- 1Department of Medicine, Surgery and Dentistry Scuola Medica Salernitana, University of Salerno, Salerno, Italy
- 2Pulmonology, Department of Biomedical Sciences, Dentistry and Morphological and Functional Imaging (BIOMORF), University of Messina, Messina, Italy
- 3Department of Medicine, Johns Hopkins University School of Medicine, Baltimore, MD, United States
Background: Posttranscriptional gene regulation (PTGR) contributes to inflammation through alterations in messenger RNA (mRNA) turnover and translation rates. RNA-binding proteins (RBPs) coordinate these processes but their role in lung inflammatory diseases is ill-defined. We evaluated the expression of a curated list of mRNA-binding RBPs (mRBPs) in selected Gene Expression Omnibus (GEO) transcriptomic databases of airway epithelium isolated from chronic obstructive pulmonary disease (COPD), severe asthma (SA) and matched control subjects, hypothesizing that global changes in mRBPs expression could be used to infer their pathogenetic roles and identify novel disease-related regulatory networks.
Methods: A published list of 692 mRBPs [Nat Rev Genet 2014] was searched in GEO datasets originated from bronchial brushings of stable COPD patients (C), smokers (S), non-smokers (NS) controls with normal lung function (n = 6/12/12) (GEO ID: GSE5058) and of (SA) and healthy control (HC) (n = 6/12) (GSE63142). Fluorescence intensity data were extracted and normalized on the medians for fold change (FC) comparisons. FCs were set at ≥ |1.5| with a false discovery rate (FDR) of ≤ 0.05. Pearson correlation maps and heatmaps were generated using tMEV tools v4_9_0.45. DNA sequence motifs were searched using PScan-ChIP. Gene Ontology (GO) was performed with Ingenuity Pathway Analysis (IPA) tool.
Results: Significant mRBP expression changes were detected for S/NS, COPD/NS and COPD/S (n = 41, 391, 382, respectively). Of those, 32% of genes changed by FC ≥ |1.5| in S/NS but more than 60% in COPD/NS and COPD/S (n = 13, 267, 257, respectively). Genes were predominantly downregulated in COPD/NS (n = 194, 73%) and COPD/S (n = 202, 79%), less so in S/NS (n = 4, 31%). Unsupervised cluster analysis identified in 4 out of 12 S the same mRBP pattern seen in C, postulating subclinical COPD. Significant DNA motifs enrichment for transcriptional regulation was found for downregulated RBPs. Correlation analysis identified five clusters of co-expressed mRBPs. GO analysis revealed significant enrichments in canonical pathways both specific and shared among comparisons. Unexpectedly, no significant mRBPs modulation was found in SA compared to controls.
Conclusions: Airway epithelial mRBPs profiling reveals a COPD-specific global downregulation of RBPs shared by a subset of control smokers, the potential of functional cooperation by coexpressed RBPs and significant impact on relevant pathogenetic pathways in COPD. Elucidation of PTGR in COPD could identify disease biomarkers or pathways for therapeutic targeting.
Introduction
RNA-binding proteins (RBPs) are key regulatory factors in post-transcriptional gene regulation (PTGR) involved in the maturation, stability, transport and degradation of cellular RNAs. RBP convey PTGR by binding to conserved regulatory elements shared by subsets of transcripts and by directing the bound targets toward cytoplasmic sites of translation or decay (1). Importantly, RBPs exert their function as part of ribonucleoprotein (mRNP) complexes, constituted by proteins and non-coding RNAs, such as microRNAs (2). Through stimulus-dependent cues, changes in mRBP composition ultimately determine the rate of target mRNA stability and translation. Therefore, understanding RBP function in disease models requires a larger evaluation of co-expression and regulatory scenarios, shaped by disease-driven triggers and signaling.
For human cancer the occurrence of aberrant RBP expression, along with altered RNA turnover and translation rates have been characterized in preclinical models, identified in human disease and further probed with in silico approaches (3, 4) leading to the identification of this class of regulators as novel disease biomarkers and as targets for small molecule-based therapeutics (5–7). Similar studies in human chronic inflammatory diseases are lagging behind, despite ample knowledge of deregulated PTGR in inflammatory responses by in vitro studies and the strong inflammatory phenotypes obtained in some of the knock-out animal models (8, 9). This knowledge chasm is also present for lung diseases, despite the strong link between chronic inflammatory diseases such as Chronic Obstructive Pulmonary Disease (COPD) and some lung cancer types (10) and the extensive overlap of RBP-regulated genes contributing to both disease process (11).
Airway epithelium is a major driver in the pathogenesis of inflammatory lung diseases such as asthma and COPD. Deregulated epithelial responses are a main therapeutic target of inhaled corticosteroids (ICS), the mainstay anti-inflammatory drug class for asthma symptom control (www.ginasthma.org) and for treating exacerbations in COPD (www.goldcopd.org). Involvement of RBPs in airway epithelial responses to inflammatory stimuli and glucocorticoid treatment has been well-characterized in vitro (12–15) but awaits evidence from patient-based studies. To this end, in a previous characterization of ARE-binding proteins expression in COPD (16) we identified a selective loss of the RBP AU-binding Factor (AUF)−1 in bronchial biopsies in stable COPD patients vs. smoker controls. Besides replicating this finding in vitro upon challenge of the epithelial cell line BEAS-2B with inflammatory cytokines and cigarette smoke extract, we confirmed this loss in primary epithelium, by interrogating a transcriptome database generated from bronchial brushings of COPD patients vs. normal smokers and non-smokers controls (17).
On these basis, we hypothesized that changes in mRNA-binding RBPs (mRBP) expression may occur on a larger scale in chronic inflammatory airways diseases, such as COPD and severe asthma (SA) and that identification of these changes can be used to infer their putative pathogenetic roles as disease-related regulatory networks, as in cancer (18). We therefore set out to evaluate the expression profile of a curated gene list of mRBPs in selected public Gene Expression Omnibus (GEO) transcriptomic databases derived from airway epithelium isolated from bronchial brushings of phenotyped patients affected by COPD, SA and relative control populations, enrolled in relevant translational studies on disease pathophysiology. In particular, we employed for COPD the same database (17) in which we found loss of AUF-1 in COPD samples vs. controls, as observed ex vivo in COPD airway biopsies (16) and a GEO database from SA and healthy controls (HC) enrolled in the Severe Asthma Research Program (SARP) study (19). The list of mRBPs we utilized for this study has been created by annotation of proteins as RBPs generated by domain search, considering known RNA binding domains [among 800 domains extracted from the protein family (Pfam) database], or including proteins known as validated partners of RNP complex (20). The mRBP expression profiles we obtained were then evaluated through gene ontology analysis to evaluate their potential relevance to disease pathogenesis; moreover, we searched for coregulated RBP expression indicative of common participation to regulatory pathways.
In airway epithelial samples from COPD patients, this approach identified a global downregulation of RBP expression that was shared by a subset of smoker control subjects; changes in mRBP expression impacted several biological pathways also involved in several aspects of COPD pathogenesis; finally, at least five groups of coregulated RBPs were identified. Importantly, airway epithelial mRBP expression was found to be much less regulated in patients with SA.
Materials and Methods
Sample Selection and Data Processing
We selected the following two trascriptomic databases generated from human airway epithelial cells obtained by bronchial brushings, downloaded from the GEO public repository of high-throughput gene expression data (Figure 1A):
• GEO ID: GSE5058. COPD patients (C), Smokers (S) and Non-Smokers (NS) as controls with normal al lung function (NLF) (n = 6/12/12, respectively) (17);
• GEO ID: GSE63142. Patients with severe Asthma (SA), healthy control subjects (HC) (n = 6/12, respectively, randomly selected from databes to match number of cases considered in GSE5058) (19).
• GEO ID: GSE8545. Patients with stable COPD (C), Smokers (S) and Non-Smokers (NS) as controls with normal lung function (NLF) (n = 18/18/18, respectively) (21). This dataset has been utilized to validate the RBP expression profile in COPD/S identified in GSE5058 (Figure 8).
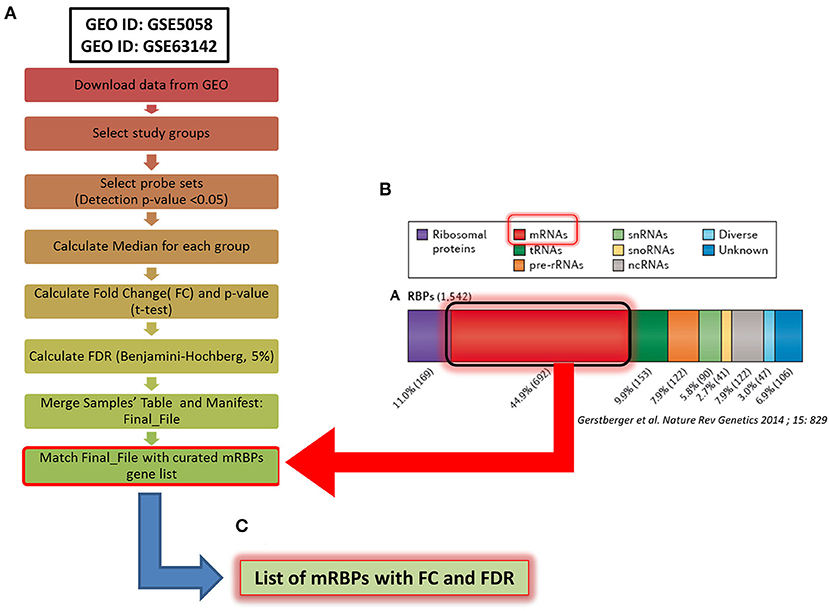
Figure 1. Methodological flowchart of the study for expression profiling of mRNA-binding proteins (mRBPs) in airway epithelium transcriptomic studies in COPD and severe asthma (SA). (A) Flowchart for data analysis of the trascriptomic datasets obtained in the Gene Expression Omnibus (GEO) public database (see Methods). Briefly, fluorescence intensity data (raw data) from the chosen datasets (NS, S, stable COPD for GSE5058; SA and HC for GSE63142) were extracted and processed to calculate the probes fluorescence compared to the background. Probes with at least one detection p < 0.05 in all three groups were considered for further analysis. Data were then normalized by the median to calculate fold change (FC) expression among groups (S/NS, COPD/NS, COPD/S; SA/HC); only those showing a False Discovery Rate (FDR) ≤ 0.05 in each comparison were considered, forming a Final File for each GSE. Concurrently, a curated gene list of 692 RBPs binding to mRNA (mRBPs) was downloaded from a published census of RNA-binding proteins (20) (B) and searched in the Final File, producing a dataset of mRBPs with statistically significant fold changes in disease state vs. controls (C).
The Affymetrix Human Genome U133 Plus 2.0 Array platform was used for both COPD studies while the SA study was performed using Agilent-014850 Whole Human Genome Microarray 4x44K G4112F. Fluorescence intensity data from individual datasets (raw data) were extracted and processed applying the standard Affimetrix MAS5 algorithm to calculate the fluorescence of the single probes compared to the background. Probes with at least one detected p < 0.05 in all three groups were considered for further analysis. Data were then normalized by the median to calculate fold change (FC) expression among groups; only those showing a False Discovery Rate (FDR) ≤ 0.05 in each comparison were considered. As genes are represented on the array platforms by multiple probes spanning different transcript portions, only genes with consistency across probes ≥ 67% were considered for final analysis. The curated gene list of 692 RBPs binding to mRNA (mRBPs) published in a general census of RNA-binding proteins was downloaded from the original file (https://www.nature.com/articles/nrg3813#MOESM25, Supplemetary Information S3) (20) and searched in the final FC files (Figure 1B), producing a list of mRBPs regulated in disease state vs. controls (Figure 1C). The analysis first identified statistically significant genes regardless of fold change value, which were denominated Differentially Expressed Genes (DEG). Then, FCs threshold was set at ≥ |1.5| for identification of genes denominated Significant FC/Differentially Expressed Genes (SDEG) as in standard array analysis.
For the validation analysis, COPD and S data (n = 18/18) were extracted from GSE8545 dataset. The GEO2R tool was used for FC data analysis (22).
Generation of Venn diagram analysis for overlapping/unique gene lists was performed using Venny 2.1 (23). Pearson correlation matrix generation was produced using R version 3.6.2. Pearson correlation maps for mRBPs expression changes and heatmaps were generated using tMEV tools v4_9_0.45 (24, 25).
