- 1Mayo Clinic Vaccine Research Group, Mayo Clinic, Rochester, MN, United States
- 2Icahn School of Medicine at Mount Sinai, New York, NY, United States
The development of vaccines, which prime the immune system to respond to future infections, has led to global declines in morbidity and mortality from dreadful infectious communicable diseases. However, many pathogens of public health importance are highly complex and/or rapidly evolving, posing unique challenges to vaccine development. Several of these challenges include an incomplete understanding of how immunity develops, host and pathogen genetic variability, and an increased societal skepticism regarding vaccine safety. In particular, new high-dimensional omics technologies, aided by bioinformatics, are driving new vaccine development (vaccinomics). Informed by recent insights into pathogen biology, host genetic diversity, and immunology, the increasing use of genomic approaches is leading to new models and understanding of host immune system responses that may provide solutions in the rapid development of novel vaccine candidates.
Introduction
Infectious diseases can lead to illness, human suffering, economic costs, medical complications, hospitalization, disability, and death. Besides sanitation and clean water, vaccines have had the greatest impact on human health and longevity (1). The cost of vaccine-preventable diseases (VPD) just in the USA during 2015 was estimated at $9 billion (2). From 2011–2020, one model estimated that 23.3 million deaths worldwide will have been averted by vaccines (3). In the 2017–2018 influenza season, it is estimated that almost one million Americans were hospitalized and 90,000 died due to influenza (4). Worldwide, it is estimated that, between 2000 and 2014, 17.1 million deaths due to measles were averted by the use of the measles vaccine (5).
While there are over 1,400 known species of human pathogens with more being discovered every year, in the US, licensed vaccines exist for only 26 pathogens (6). Preventing infections with vaccines is a complex, costly, and lengthy process that requires overcoming multiple challenges before resulting in a safe and effective product (Box 1). Historically, vaccine development has followed an empiric “isolate, inactivate and inject” paradigm, whereby the disease-causing pathogen or its disease-mediating entity (e.g., a toxin) is identified, inactivated, and injected in order to elicit a protective immune response (7–9). This empiric method, developed before the genetic revolution, enabled the development of many early and effective vaccines against pathogens such as influenza, tetanus, diphtheria, and pertussis. In the case of viruses, including smallpox, measles, mumps, rubella, and smallpox, a parallel approach has been to substitute inactivation with attenuation.
Box 1. Current Challenges Facing Vaccine Development Efforts.
The creation of new vaccines is a slow, systematic, expensive, and laborious process that requires coordination between scientists, physicians, public health officials, industry and vaccine developers, and society. These shareholders must work together in order for us to overcome the listed challenges in order to successfully development safe and effective vaccines that see widespread use.
• High (and increasing) costs for vaccine development (~$700 million–$1 billion)
• Vaccine hesitancy
• More stringent safety requirements
• Societal expectations of 100% efficacy
• Need to maintain cold-chain for vaccines
• Increasing requirements for single dose efficacy
• Need for rapid response to global outbreaks
• Limited number of vaccine manufacturers
• Product development time (typically ~10 years)
• Current pathogens require more complicated vaccines
• Low efficacy of some licensed vaccines
• Business models prioritize vaccines by market potential, not by public health need
• Aging world population that respond poorly to most vaccines (immunosenescence)
• Limited number of approved and acceptable adjuvants
• Concurrent health problems in developing world that compromise immune response (nutrition, co-infection)
• Incomplete or inadequate understanding of biology, pathogenesis, and/or immunology of emerging pathogens
• Inability to properly attenuate pathogens OR risk of reversion to wild type organism
• Humoral immune responses do not always correlate with protection
• Inappropriate/harmful immune response (formalin-inactivated RSV products) or enhanced disease upon re-infection (Dengue)
• Inadequate durability of immune response (ex. Pertussis)
While this empiric approach has led to tremendous successes, the work is far from finished; major, significant barriers remain (Figure 1). This review focuses on five of these barriers: an incomplete understanding of how immunity develops, host and pathogen genetic variability, problems related to vaccine safety, and both environmental (e.g., nutrition, obesity) and geographic factors (e.g., maintaining a cold chain in Sub-Saharan Africa, co-infection in tropical climates) that compromise vaccine usage or efficacy. Because of these barriers, the traditional empiric approach has been ineffective for developing vaccines against hypervariable and highly complex pathogens, such as Mycobacterium tuberculosis, malaria-causing Plasmodium, hookworm, HIV, HCV, coronaviruses, among others. This is due to their complex life cycles, the ability of these pathogens to rapidly alter their surface proteins (i.e., antigenic variation) and other mechanisms by which the pathogen can evade host detection and the host immune response. Complex immunology can also be a barrier; for example, the recent demonstration of antibody-dependent disease enhancement which has hindered the development and use of the recently licensed Dengue vaccine (10).
