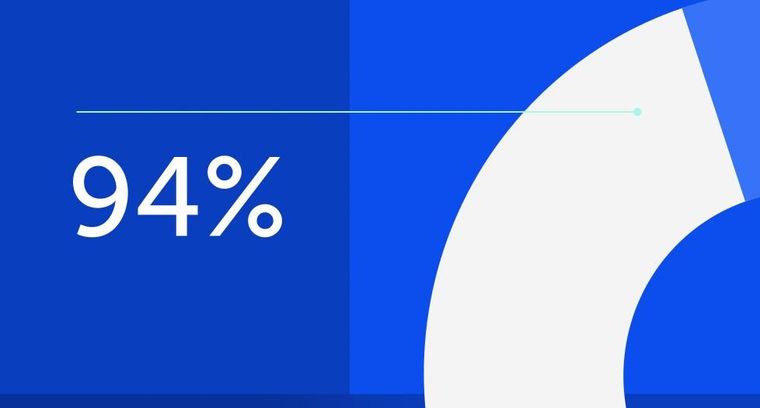
94% of researchers rate our articles as excellent or good
Learn more about the work of our research integrity team to safeguard the quality of each article we publish.
Find out more
ORIGINAL RESEARCH article
Front. Immunol., 17 May 2021
Sec. NK and Innate Lymphoid Cell Biology
Volume 12 - 2021 | https://doi.org/10.3389/fimmu.2021.599805
Background: Dengue virus (DENV) infection has a global impact on public health. The clinical outcomes (of DENV) can vary from a flu-like illness called dengue fever (DF), to a more severe form, known as dengue hemorrhagic fever (DHF). The underlying innate immune mechanisms leading to protective or detrimental outcomes have not been fully elucidated. Helper innate lymphoid cells (hILCs), an innate lymphocyte recently discovered, functionally resemble T-helper cells and are important in inflammation and homeostasis. However, the role of hILCs in DENV infection had been unexplored.
Methods: We performed flow cytometry to investigate the frequency and phenotype of hILCs in peripheral blood mononuclear cells from DENV-infected patients of different disease severities (DF and DHF), and at different phases (febrile and convalescence) of infection. Intracellular cytokine staining of hILCs from DF and DHF were also evaluated by flow cytometry after ex vivo stimulation. Further, the hILCs were sorted and subjected to transcriptome analysis using RNA sequencing. Differential gene expression analysis was performed to compare the febrile and convalescent phase samples in DF and DHF. Selected differentially expressed genes were then validated by quantitative PCR.
Results: Phenotypic analysis showed marked activation of all three hILC subsets during the febrile phase as shown by higher CD69 expression when compared to paired convalescent samples, although the frequency of hILCs remained unchanged. Upon ex vivo stimulation, hILCs from febrile phase DHF produced significantly higher IFN-γ and IL-4 when compared to those of DF. Transcriptomic analysis showed unique hILCs gene expression in DF and DHF, suggesting that divergent functions of hILCs may be associated with different disease severities. Differential gene expression analysis indicated that hILCs function both in cytokine secretion and cytotoxicity during the febrile phase of DENV infection.
Conclusions: Helper ILCs are activated in the febrile phase of DENV infection and display unique transcriptomic changes as well as cytokine production that correlate with severity. Targeting hILCs during early innate response to DENV might help shape subsequent immune responses and potentially lessen the disease severity in the future.
Dengue virus (DENV) infection is a serious public health threat, especially in tropical and subtropical areas. This important mosquito-borne virus infects approximately 390 million people annually (1). Clinical manifestations range from asymptomatic, mild dengue fever (DF) to severe life-threatening dengue hemorrhagic fever (DHF) and dengue shock syndrome (DSS) (2). Currently, there is no specific treatment. The only licensed vaccine, Dengvaxia, showed limited efficacy and inadvertently increased hospitalization rate in children and dengue-naive vaccinees (3–5). The high prevalence, absence of specific treatment and lack of effective vaccine result in prominent global burden, clinically, and economically. This is in part due to the inadequate understanding of immune responses to DENV infection.
Complex interactions between DENV and host immune responses lead to the various outcomes of the infection. While protective immune response is required for viral clearance and resolution of the infection, detrimental response results in increased viral propagation, cytokine storm, plasma leakage, and severe disease outcome (6–9). DENV is a single stranded positive-sense RNA virus in the Flaviviridae family. In humans, there are 4 DENV serotypes. Secondary heterotypic infection is associated with an increased chance of developing severe disease (10, 11), likely due to pathogenic memory T and B cell response from previous infection known as T cell antigenic sin (9, 12, 13) and antibody-dependent enhancement (14, 15). Innate and innate-like responses to DENV infection are not only crucial as the first line of defense but also influence subsequent adaptive T and B cell responses (16). Beside the role of innate-like T cells, NKT (17–19) and MAITs (20, 21), several lines of evidence suggested the important roles of various innate responses in DENV infection and viral evasion strategies, in particular type I IFN (22–24), monocytes, macrophages and dendritic cells (25), mast cells (18, 26), as well as NK cells (27). However, the roles of innate lymphoid cells (ILCs), a very important and most recently discovered innate immune cells, in DENV infection has never been investigated.
ILCs are innate counterparts of T cells that can respond rapidly, orchestrate early innate responses, and shape subsequent adaptive responses (28–35). Unlike T cells, they do not express T-cell receptors, thus do not respond in an antigen-specific manner. ILCs comprise NK cells, helper ILCs (hILCs), and lymphoid tissue inducers (LTi). Helper ILCs are classified into ILC1, ILC2, and ILC3, based on expression of major transcription factors and their signature cytokines, which resemble those of Th1, Th2 and Th17/Th22 cell subsets. In general, ILC1 produces IFN-γ; ILC2 produces IL-4, IL-5 and IL-13; and ILC3 produces IL-17A and/or IL-22 (32, 33, 36, 37). Recent evidence also showed the plasticity of ILCs that enable them to promptly respond to environmental changes (38). hILCs have been shown to play both protective and detrimental roles in various diseases, including allergy (39–41), autoimmunity (42–44), cancers (45–48), inflammation and infectious diseases caused by various pathogens including viruses (49–55).
While the critical roles of hILCs have been demonstrated in several viral infections, most studies were done in murine models. These include diverse roles in host protection, immunopathology, and tissue homeostasis in influenza A virus (IAV) (56–60), respiratory syncytial virus (RSV) (61–63), rhinovirus (64, 65), herpes simplex virus (HSV) (66), rota virus (67), and mouse cytomegalovirus (MCMV) (68–70). Because mouse and human hILCs differ significantly (71), study in the human system is critical. Using human hILCs co-cultured with viruses in vitro, hILCs were shown to respond to rhinovirus (72), IAV (56, 58), and human cytomegalovirus (HCMV) (73). However, the study of hILCs in natural viral infection in humans has been limited to those of HIV infection (74).
Here, we investigated the potential roles of hILCs in natural human DENV infection using clinical samples from a well-characterized DENV-infected patient cohort. Flow cytometric analysis showed marked hILCs activation during the febrile phase which diminished at convalescence in both DF and DHF, while hILC number and subset composition remained unchanged. Upon ex vivo stimulation, hILCs from febrile phase DHF produced more cytokine than those of DF. Furthermore, global gene expression analysis revealed upregulation of different sets of genes in the febrile phase of DF and DHF patients. These results suggested that hILCs play a role in response to febrile phase of DENV infection and that diverged hILCs functional responses were associated with different clinical outcomes of the infection. A better understanding of hILCs within the complex host-viral interaction in the pathogenesis of DENV infection may contribute to future development of effective preventative and therapeutics approaches.
This study used human samples collected from Dengue Research Framework for Resisting Epidemics in Europe (DENFREE) (75). The DENFREE (Thailand) (76) study was approved by the Institutional Review Board of Faculty of Medicine Vajira Hospital (No.015/12) and the Faculty of Tropical Medicine Mahidol University (TMEC 13-041). All subjects or their legal guardians signed written informed consent prior to study participation. The use of archived DENFREE samples in this study was approved by the ethical committee of the Faculty of Medicine, Ramathibodi hospital (COA.MURA2019/603).
Blood samples were collected from DENV-infected patients who presented with febrile illness with confirmed presence of DENV RNA in plasma by RT-PCR, as previously described (77, 78). The DENV-infected patients were classified according to WHO 1997 classification criteria into dengue fever (DF) and dengue hemorrhagic fever (DHF).
Samples from two timepoints were evaluated in this study (Supplementary Table 1). Febrile phase samples were collected one day before fever subsided to represent the febrile phase of DENV infection. Convalescent phase samples were collected two months after hospital discharge (Figure 1A). Peripheral blood mononuclear cells (PBMCs) were isolated using density gradient centrifugation (Lymphoprep, STEMCELL Technologies, 07851). Aliquots of PBMCs were then cryopreserved in freezing media (90% FBS, 10% DMSO) in liquid nitrogen until used. PBMCs from 10 DF and 10 DHF patients at both febrile and matched convalescent phases (when available) were used for surface flow cytometric experiments (Figures 1, 2). A subset of these samples (3 DF and 5 DHF patients) was used for hILC cell sorting experiment in which 3 DF and 3 DHF samples proceeded to RNA sequencing (RNA-seq) experiment (Figures 4, 5). The cDNA from sorted hILCs (3 DF and 5 DHF patients) were used for qPCR experiment. A different set of PBMCs at febrile phase (10 DF and 14 DHF patients) were used for intracellular cytokine staining experiment (Figure 3).
