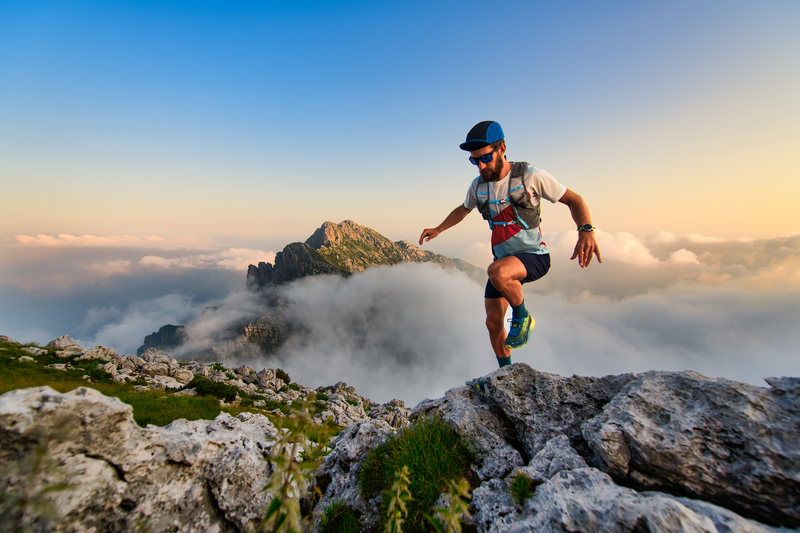
94% of researchers rate our articles as excellent or good
Learn more about the work of our research integrity team to safeguard the quality of each article we publish.
Find out more
ORIGINAL RESEARCH article
Front. Immunol. , 04 December 2020
Sec. Vaccines and Molecular Therapeutics
Volume 11 - 2020 | https://doi.org/10.3389/fimmu.2020.603337
Insights into the potential candidate hub genes may facilitate the generation of safe and effective immunity against seasonal influenza as well as the development of personalized influenza vaccines for the elderly at high risk of influenza virus infection. This study aimed to identify the potential hub genes related to the immune induction process of the 2018/19 seasonal quadrivalent inactivated influenza vaccines (QIVs) in the elderly ≥60 years by using weighted gene co-expression network analysis (WGCNA). From 63 whole blood samples from16 elderly individuals, a total of 13,345 genes were obtained and divided into eight co-expression modules, with two modules being significantly correlated with vaccine-induced immune responses. After functional enrichment analysis, genes under GO terms of vaccine-associated immunity were used to construct the sub-network for identification and functional validation of hub genes. MCEMP1 and SPARC were confirmed as the hub genes with an obvious effect on QIVs-induced immunity. The MCEMP1 expression was shown to be negatively correlated with the QIVs-associated reactogenicity within 7 days after vaccination, which could be suppressed by the CXCL 8/IL-8 and exacerbated by the Granzyme-B cytotoxic mediator. Meanwhile, the SPARC expression was found to increase the immune responses to the QIVs and contribute to the persistence of protective humoral antibody titers. These two genes can be used to predict QIVs-induced adverse reaction, the intensity of immune responses, and the persistence of humoral antibody against influenza. This work has shed light on further research on the development of personalized QIVs with appropriate immune responses and long-lasting immunity against the forthcoming seasonal influenza.
In the industrialized countries, influenza associated deaths usually occur in adults ≥ 65 yrs (years) (1). Based on the estimation model of influenza-related mortality ratios, the risk of death associated with seasonal influenza is higher in the age group ≥65 yrs than in the age group <65 yrs annually, resulting in a tremendous disease burden in the regions with more aging populations (2). Studies have shown that immunosenescence may decrease the capacity of the immune system and increase influenza associated morbidity and mortality (3, 4). Although influenza vaccination can protect against infection and its complications, as well as reduce the risk of hospitalization and the severity of influenza-related diseases, the effectiveness and efficacy of seasonal influenza vaccines varied with vaccination coverage, season, sex, health status, race/ethnicity and age (5, 6). The critical factor of aging immunity contributed to a lower cell-mediated immune response to the influenza vaccination in the elderly individuals > 65 yrs than in those < 65 yrs, leading to the failure to offer sufficient protection against seasonal circulating influenza virus (7–10), with an over 31% decrease observed in the vaccine effectiveness in the elderly individuals aged 65 yrs or above in the two A(H3N2)-dominated influenza seasons (11), due to the poorly adapted B cell responses (12) and a rapid decline in the antibodies induced by the trivalent seasonal influenza vaccine (13, 14). China has entered an aging society, with 18.1% of its population or 253.88 million individuals aged 60 yrs and above as of January 2020, suggesting the necessity to understand the transcriptomic mechanism of immunity induction by the quadrivalent influenza vaccine in the elderly individuals and explore the potential hub genes and signaling pathways for the development of effective quadrivalent seasonal influenza vaccines (15).
Several systems vaccinology studies have been performed in humans to elucidate the immunogenicity of seasonal influenza vaccines from multidimensional levels in adults, identifying early IFN signatures at the transcriptome level (16) and inducing positive memory B cells through early activation of circulating memory T follicular helper cells(ICOS+CXCR3+CXCR5+CD4+ T cells, Tfh) (17), which contributed to a higher antibody response and long-lasting humoral immunity, respectively. This novel systems vaccinology approach focused on the interaction between vaccines and the entire host systems (18). Systems vaccinology provides a powerful tool to analyze a large amount of biological data from omics technology to reconstruct the biological processes contributing to the success or failure of the influenza vaccines for the elderly and establish a standard influenza vaccine prototype.
To obtain critical immune-related information from the intricate mechanisms related to the immune responses to influenza vaccines, a powerful analytical method is needed to mine vaccine-induced immunity information from large omics datasets. Convenient and inexpensive access to human peripheral blood for accurate diagnosis and monitoring of post-immunization conditions suggests the need to identify reliable biomarkers from peripheral blood to assess the effectiveness and safety of QIVs in the elderly after inoculation. Several valuable biomarkers were identified by using next-generation sequencing, such as GCN2 (general control nonderepressible 2 kinase), with a strong correlation between its expression and the enhancement of antigen presentation (19). It is a tough challenge to identify one single gene signature or biomarker to understand or predict vaccine response through paired sample analysis of differentially expressed genes. As the subjects with baseline heterogeneity at the transcriptome level, this limitation is inevitable, resulting in the loss of useful information, low abundance or no statistical fold-change. Peripheral whole blood contains various types of immune cells, which can provide a large amount of RNA expression data, but require effective analytical methods to compress these high-dimensional gene expression data into a few modules of eigengenes with a close functional relationship.
Weighted Gene Co-expression Network Analysis (WGCNA) is commonly used to explore both weighted and un-weighted gene correlation networks from RNA-Seq data, such as a hierarchical clustering analytical method to measure the functional relationship between genes or modules (20). This computational methodology can be used to reconstruct a complete picture of vaccine-induced immune responses from a network-focused perspective and provide systematic insights without losing any information in paired sample analysis. WGCNA has been effectively used to identify disease-associated co-expression modules and eigengenes, and interpret large amounts of RNA-Seq data as biological process information (21, 22). To our best knowledge, few studies have been performed by using this methodology to investigate the vaccine-induced immunity, compare, or verify the results of previous systems vaccinology studies in seasonal influenza vaccination.
The purpose of this study was to identify the potential candidate hub genes and functionally enriched pathways in the immune responses of the elderly to QIVs by WGCNA analysis of the RNA-Seq data sampled from the elderly individuals aged ≥60 yrs after one dosage of QIV vaccination. The dynamic and kinetic changes of transcriptional RNA expression and humoral antibody titer in these individuals were monitored by collecting venous blood samples at four time points pre- and post-vaccination as the immunological processes induced by influenza vaccines. Finally, 8 modules were constructed by WGCNA with the progression of QIV immunization to identify the potential hub genes and functionally enriched pathways.
The study population and laboratory detection protocols described below are based on those published in previous studies (23, 24).
Briefly, a total of 1920 medically healthy subjects were enrolled into a randomized, double-blind, controlled phase III, and non-inferiority clinical trial in the southeast of China [Registration Numbers: CTR20190846; subjects ≥ 60 yrs; perturbation: the 2018/19 seasonal quadrivalent inactivated influenza split vaccines (QIVs)]. From this enrolled population, 60 elderly participants were randomly selected for cell-mediated immunity analysis, and 16 of them with distinct demographic characteristics and HAI titers were chosen to identify the intricate mechanisms related to various immune phenotypes by multidimensional analyses, such as whole blood transcriptome and plasma cytokine expression.