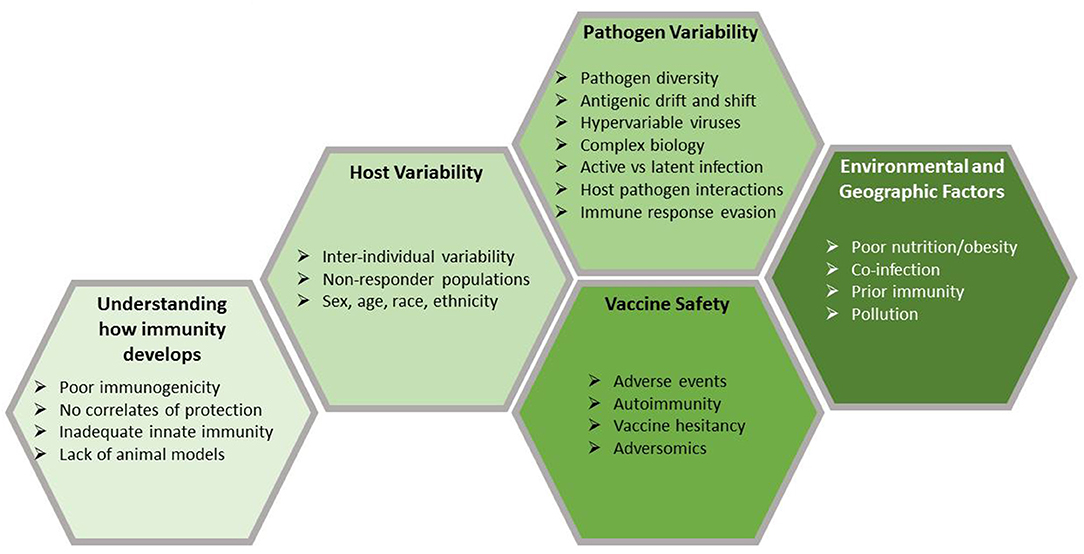
Figure 1. Barriers to vaccine development. Vaccines are the most effective public health tool for controlling infectious diseases. Despite considerable success, there is room for improvement in many current vaccines and there are a large number of new and re-emerging pathogens for which we do not have effective vaccines. Vaccine development faces a number of challenges, many of which are presented here. Developing vaccines to combat current and future pathogens will require us to overcome those challenges and recent developments in genomic technologies may provide the solutions that we need.
In response to the challenges posed by these barriers, novel approaches such as vaccinomics (which aims to understand genomic and systems-level data to elucidate the basis of inter-individual variations in immune responses), reverse vaccinology (which uses genetic sequence information to identify immunogenic antigens), and structure-based vaccine design have been developed to take advantage of high-dimensional tools and techniques and generate novel data that can be leveraged to create new vaccine products (Figure 2) (11–13). In the past decade, new vaccines, including the licensed Meningococcus B vaccine, have been designed and developed using such genomics-based approaches (14, 15). With the increasing sophistication and decreased expense of gene-based assays and next-generation sequencing technologies, genomics is accelerating the development of new vaccines in the twenty-first century—closely paralleling the application of genomics to other aspects of human medicine, such as individualized medicine.