Figure 1 Frequencies of hILCs during the course of DENV infection. (A) Study design (B) Flow cytometry analysis gating strategy for hILCs and their subsets from PBMC. (C, D) Percentage of total hILCs and hILC subsets in febrile phase of dengue fever (DF), dengue hemorrhagic fever (DHF) patients and healthy donors (HC). ILC subsets frequencies were determined by percentage of CD45+ lymphocyte (C) or total hILCs (D). (E, F) Percentage of total hILCs and ILC subsets of matched samples at febrile and convalescent phases of DF patients. ILC subset frequencies were determined by percentage of CD45+ lymphocyte (E) or total hILCs (F). (G, H) Percentage of total hILCs and ILC subsets of matched samples at febrile and convalescent phases of DHF patients. ILC subsets frequencies were determined by percentage of CD45+ lymphocyte (G) or total hILCs (H). The results were presented as Median ± IQR. Data were analyzed using Mann-Whitney test (C, D) or Wilcoxon matched-pairs signed rank test (E–H) (**p < 0.01).
Figure 2 Activation of hILCs during the febrile phase of DENV infection. (A) Representative contour plot of CD69 expression (light grey, blue, red, and orange) as compared with isotype control (dark grey) on total hILC and each hILC subsets in febrile phase and convalescent phases. (B) Percentage of CD69+ (upper panel) and ΔMFI (CD69 MFI - isotype control MFI) (lower panel) for total hILCs and each hILC subset in febrile phase of dengue fever (DF) patients and dengue hemorrhagic fever (DHF) patients compared to healthy donors (HC). (C) Percentage of CD69+ and (D) ΔMFI for total and hILC subsets of febrile phase compared to matched convalescent of DF (upper panel) and DHF (lower panel). Each line connected data of the same patient between two timepoints. Wilcoxon matched-pairs signed rank test was used for statistical comparison, p < 0.05 was considered as a statistically significant difference. (E) Percentage of CD69+ (upper panel) and ΔMFI (lower panel) for total and hILC subsets in convalescent phase of DF and DHF patients compared to healthy donors (HC). The results were presented as Median ± IQR. Data were analyzed using Mann-Whitney test (B, E) or Wilcoxon matched-pairs signed rank test (C, D) (*p < 0.05, **p < 0.01, ***p < 0.001, ****p < 0.0001).
Figure 3 Intracellular cytokine staining of hILCs from febrile DF and DHF after PMA/ionomycin ex vivo stimulation (A) Flow cytometry analysis gating strategy of total hILCs from PBMC. (B) Representative contour plots of IFN-γ, IL-4, IL-13, IL-17A, and IL-10 expression (red) overlaid on FMO control (grey). (C) Percentage of IFN-γ, IL-4, IL-13, IL-17A, and IL-10 expression of hILCs in febrile phase of DF and DHF patients. The results were presented as median ± IQR. Data were analyzed using Mann-Whitney test (*p < 0.05, **p < 0.01; ns, not significant).
Cryopreserved PBMCs were thawed at 37°C with warm RPMI-1640 (Gibco, 11875119) supplemented with 10% FBS (Gibco, 10099141). After washing twice with RPMI, cell viability was assessed using trypan blue exclusion assay. Cell viability of all samples in this study exceeded 90%. PBMCs were stained with LIVE/DEAD fixable violet (Invitrogen, L34963) according to the manufacturer’s protocol. Subsequently, Fc blocking with Human TruStain FcX™ (Biolegend, 422302) and cell surface staining were performed. The fluorophore-conjugated antibodies for cell surface staining comprise of lineage cocktail (CD3, CD14, CD16, CD19, CD20, CD56) (FITC), CD117 (PE), CRTH2 (Alexa Fluor 647), CD127 (APC/Cy7), CD45 (V500), and CD69 (Alexa Fluor 700) (for list of antibodies see Supplementary Table 2). Fluorescence minus one (FMO) and isotype controls (mIgG1-Alexa Fluor 700, BD Biosciences) were used to evaluate CD69 expression. Surface staining was performed on ice for 30 minutes in FACS Buffer (2% FBS and 0.25 mM EDTA in PBS). Stained samples were then acquired with the CytoFLEX flow cytometer (Beckman Coulter) using CytExpert version 2.3. FlowJo version 10 (FlowJo, LLC) and GraphPad Prism version 7 were used for data analysis. hILCs were identified within the lymphocyte region on the basis of their forward and side scatter profiles (FSClow and SSClow) in conjunction with CD45highSSClow after excluding doublets and dead cells. Total hILCs were gated as CD45+Lin−CD127+ cells. The hILC subsets were then identified within total hILCs as follows: CD117-hILCs as ILC1, CD161+CRTH2+hILCs as ILC2, and CD117+hILCs as ILC3 (Figure 1B).
Cryopreserved PBMCs were thawed and processed as described above. Fc blocking with Human TruStain FcX™ (Biolegend, 422302) was performed according to the manufacturer’s protocol. Cell surface staining with lineage cocktail (FITC) and CD127 (APC/Fire750) was performed at RT for 15 minutes. PBMCs were then stimulated with PMA (50 ng/ml) and Ionomycin (1 ug/ml) for 4 hours with protein transport inhibitor, BD GolgiPlug (BD, 555029). PBMCs were stained with Zombie Violet Fixable Viability Kit (Biolegend, 423114) before undergoing intracellular cytokine staining with BD Cytofix/Cytoperm™ (BD, 554714) according to manufacturer’s protocol. IFN-γ (PE), IL-4 (BV510), IL-13 (PerCP/Cy5.5), IL-17A (Alexa Fluor 700), and IL-10 (Alexa Fluor 647) were used for intracellular cytokine staining for 30 minutes on ice (see Supplementary Table 2 for a list of antibodies used). Stained samples were then acquired and analyzed with CytExpert version 2.3 and GraphPad Prism version 7, in comparison to fluorescence minus one (FMO) control.
PBMCs from 3 DF and 5 DHF patients at febrile and convalescent phases were sorted for hILCs using a FACS Aria III instrument (BD Biosciences) for hILCs RNA-seq and qPCR experiments. PBMCs were stained with Lineage cocktail antibodies (CD3, CD14, CD16, CD19, CD20, CD56) (FITC) and CD127 (APC/Cy7). After staining, cells were washed and resuspended in a cold FACS buffer (2% FBS and 0.25 mM EDTA in PBS). Immediately before sorting, cells were filtered through a 35-μm strainer. Helper ILCs were gated as Lin-CD127+ cells within the lymphocyte gate (FSClowSSClow) after exclusion of doublets and sorted directly into in 96-well plates with 4 μl of lysis buffer composed of 0.4% Triton-X 100 (Calbiochem, 648466), recombinant RNase inhibitor (Clonetech, 2313B), and 10 mM dNTP mix (Bioline, 39053). Sorting mode was set to single cell resolution in order to gain the highest purity. Fifty hILCs were sorted into each well. Approximately 3-5 wells were obtained per sample. Plates were immediately spun down after sorting, then sealed and snapped frozen with dry ice before moving to -80°C freezer for storage until further processing within 2-3 days.
The generation of full-length transcriptomes from a low number of cells per sample was performed based on the SMART-seq2 protocol (79), with modifications. Briefly, mRNA was captured using poly-dT oligonucleotides and reverse-transcribed into full-length cDNA using the described template-switching oligonucleotide and SMARTScribe (Clonetech, 639537). cDNA was amplified by PCR (PCRmax Alpha Cycler 2) for 14 cycles using the KAPA HiFi HotStart Readymix (Roche, KK2601, 07958927001) and then purified using AMPure XP (Beckman Coulter, A63881) magnetic beads at 0.8:1 (vol/vol) ratio. For each well, cDNA quality was assessed with Agilent Tapestation (expected peak ~ 1.5-2 kb). cDNA profiles containing short fragments (< 500 bp), possibly due to RNA degradation, were excluded. cDNA from three wells of the same sample were pooled and normalized to 300 pg/ul for subsequent library construction using the Nextera XT library preparation kit (Illumina, FC-131-1096) and the index kits (Illumina, FC-131-2001). Each library’s size was assessed by Agilent Tapestation. The libraries were sequenced using the Illumina HiSeq platform (Macrogen, South Korea) with paired-end 150-bp read length and coverage of approximately 30 million reads per sample.
RNA from sorted hILCs were reverse transcribed and preprocessed into cDNA as described above. cDNA was normalized to 5 ng/ul for each PCR reaction. Quantitative PCR reactions were prepared with Q5 High-Fidelity 2X Master Mix (NEB, M0492L) and SYBR green I (Roche, 11988131001). Oligos used for qPCR are listed in Supplementary Table 3. All qPCR reactions were performed using the Rotor-Gene Q real-time cycler (Qiagen). The specificity of the reaction was verified by melting curve analysis. Delta Ct value for each gene is compared to ACTB.
Nextera adapter sequences were removed using Trimmomatic version 0.36 (80). Trimmed reads were mapped and aligned using HISAT2 (81), with GRCh38 as the reference genome. Normalized relative transcript abundances as Transcripts Per Kilobase Million (TPM) were obtained using StringTie (82). Raw read counts were obtained with the HTseq-count version 0.6.1p1 (83).
