- 1Department of Rheumatology and Clinical Immunology, University Hospital Schleswig-Holstein—Campus Lübeck, Lübeck, Germany
- 2Institute of Clinical Molecular Biology, Christian-Albrechts-University Kiel, Kiel, Germany
- 3Lübeck Institute of Experimental Dermatology (LIED), University of Lübeck, Lübeck, Germany
- 4Sorbonne Université, INSERM, Immunology-Immunopathology-Immunotherapy (i3), Paris, France
- 5Institute of Immunology, Christian-Albrechts-University Kiel, Kiel, Germany
Immunophenotyping on the molecular and cellular level is a central aspect for characterization of patients with inflammatory diseases, both to better understand disease etiopathogenesis and based on this to develop diagnostic and prognostic biomarkers which allow patient stratification and tailor-made treatment strategies. Technology-driven developments have considerably expanded the range of analysis tools. Especially the analysis of adaptive immune responses, often regarded as central though mostly poorly characterized disease drivers, is a major focus of personalized medicine. The identification of the disease-relevant antigens and characterization of corresponding antigen-specific lymphocytes in individual patients benefits significantly from recent developments in cytometry by sequencing and proteomics. The aim of this workshop was to identify the important developments for state-of-the-art immunophenotyping for clinical application and precision medicine. We focused here on recent key developments in analysis of antigen-specific lymphocytes, sequencing, and proteomics approaches, their relevance in precision medicine and the discussion of the major challenges and opportunities for the future.
Introduction
Immunophenotyping is a key method for monitoring and diagnosing of immunological diseases, such as infectious diseases, chronic inflammatory and autoimmune diseases and allergy. For many of these the actual disease cause is still elusive or as in the case of COVID-19 the origin and contribution of potentially pathogenic immune reactions to severe disease. Classically immune monitoring is accomplished via measurement of serum biomarkers, including acute phase proteins, serum cytokines, or antigen-specific serum antibodies. However, a deeper understanding of the underlying disease cause and the pathogenic mechanisms, requires the identification and characterization of the responsible immune cells and their interaction partners. Most of these diseases are restricted to specific organs or antigens and there is plenty of, though mostly indirect, evidence that antigen-specific lymphocytes are the pathogenic drivers of disease. However, due to technological restrictions these cells are barely characterized and in many inflammatory and autoimmune diseases the target antigens of the pathogenic immune reaction are still unknown. This is a major roadblock for development of personalized medicine, that is disease- and patient-specific diagnostics and therapies targeting the disease cause rather than the symptoms.
Thus a major aim of immunophenotyping in the age of personalized medicine, diagnostics and therapy is the development of tools allowing to dissect the immune response on the level of antigen-specific T and B cells, their relevant interaction partners and effector molecules. During a workshop on immunophenotyping in inflammatory diseases, which took place at the international symposium of the Cluster of Excellence “Precision Medicine in Chronic Inflammation (PMI)” of the universities of Kiel and Lübeck on February 18th 2020 in Hamburg, Germany, recent developments facilitating such antigen-specific approaches and beyond were discussed.
State-of-the-Art and Key Developments
Flow-Cytometry and Analysis of Antigen-Specific Lymphocytes
The method of choice for detailed characterization of immune cell composition and phenotype is flow-cytometry, which does not only allow rapid quantitation of the main leukocyte subsets but also their detailed phenotypic characterization, including the differentiation of the complex cosmos of lymphocyte subpopulations at a single cell level. A state-of-art overview of currently available cytometric technologies can be found under (1). Instrumental to this has been the steadily increasing numbers of parameters, which can be measured from individual cells. Theoretically > 40 parameters per cell are currently possible, although this is still not part of the clinical routine. However, recent developments especially of multi-laser instruments or spectral cytometers have significantly improved the ease of use, especially for the combination of various fluorescent dyes within multiplex panels (1–4). This may bring multi-parameter flow-cytometry closer to the clinical diagnostic lab reality.
Identification of antigen-specific lymphocytes is a challenge due to their low frequency and the availability of antigen-reagents allowing direct labeling of their antigen-receptor (5, 6). Although fluorescently tagged antigens can in principle be used to directly label B cells these are still no routine reagents and staining procedures (1). Even more challenging the exact peptide/MHC combinations need to be defined to generate the recombinant peptide/MHC-multimers for antigen-specific T cell staining. This is a significant difficulty especially for the analysis of human T cells due to the high HLA-diversity in general and in particular for CD4+ T cells due to low quality MHC class II reagents, complex antigens or pathogens and/or unknown peptide targets. Therefore especially human CD4+ T cell analysis is mainly based on functional read-outs, following in vitro stimulation with antigenic peptides, proteins or protein extracts. Several technologies to detect antigen-reactive T cells have been developed (1, 5, 6). A common problem for all antigen-specific assays is the low frequency of target cells. A frequent solution to this is the in vitro expansion of rare T and B cells prior to analysis. However, this introduces an unpredictable bias with regard to phenotype, function and selection of certain cell subsets. Direct approaches utilizing minimal stimulation times (5–7 h) combined with powerful rare cells enrichment strategies, e.g., via magnetic cell separation, provide a more direct view (7–9). Rapid induction of CD154 expression in all conventional T helper cells (Tcon) (10, 11) and of CD137 in Tregs (12) within a short time window of 5–7 h after in vitro antigen stimulation has proven to be highly sensitive and specific to identify essentially all reactive CD4+ T cells. This can be combined with magnetic enrichment strategies, termed antigen-specific T cell enrichment (ARTE) (7). This technology has been used to characterize human CD4+ T cell responses in a number of clinical settings, including allergy (13), IBD (13, 14) and infectious diseases (15). The unique strength of direct enrichment-based approaches is the access to highly purified antigen-specific T cells, e.g., via fluorescence-based flow-sorting technologies, which is ideal for combination with downstream “-omics” technologies, allowing their deep molecular profiling as well as functional analyses in unparalleled resolution (13, 14, 16) (Figures 1B, C). This includes TCR sequencing to determine T cell clonality and/or TCR affinity measurements (13, 14, 16) (Figures 1A, C). The particular relevance of activation-based assays has been highlighted by recent data on SARS-CoV-2-specific T cells which allowed rapid identification of target proteins and overall T cell reactivity in patients (17–19) as well as clonality and affinity differences between mild and severe COVID-19 (20). In general B/TCR sequencing provides unique insight in B and T cell composition as outlined below. However, it has to be emphasized here, that the combination of antigen-specific B/T cell sorting with antigen-receptor sequencing is particularly powerful. Antigen-receptor sequences from cells with known specificity can be used as molecular identifiers to track antigen-specific clones in samples from the same patient. This has been used, for example, to track the modulation of antigen-specific T cells in bulk TCR sequencing data from patients with multiple sclerosis during pregnancy (21). Similarly, known TCR sequences from paired blood and tissue samples will allow identification of antigen-specific T cells from bulk or single cell RNA sequencing data. The latter approach may allow to follow and deeply characterize antigen-specific T cells even in small tissue samples.
