- 1MRC Human Immunology Unit, Medical Research Council (MRC) Weatherall Institute of Molecular Medicine (WIMM), John Radcliffe Hospital, University of Oxford, Oxford, United Kingdom
- 2MRC WIMM Centre for Computational Biology, Medical Research Council (MRC) Weatherall Institute of Molecular Medicine, John Radcliffe Hospital, University of Oxford, Oxford, United Kingdom
- 3Translational Gastroenterology Unit, John Radcliffe Hospital, Oxford, United Kingdom
Adaptive immune recognition is mediated by specific interactions between heterodimeric T cell receptors (TCRs) and their cognate peptide-MHC (pMHC) ligands, and the methods to accurately predict TCR:pMHC interaction would have profound clinical, therapeutic and pharmaceutical applications. Herein, we review recent developments in predicting cross-reactivity and antigen specificity of TCR recognition. We discuss current experimental and computational approaches to investigate cross-reactivity and antigen-specificity of TCRs and highlight how integrating kinetic, biophysical and structural features may offer valuable insights in modeling immunogenicity. We further underscore the close inter-relationship of these two interconnected notions and the need to investigate each in the light of the other for a better understanding of T cell responsiveness for the effective clinical applications.
Introduction
Specific molecular interactions between heterodimeric T cell receptors (TCRs) and their cognate peptide-MHC (pMHC) ligands contribute to the nature of ensuing adaptive immune response. A better understanding of TCR:pMHC interaction is required to be able to harness adaptive T cell immunity effectively for vaccines and therapeutics. Unfortunately, the mechanisms underpinning cross-reactivity and antigen specificity of peptide-specific TCRs remain puzzling, and leaves the community with an incomplete picture of T cell recognition.
Cross-reactivity is defined as the capacity of a TCR to recognize more than one peptide-MHC molecule. The idea was first postulated by Matzinger and Bevan (1) and later gained traction via Don Mason who challenged the dominant clonal selection theory arguing a highly incompetent immune system if a TCR was able to recognize only a single pMHC complex (2), and Andrew Sewell who empirically measured the necessity of cross-reactivity given the insufficient number of TCRαβ to protect against a wide spectrum of pathogen by comparing the number of potential foreign pMHC complexes a T cell might encounter and the number of TCRs available (3).
Although it is known that T cells can recognize peptide and non-peptide antigens, it is now well-accepted that peptide-specific TCRs exhibit high levels of cross-reactivity. In fact, it has been proposed that a single TCR can recognize 104-107 different MHC-associated epitopes (2). However, it has also been illustrated that once a TCR reacts with a specific peptide-MHC complex, the probability of it reacting with another randomly chosen peptide reduces to ~10−4 (4). Thus, TCR recognition of pMHC complexes is both cross-reactive, given the high number of total epitopes that could be bound, and at the same time, highly specific considering the low frequency of epitopes that can be recognized by a given TCR.
Recent biological and computational advances to screen antigenic peptides and profile TCR repertoires have greatly improved our understanding of the TCR:pMHC interaction. However, the picture is far from complete. As yet it is not possible to, (a) predict TCRs recognizing a given antigen, or (b) predict antigens recognized by a given TCR. Methods to accurately predict biological specificity or cross-reactivity would have profound clinical, therapeutic and pharmaceutical applications in designing cellular therapies for fighting cancer, autoimmune and infectious diseases.
New biological methodologies have enabled definition of cross-reactive peptides using high throughput screens against a series of TCR molecules and some can screen whole cells (5–14). In fact, recently developed labeled pMHC multimers coupled with single cell sequencing facilitated screening of an array of immunogenic peptides in a relatively high-throughput manner, but this is still limited by practical synthesis of pMHC multimers (6). Additionally, kinetic, biophysical and structural studies provide insights on the complex landscape of TCR:pMHC interaction from different angles. However, apart from few attempts to predict immunogenicity, algorithms to predict breadth and/or constituents of the cross-reactome are still in their infancy.
Understanding the underlying mechanisms of common antigen specificity of TCRs, on the other hand, has been the focus of key research over the past few years. A number of recent studies have demonstrated the plausibility of identifying shared motifs amongst tetramer-specific TCRs (15–18) to explain shared antigen specificity. By the advancement of high throughput sequencing technologies for both bulk and single cell immune repertoire profiling, lack of biological data is becoming less of a challenge, allowing us to simultaneously project TCR profiles of T cells along with their antigen specificity, functional states and gene expression levels (19, 20). Therefore, part of the challenge turns into developing sophisticated mathematical and computational models to understand high dimensional and occasionally sparse datasets.
Although cross-reactivity and common antigen-specificity have been investigated individually, understanding the relationship of the two closely interconnected notions seems to be underrepresented in the research community. Whereas, in order to set a foundation for better understanding T cell responsiveness for effective clinical applications, these two pillars of the adaptive immunity can be and should be investigated together and each in the light of the other.
Here we review the recent advances in the understanding of both cross-reactivity and common specificity of T cell recognition mainly from a computational perspective. We will discuss current experimental and computational approaches to investigate cross-reactivity, and highlight how integrating kinetic, biophysical and structural features may offer valuable insights in modeling immunogenicity against TCRs. We will then discuss the progress and limitations in assigning antigen-specific TCRs based on their shared features. Lastly, we will underscore the close inter-relationship of these two principles and how recent single cell technologies are poised to shed further in this area.
Predicting Cross-Recognition Potential of T Cell Receptors
Cross-Reactivity of TCR Is a Double-Edged Sword
TCR cross-reactivity, which was coined in the late twentieth and early twenty first century, has become recognized as a common feature of TCR recognition (2, 3, 21–23) and a single TCR is estimated to bind >106 different MHC-bound peptides (24). A repertoire of highly cross-reactive T cells can effectively screen for numerous antigenic peptides and minimize the risk of pathogen escape from immune surveillance. Nonetheless, cross-reactivity is a double-edged sword: while a highly cross-reactive T cell can effectively screen for a wide spectrum of epitopes, this may also lead to dysregulated T cell responses potentially contributing to allergy, immunopathology, autoimmunity and chronic infection (25–28).
Prior exposure of degenerate T cells can induce polarized response to a pathogen or vaccination (29, 30). Heterologous immunity has been reported between related pathogens with high sequence similarity as well as unrelated pathogens with minimal sequence overlap (31–35) giving both positive and negative effects (36, 37). There is accumulating evidence that genetic background, private TCR specificities and immunological history are key factors contributing to the final outcome of antigen exposure—whether to confer protective immunity or induce damaging immunopathology (25, 38, 39). It is of note that peptide recognition is not a simple on/off event, and that the same T cell can respond in different ways to modified peptides, by for example, pMHC affinity and dose thresholds (40, 41), co-stimulatory molecules (42), and hierarchical organization of thresholds (43, 44).
While naïve T cells expressing self-reactive TCRs survive due to the low avidity or low expression of peptides derived from self-proteins (45), immune tolerance may be broken if T cells are activated by cross-recognition of pathogenic peptides. This results in memory T cells that are potentially stimulated even at 50x lower peptide concentrations (46, 47). Such a phenomenon, known as molecular mimicry, may occur via induced fitting by TCR or pMHC, altered TCR:pMHC docking geometry, and/or structural degeneracy leading to cross-recognition of low-affinity TCR:pMHC, thus potentially leading to breakdown of tolerance (48–53).
From a clinical perspective, recent immunotherapy trials have highlighted off-target toxicities triggered by cross-reactivity of high affinity TCRs, where adoptive T cell transfer trials with high-avidity DMF5 TCR targeting the HLA-A*02:01 MART-1 melanoma peptide showed a greater promise than DMF4 TCR for cancer treatment but also triggered autotoxicities (54). In addition, adoptive T cell transfer targeting melanoma-associated antigen 3 (MAGE-A3) peptide demonstrated severe cardiac toxicity, attributed to recognition of unrelated peptide derived from self-protein Titin displayed on HLA-A*01:01 on the surface of healthy cardiac cells (55). This seemingly unpredictable off-target toxicity mediated by T cell cross-reactivity highlights the requirement to mitigate against autoimmunity deriving from TCR-based immunotherapy.
Technologies to Elucidate the Landscape of Cross-Reactivity
Inability to detect potential toxicities through initial safety evaluation highlighted the need to develop technologies to assess the cross-recognition potential of each TCRs engineered for clinical uses. In recent years, technologies to extensively characterize the recognition pattern of TCR:pMHC have emerged [reviewed in (56)].
Briefly, large combinatorial peptide libraries (8, 9, 24) along with peptide-MHC display systems (7, 10–13) enabled unbiased screening of pooled pMHCs against TCRs to determine positional amino acid preferences. Combinatorial peptide libraries containing thousands to millions of peptides have been utilized to identify cognate peptides, estimate the cross-recognition potential of TCRs, and further characterize structural and/or biochemical relatedness between peptides recognized by the same TCR (10, 57–60).
With the help of combinatorial peptide libraries and single amino acid analogs, the “hotspots” crucial for potential off-target cross-reactivity have been characterized (61–63). For instance, Border et al. demonstrated the capability of single amino acid analogs (called “X-scans”) to differentiate cross-recognition potential of two affinity-enhanced TCRs which would otherwise appear similarly potent and specific (64).
While binding of recombinant TCR and pMHC molecules provide essential information, previous studies reported high-affinity, yet non-stimulatory, interactions occur with high frequency in the human T cell repertoire (65, 66). In recent years, several cell-based platforms have been developed for TCR antigen discovery, using T cell clones or TCR-transduced T cells, for a better reflection of in vivo systems without requirement for a soluble TCR (7, 11–14). One example is signaling and antigen-presenting bifunctional receptors (SABRs), where a signaling domain has been introduced to in the MHC-I molecule, leading to green fluorescence protein (GFP) expression following TCR:pMHC interaction (11). Compared to conventional yeast display system, these approaches enabled a rapid identification of antigens expressed from large peptide libraries transduced into the target cells. However, there remains a number of limitations.
Limitations of the Current Technologies
Although recent approaches provide increased flexibility to investigate the degeneracy of TCRs, they remain limited in (i) the number of possible TCRs that can be tested against peptide libraries in a single experiment, (ii) the number of peptides compared to the actual number of ligands that might be encountered, (iii) the need to prepare a new peptide library for each analysis of pMHC specificity, (iv) the high number of false positive and negative peptides resulted from screening, and (v) often the requirement to generate individual recombinant TCR, T cell clones, or reporter cells expressing TCR for screening. Some approaches in ongoing development do offer the potential to obtain high-throughput biological data using primary unmodified polyclonal T cells (7).
Moreover, current strategies of generating a single amino acid analog library rely on replacing a pre-established peptide target with one amino acid at a time. However, such an approach may underscore the possibility of duplex or triplex amino acid substitutions or even largely different peptides to trigger a TCR response (67). Therefore, interpretation of the results should reflect that it may merely be a window of estimated cross-reactivity.
Expanding Knowledge of TCR:pMHC Interactions by in silico Modeling
In silico modeling may enhance the utility of experimental data for assessing TCR binding degeneracy. Associating the information gained from the aforementioned technologies with the knowledge of the human proteome and the HLA presentation potential through implementation of mathematical modeling approaches might provide valuable insights on the relationship between antigen specificity and cross-recognition potential of TCRs.
Moreover, in silico investigations may suggest clues to yet unsolved problems and help define how ubiquitous previously observed phenomena are, such as publicness of cross-reactive TCRs, different extent of cross-reactivity between featured and featureless peptides, the role of dominant peptides in TCR repertoire organization and preferential directionality of antigen specificity.
For example, Kasprowicz et al. observed preferential directionality from Hepatitis C Virus (HCV) to Influenza A Virus (IAV) i.e., a T cell primed with an HCV-derived peptide was capable of recognizing an IAV-derived peptide but the opposite was not true (68). Correspondingly, recent studies suggest that heterologous immunity is greatly influenced by private specificities and immunological history (39, 69, 70). However, due to scarcity of data and cost associated with generating the data, it is difficult to assay the prevalence and understand the underlying principle of antigen-driven repertoire convergence in an experimental setup. In this regard, in silico approaches may be more suitable for identifying patterns and testing hypotheses on factors driving observed phenomena.
Indeed, several groups have started to use modeling approaches to test various hypotheses on TCR:pMHC interaction propensities (38, 71, 72). For instance, Xu and Jo utilized a simple string model to evaluate a trade-off between rapid screening and dissociation penalty, and have shown that while a highly cross-reactive TCR detects correct peptides in a short period of time with the help of its degeneracy, it takes much longer to release from an incorrectly bound peptide (71).
In addition to models predicting TCR:pMHC interactions, models to relate TCR:pMHC binding parameters and antigen doses to T cell response have also been proposed [reviewed in (73)]. Recently, Fernandes et al. utilized partial differential equations to study the underlying mechanism of ligand discrimination and TCR triggering based on two physical properties, (i) TCR dwell time in the absence of large tyrosine phosphatase, and (ii) spatial constraints on the contact area, and found that topographically constrained T cell contacts allow, and may even be essential, for ligand discrimination by T cells (72). Although these mathematical models are built upon underlying assumptions e.g., a positive correlation between binding affinity and the extent of TCR cross-reactivity, provided that assumptions are evidence-based and reasonable, such modeling approaches will be a valuable strategy to quickly test hypothesis on cross-recognition potential.
Approaches to Predict Immunogenicity From Experimental Data
In 1998, Don Mason argued in favor of the necessity for cross-reactivity (2) with an incredibly high number of peptides potentially generated from the 20 amino acids (>1018 peptides) and a relatively limited number of unique TCRs in an individual (in the range of 106-108) (3, 74, 75). Moreover, the possibility of post-translational modification, peptide processing, HLA presentation and altered T cell functions attributes additional factors to deciphering T cell targets (76–82).