The 1920 medically healthy recipients were immunized intramuscularly in the deltoid muscle of the nondominant arm with one dosage of the 2018/19 seasonal quadrivalent inactivated influenza split vaccines {QIVs, the experiment vaccine by Wuhan Institute of Biological Products Co., Ltd., lot: SH201805649; the control vaccine by Hualan Biological Engineering Co., Ltd., lot: 201809B033; containing the A/Michigan/45/2015 NYMC X-275A [H1N1; an A/Michigan/45/2015(H1N1)pdm09 like virus], A/Singapore/INFIMH-16-0019/2016 IVR-186 [H3N2; an A/Singapore/INFIMH-16-0019/2016(H3N2) like virus], B/Phuket/3073/2013 wild type virus [B Yamagata lineage], and B/Colorado/06/2017 wild-type virus [B Victoria lineage]}. Both the chicken egg-derived QIVs adopted the same vaccine production process with standard dosage formulation of 15 µg per virus strain.
The whole blood samples (~ 4 ml each) of the 60 subjects were promptly collected at day 0 (pre-vaccination), 3, 28, and 180 post-vaccination in conventional vacuum tubes with EDTA-K2 anticoagulant by professional phlebotomists in Gaozhou Center for Disease Control and Prevention, where the clinical study was conducted (Gaozhou, Guangdong, China).
Serum samples from subjects inoculated with QIVs were obtained pre-vaccination and 28 days after immunization. Hemagglutination-inhibiting (HAI) assay was applicated for immunogenicity of the vaccine against vaccine homologous formulations.
The HAI assay applied in accordance with standard WHO procedures (25) to assess immune responses post-vaccination. A 1% suspension of chicken erythrocytes and 4 HA units/25 μl of corresponding influenza virus antigens were employed in the detection of functional antibody titers. Serum samples were treated with receptor destroying enzyme and tested in duplicate in serial 2-fold dilutions initiating from 1:10. And each test plate contained both negative and positive serum controls. The HI titer was defined as the inverse of the highest serum dilution to inhibit hemagglutination. For the convenience of calculation and statistical analysis, HI antibody titers below 10 were treated as 5.HI antibody titer ≥40 was defined as seroprotection/seropositive, which was deemed to provide humoral immune protection against specific influenza viruses.
Before this clinical study, a written informed consent was obtained from each subject. The clinical protocols were approved by the Clinic Institutional Ethics Review Board of Guangdong Centers for Disease Control and Prevention, and the study was performed according to the local institutional ethics committee guidelines. The trial was registered with China Drug Trials.org.cn (Registration Numbers: CTR20190846; subjects ≥ 60 years).
RNA-Seq Expression data in our study were deposited at the National Center for Biotechnology Information Gene Expression Omnibus (GEO) public repository (accession number: GSE151558), to review GEO accession GSE151558 at https://www.ncbi.nlm.nih.gov/geo/query/acc.cgi?acc=GSE151558.
The mRNA sequencing was performed as previously reported (26, 27). Briefly, total RNA was extracted from each EDTA-K2 anticoagulant supplemented with whole blood sample using TRIzol reagent (Bio Basic Inc., Canada) according to the manufacturer’s instructions, followed by constructing the sequencing libraries and isolating poly-A RNA from total RNA by Poly-T oligo-attached magnetic beads (Invitrogen, CA, USA). Paired-end read sequencing was performed on an Illumina Hiseq platform (Illumina NovaSeq 6000), and 125/150 bp paired-end reads were generated. Hisat2 v2.0.5 was used to build the index of the reference genome (hg19) and align the paired-end clean reads to the reference genome. The variability in RNA-seq data was removed with the Conditional Quantile Normalization (CQN) method (28). The number of reads mapped in the reference genome was counted by FeatureCounts version (1.5.0-p3). Subsequent analyses were based on these normalized values, including 18,849 genes with counts over 0 at one of these four time points (Days 0, 3, 28, and 180).
The WGCNA algorithm was used to construct the hierarchical clusters containing genes with similar or identical functions using the standard gene expression data obtained at the four time points (Days 0, 3, 28, and 180) as previously reported (29). Prior to similarity matrix construction, all pairwise genes were calculated for Pearson’s correlation to show the similarity between gene expression profiles, followed by transforming the similarity matrix into an adjacency matrix using the soft power adjacency function approach , which can exhibit the potential factorization superiority and maintain the integrity of omics information. The optimal parameter β (the soft threshold β=2 was chosen in this analysis, with a minimum module size of 30 genes) was chosen to satisfy the scale-free topology appropriately. The topological overlap matrix (TOM= ωij) was converted into a dissimilarity measure by the following equation based on the adjacency function parameter, and the TOM-based dissimilarity measure and average linkage hierarchical clusters were applied in the definition of gene modules. Each module of eigengenes represented the function of a module, which was used to generate the correlation coefficients between modules and merge the highly correlated modules (a Pearson correlation coefficient of over 0.75). Finally, the Spearman correlation between these clustering eigengenes and the immune status of the subjects was calculated to reveal the underlying mechanisms involved in vaccine-induced immunity and immunosenescence of the elderly individuals ≥ 60 yrs as well as the related hub genes and functionally enriched pathways.
To investigate the correlation of the divided co-expression modules, the eigengenes of each corresponding module were used for interaction analysis and heat map visualization. The main objective of this co-expression network analysis was to establish the relationship between these co-expression modules and the immune status of the elderly and identify the hub genes contributing to the modular connectivity and functionality. The traits of immune status in the elderly included gender, age, medical history, basal body temperature, general reaction, body mass index (BMI), blood pressure differential, and seroprotection against the four influenza vaccine strains (H1N1, H3N2, BY and BV), and the correlation between co-expression modules and these traits was calculated. The co-expression modules of a significant correlation to the traits related to influenza vaccine-induced immunity were defined as playing a critical role in the process of effective active immunization with QIVs. Besides, genes with messy expression in the grey module of high correlation with these traits may contribute to diagnose the immunogenicity of QIVs in the elderly. The subsequent analysis focused on the gene co-expression modules of the highest correlation to the traits described above.
The co-expression genes in the modules of interest were extracted for subsequent gene enrichment and network analyses to identify the genes involved in important biological regulation and signaling pathways contributing to efficient influenza vaccine-induced immune responses (30). Cluster Profiler R package was used to test the statistical enrichment of genes in Gene Ontology (GO) and Kyoto Encyclopedia of Genes and Genomes (KEGG) pathways (http://www.genome.jp/kegg/), where adjusted P-value < 0.05 was considered significantly enriched and the top 10 significant terms were visualized.
Differentially expressed gene analysis was employed in the expression profiles of critical genes among the older adults with variant immune responses to QIVs, using the DESeq2 R package (1.16.1) based on the approach of Benjamini and Hochberg, with cut-off criterion set as FDR (false discovery rate, padj) <0.05 and |log2fold change| >1 (31).
The hub genes in modules with higher correlation to the subject traits and efficient immune responses to the influenza vaccination may contribute to understanding the underlying transcriptional mechanisms of influenza vaccine response in the elderly. After GO enrichment analysis of the subject traits-correlated modules, the hub genes were excavated from the divided clusters with significant enrichment in immunity-related GO terms by constructing the sub-network. Cytoscape version 3.7.2 software was applied for the gene sub-network visualization based on its plug-in cytohubba function (32, 33), and the genes with high Maximal Clique Centrality (MCC) values were defined as hub genes. Subsequently, the hub genes in each corresponding module were excavated and imported into the STRING database (Version: 11.0) to obtain sub-network from the six protein-protein interaction (PPI) sources (Textmining, Experiments, Databases, Co−expression, Neighborhood, Gene Fusion) with a high confidence of not less than 0.4 and disconnected nodes hidden in the network for exploring Protein-Protein Interactions. Gene significance (GS) was denoted as correlation of gene expression profile with one subject trait, while the transcript module membership (MM) score was calculated by correlating the module eigengene with intramodular corresponding gene transcript expression. These associated functions and the index of screened hub genes could add weight to the identification robustness of indispensable regulatory impacts of interested hub genes on the immunity induced by influenza vaccines. For each trait in the elderly, the driver genes of corresponding modules were screened out by the highest absolute values of GS and MM generated by WGCNA.
Briefly, total RNA was extracted from whole blood sample using combined protocol with TRIzol and Takara MiniBest universal RNA extraction kit (Takara Bio Inc., Japan). Using PrimeScript™ RT Master Mix (Perfect Real Time) (Takara Bio Inc., Japan) to synthesize cDNA from 250 ng of total RNA in each sample, according to the manufacturer’s protocol. The housekeeping gene GAPDH (glyceraldehyde-3-phosphate dehydrogenase) was employed as internal reference. The primers of two genes were designed as follows: MCEMP1 forward, 5’-AGCCATCCTGAGCCTGTA-3’ and MCEMP1 reverse, 5’-TGCCTGCTAATGTCGTCT-3’; SPARC forward, 5’-CAGAACCACCACTGCAAACA-3’ and SPARC reverse, 5’- AAGTGGCAGGAAGAGTCGAA-3’. The SYBR Green Real time PCR Master Mix (Takara Bio Inc., Japan) was used to perform the qRT-PCR detection according to the manufacturer’s instructions and previous study (34). Each sample was detected in triplicate and the data was displayed as Mean FC (Fold Change) value and the Standard Error (SEM) relative to the expression of GAPDH gene based on 2−ΔΔCT method (35).