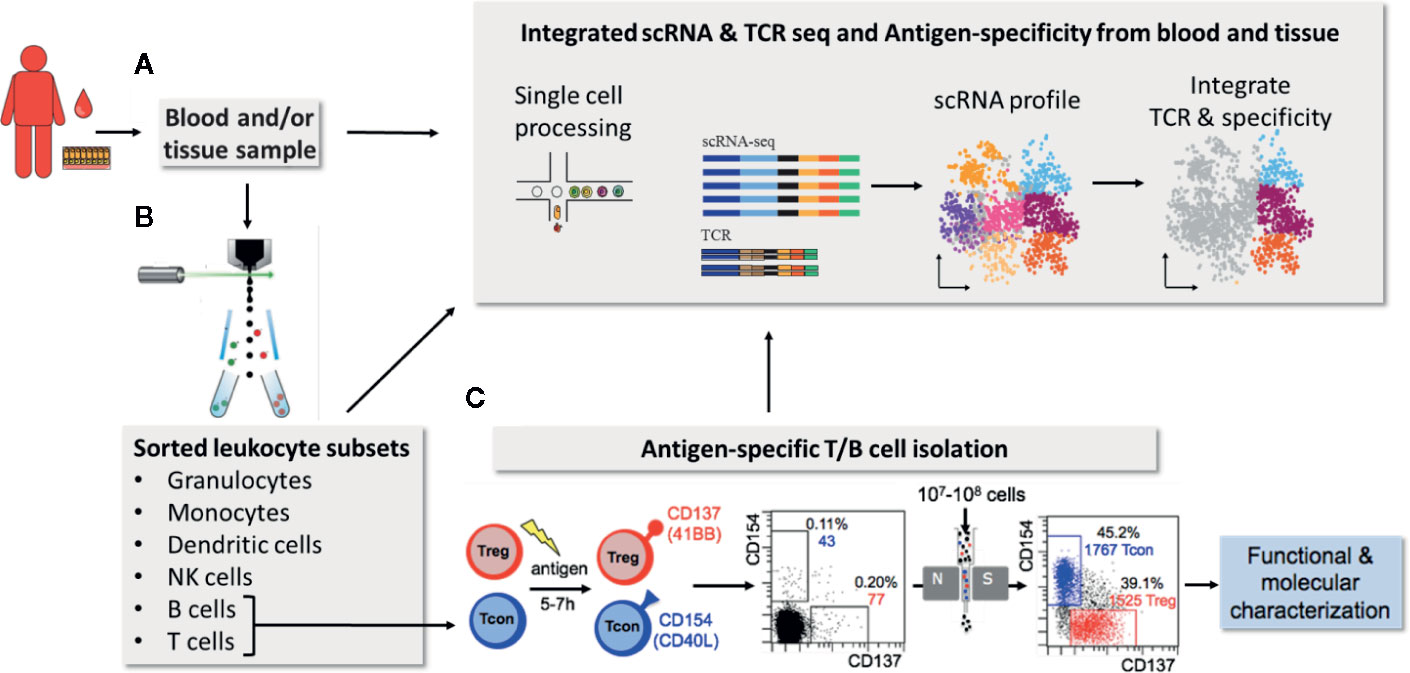
Figure 1 Integrated scRNA & TCR seq and antigen-specificity from blood and tissue. (Single cell) RNAseq provides unique multi-parameter information of single cells (A) or sorted cellular subsets (B). Further the combination of antigen-receptor sequencing with scRNASeq allows correlation of RNA expression with clonal lymphocyte populations (A). In addition, antigen-receptor sequences derived from antigen-specific lymphocytes of the same donor, obtained via combined magnetic pre-enrichment and fluorescence based flow-sorting, allows to integrate antigen-specificity and certain functional aspects (C). TCR/BCR information from antigen-specific lymphocytes may also be used to track antigen-specific cells within small tissue samples.
TCR Repertoire Analysis
Clonal expansion is one of the hallmarks of adaptive immune responses. Therefore T cell receptor (TCR) analyses carry great potential for investigating the specificity of the T cell immune response under physiological and pathophysiological conditions. There are however multiple issues to consider in advance. (i) The first is about numbers. As outlined above antigen-specific lymphocytes are rare. From the possible 1019 theoretically possible combinations (22), TCR selection during thymopoiesis selects for each individual a repertoire of approximately 107 to 108 unique TCRs (23). Thus looking for specific TCRs, even expanded ones, resembles searching for a needle in a haystack. (ii) The second is about sampling: where, and how much? Indeed, how much of the immune response targeting a pulmonary infection is represented in 105 cells from blood? (iii) The third is about T cell functional diversity. As an example, since Treg and effector T cells have opposite effects, what would mean the identification of a given TCR if you cannot assign it to one or the other cell population? (iv) The fourth is about technology: TCRs are made of alpha and beta chains that cannot (accurately) be paired by knowledge. Only single-cell sequencing can provide paired chains. Also, the accuracy of the technology that heavily relies on PCR amplification and its capacity to detect rare clonotypes might affect results (24). Despite all these limitations, TCR sequencing carries enormous potential in particular in combination with cytometric techniques discussed above. There are currently two major approaches to TCR studies: (i) global TCR repertoire assessment from a large number of cells, classically applied to study peripheral blood T cells, and which can generate millions of alpha and beta unpaired TCRs per sample, and (ii) single-cell sequencing of smaller samples, such as tissue infiltrating cells, which generates in the order of 103-104 clonotypes. Global TCR studies are providing much larger repertoires, from which the challenge is to identify the relevant TCRs. Numerous algorithms have been and are still developed to extract relevant information, such as repertoire diversity, expansions of rare cells and cross-reactivity (25–30). They, for example, allowed to identify the TCRs responding to vaccination (31) and in our experiments identified TCRs are specific and even predictive of given autoimmune diseases (David Klatzmann, unpublished observations). In contrast, single-cell studies have the advantages to relate TCRs directly to a setting/tissue or an ongoing immune response and to provide their paired alpha and beta chains (32). Noteworthy, it also allows to concomitantly investigate, (i) the binding of dextramers or MHC-multimers to give clues on the cell specificity, and (ii) the single-cell transcriptome to provide, for example, the activation status of cells with specific TCRs (32, 33).
