- 1Department of Computational Medicine and Bioinformatics, University of Michigan, Ann Arbor, MI, United States
- 2Unit for Laboratory Animal Medicine, Department of Microbiology and Immunology, University of Michigan, Ann Arbor, MI, United States
To ultimately combat the emerging COVID-19 pandemic, it is desired to develop an effective and safe vaccine against this highly contagious disease caused by the SARS-CoV-2 coronavirus. Our literature and clinical trial survey showed that the whole virus, as well as the spike (S) protein, nucleocapsid (N) protein, and membrane (M) protein, have been tested for vaccine development against SARS and MERS. However, these vaccine candidates might lack the induction of complete protection and have safety concerns. We then applied the Vaxign and the newly developed machine learning-based Vaxign-ML reverse vaccinology tools to predict COVID-19 vaccine candidates. Our Vaxign analysis found that the SARS-CoV-2 N protein sequence is conserved with SARS-CoV and MERS-CoV but not from the other four human coronaviruses causing mild symptoms. By investigating the entire proteome of SARS-CoV-2, six proteins, including the S protein and five non-structural proteins (nsp3, 3CL-pro, and nsp8-10), were predicted to be adhesins, which are crucial to the viral adhering and host invasion. The S, nsp3, and nsp8 proteins were also predicted by Vaxign-ML to induce high protective antigenicity. Besides the commonly used S protein, the nsp3 protein has not been tested in any coronavirus vaccine studies and was selected for further investigation. The nsp3 was found to be more conserved among SARS-CoV-2, SARS-CoV, and MERS-CoV than among 15 coronaviruses infecting human and other animals. The protein was also predicted to contain promiscuous MHC-I and MHC-II T-cell epitopes, and the predicted linear B-cell epitopes were found to be localized on the surface of the protein. Our predicted vaccine targets have the potential for effective and safe COVID-19 vaccine development. We also propose that an “Sp/Nsp cocktail vaccine” containing a structural protein(s) (Sp) and a non-structural protein(s) (Nsp) would stimulate effective complementary immune responses.
Introduction
The emerging Coronavirus Disease 2019 (COVID-19) pandemic poses a massive crisis to global public health. As of March 11, 2020, there were 118,326 confirmed cases and 4,292 deaths, according to the World Health Organization (WHO), and WHO declared the COVID-19 as a pandemic on the same day. On May 12, WHO reported 4,088,848 confirmed COVID-19 cases and 283,153 deaths globally, showing a dramatic increase in terms of case and death numbers. The causative agent of the COVID-19 disease is the severe acute respiratory syndrome coronavirus 2 (SARS-CoV-2). Coronaviruses can cause animal diseases such as avian infectious bronchitis caused by the infectious bronchitis virus (IBV), and pig transmissible gastroenteritis caused by a porcine coronavirus (1). Bats are commonly regarded as the natural reservoir of coronaviruses, which can be transmitted to humans and other animals after genetic mutations. There are seven known human coronaviruses, including the novel SARS-CoV-2. Four of them (HCoV-HKU1, HCoV-OC43, HCoV-229E, and HCoV-NL63) have been circulating in the human population worldwide and cause mild symptoms (2). Coronavirus became prominent after Severe Acute Respiratory Syndrome (SARS) and Middle East Respiratory Syndrome (MERS) outbreaks. In 2003, the SARS disease caused by the SARS-associated coronavirus (SARS-CoV) infected over 8,000 people worldwide and was contained in the summer of 2003 (3). SARS-CoV-2 and SARS-CoV share high sequence identity (4). The MERS disease infected more than 2,000 people, which is caused by the MERS-associated coronavirus (MERS-CoV) and was first reported in Saudi Arabia and spread to several other countries since 2012 (5).
Great efforts have been made to develop and manufacture COVID-19 vaccines, and these efforts in pushing the vaccine clinical trials are phenomenal (Table 1). Coronaviruses are positively-stranded RNA viruses with its genome packed inside the nucleocapsid (N) protein and enveloped by the membrane (M) protein, envelope (E) protein, and the spike (S) protein (6). While many coronavirus vaccine studies targeting different structural proteins were conducted, most of these efforts eventually ceased soon after the outbreak of SARS and MERS. With the recent COVID-19 pandemic outbreak, it is urgent to resume the coronavirus vaccine research. As the immediate response to the on-going pandemic, the first testing in humans of the mRNA-based vaccine targeting the S protein of SARS-CoV-2 (ClinicalTrials.gov Identifier: NCT04283461, Table 1) started on March 16, 2020. As the most superficial and protrusive protein of the coronaviruses, S protein plays a crucial role in mediating virus entry. In the SARS and MERS vaccine development, the full-length S protein and its S1 subunit (which contains receptor binding domain) have been frequently used as the vaccine antigens due to their ability to induce neutralizing antibodies that prevent host cell entry and infection.
However, the current coronavirus vaccines, including S protein-based vaccines, might have issues in the lack of inducing complete protection and possible safety concerns (7, 8). Most existing SARS/MERS vaccines were reported to induce neutralizing antibodies and partial protection against the viral challenges in animal models (Table 2). A recent study reported that adenovirus vaccine vector encoding full-length MERS-CoV S protein (ChAdOx1 MERS) showed protection upon MERS-CoV challenge in rhesus macaques (9). Nonetheless, it is desired for a COVID-19 vaccine to induce complete protection or sterile immunity. Moreover, it has become increasingly clear that multiple immune responses, including those induced by humoral or cell-mediated immunity, are responsible for correlates of protection than antibody titers alone (10). Both killed SARS-CoV whole virus vaccine and adenovirus-based recombinant vector vaccines expressing S or N proteins induced neutralizing antibody responses but did not provide complete protection in animal model (11). A study has shown increased liver pathology in the vaccinated ferrets immunized with modified vaccinia Ankara-S recombinant vaccine (12). The safety and efficacy of these vaccination strategies have not been fully tested in human clinical trials, but safety could be a major concern. Therefore, novel strategies are needed to enhance the efficacy and safety of COVID-19 vaccine development.
In recent years, the development of vaccine design has been revolutionized by the reverse vaccinology (RV), which aims to first identify promising vaccine candidate through bioinformatics analysis of the pathogen genome. RV has been successfully applied to vaccine discovery for pathogens such as Group B meningococcus and led to the license Bexsero vaccine (13). Among current RV prediction tools (14, 15), Vaxign is the first web-based RV program (16) and has been used to predict vaccine candidates against different bacterial and viral pathogens (17–19). Recently we have also developed a machine learning approach called Vaxign-ML to enhance prediction accuracy (20).
In this study, we first surveyed the existing coronavirus vaccine development status, and then applied the Vaxign and Vaxign-ML RV approaches to predict COVID-19 protein candidates for vaccine development. We identified six possible adhesins, including the structural S protein and five other non-structural proteins, and three of them (S, nsp3, and nsp8 proteins) were predicted to induce high protective immunity. The S protein was predicted to have the highest protective antigenicity score, and it has been extensively studied as the target of coronavirus vaccines by other researchers. The sequence conservation and immunogenicity of the multi-domain nsp3 protein, which was predicted to have the second-highest protective antigenicity score yet, was further analyzed in this study. Based on the predicted structural S protein and non-structural proteins (including nsp3) using reverse vaccinology and machine learning, we proposed and discussed a cocktail vaccine strategy for rational COVID-19 vaccine development.
Results
Published Research and Clinical Trial Coronavirus Vaccine Studies
To better understand the current status of coronavirus vaccine development, we systematically surveyed the development of vaccines for coronavirus from the ClinicalTrials.gov database and PubMed literature. There were only three SARS-CoV and six MERS-CoV vaccine clinical trials (Table 1), and extensive effort has been made to develop COVID-19 vaccines in response to the current pandemic. Seven representative vaccine clinical trials were presented in Table 1, including inactivated whole virus vaccine and S protein-derived vaccine. Well-established vaccines targeting pathogens other than SARS-CoV-2 are also under investigation, such as measles (NCT04357028) and BCG (NCT04327206), which may induce strong immune responses and provide non-specific protective effects against SARS-CoV-2 infection (21).