The concentrations of plasma cytokines (CCL4, Granzyme B, HGF, IFN-alpha, IFN-beta, IFN-gamma, CXCL 8/IL-8, IL-10, etc.) were detected using the xMAP technology according to the manufacturer’s instructions (LXSAHM-20, R&D Systems Inc.). Briefly, plasma samples were incubated for 2 h with antibodies conjugated to microspheres at room temperature, followed by incubation for 1 h with biotinylated antibodies and then 30 min with streptavidin-phycoerythrin fluorescent conjugate (SA-PE). The Luminex® 200TM instrument (Luminex Corp.) was used to detect the signal intensity for each microsphere added to the protein samples. In this study, we only focused on two cytokines (CXCL 8/IL-8 and Granzyme-B) to demonstrate the dynamic status of pro-inflammation and cell-mediated immunity pre- and post-vaccination in the elderly.
Statistical analyses were conducted using IBM SPSS Statistics 26.0.0.0 (SPSS Software). A least significant difference (LSD) post hoc-test in one-way ANOVA was employed to compare multiple groups, with a 95% confidence interval.
1920 medically stable adults aged 60 years and older were enrolled into the clinical study. Sixty subjects randomly selected from this clinical trial, with no significant medically healthy differences pre-vaccination (Table 1). The immunogenicity results could meet the European criteria (Committee for Medicinal Products for Human Use (CHMP) guidelines) against the four vaccine strains (H1N1, N3N2, BYAM strain, and BVIC strain) at day 28 after immunization (Table 2). Specifically, the Seroprotection Rates (SPRs) against H1N1, N3N2, BVIC, and BYAM strain ranged in 94.38%–98.28%, the Seroconversion Rates (SCRs) against these four strains ranged in 74.14%–87.93%, and the Geometric Mean Increase (GMI) of antibody against these four strains increased 6.01–18.25 folds. The ranged existing immunity against influenza virus among the older subjects before vaccination, not only exhibited a history of influenza vaccination and/or infection, but also highlighted the individual variability in immune system. Subsequently, 16 subjects demonstrated distinct immune response phenotypes, were chosen for the integration analysis of the correlation of various phenotypes with transcriptional RNA expression.
Table 2 The serological test results of QIVs against the vaccine strains (H1N1, N3N2, BYAM strain, and BVIC strain) by hemagglutinin inhibition assay (HAI).
The median age and age range of the 60 subjects selected were 67 yrs and 60–80 yrs, respectively, with female accounting for 43.3%. The detailed traits of immune status among the 16 older adults were listed in the Table S1. The pre-existing antibody titer against BYAM strain was higher than that against the other three vaccine strains (H1N1, H3N2, BVIC) in the older adults.
Prior to the construction of hierarchical clusters, 18,849 genes were selected after normalization of raw gene counts, excluding the genes with no expression in all samples. A total of 64 samples were collected from 16 of the 60 subjects at four time points pre- and post-immunization, with one sample not collected at the fourth time point, thus 63 samples for further WGCNA clustering analysis.
To identify the hub genes which may regulate the immune responses to the QIVs in the elderly subjects, the similarly expressed genes in these samples were clustered into eight co-expression modules and marked with corresponding module colors, while the other genes were grouped into the grey module. Using the soft thresholding power (β=2) in this algorithm, the co-expression network could satisfy the approximate scale-free topology criterion with R2 > 0.80 while maintaining a high mean connectivity with enough information (Figure 1). We merged the modules of eigengenes with a correlation coefficient of over 0.75 (Figure 2), and the size of the co-expression modules ranged from 82 to 9,579 genes.
Figure 1 Plots of the Scale Free Topology Fitting Index (A) and the Mean Connectivity (B). Each point was marked by the corresponding soft thresholding parameter β. β=2 was chosen as it could satisfy the criteria of a high R2 and a high mean number of connections.
Figure 2 Modules for the progression of QIVs-induced immunity in the elderly. (A) Cluster Dendrogram of transcriptional expression genes with top 50% variance generated by using the TOM-based dissimilarity measure calculated as The modules were labeled with different colors below the cluster dendrogram; the “Dynamic Tree Cut” row denoted the original clustering division and the ‘Merged Colors’ row displayed the final modules after merging the high eigengenes-based correlation coefficient modules. (B) Dendrogram of eigengenes in corresponding modules calculated using Pearson’s correlation. (C) Adjacency heatmap of co-expression modules of eigengenes. Red represents high adjacency (positive correlation) and blue represents low adjacency (negative correlation). The meta-modules are along the diagonal.
After merging the highly correlated modules, the co-expression genes were clustered into eight modules with similar gene expression patterns and labeled with different colors (Figure 2A), including the modules of Pink (82 genes), Black (163 genes), Red (173 genes), Green (487 genes), Yellow (613 genes), Brown (951 genes), Blue (1297 genes), and Turquoise (9579 genes), respectively. Grey module contained 5,504 genes that did not have the similar expression pattern with any modules. Genes within the corresponding modules indicated higher correlation than the genes between different modules from the network heatmap plot of selected genes (Figure S1). After visualizing the correlation of these eight modules, two clusters were yielded and each cluster could be further divided into two sub-clusters as displayed in (Figures 2B, C).
In order to correlate the network modules with the health status of the elderly subjects and the immune responses induced by QIVs, the correlation coefficients between modules and traits were calculated. Six of these traits were slightly correlated with one or more modules, and the trait-module relationships with P ≤ 0.1 were further analyzed (Figure 3). Taking the trait Gender as an example, it was correlated with the Black (cor=0.29 P=0.02) and Red Module (cor=0.28, P=0.03), while the character of QIVs-induced immunity against BY vaccine strain was related to three modules, including Green (cor=-0.22, P=0.08), Black (cor=-0.26, P=0.04), and Grey (cor=0.24, P=0.06) modules. From the perspective of module-trait relationship, the Brown module was negatively correlated with General Reaction involved in the local swelling, headache, and fever (cor=-0.2, P=0.1). The Green module was positively related with the Age of the subjects (cor=0.25, P=0.05), and negatively correlated with the two traits of General Reaction (cor=-0.22, P=0.08) and QIVs-induced immunity against BY vaccine strain (cor=-0.22, P=0.08). The Black module was positively correlated with the Gender character (cor=0.29, P=0.02) and also negatively correlated with the two traits of General Reaction (cor=-0.19, P=0.1) and pre-existing immunity against BY strain (cor=-0.26, P=0.04). The Red module was positively related to the traits of Gender (cor=0.28, P=0.03) and Medical History (cor=0.19, P=0.1). The Yellow module was only positively correlated with the character of General Reaction (cor=0.18, P=0.1), while Grey module was significantly and negatively correlated with the Age of the subjects (cor=-0.3, P=0.02). These results indicate that the traits, including gender and age in the elderly, may play a key role in the immune outcomes induced by QIVs, and a set of genes from these co-expression modules may help prognose the vaccine-related adverse reaction and contribute to the persistence of protective antibody level against influenza BY vaccine virus strain.
Figure 3 Heatmap plot of module-trait relationships. The correlation coefficients of module eigengenes with different health status and QIVs-induced immunity were calculated to identify the potential biological functions of each module. Each cell displayed the corresponding correlation coefficient and (P-value), and the cells with P ≤ 0.1 were highlighted in the box. The color depth indicated the intensity of correlation by the color legend (right), with red representing the positive correlation and blue representing the negative correlation. The gene cluster modules correlated with external gene information were labeled by summarizing their main functionality in red text.
These five co-expression modules significantly related with the external gene information were used for GO and KEGG gene enrichment analysis, and the hub genes in each module were excavated based on PPI analysis. The immunological themes of these co-expressed modules were identified by GO term enrichment analysis and the top six enrichment GO terms are shown in Table 3. The Brown module was highly enriched in the biological categories involved in interleukin production, including interleukin-8, interleukin-1 beta and interleukin-6, as well as biological signal transduction, including MyD88-dependent toll-like receptor signaling pathway and the regulation of cysteine-type endopeptidase activity involved in apoptotic process. The Green module was highly enriched in immune responses involved in neutrophil activation, including immune response, phagocytosis, acute inflammatory response, negative regulation of mononuclear cell proliferation, as well as the components of intracellular organelles such as ficolin-1-rich granule and secretory granule lumen. The Black module contained the co-expressed genes related to the regulation of body fluid levels, leukocyte migration, secretory granule lumen, secretory granule lumen and SH3/SH2 adaptor activity. The Red module was enriched in genes related to myeloid cell homeostasis, myeloid cell differentiation, protoporphyrinogen IX metabolic process, and homeostasis of number of cells, while the Yellow module contained genes involved in the cellular components of myofilaments.