Bulk and single cell TCR sequencing are truly complementary and even synergistic. Bulk TCR sequencing allows to provide general information on the repertoire, with indices reflecting its diversity and “normality” (25–30). Also, appropriate algorithms can identify thousands of TCRs of potential interest, based for example on their frequency in the sample compared to their theoretical probability of generation (34). The relevance of these sequences can further be explored by blasting them with databases of TCRs with known specificities (35, 36). On the other hand, single-cell sequencing, despite its limitation in terms of the number of sequences, has the advantage of providing the paired sequences in link to a specific setting. For example, the T cells present in bronchoalveolar lavages of patients with lung infection (33) or the cerebro-spinal fluid of patients with neurogenerative diseases can reasonably be assumed to be part of the ongoing immune reaction associated with the condition. Thus, as a recommendation, we would suggest the following:
● To sequence the bulk repertoire of at least 105 purified/enriched T cell populations, at least always separating Treg from Teffs, and using a robust method (24).
● To refrain from performing single-cell TCR sequencing from peripheral blood except if relevant subsets can be enriched, i.e., by antigen-specific cytometry assays as described above.
● To perform single-cell TCR sequencing on samples originating from a small cell population, whether this comes from their location or their expression of relevant markers.
Besides, one should make sure to analyze the obtained datasets with experts of the field and not forget to make their datasets public such as not to waste precious and costly results (37). Indeed, even if we could analyze the entire TCR repertoire of billions of humans bearing each 1012 T cells, we would still be far from approaching the 1061 different sequences that theoretically could be produced by the T cell machinery (38). Thus, any TCR sequence that was actually produced (i.e., detected in a sample) had dramatically much smaller chances to appear that the reader has to win the lottery, and thus represents extremely valuable knowledge (from theory to reality) that must be memorized.
RNA Sequencing
High-throughput sequencing methods have been the key to disentangle immune phenotypes of inflammatory diseases for decades. Advances in mapping disease activity, identification of early disease, and therapy-related molecular markers have been made possible by the widespread methodology of bulk RNA-seq, a robust and nowadays inexpensive method of sequencing the transcriptome. Despite the effort, the current number of direct applications of molecular-based disease diagnostic and treatment remains limited (39). One explanation for the poor output of bulk RNA-seq-driven methods is that it solely depicts the transcriptome of a homogeneous and unidentifiable agglomerate of different cell types. Thus, masking cell-specific transcription changes and neglecting the impact of cell type proportions in diseased tissues. Moreover, skewed levels of expression of molecular markers are expected when researchers focus on the immune compartment and particular aberrant proportions in inflamed tissues (40–42).
A possible approach to overcome the lack of cell-specificity is the implementation of single-cell sequencing (scRNA-seq), which generates a transcriptional landscape by cell type. This methodology is capable of acquiring up to 80,000 cells per run, enabling unprecedented power to study patterns of expression of even rare cell types linked to inflammatory diseases (43). Moreover, researchers can customize cell type identification based on their need, by using cell-specific signature genes (e.g., MKI67 high expression for proliferative lymphocytes) coupled with numerous reference transcriptomic datasets (44). Recent technological advances have also provided access to new layers of information by merging cell-specific expression with TCR or BCR information (45, 46) and one can even produce large-scale immunophenotyping panels of tens to hundreds of antibodies (47). This type of analysis leads to the unbiased classification of T or B cell populations of interest and the relationship TCR or BCR with the respectively cell-specific transcription. However, this methodology not only comes with a very high price tag but also with its own set of challenges [for review please see (48)]: (i) scRNA-seq only represents a subset of tissue, thus rare cell-types, such as antigen-specific lymphocytes might be missed by chance if the number of cells acquired is not suitable (49). (ii) Experimentally, the methods are prone to batch effects, e.g., time from acquiring sample to sample preparation is inversely correlated with sample quality and stability of expression data (50), different technologies and protocols may also acquire different cell proportions and gene counts (51). (iii) Despite the robust methods available for PBMCs, dissociation of fragile tissues such as the brain or intestinal epithelium, might be problematic and carry on skewed assessments of the tissue (52). (iv) Specific to TCR applications, the reads might be aligned to an incomplete “reference” genome that might also be biased by somatic rearrangements and mutations (45).
Regardless of the methodological pitfalls, crucial advances in immune profiling and particularly in mapping inflammatory diseases have been pinned to scRNA-seq technology: by integrating scRNA-seq and antigen-receptor sequencing (Figure 1A), Boland et al. have contributed to resolving clonal relationships of the PBMCs and the intestinal mucosa in ulcerative colitis by coupling an enrichment for IgG1+ in plasma cells in the inflamed tissue with a specific γδ T cell subset in the peripheral blood of patients (53). This publication elegantly highlights how combining gene expression data paired with receptor sequence enables re-expression and testing for antigen binding and function of potentially relevant molecular markers. Taken all together, scRNA-seq has quickly become the go-to technology for immune profiling of individual cells on a large scale.