There are two primary design strategies for coronavirus vaccine development: the usage of the whole virus or genetically engineered vaccine antigens that can be delivered through different formats. The whole virus vaccines include inactivated (22) or live-attenuated vaccines (23, 24) (Table 2). The two live attenuated SARS vaccines mutated the exoribonuclease and envelop protein to reduce the virulence and/or replication capability of the SARS-CoV. Recent works also showed promising development of three types of SARS-CoV-2 vaccines, including inactivated whole virus vaccine (25), RNA vaccine (26), and virus-like particles (VLP) vaccine (27) (Table 2). Overall, the whole virus vaccines can induce a strong immune response and protect against coronavirus infections. Genetically engineered vaccines that target specific coronavirus proteins are often used to improve vaccine safety and efficacy. The coronavirus antigens such as S protein, N protein, and M protein can be delivered as recombinant DNA vaccine and viral vector vaccine (Table 2).
From experimentally identified immune responses induced by coronavirus vaccines, we found evidence of the protective roles of both antibody and cell-mediated immunity (28, 29). The protective role of the neutralizing antibody to coronavirus S protein has been demonstrated by the experimental result that a passive transfer of the serum from mice immunized with MVA/S to naïve mice reduced the replication of challenged SARS-CoV in the respiratory tract (28). Here the MVA/S is the highly attenuated modified vaccinia virus Ankara (MVA) containing the gene encoding full-length SARS-CoV S protein. The antibodies developed in the mice immunized with MVA/S could also bind to the S1 domain of S and neutralize SARS-CoV in vitro. Passive transfer of anti-S neutralizing antibody also offered protection against SARS-CoV (30). However, antibody responses in patients previously infected with respiratory viruses, including SARS-CoV and MERS-CoV, tend to be short-lived (31). Instead, T cell responses are often long-lived by targeting conserved proteins and showed to have a significant correlation in protective immunity against influenza virus infection (32). SARS-CoV-specific memory T cells but not antibody-producing B cells could be detected in patients 6 years after SARS-CoV infection (33). A further study showed that respiratory tract memory CD4+ T cells specific for an epitope the nucleocapsid (N) protein of SARS-CoV provided protection against virulent challenge with SARS-CoV and MERS-CoV (29). CD8+ T cells were also found to be crucial for the clearance of SARS-CoV and MERS-CoV infections (34, 35). Therefore, our vaccine prediction would target those viral antigens with the ability to induce protective neutralizing antibody and/or T cell responses.
SARS-CoV-2 N Protein Sequence Is Conserved With the N Protein From SARS-CoV and MERS-CoV
We first used the Vaxign analysis framework (16, 20) to compare the full proteomes of seven human coronavirus strains (SARS-CoV-2, SARS-CoV, MERS-CoV, HCoV-229E, HCoV-OC43, HCoV-NL63, and HCoV-HKU1). The proteins of SARS-CoV-2 were used as the seed for the pan-genomic comparative analysis. The Vaxign pan-genomic analysis reported only the N protein in SARS-CoV-2 having high sequence similarity among the more severe form of coronavirus (SARS-CoV and MERS-CoV), while having low sequence similarity among the more typically mild HCoV-229E, HCoV-OC43, HCoV-NL63, and HCoV-HKU1. The sequence conservation suggested the potential of N protein as a candidate for the cross-protective vaccine against SARS and MERS. The N protein was also evaluated and used for vaccine development (Table 2). As a protein inside the viral envelope, the N protein packs the coronavirus RNA to form the helical nucleocapsid in virion assembly. This protein is more conserved than the S protein and was reported to induce a humoral and cellular immune response against coronavirus infections (36). A conserved CD4+ T cell epitope in the SARS-CoV N was also found important for the induction of protection against the challenge of SARS-CoV or MERS-CoV (29). However, a study also showed the linkage between N protein and severe pneumonia or other serious liver failures, suggesting N protein-induced pathogenesis and possible adverse effects caused by N protein-derived vaccines (37).
Six Adhesive Proteins in SARS-CoV-2 Identified as Potential Vaccine Targets
The Vaxign RV analysis predicted six SARS-CoV-2 proteins (S protein, nsp3, 3CL-PRO, and nsp8-10) as adhesive proteins (Table 3). Adhesin plays a critical role in the virus adhering to the host cell and facilitating the virus entry to the host cell (38), which has a significant association with the vaccine-induced protection (39). In SARS-CoV-2, S protein was predicted to be adhesin, matching its primary role in virus entry. The structure of SARS-CoV-2 S protein was determined (40) and reported to contribute to the host cell entry by interacting with the angiotensin-converting enzyme 2 (ACE2) (41). Besides S protein, the other five predicted adhesive proteins were all non-structural proteins. In particular, nsp3 is the largest non-structural protein of SARS-CoV-2 comprises various functional domains (42).
Three Adhesin Proteins Were Predicted to Induce Strong Protective Immunity
The recently published Vaxign-ML pipeline was applied to compute the protegenicity (protective antigenicity) score and predict the induction of protective immunity by a vaccine candidate (20). Vaxign-ML predicts the protegenicity score using an optimized supervised machine learning model with manually annotated training data consisted of bacterial and viral protective antigens. These protective antigens were tested to be protective in at least one animal challenge model (43). The performance of the Vaxign-ML models was evaluated (Table S1 and Figure S1), and the best performing model had a weighted F1-score and Matthew's correlation coefficient of 0.94 and 0.66, respectively, in nested cross-validation. Using the optimized Vaxign-ML model, we predicted three proteins (S protein, nsp3, and nsp8) as vaccine candidates with significant protegenicity scores (Table 3). The S protein was predicted to have the highest protegenicity score, which is consistent with the experimental observations reported in the literature. The nsp3 protein is the second most promising vaccine candidate besides S protein. There was currently no study of nsp3 as a vaccine target. The structure and functions of this protein have various roles in coronavirus infection, including replication and pathogenesis (immune evasion and virus survival) (42). Therefore, we selected nsp3 for further investigation, as described below.
Nsp3 as a Vaccine Candidate
The multiple sequence alignment and the resulting phylogeny of nsp3 protein showed that this protein in SARS-CoV-2 was more closely related to the human coronaviruses SARS-CoV and MERS-CoV, and bat coronaviruses BtCoV/HKU3, BtCoV/HKU4, and BtCoV/HKU9. We studied the genetic conservation of nsp3 protein (Figure 1A) in seven human coronaviruses and eight coronaviruses infecting other animals (Table S2). The five human coronaviruses, SARS-CoV-2, SARS-CoV, MERS-CoV, HCoV-HKU1, and HCoV-OC43, belong to the beta-coronavirus while HCoV-229E and HCoV-NL63 belong to the alpha-coronavirus. The HCoV-HKU1 and HCoV-OC43, as the human coronavirus with mild symptoms clustered together with murine MHV-A59. The more severe form of human coronavirus SARS-CoV-2, SARS-CoV, and MERS-CoV grouped with three bat coronaviruses BtCoV/HKU3, BtCoV/HKU4, and BtCoV/HKU9.