Table 3 The enrichment of genes from co-expression modules identified by WGCNA in immune-related Gene Ontology (GO) terms.
Furthermore, the potential pathways associated with QIVs-induced immunity in these five modules were investigated by KEGG enrichment analysis. The Brown module was enriched in genes involved in Influenza A (hsa05164, padj=8.23E-06), NOD-like receptor signaling pathway (hsa04621, padj=2.05E-05), Th17 cell differentiation (hsa04659, padj=0.002), Toll-like receptor signaling pathway (hsa04620, padj=0.002), Th1 and Th2 cell differentiation (hsa04658, padj= 0.0118), and Chemokine signaling pathway (hsa04062, padj=0.0465). The Green module genes were enriched in the Fc gamma R-mediated phagocytosis (hsa04666, padj=0.0199), while the Black module co-expression genes participated in Platelet activation (hsa04611, padj=0.0001), Complement and coagulation cascades (hsa04610, padj=0.0284), ECM-receptor interaction (hsa04512, padj=0.0284), and Chemokine signaling pathway (hsa04062, padj=0.0284). The pathway Mitophagy (hsa04137, padj=0.0088) was identified in the Red module, while the Yellow module genes were not enriched in any KEGG term.
The correlation coefficients between co-expression modules and traits revealed the Green module with the most significant positive and negative correlation to Age and General Reaction within 7 days, in contrast to the most significant positive and negative correlation to Gender and pre-existing immunity against BY vaccine strain for the Black module, respectively. Therefore, these two modules were the priority for further sub-network analysis and key gene identification, and they were designated as top age-related module and top gender-related module, respectively.
The differential expression genes (DEGs) in the subjects with gender and age differences were investigated to identify the intersectional DEGs from the most trait-related gene clusters. In the top age-related module, 2 up-regulated genes were screened in the subjects ≤ 65 yrs versus those > 65 yrs. In the top gender-related module, 81 activated genes were identified in the female group versus the male group. Meanwhile, in the top age-related gene cluster, 23 DEGs were generated in subjects with vaccination-induced general reaction within 7 days versus those with no general reaction, including two up-regulated and 21 down-regulated genes. In the top gender-related gene cluster, only 10 down-regulated genes were screened in the group with seroprotection against BY strain versus the group with no seroprotection against BY strain pre-vaccination. The traits-related DEGs from these modules are shown in Table S2.
PPI analysis and sub-network construction were performed for genes enriched in the GO terms relevant to vaccination-induced immunity. The sub-network was constructed using the MCL clustering method with inflation parameter set as 3, and the MCODE plugin of Cytoscape was conducted to identify the key clusters of the PPI network. By ranking the genes with the MCC value, 10 hub genes were identified in each module (Figure 4) and no statistical difference of MCC score occurred in the top 8 genes of each module (Table S3). According to the PPI analysis and gene functional annotation, MCEMP1 and SPARC were deemed as the key genes with most significant relation to QIVs-induced immunity in the top age-related module and top gender-related module, respectively.
Figure 4 Sub-network of the top 10 hub genes extracted based on the GO terms of the two modules with a significant correlation with the QIVs-induced immunity. The nodes indicate the genes, and the edges indicate the degree of correlation. The top 10 hub genes were ranked by the MCC value, and the intensity of red color represented a higher MCC value. (A) Sub-network derived from the Green gene cluster; (B) Sub-network derived from the Black gene cluster.
To further add weight to the key role of these two genes in the immune responses to QIVs, we analyzed the expression level of MCEMP1 and SPARC in different character groups of the subjects ≥ 60 yrs. The expression of MCEMP1 reached the peak at day 3 post-vaccination either in NM65yrs (no more than 65 years) group or M65yrs (more than 65 years) group, with higher expression level identified in NM65yrs group at day 3 and 180 after influenza immunization (Figure 5A). Meanwhile, the expression of MCEMP1 was significantly higher in the no general reaction (NGR) group than in the QIVs-associated general reaction (GR) group at day 3 post-immunization (p=0.025) (Figure 5B). The other key gene SPARC for immune differentiation showed obviously higher expression in the female group than in the male group at four time points, reaching the maximal value at day 3 post-vaccination in the female group (p=0.005) and day 28 in the male groups (p=0.023) (Figure 5C). Furthermore, the SPARC gene showed significantly lower expression in the SP group (with seroprotection or pre-existing immunity against influenza BY vaccine strain) than in the NSP group (with no seroprotection against BY strain pre-vaccination) at day 3 (p=0.025) (Figure 5D). The differential expression analysis results demonstrated that the MCEMP1 and SPARC genes could contribute to the development of QIVs-related immunity.
Figure 5 Kinetic characteristics of MCEMP1 and SPARC expression in different trait groups. The differential transcriptional expression of MCEMP1 in (A) different age groups and (B) general reaction (induced by vaccination within 7 days) groups. The differential transcriptional expression of the SPARC gene in (C) different gender groups and (D) seroprotection against BY influenza vaccine strain (existing immunity before vaccination) groups. Statistical significance was considered as p-value <0.05. FPKM, expected number of Fragments Per Kilobase of transcript sequence per Millions base pairs sequenced; M65yrs, more than 65 years old; NM65yrs, no more than 65 years old; GR, general reaction within 7 days after QIVs vaccination; NGR, no general reaction within 7 days after QIVs vaccination; NSP, no seroprotection against BY strain pre-vaccination; SP, seroprotection against BY strain pre-vaccination.
To verify the RNA-Seq results of differential expression of MCEMP1 and SPARC genes among different trait groups, qRT-PCR assay was conducted, and the gene expression patterns of MCEMP1 and SPARC genes were shown to be consistent with the transcriptome results in distinguishing the General Reaction Groups and Seroprotection Groups respectively (Figure 6). At day 3 post-vaccination, the relative expression level of MCEMP1 gene was significantly higher in the no general reaction (NGR) group (p=0.04915) (Figure 6B), in contrast to a significantly lower relative expression level of the SPARC gene in the SP group (p=0.038244) (Figure 6D).
Figure 6 Relative expression level of MCEMP1 and SPARC genes in different trait groups. The relative expression of MCEMP1 gene among (A) different age groups and (B) general reaction (induced by vaccination within 7 days) groups. The relative expression of SPARC gene among (C) different gender groups and (D) seroprotection against BY influenza vaccine strain (existing immunity before vaccination) groups. The expression level of GAPDH was used as an internal control. Each sample was detected in triplicate. Each bar and error bar represent Mean FC (Fold Change) value and the Standard Error (SEM), respectively. Statistical significance was considered as p-value <0.05. FPKM, expected number of Fragments Per Kilobase of transcript sequence per Millions base pairs sequenced; M65yrs, more than 65 years old; NM65yrs, no more than 65 years old; GR, general reaction within 7 days after QIVs vaccination; NGR, no general reaction within 7 days after QIVs vaccination; NSP, no seroprotection against BY strain pre-vaccination; SP, seroprotection against BY strain pre-vaccination.
The interferon (IFN-alpha<1.19 pg/ml, IFN-beta<1.57pg/ml, and IFN-gamma<6.46 pg/ml) and IL-10 (<1.02 pg/ml) concentrations among most plasma samples were lower than the detection limit. The dynamic expression of CCL4 and HGF cytokines could identify the peak level of vaccine-induced immune responses at day 3 post-vaccination, but could not significantly distinguish the different immune phenotypes and vaccine-related immune progression stages.
The potential immunological functions of these two genes were investigated by analyzing the regulation of the lymphocyte activity and inflammation by the genes-related immunoglobulin and/or cytokines using the plasma samples from the elderly subjects. Previous studies have shown that lower expression of MCEMP1 enhanced the T cell activity and NK cell activity while suppressing the secretion of inflammation-induced cytokine in a mouse model with sepsis, which was identified as a type II transmembrane protein in human mast cells, exerting functionality by secreting multiple preformed inflammatory mediators (36, 37). Generally, SPARC is a non-structure matrix-related glycoprotein, contributing to the organization and regulation of extracellular matrix (ECM) networks, including cell shape, cell migration, composition of ECM, and molecular signal transduction (38–40), while SPARC not only serves as a prediction biomarker for various cancers, but also shows a significant effect on the immune cell responses, i.e., its overexpression could inhibit the migration of dendritic cells (DCs) and subsequently obstruct the development of adaptive immunity and promote inflammation (41–43). Therefore, we analyzed the relevant cytokines and categorized them as pro-inflammation (CXCL 8/IL-8) and cell-mediated immunity (Granzyme-B).