After removal of absent features (zero counts in all samples), the raw counts were then imported to DESeq2 version 1.24.0 (84) to identify differentially expressed (DE) genes. DE analysis was performed by comparing the febrile phase and convalescent phase samples of the same individuals (“paired samples” in the design formula of DESeq2), so the biases between the donors were internally normalized. Wald-test p values were adjusted for multiple testing using the Benjamini-Hochberg method, and genes with adjusted p values less than 0.01 and with log2 fold changes greater than 2 or less than -2, were considered significantly differentially expressed between the two phases. Because of the low sample numbers, which may cause high variability within each sample group, shrinkage estimator ‘apeglm’ was applied to re-estimate the log2 fold change. Apeglm estimates the effect size more accurately, especially when read counts are low and highly variable (85). Genes with adjusted p values less than 0.01 and with re-estimated log2 fold changes greater than 2 or less than -2, were considered statistically significant. Functional gene set analysis was assessed using the Gene Ontology (GO) biological process analysis with gprofiler (86). ComplexHeatmap (87) was used to generate heatmap plots for visualization.
To investigate the role of hILCs during DENV infection, we first examined the frequency of hILCs and hILC subsets in PBMC from DF and DHF patients during febrile phase of DENV infection, as compared to those at the convalescence and also to healthy controls (HC), using flow cytometry (Figures 1A, B). There was no significant difference in terms of total hILC frequency among febrile DF (median 0.12, IQR 0.07 - 0.16), DHF (median 0.14, IQR 0.12 - 0.23), and HC (median 0.22, IQR 0.13 - 0.29) (Figure 1C). No obvious change in hILC subset distribution was observed, with the exception of a lower percentage of ILC3 in the febrile DF patients (% of CD45+ median 0.03, IQR 0.02 - 0.06; % of hILCs median 22.80, IQR 18.33 - 45.23), when compared to HC (% of CD45+ median 0.10, IQR 0.08 - 0.12; % of hILCs median 53.10, IQR 40.30 - 60.10) (p < 0.01) (Figures 1C, D). Furthermore, the frequency of total hILCs and hILC subsets were not significantly different when compared between the febrile phase of DENV infection and the convalescence of the same patient, regardless of disease severity (Figures 1E–H). Thus, hILC frequency did not change during DENV infection.
To investigate whether hILCs were activated during DENV infection, the expression of CD69 (in comparison to FMO control) on hILCs was investigated (Figure 2A). The percentage of CD69+ hILCs, when compared to that of the healthy donors (median 3.56, IQR 2.38 - 5.04), were significantly higher in both febrile DF (median 21.95, IQR 10.72 - 32.13, p < 0.001) and febrile DHF (median 21.55, IQR 12.58 - 33.05, p < 0.001) (Figure 2B, upper panel). The changes in CD69 mean fluorescence intensity (ΔMFI) showed a similar result (Figure 2B, lower panel). However, the expression level of CD69 was not different between hILCs of DF and DHF patients at the same time points. Expression of CD69 on ILC1 (DF median 25.50, IQR 13.73 - 31.03; DHF median 27.25, IQR 18.85 - 31.93; HC median 0.95, IQR 0.00 - 2.17), ILC2 (DF median 14.70, IQR 7.36 - 31.40; DHF median 16.65, IQR 6.67 - 35.13; HC median 1.44, IQR 0.80 - 2.40), and ILC3 (DF median 36.35, IQR 19.40 - 55.65; DHF median 30.90, IQR 21.75 - 38.45; HC median 1.30, IQR 0.86 - 3.58) were significantly higher during febrile DF and DHF when compared to HC.
In addition, analysis of hILC activation kinetics by comparing expression levels of CD69 between the febrile and convalescent samples from the same patient showed a decrease in hILC activation when disease subsided (Figures 2C, D, left most column). Further analysis on all hILC subsets show similar results of decreased activation in the convalescence (Figures 2C, D). Interestingly, low level of hILC activation seemed to persist in the convalescent phase as their CD69 expression was still higher than those of healthy donors (Figure 2E). These results suggest that hILCs were highly activated during the febrile phase of DENV infection, and the activation diminished to a low level during the convalescent phase of infection.
To assess the functions of hILCs, we performed intracellular cytokine staining of hILCs from febrile DF and DHF patients, after ex-vivo stimulation. The expression of IFN-γ, IL-4 and IL-13, and IL-17A (representative functional cytokines of ILC1, ILC2, and ILC3, respectively) were evaluated on total hILCs (Figures 3A, B). In addition, IL-10 was also evaluated. The percentage of hILCs expressing IFN-γ was higher in DHF (median 23.85, IQR 15.05 - 28.43) than DF (median 14.42, IQR 12.94 - 19.92) (p = 0.042) (Figure 3C). Likewise, the percentage of hILCs producing IL-4 was also higher in DHF (median 7.86, IQR 6.98 - 11.94) compared to DF (median 5.23, IQR 4.08 - 6.19) (p = 0.003). A very small percentage of hILCs produce IL-17A (median 2.11 in DHF and median 1.21 in DF) (p = 0.036) (Figure 3C). No statistical differences in IL-13 and IL-10 production were observed among hILCs from DHF and DF (Figure 3C). This result suggests that hILCs from febrile DHF are functionally active and capable of producing their cytokines, more than in DF.
To explore the molecular functions of hILCs in febrile phase of DENV infection, we next examined the global gene expression profile using RNA-seq of FACS-sorted hILCs from samples of 3 DF patients, 3 DHF patients in febrile phase and matched convalescent phase of the same patients.
As expected, hILC signature genes, including KLRB1 and IL7R, were detected in hILCs from all samples (Figure 4A). Meanwhile, the signature genes of hILC subsets (GATA3, KIT, AHR, PTGDR2, TBX21) were expressed at varying levels between samples (Figure 4A), likely due to the differences in the hILC subset composition among samples (Figures 1C, D). The gene markers for T cell, B cell, NK cell, and monocyte were rarely expressed in the hILCs. Together, these data further verified the identity of hILCs. Importantly, CD69 gene expression was upregulated in hILCs from febrile DF and febrile DHF, when compared to convalescence (Figure 4B lower panel), similarly to CD69 surface protein upregulation measured by flow cytometry (Figure 4B upper panel). Gene expression data from RNA-seq and protein expression data showed similar trends when compared between the two severities (DF vs DHF) and timepoints (febrile vs convalescence) (Figure 4B). Correlation analyses of global gene expression using unsupervised hierarchical clustering between samples showed that the febrile phase samples were clustered together (Figure 4C). However, the overall expression profiles cannot clearly distinguish the different disease severities. Principal component analysis (PCA) similarly showed hILCs from febrile phase samples clustered together and away from convalescent samples on PC2 (Figure 4D).
Figure 4 Transcriptome analysis of hILCs from DENV-infected patients. (A) Heatmap showing expression level (in TPM) of known hILCs and their subsets’ signature genes as compared to those of T cells, B cells and NK cells in each sample arranged according to disease severity (DF, DHF) and timepoints (febrile, convalescence). (B) Bar plots show mean MFI from previous flow cytometry experiment (upper panel) and gene expression levels in TPM (lower panel) for CD69, CD161 (KLRB1), c-kit (CD117, KIT), and CRTH2 (CD294, PTGDR2). (C) Correlation of global transcriptome profiles of hILCs in all samples. Unbiased hierarchical clustering shows high correlation amongst samples according to timepoints (febrile phase clustered together and away from convalescence). (D) PCA plot with top contribution genes for PC1 and PC2 shows separation between febrile and convalescent phase on PC2 which were contributed by the expression of CD69, EGR1, IFIT3, NFKBIA, and IFI44L. Sample number indicated on top of heatmap; DF1_F (DF sample number 1, febrile phase), DF1_C (DF, sample number 1, convalescent phase).
To further explore the functions of hILCs in febrile phase of DENV infection, we next performed pairwise differential gene expression analysis, comparing the differences between febrile and convalescent samples of the same patients in the DF and DHF groups (Figure 5A and Supplementary Figures 1A, C). Overall, a total of 261 and 228 genes were upregulated in the febrile phase of DF and DHF, respectively. Surprisingly, only 16 genes were upregulated in both DF and DHF, suggesting diverged functional responses of hILCs in different severity outcomes. Genes that were upregulated in the febrile phase of both DF and DHF, and those uniquely upregulated in DF or DHF are listed in Supplementary Tables 4, 5. We next performed functional gene set analysis using an over-representative test on the differentially expressed genes in the febrile DF (Figure 5B) and DHF (Figure 5C). In DF, the modules that were upregulated include pyruvate metabolic process, activation of JAK activity, positive regulation of TNF superfamily cytokine, and positive regulation of cytokine production (Figure 5D). In contrast, pathways that were upregulated in DHF include small molecule metabolic process, organophosphate and nucleotide/nucleoside biosynthetic process as well as nuclear mRNA surveillance of mRNA 3’-end process (Figure 5E).