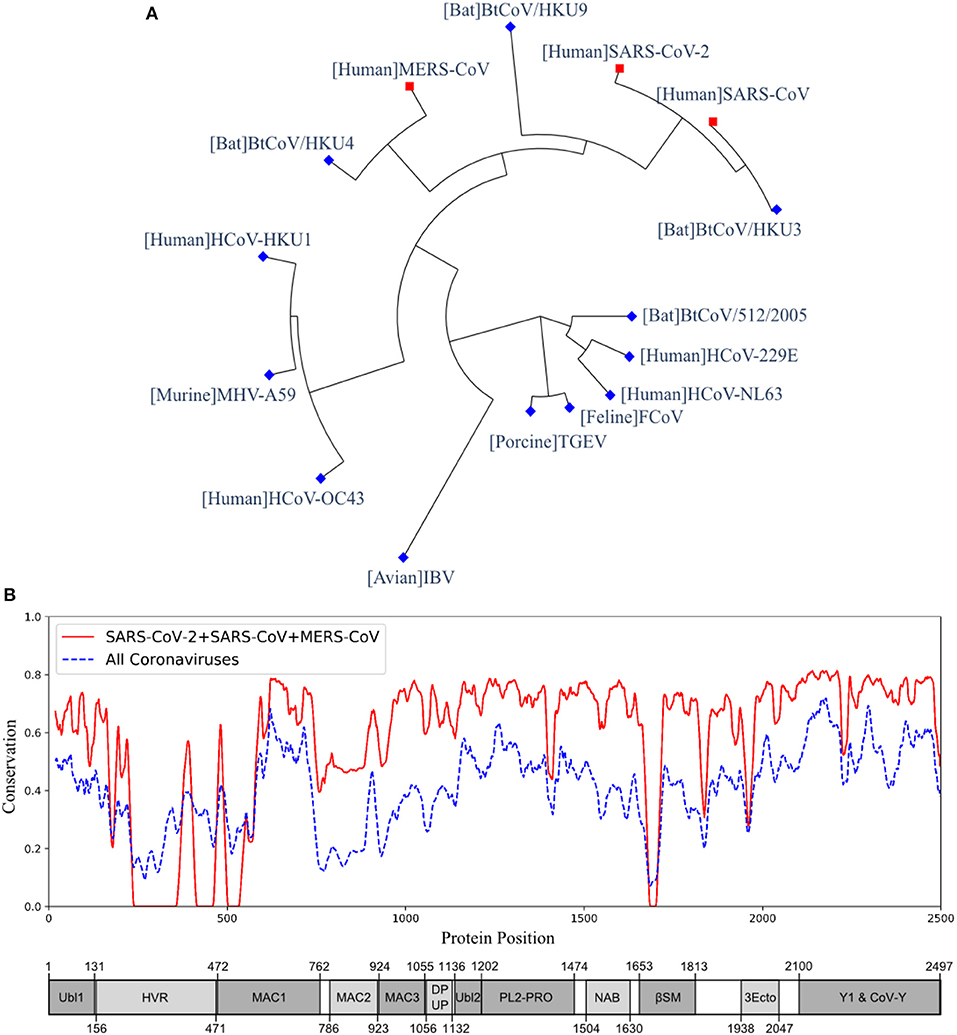
Figure 1. The phylogeny and sequence conservation of coronavirus nsp3. (A) Phylogeny of 15 strains based on the nsp3 protein sequence alignment and phylogeny analysis. (B) The conservation of nsp3 among different coronavirus strains. The red line represents the conservation among the four strains (SARS-CoV, SARS-CoV-2, MERS, and BtCoV-HKU3). The blue line was generated using all the 15 strains. The bottom part represents the nsp3 peptides and their sizes. The phylogenetically close four strains have more conserved nsp3 sequences than all the strains being considered.
When evaluating the amino acid conservations relative to the functional domains in nsp3, all protein domains, except the hypervariable region (HVR), macro-domain 1 (MAC1) and beta-coronavirus-specific marker βSM, showed higher conservation in SARS-CoV-2, SARS-CoV, and MERS-CoV (Figure 1B). The amino acid conservation between the major human coronavirus (SARS-CoV-2, SARS-CoV, and MERS-CoV) was plotted and compared to all 15 coronaviruses used to generate the phylogenetic of nsp3 protein (Figure 1B). The SARS-CoV domains were also plotted (Figure 1B), with the relative position in the multiple sequence alignment (MSA) of all 15 coronaviruses (Table S3 and Figure S2).
The immunogenicity of nsp3 protein in terms of T cell MHC-I & MHC-II and linear B cell epitopes was also investigated. There were 28 and 42 promiscuous epitopes predicted to bind the reference MHC-I & MHC-II alleles, which covered the majority of the world population, respectively (Tables S4, S5). In terms of linear B cell epitopes, there were 14 epitopes with BepiPred scores over 0.55 and had at least ten amino acids in length (Table 4). The 3D structure of SARS-CoV-2 protein was plotted and highlighted with the T cell MHC-I & MHC-II, and linear B cell epitopes (Figure 2). The predicted B cell epitopes were more likely located on the surface of the nsp3 protein. Most of the predicted MHC-I & MHC-II epitopes were embedded inside the protein. The sliding averages of T cell MHC-I & MHC-II and linear B cell epitopes were plotted with respect to the tentative SARS-CoV-2 nsp3 protein domains using SARS-CoV nsp3 protein as a reference (Figure 3). The ubiquitin-like domain 1 and 2 (Ubl1 and Ubl2) only predicted to have MHC-I epitopes. The Domain Preceding Ubl2 and PL2-PRO (DPUP) domain had only predicted MHC-II epitopes. The PL2-PRO contained both predicted MHC-I and MHC-II epitopes, but not B cell epitopes. In particular, the TM1, TM2, and AH1 were predicted helical regions with high T cell MHC-I and MHC-II epitopes (44). The TM1 and TM2 are transmembrane regions passing the endoplasmic reticulum (ER) membrane. The HVR, MAC2, MAC3, nucleic-acid binding domain (NAB), βSM, Nsp3 ectodomain; (3Ecto), Y1, and CoV-Y domain contained predicted B cell epitopes. Finally, the Vaxign RV framework also predicted two regions (position 251-260 and 329-337) in the MAC1 domain of the nsp3 having high sequence similarity to the human mono-ADP-ribosyltransferase PARP14 (NP_060024.2).
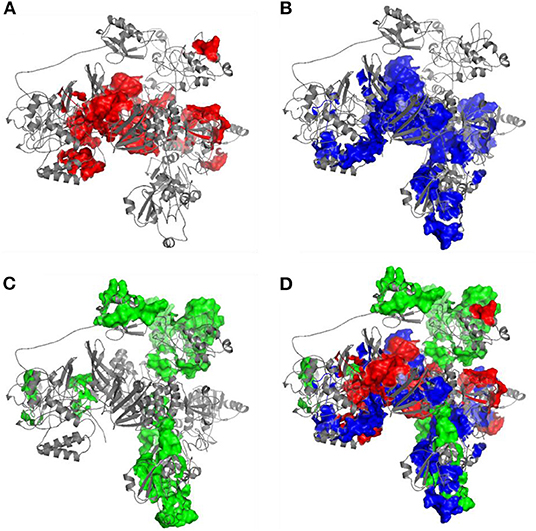
Figure 2. Predicted 3D structure of nsp3 protein highlighted with (A) MHC-I T cell epitopes (red), (B) MHC-II (blue) T cell epitopes, (C) linear B cell epitopes (green), and the (D) merged epitopes. The B cell epitopes are more exposed on the protein surface while the T cell MHC-I and MHC-II epitopes are more located within the protein.