Enrichment for DNA sequence motifs for transcription factors binding sites, according to motif descriptors in the JASPAR database, was identified using PScanChIP (26) with the following parameters: “Organism: Homo Sapiens,” “Assembly: hg38,” “Background: Promoters,” “Descriptors: Jaspar 2018 NR.” Promoter regions have been calculated as the range from +1,500 bp upstream to −500 bp downstream of the transcription start site (TSS) of all the submitted gene list. Only over-represented motifs with p ≤ 0.05 were considered.
Gene Ontology (GO)
GO analysis was performed with Ingenuity Pathway Analysis (IPA) software on microarray probes of RBPs identified as DEG. The significance values for the canonical pathways is calculated by Fisher's exact test right-tailed. The prediction of activation or inhibition of Canonical Pathways was calculated by z-score (27) as follows:
Statistical Analysis
Statistical analysis was performed using GraphPad Prism 5 (GraphPad Software Inc.).
Results
Expression Profile of mRBPs in Airway Epithelial Transcriptomic Database of Patients With Stable COPD Compared to Non-smoker and Smoker Control Subjects
The mRBPs gene list was searched in the GSE5058 dataset to identify disease-dependent mRBP gene expression in patients with established COPD compared to control groups, clinically phenotyped as shown (Figure 2A). Comparisons of expression levels for the smoking control group vs. non-smoking control group dataset (S/NS) and of COPD patients vs. both NS and S group datasets (COPD/NS, COPD/S) were performed. The number of regulated mRBP genes is reported along with corresponding array probes, which detect different fragments of each gene sequence and generate the fluorescence intensity raw data. We first calculated regulated genes with statistically significant changes regardless of fold change (FC) value, defined as Differentially Expressed Genes (DEG) (Figure 2B). These data revealed an overall greater expression of mRBPs in COPD patients vs. both NS and S control groups, about 9-fold higher than that triggered by smoke exposure alone. The mRBP genes displaying a statistically significant FC value ≥|1.5|, denominated Significant FC/Differentially Expressed Genes (SDEG) were further selected (Figure 2C). Approximately 70% of the mRBP DEG genes were included in this category when COPD samples were compared to both control samples (COPD/NS, COPD/S), while only 30% of S/NS DEG genes were upregulated over this threshold. A small number of genes were excluded from further computing for probe discordance ≥ 67% (n = 30/267, 11% for COPD/NS; 10/257 for COPD/S, 3%). The SDEG profiles indicate that the majority of mRBP genes in COPD were downregulated compared to both controls (n = 194/267, 73% for COPD/NS; n = 202/257, 79% for COPD vs. S) while in S/NS only 30% (n = 4/13) were downregulated (Figure 3). Table 1 lists the main functions, published or described in GeneCards (80) for mRBP SDEG with the highest numerical FC value in COPD vs. both control groups.
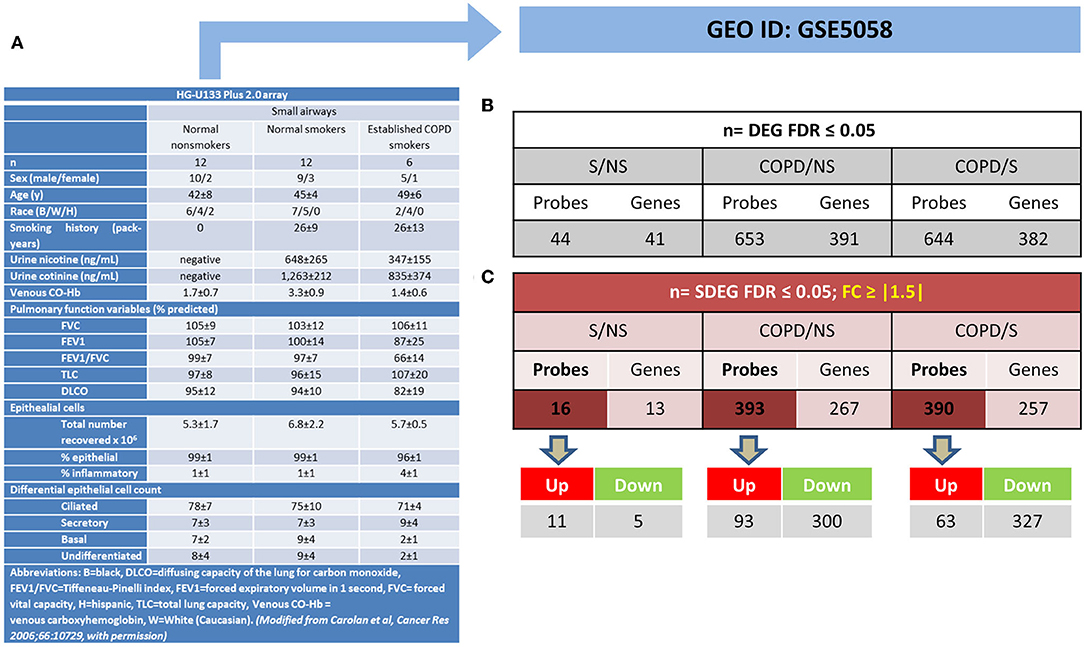
Figure 2. Regulated mRBP expression in Small Airway Epithelial Transcriptomics in stable COPD. (A) Clinical and spirometric phenotyping of COPD smoker patients (COPD), non-smokers (NS), and smokers (S) with normal lung function (NLF) as control cohorts providing small airway epithelial cells by bronchial brushings for trascriptomic analysis reported in GEO GSE5058 [modified from (17) with permission] utilized in this study for mRBP expression analysis. (B,C) For each gene, multiple probes spanning different gene regions are represented on array platforms. Panels show the numbers of mRBP probes and corresponding genes obtained after FDR filtering (see Figure 1A). (B) Statistically significant, Differentially Expressed mRBP Genes, regardless of fold change value (DEG) and (C). Statistically Significant/Differential Expressed mRBP Genes with differential expression set at ≥ |1.5) (SDEG) obtained comparing datasets from S vs. NS, COPD vs. S and vs. NS. Number of Up- or down-regulated SDEG probes are shown below.
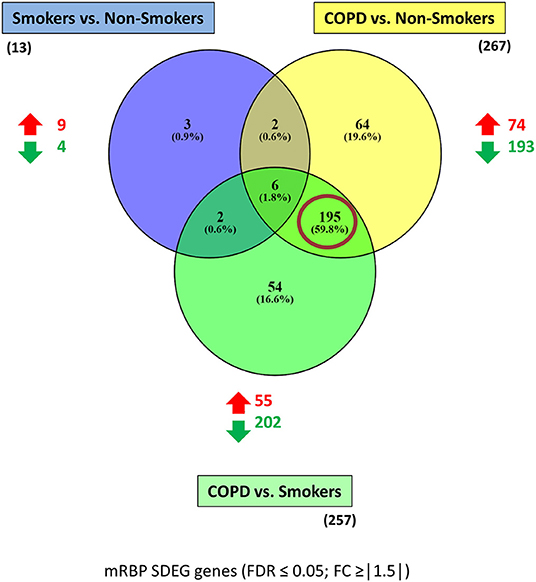
Figure 3. mRBP SDEG genes in small airway epithelium of stable COPD patients vs. non-smoking and smoking control subjects: unique and overlapping regulated expression. Venn diagram generated with Venny 2.1 (23), showing selective and shared mRBPs SDEG genes (FDR ≤ 0.05; FC ≥ |1.5|) among the three comparisons. The number of up-and down-regulated mRBPs for each comparison are shown by red/green arrows; the total number is indicated in parenthesis. Red circle highlights the predominant number of SDEG differentially expressed in COPD, regardless the smoking status of controls.
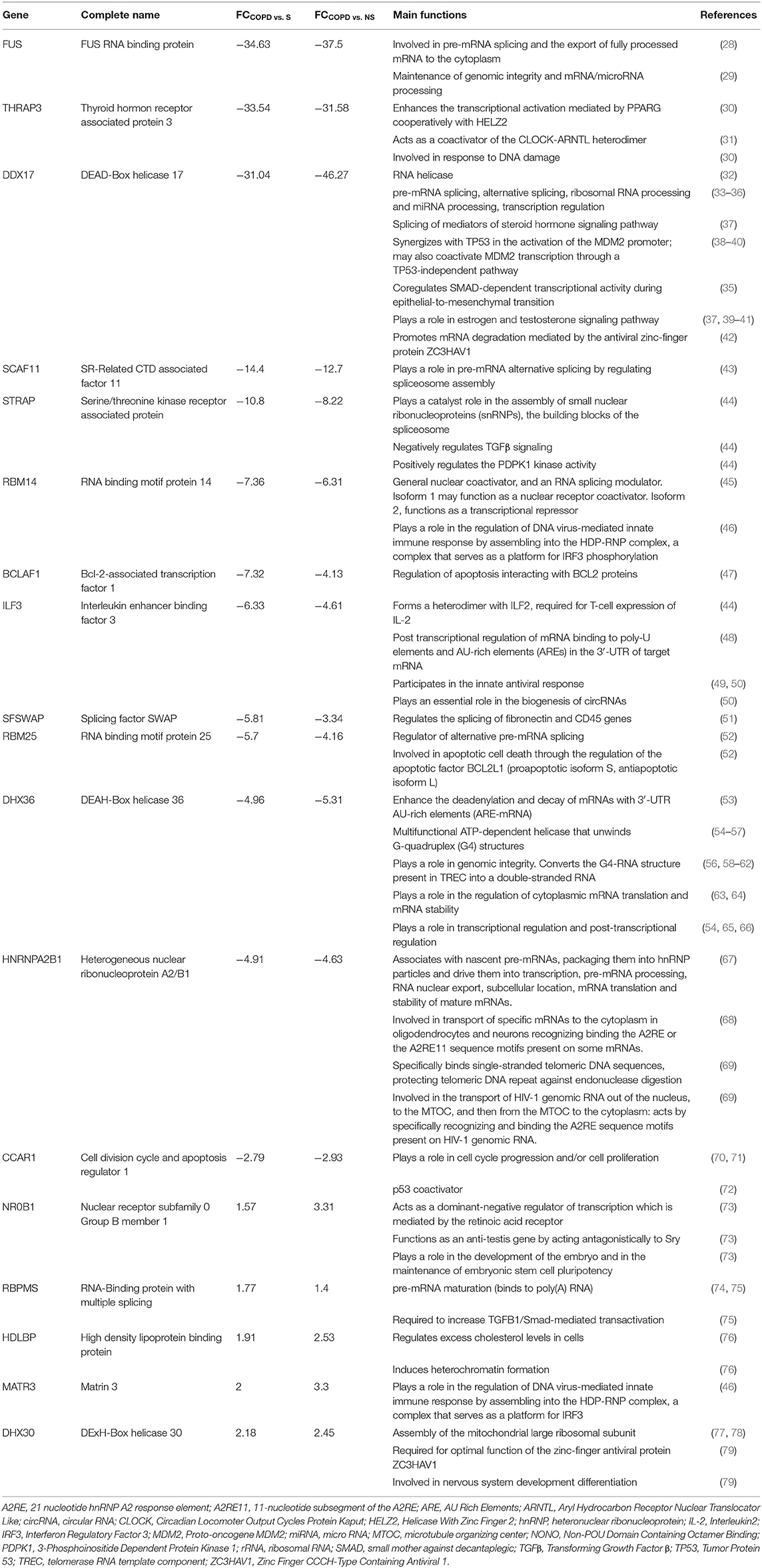
Table 1. Regulated RBPs in COPD vs. normal non-smokers and smokers: selected list with known functions.
The three gene groups (NS/S, COPD/NS, COPD/S) were intersected to identify both unique and overlapping mRBP expression profiles. As shown in the Venn's diagram (Figure 3), 195 SDEG genes are shared between the COPD patients vs. both NS and S groups, pointing at a distinctive mRBP signature driven by COPD beyond the active exposure to cigarette smoke.
Using the larger DEG lists, the three gene sets were then analyzed by IPA software probing changes in the categories of canonical pathways and intersected, (Figure 4) as done for the SDEG gene groups. Five out of eight canonical pathways impacted by COPD were shared by comparisons to NS and S groups (Figure 4A). Calculation of the z-score parameter yielded a predictive assessment of the downstream effect - activation or inactivation - exerted by the identified RBP profile on the metabolic pathways (Figure 4B). Table 2 lists the regulated mRBPs impacting upon the canonical pathways shown in Figure 4B.