Figure 5 Differential gene expression analysis of hILCs between febrile and convalescent phase of DENV infection (A) Plot comparing the fold change of DF (febrile over convalescent phase) (Y axis) to the fold change of DHF (febrile over convalescent phase) (X axis) depicts transcripts differentially expressed between disease severity and timepoint. Colored dots denote transcripts that are differentially expressed at least 2-fold higher as well as adjust p value less than 0.01. Blue or green colored dots denote differentially expressed in DF or DHF respectively. Red colored dots denote transcripts that are differentially expressed by both DF and DHF. (B, C) Bar plots show significant GO term enrichment from DE genes comparing febrile and convalescent phases in (B) DF and (C) DHF patients. (D, E) Violin plots show fold change of expression levels of matched samples between febrile and convalescent phases. Each dot represents a gene in the designated GO term by each patient. (D) show enriched GO term in DF patients and (E) shows GO term enriched in DHF patients. (F) Venn diagram shows number of differentially expressed genes in DF (febrile over convalescent phase), DHF (febrile over convalescent phase) and both after shrinkage algorithm ‘apeglm’ was applied. Immune-related genes are listed beside the diagram. (G) Expression levels of HAVCR2, TRIM21, GZMB, SLAMF7, and IFNG by quantitative PCR (delta Ct value (dCt) = Ct value of ACTB - Ct value of interested gene). Bar showing median ± IQR.
To explore the diverse roles of hILCs during the febrile phase in DF and DHF with higher stringency, we applied shrinkage estimator ‘apeglm’ to analyse differential gene expression (85). After re-estimation of log2 fold change, only EGR1 is upregulated in both febrile DF and DHF. The top immune-related genes uniquely upregulated in febrile DF (as compared to convalescence) were IFIT1, IFI44L, HAVCR2 (Tim3) while those uniquely upregulated in febrile DHF were GZMB, SLAMF7, RORC, TRIM21, and IL15RA. (Figure 5F, Supplementary Figure 1B, D and 2 and Supplementary Tables 6, 7).
To corroborate the transcriptome analysis, we performed qPCR of HAVCR2, GZMB, SLAMF7 and TRIM21 from sorted hILCs as representatives of genes that were upregulated in DF or DHF. We observed a trend of higher HAVCR2 expression in DF patients and higher TRIM21 expressions in DHF patients (Figure 5G), as was observed in the transcriptome results. However, the expression of GZMB and SLAMF7 were highly variable and no trend was observed between severities. The expression of IFNG as a representative cytokine gene of ILC1 was also show higher trend in DHF than DF (Figure 5G), consistently with the intracellular cytokine staining result. Taken together, our results suggest that in response to the DENV infection, hILCs were activated during the febrile phase in both DF and DHF, but demonstrated divergent transcriptomic responses, implying different functional roles in response to DENV infection.
To the best of our knowledge, this study showed the first evidence of hILC responses in human DENV infection, a pressing public health problem worldwide. By assessing blood samples from a well-characterized human DENV infection cohort, we found that hILCs were activated in the febrile phase of DENV infection and their cytokine production as well as transcriptional profiles in the febrile phase were distinct between DF and DHF patients.
Our results showed that the frequency of hILCs in the febrile phase of DENV infection remained unchanged when compared to matched convalescent samples, and to healthy control samples. This differs from acute HIV infection, where the number of circulating hILC were depleted irreversibly during acute infection likely from apoptosis (74). In animal models, hILC frequency was found to increase during some viral infections [IAV (56), rotavirus (67), late phase of MCMV (69), and rhinovirus (72)], but decrease in others [early phase of MCMV (69) and SIV (88)]. Although hILC frequency and subset composition remained unchanged in DENV infection, we found that hILCs were activated during the febrile phase. (Figure 2)
The activation of hILCs, as demonstrated by CD69 upregulation, was clearly observed during the febrile phase of DENV infection in both DF and DHF. ILC activation has also been observed in several other viral infections. For instance, lung ILC1 was previously shown to be activated in IAV infection along with the upregulation of IFN-γ (59). In addition, the upregulation of CD69 was found in circulating hILCs in early HIV infection (74). hILCs are generally known to be activated by cytokines depending on hILC subsets (32, 33). In IAV infection, lung ILC2 senses the alarmins from lung macrophages and subsequently secretes IL-13. In rotavirus infection, ILC3 responds to IL-1α secreted from intestinal epithelial cells (67). However, it is still unclear how hILCs are activated in the context of systemic viral infections, including DENV.
Our results showed that hILCs from the febrile phase of DHF produce more cytokines than those of DF. Cytokine storm is known to contribute to dengue severity (6, 9). While the higher serum level of IFN-γ and IL-4 have been previously observed in severe dengue patients (89), the cellular source of these cytokines especially IFN-γ are believed to be T cells (9, 90). We here showed that hILCs can secrete these cytokines during the febrile phase of infection, thus may contribute as the early source of these cytokines and likely play roles in shaping downstream adaptive T cell responses as previously shown in other models (91).
Our transcriptomic analysis suggests that the functions of hILCs likely differ between DF and DHF, as demonstrated by their distinct transcriptomic profiles. We first verified the sorted hILCs identity by observing high expression of hILCs combined gene sets, PTPRC (CD45), IL7R (CD127), and KLRB1 (CD161), in all samples. We note some low level expression of conventional T/NK cell markers, CD3E, GZMB, and SLAMF7; these genes were also found to be lowly expressed in human hILCs in public transcriptomic datasets previously reported (Supplementary Figure 3A) (92). We next assessed transcriptomic profiles and observed clear distinction between hILCs from the febrile and convalescent phases, which were separated mainly by the expression of CD69 and interferon-stimulated genes (IFIT3, IFI44L, EGR1), similarly to previous reports on whole PBMC transcriptome analysis in DENV-infected patients (93, 94). GO term analysis showed enrichment in different modules in the febrile DF and DHF. In DF, pyruvate metabolic process, activation of JAK activity, positive regulation of TNF superfamily cytokine, and positive regulation of cytokine production were upregulated, suggesting the activation of cytokine pathways, consistent with a previous report on whole blood transcriptome (95). On the other hand, upregulated genes in DHF were functionally enriched for organophosphate and nucleotide/nucleoside biosynthetic processes as well as nuclear mRNA surveillance of mRNA 3’-end processes. These suggest that hILCs were metabolically active and increased their transcription and translation. The activation of the transcription pathway was also found in ILC3 in HSV infection (66). Because the GO term analysis is relatively broad and not specific to hILC biology, we next examined DEG by focusing on immune-related genes that were upregulated in the febrile phase when compared to matched convalescent samples, especially those that are different between the febrile DF and DHF samples. Surprisingly, only EGR1 was upregulated in both severities. EGR1 is known to regulate IL-2 and TNFα production (96–98). In flavivirus infection, EGR1 was found to be commonly upregulated in TEM and TEMRA in both DENV and Zika virus infection (99). Furthermore, upon in vitro activation of T cells with DENV peptides, both activated CD4+ (100) and CD8+ T cells (101) upregulated EGR1 expression. Thus, EGR1 likely regulates proinflammatory cytokine production in hILCs during the febrile phase of DENV infection.
Our DEG analysis also suggests that hILC responses may be more tightly regulated in DF while relatively less so in DHF. In DF, febrile phase hILCs upregulated type I IFN response genes together with the negative regulators IFI44L and HAVCR2 (Tim-3), suggestive of a “regulated response”. Interferon response gene, IFIT1, is known for its antiviral activity (102) while IFI44L is a feedback regulator of antiviral response (103). In human decidua, Tim-3 was found to be expressed in ILC3 and regulated IL-22, IL-8, and TNFα cytokine production important in maintaining feto-maternal tolerance (104). Tim-3, together with PD-1 and Tigit are considered check-point or inhibitory receptors on ILCs and NK (105). Quantification of Tim-3 expression by qPCR showed a similar trend of higher expression in DF but the difference did not reach statistical significance likely due to low number of samples. On the contrary, in the febrile phase of DHF samples, we observed upregulation of TRIM21, GZMB, IL15RA, and SLAMF7 indicating pro-inflammatory response and metabolic activation. TRIM21 was shown to respond to HCV and coxsackievirus B3 by interacting with MAVS (106). It sustains IRF3, thus positively regulates type I IFN antiviral response, and also activates proinflammatory cytokines TNFα, IL-6 (107, 108). Granzyme B is well known for its role in cytotoxic activity important in eradicating viral-infected cells (109). IL-15Rα, a receptor subunit for IL-15, is important for ILC development and activation especially in ILC1 (110). While SLAMF7 function has not been characterized in hILCs, SLAMF7 was found to express in ILC1 when we curated publicly available human circulating hILC RNA-seq data (92) (Supplementary Figure 3A). Interestingly, when we mapped the upregulated genes during the febrile phase of both DF and DHF, all were found to be relatively enriched in healthy ILC1 subset (Supplementary Figure 3B). This is consistent with the existing concept that ILC1 is the main ILC subset responding to viral infection. Taken together, hILCs likely participate in response to DENV through both cytokine-mediated response and cytotoxicity. Our data also suggest that a relatively loosely regulated activation of hILCs might be associated with severe DHF, while activation with regulated response may be associated with mild DF.