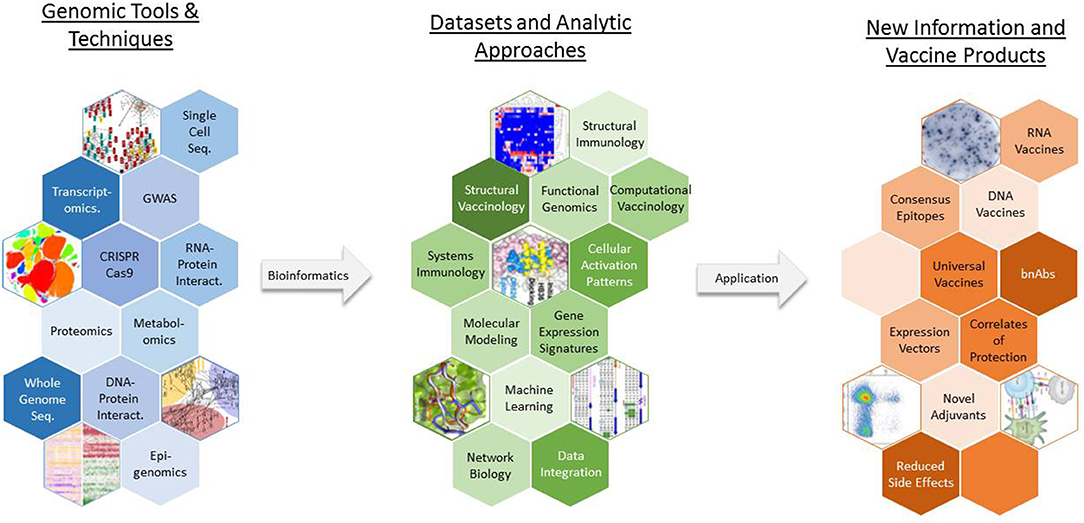
Figure 2. The use of vaccinomics in new vaccine development. A wide array of genomic tools and techniques are available for researchers to study various aspects of pathogen biology and host physiology and immune response. Vaccinomics and similar approaches represent toolboxes that contain a specific assortment of laboratory assays, statistical analysis routines, bioinformatic methods, and computational models that can be used to generate an appropriate dataset and extract biologically meaningful results from the data. GWAS, genome wide association study; bnAbs, broadly neutralizing antibodies.
Genetics has expanded far beyond the simple nucleic acid sequence of a given organism. While it primarily deals with individual genes, it also includes the myriad regulatory mechanisms that control gene expression (16–22). Similarly, genomics has also expanded in scope to include the comprehensive characterization of gene expression, regulation, interdependency, pre- and post-transcriptional modifications, gene editing, epistasis, complementarity, pleiotropy, and other complex interactions (23).
Genomics is not the only area that has undergone remarkable transformation recently in terms of the technologies and platforms that can be used to design, create, and study vaccines. Examples include the following: mass cytometry, which allows for incredibly complex immunophenotyping (24, 25); proteomics and mass spectrometry (26–30); and metabolomics, which has been closely linked to immunologic function and vaccine response (31–33). However, in this focused review, we will explore how genomics and recent genomic technologies have impacted vaccine development and may provide solutions to both the long-standing barriers in vaccine design and the new challenges posed by new and re-emerging pathogens of public health importance. Creative application of these tools and the biological insights that they provide are poised to truly revolutionize how we design, develop, test, and deploy vaccines (Figure 3).