The kinetic characteristics of these two cytokines can reflect the potential progression of inflammation status and cell-mediated immunity after immunization (Figure 7). Consistent with previous studies, GR group showed a lower expression of MCEMP1, leading to the suppression of CXCL 8/IL-8 and the increased expression of Granzyme-B (Figures 7C, D). The overexpression of SPACR in the female group and NSP group promoted inflammation with a high concentration of CXCL 8/IL-8 cytokine (Figures 7E, G), while inhibiting the cell-mediated immunity with a low level of Granzyme-B (Figures 7F, H). However, compared with the protein level, the kinetic characteristics of the mRNA expression of these two cytokine genes in the venous blood cells failed to show obvious difference in the different trait groups (age, gender, general reaction, and pre-existing immunity), and thus the cytokine concentrations rather than the mRNA expression levels were used to evaluate the immune responses, as the plasma cytokines (CXCL 8/IL-8 and Granzyme-B) are derived from a variety of cells (Figure S2).
Figure 7 Kinetic characteristics of CXCL 8/IL-8 and Granzyme-B secretory cytokines after QIVs vaccination in the plasma in different trait groups at four time points (days 0, 3, 28, and 180). Elderly subjects with specific traits were categorized into four trait groups, including age group (A, B), vaccination-related general reaction group (C, D), gender group (E, F), and existing seroprotection against BY group (G, H). Only one subject was excluded from the cytokine response analysis due to lack of the fourth blood draw. Statistical significance was considered as p-value <0.05. M65yrs, more than 65 years old; NM65yrs, no more than 65 years old; GR, general reaction within 7 days after QIVs vaccination; NGR, no general reaction within 7 days after QIVs vaccinations; SP, seroprotection against BY strain pre-vaccination; NSP, no seroprotection against BY strain pre-vaccination; SP, seroprotection against BY strain pre-vaccination.
The weaker immune responses to TIV were identified not only in infants, but also in the elderly individuals, when compared with adults (44). The efficacy of inclusion of MF59 (an oil-in-water emulsion adjuvant) in trivalent seasonal influenza vaccines was further assessed in immunological naïve infants, which showed a similar transcriptional response in infants as that in adults vaccinated with TIVs, and a positive correlation of early gene signatures of innate immunity at day 1 (including antiviral IFN genes, dendritic cell, etc.) with higher serum antibody titers at day 28 (45, 46). Previously identified biomarkers and gene signatures could only predict humoral antibody responses to influenza vaccine in young adults, but not in the elderly, due to the expression of CXCR5 and programmed death-1 (PD-1) genes in circulating Tfh cells (47). Furthermore, the immune response of the elderly to influenza vaccines is highly heterogeneous, with low or no immune responses detected in some of them, which was not driven by the absence or presence of pre-existing immunity to influenza virus (48, 49). This heterogeneity highlights the necessity to investigate the transcriptional basis for the variability of immune responses to influenza vaccination in the elderly.
Safe and effective QIV vaccination is an urgent demand for the populations with a high risk of influenza infection, especially the elderly individuals ≥ 60 yrs, and the progression of vaccine-induced immunity is a network process, highlighting the importance of effector cytokines in bridging the interaction of multiple immune cells. In this study, the biological information on immune responses to QIVs was excavated based on gene co-expression patterns through WGCNA analysis of the transcriptomic data from 63 vascular blood samples. These blood samples were collected from 16 subjects at 4 time points pre- and post-immunization, excluding one sample due to lack of the fourth blood sampling. Eight co-expression modules were generated by hierarchical clustering analysis, with the cluster size ranging from 82 to 9,579 genes. Two of the eight gene clusters (Green, Black, a total of 650 genes) were significantly correlated with individual characteristics of age and gender, as well as the QIVs-induced immune responses involved in general reaction within 7 days and pre-existing immunity against BY vaccine strain.
By correlating the two gene clusters to the traits of the elderly individuals before and after influenza vaccination, the Green module was shown to be significantly positively correlated with the age of the subjects and negatively correlated with general reaction (reactogenicity). Meanwhile, the Black module was identified to have a significant positive correlation with gender dimorphism and negative correlation with pre-existing immunity against BY vaccine strain. After correlation analysis, potential hub genes were identified by GO and KEGG functional enrichment analysis. Results indicated that the genes with a similar expression pattern in the top age-related module were significantly enriched in the initial immune responses to QIVs, including phagocytosis, acute inflammatory response, negative regulation of mononuclear cell proliferation, while the genes in the top gender-related module were enriched in the cell-mediated immunity. From these two highly trait-related modules, the intersectional DEGs were excavated, with 2, 81, 23, and 10 DEGs being identified in the age, gender, general reaction, and pre-existing immunity groups, respectively. The DEGs correlated with individual characteristics were up-regulated during the immune process, while most of the DEGs related with vaccine-induced responses were down-regulated, which could be used as the potential biomarkers for the individual vaccination strategy in the elderly.
To determine the hub genes in the two modules, the genes enriched in the GO terms related to the immune responses induced by QIVs were confirmed by PPI analysis and sub-network construction. The integrated functional analysis revealed MCEMP1 and SPARC as the hub genes during the development of safe and effective immunity related to influenza vaccines in the top age-related module and top gender-related module, respectively. As few studies have been performed on the association of MCEMP1 and SPARC genes with influenza vaccine responses thus far, the transcriptional expression level of the two hub genes was analyzed based on the FPKM value, and the potential regulatory roles of MCEMP1 and SPARC in the immunity against influenza were confirmed by analyzing the dynamic activity of the related effector cytokines CXCL 8/IL-8 and Granzyme-B.
MCEMP1 showed a significantly higher expression in the NGR group than in the GR group, reaching the peak value at day 3 post-vaccination, highlighting the regulatory effect of dynamically expressed MCEMP1 on the safety and tolerability to the influenza vaccines. Furthermore, the transcriptional expression level of SPARC was obviously higher in the female group than in the male group, reaching the peak in the former group at day 3 and in the latter group at day 28, implying higher humoral antibody and immune responses to influenza vaccines in the females (50). Intriguingly, the SP group displayed lower SPARC expression than NSP group, further confirming that excessive SPARC expression may lead to the decline of protective humoral antibody level, while SPARC deficiency may impair B lymphopoiesis (51, 52).
The MCEMP1 gene is located on human chromosome 19 band p13.3, and genes adjacent to MCEMP1 may contribute to the immune regulation of cell surface receptors, including transient expression of CD23 molecules (IgE low-affinity receptors) (36). The MCEMP1 gene encodes mast cell-expressed membrane protein 1 and can be expressed in mast cells, macrophages and even the brain tissue (53, 54). The effect of MCEMP1 on QIVs-induced immunity in elderly populations remains to be elucidated, although growing studies demonstrated its diagnostic capacity on the progression of stroke (53), and the promotion effect of its high expression on inflammation and sepsis (55).
The SPARC gene is located on human chromosome 5 band q31-q33, and its neighboring genes contributed to the immune regulation based on the transcriptional level, such as MFAP1 required for pre-mRNA processing, activation of T and NK cells (IL12B gene), as well as proliferation and differentiation of mononuclear phagocytes (CSF1R) (56). Human SPARC known as a secretory protein was detected among a variety of tissues, including blood, lung, and skin tissues (57). The functional role of the SPARC protein in the development of QIVs-induced immunity is being clarified, and SPARC has been the topic of various recent studies, including tumorigenesis (58), wound healing (39), lymphocytes activity (40, 59), and innate immunity (60, 61).
There are many limitations in the measurement of QIV efficacy in the elderly using only antibody titers against influenza, suggesting that cell-mediated immunity is essential for detecting anti-influenza efficacy (62). Previous studies have demonstrated the key immunological functions of the proteins encoded by the two genes. Combined with gene functional enrichment and individual characteristic analysis, we testified the immune process related to inflammation promotion and cell-mediated immunity, in which effector cytokines CXCL 8/IL-8 and Granzyme-B were selected to monitor the dynamic immune responses of inflammation and cell immunity pre- and post-immunization, respectively. Chemokine CXCL 8/IL-8 may affect vaccination as a molecular adjuvant, and the trivalent inactivated vaccine (TIV) would influence the serum cytokine level, but the live attenuated influenza vaccine (LAIV) would not (63), which was supported by the suppression of cytokine response patterns in severe pandemic 2009 H1N1 among hospitalized adults (64). In previous studies, Granzyme-B was shown as a cytolytic mediator contributing to the activation of cytotoxic T lymphocyte (CTL) and the elimination of influenza-infected cells, which can be used in diagnosis of influenza illness and influenza-induced fever (r= 1.000; p= 0.01) in the vaccinated elderly (65).