There are potential limitations of this study and future study is needed. First, we could not sort the hILC subsets separately for transcriptome analysis due to a limited number of hILCs in the samples. Future study using single-cell RNA sequencing could help elucidate the role of heterogeneous subsets of hILCs with higher resolution. In addition, future studies with a larger number of samples would be required to confirm our findings. Secondly, we could not detect hILC signature cytokine (IFN-γ, IL-4, IL-13, IL-17A) gene expression in RNA-seq data. This might be due to the low number of cells (150 cells per sample) as we observed a better, but not clear, expression of cytokine genes when we curated healthy hILC data from Li and colleagues (92) (Supplementary Figure 3A) which sorted 1,000 cells for RNA-seq experiment. In support of this hypothesis, another study with low number of cells also could not detect most of these genes in HIV infected hILCs data (74) (Supplementary Figure 4). Other possibilities include low cDNA conversion rate on these genes as well as insufficient sequencing depth. Nevertheless, intracellular cytokine staining and qPCR data helped to confirm that these cytokines were produced by hILCs during DENV infection. Finally, the cross-talks between hILCs and other cells are important aspects that are beyond the scope of the current study and warrant further investigations.
In summary, we provide the first evidence of hILC activation in human DENV infection and their distinct cytokine production and transcriptional profiles in the febrile phase of DF and DHF. While hILCs likely participate in antiviral defense against DENV infection, an uncontrolled response may be pathogenic and affect disease severity. Further investigations into the differential responses of hILCs in DENV infection will help us to better understand the protective response in DF and the pathogenic response in severe DHF. These could form the foundation for future applications, such as targeting pathogenic hILCs early in innate immune response to prevent disease progression to severe form, or harnessing hILCs to achieve optimally regulated immune response in vaccination strategy (111).
DENFREE Thailand: Anavaj Sakuntabhai (Functional Genetics of Infectious Diseases Unit, Institut Pasteur, Paris, France, Centre National de la Recherche Scientifique (CNRS), UMR2000, Paris, France), Pratap Singhasivanon (Department of Tropical Hygiene, Faculty of Tropical Medicine, Mahidol University, Bangkok, Thailand), Swangjit Suraamornkul (Endocrinology Division, Department of Medicine, Faculty of Medicine Vajira Hospital, Navamindradhiraj University, Bangkok, Thailand), Tawatchai Yingtaweesak (Thasongyang Hospital, Tak, Thailand), Khajohnpong Manopwisedjaroen (Department of Microbiology, Faculty of Science, Mahidol University, Bangkok, Thailand).
RNA-seq data discussed in this publication have been deposited in NCBI's Gene Expression Omnibus and are accessible through GEO Series accession number GSE155672. All RNA-seq analyses and visualizations were performed in R version 3.6. Computing code is available at github repository: https://github.com/vclabsysbio/hILCs_DENV.
The studies involving human participants were reviewed and approved by Institutional Review Board of Faculty of Medicine Vajira Hospital (No.015/12), Faculty of Tropical Medicine Mahidol University (TMEC 13-041), and Faculty of Medicine, Ramathibodi hospital (COA.MURA2019/603). Written informed consent to participate in this study was provided by the participants’ legal guardian/next of kin.
PM conceptualized and designed the experiments. DENFREE Thailand provided clinical samples. PM and FL supervised wet lab experiments. TP performed the experiments with WC and AO support. TP performed bioinformatic analyses. VC and PM supervised bioinformatic analyses. PM, OM, VC, and TP analyzed and interpreted data. PM, OM, and TP wrote the manuscript. All authors contributed to the article and approved the submitted version.
This work was supported by European Union Seventh Framework Program (EU/FP7 under Grant Agreement #282378 (DENFREE) to PM and DENFREE Thailand. The PM lab was supported by Anandamahidol foundation, Thailand Research Fund Grant for New Scholar (TRG5880121) and Royal Society-Newton Mobility Grant (NI170094) through the Office of Higher Education (Thailand) and the Royal Society (UK). The VC lab was supported by the Newton Advanced Fellowship through Thailand Research Fund (DBG60800003) and Royal Society (NA160153), and the Thailand Research Fund Grant for New Scholar (MRG6080235). TP was supported by the Scholarship for Young Scientists (2017), Faculty of Science, Mahidol University. This research project was supported by Mahidol University (Basic Research Fund: fiscal year 2021). WC was supported by The Royal Golden Jubilee (RGJ) Ph.D. Program Scholarship (Grant No. PHD/0115/2558) from Thailand Science Research and Innovation (TSRI).
The authors declare that the research was conducted in the absence of any commercial or financial relationships that could be construed as a potential conflict of interest.
The authors are grateful to Dr. Bunpote Siridechadilok and the National Center for Genetic Engineering and Biotechnology (BIOTEC) for the FACSAria III instrument. We are also grateful for Dr. Thaneeya Duangchinda for her assistance with cytometer operation and cell sorting. We thank Walairat Thuncharoen for her help as a lab manager, Nada Pitabut and the nursing team for DENFREE cohort management and patient recruitment/care, Kawinnat Sue-ob, Pakkanan Chansongkrow, and Napaporn Sriden for initial support on RNA-seq analysis. We thank Ataco (Chindasook Group, Thailand) and the central instrument facility (CIF), Faculty of Science, Mahidol University for instrumental support. High-Performance Computing was supported by Integrative Computational BioScience Center (ICBS), Mahidol University.
The Supplementary Material for this article can be found online at: https://www.frontiersin.org/articles/10.3389/fimmu.2021.599805/full#supplementary-material
1. Bhatt S, Gething PW, Brady OJ, Messina JP, Farlow AW, Moyes CL, et al. The Global Distribution and Burden of Dengue. Nature (2013) 496:504–7. doi: 10.1038/nature12060
2. World Health Organization. Dengue Haemorrhagic Fever: Diagnosis, Treatment, Prevention and Control. Geneva: World Health Organization (1997).
3. World Health Organization. GACVS Statement on Dengvaxia ® (Cyd-Tdv) (2017). Available at: https://www.who.int/vaccine_safety/committee/GACVS-StatementonDengvaxia-CYD-TDV/en/ (Accessed May 15, 2020).
4. Halstead SB. Safety Issues From a Phase 3 Clinical Trial of a Live-Attenuated Chimeric Yellow Fever Tetravalent Dengue Vaccine. Hum Vaccines Immunother (2018) 14:2158–62. doi: 10.1080/21645515.2018.1445448
5. Sridhar S, Luedtke A, Langevin E, Zhu M, Bonaparte M, Machabert T, et al. Effect of Dengue Serostatus on Dengue Vaccine Safety and Efficacy. N Engl J Med (2018) 379:327–40. doi: 10.1056/NEJMoa1800820
6. Screaton G, Mongkolsapaya J, Yacoub S, Roberts C. New Insights Into the Immunopathology and Control of Dengue Virus Infection. Nat Rev Immunol (2015) 15:745–59. doi: 10.1038/nri3916