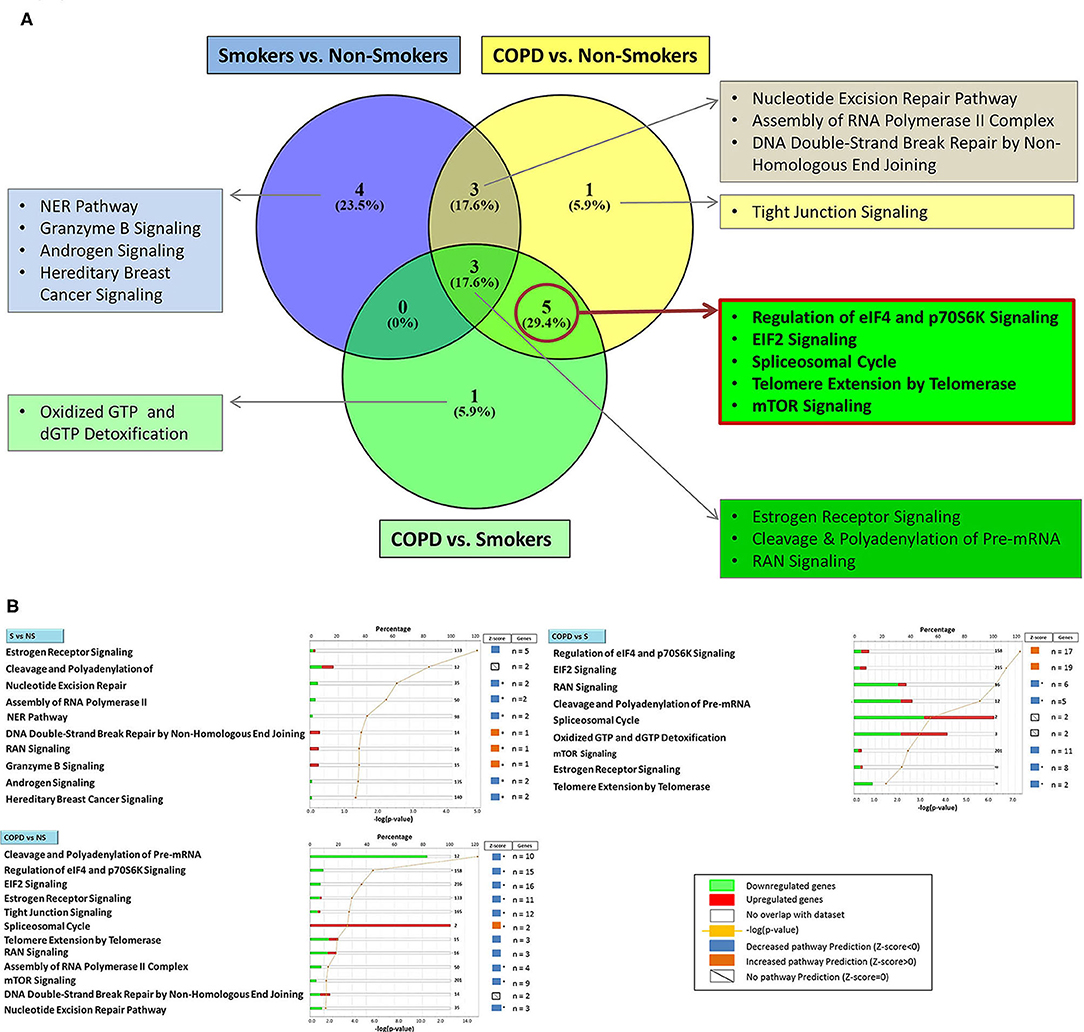
Figure 4. Genome Ontology analysis of mRBPs expression in small airway epithelium of stable COPD patients vs. non-smoking and smoking control subjects: involvement in established COPD pathogenic pathways. Ingenuity Pathway Analysis (IPA) of mRBP in COPD GSE5058 Dataset. (A) Venn diagram showing selective and shared canonical pathways among the group comparisons, calculated by Ingenuity Pathway Analysis (IPA) on DEGs for increased statistical power and listed as in Figure 3. In evidence (red lined rectangle) the pathways enriched in COPD vs. both NS and S control groups. (B) List of canonical pathways identified for each group comparisons. For each pathway, bargraphs indicate the number of RBP genes associated with the pathway (total number listed at the end of the bar) that were found up (red)- or down (green)-regulated in the dataset; z-score (on the right) predicts pathway repression (blue) or activation (orange) given by the expression profile (see Methods); far right: genes n = indicates number of regulated mRBP genes involved for each pathway (also listed in Table 2). * z-score ≥ |1|.
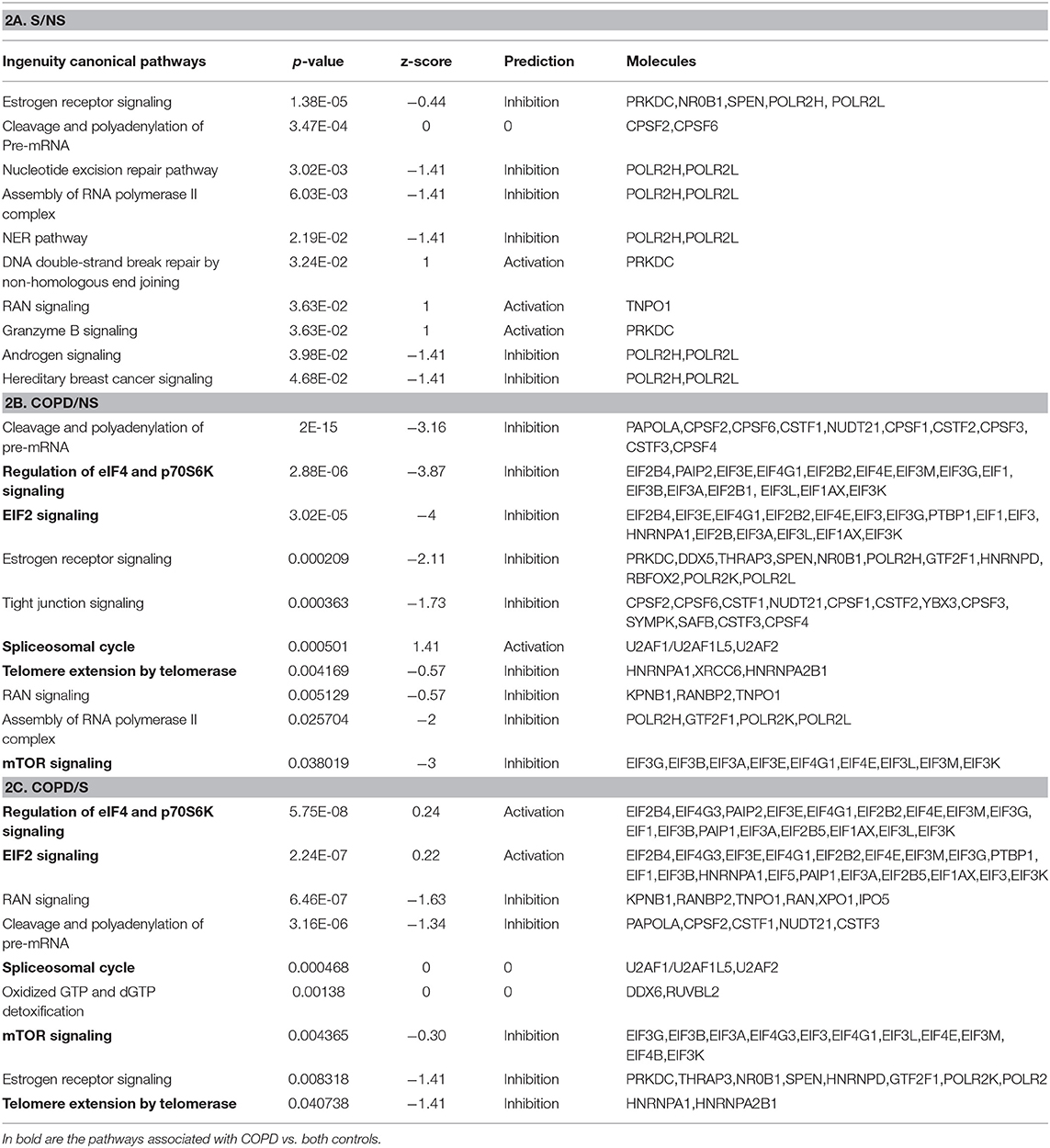
Table 2. GO analysis by IPA indicating canonical pathways in which RBP enrichment is significant for each comparison, with predicted functional outcome indicated by z-score (See Methods for details), and RBP molecules involved.
We then selected the gene set obtained for the comparison COPD/S (n = 409 SDEG probes) to perform an unsupervised clustering analysis of each subject's mRBP expression profile for the three groups (NS, S, COPD), using Pearson's hierarchical clusters/complete linkage method. Results were represented in a heatmap generated with the T-MeV software (Figure 5) (24, 25). As expected, the heatmap clearly showed how the expression of mRBPs appears predominantely diminished compared to NS and most of S subjects; interestingly, it also identified in the S group a subset of four subjects displaying an expression profile highly homologous to the one identified in COPD patients (Figure 5A). This similarity was confirmed by performing unsupervised cluster analysis for both genes and subjects using an Euclidean distance metrics (Figure 5B). This analysis confirmed that the RBP expression profile of this S subgroup indeed clustered with the samples from COPD patients.
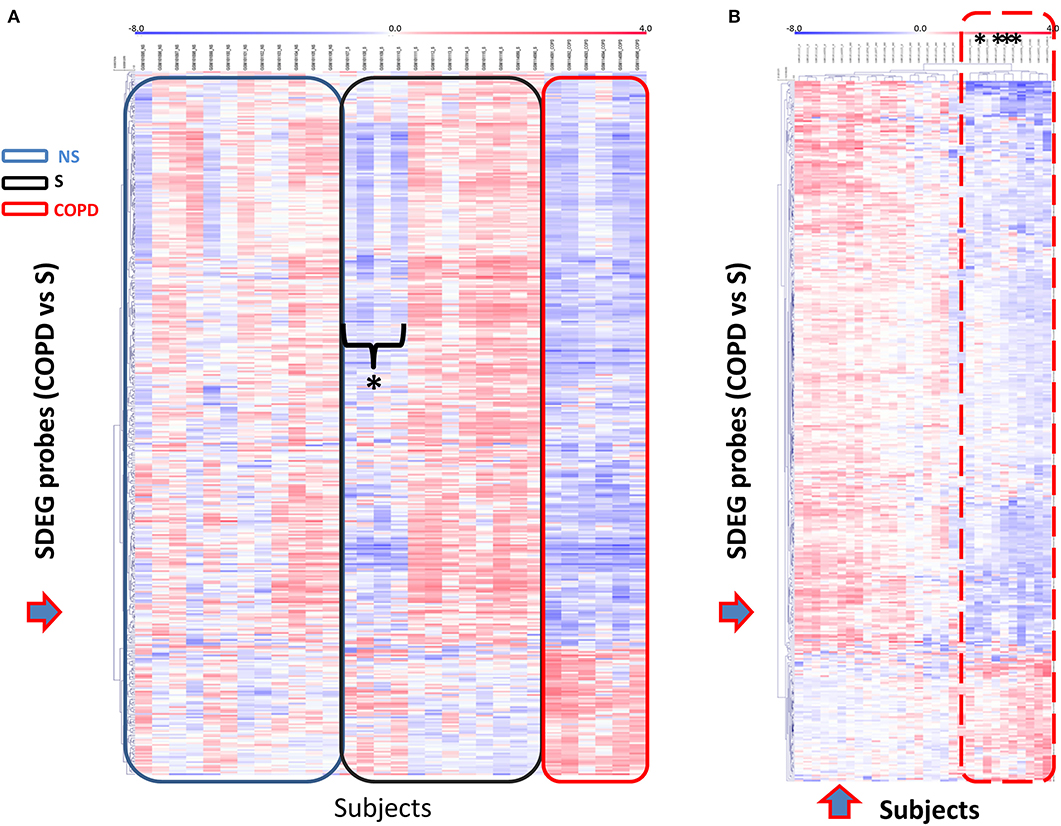
Figure 5. Unsupervised gene cluster analysis across the individual samples from GSE5058 dataset identifies selective global mRBPs repression in COPD patients shared by a subset of smoker controls. (A) Unsupervised clustering analysis applied to (blue arrow) SDEG probe list identified in COPD/S (n = 409). Heatmap shows SDEG probes' fluorescence intensity value (blue < 0, reduced: red > 0, increased). The data were normalized on the median and log2-trasformed for relative fold changes (See Methods). (B) Unsupervised clustering analysis applied to both SDEG probe list and individual samples (blue arrows). Asterisks indicate the SDEG profiles of four smokers with NLF, clustering with those of COPD patients indicated by the dotted line.