Several attempts to estimate the polyspecificity of TCRs have been performed. These include: (i) generation of mutant peptides with amino acid substitutions and testing the impact of substitution on T cell activation and/or cytotoxicity (83–85), (ii) scanning combinatorial peptide libraries to find cross-reactive peptides against a TCR of known antigen specificity (24, 57, 59, 60, 86, 87), and (iii) scanning peptides or pMHC multimers derived from the host (e.g., human) or pathogen proteome to test cross-recognition potential (5–7, 88–92). Although functional readouts may not have captured all binding, the readout from these approaches allowed identification of essential interaction residues in TCR:pMHC, which were applied to predict polyspecificity.
For instance, in a recent TCR fingerprinting study, Karapetyan et al. investigated which amino acids at each position are essential for 1G4 TCR binding, activation and killing by sequentially replacing every amino acid position outside of anchor positions 2 and 9 with 19 alternative amino acids. The peptides were analyzed using three in vitro assays examining binding of NY-ESOc259 TCR to peptide-MHC complexes, activation of TCR-expressing cells and killing of target cells. Based on the experimental measurements, they constructed positional weight matrices (PWMs) for three in vitro assays and utilized PWM-defined kernel along with NetMHCpan v3.0, an algorithm to predict MHC binding, to predict peptides with high TCR recognition score. By applying the algorithm to 336,921 predicted HLA-A*02:01 binding 9-mer peptides, they demonstrated a strong activation of primary T cells out of the top scoring peptides.
Instead of scanning a single TCR, a few algorithms have been designed to predict immunogenicity of a peptide against a pool of TCRs by the use of sequences (93), positional information (94, 95) and/or physicochemical properties (96, 97) (Table 1). Pogorelyy et al. classified immunogenic vs. non-immunogenic peptides in Kidera feature space by transforming epitope sequences into a vector of sum for each of 10 Kidera factors that encode physicochemical properties of amino acids. Similarly, Ogishi et al. incorporated Amino Acid index database (AAindex) (110) and other physicochemical properties describing features determinant of immunogenicity, then compressed the most predictive peptide descriptors and contact potential profiling (CPP)-based features into a linear coordinate system through a machine-learning technique known as Extremely Randomized Tree (ERT) algorithm (96). Of interest, they hypothesized that immunodominant epitopes share intrinsic patterns which render them more prone to be recognized by the immune system of multiple individuals and focused on identifying these prominent features.
In order to predict antigens with high potential for cross-reactivity and off-target toxicity, Jaravine et al. developed Expitope 2.0 that allow analysis of tissue-specific gene expression pattern and prediction of potential side effect in normal tissue, with the ultimate aim of selecting a safer pool of vaccine targets for personalized immunotherapy (89, 111). Zhang et al. applied tetramer-associated T cell receptor sequencing (TetTCR) to resolve up to five cross-reactive peptides per cell and identified patterns associated with TCR cross-reactivity (6). Similarly, Bentzen et al. utilized experimental data and developed an algorithm named Find Individual Motif Occurrence (FIMO) software to create a priority score inferring the likelihood of cross-recognition (5). From each Shannon logo, cross-reactive peptides were predicted from corresponding position-specific scoring matrix (PSSM) using FIMO, and the human proteome was searched for sequences that match each logo.
Discriminative Features Governing TCR:pMHC Interaction
Although there have been several attempts to predict immunogenicity, the dual nature of the peptide-specific TCR recognition interface, comprised of both peptide and MHC, makes predicting interaction between TCR and pMHC uniquely challenging. While much of T cell specificity is determined by the promiscuous peptides due to a relatively invariant interaction with MHC molecule (112, 113), it has been demonstrated that TCR:pMHC recognition is influenced by peptide length, physicochemical properties, amino acid sequence especially at central and anchor residues, MHC haplotype and structural landscape (114). Over the years, TCR:pMHC interactions have been extensively studied, thus providing a wealth of data for modeling to be performed from different perspectives (Figure 1). In the following subsections, we will describe a number of discriminative features shown to associate with immunogenicity.
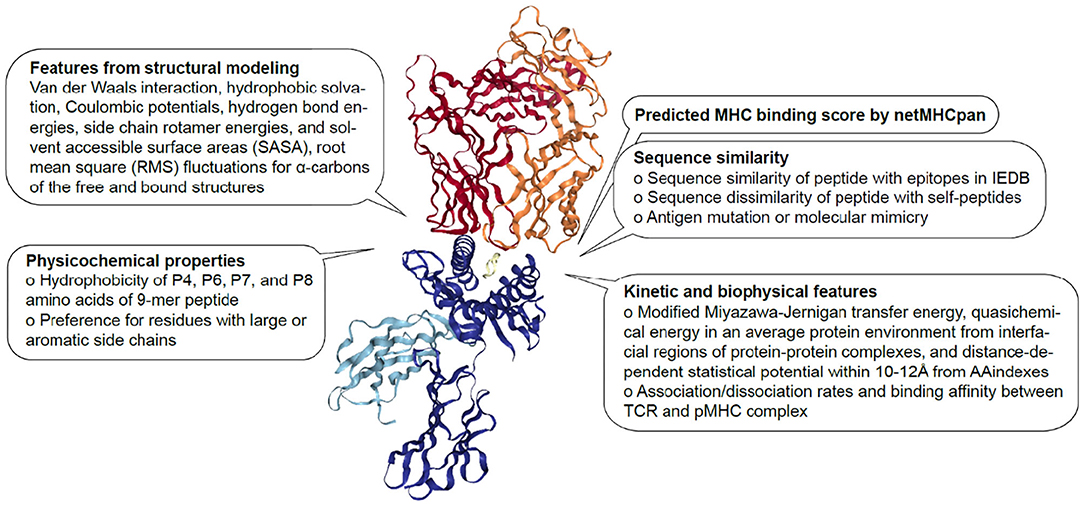
Figure 1. Features associated with TCR:pMHC interaction. Description of sequence-based, structural, kinetic, and biophysical features previously found to be associated with pMHC recognition by TCR The diagram is 1G4 TCR bound to NY-ESO-1/HLA-A*02:01 (PDB 2BNR) where TCRα, TCRβ, MHC, β2-microglobulin and peptide are colored in orange, red, blue, light blue, and yellow, respectively.
Biophysical and Kinetic Features
In addition to the discovery of hotspot residues through TCR sequence alignments (16), biophysical studies revealed that some interactions at the pMHC surface seem more important in triggering a T cell responses (112, 115). This raised a hypothesis that even TCRs sharing a similar TCR footprint may have their unique “interaction profile” (38), and claimed that while conventional hotspots were attributed to amino acid residues, the concept of hotspot should be expanded to account for interaction features, such as hydrogen bonds, van der Waals forces, pockets and coordination of water molecules (115, 116).
A collective effort has identified biological and physical parameters that modulate TCR:pMHC engagement and T cell response [reviewed in (117)], which include binding affinity, association and dissociation rates, half-life of interaction, changes in heat capacity, competition for CD3 molecules and conformational adaptability of TCRs (117–132). Taking these biophysical and kinetic features into account may help to effectively reflect the two-dimensional (2D) and dynamic in vivo system whilst modeling TCR:pMHC interactions.
Although we are not currently in the position to perform an ab initio prediction based on biophysical and/or structural data, recent studies have started to incorporate physical and chemical properties in developing a generalized TCR scoring function. In a multi-linear regression model, Riley et al. utilized 16 full-atom Rosetta terms (133, 134) and six flexibility terms as predictor variables and experimental binding energies as response variables to estimate the effect of point mutations on thermodynamic stability of the TCR. They identified six significant features, 4 structural terms (van der Waals attractive and repulsive forces, solvation energies and sidechain hydrogen bonding) and 2 flexibility terms (root mean square (RMS) fluctuations for α-carbons of the free and bound structures) contributing to improved performance of the scoring function.
In another application, Haider et al. aimed to engineer an affinity enhanced A6 TCR targeting Tax peptide/HLA-A2 complex (135). They created a set of 219 fitted scoring functions using kinetic and potential energy terms and identified a function (named ZAFFI score) best capable of reproducing affinity changes upon 648 mutations on ovomucoid turkey inhibitor molecule. This work was followed by Pierce et al. identifying an improved scoring function (ZAFFI 1.1) having a higher correlation for a set of DF5 point mutations (136). ZAFFI 1.1 includes six terms: van der Waals attractive and repulsive components, desolvation, intra-residue clash, hydrogen bonding and Coulombic electrostatic force.
In a recent review, Spear et al. have highlighted the significance of considering the previously unappreciated complex relationship between kinetic, cellular and structural patterns that modulate antigen specificity and TCR cross-reactivity in designing TCRs (117). Likewise, such parameters should be taken into account in modeling TCR:pMHC cross-recognition propensities as well as antigen specificity.
Features From Structural Modeling
The 3D crystal structures of T cell receptor and their cognate pMHCs have been resolved and deposited in protein database (PDB) (137). The structural T cell receptor database (STCRDab) contains >340 PDB entries and >480 αβTCR structures and facilitates analysis and visualization of TCR structures as well as individual CDR loops (138). This database includes information about MHC type, antigen specificity, Vα-Vβ pairing, orientation, dissociation rate (Kd) and CDR type. Additionally, TCR3d provides information on germline gene usage, antigen binding mode and interface features (139).
Based on the cognate peptide, MHC and TCR structures in the aforementioned database, there have been a number of attempts to accurately predict peptide-MHC conformations, including docking algorithms (140, 141), protein threading (142), all-atom molecular dynamics (MD) simulations (143–145), energy minimization (146) and hybrid of these approaches (147). Likewise, approaches to model pMHC-TCR include MD or Monte Carlo simulations, TCR:pMHC hydrogen bond network analysis (148, 149), binding free energy simulation (150) and CDR loop characterization (130). Both rigid and flexible docking protocols have been proposed to assemble unbound structures (151).
The features retrieved from structural modeling were utilized to predict TCR:pMHC complex formation (151, 152). Mendes et al. applied electrostatic potential and topography data to conduct structure-based prediction among viral epitopes. By using structural features as input for a multivariate statistical model, they showed that use of accessible surface area (ASA, Table 3) adds value to infer immunogenicity and cross-recognition potential. Similarly, Riley et al. showed that hydrophobic SASA and hydrophobic solvation energy values at peptide positions 5, 7, and 8 were in the top 10% of all weights in the neural network for predicting immunogenicity (109).
Recent structural studies have emphasized the importance of structural and physicochemical homology in T cell receptor cross-reactivity (112, 153–160). For example, screening libraries of ligands against 2B4 and 42F3 TCRs revealed that peptides containing sequence motifs at specific positions were found to participate in similar TCR contact networks (112, 153). Collectively, the shared peptide conformation and core residues were shown to limit structural diversity and facilitate cross-recognition.
However, Riley et al. questioned the notion that the pools of ligands for a given TCR is built around core regions of restricted structural and chemical space, and showed that T cell receptors can also cross-react between ligands with little structural or physicochemical commonalities. They demonstrated that the DMF5 TCR can cross-react with divergent antigens by unanticipated rearrangements in peptide and presenting MHC molecules, including binding-induced peptide register shifts. Although dramatic rearrangements did not translate into molecular mimicry, this TCR was capable of cross-reacting with distinct classes of epitopes. Likewise, cross-reactivity has been observed from unrelated pathogens even with a low level of structural homology (31, 33, 68, 69, 161, 162).
These findings suggest that while structural homology may inform cross-recognition potential of peptides having the same structural configuration, current methods are suboptimal in predicting polyspecificity across different classes. Moreover, amino acid mutations at positions distant from direct recognition sites may also have a substantial effect on TCR:pMHC interaction e.g., change in binding parameters and/or structural conformation, and can only be validated by experimentation (163). Altogether these may imply an immense breadth of promiscuity beyond our expectations based on current understanding.
Elements to Consider in Modeling Immunogenicity or Cross-Reactivity of TCRs
Given the limitations in the current methods to reflect and predict TCR:pMHC recognition, here we describe a few considerations to make in building algorithms to predict immunogenicity or cross-recognition potential.
First, a key challenge in developing machine learning and statistical models to predict immunogenicity is the lack of true negative datasets for TCR-epitope interaction as well as cross-reactivity information. Several groups tackled this limitation by simulating a background or negative data (93, 96, 97, 164). Jurtz et al. approached the problem by creating incorrect combinations of TCRs and peptides i.e., linking TCR sequences with a random peptide different from the cognate target, and produced a balanced set of positive and negative data. Alternatively, Ogishi et al. retrieved the latest set of all characterized peptides and examined coexistence of positive and negative assay results to classify immunogenicity in a population-level (96). Given the limited coverage of cross-reactivity spectrum, a rational simulation would supplement the true negative data for training a classifier.
Additionally, the existing datasets are in a binary format of being immunogenic or non- immunogenic, whereas it is evident that the T cell response is a continuum and comes in different flavors from a mild to a very strong response and varies in functional outcomes such as differential cytokine production. Quantitative T cell response measures associated with each epitope will open a new avenue for rigorous modeling.
Second, current distance measures are mainly context specific and do not capture the true immunogenic capacity of the input peptides. For example, Grouping Lymphocyte Interactions by Paratope Hotspots (GLIPH) and TCRDist that are aimed to detect common antigen specificity groups may not be effective in estimating breadth and/or constituents of the cross-reactome. Cancer specific immunogenic neoantigens that are used for cancer vaccine targets are mainly different from the wild type by only a single point mutation. Engineered affinity-enhanced TCRs have recently been shown to generate unpredicted cross-reactivity even by a single amino acid substitution (64, 165, 166). As such, a naive sequence-based metrics such as Euclidean distance may pose limitation and thus development of a distinct distance metric for evaluating cross-recognition potential may be required.