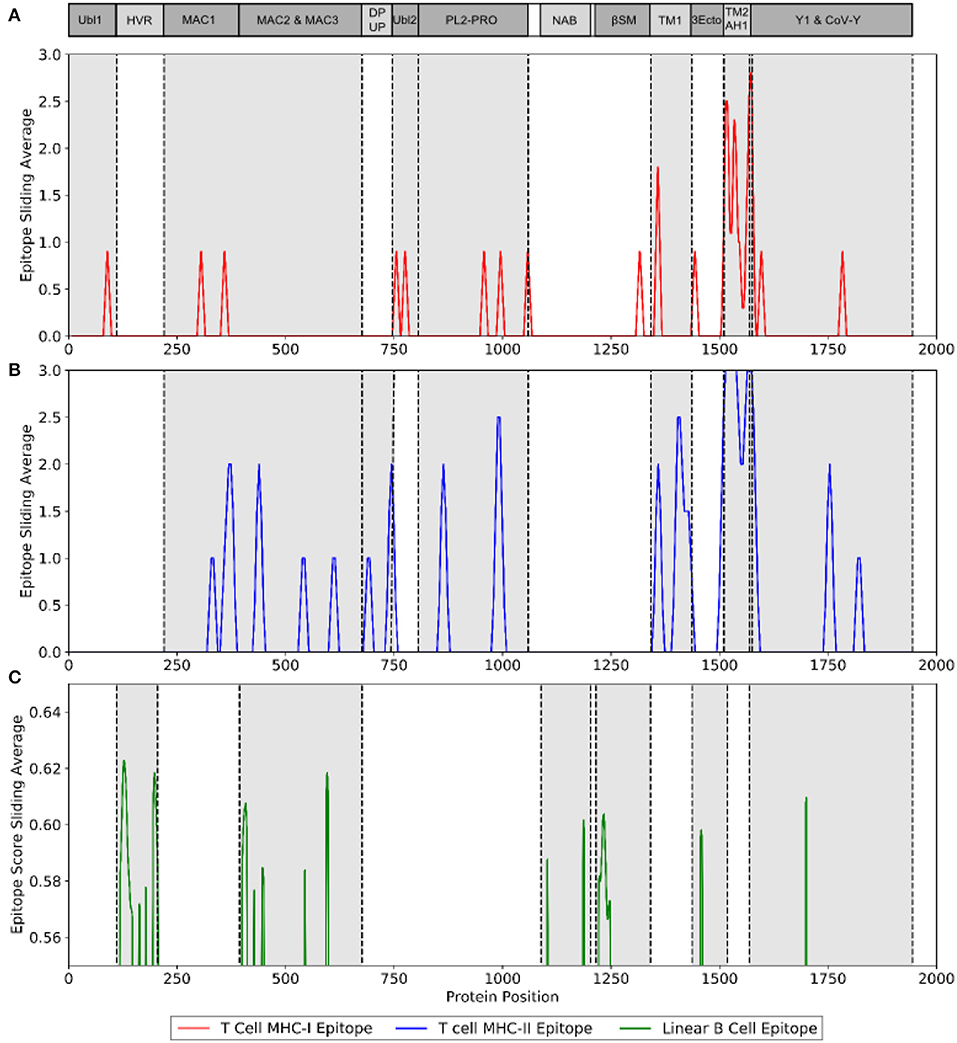
Figure 3. Immunogenic region of nsp3 between SARS-CoV-2 and the four conservation strains. (A) MHC-I (red) T cell epitope (B) MHC-II (blue) T cell epitope (C) linear B cell epitope (green).
Discussion
Our prediction of the potential SARS-CoV-2 antigens, which could induce protective immunity, provides a timely analysis for the vaccine development against COVID-19. Currently, most coronavirus vaccine studies use the whole inactivated or attenuated virus, or target the structural proteins such as the spike (S) protein, nucleocapsid (N) protein, and membrane (M) protein (Table 2). But the inactivated or attenuated whole virus vaccine might cause strong adverse events. On the other hand, vaccines targeting the structural proteins induce a robust immune response (36, 45, 46). In some studies, these structural proteins, including the S and N proteins, were reported to associate with the pathogenesis of coronavirus (37, 47) and might raise safety concern (12). Recently, the epitopes of the SARS-CoV-2 were computationally predicted and evaluated by sequence homology analysis of SARS-CoV and MERS-CoV epitopes (48). Following this study, the predicted T cell MHC-I and MHC-II epitopes of SARS-CoV-2 was experimentally evaluated using the “megapools” approach and both CD4+ and CD8+ responses were detected (49). The present work is complementary but not overlapping with the recent reports. Our study applied state-of-the-art Vaxign reserve vaccinology (RV) and Vaxign-ML machine learning strategies to the entire SARS-CoV-2 proteomes, including both structural and non-structural proteins for vaccine candidate prediction. Our results indicate, for the first time, that many non-structural proteins could be used as potential vaccine candidates.
The SARS-CoV-2 S protein was identified by our Vaxign and Vaxign-ML analysis as the most favorable vaccine candidate. First, the Vaxign RV framework predicted the S protein as a likely adhesin, which is consistent with the role of S protein for the invasion of host cells. Second, our Vaxign-ML predicted that the S protein had a high protective antigenicity score. These results confirmed the role of S protein as the important target of COVID-19 vaccines. However, targeting only the S protein may induce high serum-neutralizing antibody titers but cannot induce complete protection (11). In addition, HCoV-NL63 also uses S protein and employs the angiotensin-converting enzyme 2 (ACE2) for cellular entry, despite markedly weak pathogenicity (50). This suggests that the S protein is not the only factor determining the infection level of a human coronavirus. Thus, alternative vaccine antigens may be considered as potential targets for COVID-19 vaccines.
Among the five non-structural proteins being predicted as potential vaccine candidates, the nsp3 protein was predicted to have second-highest protective antigenicity score, adhesin property, promiscuous MHC-I & MHC-II T cell epitopes, and B cell epitopes. The nsp3 is the largest non-structural protein that includes multiple functional domains related to viral pathogenesis (42). The multiple sequence alignment of nsp3 also showed higher sequence conservation in most of the functional domains in SARS-CoV-2, SARS-CoV, and MERS-CoV, than in all 15 coronavirus strains (Figure 1B). Besides the nsp3 protein, our study also predicted four additional non-structural proteins (3CL-pro, nsp8, nsp9, and nsp10) as possible vaccine candidates based on their adhesin probabilities, and the nsp8 protein was also predicted to have a significant protective antigenicity score.
However, these predicted non-structural proteins (nsp3, 3CL-pro, nsp8, nsp9, and nsp10) are not part of the viral structural particle, and all the current SARS/MERS/COVID-19 vaccine studies target the structural (S/M/N) proteins. Although structural proteins are commonly used as viral vaccine candidates, non-structural proteins correlate to vaccine protection. The non-structural protein NS1 was found to induce protective immunity against infections by flaviviruses (51). Since NS1 is not part of the virion, antibodies against NS1 have no neutralizing activity but some exhibit complement-fixing activity (52). However, passive transfer of anti-NS1 antibody or immunization with NS1 conferred protection (53). The anti-NS1 antibody could also reduce viral replication by complement-dependent cytotoxicity of infected cells, block NS1-induced pathogenic effects, and attenuate NS1-induced disease development during the critical phase (54). Finally, NS1 is not a structural protein and the anti-NS1 antibody will not induce antibody-dependent enhancement (ADE), which is a virulence factor and a risk factor causing many adverse events (54). In addition to the induction of antibody responses, non-structural proteins of viruses could induce virus-specific T cells, especially cytotoxic T lymphocytes, that are important to control viral infection. The non-structural proteins of the hepatitis C virus were reported to induce HCV-specific vigorous and broad-spectrum T-cell responses (55). The non-structural HIV-1 gene products were also shown to be valuable targets for prophylactic or therapeutic vaccines (56). Therefore, it is reasonable to hypothesize that the SARS-CoV-2 non-structural proteins (e.g., nsp3) are possible vaccine targets, which might induce cell-mediated or humoral immunity necessary to prevent viral invasion and/or replication.
The SARS-CoV-2 nsp3 protein was recently reported to account for the virus-specific T cell response. Grifoni et al. showed that the three major structural (S/M/N) proteins accounted for 59% of the total CD4+ T cell response in COVID-19 recovered patients while other non-structural proteins, including nsp3, also accounted for the response (49). In addition, SARS-CoV-2-reactive CD4+ T cells could be detected in a large portion of unexposed individuals, suggesting cross-reactive T cell recognition between SARS-CoV-2 and the other coronaviruses that only cause common cold. In our study, the nsp3 protein showed sequence conservation among the 15 coronaviruses, and particularly, the protein shared higher similarity among the more severe form of coronavirus (SARS-CoV, MERS-CoV, and SARS-CoV-2) (Figure 2). The preexisting immunity against the mild human coronaviruses might offer cross-protection to the SARS-CoV-2 infected individuals (49). In spite of that, none of the non-structural proteins have been evaluated as vaccine candidates, and the feasibility of these proteins as vaccine targets are subject to further experimental verification.