To initiate mechanistic understanding of predominant RBP downregulation in subjects with stable COPD and in selected smokers with normal lung function, we conducted for the RBP gene set obtained for COPD/S a search for transcription factor (TF) binding motif within promoter regions. Significant binding site enrichment for several TFs (Figure 6, listed in Supplementary Table 1) was exclusively found for the genes downregulated in their expression.
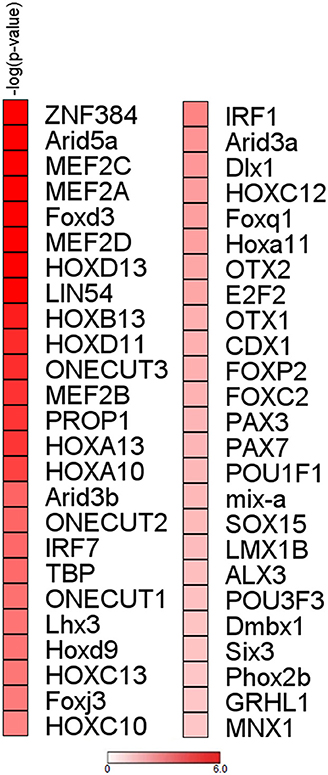
Figure 6. Analysis of upstream regions of mRBP genes regulated in COPD/S identifies enrichment of transcription factors binding sites for downregulated genes. Enrichment analysis of transcription factor binding sites for COPD/S SDEG gene list (n = 257) within the promoter regions orderd by -log(p-value). Promoter regions have been calculated as the range from +1,500 bp upstream to −500 bp downstream of the gene transcription start site (TSS). Only over-represented motifs with p ≤ 0.05 were considered.
As mRBPs exert their function by dinamically assembling in RNP complexes, the same gene dataset (SDEG in COPD/S) was then searched for RBPs with correlated expression, which may indicate disease-driven, coordinated target regulation (20). Pearson correlation map showed at least five highly correlated mRBP clusters (r ≥ 0.70) (Figure 7A). Clusters of RBP gene lists and their FC values between COPD and both S/NS control populations are listed in Supplementary Table 2. Canonical pathway analysis indicated that RBP genes in clusters commonly impacted upon RAN signaling and Telomere Extension by Telomerase, along with more cluster- restricted influence on other pathways, such as inhibition of ARE-mediated mRNA degradation and IL-15 expression (Table 3). In particular, cluster 3 included 40 genes, among which was included HNRNPD, coding for the RBP AUF-1 that we previously identified as repressed in small airway epithelium in airway biopsies of an independent subject cohort of COPD patients compared to smoker controls (16). For cluster 3, IPA analysis identified enrichment of genes involved in RAN signaling, telomere extension, IL-15 expression (Figure 7B). Figure 7C shows normalized fold changes across the three groups for eight representative mRBPs, including HNRNPD, contained in cluster 3.
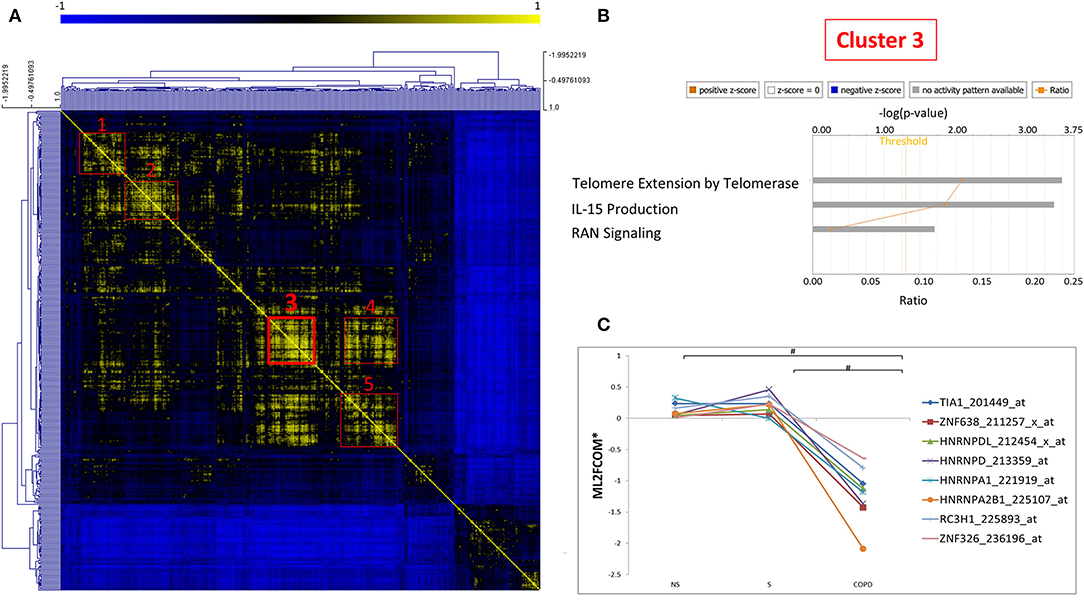
Figure 7. Correlation map identifies five clusters of coexpressed RBPs in COPD. (A) Pearson Correlation maps of SDEG probe list (n = 409 in COPD/S) across all samples, with R value set as (r ≥ 0.7) identifies at least five clusters of coexpressed mRBPs (red squares). (B) Representative GO analysis of cluster 3 (n = 42 SDEG) (full GO cluster analysis in Table 3). (C) Representative expression profile of selected mRBPs coexpressed in cluster 3 (full cluster expression profiles in Supplementary Table 2). #p ≤ 0.05. *Mean of Log2 (fold change over median).
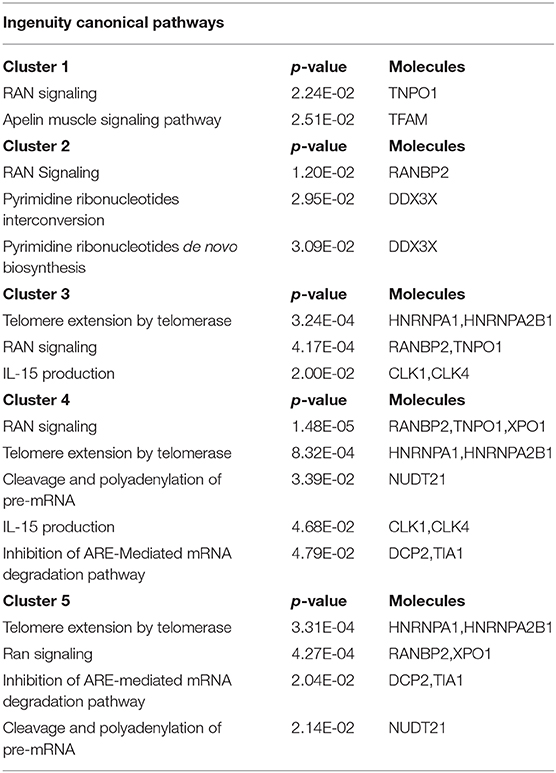
Table 3. Canonical Pathways identified by IPA analysis of mRBPs clusters 1 to 5 as shown in Figure 7.
We then submitted the mRBP profile obtained in the COPS/S, used also to identify gene clusters, to validation in an independent transcriptomic dataset of small airway epithelium obtained from bronchial brushing of NS, S and COPD (GOLD stages I or II) [n = 18 each cohort, deposited in public GEO repository as GSE 8545 (21). Analysis of COPD/S in GSE 8545 largely confirmed the global downregulation of RBP in COPD/S, with 56% of probe sets downregulated with an FC ≤ - 1.5 (217/390) vs. 80% (327/390) downregulated in GSE5058. Importantly, the comparison reproduced differential expression for all RBP cluster genes (listed in Table 3) identified by IPA as having significant impact on canonical pathways (Figure 8).
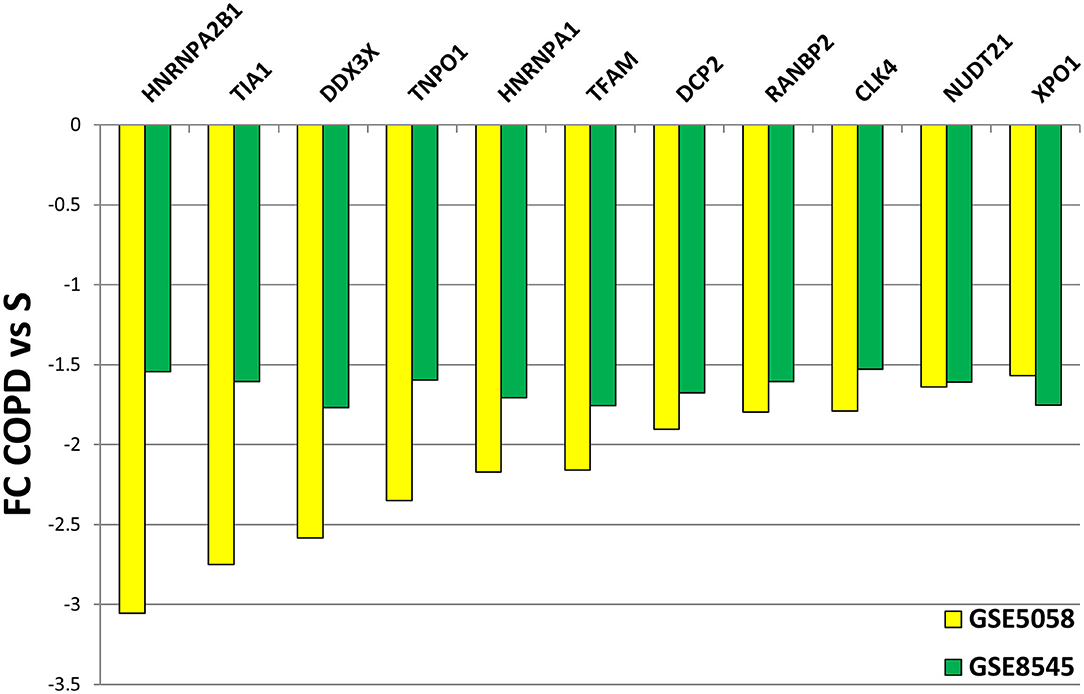
Figure 8. Validation of differential expression for RBP genes in GSE8545 COPD/S dataset. Comparison of FC values for COPD/S mRBP genes between GSE5058 (test dataset) and GSE8545 (validation dataset; See Methods) for the RBP cluster genes (listed in Table 3) identified by IPA as having significant impact on canonical pathways.
Expression Profile of mRBPs in Bronchial Epithelium of Patients With Severe Asthma Compared to Control Subjects
Transcriptomic data in airway epithelium from bronchial brushing of patients with severe asthma (SA) and healthy controls (HCs) were searched for mRBP expression using the same methodology (Figure 1). Clinical characteristics of the original study, from which we extracted GEO data only for SA and HC groups, are shown in Supplementary Table 3. We randomly extracted from the GSE63142 dataset (19) the same number of patients of the GSE5058 COPD dataset (n = 6 SA, n = 12 HCs). Only 30 probes (corresponding to 29 genes) were differentially expressed (DEG) in SA vs. HCs, but none of the DEG genes changed by at least 50% compared with HCs, thus none was categorized as SDEG (FDR ≤ 0.05; FC ≥ |1.5|). We then extended the search to the entire number of subjects of the datasets (n = 56 SA and 27 HCs) and identified as DEG 68 gene probes corresponding to 62 genes; as for the previous analysis, none of the DEG was categorizable as SDEG (genes listed in Supplementary Table 4).
Discussion
In the present study, global changes in mRBP gene expression in human airway epithelium were evaluated, for the first time, using public gene array databases derived from bronchial brushings of patients affected by two major chronic lung inflammatory diseases, COPD and severe asthma, and their relative control subjects. We report that significant changes were largely exclusive to samples from COPD and that they were mostly due to downregulated mRBP expression, a feature that was found also in a subset of control smokers. These changes were associated with the occurrence of several clusters of co-regulated mRBPs, and they impacted relevant pathways deemed pathogenic for COPD.