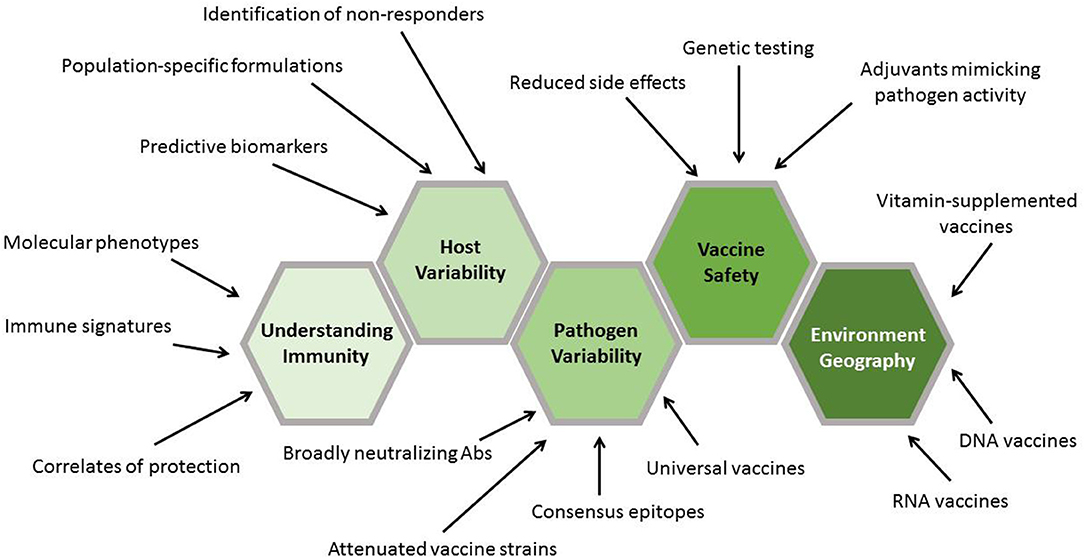
Figure 3. Contribution of genomics to vaccine development stages. The results generated by genomic approaches inform each stage of vaccine development. Initial genomics work plays a critical role in discovering new vaccine targets. This is followed by the characterization of these targets in terms of their ability to generate protective immune responses. Validation of the findings in genetically diverse populations is aided by information gained through genomics approaches. Finally, application of the findings assists in the design and completion of phase I–IV clinical trials.
Barrier #1: Incomplete Understanding of How Immunity Develops
The human immune system is incredibly complex, with multiple tissues and organs, dozens of different signaling pathways (34), hundreds of different cells (35), thousands of different effector molecules, and an effectively infinite ability to recognize foreign antigens (36)—all of which must be “choreographed” effectively, kinetically, and in proper sequence. Immunologists have developed a large and comprehensive (but by no means complete) catalog of the individual parts that make up the system (37–39); however, our reductionist understanding of how these parts collaboratively function as a “system” has lagged behind (40). While we understand what most of the parts do individually, we have more trouble understanding how each component inter-relates and collectively contributes to the development of immunity at the systems-level (41, 42). In short, we do not comprehensively understand the rules governing the behavior of the system and therefore cannot reliably and consistently predict the outcome of a given infection or vaccination (43). Developing this understanding is a critical first step in our ability to predict and eventually manipulate the immune system in order to achieve the desired outcome of protective immunity (44).
This is the knowledge gap that systems biology and vaccinomics paradigms seek to fill by capturing complex relationships among immune components as the host responds to infection or vaccination—rather than simple reductionist approaches to single components of the system. These studies have been made possible by our growing ability to measure increasingly larger and more complete collections of molecules. For example, instead of a single quantitative PCR reaction, we can use next generation sequencing to simultaneously sequence millions of different DNA or RNA molecules (45, 46). We can characterize comprehensive changes within each cell's entire transcriptome, epigenome, proteome, metabolome, and multiple other “-omes” (47–49). We can characterize the cell types and sub-types involved in the response, their phenotype, their activation state, and their biological functions (50, 51). We can capture the signals generated by multiple signal-transduction pathways inside each cell (52) and observe the communication occurring between cells in normal health (53) and during infection (54). Collectively, these technologies have been applied to understanding immune function, host-pathogen interactions, pathogen genetics, and pathogenesis in unprecedented detail (55–58).