This is probably the first study regarding the correlation of these two genes (MCEMP1, SPARC) with individual traits and immune responses in the development of effective immunity against influenza after QIVs inoculation. In our study, MCEMP1 was shown to contribute significantly to the reactogenicity within 7 day after QIVs vaccination, with higher Granzyme-B secretion and lower CXCL 8/IL-8 expression in the GR group (with vaccine associated adverse reactions) than in the NGR group (with good tolerability). Interestingly, the SPARC gene expression level could distinguish the females from males, which reached a peak at day 28 in the male group but at day 3 in the female group. Humoral and cell-mediated immunity showed a higher CXCL 8/IL-8 secretion and a lower Granzyme-B expression, which was consistent with previous studies reporting that females typically generate higher humoral antibody responses to seasonal influenza vaccines while the males sustain longer-lasting seroprotective antibody titers (50, 66, 67). The kinetic characteristics of the SPARC gene expression pattern in the NSP group were in accordance with the trait of the female group (as the NSP group mainly consisted of elderly females) and the secretion level of these two cytokines. Additionally, GO enrichment analysis indicated that MCEMP1 mainly contributed to the neutrophil-mediated immunity, including neutrophil degranulation, granulocyte activation, neutrophil activation involved in immune response, formulation of specific granules and secretory granule membranes involved in mediating the immune process. Meanwhile, SPARC was shown to participate in the immune responses to the pathogen-derived antigens (such as lipopolysaccharide and molecules of a bacterial origin) and was involved in the lumen development of cytoplasmic vesicles and secretory granules. From these results, it can be concluded that the MCEMP1 and SPARC genes may have an effect on the development of protective humoral and cell immunity against influenza in the elderly. These two genes could not only be used as biomarkers in the prediction of adverse reaction and immunogenicity before influenza vaccination, but also provide gender characteristics for further research to improve the QIV efficacy in the development of personalized influenza vaccines. Furthermore, due to the insufficient research on potential biomarkers for the evaluation of influenza vaccine efficacy and safety, MCEMP1 and SPARC genes in our study may provide a good reference for further studies.
This study has a few limitations that warrant further investigation. First, our study lacks a large sample size covering different races of older people, and thus the specificity of MCEMP1 and SPARC genes could not be synthetically evaluated. Second, if the threshold concentration can significantly differentiate populations with different immune response phenotypes, then the QIVs-related reactogenicity biomarker MCEMP1 and immune persistent biomarker SPARC will have greater utility. In our study cohort, the elderly enrolled demonstrated a history of influenza virus vaccination and/or infection, based on the existing immunity to four vaccine strains before inoculation, and blank samples (with non-existing immunity) should be collected to evaluate the utility of these two biomarkers (MCEMP1 and SPARC) in guiding the clinical use of influenza vaccines covering a wider population. Third, the underlying mechanisms of MCEMP1 and SPARC genes contributing to the development of protective immunity after influenza vaccination should have been rigorously verified in multidimensions, especially the regulation of effector cytokines (CXCL 8/IL-8, Granzyme-B). Due to the small size of the current study, our findings need to be further confirmed with a larger sample size and thus cannot be applied to different ethnic groups at present.
In this study, the hub genes related to the immunity against seasonal influenza viruses were identified by a comprehensive network-based analysis of transcriptome data and plasma cytokine responses in the elderly≥60 yrs. MCEMP1 and SPARC were confirmed as the hub genes with potential influence on the outcomes of QIVs-induced immunity. The MCEMP1 expression is negatively correlated with the QIVs-related reactogenicity within 7 day after vaccination, which would be suppressed by the CXCL 8/IL-8 and exacerbated by the Granzyme-B cytotoxic mediator. Meanwhile, the SPARC expression tends to increase the immune responses to the QIVs and promote the persistence of protective humoral antibody titers. These two genes can be used to predict the adverse reactions induced by QIVs, the intensity of immune responses, and the persistence of humoral antibody against influenza. This work has provided useful information for future research on the development of personalized seasonal influenza vaccines with proper immune responses and long-lasting immunity against influenza.
The datasets presented in this study can be found in online repositories. The names of the repository and accession number can be found here: https://www.ncbi.nlm.nih.gov/geo/, GSE151558.
The studies involving human participants were reviewed and approved by The Clinic Institutional Ethics Review Board of Guangdong Centers for Disease Control and Prevention. The patients/participants provided their written informed consent to participate in this study.
JY, JYZ, RF, WZ, JKZ, and XY conceived the idea of this research. JY, JYZ, RF, and TH carried out the bioinformatics and data analysis. JY, JYZ, RF, WZ, and KD, XL, PZ, and JD collaborated with the interpretation of results, discussion, and review of the manuscript. JY drafted the manuscript. All authors contributed to the article and approved the submitted version.
JY is studying for his doctorate at Wuhan Institute of Biological Products Co., Ltd. JYZ, WZ, KD, and XL are employees in the company Wuhan Institute of Biological Products Co., Ltd. TH is studying for a master’s degree at Wuhan Institute of Biological Products Co., Ltd. XY is an employee of the company China Biotechnology Co., Ltd.
The remaining authors declare that the research was conducted in the absence of any commercial or financial relationships that could be construed as a potential conflict of interest.
The Supplementary Material for this article can be found online at: https://www.frontiersin.org/articles/10.3389/fimmu.2020.603337/full#supplementary-material
1. Organization WH. Influenza (Seasonal) World Health Organization (WHO). (2018). https://www.who.int/en/news-room/fact-sheets/detail/influenza-(seasonal). cited 2020 April 29.
2. Iuliano AD, Roguski KM, Chang HH, Muscatello DJ, Palekar R, Tempia S, et al. Estimates of global seasonal influenza-associated respiratory mortality: a modelling study. Lancet (2018) 391(10127):1285–300. doi: 10.1016/S0140-6736(17)33293-2
3. Reber AJ, Chirkova T, Kim JH, Cao W, Biber R, Shay DK, et al. Immunosenescence and Challenges of Vaccination against Influenza in the Aging Population. Aging Dis (2012) 3(1):68–90.
4. Crooke SN, Ovsyannikova IG, Poland GA, Kennedy RB. Immunosenescence: A systems-level overview of immune cell biology and strategies for improving vaccine responses. Exp Gerontol (2019) 124:110632. doi: 10.1016/j.exger.2019.110632
5. Doyle JD, Chung JR, Kim SS, Gaglani M, Raiyani C, Zimmerman RK, et al. Interim Estimates of 2018-19 Seasonal Influenza Vaccine Effectiveness - United States, February 2019. MMWR Morb Mortal Wkly Rep (2019) 68(6):135–9. doi: 10.15585/mmwr.mm6806a2
6. Dong Hee L, Minkyung K, Minjoo K, Young Ju L, Hye Jin Y, Sang-Hyun L, et al. Age-dependent alterations in serum cytokines, peripheral blood mononuclear cell cytokine production, natural killer cell activity, and prostaglandin F 2α. Immunol Res (2017) 65(5):1009–16. doi: 10.1007/s12026-017-8940-0
7. McElhaney JE, Kuchel GA, Zhou X, Swain SL, Haynes L. T-Cell Immunity to Influenza in Older Adults: A Pathophysiological Framework for Development of More Effective Vaccines. Front Immunol (2016) 7:41. doi: 10.3389/fimmu.2016.00041
8. Haralambieva IH, Ovsyannikova IG, Kennedy RB, Zimmermann MT, Grill DE, Oberg AL, et al. Transcriptional signatures of influenza A/H1N1-specific IgG memory-like B cell response in older individuals. Vaccine (2016) 34(34):3993–4002. doi: 10.1016/j.vaccine.2016.06.034
9. Haralambieva IH, Painter SD, Kennedy RB, Ovsyannikova IG, Lambert ND, Goergen KM, et al. The impact of immunosenescence on humoral immune response variation after influenza A/H1N1 vaccination in older subjects. PloS One (2015) 10(3):e0122282. doi: 10.1371/journal.pone.0122282
10. Dyda A, MacIntyre CR, McIntyre P, Newall AT, Banks E, Kaldor J, et al. Factors associated with influenza vaccination in middle and older aged Australian adults according to eligibility for the national vaccination program. Vaccine (2015) 33(29):3299–305. doi: 10.1016/j.vaccine.2015.05.046
11. Puig-Barberà J, Mira-Iglesias A, Tortajada-Girbés M, López-Labrador FX, Librero-López J, Díez-Domingo J, et al. Waning protection of influenza vaccination during four influenza seasons, 2011/2012 to 2014/2015. Vaccine (2017) 35(43):5799–807. doi: 10.1016/j.vaccine.2017.09.035
12. Henry C, Zheng NY, Huang M, Cabanov A, Rojas KT, Kaur K, et al. Influenza Virus Vaccination Elicits Poorly Adapted B Cell Responses in Elderly Individuals. Cell Host Microbe (2019) 25(3):357–66.e6. doi: 10.1016/j.chom.2019.01.002
13. Kositanont U, Assantachai P, Wasi C, Puthavathana P, Praditsuwan R. Kinetics of the antibody response to seasonal influenza vaccination among the elderly. Viral Immunol (2012) 25(6):471–6. doi: 10.1089/vim.2012.0024
14. Young B, Zhao X, Cook AR, Parry CM, Wilder-Smith A, MC IC. Do antibody responses to the influenza vaccine persist year-round in the elderly? A systematic review and meta-analysis. Vaccine (2017) 35(2):212–21. doi: 10.1016/j.vaccine.2016.11.013
15. National Bureau of Statistics. 2019 statistical bulletin on national economic and social development. National Bureau of Statistics of China. Available at: http://www.stats.gov.cn/tjsj/zxfb/202002/t20200228_1728913.html. cited 2020 1 May.