We recently identified in a GEO database of airway epithelial cells from stable COPD patients a significant loss of the RBP AUF-1 compared to smoker controls, matching the loss we identified at protein level in airway samples of patients with stable COPD compared to normal smokers (16). The present study was undertaken on this basis as a broader investigation of epithelial RBP patterns comparing two lung diseases characterized by chronic inflammation and oxidative stress – COPD and SA - since preclinical studies clearly identified the role of this class of posttranscriptional regulators in these pathological processes (14, 81, 82), yet they are scarcely described in human disease driven by them. This proof-of concept study could serve as springboard for studies focused on specific PTGR pathways and molecular species exploitable as phenotype/endotype disease biomarker or for therapeutic targetability.
To generate the list of mRBPs we used for this study, Gerstberger et al. (20) searched the human genome for protein-coding genes bearing RBDs using specific statistical probability models and futher manual curation, which led to a final census of 1,542 RBPs. Strikingly, the biological functions of a third of these proteins is unknown or minimally defined, at least in human disease; some of these - such as DEAD/DEAH box helicases – were found to be significantly regulated in airway epithelium of COPD patients by our analysis.
In our study the DEAD-box RNA helicase, DDX17 was in fact among the top downregulated mRBP in COPD; its role is not yet defined in this disease. DDX17 is a nucleocytoplasmic shuttling factor that functions as RNA helicase and is involved in transcription, splicing and miRNA processing. Several studies indicate its involvement in antiviral responses: in a recent study, a significant decrease in DDX17 was found in transcriptional signature to vaccination to H1N1 influenza virus in human subjects, which correlated with antibody titter and IFN-γ production by T-cells (83). Upregulated DDX17 expression has been reported to be associated with resistance to the tyrosine kinase inhibitor drug, gefitinib in non-small cell lung cancer (NSCLC) cells (84). We also found as significantly downregulated the 3′-5′ DEAH-box helicase DHX36, also known as RHAU (RNA helicase associated with AU-rich element) (58). In addition to regulating the transport and half-life of ARE-bearing mRNAs, it has a role in the mechanisms of preservation of genome integrity and in the maintenance of telomeres. In particular, DHX36 assists the activity of the TERT (Telomerase Reverse Transcriptase) enzyme (54, 58–62). As helicase, DHX36 unwinds parallel G-quadruplex structures formed in DNA and RNA. Interestingly, a recent study indicates that ablation of DHX36 results in increased SG formation and protein kinase R (PKR/EIF2AK2) phosphorylation, indicating that DHX36 is involved in resolution of cellular stress at the level of SG (85). Moreover, in rat alveolar epithelial cells DHX36 downregulates epithelial sodium channel (ENaC) mRNA stability by binding with T-cell internal antigen-1 related protein (TIAR-1) to the transcript 3′-UTR (86).
The results from the study in COPD revealed for the first time a significant, global downregulation of mRBPs in cells from COPD patients compared to controls; interestingly, a pattern very similar was found in samples from four out of 12 smoker controls with normal lung function, as confirmed by cluster analysis. This data suggest that this expression profile could indicate subjects at higher risk related to smoke, or with disease at preclinical stage. It would be important to understand, upon validation on larger datasets, the specific mechanisms and epithelial responses affected by this mRBP profile, and whether it leads to molecular changes underpinning a specific clinical disease phenotype.
To this end, a search for common transcriptional control and global pathway analysis may assist in directing further studies on a scale larger than single-gene analysis, in particular when exploring relatively uncharted pathways.
Of note, significant enrichment for TF binding motifs in COPD/S-regulated genes was found only for downregulated RBP, further supporting a biological relevance of this global change, possibly representing a coordinated shift in response to pathogenic triggers. Overall, the TFs putatively binding to the DNA motifs identified with highest enrichment, such as the MEF2 TF family, have pleiotropic functions in cell cycle, cell differentiation, proliferation, apoptosis (87); Interestingly, ARID5A acts both as TF and as RBP according to nuclear or cytoplasmic localization (88); FoxD3 acts as tumor suppressor in lung cancer (89) while HOX genes are overexpressed in non-small cell lung cancer and postulated to act as oncogenes (90).
In our genome ontology analysis (Table 2), some of the pathways significantly affected by the changes in mRBPs are already recognized as impacted by the pathogenetic process in COPD, such as the expression/activity of the telomerase enzyme and the signaling coordinated by the kinase mTOR (Mammalian Target Of Rapamycin) (91–94); others may indicate so far under recognized disease determinants.
Telomerase is an enzyme complex that reverse-transcribes an integral RNA template in order to add short DNA repeats at the 3′-ends of telomeres. In our study, the mRBPs HnRNPA1 and HnRNPA2B were found as downregulated SDEG in COPD and this profile was predicted by IPA to inhibit telomere extension by telomerase. Early studies established that hnRNP-A1, hnRNP-A2, and hnRNP-B1 proteins can interact with telomeres and are products of two different genes (HNRNPA1 and HNRNPA2B1) but display similar structures (two RRMs and four RGG motifs in each)(95). The mRBP hnRNP A1 is the best characterized and found to be associated with human telomeres in vivo (96); depletion of hnRNP A/B proteins in human embryonic kidney 293 cell extracts greatly reduced telomerase activity, which was rescued by addition of recombinant hnRNP A1 (96). Recently, a large study conducted in a group of 576 patient with moderate-to-severe COPD found a significant relationship of absolute telomere length, measured by PCR in DNA from peripheral blood samples, with several clinical parameters such as quality of life, number of exacerbations, and mortality (97). These evidence suggest that shorter leukocyte telomere lengths could be evaluated as a biomarker for clinical outcomes in COPD. Furthermore, these two RBPs were coexpressed in cluster 3 (Figure 7), along with another known TERT-regulating factor, AUF-1 (98), which we initially documented as downregulated in COPD patients vs. controls by immunohistochemistry (16). AUF-1 positively regulates TERT1 at promoter level, but its loss may impact cellular senescence also by concomitant lack of its destabilization function for cell-cycle checkpoint mRNAs (98). Coexpression is often found among RBPs that participate to common posttranscriptional pathways; therefore, the novel mRBP clusters we identified (Figure 7 and Supplementary Table 2) can be used as starting point to infer mRBP putative regulatory roles and identify coordinated expression of targets (20). Taken together, depletion in COPD of RBPs that are crucial for telomere length, as suggested by our findings, may have a role in shaping several COPD clinical outcomes by impacting this function also in airway epithelium.
In our pathway analysis, signaling of eukariotic initiation factors (eIF) was negatively impacted by downregulation of several eIFs, an effect which was also linked to a negative influence on mTOR signaling. The expression and functions of eukariotic initiation factors (eIF) are generally regulated in aging, transformation, and growth arrest and are critically hampered in cancer and during pathogenic stress conditions (99). Acceleration of cellular aging driven by oxidative stress in COPD lung is characterized by activation of PI3K (phosphoinositide-3-kinase) and mTOR signaling, through oxidation of tyrosine phosphatases such as and SHIP-1 (SH2-containing inositol-59-phosphatase-1) and PTEN (phosphatase tensin homolog) (100). The activity of eIF4E-binding protein(BP)1/eIF4E pathway is required for mTOR-dependent G1-phase progression (101), in particular it mediates mTORC1-dependent increased expression of cyclin D1 (102). In cancer cells, decreased expression and functions of eIFs leads to inhibition of global translation and enhancement of translation of subsets of mRNAs by alternative mechanisms (99). Therefore, pathway analysis of COPD-related mRBP patterns strongly suggests a key role for altered translational regulation as a pathogenic mechanism by which oxidative stress alters specific protein levels and cellular functions. A more specific understanding of dysregulation of mRNA translation could uncover new strategies to diagnose and treat at least some forms of chronic inflammation and possibly indicate mechanisms linking COPD to lung cancer development.
Cytokines, chemokines and proinflammatory molecules secreted by senescent cells are collectively described as the senescence-associated secretory phenotype (SASP) (103). Multiple RBPs, such as Human antigen (Hu)R, AUF-1, tristetraprolin (TTP) have been shown to regulate several of the inflammatory transcripts that take also part to the SARP phenotype (12, 81, 104). In particular, CCL2, CXCL1 and IL-6 were identified among others as HuR-associated and regulated transcripts in human airway epithelial BEAS-2B cells stimulated with TNFα plus IFNγ. However, levels of TTP expression appear unchanged in airway samples from COPD patients and smoker controls as well as in epithelial in vitro models of cigarette smoke challenge, and there are conflicting evidence regarding airway epithelial HuR expression in COPD (16, 105, 106). The documented loss of epithelial AUF-1 may contribute also to SASP, besides impacting on cellular senescence, through loss of its mRNA decay-promoting function for several of its factors, such as IL-1β, TNFα, IL-6, CXCL1, VEGF (104). It is noteworthy that in our correlation analysis of COPD-regulated mRBPs, expression of T-cell intracellular antigen (TIA)-1, a critical translational repressor of TNFα (107), clusters with that of AUF-1 (Figure 7C).
Lastly, the pathway impacted by COPD-associated RBP expression in all clusters is Ras-related nuclear protein (Ran) signaling. Ran controls nucleo-cytoplasmic protein transport through the nuclear pore complex and regulates cell cycle progression (108, 109). As regulators of RNA throughout their lifespan, numerous RBPs undertake regulated nucleocytoplasmic shuttling in complex with their targets; key RBPs, such as HuR and HNRNPA1, are known to bind to transportin-1 (110). Conversely, several factors involved in Ran-mediated nucleo-cytoplasmic transport system, including transportin 1, are controlled by RBPs such as HuR (111) and by posttranscriptional control at large. As disruption of Ran expression and function is linked to most cancers, including NSCLC (112, 113), this data suggest that the impact of RBP expression changes on Ran pathway may contribute to increased risk of cancer development in a subset of COPD patients.
Unexpectedly, no significant differential mRBP expression was found when applying the mRBP list search to the database derived from bronchial brushings of SA patients vs. healthy controls. Triggers and inflammatory features of bronchial asthma have distinct features from those of COPD, however in severe asthma the inflammatory pattern is less divergent: increased oxidative stress and a poor response to corticosteroids are SA features common to COPD, especially in SA subjects who are smokers (114). Moreover, global alterations in airway epithelial-derived miRNA and polysome-bound mRNAs have been reported in other smaller SA patient cohorts compared to HC, indicating the occurrence of alteration in posttranscriptional pathways (115). Furthermore, a recent study reports that in primary airway epithelial cells harvested by bronchial brushing in SA patients, IL-17A in combination with TNFα prevents the cytoplasmic translocation of the RBP TIAR. Nuclear sequestration of TIAR halted the cytoplasmic binding and translational repression of its targets, IL-6 and CXCL8 mRNAs and correlated with corticosteroid insensitivity, in vivo neutrophilic inflammation and the post-bronchodilator FEV1 of SA patients (116). Posttranscriptional changes in asthma could be driven by a more diverse pattern of molecular species and mechanisms and changes in mRBPs localization and binding, rather that expression, may be predominant. This underscores the need to implement studies probing RBP posttranslational modifications in human airway samples or cell lines and to characterize the RBP interactome and understand its regulation of disease-related epithelial responses. More studies will be necessary to validate this significant negative finding in SA also using additional airway epithelial databases from patients with defined eosinophilic T2 phenotypes and neutrophilic or pauci-granulocytic non-T2 phenotypes (117). Moreover, evaluation of datasets derived from patients with milder asthma could add relevant information, for example to confirm a potential role of RBP modulation by glucocorticoid therapy, so far identified in human airway epithelium in vitro (12).
There are several limitations also to the results obtained for the COPD database, the main one being the small number of subjects included. In support of our findings, however, this patient and control cohort did have statistical power to support the findings of the original study. We were able to validate our main finding of global downregulation of mRBPs in COPD/S in an independent small airway epithelial cell transcriptomic study (21), in particular for coexpressed mRBP genes whose changes were deemed by IPA analys to have a significant impact on several canonical pathways. It is nevertheless essential to evaluate other independent databases with larger number of COPD subjects and controls, possibly with detailed clinical phenotyping including alpha-1-antitrypsin deficiency status, length of smoking history or time from smoking cessation as well as history of exacerbation. However, a rate-limiting factor for this validation lies in the fact that few studies have focused on transcriptomic of small airway epithelium in large number of patients. While correlation network analysis is being conducted on the transcriptome of larger COPD case/control cohorts for which lung tissue samples are available (118), other systems-biology approaches using single-cell sequencing of small airway epithelial cells are concurrently uncovering relevant biological features using brushing samples isolated in a number of COPD and control subjects equal to, or smaller than that of the study we evaluated (119, 120).
