes Care. (2020) 43:5–12. doi: 10.2337/dc19-0880
17. Brown KL, Lubieniecka JM, Armaroli G, Kessel K, Gibson KM, Graham J, et al. S100A12 serum levels and PMN counts are elevated in childhood systemic vasculitides especially involving proteinase 3 specific anti-neutrophil cytoplasmic antibodies. Front Pediatr. (2018) 6:341. doi: 10.3389/fped.2018.00341
18. Cabral DA, Uribe AG, Benseler S, O'Neil KM, Hashkes PJ, Higgins G, et al. ARCHiVe investigators network, classification, presentation, and initial treatment of Wegener's granulomatosis in childhood. Arthritis Rheum. (2009) 60:3413–24. doi: 10.1002/art.24876
19. Dolezalova P, Price-Kuehne FE, Özen S, Benseler SM, Cabral DA, Anton J, et al. Disease activity assessment in childhood vasculitis: development and preliminary validation of the Paediatric Vasculitis Activity Score (PVAS) Ann Rheum Dis. (2013) 72:1628–33. doi: 10.1136/annrheumdis-2012-202111
20. Dobin A, Davis CA, Schlesinger F, Drenkow J, Zaleski C, Jha S, et al. STAR: ultrafast universal RNA-seq aligner. Bioinformatics. (2013) 29:15–21. doi: 10.1093/bioinformatics/bts635
21. Anders S, Pyl PT, Huber W. HTSeq—a Python framework to work with high-throughput sequencing data. Bioinformatics. (2015) 31:166–9. doi: 10.1093/bioinformatics/btu638
22. Chen LS, Storey JD. Eigen-R2 for dissecting variation in high-dimensional studies. Bioinformatics. (2008) 24:2260–2. doi: 10.1093/bioinformatics/btn411
23. Leeks JT, Johnson WE, Parker HS, Jaffe AE, Storey JD. The sva package for removing batch effects and other unwanted variation in high-throughput experiments. Bioinformatics. (2012) 28:882–3. doi: 10.1093/bioinformatics/bts034
24. Love MI, Huber W, Anders S. Moderated estimation of fold change and dispersion for RNA-seq data with DESeq2. Genome Biol. (2014) 15:550. doi: 10.1186/s13059-014-0550-8
25. Zweiner I, Frisch B, Binder H. Transforming RNA-Seq data to improve the performance of prognostic gene signatures. PLoS ONE. (2014) 9:e85150. doi: 10.1371/journal.pone.0085150
26. Yu G, He QY. ReactomePA: an R/Bioconductor package for reactome pathway analysis and visualization. Molecular BioSystems. (2016) 12:477–9. doi: 10.1039/C5MB00663E
27. Xia J, Gill EE, Hancock REW. NetworkAnalyst for statistical, visual and network-based meta-analysis of gene expression data. Nat Protoc. (2015) 10:823–44. doi: 10.1038/nprot.2015.052
28. Grayson PC, Steiling K, Platt M, Berman JS, Zhang X, Xiao J, et al. Defining the nasal transcriptome in granulomatosis with polyangiitis (Wegener's). Arthritis Rheumatol. (2015) 67:2233–9. doi: 10.1002/art.39185
29. Wu D, Lim E, Francois Vaillant F, Asselin-Labat ML, Visvader JE, Smyth GK. ROAST: rotation gene set tests for complex microarray experiments. Bioinformatics. (2010) 26:2176–82. doi: 10.1093/bioinformatics/btq401
30. Wu D, Smith GK. Camera: a competitive gene set test accounting for inter-gene correlation. Nucleic Acids Res. (2012) 40:e133. doi: 10.1093/nar/gks461
31. Le S, Josse J, Husson F. FactoMineR: an R package for multivariate analysis. J. Statistical Software. (2008) 25:1–18. doi: 10.18637/jss.v025.i01
32. Lacy P. Mechanisms of degranulation in neutrophils. Allergy Asthma Clin Immunol. (2006) 2:98–108. doi: 10.1186/1710-1492-2-3-98
33. Papayannopoulos V. Neutrophil extracellular traps in immunity and disease. Nat Rev Immunol. (2018) 18:134–47. doi: 10.1038/nri.2017.105
34. Kessenbrock K, Krumbholz M, Schonermarck U, Back W, Gross WL, Werb Z, et al. Netting neutrophils in autoimmune small-vessel vasculitis. Nat Med. (2009) 15:623–5. doi: 10.1038/nm.1959
35. Yoshida M, Sasaki M, Sugisaki K, Yamaguchi Y, Yamada M. Neutrophil extracellular trap components in fibrinoid necrosis of the kidney with myeloperoxidase-ANCA-associated vasculitis. Clin Kidney J. (2013) 6:308–12. doi: 10.1093/ckj/sft048