Third, there is a considerable heterogeneity in the experimental methodologies employed in assessing T cell responses. Although standardizing T cell assays into a single readout is practically difficult, accuracy of predictive algorithms may be enhanced by reflecting the sensitivity and specificity of assays employed for characterizing each epitope.
Fourth, up to date, exhaustive screenings have been performed based on an assumption of invariant MHC interaction. However, previous studies suggested the ability of a TCR to recognize peptides bound by non-canonical HLA molecules (167, 168). In addition to cross-reactivity of virus-specific T cells to HLA-A and -B molecules, van der Zwan et al. reported cross-reactivity of HLA-B*08:01-restricted EBV-specific T cell against HLA-C*01:02 (169). From a clinical perspective, a severe off-target toxicity was reported by adoptive cell transfer of T cells targeting HLA-A*01:01 MAGE-A3 complexes by binding to a Titin-derived peptide displayed on HLA-A*01:01 (55). Thus, it may be necessary to screen for peptides bound on non-predicted HLA alleles to project the complete scope of cross-reactivity, and we need to keep in mind that the current sets of data may only reflect the tip of an iceberg.
Lastly, we need to keep in mind that while TCR:pMHC interactions exhibit a remarkable capacity of discrimination, they are often sloppy and cross-reactive. Nevertheless, as exemplified by thymic selection, weaker affinities play an essential role in underpinning the sensitive detection of a wide range of cognate antigens yet keeping it well-balanced from self-reactivity (170, 171). Moreover, low and high-affinity T cells may involve in biological processes differently in regards to e.g., effector and memory differentiation, metabolic reprogramming, and immune response in specific conditions (172, 173). Given the dynamic nature of weak interactions and their potential functional implications, we may need to divert from lessons learned from well-optimized interfaces, such as antigen-antibody binding. We should note that due to challenges involved in measuring low affinity interactions, existing data may be biased in favor of high affinity interactions (174), and may also need to reexamine scoring functions and parameters to reflect dynamic interplay of low and high-affinity T cells for an efficient immune response (175).
Predicting Common Specificity Group of T Cell Receptors
The amino acid sequence of paired TCR defines its binding specificity. However, we still do not have a full understanding of the mechanisms underpinning the recognition of pMHC complexes by their cognate TCRs. In the last few years, there have been mathematical and computational efforts to find systematic ways to cluster TCRs based on their likely antigen specificity, a phenomenon known as defining common antigen specificity groups.
To identify TCRs specific to a given antigen, one will require to sort and sequence naïve and antigen experienced T cell repertoires. Recent advances in both bulk and single cell sequencing technologies facilitates generation of such datasets in a high throughput manner. A dedicated set of algorithms and software tools will allow computational biologist to further analyze and profile TCR repertoires (176–178). This includes MIXCR and IMGT V-QUEST for assigning raw sequence reads into VJ genes and CDR3 sequences, and VDJtools (179) to compute VJ gene usage statistics as well as repertoire diversity.
Such complementary biological assays and computational platforms enabled robust generation and analysis of millions of TCRs in a single experiment. Importantly, the curated sequences have been deposited in databases such as VDJdb (180) and McPAS-TCR (181). The VDJdb contains >60,000 TCR specificity records associated with their epitope and MHC, and McPAS-TCR holds >5,000 TCRs associated with pathogenic conditions e.g., pathogen infection, cancer and autoimmunity.
The accumulation of so many antigen-specific TCR sequences, on one hand, urged the development of systematic methods to group TCR sequences according to, for example, their shared antigen specificity, and on the other hand, opened an opportunity to conduct in-depth characterization of antigen-specific TCR repertoires, find shared and conserved features and develop a distance measure that permits clustering and visualization of the TCR space (Figure 2). In the following subsections, we will be looking into a number of such methods.
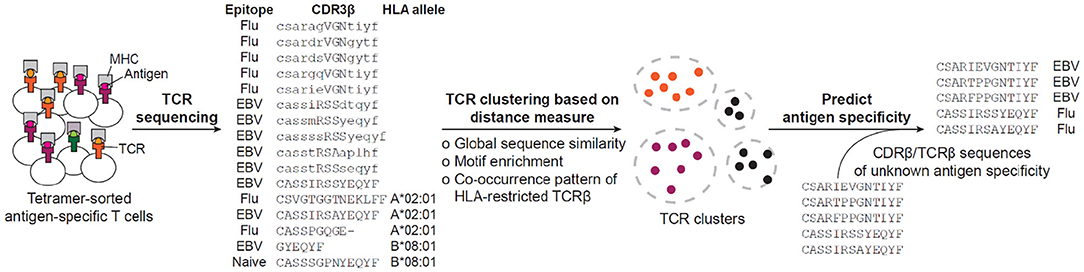
Figure 2. Current workflow for predicting antigen specificity of TCRs. The tetramer-sorted antigen specific CDR3β or TCRβ are clustered by distance measure defined by either global sequence similarity, motif enrichment or sequence co-occurrence pattern. Then, specificity clusters are investigated for their descriptive features, such as enrichment of common V-genes, CDR3 length, clonal expansions, and motif significance, to be considered in making the prediction of antigen specificity. Based on the collection of identified features, previously uncharacterized CDR3βs or TCRβs are predicted for their antigen specificity. The example sequences have been retrieved from (16, 182).
Algorithms to Predict Antigen-Specificity of TCRs
The above mentioned rationales have formed the foundation for several recent studies trying to predict specificity groups of TCRs based on their TCR or CDR sequences (15–18, 182–187) (Table 2). By analyzing the collection of TCR sequences, researchers have tried to identify shared features among antigen-specific TCRs and to develop a distance-based classifier capable of assigning previously unobserved TCRs to characterized repertoires. Here are examples of different approaches employed to predict common specificity groups of TCR.
Co-occurrence Pattern of TCR Sequences
While TCRs are rarely cross-reactive across HLA haplotypes (193), they can be highly promiscuous to different peptides presented on the same HLA (24, 112, 113) and this invariant interaction between TCR:pMHC also confers T cell specificity. Based on this principle, a recent study by DeWitt et al. showed that despite the diversity and complexity of TCR repertoire and pMHC, there exist common patterns across individuals exposed to the same disease. They leveraged this finding to cluster TCRs by their co-occurrence pattern, associated TCR clusters to HLA (i.e., HLA restriction) and predicted antigen specificity of the TCR cluster (182). Using repertoire sequencing data coupled with high-resolution MHC genotyping, they demonstrated striking imprints of common pathogens and clusters of co-occurring TCRs that may represent markers of shared immune exposure.
CDR3β Sequence Similarity
As a result of somatic recombination, TCR sequences produce three complementary determinant region (CDR) loops, where CDR1 and CDR2 of α- and β-chains are conventionally believed to govern the interaction with an MHC molecule, and hypervariable CDR3α and CDR3β loops to guide specific engagement of TCRs with MHC-bound cognate peptides (194, 195). A number of studies have observed structural rearrangement of CDR loops during TCR:pMHC interaction. The range of motion is between 0.3 and 11.4Å, where CDR3 loop generally undergoes the largest shifts (196).
Based on the understanding of CDR loops with pMHC interaction, some progress has been made in predicting specificity groups of TCRs based on the similarity of short stretches of TCR amino acid sequences, known as motifs, mainly within CDR3 region (15, 16, 18, 93, 192). Glanville et al. aligned amino acid sequences of all reported TCR:pMHC crystal structures and identified stretches of three to five contiguous amino acids at specific positions in TCRβ CDR3 to be positioned within 5Å of peptide residues. Building upon this finding, they sorted Epstein-Barr virus (EBV), cytomegalovirus (CMV) and influenza-specific T cells, performed single cell sequencing of isolated TCRs or bulk TCRβ sequencing, then again observed similarity in short sequences of CDR3s within hundreds of antigen-specific T cells. The authors proceeded to incorporate these observations into an algorithm for Grouping Lymphocyte Interactions by Paratope Hotspots (GLIPH) that allowed them to cluster TCRs with comparable levels of specificities. Along with GLIPH, several algorithms have recently been proposed such as TCRDist (15) and TCRnet (17), which also relies on CDR3s to cluster TCRs based on the amino acid sequence similarity.
Improving Accuracy of TCR Specificity Group Prediction
Although current algorithms have been applied in multiple biological contexts such as Alzheimer's disease (197), narcolepsy (198), and PD-1 blockade treatment (199), recent studies reported suboptimality of the algorithms (18) given the limited number of crystal structures concentrated around a few frequently observed viral antigens. Here we present several elements that may facilitate improvement of predictive accuracy.
Extending Current Algorithms From CDR3β Amino Acids
A number of recent studies have suggested that integrating information across all six CDRs, instead of considering CDR3α or CDRβ independently, would likely yield a higher performance (15, 16, 182). In particular, Lanzarotti et al. evaluated TCR target prediction models based on incorporation of full TCR paired sequences, 6 CDR loops and/or structural similarity (200). The best performing model was the one incorporating all CDR1, 2, 3 α and β information with greater weight given to CDR3αβ, plus adding structural information (root mean square deviation, RMSD) moderately but consistently improved the performance. Of interest, placing greater weight to CDRβ sequences over CDRα led to decreased predictive power compared to even the flat model. Thus, developing a distance measure that incorporates all CDR1, 2, 3 α and β sequences is likely to demonstrate a higher predictive performance than the current TCR specificity group algorithms.
In addition, translating CDR amino acid sequences into their physicochemical properties and using their inherent properties to cluster TCRs into specificity groups may bring another step forward. Ostmeyer et al. developed a statistical classifier of T cell receptor repertoire that distinguishes tumor tissue from patient-matched healthy tissue of the same organ (201). The classifier was based on physicochemical motifs in CDR3 of TCRβ chains. Here, 4-mer amino acid sequences were represented by their physicochemical properties using Atchley factors—polarity, secondary structure, molecular size/volume, codon diversity and electrostatic charge—and achieved classification accuracy of 93 and 94% for colorectal and breast cancer, respectively.
From previous efforts to reduce dimensionality of a large number of possibly co-linear amino acids properties into small number of orthogonal properties that maintain most of the information contained in the original set, physicochemical properties of amino acids have been characterized and summarized into e.g., 10 Kidera factors (202) and 5 Atchley factors (203). Analyzing occurrence of “physicochemical motifs” in TCRs along with structural features e.g., RMSD will bring one step closer to accurately identifying TCR specificity groups.
Application of Single Cell Technologies for Paired TCRαβ Profiling
While bulk TCR sequencing revolutionized characterization of TCR repertoire in different pathological settings e.g., tumor immunology and autoimmunity (204), β chain analysis has always been the main target due to its higher combinatorial potential and its ability to represent as “unique label” for a T cell after allelic exclusion. However, many studies highlighted the pairing of α and β chain to reflect biological function of a T cell in vivo (205, 206) and that even α chain alone can accurately differentiate T cell subsets by its function and phenotype (207, 208). Izraelson et al. narrowed TCR complexity by fixing TCRβ background and thus allowing TCR diversity and antigen specificity to be determined by TCRα chain alone. Then, using a similarity measure, R metric describing the correlation of overlapping clonotype frequencies, could “digitally” differentiate their TCRα repertoire from spleen, thymus and lymph nodes into functional T cell subsets of Treg, Teff, and naïve CD4 T cells. This illustrates that while TCRβ may operate as “unique label” of a T cell, TCRα may as well encode essential information about its phenotype, function and specificity.
Recent advancement in single cell approaches opened the door for elucidating how particular α-β pairing contributes to antigen specificity. In particular, several groups have started to implement single cell platforms for simultaneous identification of TCRαβ sequence and antigen specificity in a high-throughput manner across multiple pMHCs (5, 6, 209). For example, Bentzen et al. applied a large library of >100 DNA barcode-labeled MHC multimers to stain antigen-specific T cells, isolate T cells bound by MHC multimers using flow cytometry followed by a droplet-based single cell sequencing to capture αβTCR transcripts and the MHC-associated DNA barcodes in parallel.
The potential benefits of identifying TCR αβ pairs coupled to antigen specificities include but are not limited to: (i) identifying unique CDR3 α/β signatures dictating epitope recognition for possible applications across the field of adaptive immunity e.g., efficient design of TCRs for vaccine development or targeted immunotherapy (210), (ii) portraying T cell ancestry in response to pathogen exposure, (iii) investigating which functional T cell subsets have undergone clonal expansion in response to different antigens, (iv) examining distinct phenotypic and functional properties of T cells responded to different antigens, and (v) identifying TCRαβ heterodimers losing functional integrity in vitro, which will be useful for therapeutic applications (211).
Importantly, the large number of paired TCRαβs coupled to antigen specificity can be fed into computational models improving accuracy of prediction. The exhaustive list of recognition patterns combined with increasing structural information about TCR:pMHC interaction will assist prediction of specific TCR:pMHC interaction based on TCR sequence (10). Of interest, with increasing reports focusing on TCR repertoire of antigen-specific populations, the latest studies have started to compare predictive performance on different datasets. Thakkar et al. have analyzed repertoires from a twin pair study (212), antigen-specific data from GLIPH and TCRDist studies (15, 16) and pathology-associated data from McPAS-TCR to evaluate the trade-off between sensitivity and specificity of predictive algorithms in different pathology, antigen, MHC restriction settings (187). As discussed by Thakkar et al., while datasets were analyzed individually in the study, integrating multiple datasets and large number of paired TCRαβs should provide insights into common modalities of recognition and broader functional associations across antigens from different pathologies.
The Interface Between Common Specificity Group and Cross-Reactivity of TCR to Model the Landscape of Interaction Propensities
Despite interest in mapping the TCR:pMHC interactions, a combinatorial approach exploring the mutation space of TCRs against corresponding peptide cross-reactome has not been exhaustively performed. Thus, it would be exceptionally challenging to account for the whole range of available TCRs and surveilling pMHCs.