Moreover, we searched epithelial GEO databases for a list of RBPs selected on the basis of canonical RBDs (20). It remains to be probed the profile of other RBPs that do not contain conventional RBDs (121), which have been increasingly identified in human RNA interactome studies. These RBPs also have RNA binding activity but hold several other functions, such enzymatic activity in metabolic pathways or in RNA modification; their role in human epithelial biology is largely unknown.
Overall, the COPD-related mRBP profile found in our study suggests post-transcriptional control of epithelial gene expression as substantial, yet understudied process possibly contributing to key pathogenic mechanisms in COPD. In-depth characterization of proteins dynamically interacting with mRNAs is necessary to understand how PTGR participates to the disease process – and whether it can be targeted therapeutically. Therefore, creating a map of RBP expression is a necessary first step to then analyze epithelial mRNA-bound proteome and potential changes in disease. Focused functional analysis and validating proteomic experiments will be needed to validate the coexpression of mRBPs and to address whether expression of mRBP targets, when known, in the COPD epithelial transcriptome database would show alterations consistent with – and dependent from - the documented changes of mRBPs expression.
Data Availability Statement
The datasets presented in this study can be found in online repositories. The names of the repository/repositories and accession number(s) can be found in the article/Supplementary Material.
Author Contributions
LR implemented all analyses, generated figures, and assisted in manuscript preparation. GG and DM bioinformatic and statistical analysis. JD, IS, and AN data extraction from GEO databases and analysis of specific datasets. MP generation of Tables and referencing. AV, GC, and VC selection of databases, data mining, and manuscript editing. CS study design and manuscript preparation. All authors contributed to the article and approved the submitted version.
Funding
Fondazione con il Sud (Grant: Brains2South 2015 PDR-0224 to JD), Regione Campania, POR FESR 2014/20 RarePlatNet Project (Az. 1.2, CUP B63D18000380007) (to VC), POR Campania FESR 2007–2013–RETE DELLE BIOTECNOLOGIE IN CAMPANIA –Project Terapie Innovative di Malattie Infiammatorie croniche, metaboliche, Neoplastiche e Geriatriche – TIMING (University of Salerno, CS); FARB 2018/19 (University of Salerno, VC, and CS).
Conflict of Interest
The authors declare that the research was conducted in the absence of any commercial or financial relationships that could be construed as a potential conflict of interest.
Acknowledgments
The authors wish to thank Prof. Ian M. Adcock for critical discussions on data.
Supplementary Material
The Supplementary Material for this article can be found online at: https://www.frontiersin.org/articles/10.3389/fimmu.2020.579889/full#supplementary-material
References
1. Keene JD. RNA regulons: coordination of post-transcriptional events. Nat Rev Genet. (2007) 8:533–43. doi: 10.1038/nrg2111
2. Gehring NH, Wahle E, Fischer U. Deciphering the mRNP code: RNA-bound determinants of post-transcriptional gene regulation. Trends Biochem Sci. (2017) 42:369–82. doi: 10.1016/j.tibs.2017.02.004
3. Hitti E, Bakheet T, Al-Souhibani N, Moghrabi W, Al-Yahya S, Al-Ghamdi M, et al. Systematic analysis of AU-rich element expression in cancer reveals common functional clusters regulated by key RNA-binding proteins. Cancer Res. (2016) 76:4068–80. doi: 10.1158/0008-5472.CAN-15-3110
4. Pereira B, Billaud M, Almeida R. RNA-binding proteins in cancer: old players and new actors. Trends Cancer. (2017) 3:506–28. doi: 10.1016/j.trecan.2017.05.003
5. Wang Z, Bhattacharya A, Ivanov DN. Identification of small-molecule inhibitors of the HuR/RNA interaction using a fluorescence polarization screening assay followed by NMR validation. PLoS ONE. (2015) 10:e0138780. doi: 10.1371/journal.pone.0138780
6. Hong S. RNA binding protein as an emerging therapeutic target for cancer prevention and treatment. J Cancer Prev. (2017) 22:203–10. doi: 10.15430/JCP.2017.22.4.203
7. Patial S, Blackshear PJ. Tristetraprolin as a therapeutic target in inflammatory disease. Trends Pharmacol Sci. (2016) 37:811–21. doi: 10.1016/j.tips.2016.07.002
8. Taylor GA, Carballo E, Lee DM, Lai WS, Thompson MJ, Patel DD, et al. A pathogenetic role for tnfα in the syndrome of cachexia, arthritis, and autoimmunity resulting from tristetraprolin (TTP) deficiency. Immunity. (1996) 4:445–54. doi: 10.1016/S1074-7613(00)80411-2
9. Lu J-Y, Sadri N, Schneider RJ. Endotoxic shock in AUF1 knockout mice mediated by failure to degrade proinflammatory cytokine mRNAs. Genes Dev. (2006) 20:3174–84. doi: 10.1101/gad.1467606
10. Durham AL, Adcock IM. The relationship between COPD and lung cancer. Lung Cancer. (2015) 90:121–7. doi: 10.1016/j.lungcan.2015.08.017
11. Khabar KSA. Post-transcriptional control during chronic inflammation and cancer: a focus on AU-rich elements. Cell Mol Life Sci. (2010) 67:2937–55. doi: 10.1007/s00018-010-0383-x
12. Ishmael FT, Fang X, Galdiero MR, Atasoy U, Rigby WFC, Gorospe M, et al. Role of the RNA-binding protein tristetraprolin in glucocorticoid-mediated gene regulation. J Immunol. (2008) 180:8342. doi: 10.4049/jimmunol.180.12.8342
13. Ishmael FT, Fang X, Houser KR, Pearce K, Abdelmohsen K, Zhan M, et al. The human glucocorticoid receptor as an RNA-binding protein: global analysis of glucocorticoid receptor-associated transcripts and identification of a target RNA motif. J Immunol. (2011) 186:1189–98. doi: 10.4049/jimmunol.1001794
14. Fan J, Ishmael FT, Fang X, Myers A, Cheadle C, Huang SK, et al. Chemokine transcripts as targets of the RNA-binding protein HuR in human airway epithelium. J Immunol. (2011) 186:2482–94. doi: 10.4049/jimmunol.0903634
15. Stellato C. Glucocorticoid actions on airway epithelial responses in immunity: functional outcomes and molecular targets. J Allergy Clin Immunol. (2007) 120:1247–63. doi: 10.1016/j.jaci.2007.10.041
16. Ricciardi L, Col JD, Casolari P, Memoli D, Conti V, Vatrella A, et al. Differential expression of RNA-binding proteins in bronchial epithelium of stable COPD patients. Int J Chron Obstruct Pulmon Dis. (2018) 13:3173–90. doi: 10.2147/COPD.S166284
17. Carolan BJ, Heguy A, Harvey BG, Leopold PL, Ferris B, Crystal RG. Up-regulation of expression of the ubiquitin carboxyl-terminal hydrolase L1 gene in human airway epithelium of cigarette smokers. Cancer Res. (2006) 66:10729–40. doi: 10.1158/0008-5472.CAN-06-2224
18. Kechavarzi B, Janga SC. Dissecting the expression landscape of RNA-binding proteins in human cancers. Genome Biol. (2014) 15:R14. doi: 10.1186/gb-2014-15-1-r14
19. Modena BD, Tedrow JR, Milosevic J, Bleecker ER, Meyers DA, Wu W, et al. Gene expression in relation to exhaled nitric oxide identifies novel asthma phenotypes with unique biomolecular pathways. Am J Respir Crit Care Med. (2014) 190:1363–72. doi: 10.1164/rccm.201406-1099OC
20. Gerstberger S, Hafner M, Tuschl T. A census of human RNA-binding proteins. Nat Rev Genetics. (2014) 15:829. doi: 10.1038/nrg3813
21. Ammous Z, Hackett NR, Butler MW, Raman T, Dolgalev I, O'Connor TP, et al. Variability in small airway epithelial gene expression among normal smokers. Chest. (2008) 133:1344–53. doi: 10.1378/chest.07-2245
22. Barrett T, Wilhite SE, Ledoux P, Evangelista C, Kim IF, Tomashevsky M, et al. NCBI GEO: archive for functional genomics data sets—update. Nucleic Acids Res. (2012) 41:D991–5. doi: 10.1093/nar/gks1193
23. Oliveros JC. VENNY. An Interactive Tool for Comparing Lists With Venn Diagrams. (2007–2015) Available online at: http://bioinfogp.cnb.csic.es/tools/venny/index.html
24. Howe E, Holton K, Nair S, Schlauch D, Sinha R, Quackenbush J. MeV: MultiExperiment viewer. In: Ochs MF, Casagrande JT, Davuluri RV, editors. Biomedical Informatics for Cancer Research. Boston, MA: Springer US. (2010). p. 267–77. doi: 10.1007/978-1-4419-5714-6_15
25. Saeed AI, Sharov V, White J, Li J, Liang W, Bhagabati N, et al. TM4: a free, open-source system for microarray data management and analysis. BioTechniques. (2003) 34:374–8. doi: 10.2144/03342mt01
26. Zambelli F, Pesole G, Pavesi G. PscanChIP: finding over-represented transcription factor-binding site motifs and their correlations in sequences from ChIP-Seq experiments. Nucleic Acids Res. (2013) 41:W535–43. doi: 10.1093/nar/gkt448
27. Walter W, Sánchez-Cabo F, Ricote M. GOplot: an R package for visually combining expression data with functional analysis. Bioinformatics. (2015) 31:2912–4. doi: 10.1093/bioinformatics/btv300
28. Yamaguchi A, Takanashi K. FUS interacts with nuclear matrix-associated protein SAFB1 as well as Matrin3 to regulate splicing and ligand-mediated transcription. Sci Rep. (2016) 6:35195. doi: 10.1038/srep35195
29. Baechtold H, Kuroda M, Sok J, Ron D, Lopez BS, Akhmedov AT. Human 75-kDa DNA-pairing protein is identical to the pro-oncoprotein TLS/FUS and is able to promote D-loop formation. J Biol Chem. (1999) 274:34337–42. doi: 10.1074/jbc.274.48.34337
30. Crucs S, Chatterjee S, Gavis ER. Overlapping but distinct RNA elements control repression and activation of nanos translation. Mol Cell. (2000) 5:457–67. doi: 10.1016/S1097-2765(00)80440-2
31. Lande-Diner L, Boyault C, Kim JY, Weitz CJ. A positive feedback loop links circadian clock factor CLOCK-BMAL1 to the basic transcriptional machinery. Proc Natl Acad Sci USA. (2013) 110:16021. doi: 10.1073/pnas.1305980110
32. Mukhopadhyay R, Jia J, Arif A, Ray PS, Fox PL. The GAIT system: a gatekeeper of inflammatory gene expression. Trends Biochem Sci. (2009) 34:324–31. doi: 10.1016/j.tibs.2009.03.004
33. Hönig A, Auboeuf D, Parker MM, Malley BW, Berget SM. Regulation of alternative splicing by the ATP-dependent DEAD-Box RNA helicase p72. Mol Cell Biol. (2002) 22:5698. doi: 10.1128/MCB.22.16.5698-5707.2002
34. Dardenne E, Pierredon S, Driouch K, Gratadou L, Lacroix-Triki M, Espinoza MP, et al. Splicing switch of an epigenetic regulator by RNA helicases promotes tumor-cell invasiveness. Nat Struct Mol Biol. (2012) 19:1139–46. doi: 10.1038/nsmb.2390