16. Bucasas KL, Franco LM, Shaw CA, Bray MS, Wells JM, Nino D, et al. Early patterns of gene expression correlate with the humoral immune response to influenza vaccination in humans. J Infect Dis (2011) 203(7):921–9. doi: 10.1093/infdis/jiq156
17. Bentebibel SE, Lopez S, Obermoser G, Schmitt N, Mueller C, Harrod C, et al. Induction of ICOS+CXCR3+CXCR5+ TH cells correlates with antibody responses to influenza vaccination. Sci Trans Med (2013) 5(176):176ra32. doi: 10.1126/scitranslmed.3005191
18. Lever M, Silveira EL, Nakaya HI. Systems Vaccinology Applied to DNA Vaccines: Perspective and Challenges. Curr Issues Mol Biol (2017) 22:1–16. doi: 10.21775/cimb.022.001
19. Ravindran R, Khan N, Nakaya HI, Li S, Loebbermann J, Maddur MS, et al. Vaccine activation of the nutrient sensor GCN2 in dendritic cells enhances antigen presentation. Sci (New York NY) (2014) 343(6168):313–7. doi: 10.1126/science.1246829
20. Langfelder P, Horvath S. WGCNA: an R package for weighted correlation network analysis. BMC Bioinformatics (2008) 9:559. doi: 10.1186/1471-2105-9-559
21. Liu X, Hu AX, Zhao JL, Chen FL. Identification of Key Gene Modules in Human Osteosarcoma by Co-Expression Analysis Weighted Gene Co-Expression Network Analysis (WGCNA). J Cell Biochem (2017) 118(11):3953–9. doi: 10.1002/jcb.26050
22. Yao Q, Song Z, Wang B, Qin Q, Zhang JA. Identifying Key Genes and Functionally Enriched Pathways in Sjögren’s Syndrome by Weighted Gene Co-Expression Network Analysis. Front Genet (2019) 10:1142. doi: 10.3389/fgene.2019.01142
23. Pei G, Chen L, Zhang W. WGCNA Application to Proteomic and Metabolomic Data Analysis. Methods Enzymol (2017) 585:135–58. doi: 10.1016/bs.mie.2016.09.016
24. Voigt EA, Grill DE, Zimmermann MT, Simon WL, Ovsyannikova IG, Kennedy RB, et al. Transcriptomic signatures of cellular and humoral immune responses in older adults after seasonal influenza vaccination identified by data-driven clustering. Sci Rep (2018) 8(1):739. doi: 10.1038/s41598-017-17735-x
25. Organization WH. Global Influenza Surveillance Network. Manual for the laboratory diagnosis and virological surveillance of influenza (2011). Available at: http://whqlibdoc.who.int/publications/2011/9789241548090_eng.pdf (Accessed 13 January 2020).
26. Haralambieva IH, Oberg AL, Ovsyannikova IG, Kennedy RB, Grill DE, Middha S, et al. Genome-wide characterization of transcriptional patterns in high and low antibody responders to rubella vaccination. PloS One (2013) 8(5):e62149. doi: 10.1371/journal.pone.0062149
27. Kennedy RB, Oberg AL, Ovsyannikova IG, Haralambieva IH, Grill D, Poland GA. Transcriptomic profiles of high and low antibody responders to smallpox vaccine. Genes Immun (2013) 14(5):277–85. doi: 10.1038/gene.2013.14
28. Hansen KD, Irizarry RA, Wu Z. Removing technical variability in RNA-seq data using conditional quantile normalization. Biostatistics (2012) 13(2):204–16. doi: 10.1093/biostatistics/kxr054
29. Zhang B, Horvath S. A General Framework for Weighted Gene Co-Expression Network Analysis. Stat Appl Genet Mol Biol (2005) 4(1):17. doi: 10.2202/1544-6115.1128
30. Deist MS, Gallardo RA, Dekkers JCM, Zhou H, Lamont SJ. Novel Combined Tissue Transcriptome Analysis After Lentogenic Newcastle Disease Virus Challenge in Inbred Chicken Lines of Differential Resistance. Front Genet (2020) 11:11. doi: 10.3389/fgene.2020.00011
31. Love MI, Huber W, Anders S. Moderated estimation of fold change and dispersion for RNA-seq data with DESeq2. Genome Biol (2014) 15(12):550. doi: 10.1186/s13059-014-0550-8
32. Shannon P, Markiel A, Ozier O, Baliga NS, Wang JT, Ramage D, et al. Cytoscape: a software environment for integrated models of biomolecular interaction networks. Genome Res (2003) 13(11):2498–504. doi: 10.1101/gr.1239303
33. Chin CH, Chen SH, Wu HH, Ho CW, Ko MT, Lin CY. cytoHubba: identifying hub objects and sub-networks from complex interactome. BMC Syst Biol (2014) 8 Suppl 4:S11. doi: 10.1186/1752-0509-8-S4-S11
34. Kim A, Yoon D, Lim Y, Roh HJ, Kim S, Park CI, et al. Co-Expression Network Analysis of Spleen Transcriptome in Rock Bream (Oplegnathus fasciatus) Naturally Infected with Rock Bream Iridovirus (RBIV). Int J Mol Sci (2020) 21(5):1707. doi: 10.3390/ijms21051707
35. Livak KJ, Schmittgen TD. Analysis of relative gene expression data using real-time quantitative PCR and the 2(-Delta Delta C(T)) Method. Methods (2001) 25(4):402–8. doi: 10.1006/meth.2001.1262
36. Li K, Wang SW, Li Y, Martin RE, Li L, Lu M, et al. Identification and expression of a new type II transmembrane protein in human mast cells. Genomics (2005) 86(1):68–75. doi: 10.1016/j.ygeno.2005.03.006
37. Chen JX, Xu X, Zhang S. Silence of long noncoding RNA NEAT1 exerts suppressive effects on immunity during sepsis by promoting microRNA-125-dependent MCEMP1 downregulation. IUBMB Life (2019) 71(7):956–68. doi: 10.1002/iub.2033
38. Chlenski A, Guerrero LJ, Salwen HR, Yang Q, Tian Y, Morales La Madrid A, et al. Secreted protein acidic and rich in cysteine is a matrix scavenger chaperone. PloS One (2011) 6(9):e23880. doi: 10.1371/journal.pone.0023880
39. Goldblum SE, Ding X, Funk SE, Sage EH. SPARC (secreted protein acidic and rich in cysteine) regulates endothelial cell shape and barrier function. Proc Natl Acad Sci U S A (1994) 91(8):3448–52. doi: 10.1073/pnas.91.8.3448
40. Alvarez MJ, Prada F, Salvatierra E, Bravo AI, Lutzky VP, Carbone C, et al. Secreted protein acidic and rich in cysteine produced by human melanoma cells modulates polymorphonuclear leukocyte recruitment and antitumor cytotoxic capacity. Cancer Res (2005) 65(12):5123–32. doi: 10.1158/0008-5472.Can-04-1102
41. Riley HJ, Bradshaw AD. The Influence of the Extracellular Matrix in Inflammation: Findings from the SPARC-Null Mouse. Anat Rec (Hoboken) (2020) 303(6):1624–9. doi: 10.1002/ar.24133
42. Rotta G, Matteoli G, Mazzini E, Nuciforo P, Colombo MP, Rescigno M. Contrasting roles of SPARC-related granuloma in bacterial containment and in the induction of anti-Salmonella typhimurium immunity. J Exp Med (2008) 205(3):657–67. doi: 10.1084/jem.20071734
43. Toba H, de Castro Brás LE, Baicu CF, Zile MR, Lindsey ML, Bradshaw AD. Secreted protein acidic and rich in cysteine facilitates age-related cardiac inflammation and macrophage M1 polarization. Am J Physiol Cell Physiol (2015) 308(12):C972–82. doi: 10.1152/ajpcell.00402.2014
44. Chen WH, Cross AS, Edelman R, Sztein MB, Blackwelder WC, Pasetti MF. Antibody and Th1-type cell-mediated immune responses in elderly and young adults immunized with the standard or a high dose influenza vaccine. Vaccine (2011) 29(16):2865–73. doi: 10.1016/j.vaccine.2011.02.017
45. Nakaya HI, Clutterbuck E, Kazmin D, Wang L, Cortese M, Bosinger SE, et al. Systems biology of immunity to MF59-adjuvanted versus nonadjuvanted trivalent seasonal influenza vaccines in early childhood. Proc Natl Acad Sci U S A (2016) 113(7):1853–8. doi: 10.1073/pnas.1519690113