Depicting the cross-recognition of TCRs and pMHCs in >10 (6) space, modeling TCR:pMHC landscape should be taken as a cross-talk between unique and representative clusters of pMHCs and TCRs rather than individual entities (Figure 3). However, as discussed by Bradley and Thomas we currently do not know whether TCRs closely related by antigen specificity algorithms e.g., GLIPH or TCRDist are expected to have similar cross-reactome (213). An intuitive answer would be that TCRs with a shorter distance, especially those within the same cluster, will have a greater overlap of cross-reactive peptides. However, as elucidated by structural studies, sequence similarity cannot adequately represent cross-recognition potential as TCRs may have multiple configurations for different classes of epitopes (67).
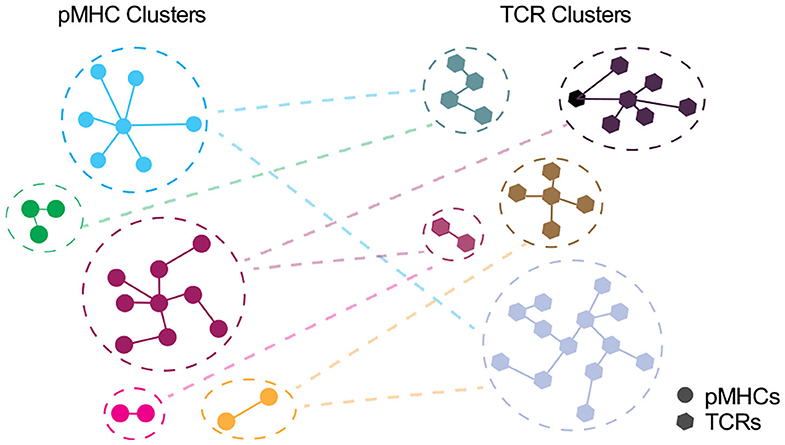
Figure 3. Interplay between unique clusters of pMHCs and TCRs. In an ideal world with an accurate distance measure, pMHCs in the same cluster should share the common specificity toward TCRs and vice versa. Each node denotes pMHC (circle) or TCR (polygon) entities and edge denote the distance with the closest pMHC or TCR, respectively.
Therefore, modeling this dynamic interplay may require the development of an accurate distance measure to group TCRs informative of their antigen specificity and/or cross-reactivity. This will require assessment of all identified features, such as paired TCRαβ sequences, n-mer motifs, physicochemical properties as well as structural, physical and kinetic parameters, to derive a minimum set of features with maximum association to immunogenicity. These features will become a toolkit for developing TCR and pMHC distance measures to discriminate >106 TCRs and >1018 peptides into designated clusters. Following the classification of clusters, the relationship between TCR and pMHC clusters can further be explored—it may segregate into a linear function or may yield an indistinct pattern where even the repertoires for closely related epitopes have divergent landscape with a very limited overlap.
Conclusion and Outlook
Here we discuss two fundamental principles of TCR:pMHC interaction, antigen specificity and TCR cross-reactivity. Modeling the underlying principles by cellular, kinetic, and structural features will deepen our understanding on the organizational principle of TCR repertoires.
Recent technological advancements have opened doors for screening antigen-specific TCRs and cross-reactive peptides in a high-throughput manner. In particular, MHC multimer screening in combination with multimodal single cell technologies increased the breadth of T cell analysis by allowing integration of antigen specificity with immune repertoire, transcriptomic and proteomic profiling (7, 19, 20). Further developments in biological systems will provide larger training sets for the in silico analyses. These analyses simultaneously give information on: (i) paired TCR sequences, (ii) pMHC specificities across a large epitope library, and (iii) transcriptomics and proteomics measurements of single T cells profiled in parallel. The multi-omics integration will enable in-depth analysis of phenotypic and functional states of each T cell and correlate with their TCR sequences and pMHC interaction.
The present algorithms have not distinguished TCR repertoires by their functional subsets, such as CD4+ and CD8+ T cells with pro-inflammatory or regulatory functions, largely due to lack of sufficient annotations. Given that each subsets have distinct dynamics according to pathogenic conditions, e.g., viral infection, cancer or autoimmunity, utilization of subset-specific TCR repertoire may further improve predictability of epitope immunogenicity (96). In this regard, the recent efforts to integrate TCR sequencing with transcriptomic and proteomic profiling in a single cell level will enrich present-day datasets (19, 20).
Along with an increasing wealth of experimental and sequencing data, there have been advancements in in silico approaches to analyze, model and predict TCR:pMHC interaction. Further efforts will provide insights into specific TCR recognition and organizational principles of the repertoire and support a wide range of applications from discovering potential drivers of allergy, autoimmunity and tolerance (160, 214–216) to identifying cancer neoantigens and developing personalized vaccines (217–219).
For instance, recent studies have focused on a rational computer-aided approach to TCR engineering as a more predictable and safer approach to TCR design (135, 136, 220–222). They used a fine manipulation of structural topography of TCR:pMHC interaction and specific kinetic parameters to better control the potential for cross-reactivity (223). Another study exploited structure-guided computational design of DMF5 TCR by using both “positive design” to enhance peptide-centric binding and “negative design” to weaken interaction with the MHC (136). While the positive design alone introduced new cross-reactivities thus weakened T cell potency, a combination of both positive and negative design maintained the recognition potential whilst cross-reactivity toward other MART-1 homologs was reduced and cross-reactivity against more divergent class of epitopes was eliminated.
Ultimately, building a complete map portraying the TCR:pMHC interface will provide opportunities to describe the response to dynamic interactions in the immune system. The examples include: (i) dynamic changes of antigen-specific TCR repertoire after adoptive transfer (224), (ii) mechanism of molecular mimicry and preferential directionality in antigen specificity, and (iii) influence of private repertoire and immunological history on antigen specificity. Finally, an extensive understanding of dynamic T cell response will allow development of personalized treatments by taking into account the individual's endogenous ability to target a given disease-specific antigen as well as the personal risk of autoimmunity.
Author Contributions
HK conceived and designed the study. CL conducted literature review. HK and CL wrote the manuscript with contributions from GN, MS, GO, and AS. HK and AS supervised the project. All authors contributed to the interpretation of the observations.
Funding
This work has been supported by Medical Research Council UK. HK, MS, and GN were funded by the MRC HIU core grant. CL was funded by UK National Institute of Health Research (NIHR). AS was funded by a Wellcome Investigator Award (219523/Z/19/Z), the UK Medical Research Council, NIHR, awards from Bristol-Myers Squibb and UCB. AS is an NIHR Senior Investigator and acknowledges support from the Oxford NIHR Biomedical Research Centre. The views expressed are those of the author(s) and not necessarily those of the NHS, The NIHR, or the Department of Health, UK.
Conflict of Interest
GO has served on advisory boards or holds consultancies or equity with Eli Lilly, Novartis, Janssen, Sanofi, Orbit Discovery and UCB Pharma, and has undertaken clinical trials with Atopix, Regeneron/Sanofi, Roche.
The remaining authors declare that the research was conducted in the absence of any commercial or financial relationships that could be construed as a potential conflict of interest.
Acknowledgments
HK would like to dedicate this study to Prof. Cerundolo who introduced him to the amazing world of T cell immunology. We wish to thank Omer Dushek, Agne Antanaviciute, Paul Buckley, Jeongmin Woo and Isaac Woodhouse for critical reading of the manuscript.
References
1. Matzinger P, Bevan MJ. Why do so many lymphocytes respond to major histocompatibility antigens? Cell Immunol. (1977) 29:1–5. doi: 10.1016/0008-8749(77)90269-6
2. Mason D. A very high level of crossreactivity is an essential feature of the T-cell receptor. Immunol Today. (1998) 19:395–404. doi: 10.1016/S0167-5699(98)01299-7
3. Sewell AK. Why must T cells be cross-reactive? Nat Rev Immunol. (2012) 12:669–77. doi: 10.1038/nri3279
4. Frank SA. Immunology and Evolution of Infectious Disease. Princeton, NJ: Princeton University Press (2002).
5. Bentzen AK, Such L, Jensen KK, Marquard AM, Jessen LE, Miller NJ, et al. T cell receptor fingerprinting enables in-depth characterization of the interactions governing recognition of peptide–MHC complexes. Nat Biotechnol. (2018) 36:1191–6. doi: 10.1038/nbt.4303
6. Zhang S-Q, Ma K-Y, Schonnesen AA, Zhang M, He C, Sun E, et al. High-throughput determination of the antigen specificities of T cell receptors in single cells. Nat Biotechnol. (2018) 36:1156–9. doi: 10.1038/nbt.4282
7. Pan X, Huang L-C, Dong T, Peng Y, Cerundolo V, McGowan S, et al. Combinatorial HLA-peptide bead libraries for high throughput identification of CD8+ T cell specificity. J Immunol Methods. (2014) 403:72–78. doi: 10.1016/j.jim.2013.11.023
8. Bijen HM, van der Steen DM, Hagedoorn RS, Wouters AK, Wooldridge L, Falkenburg JHF, et al. Preclinical strategies to identify off-target toxicity of high-affinity TCRs. Mol Ther. (2018) 26:1206–14. doi: 10.1016/j.ymthe.2018.02.017
9. Ekeruche-Makinde J, Clement M, Cole DK, Edwards ESJ, Ladell K, Miles JJ, et al. T-cell receptor-optimized peptide skewing of the T-cell repertoire can enhance antigen targeting. J Biol Chem. (2012) 287:37269–81. doi: 10.1074/jbc.M112.386409
10. Gee MH, Han A, Lofgren SM, Beausang JF, Mendoza JL, Birnbaum ME, et al. Antigen identification for orphan T cell receptors expressed on tumor-infiltrating lymphocytes. Cell. (2018) 172:549–63.e16. doi: 10.1016/j.cell.2017.11.043
11. Joglekar AV, Leonard MT, Jeppson JD, Swift M, Li G, Wong S, et al. T cell antigen discovery via signaling and antigen-presenting bifunctional receptors. Nat Methods. (2019) 16:191–8. doi: 10.1038/s41592-018-0304-8
12. Kisielow J, Obermair F-J, Kopf M. Deciphering CD4 + T cell specificity using novel MHC–TCR chimeric receptors. Nat Immunol. (2019) 20:652–62. doi: 10.1038/s41590-019-0335-z
13. Li G, Bethune MT, Wong S, Joglekar AV, Leonard MT, Wang JK, et al. T cell antigen discovery via trogocytosis. Nat Methods. (2019) 16:183–90. doi: 10.1038/s41592-018-0305-7
14. Kula T, Dezfulian MH, Wang CI, Abdelfattah NS, Hartman ZC, Wucherpfennig KW, et al. T-scan: a genome-wide method for the systematic discovery of T cell epitopes. Cell. (2019) 178:1016–28.e13. doi: 10.1016/j.cell.2019.07.009
15. Dash P, Fiore-Gartland AJ, Hertz T, Wang GC, Sharma S, Souquette A, et al. Quantifiable predictive features define epitope-specific T cell receptor repertoires. Nature. (2017) 547:89–93. doi: 10.1038/nature22383
16. Glanville J, Huang H, Nau A, Hatton O, Wagar LE, Rubelt F, et al. Identifying specificity groups in the T cell receptor repertoire. Nature. (2017) 547:94–8. doi: 10.1038/nature22976
17. Pogorelyy MV, Shugay M. A framework for annotation of antigen specificities in high-throughput T-cell repertoire sequencing studies. Front Immunol. (2019) 10:2159. doi: 10.3389/fimmu.2019.02159