Proteomics Approaches
In addition to multicolor flow-cytometry and transcriptomics, proteomics of sorted cell subsets and various tissues allow to provide detailed insights into pathology-associated changes in complex immune-mediated diseases (54). There are a multitude of proteomic techniques that can be used to analyze changes of protein expression; e.g., mass spectrometry-based proteomics (55, 56). This, however, requires a high degree of specialization and access to respective core facilities. Thus, we here focus on proteomic techniques that can, in principle, be applied in most laboratories: (i) Bead-based multiplex arrays for flow cytometry to determine cytokine concentrations, (ii) protein arrays for autoantibody profiling, (iii) proximity extension assay proteomics, (iv) multi-parameter immunohistochemistry, and (v) determination of kinase activity.
Bead-based multiplex arrays for flow cytometry have become a standard technology for the fast and high-throughput analysis of cytokine concentrations in biological samples, such as blood or cell culture supernatants (57, 58).
Several companies offer auto-antigen protein arrays that allow the simultaneous detection of multiple autoantibodies from human serum samples, including the immunoglobulin subtype differentiation (59). These led to the identification of autoantibodies in diseases that are currently not considered as autoimmune diseases, e.g., chronic obstructive pulmonary disease (60) or neuropsychiatric diseases (61). Furthermore, autoantibody arrays can be used to identify additional autoantibody reactivity beyond known autoantibodies, for example in pemphigus (62, 63). However, the mere detection of autoantibodies in any given population is usually not informative because autoantibodies can be detected at high frequency also in healthy blood donors (64, 65) and, are even present in IVIG preparations (66) used to treat autoimmune diseases (67). Thus, in depth characterization of their functions and of the corresponding B and T cells is mandatory.
Proximity extension assay (PEA) proteomics allow to identify up to 1500 human proteins in any solution. The assay is optimized for serum, but use of lysed tissue extracts has also been described (68, 69). For PEA, samples are incubated with a pair of oligonucleotide-labeled antibodies binding to different epitopes of a specific protein. When these antibodies are in close proximity, they hybridize, and are extended by a DNA polymerase. This unique DNA barcode is then amplified by PCR, whereby the amount of each specific DNA barcode in quantified by PCR (70). Regarding mouse samples, a limited number of proteins (90–100) can be analyzed using this technique. However, up to now, the majority of published reports on PEA have investigated patients with chronic inflammatory skin conditions, but not with systemic inflammatory or autoimmune diseases (69, 71–74). Based on our own experience, we believe that the PEA technology will increasingly be used to study alterations of proteomic signatures in chronic inflammatory diseases, and thus will significantly contribute to the understanding of disease pathogenesis. PEA also offers the possibility to stratify certain patients according to their proteomic signature, and/or allow to predict treatment responses.
While all of the above technologies are primarily used to identify proteomic signatures in blood, multi-parameter immunohistochemistry enables to determine protein expression in tissues. One platform is the multi-epitope ligand cartography (MELC) robot technology that has been described in 2006 (75). The principle behind MELC is repeated staining and subsequent bleaching of histology specimens with automated image recording. Interestingly, only few papers have been published using this technique. Overall, we believe that multi-parameter immunohistochemistry is a potentially powerful tool to understand both protein expression and location, however, may not be suitable for routine diagnostics due to high costs and low sample throughput.
Aberrant kinase activation, in particular of the Janus Kinase (JAK) family, has been identified as a therapeutic target for an increasing number of autoimmune diseases (76–78). A relatively novel method to determine kinase activity in cell culture, as well as tissue or blood samples, is a peptide microarray, commercialized under the name of PamGene (79). In this assay, either serine or threonine-containing peptides are spotted on a microarray. After application of the cell or tissue homogenates, kinases within these phosphorylate their respective substrates. Substrate phosphorylation is detected over time using specific antibodies. Based on the phosphorylation pattern of substrates, the activity of kinases can be determined. This peptide microarray for detection of kinase activity can, among others, be used for the identification of novel therapeutic targets (80, 81), discovery of biomarkers (82, 83), and understanding of physiological immune functions (84). The main focus of the PamGene has so far, however, been in the field of cancer research. Based on our own experience with this technique, we expect that this microarray technique will significantly contribute to our understanding of the pathogenesis of chronic inflammatory and autoimmune diseases in the near future.
Perspective
Despite significant progress in translational and personalized medicine in the last decade, there is still an urgent need to identify disease-specific signatures and individual biomarkers allowing patient stratification, prediction of responses to therapy including the assessment of dynamic changes and the identification of novel therapeutic targets. In addition, it should be mentioned that data validation and knowledge transfer into clinical routine is often missing in the majority of applied approaches. Modern high-throughput technologies can be extremely useful and time saving in immunological research and become increasingly relevant especially for precision medicine, but are also highly cost intensive. Thus, the right method or combination of methods suitable to adequately address the questions of your study needs to be carefully selected to get a reasonable balance between costs and benefits. On the other hand one has to consider in advance which relevant information and conclusions can be extracted from multi-parameter data sets and how these data can be combined and integrated in a biological and clinical context. The involvement of experienced system biologist and biostatisticians in the planning of a study is therefore highly recommended in order to get most information from the applied methods.
Author Contributions
JH, JB, RL, DK, and AS equally contributed to this review according to their area of expertise and with regard to content and structure of the manuscript. All authors contributed to the article and approved the submitted version
Funding
This work was supported by German Research Foundation grants EXC2167.
Conflict of Interest
The authors declare that the research was conducted in the absence of any commercial or financial relationships that could be construed as a potential conflict of interest.
Acknowledgments
We thank all speakers and participants of the workshop for their excellent contributions and fruitful discussions.