46. Patel SS, Bizjajeva S, Lindert K, Heijnen E, Oberye J. Cumulative clinical experience with MF59-adjuvanted trivalent seasonal influenza vaccine in young children. Int J Infect Dis (2019) 85S:S26–38. doi: 10.1016/j.ijid.2019.05.009
47. Herati RS, Reuter MA, Dolfi DV, Mansfield KD, Aung H, Badwan OZ, et al. Circulating CXCR5+PD-1+ response predicts influenza vaccine antibody responses in young adults but not elderly adults. J Immunol (Baltimore Md 1950) (2014) 193(7):3528–37. doi: 10.4049/jimmunol.1302503
48. García-Sastre A. Systems vaccinology informs influenza vaccine immunogenicity. Proc Natl Acad Sci U S A (2016) 113(7):1689–91. doi: 10.1073/pnas.1525361113
49. Lambert ND, Ovsyannikova IG, Pankratz VS, Jacobson RM, Poland GA. Understanding the immune response to seasonal influenza vaccination in older adults: a systems biology approach. Expert Rev Vaccines (2012) 11(8):985–94. doi: 10.1586/erv.12.61
50. Klein SL, Marriott I, Fish EN. Sex-based differences in immune function and responses to vaccination. Trans R Soc Trop Med Hyg (2015) 109(1):9–15. doi: 10.1093/trstmh/tru167
51. Luo Z, Zhou Y, Luo P, Zhao Q, Xiao N, Yu Y, et al. SPARC deficiency affects bone marrow stromal function, resulting in impaired B lymphopoiesis. J Leukoc Biol (2014) 96(1):73–82. doi: 10.1189/jlb.1A0713-415RR
52. Rempel SA, Hawley RC, Gutierrez JA, Mouzon E, Bobbitt KR, Lemke N, et al. Splenic and immune alterations of the Sparc-null mouse accompany a lack of immune response. Genes Immun (2007) 8(3):262–74. doi: 10.1038/sj.gene.6364388
53. Raman K, O’Donnell MJ, Czlonkowska A, Duarte YC, Lopez-Jaramillo P, Penaherrera E, et al. Peripheral Blood MCEMP1 Gene Expression as a Biomarker for Stroke Prognosis. Stroke (2016) 47(3):652–8. doi: 10.1161/STROKEAHA.115.011854
54. Strbian D, Karjalainen-Lindsberg ML, Tatlisumak T, Lindsberg PJ. Cerebral mast cells regulate early ischemic brain swelling and neutrophil accumulation. J Cereb Blood Flow Metab (2006) 26(5):605–12. doi: 10.1038/sj.jcbfm.9600228
55. Xie W, Chen L, Chen L, Kou Q. Silencing of long non-coding RNA MALAT1 suppresses inflammation in septic mice: role of microRNA-23a in the down-regulation of MCEMP1 expression. Inflammation Res (2020) 69(2):179–90. doi: 10.1007/s00011-019-01306-z
56. Boultwood J, Strickson AJ, Jabs EW, Cheng JF, Fidler C, Wainscoat JS. Physical mapping of the human ATX1 homologue (HAH1) to the critical region of the 5q- syndrome within 5q32, and immediately adjacent to the SPARC gene. Hum Genet (2000) 106(1):127–9. doi: 10.1007/s004399900215
57. Wang B, Chen K, Xu W, Chen D, Tang W, Xia TS. Integrative genomic analyses of secreted protein acidic and rich in cysteine and its role in cancer prediction. Mol Med Rep (2014) 10(3):1461–8. doi: 10.3892/mmr.2014.2339
58. Inoue M, Senju S, Hirata S, Ikuta Y, Hayashida Y, Irie A, et al. Identification of SPARC as a candidate target antigen for immunotherapy of various cancers. Int J Cancer (2010) 127(6):1393–403. doi: 10.1002/ijc.25160
59. Chang CH, Yen MC, Liao SH, Hsu YL, Lai CS, Chang KP, et al. Secreted Protein Acidic and Rich in Cysteine (SPARC) Enhances Cell Proliferation, Migration, and Epithelial Mesenchymal Transition, and SPARC Expression is Associated with Tumor Grade in Head and Neck Cancer. Int J Mol Sci (2017) 18(7):1556. doi: 10.3390/ijms18071556
60. Bradshaw AD. Diverse biological functions of the SPARC family of proteins. Int J Biochem Cell Biol (2012) 44(3):480–8. doi: 10.1016/j.biocel.2011.12.021
61. Tanaka M, Takagi T, Naito Y, Uchiyama K, Hotta Y, Toyokawa Y, et al. Secreted protein acidic and rich in cysteine functions in colitis via IL17A regulation in mucosal CD4(+) T cells. J Gastroenterol Hepatol (2018) 33(3):671–80. doi: 10.1111/jgh.13842
62. McElhaney JE, Gentleman B. Cell-Mediated Immune Response to Influenza Using Ex Vivo Stimulation and Assays of Cytokine and Granzyme B Responses. Methods Mol Biol (Clifton NJ) (2015) 1343:121–41. doi: 10.1007/978-1-4939-2963-4_11
63. Ramakrishnan A, Althoff KN, Lopez JA, Coles CL, Bream JH. Differential serum cytokine responses to inactivated and live attenuated seasonal influenza vaccines. Cytokine (2012) 60(3):661–6. doi: 10.1016/j.cyto.2012.08.004
64. Lee N, Wong CK, Chan PK, Chan MC, Wong RY, Lun SW, et al. Cytokine response patterns in severe pandemic 2009 H1N1 and seasonal influenza among hospitalized adults. PloS One (2011) 6(10):e26050. doi: 10.1371/journal.pone.0026050
65. Shahid Z, Kleppinger A, Gentleman B, Falsey AR, McElhaney JE. Clinical and immunologic predictors of influenza illness among vaccinated older adults. Vaccine (2010) 28(38):6145–51. doi: 10.1016/j.vaccine.2010.07.036
66. Kovats S. Estrogen receptors regulate innate immune cells and signaling pathways. Cell Immunol (2015) 294(2):63–9. doi: 10.1016/j.cellimm.2015.01.018
Keywords: weighted gene co-expression network analysis (WGCNA), hub genes, quadrivalent inactivated influenza vaccines (QIVs), MCEMP1, SPARC, reactogenicity
Citation: Yang J, Zhang J, Fan R, Zhao W, Han T, Duan K, Li X, Zeng P, Deng J, Zhang J and Yang X (2020) Identifying Potential Candidate Hub Genes and Functionally Enriched Pathways in the Immune Responses to Quadrivalent Inactivated Influenza Vaccines in the Elderly Through Co-Expression Network Analysis. Front. Immunol. 11:603337. doi: 10.3389/fimmu.2020.603337
Received: 08 September 2020; Accepted: 06 November 2020;
Published: 04 December 2020.
Edited by:
Bertrand Kaeffer, Institut National de Recherche pour l’agriculture, l’alimentation et l’environnement (INRAE), FranceReviewed by:
John F. Alcorn, University of Pittsburgh, United StatesCopyright © 2020 Yang, Zhang, Fan, Zhao, Han, Duan, Li, Zeng, Deng, Zhang and Yang. This is an open-access article distributed under the terms of the Creative Commons Attribution License (CC BY). The use, distribution or reproduction in other forums is permitted, provided the original author(s) and the copyright owner(s) are credited and that the original publication in this journal is cited, in accordance with accepted academic practice. No use, distribution or reproduction is permitted which does not comply with these terms.
*Correspondence: Xiaoming Yang, eWFuZ3hpYW9taW5nenNAaG90bWFpbC5jb20=
Disclaimer: All claims expressed in this article are solely those of the authors and do not necessarily represent those of their affiliated organizations, or those of the publisher, the editors and the reviewers. Any product that may be evaluated in this article or claim that may be made by its manufacturer is not guaranteed or endorsed by the publisher.
Research integrity at Frontiers
Learn more about the work of our research integrity team to safeguard the quality of each article we publish.