35. Dardenne E, Polay Espinoza M, Fattet L, Germann S, Lambert M-P, Neil H, et al. RNA helicases DDX5 and DDX17 dynamically orchestrate transcription, miRNA, and splicing programs in cell differentiation. Cell Rep. (2014) 7:1900–13. doi: 10.1016/j.celrep.2014.05.010
36. Germann S, Gratadou L, Zonta E, Dardenne E, Gaudineau B, Fougère M, et al. Dual role of the ddx5/ddx17 RNA helicases in the control of the pro-migratory NFAT5 transcription factor. Oncogene. (2012) 31:4536–49. doi: 10.1038/onc.2011.618
37. Samaan S, Tranchevent L-C, Dardenne E, Polay Espinoza M, Zonta E, Germann S, et al. The Ddx5 and Ddx17 RNA helicases are cornerstones in the complex regulatory array of steroid hormone-signaling pathways. Nucleic Acids Res. (2013) 42:2197–207. doi: 10.1093/nar/gkt1216
38. Shin S, Janknecht R. Concerted activation of the Mdm2 promoter by p72 RNA helicase and the coactivators p300 and P/CAF. J Cell Biochem. (2007) 101:1252–65. doi: 10.1002/jcb.21250
39. Mooney SM, Grande JP, Salisbury JL, Janknecht R. Sumoylation of p68 and p72 RNA helicases affects protein stability and transactivation potential. Biochemistry. (2010) 49:1–10. doi: 10.1021/bi901263m
40. Mooney SM, Goel A, D'Assoro AB, Salisbury JL, Janknecht R. Pleiotropic effects of p300-mediated acetylation on p68 and p72 RNA helicase. J Biol Chem. (2010) 285:30443–52. doi: 10.1074/jbc.M110.143792
41. Dutertre M, Gratadou L, Dardenne E, Germann S, Samaan S, Lidereau R, et al. Estrogen regulation and physiopathologic significance of alternative promoters in breast cancer. Cancer Res. (2010) 70:3760. doi: 10.1158/0008-5472.CAN-09-3988
42. Chen G, Guo X, Lv F, Xu Y, Gao G. p72 DEAD box RNA helicase is required for optimal function of the zinc-finger antiviral protein. Proc Natl Acad Sci USA. (2008) 105:4352. doi: 10.1073/pnas.0712276105
43. Sampath P, Mazumder B, Seshadri V, Fox PL. Transcript-selective translational silencing by gamma interferon is directed by a novel structural element in the ceruloplasmin mRNA 3′ untranslated region. Mol Cell Biol. (2003) 23:1509–19. doi: 10.1128/MCB.23.5.1509-1519.2003
44. Mazumder B, Seshadri V, Imataka H, Sonenberg N, Fox PL. Translational silencing of ceruloplasmin requires the essential elements of mRNA circularization: poly(A) tail, poly(A)-binding protein, and eukaryotic translation initiation factor 4G. Mol Cell Biol. (2001) 21:6440–9. doi: 10.1128/MCB.21.19.6440-6449.2001
45. Iwasaki T, Chin WW, Ko L. Identification and characterization of RRM-containing Coactivator Activator (CoAA) as TRBP-interacting protein, and its splice variant as a Coactivator Modulator (CoAM). J Biol Chem. (2001) 276:33375–83. doi: 10.1074/jbc.M101517200
46. Morchikh M, Cribier A, Raffel R, Amraoui S, Cau J, Severac D, et al. HEXIM1 and NEAT1 long non-coding RNA form a multi-subunit complex that regulates DNA-mediated innate immune response. Mol Cell. (2017) 67:387–99.e5. doi: 10.1016/j.molcel.2017.06.020
47. Kapasi P, Chaudhuri S, Vyas K, Baus D, Komar AA, Fox PL, et al. L13a blocks 48S assembly: role of a general initiation factor in mRNA-specific translational control. Mol Cell. (2007) 25:113–26. doi: 10.1016/j.molcel.2006.11.028
48. Tran H, Schilling M, Wirbelauer C, Hess D, Nagamine Y. Facilitation of mRNA deadenylation and decay by the exosome-bound, DExH protein RHAU. Mol Cell. (2004) 13:101–11. doi: 10.1016/S1097-2765(03)00481-7
49. Harashima A, Guettouche T, Barber GN. Phosphorylation of the NFAR proteins by the dsRNA-dependent protein kinase PKR constitutes a novel mechanism of translational regulation and cellular defense. Genes Dev. (2010) 24:2640–53. doi: 10.1101/gad.1965010
50. Li X, Liu C-X, Xue W, Zhang Y, Jiang S, Yin Q-F, et al. Coordinated circRNA biogenesis and function with NF90/NF110 in viral infection. Mol Cell. (2017) 67:214–27.e7. doi: 10.1016/j.molcel.2017.05.023
51. Arif A, Jia J, Mukhopadhyay R, Willard B, Kinter M, Fox PL. Two-site phosphorylation of EPRS coordinates multimodal regulation of noncanonical translational control activity. Mol Cell. (2009) 35:164–80. doi: 10.1016/j.molcel.2009.05.028
52. Rahman MA, Masuda A, Ohe K, Ito M, Hutchinson DO, Mayeda A, et al. HnRNP L and hnRNP LL antagonistically modulate PTB-mediated splicing suppression of CHRNA1 pre-mRNA. Sci Rep. (2013) 3:2931. doi: 10.1038/srep02931
53. Damianov A, Ying Y, Lin C-H, Lee J-A, Tran D, Vashisht Ajay A, et al. Rbfox proteins regulate splicing as part of a large multiprotein complex LASR. Cell. (2016) 165:606–19. doi: 10.1016/j.cell.2016.03.040
54. Giri B, Smaldino PJ, Thys RG, Creacy SD, Routh ED, Hantgan RR, et al. G4 resolvase 1 tightly binds and unwinds unimolecular G4-DNA. Nucleic Acids Res. (2011) 39:7161–78. doi: 10.1093/nar/gkr234
55. Vaughn JP, Creacy SD, Routh ED, Joyner-Butt C, Jenkins GS, Pauli S, et al. The DEXH protein product of the DHX36 gene is the major source of tetramolecular quadruplex G4-DNA resolving activity in HeLa cell lysates. J Biol Chem. (2005) 280:38117–20. doi: 10.1074/jbc.C500348200
56. Chalupníková K, Lattmann S, Selak N, Iwamoto F, Fujiki Y, Nagamine Y. Recruitment of the RNA helicase RHAU to stress granules via a unique RNA-binding domain. J Biol Chem. (2008) 283:35186–98. doi: 10.1074/jbc.M804857200
57. Lattmann S, Giri B, Vaughn JP, Akman SA, Nagamine Y. Role of the amino terminal RHAU-specific motif in the recognition and resolution of guanine quadruplex-RNA by the DEAH-box RNA helicase RHAU. Nucleic Acids Res. (2010) 38:6219–33. doi: 10.1093/nar/gkq372
58. Meier M, Patel TR, Booy EP, Marushchak O, Okun N, Deo S, et al. Binding of G-quadruplexes to the N-terminal recognition domain of the RNA helicase associated with AU-rich element (RHAU). J Biol Chem. (2013) 288:35014–27. doi: 10.1074/jbc.M113.512970
59. Lattmann S, Stadler MB, Vaughn JP, Akman SA, Nagamine Y. The DEAH-box RNA helicase RHAU binds an intramolecular RNA G-quadruplex in TERC and associates with telomerase holoenzyme. Nucleic Acids Res. (2011) 39:9390–404. doi: 10.1093/nar/gkr630
60. Booy EP, Meier M, Okun N, Novakowski SK, Xiong S, Stetefeld J, et al. The RNA helicase RHAU (DHX36) unwinds a G4-quadruplex in human telomerase RNA and promotes the formation of the P1 helix template boundary. Nucleic Acids Res. (2012) 40:4110–24. doi: 10.1093/nar/gkr1306
61. Booy EP, McRae EKS, McKenna SA. Biochemical characterization of G4 quadruplex telomerase RNA unwinding by the RNA helicase RHAU. In: Boudvillain M, editor. RNA Remodeling Proteins: Methods and Protocols. New York, NY: Springer. (2015). p. 125–35. doi: 10.1007/978-1-4939-2214-7_9
62. Sexton AN, Collins K. The 5′ guanosine tracts of human telomerase RNA are recognized by the G-quadruplex binding domain of the RNA helicase DHX36 and function to increase RNA accumulation. Mol Cell Biol. (2011) 31:736. doi: 10.1128/MCB.01033-10
63. Booy EP, Howard R, Marushchak O, Ariyo EO, Meier M, Novakowski SK, et al. The RNA helicase RHAU (DHX36) suppresses expression of the transcription factor PITX1. Nucleic Acids Res. (2013) 42:3346–61. doi: 10.1093/nar/gkt1340
64. Nie J, Jiang M, Zhang X, Tang H, Jin H, Huang X, et al. Post-transcriptional regulation of Nkx2-5 by RHAU in heart development. Cell Rep. (2015) 13:723–32. doi: 10.1016/j.celrep.2015.09.043
65. Huang W, Smaldino PJ, Zhang Q, Miller LD, Cao P, Stadelman K, et al. Yin Yang 1 contains G-quadruplex structures in its promoter and 5′-UTR and its expression is modulated by G4 resolvase 1. Nucleic Acids Res. (2011) 40:1033–49. doi: 10.1093/nar/gkr849
66. Newman M, Sfaxi R, Saha A, Monchaud D, Teulade-Fichou M-P, Vagner S. The G-quadruplex-specific RNA helicase DHX36 regulates p53 pre-mRNA 3′-end processing following UV-induced DNA damage. J Mol Biol. (2017) 429:3121–31. doi: 10.1016/j.jmb.2016.11.033
67. He Y, Smith R. Nuclear functions of heterogeneous nuclear ribonucleoproteins A/B. Cell Mol Life Sci. (2009) 66:1239–56. doi: 10.1007/s00018-008-8532-1
68. Munro TP, Magee RJ, Kidd GJ, Carson JH, Barbarese E, Smith LM, et al. Mutational analysis of a heterogeneous nuclear ribonucleoprotein A2 response element for rna trafficking. J Biol Chem. (1999) 274:34389–95. doi: 10.1074/jbc.274.48.34389
69. Coelho MB, Attig J, Bellora N, König J, Hallegger M, Kayikci M, et al. Nuclear matrix protein Matrin3 regulates alternative splicing and forms overlapping regulatory networks with PTB. EMBO J. (2015) 34:653–68. doi: 10.15252/embj.201489852
70. Rishi AK, Zhang L, Boyanapalli M, Wali A, Mohammad RM, Yu Y, et al. Identification and characterization of a cell cycle and apoptosis regulatory protein-1 as a novel mediator of apoptosis signaling by retinoid CD437. J Biol Chem. (2003) 278:33422–35. doi: 10.1074/jbc.M303173200
71. Seo W-Y, Jeong BC, Yu EJ, Kim HJ, Kim S-H, Lim JE, et al. CCAR1 promotes chromatin loading of androgen receptor (AR) transcription complex by stabilizing the association between AR and GATA2. Nucleic Acids Res. (2013) 41:8526–36. doi: 10.1093/nar/gkt644
72. Nakamura H, Kawagishi H, Watanabe A, Sugimoto K, Maruyama M, Sugimoto M. Cooperative role of the RNA-binding proteins Hzf and HuR in p53 activation. Mol Cell Biol. (2011) 31:1997–2009. doi: 10.1128/MCB.01424-10
73. Cho SJ, Zhang J, Chen X. RNPC1 modulates the RNA-binding activity of, and cooperates with, HuR to regulate p21 mRNA stability. Nucleic Acids Res. (2010) 38:2256–67. doi: 10.1093/nar/gkp1229
74. Sun Y, Ding L, Zhang H, Han J, Yang X, Yan J, et al. Potentiation of smad-mediated transcriptional activation by the RNA-binding protein RBPMS. Nucleic Acids Res. (2006) 34:6314–26. doi: 10.1093/nar/gkl914
75. Teplova M, Farazi TA, Tuschl T, Patel DJ. Structural basis underlying CAC RNA recognition by the RRM domain of dimeric RNA-binding protein RBPMS. Q Rev Biophys. (2016) 49:e1. doi: 10.1017/S0033583515000207
76. Wang Isabel X, So E, Devlin James L, Zhao Y, Wu M, Cheung Vivian G. ADAR regulates RNA editing, transcript stability, and gene expression. Cell Rep. (2013) 5:849–60. doi: 10.1016/j.celrep.2013.10.002
77. Antonicka H, Shoubridge Eric A. Mitochondrial RNA granules are centers for posttranscriptional RNA processing and ribosome biogenesis. Cell Rep. (2015) 10:920–32. doi: 10.1016/j.celrep.2015.01.030
78. Lessel D, Schob C, Küry S, Reijnders MRF, Harel T, Eldomery MK, et al. De novo missense mutations in DHX30 impair global translation and cause a neurodevelopmental disorder. Am J Hum Genet. (2017) 101:716–24. doi: 10.1016/j.ajhg.2017.09.014