18. Zhang H, Liu L, Zhang J, Chen J, Ye J, Shukla S, et al. Investigation of antigen-specific T-cell receptor clusters in human cancers. Clin Cancer Res. (2019) 26:1359–71. doi: 10.1158/1078-0432.CCR-19-3249
19. Peterson VM, Zhang KX, Kumar N, Wong J, Li L, Wilson DC, et al. Multiplexed quantification of proteins and transcripts in single cells. Nat Biotechnol. (2017) 35:936–9. doi: 10.1038/nbt.3973
20. Stoeckius M, Hafemeister C, Stephenson W, Houck-Loomis B, Chattopadhyay PK, Swerdlow H, et al. Simultaneous epitope and transcriptome measurement in single cells. Nat Methods. (2017) 14:865–8. doi: 10.1038/nmeth.4380
21. Cornberg M, Clute SC, Watkin LB, Saccoccio FM, Kim S-K, Naumov YN, et al. CD8 T cell cross-reactivity networks mediate heterologous immunity in human EBV and murine vaccinia virus infections. J Immunol. (2010) 184:2825–38. doi: 10.4049/jimmunol.0902168
22. Degauque N, Brouard S, Soulillou J-P. Cross-reactivity of TCR repertoire: current concepts, challenges, and implication for allotransplantation. Front Immunol. (2016) 7:89. doi: 10.3389/fimmu.2016.00089
23. Wucherpfennig KW, Allen PM, Celada F, Cohen IR, De Boer R, Garcia KC, et al. Polyspecificity of T cell and B cell receptor recognition. Semin Immunol. (2007) 19:216–24. doi: 10.1016/j.smim.2007.02.012
24. Wooldridge L, Ekeruche-Makinde J, Berg HA. A single autoimmune T cell receptor recognizes more than a million different peptides. J Biol Chem. (2012) 287:1168–77. doi: 10.1074/jbc.M111.289488
25. Cornberg M, Kenney LL, Chen AT, Waggoner SN, Kim S-K, Dienes HP, et al. Clonal exhaustion as a mechanism to protect against severe immunopathology and death from an overwhelming CD8 T cell response. Front Immunol. (2013) 4:475. doi: 10.3389/fimmu.2013.00475
26. Pomés A, Schulten V. Cross-reactivity in allergy: a double-edged sword. Allergy. (2020) 75:9–11. doi: 10.1111/all.13993
27. Selin LK, Cornberg M, Brehm MA, Kim S-K, Calcagno C, Ghersi D, et al. CD8 memory T cells: cross-reactivity and heterologous immunity. Semin Immunol. (2004) 16:335–47. doi: 10.1016/j.smim.2004.08.014
28. Welsh RM, Fujinami RS. Pathogenic epitopes, heterologous immunity and vaccine design. Nat Rev Microbiol. (2007) 5:555–63. doi: 10.1038/nrmicro1709
29. Chen AT, Cornberg M, Gras S, Guillonneau C, Rossjohn J, Trees A, et al. Loss of anti-viral immunity by infection with a virus encoding a cross-reactive pathogenic epitope. PLoS Pathog. (2012) 8:e1002633. doi: 10.1371/journal.ppat.1002633
30. Wlodarczyk MF, Kraft AR, Chen HD, Kenney LL, Selin LK. Anti–IFN-γ and peptide-tolerization therapies inhibit acute lung injury induced by cross-reactive influenza a–specific memory T cells. J Immunol. (2013) 190:2736–46. doi: 10.4049/jimmunol.1201936
31. Acierno PM, Newton DA, Brown EA, Maes LA, Baatz JE, Gattoni-Celli S. Cross-reactivity between HLA-A2-restricted FLU-M1:58–66 and HIV p17 GAG:77–85 epitopes in HIV-infected and uninfected individuals. J Transl Med. (2003) 1:3. doi: 10.1186/1479-5876-1-3
32. Che JW, Selin LK, Welsh RM. Evaluation of non-reciprocal heterologous immunity between unrelated viruses. Virology. (2015) 482:89–97. doi: 10.1016/j.virol.2015.03.002
33. Clute SC, Naumov YN, Watkin LB, Aslan N, Sullivan JL, Thorley-Lawson DA, et al. Broad cross-reactive TCR repertoires recognizing dissimilar Epstein-Barr and influenza A virus epitopes. J Immunol. (2010) 185:6753–64. doi: 10.4049/jimmunol.1000812
34. Setia MS, Steinmaus C, Ho CS, Rutherford GW. The role of BCG in prevention of leprosy: a meta-analysis. Lancet Infect Dis. (2006) 6:162–70. doi: 10.1016/S1473-3099(06)70412-1
35. Stewart AJ, Devlin PM. The history of the smallpox vaccine. J Infect. (2006) 52:329–34. doi: 10.1016/j.jinf.2005.07.021
36. Su LF, Kidd BA, Han A, Kotzin JJ, Davis MM. Virus-specific CD4+ memory-phenotype T cells are abundant in unexposed adults. Immunity. (2013) 38:373–83. doi: 10.1016/j.immuni.2012.10.021
37. Welsh RM, Che JW, Brehm MA, Selin LK. Heterologous immunity between viruses. Immunol Rev. (2010) 235:244–66. doi: 10.1111/j.0105-2896.2010.00897.x
38. Antunes DA, Rigo MM, Freitas MV, Mendes MFA, Sinigaglia M, Lizée G, et al. Interpreting T-cell cross-reactivity through structure: implications for TCR-based cancer immunotherapy. Front Immunol. (2017) 8:1210. doi: 10.3389/fimmu.2017.01210
39. Nie S, Lin S-J, Kim S, Welsh RM, Selin LK. Pathological features of heterologous immunity are regulated by the private specificities of the immune repertoire. Am J Pathol. (2010) 176:2107–112. doi: 10.2353/ajpath.2010.090656
40. Valitutti S, Müller S, Dessing M, Lanzavecchia A. Different responses are elicited in cytotoxic T lymphocytes by different levels of T cell receptor occupancy. J Exp Med. (1996) 183:1917–21. doi: 10.1084/jem.183.4.1917
41. van den Berg HA, Ladell K, Miners K, Laugel B, Llewellyn-Lacey S, Clement M, et al. Cellular-level versus receptor-level response threshold hierarchies in T-cell activation. Front Immunol. (2013) 4:250. doi: 10.3389/fimmu.2013.00250
42. Price DA, Sewell AK, Dong T, Tan R, Goulder PJR, Rowland-Jones SL, et al. Antigen–specific release of β-chemokines by anti-HIV-1 cytotoxic T lymphocytes. Curr Biol. (1998) 8:355–8. doi: 10.1016/S0960-9822(98)70138-1
43. Hemmer B, Stefanova I, Vergelli M, Germain RN, Martin R. Relationships among TCR ligand potency, thresholds for effector function elicitation, and the quality of early signaling events in human T cells. J Immunol. (1998) 160:5807–14.
44. Abu-Shah E, Trendel N, Kruger P, Nguyen J, Pettmann J, Kutuzov M, et al. Human CD8+ T cells exhibit a shared antigen threshold for different effector responses. bioRxiv. (2020) doi: 10.1101/2020.04.24.059766
45. Starr TK, Jameson SC, Hogquist KA. Positive and negative selection of T cells. Annu Rev Immunol. (2003) 21:139–76. doi: 10.1146/annurev.immunol.21.120601.141107
46. Curtsinger JM, Lins DC, Mescher MF. CD8+ memory T cells (CD44high, Ly-6C+) are more sensitive than naive Cells (CD44low, Ly-6C–) to TCR/CD8 signaling in response to antigen. J Immunol. (1998) 160:3236–43.
47. Veiga-Fernandes H, Walter U, Bourgeois C, McLean A, Rocha B. Response of naïve and memory CD8 + T cells to antigen stimulation in vivo. Nat Immunol. (2000) 1:47–53. doi: 10.1038/76907
48. Albert LJ, Inman RD. Molecular mimicry and autoimmunity. N Engl J Med. (1999) 341:2068–74. doi: 10.1056/NEJM199912303412707
49. Oldstone MBA. Molecular mimicry and immune-mediated diseases. FASEB J. (1998) 12:1255–65. doi: 10.1096/fasebj.12.13.1255
50. Pender MP, Csurhes PA, Burrows JM, Burrows SR. Defective T-cell control of Epstein–Barr virus infection in multiple sclerosis. Clin Transl Immunol. (2017) 6:e126. doi: 10.1038/cti.2016.87
51. Wim Ang C, Jacobs BC, Laman JD. The Guillain–Barré syndrome: a true case of molecular mimicry. Trends Immunol. (2004) 25:61–66. doi: 10.1016/j.it.2003.12.004
52. Wucherpfennig KW, Strominger JL. Molecular mimicry in T cell-mediated autoimmunity: viral peptides activate human T cell clones specific for myelin basic protein. Cell. (1995) 80:695–705. doi: 10.1016/0092-8674(95)90348-8
53. Zhao Z-S, Granucci F, Yeh L, Schaffer PA, Cantor H. Molecular mimicry by herpes simplex virus-type 1: autoimmune disease after viral infection. Science. (1998) 279:1344–7. doi: 10.1126/science.279.5355.1344
54. Johnson LA, Morgan RA, Dudley ME, Cassard L, Yang JC, Hughes MS, et al. Gene therapy with human and mouse T-cell receptors mediates cancer regression and targets normal tissues expressing cognate antigen. Blood. (2009) 114:535–46. doi: 10.1182/blood-2009-03-211714
55. Raman MCC, Rizkallah PJ, Simmons R, Donnellan Z, Dukes J, Bossi G, et al. Direct molecular mimicry enables off-target cardiovascular toxicity by an enhanced affinity TCR designed for cancer immunotherapy. Sci Rep. (2016) 6:18851. doi: 10.1038/srep18851
56. Bentzen AK, Hadrup SR. T-cell-receptor cross-recognition and strategies to select safe T-cell receptors for clinical translation. Immuno Oncol Technol. (2019) 2:1–10. doi: 10.1016/j.iotech.2019.06.003
57. Frankild S, Boer RJ, de Lund O, Nielsen M, Kesmir C. Amino acid similarity accounts for T cell cross-reactivity and for “Holes” in the T cell repertoire. PLoS ONE. (2008) 3:e1831. doi: 10.1371/journal.pone.0001831
58. Ishizuka J, Grebe K, Shenderov E, Peters B, Chen Q, Peng Y, et al. Quantitating T cell cross-reactivity for unrelated peptide antigens. J Immunol. (2009) 183:4337–45. doi: 10.4049/jimmunol.0901607
59. Nino-Vasquez JJ, Allicotti G, Borras E, Wilson DB, Valmori D, Simon R, et al. A powerful combination: the use of positional scanning libraries and biometrical analysis to identify cross-reactive T cell epitopes. Mol Immunol. (2004) 40:1063–74. doi: 10.1016/j.molimm.2003.11.005
60. Rubio-Godoy V, Dutoit V, Zhao Y, Simon R, Guillaume P, Houghten R, et al. Positional scanning-synthetic peptide library-based analysis of self- and pathogen-derived peptide cross-reactivity with tumor-reactive melan-a-specific CTL. J Immunol. (2002) 169:5696–707. doi: 10.4049/jimmunol.169.10.5696
61. Harris DT, Wang N, Riley TP, Anderson SD, Singh NK, Procko E, et al. Deep mutational scans as a guide to engineering high affinity T cell receptor interactions with peptide-bound major histocompatibility complex. J Biol Chem. (2016) 291:24566–78. doi: 10.1074/jbc.M116.748681
62. Pan R-Y, Chu M-T, Wang C-W, Lee Y-S, Lemonnier F, Michels AW, et al. Identification of drug-specific public TCR driving severe cutaneous adverse reactions. Nat Commun. (2019) 10:3569. doi: 10.1038/s41467-019-11396-2
63. Stadinski BD, Shekhar K, Gómez-Touriño I, Jung J, Sasaki K, Sewell AK, et al. Hydrophobic CDR3 residues promote the development of self-reactive T cells. Nat Immunol. (2016) 17:946–55. doi: 10.1038/ni.3491
64. Border EC, Sanderson JP, Weissensteiner T, Gerry AB, Pumphrey NJ. Affinity-enhanced T-cell receptors for adoptive T-cell therapy targeting MAGE-A10: strategy for selection of an optimal candidate. OncoImmunology. (2019) 8:e1532759. doi: 10.1080/2162402X.2018.1532759
65. Sibener LV, Fernandes RA, Kolawole EM, Carbone CB, Liu F, McAffee D, et al. Isolation of a structural mechanism for uncoupling T cell receptor signaling from peptide-MHC binding. Cell. (2018) 174:672–87.e27. doi: 10.1016/j.cell.2018.06.017
66. Adams JJ, Narayanan S, Liu B, Birnbaum ME, Kruse AC, Bowerman NA, et al. T Cell receptor signaling is limited by docking geometry to peptide-major histocompatibility complex. Immunity. (2011) 35:681–93. doi: 10.1016/j.immuni.2011.09.013
67. Riley TP, Hellman LM, Gee MH, Mendoza JL, Alonso JA, Foley KC, et al. T cell receptor cross-reactivity expanded by dramatic peptide–MHC adaptability. Nat Chem Biol. (2018) 14:934–42. doi: 10.1038/s41589-018-0130-4
68. Kasprowicz V, Ward SM, Turner A, Grammatikos A, Nolan BE, Lewis-Ximenez L, et al. Defining the Directionality and Quality of Influenza Virus–Specific CD8+ T Cell Cross-Reactivity in Individuals Infected with Hepatitis C Virus. (2008). Available online at: https://www.jci.org/articles/view/33082/pdf.