Besides the immunogenicity, safety is also an important factor of a successful COVID-19 vaccine. One of the safety issues of COVID-19 vaccines might occur due to vaccine delivery (e.g., vectors, adjuvants, formulation doses, or route of administration), which cannot be evaluated by the machine learning approach presented in this study. In addition, the nsp3 and other viral adhesive proteins with sequence homology to the host cell adhesion molecules might also cause auto-reactivity with self-antigen or induce T regulatory, leading to low responsiveness of the host to the virus. By applying Vaxign and epitope predictions, our study found that the MAC1 domain of nsp3 protein share sequence homology with the human mono-ADP-ribosyltransferase PARP14, and there is no predicted T cell MHC-I, MHC-II, and linear B cell epitopes within the aligned region.
In addition to vaccines expressing a single or a combination of structural proteins, here we propose an “Sp/Nsp cocktail vaccine” as an effective strategy for COVID-19 vaccine development. A typical cocktail vaccine includes more than one antigen to cover different aspects of protection (57, 58). The licensed Group B meningococcus Bexsero vaccine, which was developed via reverse vaccinology, contains three protein antigens (13). To develop an efficient and safe COVID-19 cocktail vaccine, an “Sp/Nsp cocktail vaccine,” which mixes a structural protein(s) (Sp, such as S protein) and a non-structural protein(s) (Nsp, such as nsp3) could induce more favorable protective immune responses than vaccines expressing a structural protein(s). Current COVID-19 vaccines mostly target on the S protein with various types of delivery systems (such as recombinant virus vectors) (Table 1), and none of the non-structural proteins has not been used. The benefit of a cocktail vaccine strategy could induce immunity that can protect the host against not only the S-ACE2 interaction and viral entry to the host cells, but also protect against the accessary non-structural adhesin proteins (e.g., nsp3), which might also be vital to the viral entry and replication. The usage of more than one antigen allows us to reduce the volume of each antigen and thus to reduce the induction of adverse events. Nonetheless, the potential and safety of the proposed “Sp/Nsp cocktail vaccine” strategy need to be experimentally validated.
For rational COVID-19 vaccine development, it is critical to understand the fundamental host-coronavirus interaction and protective immune mechanism (7). Such understanding may not only provide us guidance in terms of antigen selection but also facilitate our design of vaccine formulations. For example, an important foundation of our prediction in this study is based on our understanding of the critical role of adhesin as a virulence factor as well as protective antigen. The choice of DNA vaccine, recombinant vaccine vector, and another method of vaccine formulation is also deeply rooted in our understanding of pathogen-specific immune response induction. Different experimental conditions may also affect results (59, 60). Therefore, it is crucial to understand the underlying molecular and cellular mechanisms for rational vaccine development.
Methods
Annotation of Literature and Database Records
We annotated peer-reviewed journal articles stored in the PubMed database and the ClinicalTrials.gov database. From the peer-reviewed articles, we identified and annotated those coronavirus vaccine candidates that were experimentally studied and found to induce protective neutralizing antibody or provided immunity against virulent pathogen challenge.
Vaxign and Vaxign-ML Reverse Vaccinology Prediction
The SARS-CoV-2 sequence was obtained from NCBI. All the proteins of six known human coronavirus strains, including SARS-CoV, MERS-CoV, HCoV-229E, HCoV-OC43, HCoV-NL63, and HCoV-HKU1 were extracted from Uniprot proteomes (61). The full proteomes of these seven coronaviruses were then analyzed using the Vaxign reverse vaccinology pipeline (16, 20). The Vaxign program predicted serval biological features, including adhesin probability (62), transmembrane helix (63), orthologous proteins (64), protein functions (16), and Vaxign-ML protegenicity score (20).
The Vaxign-ML protegenicity score was calculated following a similar methodology described in the Vaxign-ML. In brief, the positive samples in the training data included 397 bacterial and 178 viral protective antigens (PAgs) recorded in the Protegen database (43) after removing homologous proteins with over 30% sequence identity. There were 4,979 negative samples extracted from the corresponding pathogens' Uniprot proteomes (61) with sequence dis-similarity to the PAgs, as described in previous studies (65–67). Homologous proteins in the negative samples were also removed. The proteins in the resulting dataset were annotated with biological and physicochemical features. The biological features included adhesin probability (62), transmembrane helix (63), and immunogenicity (68). The physicochemical features included the compositions, transitions, and distributions (69), quasi-sequence-order (70), Moreau-Broto auto-correlation (71, 72), and Geary auto-correlation (73) of various physicochemical properties such as charge, hydrophobicity, polarity, and solvent accessibility (74). Five supervised ML classification algorithms, including logistic regression, support vector machine, k-nearest neighbor, random forest (75), and extreme gradient boosting (XGB) (76) were trained on the annotated proteins dataset. The performance of these models was evaluated using a nested 5-fold cross-validation (N5CV) based on the area under receiver operating characteristic curve, precision, recall, weighted F1-score, and Matthew's correlation coefficient. The best performing XGB model was selected to predict the protegenicity score of all SARS-CoV-2 isolate Wuhan-Hu-1 (GenBank ID: MN908947.3) proteins, downloaded from NCBI. The protegenicity score is the percentile rank score from the Vaxign-ML classification model. A protein with higher protegenicity score is considered as stronger vaccine candidate with higher utility toward protection. In addition, using the protegenicity score of 0.9 as a threshold resulted in the highest prediction performance with weighted F1-score = 0.94 in N5CV.
Phylogenetic Analysis
The protein nsp3 was selected for further investigation. The nsp3 proteins of 14 coronaviruses besides SARS-CoV-2 were downloaded from the Uniprot (Table S2). Multiple sequence alignment of these nsp3 proteins was performed using MUSCLE (77) and visualized via SEAVIEW (78). The phylogenetic tree was constructed using PhyML (79), and the amino acid conservation was estimated by the Jensen-Shannon Divergence (JSD) (80). The JSD score was also used to generate a sequence conservation line using the nsp3 protein sequences from 4 or 13 coronaviruses.
Immunogenicity Analysis
The immunogenicity of the nsp3 protein was evaluated by the prediction of T cell MHC-I and MHC-II, and linear B cell epitopes. For T cell MHC-I epitopes, the IEDB consensus method was used to predicting promiscuous epitopes binding to 4 out of 27 MHC-I reference alleles with consensus percentile ranking <1.0 score (68). For T cell MHC-II epitopes, the IEDB consensus method was used to predicting promiscuous epitopes binding to more than half of the 27 MHC-II reference alleles with consensus percentile ranking <10.0. The MHC-I and MHC-II reference alleles covered a wide range of human genetic variation representing the majority of the world population (81, 82). The linear B cell epitopes were predicted using the BepiPred 2.0 with a cutoff of 0.55 score (83). Linear B cell epitopes with at least 10 amino acids were mapped to the predicted 3D structure of SARS-CoV-2 nsp3 protein visualized via PyMol (84). The predicted count of T cell MHC-I and MHC-II epitopes, and the predicted score of linear B cell epitopes were computed as the sliding averages with a window size of ten amino acids. The nsp3 protein 3D structure was predicted using C-I-Tasser (85) available in the Zhang Lab webserver (https://zhanglab.ccmb.med.umich.edu/C-I-TASSER/2019-nCov/).
Data Availability Statement
All datasets generated for this study are included in the article/Supplementary Material.
Author Contributions
EO and YH contributed to the study design. EO, MW, and AH collected the data. EO performed bioinformatics analysis. EO, MW, and YH wrote the manuscript. All authors performed result interpretation, discussed, and reviewed the manuscript.
Funding
This work has been supported by the NIH-NIAID grants 1R01AI081062 and 1UH2AI132931. The article-processing charge for this article was paid by a discretionary fund from Dr. William King, the director of the Unit for Laboratory Animal Medicine (ULAM) in the University of Michigan. This manuscript has been released as a pre-print at https://www.biorxiv.org/content/10.1101/2020.03.20.000141v2 (86).
Conflict of Interest
The authors declare that the research was conducted in the absence of any commercial or financial relationships that could be construed as a potential conflict of interest.