7. Murphy BR, Whitehead SS. Immune Response to Dengue Virus and Prospects for a Vaccine. Annu Rev Immunol (2011) 29:587–619. doi: 10.1146/annurev-immunol-031210-101315
8. St. John AL, Rathore APS. Adaptive Immune Responses to Primary and Secondary Dengue Virus Infections. Nat Rev Immunol (2019) 19:218–30. doi: 10.1038/s41577-019-0123-x
9. Rothman AL. Immunity to Dengue Virus: A Tale of Original Antigenic Sin and Tropical Cytokine Storms. Nat Rev Immunol (2011) 11:532–43. doi: 10.1038/nri3014
10. Mangada MM, Rothman AL. Altered Cytokine Responses of Dengue-Specific CD4+ T Cells to Heterologous Serotypes. J Immunol (2005) 175:2676–83. doi: 10.4049/jimmunol.175.4.2676
11. Sangkawibha N, Rojanasuphot S, Ahandrik S, Viriyapongse S, Jatanasen S, Salitul V, et al. Risk Factors in Dengue Shock Syndrome: A Prospective Epidemiologic Study in Rayong, Thailand. Am J Epidemiol (1984) 120:653–69. doi: 10.1093/oxfordjournals.aje.a113932
12. Mongkolsapaya J, Dejnirattisai W, Xu X, Vasanawathana S, Tangthawornchaikul N, Chairunsri A, et al. Original Antigenic Sin and Apoptosis in the Pathogenesis of Dengue Hemorrhagic Fever. Nat Med (2003) 9:921–7. doi: 10.1038/nm887
13. Halstead SB, Rojanasuphot S, Sangkawibha N. Original Antigenic Sin in Dengue. Am J Trop Med Hyg (1983) 32:154–6. doi: 10.4269/ajtmh.1983.32.154
14. Guzman MG, Alvarez M, Halstead SB. Secondary Infection as a Risk Factor for Dengue Hemorrhagic Fever/Dengue Shock Syndrome: An Historical Perspective and Role of Antibody-Dependent Enhancement of Infection. Arch Virol (2013) 158:1445–59. doi: 10.1007/s00705-013-1645-3
15. Chan KR, Ong EZ, Tan HC, Zhang SL-X, Zhang Q, Tang KF, et al. Leukocyte Immunoglobulin-Like Receptor B1 is Critical for Antibody-Dependent Dengue. Proc Natl Acad Sci (2014) 111:2722–7. doi: 10.1073/pnas.1317454111
16. King CA, Wegman AD, Endy TP. Mobilization and Activation of the Innate Immune Response to Dengue Virus. Front Cell Infect Microbiol (2020) 10:574417. doi: doi: 10.3389/fcimb.2020.574417
17. Kamaladasa A, Wickramasinghe N, Adikari TN, Gomes L, Shyamali NLA, Salio M, et al. Expansion of Highly Activated Invariant Natural Killer T Cells With Altered Phenotype in Acute Dengue Infection: Expansion of iNKT Cells. Clin Exp Immunol (2016) 185:228–38. doi: 10.1111/cei.12778
18. St. John AL, Rathore APS, Yap H, Ng M-L, Metcalfe DD, Vasudevan SG, et al. Immune Surveillance by Mast Cells During Dengue Infection Promotes Natural Killer (NK) and NKT-cell Recruitment and Viral Clearance. Proc Natl Acad Sci (2011) 108:9190–5. doi: 10.1073/pnas.1105079108
19. Matangkasombut P, Chan-in W, Opasawaschai A, Pongchaikul P, Tangthawornchaikul N, Vasanawathana S, et al. Invariant NKT Cell Response to Dengue Virus Infection in Human. PloS Negl Trop Dis (2014) 8:e2955. doi: 10.1371/journal.pntd.0002955
20. Paquin-Proulx D, Avelino-Silva VI, Santos BAN, Silveira Barsotti N, Siroma F, Fernandes Ramos J, et al. MAIT Cells are Activated in Acute Dengue Virus Infection and After In Vitro Zika Virus Infection. PloS Negl Trop Dis (2018) 12:e0006154. doi: 10.1371/journal.pntd.0006154
21. STOP-HCV consortium, van Wilgenburg B, Scherwitzl I, Hutchinson EC, Leng T, Kurioka A, et al. MAIT Cells are Activated During Human Viral Infections. Nat Commun (2016) 7:11653. doi: 10.1038/ncomms11653
22. Kao Y-T, Lai MMC, Yu C-Y. How Dengue Virus Circumvents Innate Immunity. Front Immunol (2018) 9:2860. doi: 10.3389/fimmu.2018.02860
23. Uno N, Ross TM. Dengue Virus and the Host Innate Immune Response. Emerg Microbes Infect (2018) 7:1–11. doi: 10.1038/s41426-018-0168-0
24. Green AM, Beatty PR, Hadjilaou A, Harris E. Innate Immunity to Dengue Virus Infection and Subversion of Antiviral Responses. J Mol Biol (2014) 426:1148–60. doi: 10.1016/j.jmb.2013.11.023
25. Schmid MA, Diamond MS, Harris E. Dendritic Cells in Dengue Virus Infection: Targets of Virus Replication and Mediators of Immunity. Front Immunol (2014) 5:647. doi: 10.3389/fimmu.2014.00647
26. Avirutnan P, Matangkasombut P. Unmasking the Role of Mast Cells in Dengue. eLife (2013) 2:e00767. doi: 10.7554/eLife.00767
27. Zimmer CL, Cornillet M, Solà-Riera C, Cheung K-W, Ivarsson MA, Lim MQ, et al. NK Cells are Activated and Primed for Skin-Homing During Acute Dengue Virus Infection in Humans. Nat Commun (2019) 10:3897. doi: 10.1038/s41467-019-11878-3
28. Spits H, Di Santo JP. The Expanding Family of Innate Lymphoid Cells: Regulators and Effectors of Immunity and Tissue Remodeling. Nat Immunol (2011) 12:21–7. doi: 10.1038/ni.1962
29. Spits H, Artis D, Colonna M, Diefenbach A, Di Santo JP, Eberl G, et al. Innate Lymphoid Cells — a Proposal for Uniform Nomenclature. Nat Rev Immunol (2013) 13:145–9. doi: 10.1038/nri3365
30. Spits H, Cupedo T. Innate Lymphoid Cells: Emerging Insights in Development, Lineage Relationships, and Function. Annu Rev Immunol (2012) 30:647–75. doi: 10.1146/annurev-immunol-020711-075053
31. Hazenberg MD, Spits H. Human Innate Lymphoid Cells. Blood (2014) 124:700–9. doi: 10.1182/blood-2013-11-427781
32. Artis D, Spits H. The Biology of Innate Lymphoid Cells. Nature (2015) 517:293–301. doi: 10.1038/nature14189
33. Eberl G, Colonna M, Di Santo JP, McKenzie ANJ. Innate Lymphoid Cells: A New Paradigm in Immunology. Science (2015) 348:aaa6566–aaa6566. doi: 10.1126/science.aaa6566
34. Vivier E, Artis D, Colonna M, Diefenbach A, Di Santo JP, Eberl G, et al. Innate Lymphoid Cells: 10 Years on. Cell (2018) 174:1054–66. doi: 10.1016/j.cell.2018.07.017
35. Ebihara T. Dichotomous Regulation of Acquired Immunity by Innate Lymphoid Cells. Cells (2020) 9:1193. doi: 10.3390/cells9051193
36. Parodi M, Favoreel H, Candiano G, Gaggero S, Sivori S, Mingari MC, et al. Nkp44-Nkp44 Ligand Interactions in the Regulation of Natural Killer Cells and Other Innate Lymphoid Cells in Humans. Front Immunol (2019) 10:719. doi: 10.3389/fimmu.2019.00719
37. Rahman MA, Ko E-J, Enyindah-Asonye G, Helmold Hait S, Hogge C, Hunegnaw R, et al. Differential Effect of Mucosal NKp44+ Innate Lymphoid Cells and Δγ Cells on Simian Immunodeficiency Virus Infection Outcome in Rhesus Macaques. J Immunol (2019) 203:2459–71. doi: 10.4049/jimmunol.1900572
38. Bal SM, Golebski K, Spits H. Plasticity of Innate Lymphoid Cell Subsets. Nat Rev Immunol (2020) 20:552–65. doi: 10.1038/s41577-020-0282-9
39. Morita H, Moro K, Koyasu S. Innate Lymphoid Cells in Allergic and Nonallergic Inflammation. J Allergy Clin Immunol (2016) 138:1253–64. doi: 10.1016/j.jaci.2016.09.011
40. Stier MT, Peebles RS. Innate Lymphoid Cells and Allergic Disease. Ann Allergy Asthma Immunol (2017) 119:480–8. doi: 10.1016/j.anai.2017.08.290
41. Helfrich S, Mindt BC, Fritz JH, Duerr CU. Group 2 Innate Lymphoid Cells in Respiratory Allergic Inflammation. Front Immunol (2019) 10:930. doi: 10.3389/fimmu.2019.00930
42. Shikhagaie MM, Germar K, Bal SM, Ros XR, Spits H. Innate Lymphoid Cells in Autoimmunity: Emerging Regulators in Rheumatic Diseases. Nat Rev Rheumatol (2017) 13:164–73. doi: 10.1038/nrrheum.2016.218
43. Xiong T, Turner J-E. Innate Lymphoid Cells in Autoimmunity and Chronic Inflammatory Diseases. Semin Immunopathol (2018) 40:393–406. doi: 10.1007/s00281-018-0670-4