69. Cornberg M, Wedemeyer H. Hepatitis C virus infection from the perspective of heterologous immunity. Curr Opin Virol. (2016) 16:41–8. doi: 10.1016/j.coviro.2016.01.005
70. Gil A, Kenney LL, Mishra R, Watkin LB, Aslan N, Selin LK. Vaccination and heterologous immunity: educating the immune system. Trans R Soc Trop Med Hyg. (2015) 109:62–9. doi: 10.1093/trstmh/tru198
71. Xu J, Jo J. Broad cross-reactivity of the T-cell repertoire achieves specific and sufficiently rapid target searching. J Theor Biol. (2019) 466:119–27. doi: 10.1016/j.jtbi.2019.01.025
72. Fernandes RA, Ganzinger KA, Tzou JC, Jönsson P, Lee SF, Palayret M, et al. A cell topography-based mechanism for ligand discrimination by the T cell receptor. Proc Natl Acad Sci USA. (2019) 116:14002–10. doi: 10.1073/pnas.1817255116
73. Dushek O, van der Merwe PA. An induced rebinding model of antigen discrimination. Trends Immunol. (2014) 35:153–8. doi: 10.1016/j.it.2014.02.002
74. Arstila TP, Casrouge A, Baron V, Even J, Kanellopoulos J, Kourilsky P. A direct estimate of the human αβ T cell receptor diversity. Science. (1999) 286:958–61. doi: 10.1126/science.286.5441.958
75. Robins HS, Campregher PV, Srivastava SK, Wacher A, Turtle CJ, Kahsai O, et al. Comprehensive assessment of T-cell receptor β-chain diversity in αβ T cells. Blood. (2009) 114:4099–107. doi: 10.1182/blood-2009-04-217604
76. Chen W, Yewdell JW, Levine RL, Bennink JR. Modification of cysteine residues in vitro and in vivo affects the immunogenicity and antigenicity of major histocompatibility complex class I–restricted viral determinants. J Exp Med. (1999) 189:1757–64. doi: 10.1084/jem.189.11.1757
77. Green RS, Stone EL, Tenno M, Lehtonen E, Farquhar MG, Marth JD. Mammalian N-glycan branching protects against innate immune self-recognition and inflammation in autoimmune disease pathogenesis. Immunity. (2007) 27:308–20. doi: 10.1016/j.immuni.2007.06.008
78. Hill JA, Bell DA, Brintnell W, Yue D, Wehrli B, Jevnikar AM, et al. Arthritis induced by posttranslationally modified (citrullinated) fibrinogen in DR4-IE transgenic mice. J Exp Med. (2008) 205:967–79. doi: 10.1084/jem.20072051
79. Meadows L, Wang W, den Haan JMM, Blokland E, Reinhardus C, Drijfhout JW, et al. The HLA-A*0201-restricted H-Y antigen contains a posttranslationally modified cysteine that significantly affects T cell recognition. Immunity. (1997) 6:273–81. doi: 10.1016/S1074-7613(00)80330-1
80. Mohammed F, Cobbold M, Zarling AL, Salim M, Barrett-Wilt GA, Shabanowitz J, et al. Phosphorylation-dependent interaction between antigenic peptides and MHC class I: a molecular basis for the presentation of transformed self. Nat Immunol. (2008) 9:1236–43. doi: 10.1038/ni.1660
81. Petersen J, Wurzbacher SJ, Williamson NA, Ramarathinam SH, Reid HH, Nair AKN, et al. Phosphorylated self-peptides alter human leukocyte antigen class I-restricted antigen presentation and generate tumor-specific epitopes. Proc Natl Acad Sci USA. (2009) 106:2776–81. doi: 10.1073/pnas.0812901106
82. Ramarathinam SH, Gras S, Alcantara S, Yeung AWS, Mifsud NA, Sonza S, et al. Identification of native and posttranslationally modified HLA-B*57:01-restricted HIV envelope derived epitopes using immunoproteomics. Proteomics. (2018) 18:1700253. doi: 10.1002/pmic.201700253
83. Gotch F, McMichael A, Rothbard J. Recognition of influenza A matrix protein by HLA-A2-restricted cytotoxic T lymphocytes. Use of analogues to orientate the matrix peptide in the HLA-A2 binding site. J Exp Med. (1988) 168:2045–57. doi: 10.1084/jem.168.6.2045
84. Hu N, D'Souza C, Cheung H, Lang H, Cheuk E, Chamberlain JW. Highly conserved pattern of recognition of influenza A wild-type and variant CD8+ CTL epitopes in HLA-A2+ humans and transgenic HLA-A2+/H2 class I-deficient mice. Vaccine. (2005) 23:5231–44. doi: 10.1016/j.vaccine.2005.07.032
85. Petrova GV, Naumova EN, Gorski J. The polyclonal CD8 T cell response to influenza M158–66 generates a fully connected network of cross-reactive clonotypes to structurally related peptides: a paradigm for memory repertoire coverage of novel epitopes or escape mutants. J Immunol. (2011) 186:6390–7. doi: 10.4049/jimmunol.1004031
86. Rosa CL, Krishnan R, Markel S, Schneck JP, Houghten R, Pinilla C, et al. Enhanced immune activity of cytotoxic T-lymphocyte epitope analogs derived from positional scanning synthetic combinatorial libraries. Blood. (2001) 97:1776–86. doi: 10.1182/blood.V97.6.1776
87. Wooldridge L, Laugel B, Ekeruche J, Clement M, Berg HA, van den Price DA, et al. CD8 controls T cell cross-reactivity. J Immunol. (2010) 185:4625–32. doi: 10.4049/jimmunol.1001480
88. Hemmer B, Gran B, Zhao Y, Marques A, Pascal J, Tzou A, et al. Identification of candidate T-cell epitopes and molecular mimics in chronic Lyme disease. Nat Med. (1999) 5:1375–82. doi: 10.1038/70946
89. Jaravine V, Mösch A, Raffegerst S, Schendel DJ, Frishman D. Expitope 2.0: a tool to assess immunotherapeutic antigens for their potential cross-reactivity against naturally expressed proteins in human tissues. BMC Cancer. (2017) 17:892. doi: 10.1186/s12885-017-3854-8
90. Karapetyan AR, Chaipan C, Winkelbach K, Wimberger S, Jeong JS, Joshi B, et al. TCR Fingerprinting and off-target peptide identification. Front Immunol. (2019) 10:2501. doi: 10.3389/fimmu.2019.02501
91. Oseroff C, Kos F, Bui H-H, Peters B, Pasquetto V, Glenn J, et al. HLA class I-restricted responses to vaccinia recognize a broad array of proteins mainly involved in virulence and viral gene regulation. Proc Natl Acad Sci USA. (2005) 102:13980–5. doi: 10.1073/pnas.0506768102
92. Sylwester AW, Mitchell BL, Edgar JB, Taormina C, Pelte C, Ruchti F, et al. Broadly targeted human cytomegalovirus-specific CD4+ and CD8+ T cells dominate the memory compartments of exposed subjects. J Exp Med. (2005) 202:673–85. doi: 10.1084/jem.20050882
93. Jurtz VI, Jessen LE, Bentzen AK, Jespersen MC, Mahajan S, Vita R, et al. NetTCR: sequence-based prediction of TCR binding to peptide-MHC complexes using convolutional neural networks. bioRxiv. (2018) 433706. doi: 10.1101/433706
94. Smith CC, Chai S, Washington AR, Lee SJ, Landoni E, Field K, et al. Machine-learning prediction of tumor antigen immunogenicity in the selection of therapeutic epitopes. Cancer Immunol Res. (2019) 7:1591–604. doi: 10.1158/2326-6066.CIR-19-0155
95. Tung C-W, Ziehm M, Kämper A, Kohlbacher O, Ho S-Y. POPISK: T-cell reactivity prediction using support vector machines and string kernels. BMC Bioinformatics. (2011) 12:446. doi: 10.1186/1471-2105-12-446
96. Ogishi M, Yotsuyanagi H. Quantitative prediction of the landscape of T cell epitope immunogenicity in sequence space. Front Immunol. (2019) 10:827. doi: 10.3389/fimmu.2019.00827
97. Pogorelyy MV, Fedorova AD, McLaren JE, Ladell K, Bagaev DV, Eliseev AV, et al. Exploring the pre-immune landscape of antigen-specific T cells. Genome Med. (2018) 10:68. doi: 10.1186/s13073-018-0577-7
98. Calis JJA, Maybeno M, Greenbaum JA, Weiskopf D, Silva ADD, Sette A, et al. Properties of MHC class I presented peptides that enhance immunogenicity. PLoS Comput Biol. (2013) 9:e1003266. doi: 10.1371/journal.pcbi.1003266
99. Assarsson E, Sidney J, Oseroff C, Pasquetto V, Bui H-H, Frahm N, et al. A quantitative analysis of the variables affecting the repertoire of T cell specificities recognized after vaccinia virus infection. J Immunol. (2007) 178:7890–901. doi: 10.4049/jimmunol.178.12.7890
100. Kotturi MF, Botten J, Sidney J, Bui H-H, Giancola L, Maybeno M, et al. A Multivalent and cross-protective vaccine strategy against arenaviruses associated with human disease. PLoS Pathog. (2009) 5:e1000695. doi: 10.1371/journal.ppat.1000695
101. Trolle T, Nielsen M. NetTepi: an integrated method for the prediction of T cell epitopes. Immunogenetics. (2014) 66:449–56. doi: 10.1007/s00251-014-0779-0
102. Karosiene E, Lundegaard C, Lund O, Nielsen M. NetMHCcons: a consensus method for the major histocompatibility complex class I predictions. Immunogenetics. (2012) 64:177–86. doi: 10.1007/s00251-011-0579-8
103. Jørgensen KW, Rasmussen M, Buus S, Nielsen M. NetMHCstab – predicting stability of peptide–MHC-I complexes; impacts for cytotoxic T lymphocyte epitope discovery. Immunology. (2014) 141:18–26. doi: 10.1111/imm.12160
104. Chowell D, Krishna S, Becker PD, Cocita C, Shu J, Tan X, et al. TCR contact residue hydrophobicity is a hallmark of immunogenic CD8+ T cell epitopes. Proc Natl Acad Sci USA. (2015) 112:E1754–62. doi: 10.1073/pnas.1500973112
105. Łuksza M, Riaz N, Makarov V, Balachandran VP, Hellmann MD, Solovyov A, et al. A neoantigen fitness model predicts tumour response to checkpoint blockade immunotherapy. Nature. (2017) 551:517–20. doi: 10.1038/nature24473
106. Bjerregaard A-M, Nielsen M, Jurtz V, Barra CM, Hadrup SR, Szallasi Z, et al. An analysis of natural T cell responses to predicted tumor neoepitopes. Front Immunol. (2017) 8:1566. doi: 10.3389/fimmu.2017.01566
107. Shen W-J, Wong H-S, Xiao Q-W, Guo X, Smale S. Towards a mathematical foundation of immunology and amino acid chains. arXiv [Preprint]. ArXiv:12056031 Cs Q-Bio Stat (2012). Available online at: https://arxiv.org/abs/1205.6031
108. Klinger M, Pepin F, Wilkins J, Asbury T, Wittkop T, Zheng J, et al. Multiplex identification of antigen-specific T cell receptors using a combination of immune assays and immune receptor sequencing. PLoS ONE. (2015) 10:e0141561. doi: 10.1371/journal.pone.0141561
109. Riley TP, Keller GLJ, Smith AR, Davancaze LM, Arbuiso AG, Devlin JR, et al. Structure based prediction of neoantigen immunogenicity. Front Immunol. (2019) 10:2047. doi: 10.3389/fimmu.2019.02047
110. Kawashima S, Kanehisa M. AAindex: amino acid index database. Nucleic Acids Res. (2000) 28:374. doi: 10.1093/nar/28.1.374
111. Haase K, Raffegerst S, Schendel DJ, Frishman D. Expitope: a web server for epitope expression. Bioinformatics. (2015) 31:1854–6. doi: 10.1093/bioinformatics/btv068
112. Adams JJ, Narayanan S, Birnbaum ME, Sidhu SS, Blevins SJ, Gee MH, et al. Structural interplay between germline interactions and adaptive recognition determines the bandwidth of TCR-peptide-MHC cross-reactivity. Nat Immunol. (2016) 17:87–94. doi: 10.1038/ni.3310
113. Riley TP, Baker BM. The intersection of affinity and specificity in the development and optimization of T cell receptor based therapeutics. Semin Cell Dev Biol. (2018) 84:30–41. doi: 10.1016/j.semcdb.2017.10.017
114. Ekeruche-Makinde J, Miles JJ, van den Berg HA, Skowera A, Cole DK, Dolton G, et al. Peptide length determines the outcome of TCR/peptide-MHCI engagement. Blood. (2013) 121:1112–23. doi: 10.1182/blood-2012-06-437202
115. Song I, Gil A, Mishra R, Ghersi D, Selin LK, Stern LJ. Broad TCR repertoire and diverse structural solutions for recognition of an immunodominant CD8 + T cell epitope. Nat Struct Mol Biol. (2017) 24:395–406. doi: 10.1038/nsmb.3383
116. Cuendet MA, Zoete V, Michielin O. How T cell receptors interact with peptide-MHCs: a multiple steered molecular dynamics study. Proteins Struct Funct Bioinform. (2011) 79:3007–24. doi: 10.1002/prot.23104
117. Spear TT, Evavold BD, Baker BM, Nishimura MI. Understanding TCR affinity, antigen specificity, and cross-reactivity to improve TCR gene-modified T cells for cancer immunotherapy. Cancer Immunol Immunother. (2019) 68:1881–9. doi: 10.1007/s00262-019-02401-0
118. Stone JD, Chervin AS, Kranz DM. T-cell receptor binding affinities and kinetics: impact on T-cell activity and specificity. Immunology. (2009) 126:165–76. doi: 10.1111/j.1365-2567.2008.03015.x
119. Krogsgaard M, Prado N, Adams EJ, He X, Chow D-C, Wilson DB, et al. Evidence that structural rearrangements and/or flexibility during TCR binding can contribute to T cell activation. Mol Cell. (2003) 12:1367–78. doi: 10.1016/S1097-2765(03)00474-X
120. Degano M, Garcia KC, Apostolopoulos V, Rudolph MG, Teyton L, Wilson IA. A functional hot spot for antigen recognition in a superagonist TCR/MHC complex. Immunity. (2000) 12:251–61. doi: 10.1016/S1074-7613(00)80178-8
121. Kalergis AM, Nathenson SG. Altered peptide ligand-mediated TCR antagonism can be modulated by a change in a single amino acid residue within the CDR3β of an MHC class I-restricted TCR. J Immunol. (2000) 165:280–85. doi: 10.4049/jimmunol.165.1.280
122. Liu B, Chen W, Evavold BD, Zhu C. Accumulation of dynamic catch bonds between TCR and agonist peptide-MHC triggers T cell SIGNALING. Cell. (2014) 157:357–68. doi: 10.1016/j.cell.2014.02.053
123. Lyons GE, Moore T, Brasic N, Li M, Roszkowski JJ, Nishimura MI. Influence of human CD8 on antigen recognition by T-cell receptor–transduced cells. Cancer Res. (2006) 66:11455–61. doi: 10.1158/0008-5472.CAN-06-2379
124. Moore TV, Lyons GE, Brasic N, Roszkowski JJ, Voelkl S, Mackensen A, et al. Relationship between CD8-dependent antigen recognition, T cell functional avidity, and tumor cell recognition. Cancer Immunol Immunother. (2008) 58:719–28. doi: 10.1007/s00262-008-0594-2
125. Pryshchep S, Zarnitsyna VI, Hong J, Evavold BD, Zhu C. Accumulation of serial forces on TCR and CD8 frequently applied by agonist antigenic peptides embedded in MHC molecules triggers calcium in T cells. J Immunol. (2014) 193:68–76. doi: 10.4049/jimmunol.1303436
126. Spear TT, Nagato K, Nishimura MI. Strategies to genetically engineer T cells for cancer immunotherapy. Cancer Immunol Immunother. (2016) 65:631–49. doi: 10.1007/s00262-016-1842-5
127. Spear TT, Wang Y, Foley KC, Murray DC, Scurti GM, Simms PE, et al. Critical biological parameters modulate affinity as a determinant of function in T-cell receptor gene-modified T-cells. Cancer Immunol Immunother. (2017) 66:1411–24. doi: 10.1007/s00262-017-2032-9
128. Spear TT, Wang Y, Smith TW, Simms PE, Garrett-Mayer E, Hellman LM, et al. Altered peptide ligands impact the diversity of polyfunctional phenotypes in T cell receptor gene-modified T cells. Mol Ther. (2018) 26:996–1007. doi: 10.1016/j.ymthe.2018.01.015
129. Thomson CT, Kalergis AM, Sacchettini JC, Nathenson SG. A structural difference limited to one residue of the antigenic peptide can profoundly alter the biological outcome of the TCR-peptide/MHC class I interaction. J Immunol. (2001) 166:3994–7. doi: 10.4049/jimmunol.166.6.3994
130. Tsuchiya Y, Namiuchi Y, Wako H, Tsurui H. A study of CDR3 loop dynamics reveals distinct mechanisms of peptide recognition by T-cell receptors exhibiting different levels of cross-reactivity. Immunology. (2018) 153:466–78. doi: 10.1111/imm.12849
131. Valitutti S, Müller S, Cella M, Padovan E, Lanzavecchia A. Serial triggering of many T-cell receptors by a few peptide–MHC complexes. Nature. (1995) 375:148–51. doi: 10.1038/375148a0
132. Aleksic M, Dushek O, Zhang H, Shenderov E, Chen J-L, Cerundolo V, et al. Dependence of T cell antigen recognition on T cell receptor-peptide MHC confinement time. Immunity. (2010) 32:163–74. doi: 10.1016/j.immuni.2009.11.013
133. Leaver-Fay A, O'Meara MJ, Tyka M, Jacak R, Song Y, Kellogg EH, et al. Chapter six - scientific benchmarks for guiding macromolecular energy function improvement. In: Keating AE, editor. Methods in Enzymology, Vol. 523. Oxford: Academic Press (2013). p. 109–43.