Supplementary Material
The Supplementary Material for this article can be found online at: https://www.frontiersin.org/articles/10.3389/fimmu.2020.01581/full#supplementary-material
References
1. Perlman S, Netland J. Coronaviruses post-SARS: update on replication and pathogenesis. Nat Rev Microbiol. (2009) 7:439–50. doi: 10.1038/nrmicro2147
2. Cabeça TK, Granato C, Bellei N. Epidemiological and clinical features of human coronavirus infections among different subsets of patients. Influenza Other Respir Viruses. (2013) 7:1040–7. doi: 10.1111/irv.12101
3. Lu R, Zhao X, Li J, Niu P, Yang B, Wu H, et al. Genomic characterisation and epidemiology of 2019 novel coronavirus: implications for virus origins and receptor binding. Lancet. (2020) 395:565–74. doi: 10.1016/S0140-6736(20)30251-8
4. Lai CC, Shih TP, Ko WC, Hsueh PR. Severe acute respiratory syndrome coronavirus 2 (SARS-CoV-2) coronavirus disease-2019 (COVID-19): the epidemic the challenges. Int J Antimicrob Agents. (2020) 55:105924. doi: 10.1016/j.ijantimicag.2020.105924
5. Chan JFW, Lau SKP, To KKW, Cheng VCC, Woo PCY, Yue KY. Middle east respiratory syndrome coronavirus: another zoonotic betacoronavirus causing SARS-like disease. Clin Microbiol Rev. (2015) 28:465–522. doi: 10.1128/CMR.00102-14
6. Li F. Structure, function, and evolution of Coronavirus Spike Proteins. Annu Rev Virol. (2016) 3:237–61. doi: 10.1146/annurev-virology-110615-042301
7. Roper RL, Rehm KE. SARS vaccines: where are we? Expert Rev Vaccines. (2009) 8:887–98. doi: 10.1586/erv.09.43
8. De Wit E, Van Doremalen N, Falzarano D, Munster VJ. SARS and MERS: recent insights into emerging coronaviruses. Nat Rev Microbiol. (2016) 14:523–34. doi: 10.1038/nrmicro.2016.81
9. van Doremalen N, Haddock E, Feldmann F, Meade-White K, Bushmaker T, Fischer R, et al. A single dose of ChAdOx1 MERS provides broad protective immunity against a variety of MERS-CoV strains. bioRxiv [Preprint]. (2020) doi: 10.1101/2020.04.13.036293v1
10. Plotkin SA. Updates on immunologic correlates of vaccine-induced protection. Vaccine. (2020) 38:2250–7. doi: 10.1016/j.vaccine.2019.10.046
11. See RH, Petric M, Lawrence DJ, Mok CPY, Rowe T, Zitzow LA, et al. Severe acute respiratory syndrome vaccine efficacy in ferrets: whole killed virus and adenovirus-vectored vaccines. J Gen Virol. (2008) 89:2136–46. doi: 10.1099/vir.0.2008/001891-0
12. Weingartl H, Czub M, Czub S, Neufeld J, Marszal P, Gren J, et al. Immunization with modified vaccinia virus ankara-based recombinant vaccine against severe acute respiratory syndrome is associated with enhanced hepatitis in ferrets. J Virol. (2004) 78:12672–6. doi: 10.1128/jvi.78.22.12672-12676.2004
13. Folaranmi T, Rubin L, Martin SW, Patel M, MacNeil JR. Use of serogroup B meningococcal vaccines in persons aged≥ 10 years at increased risk for serogroup B meningococcal disease: recommendations of the Advisory Committee on Immunization Practices, 2015. MMWR Morb Mortal Wkly Rep. (2015) 64:608–12.
14. He Y, Rappuoli R, De Groot AS, Chen RT. Emerging vaccine informatics. J Biomed Biotechnol. (2010) 2010:1–26. doi: 10.1155/2010/218590
15. Dalsass M, Brozzi A, Medini D, Rappuoli R. Comparison of open-source reverse vaccinology programs for bacterial vaccine antigen discovery. Front Immunol. (2019) 10:113. doi: 10.3389/fimmu.2019.00113
16. He Y, Xiang Z, Mobley HLT. Vaxign: the first web-based vaccine design program for reverse vaccinology and applications for vaccine development. J Biomed Biotechnol. (2010) 2010:1–15. doi: 10.1155/2010/297505
17. Xiang ZA, He YO. Genome-wide prediction of vaccine targets for human herpes simplex viruses using Vaxign reverse vaccinology BMC Bioinformatics. (2013) 14:1–10. doi: 10.1186/1471-2105-14-S4-S2
18. Singh R, Garg N, Shukla G, Capalash N, Sharma P. Immunoprotective efficacy of acinetobacter baumannii outer membrane protein, filf, predicted in silico as a potential vaccine candidate. Front Microbiol. (2016) 7:158. doi: 10.3389/fmicb.2016.00158
19. Navarro-Quiroz E, Navarro-Quiroz R, España-Puccini P, Villarreal JL, Perez AD, Ponce CF, et al. Prediction of epitopes in the proteome of Helicobacter pylori. Glob J Health Sci. (2018) 10:148. doi: 10.5539/gjhs.v10n7p148
20. Ong E, Wang H, Wong MU, Seetharaman M, Valdez N, He Y. Vaxign-ML: supervised machine learning reverse vaccinology model for improved prediction of bacterial protective antigens. Bioinformatics. (2020) 36:3185–91. doi: 10.1093/bioinformatics/btaa119
21. Redelman-Sidi G. Could BCG be used to protect against COVID-19? Nat Rev Urol. (2020) 17:316–7. doi: 10.1038/s41585-020-0325-9
22. See RH, Zakhartchouk AN, Petric M, Lawrence DJ, Mok CPY, Hogan RJ, et al. Comparative evaluation of two severe acute respiratory syndrome (SARS) vaccine candidates in mice challenged with SARS coronavirus. J Gen Virol. (2006) 87:641–50. doi: 10.1099/vir.0.81579-0
23. Graham RL, Becker MM, Eckerle LD, Bolles M, Denison MR, Baric RS. A live, impaired-fidelity coronavirus vaccine protects in an aged, immunocompromised mouse model of lethal disease. Nat Med. (2012) 18:1820. doi: 10.1038/nm.2972
24. Fett C, DeDiego ML, Regla-Nava JA, Enjuanes L, Perlman S. Complete protection against severe acute respiratory syndrome coronavirus-mediated lethal respiratory disease in aged mice by immunization with a mouse-adapted virus lacking E Protein. J Virol. (2013) 87:6551–9. doi: 10.1128/jvi.00087-13
25. Gao Q, Bao L, Mao H, Wang L, Xu K, Yang M, et al. Rapid development of an inactivated vaccine for SARS-CoV-2. bioRxiv [Preprint]. doi: 10.1101/2020.04.17.046375v1 (2020)
26. McKay PF, Hu K, Blakney AK, Samnuan K, Bouton CR, Rogers P, et al. Self-amplifying RNA SARS-CoV-2 lipid nanoparticle vaccine induces equivalent preclinical antibody titers and viral neutralization to recovered COVID-19 patients. bioRxiv [Preprint]. (2020). doi: 10.1101/2020.04.22.055608v1