36. Urban CF, Ermert D, Schmid M, Abu-Abed U, Goosmann C, Nacken W, et al. Neutrophil extracellular traps contain calprotectin, a cytosolic protein complex involved in host defense against Candida albicans. PLoS Pathog. (2009) 5:e1000639. doi: 10.1371/journal.ppat.1000639
37. Jin H, Arase N, Hirayasu K, Kohyama M, Suenaga T, Saito F, et al. Autoantibodies to IgG/HLA class II complexes are associated with rheumatoid arthritis susceptibility. Proc Natl Acad Sci. (2014) 111:3787–92. doi: 10.1073/pnas.1401105111
38. Zeyda M, Huber J, Prager G, Stulnig TM. Inflammation correlates with markers of T-cell subsets including regulatory T cells in adipose tissue from obese patients. Obesity. (2011) 19:743–8. doi: 10.1038/oby.2010.123
39. Hirakara K, Nakayama T. CD4+ T-cell subsets in inflammatory diseases: beyond the Th1/Th2 paradigm. Int Immunol. (2016) 28:163–71. doi: 10.1093/intimm/dxw006
40. Nguyen LP, Pan J, Dinh TT, Hadeiba H, O'Hara III E, Ebtikar A, et al. Role and species-specific expression of colon T cell homing receptor GPR15 in colitis. Nat Immunol. (2015) 16:207–13. doi: 10.1038/ni.3079
41. Endo Y, Hirahara K, Iinuma T, Shinoda K, Tumes DJ, Asou HK, et al. The interleukin-33-p38 kinase axis confers memory T helper 2 cell pathogenicity in the airway. Immunity. (2015) 42:294–308. doi: 10.1016/j.immuni.2015.01.016
42. Mitson-Salazar A, Yin Y, Wansley DL, Young M, Bolan H, Arceo S, et al. Hematopoietic prostaglandin D synthase defines a proeosinophilic pathogenic effector human T(H)2 cell subpopulation with enhanced function. J Allergy Clin Immunol. (2016) 137:907–18. doi: 10.1016/j.jaci.2015.08.007
43. Friedman MA, Choi D, Planck SR, Rosenbaum JT, Sibley CH. Gene expression pathways across multiple tissues in antineutrophil cytoplasmic antibody-associated vasculitis reveal core pathways of disease pathology. J. Rheumatol. (2019) 46:609–15. doi: 10.3899/jrheum.180455
44. Westwell-Roper C, Lubienieka JM, Brown KL, Morishita KA, Mammen C, Wagner-Weiner L, et al. Clinical practice variation and need for pediatric-specific treatment guidelines among rheumatologists caring forchildren with ANCA-associated vasculitis: an international clinician survey. Pediatr Rheumatol Online J. (2017) 15:61. doi: 10.1186/s12969-017-0191-z
Keywords: vasculitis, neutrophils, transcriptome, inflammation, ANCA
Citation: Gill EE, Smith ML, Gibson KM, Morishita KA, Lee AHY, Falsafi R, Graham J, Foell D, Benseler SM, Ross CJ, Luqmani RA, Cabral DA, Hancock REW, Brown KL and the PedVas Initiative Investigators (2021) Different Disease Endotypes in Phenotypically Similar Vasculitides Affecting Small-to-Medium Sized Blood Vessels. Front. Immunol. 12:638571. doi: 10.3389/fimmu.2021.638571
Received: 07 December 2020; Accepted: 01 February 2021;
Published: 22 February 2021.
Edited by:
Joshua Daniel Ooi, Monash University, AustraliaReviewed by:
Carlo Chizzolini, Université de Genève, SwitzerlandJillian M. Richmond, University of Massachusetts Medical School, United States
Copyright © 2021 Gill, Smith, Gibson, Morishita, Lee, Falsafi, Graham, Foell, Benseler, Ross, Luqmani, Cabral, Hancock, Brown and the PedVas Initiative Investigators. This is an open-access article distributed under the terms of the Creative Commons Attribution License (CC BY). The use, distribution or reproduction in other forums is permitted, provided the original author(s) and the copyright owner(s) are credited and that the original publication in this journal is cited, in accordance with accepted academic practice. No use, distribution or reproduction is permitted which does not comply with these terms.
*Correspondence: David A. Cabral, dcabral@cw.bc.ca
†These authors have contributed equally to this work