134. Riley TP, Ayres CM, Hellman LM, Singh NK, Cosiano M, Cimons JM, et al. A generalized framework for computational design and mutational scanning of T-cell receptor binding interfaces. Protein Eng Des Sel. (2016) 29:595–606. doi: 10.1093/protein/gzw050
135. Haidar JN, Pierce B, Yu Y, Tong W, Li M, Weng Z. Structure-based design of a T cell receptor leads to nearly 100-Fold improvement in binding affinity for pepMHC. Proteins. (2009) 74:948–60. doi: 10.1002/prot.22203
136. Pierce BG, Hellman LM, Hossain M, Singh NK, Kooi CWV, Weng Z, et al. Computational design of the affinity and specificity of a therapeutic T cell receptor. PLoS Comput Biol. (2014) 10:e1003478. doi: 10.1371/journal.pcbi.1003478
137. Berman HM, Westbrook J, Feng Z, Gilliland G, Bhat TN, Weissig H, et al. The protein data bank. Nucleic Acids Res. (2000) 28:235–42. doi: 10.1093/nar/28.1.235
138. Leem J, de Oliveira SHP, Krawczyk K, Deane CM. STCRDab: the structural T-cell receptor database. Nucleic Acids Res. (2018) 46:D406–12. doi: 10.1093/nar/gkx971
139. Gowthaman R, Pierce BG. TCR3d: the T cell receptor structural repertoire database. Bioinformatics. (2019) 35:5323–5. doi: 10.1093/bioinformatics/btz517
140. Desmet J, Wilson IA, Joniau M, De Maeyer M, Lasters I. Computation of the binding of fully flexible peptides to proteins with flexible side chains. FASEB J. (1997) 11:164–72. doi: 10.1096/fasebj.11.2.9039959
141. Leach AR. Ligand docking to proteins with discrete side-chain flexibility. J Mol Biol. (1994) 235:345–56. doi: 10.1016/S0022-2836(05)80038-5
142. Bui H-H, Schiewe AJ, Grafenstein H. von and haworth is. Structural prediction of peptides binding to MHC class I molecules. Proteins Struct Funct Bioinform. (2006) 63:43–52. doi: 10.1002/prot.20870
143. Fagerberg T, Cerottini J-C, Michielin O. Structural prediction of peptides bound to MHC class I. J Mol Biol. (2006) 356:521–46. doi: 10.1016/j.jmb.2005.11.059
144. Monje-Galvan V, Warburton L, Klauda JB. Setting up all-atom molecular dynamics simulations to study the interactions of peripheral membrane proteins with model lipid bilayers. In: Drin G, editor. Intracellular Lipid Transport: Methods and Protocols. New York, NY: Springer (2019). p. 325–39.
145. Perilla JR, Hadden JA, Goh BC, Mayne CG, Schulten K. All-atom molecular dynamics of virus capsids as drug targets. J Phys Chem Lett. (2016) 7:1836–44. doi: 10.1021/acs.jpclett.6b00517
146. Park M-S, Park SY, Miller KR, Collins EJ, Lee HY. Accurate structure prediction of peptide–MHC complexes for identifying highly immunogenic antigens. Mol Immunol. (2013) 56:81–90. doi: 10.1016/j.molimm.2013.04.011
147. Kish-Catalone TM, Lu W, Gallo RC, DeVico AL. Preclinical evaluation of synthetic −2 RANTES as a candidate vaginal microbicide to target CCR5. Antimicrob Agents Chemother. (2006) 50:1497–509. doi: 10.1128/AAC.50.4.1497-1509.2006
148. Knapp B, Alcala M, Zhang H, West CE, van der Merwe PA, Deane CM. pyHVis3D: visualising molecular simulation deduced H-bond networks in 3D: application to T-cell receptor interactions. Bioinformatics. (2018) 34:1941–3. doi: 10.1093/bioinformatics/btx842
149. Zhang H, Lim H-S, Knapp B, Deane CM, Aleksic M, Dushek O, et al. The contribution of major histocompatibility complex contacts to the affinity and kinetics of T cell receptor binding. Sci Rep. (2016) 6:35326. doi: 10.1038/srep35326
150. Wan S, Knapp B, Wright DW, Deane CM, Coveney PV. Rapid, precise, and reproducible prediction of peptide–MHC binding affinities from molecular dynamics that correlate well with experiment. J Chem Theory Comput. (2015) 11:3346–56. doi: 10.1021/acs.jctc.5b00179
151. Pierce BG, Weng Z. A flexible docking approach for prediction of T cell receptor–peptide–MHC complexes. Protein Sci. (2013) 22:35–46. doi: 10.1002/pro.2181
152. Mendes MFA, Antunes DA, Rigo MM, Sinigaglia M, Vieira GF. Improved structural method for T-cell cross-reactivity prediction. Mol Immunol. (2015) 67:303–10. doi: 10.1016/j.molimm.2015.06.017
153. Birnbaum ME, Mendoza JL, Sethi DK, Dong S, Glanville J, Dobbins J, et al. Deconstructing the peptide-MHC specificity of T cell recognition. Cell. (2014) 157:1073–87. doi: 10.1016/j.cell.2014.03.047
154. Borbulevych OY, Piepenbrink KH, Gloor BE, Scott DR, Sommese RF, Cole DK, et al. T cell receptor cross-reactivity directed by antigen-dependent tuning of peptide-MHC molecular flexibility. Immunity. (2009) 31:885–96. doi: 10.1016/j.immuni.2009.11.003
155. Borbulevych OY, Piepenbrink KH, Baker BM. Conformational melding permits a conserved binding geometry in TCR recognition of foreign and self molecular mimics. J Immunol. (2011) 186:2950–58. doi: 10.4049/jimmunol.1003150
156. Cole DK, Bulek AM, Dolton G, Schauenberg AJ, Szomolay B, Rittase W, et al. Hotspot autoimmune T cell receptor binding underlies pathogen and insulin peptide cross-reactivity. J Clin Invest. (2016) 126:2191–204. doi: 10.1172/JCI85679
157. Garboczi DN, Ghosh P, Utz U, Fan QR, Biddison WE, Wiley DC. Structure of the complex between human T-cell receptor, viral peptide and HLA-A2. Nature. (1996) 384:134–41. doi: 10.1038/384134a0
158. Hausmann S, Biddison WE, Smith KJ, Ding Y-H, Garboczi DN, Utz U, et al. Peptide recognition by two HLA-A2/Tax11–19-specific T cell clones in relationship to their MHC/Peptide/TCR crystal structures. J Immunol. (1999) 162:5389–97.
159. Shen ZT, Nguyen TT, Daniels KA, Welsh RM, Stern LJ. Disparate epitopes mediating protective heterologous immunity to unrelated viruses share peptide–MHC structural features recognized by cross-reactive T cells. J Immunol. (2013) 191:5139–52. doi: 10.4049/jimmunol.1300852
160. Yin Y, Li Y, Mariuzza RA. Structural basis for self-recognition by autoimmune T-cell receptors. Immunol Rev. (2012) 250:32–48. doi: 10.1111/imr.12002
161. Clute SC, Watkin LB, Cornberg M, Naumov YN, Sullivan JL, Luzuriaga K, et al. Cross-reactive influenza virus–specific CD8+ T cells contribute to lymphoproliferation in Epstein-Barr virus–associated infectious mononucleosis. J Clin Invest. (2005) 115:3602–12. doi: 10.1172/JCI25078
162. Kennedy PTF, Urbani S, Moses RA, Amadei B, Fisicaro P, Lloyd J, et al. The influence of T cell cross-reactivity on HCV-peptide specific human T cell response. Hepatology. (2006) 43:602–11. doi: 10.1002/hep.21081
163. Capietto A-H, Jhunjhunwala S, Pollock SB, Lupardus P, Wong J, Hänsch L, et al. Mutation position is an important determinant for predicting cancer neoantigens. J Exp Med. (2020) 217:e20190179. doi: 10.1084/jem.20190179
164. Springer I, Besser H, Tickotsky-Moskovitz N, Dvorkin S, Louzoun Y. Prediction of specific TCR-peptide binding from large dictionaries of TCR-peptide pairs. arXiv [Preprint]. bioRxiv:650861. (2020) doi: 10.1101/650861
165. Cameron BJ, Gerry AB, Dukes J, Harper JV, Kannan V, Bianchi FC, et al. Identification of a titin-derived HLA-A1–presented peptide as a cross-reactive target for engineered MAGE A3–directed T cells. Sci Transl Med. (2013) 5:197ra103. doi: 10.1126/scitranslmed.3006034
166. Morgan R, Chinnasamy N, Abate-Daga D, Gros A, Robbins P, Zheng Z, et al. Cancer regression and neurological toxicity following anti-MAGE-A3 TCR gene therapy. J Immunother. (2013) 36:133–51. doi: 10.1097/CJI.0b013e3182829903
167. Gaston JS, Rickinson AB, Epstein MA. Cross-reactivity of self-HLA-restricted Epstein-Barr virus-specific cytotoxic T lymphocytes for allo-HLA determinants. J Exp Med. (1983) 158:1804–21. doi: 10.1084/jem.158.6.1804
168. Amir AL, D'Orsogna LJA, Roelen DL, van Loenen MM, Hagedoorn RS, de Boer R, et al. Allo-HLA reactivity of virus-specific memory T cells is common. Blood. (2010) 115:3146–57. doi: 10.1182/blood-2009-07-234906
169. van der Zwan A, van der Meer-Prins EMW, van Miert PPMC, van den Heuvel H, Anholts JDH, Roelen DL, et al. Cross-reactivity of virus-specific CD8+ T cells against allogeneic HLA-C: possible implications for pregnancy outcome. Front Immunol. (2018) 9:2880. doi: 10.3389/fimmu.2018.02880
170. Ziegler A, Müller CA, Böckmann RA, Uchanska-Ziegler B. Low-affinity peptides and T-cell selection. Trends Immunol. (2009) 30:53–60. doi: 10.1016/j.it.2008.11.004
171. van der Merwe PA, Dushek O. Mechanisms for T cell receptor triggering. Nat Rev Immunol. (2011) 11:47–55. doi: 10.1038/nri2887
172. Martinez RJ, Evavold BD. Lower affinity T cells are critical components and active participants of the immune response. Front Immunol. (2015) 6:468. doi: 10.3389/fimmu.2015.00468
173. Martinez RJ, Andargachew R, Martinez HA, Evavold BD. Low-affinity CD4+ T cells are major responders in the primary immune response. Nat Commun. (2016) 7:13848. doi: 10.1038/ncomms13848
174. Gee MH, Sibener LV, Birnbaum ME, Jude KM, Yang X, Fernandes RA, et al. Stress-testing the relationship between T cell receptor/peptide-MHC affinity and cross-reactivity using peptide velcro. Proc Natl Acad Sci USA. (2018) 115:E7369–78. doi: 10.1073/pnas.1802746115
175. Rudolph MG, Stanfield RL, Wilson IA. How tCRs bind MHCs, peptides, and coreceptors. Annu Rev Immunol. (2006) 24:419–66. doi: 10.1146/annurev.immunol.23.021704.115658
176. Friedensohn S, Khan TA, Reddy ST. Advanced methodologies in high-throughput sequencing of immune repertoires. Trends Biotechnol. (2017) 35:203–14. doi: 10.1016/j.tibtech.2016.09.010
177. Heather JM, Ismail M, Oakes T, Chain B. High-throughput sequencing of the T-cell receptor repertoire: pitfalls and opportunities. Brief Bioinform. (2018) 19:554–65. doi: 10.1093/bib/bbw138
178. Miho E, Yermanos A, Weber CR, Berger CT, Reddy ST, Greiff V. Computational strategies for dissecting the high-dimensional complexity of adaptive immune repertoires. Front Immunol. (2018) 9:224. doi: 10.3389/fimmu.2018.00224
179. Shugay M, Bagaev DV, Turchaninova MA, Bolotin DA, Britanova OV, Putintseva EV, et al. VDJtools: unifying post-analysis of T cell receptor repertoires. PLoS Comput Biol. (2015) 11:e1004503. doi: 10.1371/journal.pcbi.1004503
180. Bagaev DV, Vroomans RMA, Samir J, Stervbo U, Rius C, Dolton G, et al. VDJdb in 2019: database extension, new analysis infrastructure and a T-cell receptor motif compendium. Nucleic Acids Res. (2020) 48:D1057–62. doi: 10.1093/nar/gkz874
181. Tickotsky N, Sagiv T, Prilusky J, Shifrut E, Friedman N. McPAS-TCR: a manually curated catalogue of pathology-associated T cell receptor sequences. Bioinformatics. (2017) 33:2924–9. doi: 10.1093/bioinformatics/btx286
182. DeWitt WS III, Smith A, Schoch G, Hansen JA, Matsen FA, et al. Human T cell receptor occurrence patterns encode immune history, genetic background, and receptor specificity. Elife. (2018) 7:e38358. doi: 10.7554/eLife.38358.043
183. Thomas N, Best K, Cinelli M, Reich-Zeliger S, Gal H, Shifrut E, et al. Tracking global changes induced in the CD4 T-cell receptor repertoire by immunization with a complex antigen using short stretches of CDR3 protein sequence. Bioinformatics. (2014) 30:3181–8. doi: 10.1093/bioinformatics/btu523
184. Cinelli M, Sun Best K, Heather JM, Reich-Zeliger S, Shifrut E, Friedman N, et al. Feature selection using a one dimensional naïve Bayes' classifier increases the accuracy of support vector machine classification of CDR3 repertoires. Bioinformatics. (2017) 33:951–5. doi: 10.1093/bioinformatics/btw771
185. Priel A, Gordin M, Philip H, Zilberberg A, Efroni S. Network representation of T-cell repertoire— a novel tool to analyze immune response to cancer formation. Front Immunol. (2018) 9:2913. doi: 10.3389/fimmu.2018.02913
186. Meysman P, De Neuter N, Gielis S, Bui Thi D, Ogunjimi B, Laukens K. On the viability of unsupervised T-cell receptor sequence clustering for epitope preference. Bioinformatics. (2019) 35:1461–8. doi: 10.1093/bioinformatics/bty821
187. Thakkar N, Bailey-Kellogg C. Balancing sensitivity and specificity in distinguishing TCR groups by CDR sequence similarity. BMC Bioinformatics. (2019) 20:241. doi: 10.1186/s12859-019-2864-8
188. Gordin M, Philip H, Zilberberg A, Gidoni MC, Margalit R, Clouser C, et al. Mice developing mammary tumors evolve T cell sequences shared with human breast cancer patients. arXiv [Preprint]. bioRxiv:371260. (2018) doi: 10.1101/371260
189. Edgar RC. Search and clustering orders of magnitude faster than BLAST. Bioinformatics. (2010) 26:2460–1. doi: 10.1093/bioinformatics/btq461
190. Emerson RO, DeWitt WS, Vignali M, Gravley J, Hu JK, Osborne EJ, et al. Immunosequencing identifies signatures of cytomegalovirus exposure history and HLA-mediated effects on the T cell repertoire. Nat Genet. (2017) 49:659–65. doi: 10.1038/ng.3822
191. Ester M, Kriegel H-P, Sander J, Xu X. A density-based algorithm for discovering clusters in large spatial databases with noise. In: Proceedings of the Second International Conference on Knowledge Discovery and Data Mining. Palo Alto, CA: AAAI Press (1996). p. 226–31.