27. Zha L, Zhao H, Mohsen MO, Hong L, Zhou Y, Yao C, et al. Development of a COVID-19 vaccine based on the receptor binding domain displayed on virus-like particles. bioRxiv [Preprint]. (2020). doi: 10.1101/2020.05.06.079830v2
28. Bisht H, Roberts A, Vogel L, Bukreyev A, Collins PL, Murphy BR, et al. Severe acute respiratory syndrome coronavirus spike protein expressed by attenuated vaccinia virus protectively immunizes mice. Proc Natl Acad Sci USA. (2004) 101:6641–6. doi: 10.1073/pnas.0401939101
29. Zhao J, Zhao J, Mangalam AK, Channappanavar R, Fett C, Meyerholz DK, et al. Airway memory CD4+ T cells mediate protective immunity against emerging respiratory coronaviruses. Immunity. (2016) 44:1379–91. doi: 10.1016/j.immuni.2016.05.006
30. Traggiai E, Becker S, Subbarao K, Kolesnikova L, Uematsu Y, Gismondo MR, et al. An efficient method to make human monoclonal antibodies from memory B cells: potent neutralization of SARS coronavirus. Nat Med. (2004) 10:871–5. doi: 10.1038/nm1080
31. Channappanavar R, Zhao J, Perlman S. T cell-mediated immune response to respiratory coronaviruses. Immunol Res. (2014) 59:118–28. doi: 10.1007/s12026-014-8534-z
32. Wilkinson TM, Li CKF, Chui CSC, Huang AKY, Perkins M, Liebner JC, et al. Preexisting influenza-specific CD4 + T cells correlate with disease protection against influenza challenge in humans. Nat Med. (2012) 18:274–80. doi: 10.1038/nm.2612
33. Tang F, Quan Y, Xin ZT, Wrammert J, Ma MJ, Lv H, et al. Lack of peripheral memory B cell responses in recovered patients with severe acute respiratory syndrome: a six-year follow-up study. J Immunol. (2011) 186:7264–8. doi: 10.4049/jimmunol.0903490
34. Zhao J, Zhao J, Perlman S. T cell responses are required for protection from clinical disease and for virus clearance in severe acute respiratory syndrome coronavirus-infected mice. J Virol. (2010) 84:9318–25. doi: 10.1128/jvi.01049-10
35. Coleman CM, Sisk JM, Halasz G, Zhong J, Beck SE, Matthews KL, et al. CD8+ T cells macrophages regulate pathogenesis in a mouse model of middle east respiratory syndrome. J Virol. (2017) 91:16. doi: 10.1128/jvi.01825-16
36. Zhao P, Cao J, Zhao LJ, Qin ZL, Ke JS, Pan W, et al. Immune responses against SARS-coronavirus nucleocapsid protein induced by DNA vaccine. Virology. (2005) 331:128–35. doi: 10.1016/j.virol.2004.10.016
37. Yasui F, Kai C, Kitabatake M, Inoue S, Yoneda M, Yokochi S, et al. Prior Immunization with Severe Acute Respiratory Syndrome (SARS)-Associated Coronavirus (SARS-CoV) nucleocapsid protein causes severe pneumonia in mice infected with SARS-CoV. J Immunol. (2008) 181:6337–48. doi: 10.4049/jimmunol.181.9.6337
38. Ribet D, Cossart P. How bacterial pathogens colonize their hosts and invade deeper tissues. Microbes Infect. (2015) 17:173–83. doi: 10.1016/j.micinf.2015.01.004
39. Ong E, Wong MU, He Y. Identification of new features from known bacterial protective vaccine antigens enhances rational vaccine design. Front Immunol. (2017) 8:1382. doi: 10.3389/fimmu.2017.01382
40. Wrapp D, Wang N, Corbett KS, Goldsmith JA, Hsieh, C.-L, Abiona O, et al. Cryo-EM structure of the 2019-nCoV spike in the prefusion conformation. Science. (2020) 367:1260–3. doi: 10.1126/science.abb2507
41. Letko M, Marzi A, Munster V. Functional assessment of cell entry and receptor usage for SARS-CoV-2 and other lineage B betacoronaviruses. Nat Microbiol. (2020) 5:562–9. doi: 10.1038/s41564-020-0688-y
42. Lei J, Kusov Y, Hilgenfeld R. Nsp3 of coronaviruses: structures and functions of a large multi-domain protein. Antiviral Res. (2018) 149:58–74. doi: 10.1016/j.antiviral.2017.11.001
43. Yang B, Sayers S, Xiang Z, He Y. Protegen: a web-based protective antigen database and analysis system. Nucleic Acids Res. (2011) 39:1073–8. doi: 10.1093/nar/gkq944
44. Rothbard JB, Taylor WR. A sequence pattern common to T cell epitopes. EMBO J. (1988) 7:93–100. doi: 10.1002/j.1460-2075.1988.tb02787.x
45. Shi SQ, Peng JP, Li YC, Qin C, Liang GD, Xu L, et al. The expression of membrane protein augments the specific responses induced by SARS-CoV nucleocapsid DNA immunization. Mol Immunol. (2006) 43:1791–8. doi: 10.1016/j.molimm.2005.11.005
46. Al-Amri SS, Abbas AT, Siddiq LA, Alghamdi A, Sanki MA, Al-Muhanna MK, et al. Immunogenicity of Candidate MERS-CoV DNA vaccines based on the spike protein. Sci Rep. (2017) 7:44875. doi: 10.1038/srep44875
47. Glansbeek HL, Haagmans BL, Te Lintelo EG, Egberink HF, Duquesne V, Aubert A, et al. Adverse effects of feline IL-12 during DNA vaccination against feline infectious peritonitis virus. J Gen Virol. (2002) 83:1–10. doi: 10.1099/0022-1317-83-1-1
48. Grifoni A, Sidney J, Zhang Y, Scheuermann RH, Peters B, Sette A. A sequence homology and bioinformatic approach can predict candidate targets for immune responses to SARS-CoV-2. Cell Host Microbe. (2020) 27:671–80.e2. doi: 10.1016/j.chom.2020.03.002
49. Grifoni A, Weiskopf D, Ramirez SI, Mateus J, Dan JM, Rydyznski Moderbacher C, et al. Targets of T cell responses to SARS-CoV-2 coronavirus in humans with COVID-19 disease and unexposed individuals. Cell. (2020) 181:1489–501. doi: 10.1016/j.cell.2020.05.015
50. Hofmann H, Pyrc K, Van Der Hoek L, Geier M, Berkhout B, Pöhlmann S. Human coronavirus NL63 employs the severe acute respiratory syndrome coronavirus receptor for cellular entry. Proc Natl Acad Sci USA. (2005) 102:7988–93. doi: 10.1073/pnas.0409465102
51. Salat J, Mikulasek K, Larralde O, Formanova PP, Chrdle A, Haviernik J, et al. Tick-borne encephalitis virus vaccines contain non-structural protein 1 antigen and may elicit NS1-specific antibody responses in vaccinated individuals. Vaccines. (2020) 8:81. doi: 10.3390/vaccines8010081
52. Schlesinger JJ, Brandriss MW, Walsh EE. Protection against 17D yellow fever encephalitis in mice by passive transfer of monoclonal antibodies to the nonstructural glycoprotein gp48 and by active immunization with gp48. J Immunol. (1985) 135:2805–9.