References
1. Cossarizza A, Chang HD, Radbruch A, Acs A, Adam D, Adam-Klages S, et al. Guidelines for the use of flow cytometry and cell sorting in immunological studies (second edition). Eur J Immunol (2019) 49:1457–973. doi: 10.1002/eji.201970107
2. Simoni Y, Chng MHY, Li S, Fehlings M, Newell EW. Mass cytometry: a powerful tool for dissecting the immune landscape. Curr Opin Immunol (2018) 51:187–96. doi: 10.1016/j.coi.2018.03.023
3. Futamura K, Sekino M, Hata A, Ikebuchi R, Nakanishi Y, Egawa G, et al. Novel full-spectral flow cytometry with multiple spectrally-adjacent fluorescent proteins and fluorochromes and visualization of in vivo cellular movement. Cytometry A (2015) 87:830–42. doi: 10.1002/cyto.a.22725
4. Niewold P, Ashhurst TM, Smith AL, King NJC. Spectral cytometry: a spectrum of possibilities. Cytometry A (2020). doi: 10.1002/cyto.a.24211
5. Bacher P, Scheffold A. New technologies for monitoring human antigen-specific T cells and regulatory T cells by flow-cytometry. Curr Opin Pharmacol (2015) 23:17–24. doi: 10.1016/j.coph.2015.04.005
6. Bacher P, Scheffold A. Flow-cytometric analysis of rare antigen-specific T cells. Cytometry A (2013) 83:692–701. doi: 10.1002/cyto.a.22317
7. Bacher P, Schink C, Teutschbein J, Kniemeyer O, Assenmacher M, Brakhage AA, et al. Antigen-reactive T cell enrichment for direct, high-resolution analysis of the human naive and memory Th cell repertoire. J Immunol (2013) 190:3967–76. doi: 10.4049/jimmunol.1202221
8. Brosterhus H, Brings S, Leyendeckers H, Manz RA, Miltenyi S, Radbruch A, et al. Enrichment and detection of live antigen-specific CD4(+) and CD8(+) T cells based on cytokine secretion. Eur J Immunol (1999) 29:4053–9. doi: 10.1002/(SICI)1521-4141(199912)29:12<4053::AID-IMMU4053>3.0.CO;2-C
9. Kotov D I, Jenkins MK. Peptide:MHCII Tetramer-Based Cell Enrichment for the Study of Epitope-Specific CD4(+) T Cells. Curr Protoc Immunol (2019) 125:e75. doi: 10.1002/cpim.75
10. Frentsch M, Arbach O, Kirchhoff D, Moewes B, Worm M, Rothe M, et al. Direct access to CD4+ T cells specific for defined antigens according to CD154 expression. Nat Med (2005) 11:1118–24. doi: 10.1038/nm1292
11. Chattopadhyay PK, Yu J, Roederer M. A live-cell assay to detect antigen-specific CD4+ T cells with diverse cytokine profiles. Nat Med (2005) 11:1113–7. doi: 10.1038/nm1293
12. Schoenbrunn A, Frentsch M, Kohler S, Keye J, Dooms H, Moewes B, et al. A converse 4-1BB and CD40 ligand expression pattern delineates activated regulatory T cells (Treg) and conventional T cells enabling direct isolation of alloantigen-reactive natural Foxp3+ Treg. J Immunol (2012) 189:5985–94. doi: 10.4049/jimmunol.1201090
13. Bacher P, Heinrich F, Stervbo U, Nienen M, Vahldieck M, Iwert C, et al. Regulatory T Cell Specificity Directs Tolerance versus Allergy against Aeroantigens in Humans. Cell (2016) 167:1067–78.e1016. doi: 10.1016/j.cell.2016.09.050
14. Bacher P, Hohnstein T, Beerbaum E, Rocker M, Blango MG, Kaufmann S, et al. Human Anti-fungal Th17 Immunity and Pathology Rely on Cross-Reactivity against Candida albicans. Cell (2019) 176:1340–55.e1315. doi: 10.1016/j.cell.2019.01.041
15. Bacher P, Steinbach A, Kniemeyer O, Hamprecht A, Assenmacher M, Vehreschild MJ, et al. Fungus-specific CD4(+) T cells for rapid identification of invasive pulmonary mold infection. Am J Respir Crit Care Med (2015) 191:348–52. doi: 10.1164/rccm.201407-1235LE
16. Aparicio-Soto M, Riedel F, Leddermann M, Bacher P, Scheffold A, Kuhl H, et al. TCRs with segment TRAV9-2 or a CDR3 histidine are overrepresented among nickel-specific CD4+ T cells. Allergy (2020) 75:2574–86. doi: 10.1111/all.14322
17. Grifoni A, Weiskopf D, Ramirez SI, Mateus J, Dan JM, Moderbacher CR, et al. Targets of T Cell Responses to SARS-CoV-2 Coronavirus in Humans with COVID-19 Disease and Unexposed Individuals. Cell (2020) 181:1489–501.e1415. doi: 10.1016/j.cell.2020.05.015
18. Weiskopf D, Schmitz KS, Raadsen MP, Grifoni A, Okba NMA, Endeman H, et al. Phenotype and kinetics of SARS-CoV-2-specific T cells in COVID-19 patients with acute respiratory distress syndrome. Sci Immunol (2020) 5. doi: 10.1126/sciimmunol.abd2071
19. Braun J, Loyal L, Frentsch M, Wendisch D, Georg P, Kurth P, et al. SARS-CoV-2-reactive T cells in healthy donors and patients with COVID-19. Nature (2020). doi: 10.1038/s41586-020-2598-9
21. Ramien C, Yusko EC, Engler JB, Gamradt S, Patas K, Schweingruber N, et al. T Cell Repertoire Dynamics during Pregnancy in Multiple Sclerosis. Cell Rep (2019) 29:810–15.e814. doi: 10.1016/j.celrep.2019.09.025
22. Bradley P, Thomas PG. Using T Cell Receptor Repertoires to Understand the Principles of Adaptive Immune Recognition. Annu Rev Immunol (2019) 37:547–70. doi: 10.1146/annurev-immunol-042718-041757
23. Qi Q, Liu Y, Cheng Y, Glanville J, Zhang D, Lee JY, et al. Diversity and clonal selection in the human T-cell repertoire. Proc Natl Acad Sci USA (2014) 111:13139–44. doi: 10.1073/pnas.1409155111