44. Zhou S, Li Q, Wu H, Lu Q. The Pathogenic Role of Innate Lymphoid Cells in Autoimmune-Related and Inflammatory Skin Diseases. Cell Mol Immunol (2020) 17:335–46. doi: 10.1038/s41423-020-0399-6
45. Vallentin B, Barlogis V, Piperoglou C, Cypowyj S, Zucchini N, Chene M, et al. Innate Lymphoid Cells in Cancer. Cancer Immunol Res (2015) 3:1109–14. doi: 10.1158/2326-6066.CIR-15-0222
46. Bruchard M, Ghiringhelli F. Deciphering the Roles of Innate Lymphoid Cells in Cancer. Front Immunol (2019) 10:656. doi: 10.3389/fimmu.2019.00656
47. An Z, Flores-Borja F, Irshad S, Deng J, Ng T. Pleiotropic Role and Bidirectional Immunomodulation of Innate Lymphoid Cells in Cancer. Front Immunol (2020) 10:3111. doi: 10.3389/fimmu.2019.03111
48. Wagner M, Koyasu S. Cancer Immunoediting by Innate Lymphoid Cells. Trends Immunol (2019) 40:415–30. doi: 10.1016/j.it.2019.03.004
49. Cai T, Qiu J, Ji Y, Li W, Ding Z, Suo C, et al. IL-17–Producing ST2+ Group 2 Innate Lymphoid Cells Play a Pathogenic Role in Lung Inflammation. J Allergy Clin Immunol (2019) 143:229–44.e9. doi: 10.1016/j.jaci.2018.03.007
50. Silver JS, Kearley J, Copenhaver AM, Sanden C, Mori M, Yu L, et al. Inflammatory Triggers Associated With Exacerbations of COPD Orchestrate Plasticity of Group 2 Innate Lymphoid Cells in the Lungs. Nat Immunol (2016) 17:626–35. doi: 10.1038/ni.3443
51. Carvelli J, Piperoglou C, Bourenne J, Farnarier C, Banzet N, Demerlé C, et al. Imbalance of Circulating Innate Lymphoid Cell Subpopulations in Patients With Septic Shock. Front Immunol (2019) 10:2179. doi: 10.3389/fimmu.2019.02179
52. Satoh-Takayama N, Kato T, Motomura Y, Kageyama T, Taguchi-Atarashi N, Kinoshita-Daitoku R, et al. Bacteria-Induced Group 2 Innate Lymphoid Cells in the Stomach Provide Immune Protection Through Induction of Iga. Immunity (2020) 52:635–649.e4. doi: 10.1016/j.immuni.2020.03.002
53. Löser S, Smith KA, Maizels RM. Innate Lymphoid Cells in Helminth Infections—Obligatory or Accessory? Front Immunol (2019) 10:620. doi: 10.3389/fimmu.2019.00620
54. Gladiator A, Wangler N, Trautwein-Weidner K, LeibundGut-Landmann S. Cutting Edge: Il-17–Secreting Innate Lymphoid Cells are Essential for Host Defense Against Fungal Infection. J Immunol (2013) 190:521–5. doi: 10.4049/jimmunol.1202924
55. Panda SK, Colonna M. Innate Lymphoid Cells in Mucosal Immunity. Front Immunol (2019) 10:861. doi: 10.3389/fimmu.2019.00861
56. Monticelli LA, Sonnenberg GF, Abt MC, Alenghat T, Ziegler CGK, Doering TA, et al. Innate Lymphoid Cells Promote Lung-Tissue Homeostasis After Infection With Influenza Virus. Nat Immunol (2011) 12:1045–54. doi: 10.1038/ni.2131
57. Gorski SA, Hahn YS, Braciale TJ. Group 2 Innate Lymphoid Cell Production of IL-5 is Regulated by NKT Cells During Influenza Virus Infection. PloS Pathog (2013) 9:e1003615. doi: 10.1371/journal.ppat.1003615
58. Duerr CU, McCarthy CDA, Mindt BC, Rubio M, Meli AP, Pothlichet J, et al. Type I Interferon Restricts Type 2 Immunopathology Through the Regulation of Group 2 Innate Lymphoid Cells. Nat Immunol (2016) 17:65–75. doi: 10.1038/ni.3308
59. Vashist N, Trittel S, Ebensen T, Chambers BJ, Guzmán CA, Riese P. Influenza-Activated ILC1s Contribute to Antiviral Immunity Partially Influenced by Differential Gitr Expression. Front Immunol (2018) 9:505. doi: 10.3389/fimmu.2018.00505
60. Li BWS, de Bruijn MJW, Lukkes M, van Nimwegen M, Bergen IM, KleinJan A, et al. T Cells and ILC2s are Major Effector Cells in Influenza-Induced Exacerbation of Allergic Airway Inflammation in Mice. Eur J Immunol (2019) 49:144–56. doi: 10.1002/eji.201747421
61. Saravia J, You D, Shrestha B, Jaligama S, Siefker D, Lee GI, et al. Respiratory Syncytial Virus Disease is Mediated by Age-Variable Il-33. PloS Pathog (2015) 11:e1005217. doi: 10.1371/journal.ppat.1005217
62. Stier MT, Bloodworth MH, Toki S, Newcomb DC, Goleniewska K, Boyd KL, et al. Respiratory Syncytial Virus Infection Activates IL-13–producing Group 2 Innate Lymphoid Cells Through Thymic Stromal Lymphopoietin. J Allergy Clin Immunol (2016) 138:814–24.e11. doi: 10.1016/j.jaci.2016.01.050
63. Stier MT, Goleniewska K, Cephus JY, Newcomb DC, Sherrill TP, Boyd KL, et al. Stat1 Represses Cytokine-Producing Group 2 and Group 3 Innate Lymphoid Cells During Viral Infection. J Immunol (2017) 199:510–9. doi: 10.4049/jimmunol.1601984
64. Hong JY, Bentley JK, Chung Y, Lei J, Steenrod JM, Chen Q, et al. Neonatal Rhinovirus Induces Mucous Metaplasia and Airways Hyperresponsiveness Through IL-25 and Type 2 Innate Lymphoid Cells. J Allergy Clin Immunol (2014) 134:429–439.e8. doi: 10.1016/j.jaci.2014.04.020
65. Han M, Hong JY, Jaipalli S, Rajput C, Lei J, Hinde JL, et al. Ifn-γ Blocks Development of an Asthma Phenotype in Rhinovirus-infected Baby Mice by Inhibiting Ilc2s. Am J Respir Cell Mol Biol (2016) 56:242–51. doi: 10.1165/rcmb.2016-0056OC
66. Hirose S, Wang S, Tormanen K, Wang Y, Tang J, Akbari O, et al. Roles of Type 1, 2, and 3 Innate Lymphoid Cells in Herpes Simplex Virus 1 Infection in Vitro and In Vivo. J Virol (2019) 93:e00523–19. doi: 10.1128/JVI.00523-19
67. Hernández PP, Mahlakõiv T, Yang I, Schwierzeck V, Nguyen N, Guendel F, et al. Interferon-λ and Interleukin 22 Act Synergistically for the Induction of Interferon-Stimulated Genes and Control of Rotavirus Infection. Nat Immunol (2015) 16:698–707. doi: 10.1038/ni.3180
68. Weizman O-E, Adams NM, Schuster IS, Krishna C, Pritykin Y, Lau C, et al. ILC1 Confer Early Host Protection at Initial Sites of Viral Infection. Cell (2017) 171:795–808.e12. doi: 10.1016/j.cell.2017.09.052
69. Weizman O-E, Song E, Adams NM, Hildreth AD, Riggan L, Krishna C, et al. Mouse Cytomegalovirus-Experienced ILC1s Acquire a Memory Response Dependent on the Viral Glycoprotein M12. Nat Immunol (2019) 20:1004–11. doi: 10.1038/s41590-019-0430-1
70. Picarda G, Ghosh R, McDonald B, Verma S, Thiault N, El Morabiti R, et al. Cytomegalovirus Evades Trail-Mediated Innate Lymphoid Cell 1 Defenses. J Virol (2019) 93:e00617–19. doi: 10.1128/JVI.00617-19
71. Guia S, Narni-Mancinelli E. Helper-Like Innate Lymphoid Cells in Humans and Mice. Trends Immunol (2020) 41:436–52. doi: 10.1016/j.it.2020.03.002
72. Jurak LM, Xi Y, Landgraf M, Carroll ML, Murray L, Upham JW. Interleukin 33 Selectively Augments Rhinovirus-Induced Type 2 Immune Responses in Asthmatic But Not Healthy People. Front Immunol (2018) 9:1895. doi: 10.3389/fimmu.2018.01895
73. Ising R, Weinhold S, Bennstein SB, Zimmermann A, Degistirici Ö, Kögler G, et al. HCMV Infection in a Mesenchymal Stem Cell Niche: Differential Impact on the Development of NK Cells Versus ILC3. J Clin Med (2019) 9:10. doi: 10.3390/jcm9010010
74. Kløverpris HN, Kazer SW, Mjösberg J, Mabuka JM, Wellmann A, Ndhlovu Z, et al. Innate Lymphoid Cells Are Depleted Irreversibly During Acute HIV-1 Infection in the Absence of Viral Suppression. Immunity (2016) 44:391–405. doi: 10.1016/j.immuni.2016.01.006
75. Dengue research Framework for Resisting Epidemics in Europe. Denfree Project | FP7 | CORDIS | European Commission. Available at: https://cordis.europa.eu/project/id/282378 (Accessed August 22, 2020).