79. Anantharaman A, Gholamalamdari O, Khan A, Yoon J-H, Jantsch MF, Hartner JC, et al. RNA-editing enzymes ADAR1 and ADAR2 coordinately regulate the editing and expression of Ctn RNA. FEBS Lett. (2017) 591:2890–904. doi: 10.1002/1873-3468.12795
80. Stelzer G, Rosen N, Plaschkes I, Zimmerman S, Twik M, Fishilevich S, et al. The GeneCards suite: from gene data mining to disease genome sequence analyses. Curr Protoc Bioinform. (2016) 54:1.30.1–1.3. doi: 10.1002/cpbi.5
81. Anderson P. Post-transcriptional regulons coordinate the initiation and resolution of inflammation. Nat Rev Immunol. (2010) 10:24–35. doi: 10.1038/nri2685
82. Kotb A, Yuki K, Hyeon Ho K, Myriam G. Posttranscriptional gene regulation by RNA-binding proteins during oxidative stress: implications for cellular senescence. Biol Chem. (2008) 389:243–55. doi: 10.1515/BC.2008.022
83. Skibinski DAG, Jones LA, Zhu YO, Xue LW, Au B, Lee B, et al. Induction of human T-cell and cytokine responses following vaccination with a novel influenza vaccine. Sci Rep. (2018) 8:18007. doi: 10.1038/s41598-018-36703-7
84. Li K, Mo C, Gong D, Chen Y, Huang Z, Li Y, et al. DDX17 nucleocytoplasmic shuttling promotes acquired gefitinib resistance in non-small cell lung cancer cells via activation of β-catenin. Cancer Lett. (2017) 400:194–202. doi: 10.1016/j.canlet.2017.02.029
85. Sauer M, Juranek SA, Marks J, De Magis A, Kazemier HG, Hilbig D, et al. DHX36 prevents the accumulation of translationally inactive mRNAs with G4-structures in untranslated regions. Nat Commun. (2019) 10:2421. doi: 10.1038/s41467-019-10432-5
86. Migneault F, Gagnon F, Pascariu M, Laperle J, Roy A, Dagenais A, et al. Post-transcriptional modulation of aENaC mRNA in alveolar epithelial cells: involvement of its 3′ untranslated region. Cell Physiol Biochem. (2019) 52:984–1002. doi: 10.33594/000000068
87. Pon JR, Marra MA. MEF2 transcription factors: developmental regulators and emerging cancer genes. Oncotarget. (2016) 7:2297–312. doi: 10.18632/oncotarget.6223
88. Nyati KK, Zaman MM-U, Sharma P, Kishimoto T. Arid5a, an RNA-binding protein in immune regulation: RNA stability, inflammation, and autoimmunity. Trends Immunol. (2020) 41:255–68. doi: 10.1016/j.it.2020.01.004
89. Lin C, Song W, Bi X, Zhao J, Huang Z, Li Z, et al. Recent advances in the ARID family: focusing on roles in human cancer. Onco Targets Ther. (2014) 7:315–24. doi: 10.2147/OTT.S57023
90. Plowright L, Harrington KJ, Pandha HS, Morgan R. HOX transcription factors are potential therapeutic targets in non-small-cell lung cancer (targeting HOX genes in lung cancer). Br J Cancer. (2009) 100:470–5. doi: 10.1038/sj.bjc.6604857
91. Ito K, Barnes PJ. COPD as a disease of accelerated lung aging. Chest. (2009) 135:173–80. doi: 10.1378/chest.08-1419
92. Barnes PJ. Senescence in COPD and its comorbidities. Ann Rev Physiol. (2017) 79:517–39. doi: 10.1146/annurev-physiol-022516-034314
93. Houssaini A, Breau M, Kebe K, Abid S, Marcos E, Lipskaia L, et al. mTOR pathway activation drives lung cell senescence and emphysema. JCI Insight. (2018) 3:e93203. doi: 10.1172/jci.insight.93203
94. Mercado N, Ito K, Barnes PJ. Accelerated ageing of the lung in COPD: new concepts. Thorax. (2015) 70:482. doi: 10.1136/thoraxjnl-2014-206084
95. Shishkin SS, Kovalev LI, Pashintseva NV, Kovaleva MA, Lisitskaya K. Heterogeneous nuclear ribonucleoproteins involved in the functioning of telomeres in malignant cells. Int J Mol Sci. (2019) 20:745. doi: 10.3390/ijms20030745
96. Zhang Q-S, Manche L, Xu R-M, Krainer AR. hnRNP A1 associates with telomere ends and stimulates telomerase activity. RNA. (2006) 12:1116–28. doi: 10.1261/rna.58806
97. Jin M, Lee EC, Ra SW, Fishbane N, Tam S, Criner GJ, et al. Relationship of absolute telomere length with quality of life, exacerbations, and mortality in COPD. Chest. (2018) 154:266–73. doi: 10.1016/j.chest.2018.05.022
98. Pont Adam R, Sadri N, Hsiao Susan J, Smith S, Schneider Robert J. mRNA decay factor AUF1 maintains normal aging, telomere maintenance, and suppression of senescence by activation of telomerase transcription. Mol Cell. (2012) 47:5–15. doi: 10.1016/j.molcel.2012.04.019
99. Sharma DK, Bressler K, Patel H, Balasingam N, Thakor N. Role of eukaryotic initiation factors during cellular stress and cancer progression. J Nucleic Acids. (2016) 2016:8235121. doi: 10.1155/2016/8235121
100. Barnes PJ, Baker J, Donnelly LE. Cellular senescence as a mechanism and target in chronic lung diseases. Am J Respir Crit Care Med. (2019) 200:556–64. doi: 10.1164/rccm.201810-1975TR
101. Fingar DC, Richardson CJ, Tee AR, Cheatham L, Tsou C, Blenis J. mTOR controls cell cycle progression through its cell growth effectors S6K1 and 4E-BP1/eukaryotic translation initiation factor 4E. Mol Cell Biol. (2004) 24:200. doi: 10.1128/MCB.24.1.200-216.2004
102. Averous J, Fonseca BD, Proud CG. Regulation of cyclin D1 expression by mTORC1 signaling requires eukaryotic initiation factor 4E-binding protein 1. Oncogene. (2008) 27:1106–13. doi: 10.1038/sj.onc.1210715
103. Salama R, Sadaie M, Hoare M, Narita M. Cellular senescence and its effector programs. Genes Dev. (2014) 28:99–114. doi: 10.1101/gad.235184.113
104. Moore AE, Chenette DM, Larkin LC, Schneider RJ. Physiological networks and disease functions of RNA-binding protein AUF1. Wiley Interdiscip Rev RNA. (2014) 5:549–64. doi: 10.1002/wrna.1230
105. Hassibi S, Baker J, Donnelly L, Barnes P. The RNA binding protein HuR regulates the senescence-associated secretory phenotype under conditions of oxidative stress. Eur Respir J. (2019) 54(Suppl. 63):PA2374. doi: 10.1183/13993003.congress-2019.PA2374
106. Usmani OS, Lavorini F, Marshall J, Dunlop WCN, Heron L, Farrington E, et al. Critical inhaler errors in asthma and COPD: a systematic review of impact on health outcomes. Respir Res. (2018) 19:10. doi: 10.1186/s12931-017-0710-y
107. Piecyk M, Wax S, Beck ARP, Kedersha N, Gupta M, Maritim B, et al. TIA-1 is a translational silencer that selectively regulates the expression of TNF-α. EMBO J. (2000) 19:4154–63. doi: 10.1093/emboj/19.15.4154
108. Lei EP, Silver PA. Protein and RNA export from the nucleus. Dev Cell. (2002) 2:261–72. doi: 10.1016/S1534-5807(02)00134-X
109. Cavazza T, Vernos I. The RanGTP pathway: from nucleo-cytoplasmic transport to spindle assembly and beyond. Front Cell Dev Biol. (2016) 3:82. doi: 10.3389/fcell.2015.00082
110. Rebane A, Aab A, Steitz JA. Transportins 1 and 2 are redundant nuclear import factors for hnRNP A1 and HuR. RNA. (2004) 10:590–9. doi: 10.1261/rna.5224304
111. Zhang W, Vreeland AC, Noy N. RNA-binding protein HuR regulates nuclear import of protein. J Cell Sci. (2016) 129:4025. doi: 10.1242/jcs.192096
112. Ning J, Liu W, Zhang J, Lang Y, Xu S. Ran GTPase induces EMT and enhances invasion in non-small cell lung cancer cells through activation of PI3K-AKT pathway. Oncol Res. (2013) 21:67–72. doi: 10.3727/096504013X13747716581417
113. Boudhraa Z, Carmona E, Provencher D, Mes-Masson A-M. Ran GTPase: a key player in tumor progression and metastasis. Front Cell Dev Biol. (2020) 8:345. doi: 10.3389/fcell.2020.00345
114. Barnes PJ. Immunology of asthma and chronic obstructive pulmonary disease. Nat Rev Immunol. (2008) 8:183–92. doi: 10.1038/nri2254
115. Martinez-Nunez RT, Rupani H, Platé M, Niranjan M, Chambers RC, Howarth PH, et al. Genome-wide posttranscriptional dysregulation by MicroRNAs in human asthma as revealed by frac-seq. J Immunol. (2018) 201:251. doi: 10.4049/jimmunol.1701798
116. Ravi A, Chowdhury S, Dijkhuis A, Bonta PI, Sterk PJ, Lutter R. Neutrophilic inflammation in asthma and defective epithelial translational control. Eur Respir J. (2019) 54:1900547. doi: 10.1183/13993003.00547-2019
117. Kuo C-HS, Pavlidis S, Loza M, Baribaud F, Rowe A, Pandis I, et al. A transcriptome-driven analysis of epithelial brushings and bronchial biopsies to define asthma phenotypes in U-BIOPRED. Am J Respir Crit Care Med. (2016) 195:443–55. doi: 10.1164/rccm.201512-2452OC
118. Paci P, Fiscon G, Conte F, Licursi V, Morrow J, Hersh C, et al. Integrated transcriptomic correlation network analysis identifies COPD molecular determinants. Sci Rep. (2020) 10:3361. 10:3361. doi: 10.1038/s41598-020-60228-7
119. Duclos GE, Teixeira VH, Autissier P, Gesthalter YB, Reinders-Luinge MA, Terrano R, et al. Characterizing smoking-induced transcriptional heterogeneity in the human bronchial epithelium at single-cell resolution. Sci Adv. (2019) 5:eaaw3413. doi: 10.1126/sciadv.aaw3413
120. Zuo W-l, Rostami MR, Shenoy SA, LeBlanc MG, Salit J, Strulovici-Barel Y, et al. Cell-specific expression of lung disease risk-related genes in the human small airway epithelium. Respir Res. (2020) 21:200. 21:200. doi: 10.1186/s12931-020-01442-9
Keywords: airway epithelium, chronic inflammation, COPD, posttranscriptional gene regulation, RNA binding proteins, severe asthma
Citation: Ricciardi L, Giurato G, Memoli D, Pietrafesa M, Dal Col J, Salvato I, Nigro A, Vatrella A, Caramori G, Casolaro V and Stellato C (2020) Posttranscriptional Gene Regulatory Networks in Chronic Airway Inflammatory Diseases: In silico Mapping of RNA-Binding Protein Expression in Airway Epithelium. Front. Immunol. 11:579889. doi: 10.3389/fimmu.2020.579889
Received: 03 July 2020; Accepted: 19 August 2020;
Published: 16 October 2020.
Edited by:
Girolamo Pelaia, University of Catanzaro, ItalyReviewed by:
Hartmut Kleinert, Johannes Gutenberg University Mainz, GermanyKenta Shinoda, National Institutes of Health (NIH), United States
Copyright © 2020 Ricciardi, Giurato, Memoli, Pietrafesa, Dal Col, Salvato, Nigro, Vatrella, Caramori, Casolaro and Stellato. This is an open-access article distributed under the terms of the Creative Commons Attribution License (CC BY). The use, distribution or reproduction in other forums is permitted, provided the original author(s) and the copyright owner(s) are credited and that the original publication in this journal is cited, in accordance with accepted academic practice. No use, distribution or reproduction is permitted which does not comply with these terms.
*Correspondence: Cristiana Stellato, cstellato@unisa.it