24. Barennes P, Quiniou V, Shugay M, Egorov ES, Davydov AN, Chudakov DM, et al. Benchmarking of T cell receptor repertoire profiling methods reveals large systematic biases. Nat Biotechnol (2020). doi: 10.1038/s41587-020-0656-3
25. Bolotin DA, Poslavsky S, Mitrophanov I, Shugay M, Mamedov IZ, Putintseva EV, et al. MiXCR: software for comprehensive adaptive immunity profiling. Nat Methods (2015) 12:380–1. doi: 10.1038/nmeth.3364
26. Shannon P, Markiel A, Ozier O, Baliga NS, Wang JT, Ramage D, et al. Cytoscape: a software environment for integrated models of biomolecular interaction networks. Genome Res (2003) 13:2498–504. doi: 10.1101/gr.1239303
27. Nazarov V I, Pogorelyy MV, Komech EA, Zvyagin IV, Bolotin DA, Shugay M, et al. tcR: an R package for T cell receptor repertoire advanced data analysis. BMC Bioinf (2015) 16:175. doi: 10.1186/s12859-015-0613-1
28. Sethna Z, Elhanati Y, Callan CG, Walczak AM, Mora T. OLGA: fast computation of generation probabilities of B- and T-cell receptor amino acid sequences and motifs. Bioinformatics (2019) 35:2974–81. doi: 10.1093/bioinformatics/btz035
29. Marcou Q, Mora T, Walczak AM. High-throughput immune repertoire analysis with IGoR. Nat Commun (2018) 9:561. doi: 10.1038/s41467-018-02832-w
30. Glanville J, Huang H, Nau A, Hatton O, Wagar LE, Rubelt F, et al. Identifying specificity groups in the T cell receptor repertoire. Nature (2017) 547:94–8. doi: 10.1038/nature22976
31. Pogorelyy MV, Minervina AA, Touzel MP, Sycheva AL, Komech EA, Kovalenko EI, et al. Precise tracking of vaccine-responding T cell clones reveals convergent and personalized response in identical twins. Proc Natl Acad Sci USA (2018) 115:12704–9. doi: 10.1073/pnas.1809642115
32. https://pages.10xgenomics.com/rs/446-PBO-704/images/10x_AN047_IP_A_New_Way_of_Exploring_Immunity_Digital.pdf.
33. Liao M, Liu Y, Yuan J, Wen Y, Xu G, Zhao J, et al. Single-cell landscape of bronchoalveolar immune cells in patients with COVID-19. Nat Med (2020) 26:842–4. doi: 10.1038/s41591-020-0901-9
34. Murugan A, Mora T, Walczak AM, Callan CG Jr. Statistical inference of the generation probability of T-cell receptors from sequence repertoires. Proc Natl Acad Sci USA (2012) 109:16161–6. doi: 10.1073/pnas.1212755109
35. Tickotsky N, Sagiv T, Prilusky J, Shifrut E, Friedman N. McPAS-TCR: a manually curated catalogue of pathology-associated T cell receptor sequences. Bioinformatics (2017) 33:2924–9. doi: 10.1093/bioinformatics/btx286
36. Shugay M, Bagaev DV, Zvyagin IV, Vroomans RM, Crawford JC, Dolton G, et al. VDJdb: a curated database of T-cell receptor sequences with known antigen specificity. Nucleic Acids Res (2018) 46:D419–27. doi: 10.1093/nar/gkx760
37. Corrie BD, Marthandan N, Zimonja B, Jaglale J, Zhou Y, Barr E, et al. iReceptor: A platform for querying and analyzing antibody/B-cell and T-cell receptor repertoire data across federated repositories. Immunol Rev (2018) 284:24–41. doi: 10.1111/imr.12666
38. Dupic T, Marcou Q, Walczak AM, Mora T. Genesis of the alphabeta T-cell receptor. PLoS Comput Biol (2019) 15:e1006874. doi: 10.1371/journal.pcbi.1006874
39. Ostrowski J, Dabrowska M, Lazowska I, Paziewska A, Balabas A, Kluska A, et al. Redefining the Practical Utility of Blood Transcriptome Biomarkers in Inflammatory Bowel Diseases. J Crohns Colitis (2019) 13:626–33. doi: 10.1093/ecco-jcc/jjy205
40. Parikh K, Antanaviciute A, Fawkner-Corbett D, Jagielowicz M, Aulicino A, Lagerholm C, et al. Colonic epithelial cell diversity in health and inflammatory bowel disease. Nature (2019) 567:49–55. doi: 10.1038/s41586-019-0992-y
41. He H, Suryawanshi H, Morozov P, Gay-Mimbrera J, Del Duca E, Kim HJ, et al. Single-cell transcriptome analysis of human skin identifies novel fibroblast subpopulation and enrichment of immune subsets in atopic dermatitis. J Allergy Clin Immunol (2020) 145:1615–28. doi: 10.1016/j.jaci.2020.01.042
42. Valenzi E, Bulik M, Tabib T, Morse C, Sembrat J, Trejo Bittar H, et al. Single-cell analysis reveals fibroblast heterogeneity and myofibroblasts in systemic sclerosis-associated interstitial lung disease. Ann Rheum Dis (2019) 78:1379–87. doi: 10.1136/annrheumdis-2018-214865
43. See P, Lum J, Chen J, Ginhoux F. Corrigendum: A Single-Cell Sequencing Guide for Immunologists. Front Immunol (2019) 10:278. doi: 10.3389/fimmu.2019.00278
44. Aran D, Looney AP, Liu L, Wu E, Fong V, Hsu A, et al. Reference-based analysis of lung single-cell sequencing reveals a transitional profibrotic macrophage. Nat Immunol (2019) 20:163–72. doi: 10.1038/s41590-018-0276-y
45. De Simone M, Rossetti G, Pagani M. Single Cell T Cell Receptor Sequencing: Techniques and Future Challenges. Front Immunol (2018) 9:1638. doi: 10.3389/fimmu.2018.01638
46. Goldstein LD, Chen YJ, Wu J, Chaudhuri S, Hsiao YC, Schneider K, et al. Massively parallel single-cell B-cell receptor sequencing enables rapid discovery of diverse antigen-reactive antibodies. Commun Biol (2019) 2:304. doi: 10.1038/s42003-019-0551-y