A central organizing feature of these efforts is a focus on genomics, as gene expression is considered a critical first step because immune cells recognize and react to foreign antigens. Consequently, each of these studies has a primary goal of understanding the transcriptomic changes that occur during the development of an immune response. Querec et al. first applied high-throughput data to the characterization of yellow fever vaccine response in humans (59). Systems analysis was used to discover a distinct molecular signature that predicted the neutralizing antibody (Ab) (i.e., TNFRS17 gene signature) and antigen-specific CD8+ T cell (i.e., C1QB and EIF2AK3 gene signature) responses to the live attenuated yellow fever vaccine YF-17D in humans with up to 100 and 90% accuracy, respectively (59). Because expression levels of the genes identified in this study were highly predictive of both humoral and cell-mediated immune responses, these signatures can potentially function as early biomarkers of vaccine response, efficacy, and even safety. Similarly, Dunachie et al. identified a gene expression signature that correlates with vaccine-induced protection in a human malaria challenge model in which the expression of genes associated with IFN induction and with antigen presentation correlated with protection against malaria (60).
Transcriptomic studies can reveal important factors controlling disease susceptibility and clinical outcomes during infection or vaccination. Through mechanisms that are not fully understood, clinical symptoms of dengue virus infection range from asymptomatic or mild disease (80%) to severe, life-threatening dengue hemorrhagic fever (DHF) or dengue shock syndrome (DSS). Transcriptomic profiling of the central nervous system (CNS) of mice infected with dengue identified putative innate signaling pathways (IFN signaling, IL-10, GM-CSF, PDGF), antigen processing, and complement activation signatures, which suggests that innate immune responses may serve to limit dengue virus replication in the CNS and thereby reduce disease severity (61). These findings suggest that adjuvant-mediated activation of these pathways could enhance vaccine response and/or provide therapeutic benefit. Similar gene expression studies in humans with dengue illness suggest that a transcriptomic signature detectable as early as 1 day after infection can potentially distinguish between dengue fever and the more serious dengue hemorrhagic fever (62). These results inform the development of molecular diagnostics and treatment options for patients.
Ebola virus infection is another disease where pathogenesis is not completely understood and transcriptomic analysis has revealed important insights into Ebola disease progression. Non-human primate survivors of experimental infection displayed upregulation of specific genes, including CCL8, compared to animals that succumbed to infection (63). Although the study was focused on therapeutics, the findings suggest additional correlates of protection beyond the typical antibody measures. In yet another example, microarrays have also been used to identify gene expression patterns (i.e., upregulation of NF-kB and IFNg signaling) that correlate with protection in trials with the malaria RTS,S vaccine (64). Thus, studies evaluating transcriptomic changes after infection/vaccination have provided rich insights into mechanisms of disease initiation, clinical progression, and vaccine-induced immunity (65). These studies have also identified potential correlates of protection and yielded predictive biomarkers that can be used to inform clinical care or to provide early go/no-go criteria for vaccine trials.
Systems biology studies have also provided important insights into the generation and maintenance (i.e., durability) of immune responses to many vaccines, including seasonal influenza (trivalent inactivated influenza vaccine [TIV] and MF59-adjuvanted influenza vaccine), malaria (RTS,S), meningococcal (MPSV4 and MCV4), and others (66–70). A systems biology approach comparing MF59-adjuvanted and TIV vaccine in immune-immature children (14–24-months-old, n = 90) identified significantly higher transcriptional responses to the MF59-adjuvanted vaccine and identified early innate response signatures correlated with Day 28 Ab titers (67). These include M16 (a module associated with TLR and inflammatory signaling); M11 (a module regulating monocyte function); M75 (a module controlling IFN-induced antiviral response); M156 (a module associated with Ab secreting cells); and S3 (a module with genes involved in immunoglobulin production). These findings may provide potentially generalizable molecular correlates of Ab production during early childhood (67).