192. Pogorelyy MV, Minervina AA, Shugay M, Chudakov DM, Lebedev YB, Mora T, et al. Detecting T cell receptors involved in immune responses from single repertoire snapshots. PLoS Biol. (2019) 17:e3000314. doi: 10.1371/journal.pbio.3000314
193. Buuren MM, van Dijkgraaf FE, Linnemann C, Toebes M, Chang CXL, Mok JY, et al. HLA micropolymorphisms strongly affect peptide–MHC multimer–based monitoring of antigen-specific CD8+ T cell responses. J Immunol. (2014) 192:641–8. doi: 10.4049/jimmunol.1301770
194. Cole DK, Miles KM, Madura F, Holland CJ, Schauenburg AJA, Godkin AJ, et al. T-cell receptor (TCR)-peptide specificity overrides affinity-enhancing TCR-major histocompatibility complex interactions. J Biol Chem. (2014) 289:628–38. doi: 10.1074/jbc.M113.522110
195. Feng D, Bond CJ, Ely LK, Maynard J, Garcia KC. Structural evidence for a germline-encoded T cell receptor–major histocompatibility complex interaction ‘codon'. Nat Immunol. (2007) 8:975–83. doi: 10.1038/ni1502
196. Petrova G, Ferrante A, Gorski J. Cross-reactivity of T cells and its role in the immune system. Crit Rev Immunol. (2012) 32:349–72. doi: 10.1615/CritRevImmunol.v32.i4.50
197. Gate D, Saligrama N, Leventhal O, Yang AC, Unger MS, Middeldorp J, et al. Clonally expanded CD8 T cells patrol the cerebrospinal fluid in Alzheimer's disease. Nature. (2020) 577:399–404. doi: 10.1038/s41586-019-1895-7
198. Jiang W, Birtley JR, Hung S-C, Wang W, Chiou S-H, Macaubas C, et al. In vivo clonal expansion and phenotypes of hypocretin-specific CD4 + T cells in narcolepsy patients and controls. Nat Commun. (2019) 10:5247. doi: 10.1038/s41467-019-13234-x
199. Yost KE, Satpathy AT, Wells DK, Qi Y, Wang C, Kageyama R, et al. Clonal replacement of tumor-specific T cells following PD-1 blockade. Nat Med. (2019) 25:1251–9. doi: 10.1038/s41591-019-0522-3
200. Lanzarotti E, Marcatili P, Nielsen M. T-cell receptor cognate target prediction based on paired α and β chain sequence and structural CDR loop similarities. Front Immunol. (2019) 10:2080. doi: 10.3389/fimmu.2019.02080
201. Ostmeyer J, Christley S, Toby IT, Cowell LG. Biophysicochemical motifs in T-cell receptor sequences distinguish repertoires from tumor-infiltrating lymphocyte and adjacent healthy tissue. Cancer Res. (2019) 79:1671–80. doi: 10.1158/0008-5472.CAN-18-2292
202. Kidera A, Konishi Y, Oka M, Ooi T, Scheraga HA. Statistical analysis of the physical properties of the 20 naturally occurring amino acids. J Protein Chem. (1985) 4:23–55. doi: 10.1007/BF01025492
203. Atchley WR, Zhao J, Fernandes AD, Drüke T. Solving the protein sequence metric problem. Proc Natl Acad Sci USA. (2005) 102:6395–400. doi: 10.1073/pnas.0408677102
204. De Simone M, Rossetti G, Pagani M. Single cell T cell receptor sequencing: techniques and future challenges. Front Immunol. (2018) 9:1638. doi: 10.3389/fimmu.2018.01638
205. Carter JA, Preall JB, Grigaityte K, Goldfless SJ, Jeffery E, Briggs AW, et al. Single T cell sequencing demonstrates the functional role of αβ TCR pairing in cell lineage and antigen specificity. Front Immunol. (2019) 10:1516. doi: 10.3389/fimmu.2019.01516
206. Stubbington MJT, Lönnberg T, Proserpio V, Clare S, Speak AO, Dougan G, et al. T cell fate and clonality inference from single-cell transcriptomes. Nat Methods. (2016) 13:329–32. doi: 10.1038/nmeth.3800
207. Izraelson M, Nakonechnaya TO, Moltedo B, Egorov ES, Kasatskaya SA, Putintseva EV, et al. Comparative analysis of murine T-cell receptor repertoires. Immunology. (2018) 153:133–44. doi: 10.1111/imm.12857
208. Kamga L, Gil A, Song I, Brody R, Ghersi D, Aslan N, et al. CDR3α drives selection of the immunodominant Epstein Barr Virus (EBV) BRLF1-specific CD8 T cell receptor repertoire in primary infection. PLoS Pathog. (2019) 15:e1008122. doi: 10.1371/journal.ppat.1008122
209. Bentzen AK, Marquard AM, Lyngaa R, Saini SK, Ramskov S, Donia M, et al. Large-scale detection of antigen-specific T cells using peptide-MHC-I multimers labeled with DNA barcodes. Nat Biotechnol. (2016) 34:1037–45. doi: 10.1038/nbt.3662
210. Rufer N. Molecular tracking of antigen-specific T-cell clones during immune responses. Curr Opin Immunol. (2005) 17:441–7. doi: 10.1016/j.coi.2005.06.003
211. Hinrichs CS, Restifo NP. Reassessing target antigens for adoptive T-cell therapy. Nat Biotechnol. (2013) 31:999–1008. doi: 10.1038/nbt.2725
212. Zvyagin IV, Pogorelyy MV, Ivanova ME, Komech EA, Shugay M, Bolotin DA, et al. Distinctive properties of identical twins' TCR repertoires revealed by high-throughput sequencing. Proc Natl Acad Sci USA. (2014) 111:5980–5. doi: 10.1073/pnas.1319389111
213. Bradley P, Thomas PG. Using T cell receptor repertoires to understand the principles of adaptive immune recognition. Annu Rev Immunol. (2019) 37:547–70. doi: 10.1146/annurev-immunol-042718-041757
214. Gross DM, Forsthuber T, Tary-Lehmann M, Etling C, Ito K, Nagy ZA, et al. Identification of LFA-1 as a candidate autoantigen in treatment-resistant lyme arthritis. Science. (1998) 281:703–6. doi: 10.1126/science.281.5377.703
215. Losikoff PT, Mishra S, Terry F, Gutierrez A, Ardito MT, Fast L, et al. HCV epitope, homologous to multiple human protein sequences, induces a regulatory T cell response in infected patients. J Hepatol. (2015) 62:48–55. doi: 10.1016/j.jhep.2014.08.026
216. Oseroff C, Sidney J, Vita R, Tripple V, McKinney DM, Southwood S, et al. T cell responses to known allergen proteins are differently polarized and account for a variable fraction of total response to allergen extracts. J Immunol. (2012) 189:1800–11. doi: 10.4049/jimmunol.1200850
217. Li B, Severson E, Pignon J-C, Zhao H, Li T, Novak J, et al. Comprehensive analyses of tumor immunity: implications for cancer immunotherapy. Genome Biol. (2016) 17:174. doi: 10.1186/s13059-016-1028-7
218. Ott PA, Hu Z, Keskin DB, Shukla SA, Sun J, Bozym DJ, et al. An immunogenic personal neoantigen vaccine for patients with melanoma. Nature. (2017) 547:217–21. doi: 10.1038/nature22991
219. Snyder A, Makarov V, Merghoub T, Yuan J, Zaretsky JM, Desrichard A, et al. Genetic basis for clinical response to CTLA-4 blockade in melanoma. N Engl J Med. (2014) 371:2189–99. doi: 10.1056/NEJMoa1406498
220. Hellman LM, Foley KC, Singh NK, Alonso JA, Riley TP, Devlin JR, et al. Improving T cell receptor on-target specificity via structure-guided design. Mol Ther. (2019) 27:300–13. doi: 10.1016/j.ymthe.2018.12.010
221. Malecek K, Grigoryan A, Zhong S, Gu WJ, Johnson LA, Rosenberg SA, et al. Specific increase in potency via structure-based design of a TCR. J Immunol. (2014) 193:2587–99. doi: 10.4049/jimmunol.1302344
222. Zoete V, Irving M, Ferber M, Cuendet M, Michielin O. Structure-based, rational design of T cell receptors. Front Immunol. (2013) 4:268. doi: 10.3389/fimmu.2013.00268
223. Zhao Y, Bennett AD, Zheng Z, Wang QJ, Robbins PF, Yu LYL, et al. High-affinity TCRs generated by phage display provide CD4+ T cells with the ability to recognize and kill tumor cell lines. J Immunol. (2007) 179:5845–54. doi: 10.4049/jimmunol.179.9.5845
Keywords: T cell cross reactivity, T cell specificity, epitope, antigen presentation, adaptive immune system, antigen specificity
Citation: Lee CH, Salio M, Napolitani G, Ogg G, Simmons A and Koohy H (2020) Predicting Cross-Reactivity and Antigen Specificity of T Cell Receptors. Front. Immunol. 11:565096. doi: 10.3389/fimmu.2020.565096
Received: 23 May 2020; Accepted: 07 September 2020;
Published: 22 October 2020.
Edited by:
Thomas Herrmann, Julius Maximilian University of Würzburg, GermanyReviewed by:
Brian M. Baker, University of Notre Dame, United StatesDavid A. Scheinberg, Memorial Sloan Kettering Cancer Center, United States
Copyright © 2020 Lee, Salio, Napolitani, Ogg, Simmons and Koohy. This is an open-access article distributed under the terms of the Creative Commons Attribution License (CC BY). The use, distribution or reproduction in other forums is permitted, provided the original author(s) and the copyright owner(s) are credited and that the original publication in this journal is cited, in accordance with accepted academic practice. No use, distribution or reproduction is permitted which does not comply with these terms.
*Correspondence: Hashem Koohy, aGFzaGVtLmtvb2h5QHJkbS5veC5hYy51aw==
In Memoriam: This paper is dedicated to the memory of Prof. Vincenzo Cerundolo.