47. Stoeckius M, Hafemeister C, Stephenson W, Houck-Loomis B, Chattopadhyay PK, Swerdlow H, et al. Simultaneous epitope and transcriptome measurement in single cells. Nat Methods (2017) 14:865–8. doi: 10.1038/nmeth.4380
48. Lahnemann D, Koster J, Szczurek E, McCarthy DJ, Hicks SC, Robinson MD, et al. Eleven grand challenges in single-cell data science. Genome Biol (2020) 21:31. doi: 10.1186/s13059-020-1926-6
49. Nguyen A, Khoo WH, Moran I, Croucher P II, Phan TG. Single Cell RNA Sequencing of Rare Immune Cell Populations. Front Immunol (2018) 9:1553. doi: 10.3389/fimmu.2018.01553
50. Massoni-Badosa R, Iacono G, Moutinho C, Kulis M, Palau N, Marchese D, et al. Sampling time-dependent artifacts in single-cell genomics studies. Genome Biol (2020) 21:112. doi: 10.1186/s13059-020-02032-0
51. Mereu E, Lafzi A, Moutinho C, Ziegenhain C, McCarthy DJ, Alvarez-Varela A, et al. Benchmarking single-cell RNA-sequencing protocols for cell atlas projects. Nat Biotechnol (2020) 38:747–55. doi: 10.1038/s41587-020-0469-4
52. Guillaumet-Adkins A, Rodriguez-Esteban G, Mereu E, Mendez-Lago M, Jaitin DA, Villanueva A, et al. Single-cell transcriptome conservation in cryopreserved cells and tissues. Genome Biol (2017) 18:45. doi: 10.1186/s13059-017-1171-9
53. Boland BS, He Z, Tsai MS, Olvera JG, Omilusik KD, Duong HG, et al. Heterogeneity and clonal relationships of adaptive immune cells in ulcerative colitis revealed by single-cell analyses. Sci Immunol (2020) 5. doi: 10.1126/sciimmunol.abb4432
54. Conrad K, Shoenfeld Y, Fritzler MJ. Precision health: A pragmatic approach to understanding and addressing key factors in autoimmune diseases. Autoimmun Rev (2020) 19:102508. doi: 10.1016/j.autrev.2020.102508
55. Hammers CM, Tang HY, Chen J, Emtenani S, Zheng Q, Stanley JR. Research Techniques Made Simple: Mass Spectrometry for Analysis of Proteins in Dermatological Research. J Invest Dermatol (2018) 138:1236–42. doi: 10.1016/j.jid.2018.01.001
56. Madzharova E, Sabino F, Auf dem Keller U. Exploring Extracellular Matrix Degradomes by TMT-TAILS N-Terminomics. Methods Mol Biol (2019) 1944:115–26. doi: 10.1007/978-1-4939-9095-5_8
57. Kawamoto D, Amado PPL, Albuquerque-Souza E, Bueno MR, Vale GC, Saraiva L, et al. Chemokines and cytokines profile in whole saliva of patients with periodontitis. Cytokine (2020) 135:155197. doi: 10.1016/j.cyto.2020.155197
58. Park S, You YA, Yun H, Choi SJ, Hwang HS, Choi SK, et al. Cervicovaginal fluid cytokines as predictive markers of preterm birth in symptomatic women. Obstet Gynecol Sci (2020) 63:455–63. doi: 10.5468/ogs.19131
59. Zhu H, Luo H, Yan M, Zuo X, Li QZ. Autoantigen Microarray for High-throughput Autoantibody Profiling in Systemic Lupus Erythematosus. Genomics Proteomics Bioinf (2015) 13:210–8. doi: 10.1016/j.gpb.2015.09.001
60. Ma A, Wen L, Yin J, Hu Y, Yue X, Li J, et al. Serum Levels of Autoantibodies Against Extracellular Antigens and Neutrophil Granule Proteins Increase in Patients with COPD Compared to Non-COPD Smokers. Int J Chron Obstruct Pulmon Dis (2020) 15:189–200. doi: 10.2147/COPD.S235903
61. Delunardo F, Soldati D, Bellisario V, Berry A, Camerini S, Crescenzi M, et al. Anti-GAPDH Autoantibodies as a Pathogenic Determinant and Potential Biomarker of Neuropsychiatric Diseases. Arthritis Rheumatol (2016) 68:2708–16. doi: 10.1002/art.39750
62. Kalantari-Dehaghi M, Anhalt GJ, Camilleri MJ, Chernyavsky AI, Chun S, Felgner PL, et al. Pemphigus vulgaris autoantibody profiling by proteomic technique. PLoS One (2013) 8:e57587. doi: 10.1371/journal.pone.0057587
63. Kalantari-Dehaghi M, Molina DM, Farhadieh M, Morrow WJ, Liang X, Felgner PL, et al. New targets of pemphigus vulgaris antibodies identified by protein array technology. Exp Dermatol (2011) 20:154–6. doi: 10.1111/j.1600-0625.2010.01193.x
64. Prussmann J, Prussmann W, Recke A, Rentzsch K, Juhl D, Henschler R, et al. Co-occurrence of autoantibodies in healthy blood donors. Exp Dermatol (2014) 23:519–21. doi: 10.1111/exd.12445
65. Prussmann W, Prussmann J, Koga H, Recke A, Iwata H, Juhl D, et al. Prevalence of pemphigus and pemphigoid autoantibodies in the general population. Orphanet J Rare Dis (2015) 10:63. doi: 10.1186/s13023-015-0278-x
66. Schaub A, Wymann S, Heller M, Ghielmetti M, Beleznay Z, Stadler BM, et al. Self-reactivity in the dimeric intravenous immunoglobulin fraction. Ann N Y Acad Sci (2007) 1110:681–93. doi: 10.1196/annals.1423.071
67. Hoffmann JHO, Enk AH. High-Dose Intravenous Immunoglobulin in Skin Autoimmune Disease. Front Immunol (2019) 10:1090. doi: 10.3389/fimmu.2019.01090
68. Brunner PM, Suarez-Farinas M, He H, Malik K, Wen HC, Gonzalez J, et al. Author Correction: The atopic dermatitis blood signature is characterized by increases in inflammatory and cardiovascular risk proteins. Sci Rep (2018) 8:8439. doi: 10.1038/s41598-018-26378-5