53. Gibson CA, Schlesinger JJ, Barrett ADT. Prospects for a virus non-structural protein as a subunit vaccine. Vaccine. (1988) 6:7–9. doi: 10.1016/0264-410X(88)90004-7
54. Chen HR, Lai YC, Yeh TM. Dengue virus non-structural protein 1: a pathogenic factor, therapeutic target, vaccine candidate. J Biomed Sci. (2018) 25:58. doi: 10.1186/s12929-018-0462-0
55. Ip PP, Boerma A, Regts J, Meijerhof T, Wilschut J, Nijman HW, et al. Alphavirus-based vaccines encoding nonstructural proteins of hepatitis c virus induce robust and protective T-cell responses. Mol Ther. (2014) 22:881–90. doi: 10.1038/mt.2013.287
56. Cafaro A, Tripiciano A, Picconi O, Sgadari C, Moretti S, Butt, ò S, et al. Anti-tat immunity in HIV-1 infection: effects of naturally occurring and vaccine-induced antibodies against tat on the course of the disease. Vaccines. (2019) 7:99. doi: 10.3390/vaccines7030099
57. Millet P, Campbell GH, Sulzer AJ, Grady KK, Pohl J, Aikawa M, et al. Immunogenicity of the Plasmodium falciparum asexual blood-stage synthetic peptide vaccine SPf66. Am J Trop Med Hyg. (1993) 48:424–31. doi: 10.4269/ajtmh.1993.48.424
58. Sealy R, Slobod KS, Flynn P, Branum K, Surman S, Jones B, et al. Preclinical and clinical development of a multi-envelope, DNA-virus-protein (D-V-P) HIV-1 vaccine. Int Rev Immunol. (2009) 28:49–68. doi: 10.1080/08830180802495605
59. He Y, Racz R, Sayers S, Lin Y, Todd T, Hur J, et al. Updates on the web-based VIOLIN vaccine database and analysis system. Nucleic Acids Res. (2014) 42:1124–32. doi: 10.1093/nar/gkt1133
60. Ong E, Sun P, Berke K, Zheng J, Wu G, He Y. VIO: ontology classification and study of vaccine responses given various experimental and analytical conditions. BMC Bioinformatics. (2019) 20:1–10. doi: 10.1186/s12859-019-3194-6
61. The UniProt Consortium. The Universal Protein Resource (UniProt). Nucleic Acids Res. (2008) 36:D193–7. doi: 10.1093/nar/gkl929
62. Sachdeva G, Kumar K, Jain P, Ramachandran S. SPAAN: a software program for prediction of adhesins and adhesin-like proteins using neural networks. Bioinformatics. (2005) 21:483–91. doi: 10.1093/bioinformatics/bti028
63. Krogh A, Larsson B, von Heijne G, Sonnhammer EL. Predicting transmembrane protein topology with a hidden Markov model: application to complete genomes. J Mol Biol. (2001) 305:567–80. doi: 10.1006/jmbi.2000.4315
64. Li L, Stoeckert CJ, Roos DS. OrthoMCL: identification of ortholog groups for eukaryotic genomes. Genome Res. (2003) 13:2178–89. doi: 10.1101/gr.1224503
65. Doytchinova I, Flower DR. VaxiJen: a server for prediction of protective antigens, tumour antigens and subunit vaccines. BMC Bioinformatics. (2007) 8:4. doi: 10.1186/1471-2105-8-4
66. Bowman BN, McAdam PR, Vivona S, Zhang JX, Luong T, Belew RK, et al. Improving reverse vaccinology with a machine learning approach. Vaccine. (2011) 29:8156–64. doi: 10.1016/j.vaccine.2011.07.142
67. Heinson AI, Gunawardana Y, Moesker B, Denman Hume CC, Vataga E, Hall Y, et al. Enhancing the biological relevance of machine learning classifiers for reverse vaccinology. Int J Mol Sci. (2017) 18:312. doi: 10.3390/ijms18020312
68. Fleri W, Paul S, Dhanda SK, Mahajan S, Xu X, Fleri W, et al. The immune epitope database and analysis resource in epitope discovery and synthetic vaccine design. Front Immunol. (2017) 8:278. doi: 10.3389/fimmu.2017.00278
69. Dubchak I, Muchnik I, Holbrook SR, Kim SH. Prediction of protein folding class using global description of amino acid sequence. Proc Natl Acad Sci USA. (1995) 92:8700–4. doi: 10.1073/pnas.92.19.8700
70. Chou KC. Prediction of protein subcellular locations by incorporating quasi-sequence-order effect. Biochem Biophys Res Commun. (2000) 278:477–83. doi: 10.1006/bbrc.2000.3815
71. Feng ZP, Zhang CT. Prediction of membrane protein types based on the hydrophobic index of amino acids. J Protein Chem. (2000) 19:269–75. doi: 10.1023/A:1007091128394
72. Lin Z, Pan XM. Accurate prediction of protein secondary structural content. Protein J. (2001) 20:217–20. doi: 10.1023/A:1010967008838
73. Sokal RR, Thomson BA. Population structure inferred by local spatial autocorrelation: an example from an Amerindian tribal population. Am J Phys Anthropol. (2006) 129:121–31. doi: 10.1002/ajpa.20250
74. Ong SAK, Lin HH, Chen YZ, Li ZR, Cao Z. Efficacy of different protein descriptors in predicting protein functional families. BMC Bioinformatics. (2007) 8:1–14. doi: 10.1186/1471-2105-8-300
75. Pedregosa F, Varoquaux G, Gramfort A, Michel V, Thirion B, Grisel O, et al. Scikit-learn: machine learning in python. J Mach Learn Res. (2012) 12:2825–30. doi: 10.1007/s13398-014-0173-7.2
76. Chen T, Guestrin C. XGBoost: a scalable tree boosting system. In: Proceedings of the ACM SIGKDD International Conference on Knowledge Discovery and Data Mining. San Francisco, CA. (2016) 785–94. doi: 10.1145/2939672.2939785
77. Edgar RC. MUSCLE: multiple sequence alignment with high accuracy and high throughput. Nucleic Acids Res. (2004) 32:1792–7. doi: 10.1093/nar/gkh340
78. Gouy M, Guindon S, Gascuel O. Sea view version 4: a multiplatform graphical user interface for sequence alignment and phylogenetic tree building. Mol Biol Evol. (2010) 27:221–4. doi: 10.1093/molbev/msp259
79. Lefort V, Longueville JE, Gascuel O. SMS: smart model selection in PhyML. Mol Biol Evol. (2017) 34:2422–4. doi: 10.1093/molbev/msx149
80. Capra JA, Singh M. Predicting functionally important residues from sequence conservation. Bioinformatics. (2007) 23:1875–82. doi: 10.1093/bioinformatics/btm270
81. Greenbaum J, Sideny J, Chung J, Brander C, Peter B, Sette A. Functinal classification of class II human leukocyte antigen (HLA) molecules reveals seven different supertypes and a surprising degree of repertoire sharing across supertypes. Immunogenetics. (2013) 63:325–35. doi: 10.1007/s00251-011-0513-0.Functional
82. Weiskopf D, Angelo MA, de Azeredo EL, Sidney J, Greenbaum JA, Fernando AN, et al. Comprehensive analysis of dengue virus-specific responses supports an HLA-linked protective role for CD8+ T cells. Proc Natl Acad Sci USA. (2013) 110:E2046–53. doi: 10.1073/pnas.1305227110
83. Jespersen MC, Peters B, Nielsen M, Marcatili P. BepiPred-2.0: improving sequence-based B-cell epitope prediction using conformational epitopes. Nucleic Acids Res. (2017) 45:W24–9. doi: 10.1093/nar/gkx346
84. Schrödinger L. The PyMol Molecular Graphics System, Version~1.8. (2015). Available online at: https://pymol.org (accessed May 15, 2020).
85. Zheng W, Li Y, Zhang C, Pearce R, Mortuza SM, Zhang Y. Deep-learning contact-map guided protein structure prediction in CASP13. Proteins Struct Funct Bioinformatics. (2019) 87:1149–64. doi: 10.1002/prot.25792
86. Ong E, Wong MU, Huffman A, He Y. COVID-19 coronavirus vaccine design using reverse vaccinology and machine learning. bioRxiv [Preprint]. (2020). Available at: https://www.biorxiv.org/content/10.1101/2020.03.20.000141v2 (accessed May 15, 2020).
Keywords: COVID-19, S protein, non-structural protein 3, vaccine, reverse vaccinology, machine learning, vaxign, vaxign-ML
Citation: Ong E, Wong MU, Huffman A and He Y (2020) COVID-19 Coronavirus Vaccine Design Using Reverse Vaccinology and Machine Learning. Front. Immunol. 11:1581. doi: 10.3389/fimmu.2020.01581
Received: 14 May 2020; Accepted: 15 June 2020;
Published: 03 July 2020.
Edited by:
Ken J. Ishii, University of Tokyo, JapanReviewed by:
Behazine Combadiere, INSERM U1135 Centre d'Immunologie et de Maladies Infectieuses, FranceRupsa Basu, TechnoVax Inc, United States
Copyright © 2020 Ong, Wong, Huffman and He. This is an open-access article distributed under the terms of the Creative Commons Attribution License (CC BY). The use, distribution or reproduction in other forums is permitted, provided the original author(s) and the copyright owner(s) are credited and that the original publication in this journal is cited, in accordance with accepted academic practice. No use, distribution or reproduction is permitted which does not comply with these terms.
*Correspondence: Yongqun He, eW9uZ3F1bmhAbWVkLnVtaWNoLmVkdQ==