76. Matangkasombut P, Manopwisedjaroen K, Pitabut N, Thaloengsok S, Suraamornkul S, Yingtaweesak T, et al. Dengue Viremia Kinetics in Asymptomatic and Symptomatic Infection. Int J Infect Dis (2020) 101:90–7. doi: 10.1016/j.ijid.2020.09.1446
77. Lanciotti RS, Calisher CH, Gubler DJ, Chang GJ, Vorndam AV. Rapid Detection and Typing of Dengue Viruses From Clinical Samples by Using Reverse Transcriptase-Polymerase Chain Reaction. J Clin Microbiol (1992) 30:545–51. doi: 10.1128/JCM.30.3.545-551.1992
78. Duong V, Lambrechts L, Paul RE, Ly S, Lay RS, Long KC, et al. Asymptomatic Humans Transmit Dengue Virus to Mosquitoes. Proc Natl Acad Sci U.S.A. (2015) 112:14688–93. doi: 10.1073/pnas.1508114112
79. Picelli S, Faridani OR, Björklund ÅK, Winberg G, Sagasser S, Sandberg R. Full-Length RNA-seq From Single Cells Using Smart-Seq2. Nat Protoc (2014) 9:171–81. doi: 10.1038/nprot.2014.006
80. Bolger AM, Lohse M, Usadel B. Trimmomatic: A Flexible Trimmer for Illumina Sequence Data. Bioinformatics (2014) 30:2114–20. doi: 10.1093/bioinformatics/btu170
81. Kim D, Langmead B, Salzberg SL. HISAT: A Fast Spliced Aligner With Low Memory Requirements. Nat Methods (2015) 12:357–60. doi: 10.1038/nmeth.3317
82. Pertea M, Pertea GM, Antonescu CM, Chang T-C, Mendell JT, Salzberg SL. StringTie Enables Improved Reconstruction of a Transcriptome From RNA-seq Reads. Nat Biotechnol (2015) 33:290–5. doi: 10.1038/nbt.3122
83. Anders S, Pyl PT, Huber W. Htseq—a Python Framework to Work With High-Throughput Sequencing Data. Bioinformatics (2015) 31:166–9. doi: 10.1093/bioinformatics/btu638
84. Love MI, Huber W, Anders S. Moderated Estimation of Fold Change and Dispersion for RNA-seq Data With Deseq2. Genome Biol (2014) 15:550. doi: 10.1186/s13059-014-0550-8
85. Zhu A, Ibrahim JG, Love MI. Heavy-Tailed Prior Distributions for Sequence Count Data: Removing the Noise and Preserving Large Differences. Bioinformatics (2019) 35:2084–92. doi: 10.1093/bioinformatics/bty895
86. Reimand J, Kull M, Peterson H, Hansen J, Vilo J. G:Profiler—a Web-Based Toolset for Functional Profiling of Gene Lists From Large-Scale Experiments. Nucleic Acids Res (2007) 35:W193–200. doi: 10.1093/nar/gkm226
87. Gu Z, Eils R, Schlesner M. Complex Heatmaps Reveal Patterns and Correlations in Multidimensional Genomic Data. Bioinformatics (2016) 32:2847–9. doi: 10.1093/bioinformatics/btw313
88. Xu H, Wang X, Liu DX, Moroney-Rasmussen T, Lackner AA, Veazey RS. Il-17-producing Innate Lymphoid Cells are Restricted to Mucosal Tissues and are Depleted in SIV-infected Macaques. Mucosal Immunol (2012) 5:658–69. doi: 10.1038/mi.2012.39
89. Bozza FA, Cruz OG, Zagne SMO, Azeredo EL, Nogueira RMR, Assis EF, et al. Multiplex Cytokine Profile From Dengue Patients: MIP-1beta and IFN-gamma as Predictive Factors for Severity. BMC Infect Dis (2008) 8:86. doi: 10.1186/1471-2334-8-86
90. Duangchinda T, Dejnirattisai W, Vasanawathana S, Limpitikul W, Tangthawornchaikul N, Malasit P, et al. Immunodominant T-cell Responses to Dengue Virus NS3 are Associated With DHF. Proc Natl Acad Sci U S A (2010) 107:16922–7. doi: 10.1073/pnas.1010867107
91. Sonnenberg GF, Hepworth MR. Functional Interactions Between InnateLymphoid Cells and Adaptive Immunity. Nat Rev Immunol (2019) 19:599–613. doi: 10.1038/s41577-019-0194-8
92. Li S, Morita H, Sokolowska M, Tan G, Boonpiyathad T, Opitz L, et al. Gene Expression Signatures of Circulating Human Type 1, 2, and 3 Innate Lymphoid Cells. J Allergy Clin Immunol (2019) 143:2321–5. doi: 10.1016/j.jaci.2019.01.047
93. Ubol S, Masrinoul P, Chaijaruwanich J, Kalayanarooj S, Charoensirisuthikul T, Kasisith J. Differences in Global Gene Expression in Peripheral Blood Mononuclear Cells Indicate a Significant Role of the Innate Responses in Progression of Dengue Fever But Not Dengue Hemorrhagic Fever. J Infect Dis (2008) 197:1459–67. doi: 10.1086/587699
94. Sun P, García J, Comach G, Vahey MT, Wang Z, Forshey BM, et al. Sequential Waves of Gene Expression in Patients With Clinically Defined Dengue Illnesses Reveal Subtle Disease Phases and Predict Disease Severity. PloS Negl Trop Dis (2013) 7:e2298. doi: 10.1371/journal.pntd.0002298
95. Hoang LT, Lynn DJ, Henn M, Birren BW, Lennon NJ, Le PT, et al. The Early Whole-Blood Transcriptional Signature of Dengue Virus and Features Associated With Progression to Dengue Shock Syndrome in Vietnamese Children and Young Adults. J Virol (2010) 84:12982–94. doi: 10.1128/JVI.01224-10
96. McMahon SB, Monroe JG. The Role of Early Growth Response Gene 1 (egr-1) in Regulation of the Immune Response. J Leukoc Biol (1996) 60:159–66. doi: 10.1002/jlb.60.2.159
97. Shin H-J, Lee J-B, Park S-H, Chang J, Lee C-W. T-Bet Expression is Regulated by EGR1-mediated Signaling in Activated T Cells. Clin Immunol (2009) 131:385–94. doi: 10.1016/j.clim.2009.02.009
98. Cubero FJ, Nieto N. Arachidonic Acid Stimulates Tnfα Production in Kupffer Cells Via a Reactive Oxygen species-pERK1/2-Egr1-dependent Mechanism. Am J Physiol-Gastrointest Liver Physiol (2012) 303:G228–39. doi: 10.1152/ajpgi.00465.2011
99. Grifoni A, Tian Y, Sette A, Weiskopf D. Transcriptomic Immune Profiles of Human Flavivirus-Specific T-cell Responses. Immunology (2020) 160:3–9. doi: 10.1111/imm.13161
100. Tian Y, Seumois G, De-Oliveira-Pinto LM, Mateus J, Herrera-de la Mata S, Kim C, et al. Molecular Signatures of Dengue Virus-Specific IL-10/IFN-γ Co-producing Cd4 T Cells and Their Association With Dengue Disease. Cell Rep (2019) 29:4482–95. doi: 10.1016/j.celrep.2019.11.098
101. Tian Y, Babor M, Lane J, Seumois G, Liang S, Goonawardhana NDS, et al. Dengue-Specific CD8+ T Cell Subsets Display Specialized Transcriptomic and TCR Profiles. J Clin Invest (2019) 129:1727–41. doi: 10.1172/JCI123726
102. Fensterl V, Sen GC. Interferon-Induced Ifit Proteins: Their Role in Viral Pathogenesis. J Virol (2015) 89:2462–8. doi: 10.1128/JVI.02744-14
103. DeDiego ML, Martinez-Sobrido L, Topham DJ. Novel Functions of IFI44L as a Feedback Regulator of Host Antiviral Responses. J Virol (2019) 93:e01159–19. doi: 10.1128/JVI.01159-19
104. Vacca P, Pesce S, Greppi M, Fulcheri E, Munari E, Olive D, et al. PD-1 is Expressed by and Regulates Human Group 3 Innate Lymphoid Cells in Human Decidua. Mucosal Immunol (2019) 12:624–31. doi: 10.1038/s41385-019-0141-9
105. Mariotti FR, Quatrini L, Munari E, Vacca P, Moretta L. Innate Lymphoid Cells: Expression of PD-1 and Other Checkpoints in Normal and Pathological Conditions. Front Immunol (2019) 10:910. doi: 10.3389/fimmu.2019.00910
106. Liu H, Li M, Song Y, Xu W. Trim21 Restricts Coxsackievirus B3 Replication, Cardiac and Pancreatic Injury Via Interacting With MAVS and Positively Regulating IRF3-Mediated Type-I Interferon Production. Front Immunol (2018) 9:2479. doi: 10.3389/fimmu.2018.02479
107. Yang K, Shi H-X, Liu X-Y, Shan Y-F, Wei B, Chen S, et al. Trim21 Is Essential to Sustain IFN Regulatory Factor 3 Activation During Antiviral Response. J Immunol (2009) 182:3782–92. doi: 10.4049/jimmunol.0803126
108. Watkinson RE, Tam JCH, Vaysburd MJ, James LC. Simultaneous Neutralization and Innate Immune Detection of a Replicating Virus by TRIM21. J Virol (2013) 87:7309–13. doi: 10.1128/JVI.00647-13
109. Afonina IS, Cullen SP, Martin SJ. Cytotoxic and non-Cytotoxic Roles of the CTL/NK Protease Granzyme B: Granzyme B in Apoptosis and Inflammation. Immunol Rev (2010) 235:105–16. doi: 10.1111/j.0105-2896.2010.00908.x
110. Klose CSN, Flach M, Möhle L, Rogell L, Hoyler T, Ebert K, et al. Differentiation of Type 1 ILCs From a Common Progenitor to All Helper-Like Innate Lymphoid Cell Lineages. Cell (2014) 157:340–56. doi: 10.1016/j.cell.2014.03.030
Keywords: Dengue, viral infection, innate lymphoid cells, ILCs, immune response to dengue, innate immunity, RNA-seq, transcriptome
Citation: Poonpanichakul T, Chan-In W, Opasawatchai A, Loison F, Matangkasombut O, Charoensawan V, Matangkasombut P and DENFREE Thailand (2021) Innate Lymphoid Cells Activation and Transcriptomic Changes in Response to Human Dengue Infection. Front. Immunol. 12:599805. doi: 10.3389/fimmu.2021.599805
Received: 28 August 2020; Accepted: 29 April 2021;
Published: 17 May 2021.
Edited by:
Cyril Seillet, Walter and Eliza Hall Institute of Medical Research, AustraliaReviewed by:
John Hiscott, Istituto Pasteur Italia Cenci Bolognetti Foundation, ItalyCopyright © 2021 Poonpanichakul, Chan-In, Opasawatchai, Loison, Matangkasombut, Charoensawan, Matangkasombut and DENFREE Thailand. This is an open-access article distributed under the terms of the Creative Commons Attribution License (CC BY). The use, distribution or reproduction in other forums is permitted, provided the original author(s) and the copyright owner(s) are credited and that the original publication in this journal is cited, in accordance with accepted academic practice. No use, distribution or reproduction is permitted which does not comply with these terms.
*Correspondence: Ponpan Matangkasombut, cG9ucGFuLm1hdEBtYWhpZG9sLmVkdQ==
Disclaimer: All claims expressed in this article are solely those of the authors and do not necessarily represent those of their affiliated organizations, or those of the publisher, the editors and the reviewers. Any product that may be evaluated in this article or claim that may be made by its manufacturer is not guaranteed or endorsed by the publisher.
Research integrity at Frontiers
Learn more about the work of our research integrity team to safeguard the quality of each article we publish.