69. Pavel AB, Zhou L, Diaz A, Ungar B, Dan J, He H, et al. The proteomic skin profile of moderate-to-severe atopic dermatitis patients shows an inflammatory signature. J Am Acad Dermatol (2020) 82:690–9. doi: 10.1016/j.jaad.2019.10.039
70. Lundberg M, Eriksson A, Tran B, Assarsson E, Fredriksson S. Homogeneous antibody-based proximity extension assays provide sensitive and specific detection of low-abundant proteins in human blood. Nucleic Acids Res (2011) 39:e102. doi: 10.1093/nar/gkr424
71. Glickman JW, Dubin C, Renert-Yuval Y, Dahabreh D, Kimmel GW, Auyeung K, et al. Cross-sectional study of blood biomarkers of patients with moderate to severe alopecia areata reveals systemic immune and cardiovascular biomarker dysregulation. J Am Acad Dermatol (2020). doi: 10.1016/j.jaad.2020.04.138
72. Rojahn TB, Vorstandlechner V, Krausgruber T, Bauer WM, Alkon N, Bangert C, et al. Single-cell transcriptomics combined with interstitial fluid proteomics defines cell type-specific immune regulation in atopic dermatitis. J Allergy Clin Immunol (2020) 146:1056–69. doi: 10.1016/j.jaci.2020.03.041
73. He H, Li R, Choi S, Zhou L, Pavel A, Estrada YD, et al. Increased cardiovascular and atherosclerosis markers in blood of older patients with atopic dermatitis. Ann Allergy Asthma Immunol (2020) 124:70–8. doi: 10.1016/j.anai.2019.10.013
74. Brunner PM, He R, Pavel AB, Czarnowicki T, Lefferdink R, Erickson T, et al. The blood proteomic signature of early-onset pediatric atopic dermatitis shows systemic inflammation and is distinct from adult long-standing disease. J Am Acad Dermatol (2019) 81:510–9. doi: 10.1016/j.jaad.2019.04.036
75. Schubert W, Bonnekoh B, Pommer AJ, Philipsen L, Bockelmann R, Malykh Y, et al. Analyzing proteome topology and function by automated multidimensional fluorescence microscopy. Nat Biotechnol (2006) 24:1270–8. doi: 10.1038/nbt1250
76. Solimani F, Meier K, Ghoreschi K. Emerging Topical and Systemic JAK Inhibitors in Dermatology. Front Immunol (2019) 10:2847. doi: 10.3389/fimmu.2019.02847
77. Szilveszter KP, Nemeth T, Mocsai A. Tyrosine Kinases in Autoimmune and Inflammatory Skin Diseases. Front Immunol (2019) 10:1862. doi: 10.3389/fimmu.2019.01862
78. Howell MD, Kuo F II, Smith PA. Targeting the Janus Kinase Family in Autoimmune Skin Diseases. Front Immunol (2019) 10:2342. doi: 10.3389/fimmu.2019.02342
79. Hilhorst R, Houkes L, van den Berg A, Ruijtenbeek R. Peptide microarrays for detailed, high-throughput substrate identification, kinetic characterization, and inhibition studies on protein kinase A. Anal Biochem (2009) 387:150–61. doi: 10.1016/j.ab.2009.01.022
80. Cato L, de Tribolet-Hardy J, Lee I, Rottenberg JT, Coleman I, Melchers D, et al. ARv7 Represses Tumor-Suppressor Genes in Castration-Resistant Prostate Cancer. Cancer Cell (2019) 35:401–13.e406. doi: 10.1016/j.ccell.2019.01.008
81. Tosi D, Perez-Gracia E, Atis S, Vie N, Combes E, Gabanou M, et al. Rational development of synergistic combinations of chemotherapy and molecular targeted agents for colorectal cancer treatment. BMC Cancer (2018) 18:812. doi: 10.1186/s12885-018-4712-z
82. Alack K, Weiss A, Kruger K, Horet M, Schermuly R, Frech T, et al. Profiling of human lymphocytes reveals a specific network of protein kinases modulated by endurance training status. Sci Rep (2020) 10:888. doi: 10.1038/s41598-020-57676-6
83. Noe G, Bellesoeur A, Thomas-Schoemann A, Rangarajan S, Naji F, Puszkiel A, et al. Clinical and kinomic analysis identifies peripheral blood mononuclear cells as a potential pharmacodynamic biomarker in metastatic renal cell carcinoma patients treated with sunitinib. Oncotarget (2016) 7:67507–20. doi: 10.18632/oncotarget.11686
Keywords: immunophenotyping, sequencing, proteomics, inflammation, precision medicine, TCR repertoire, antigens
Citation: Humrich JY, Bernardes JP, Ludwig RJ, Klatzmann D and Scheffold A (2020) Phenotyping of Adaptive Immune Responses in Inflammatory Diseases. Front. Immunol. 11:604464. doi: 10.3389/fimmu.2020.604464
Received: 09 September 2020; Accepted: 22 October 2020;
Published: 25 November 2020.
Edited by:
Massimo Gadina, National Institute of Arthritis and Musculoskeletal and Skin Diseases (NIAMS), United StatesReviewed by:
Phil Mccoy, National Institutes of Health (NIH), United StatesSilvia Piconese, Sapienza University of Rome, Italy
Copyright © 2020 Humrich, Bernardes, Ludwig, Klatzmann and Scheffold. This is an open-access article distributed under the terms of the Creative Commons Attribution License (CC BY). The use, distribution or reproduction in other forums is permitted, provided the original author(s) and the copyright owner(s) are credited and that the original publication in this journal is cited, in accordance with accepted academic practice. No use, distribution or reproduction is permitted which does not comply with these terms.
*Correspondence: Jens Y. Humrich, jens.humrich@uksh.de