Several adjuvants, such as MF59, AS01-4, TLR9 agonists, virosomes, and others have recently been licensed for use in human vaccines. For example, a recent Hepatitis B vaccine (Heplisav B) incorporating a TLR9 agonist has considerably improved seroconversion rates compared to other hepatitis vaccines—particularly in subjects who normally respond poorly and slowly (71). Similarly, the recently FDA-licensed MF59-adjuvanted influenza subunit vaccine (72) induces higher antibody titers, a broader humoral response, and longer persistence of influenza Ab titers than the non-adjuvanted, standard-dose influenza vaccine in older adults. This is a population that suffers the greatest burden of influenza-associated morbidity and mortality, yet has the poorest response to standard influenza vaccines (73, 74). A recent large study in 7,082 individuals (≥65 years of age) demonstrated significantly higher immunogenicity (p < 0.001, seroconversion and HAI GMT) of the MF59-adjuvanted vaccine compared to standard dose influenza vaccine (75). Similarly, the diversity, commonalities, and differences in human genetic and immune responses to two varicella zoster virus (VZV) vaccines, the live attenuated vaccine (Zostavax), and the AS01B-adjuvanted glycoprotein E vaccine (Shingrix) are being examined using systems biology approaches (31, 76). These vaccines exhibit significant differential immunogenicity and significant variations in the longevity of immunologic memory. Furthermore, the effect of age and immunosenescence is drastically different with these two vaccines. These clinically important differences provide an ideal system for studying the systems-level factors contributing to these differences and are likely to drastically improve our understanding of zoster immunology. In fact, recent reports have highlighted the novel finding that the magnitude and durability of immune responses to zoster vaccination are dependent on the abundance of both regulatory T cells and T cells expressing checkpoint markers (e.g., PD-1) (77). Systems studies examining the durability of immunity after measles, mumps, and rubella vaccination are in progress (78–84).
Structural Vaccinology
Structural biology studies have allowed investigators to map viral epitopes onto the three-dimensional structure of pathogen proteins (85, 86). Antibody-antigen complexes can also be determined, providing insights into critical antibody functionality such as neutralization or have identified critical conserved regions that can be targeted for more effective immune responses (87). Insights into conformational changes with RSV have enabled investigators to develop new vaccines that avoid the limitations of historical approaches (88) and design better immunogens (89). Another excellent example of structural vaccinology is the increasing use of virus-like-particles as vaccine platforms (90, 91).
Barrier #2: Host Variability
Genome-Wide Association Studies (GWAS)
Inter-individual variation in vaccine-specific immune response is known to be influenced by host gene polymorphisms. This genetic variability of the human population gave rise to vaccine-immunogenetics research focused on finding important genetic variants associated with variations in immune responses by assessing relationships between variability in immune response to vaccines and genetic factors. Certainly, population-based candidate gene association studies of vaccine-specific immune responses are beginning to reveal and explain how—and to what degree—variations in innate and adaptive immune responses following vaccination are determined by gene polymorphisms (92, 93). While a candidate gene approach was thought most efficient in the past decade, it is clear that a GWAS is an unbiased, agnostic approach that serves as a critical step in the research by identifying genetic variants impacting immunity and supporting a novel paradigm by which vaccine development could occur (92, 94, 95). A GWAS allows the identification of individual and groups of genes and genetic variants (SNPs, or single nucleotide polymorphisms) that are associated with specific markers of vaccine-induced immunity. At the systems level, genotype/phenotype computational models that integrate numerous additive and epistatic marker effects are needed. The evidence thus far suggests that the effect of one gene/allele depends on the presence of another gene/allele that may control a phenotype (e.g., epistatic interactions). The integration of epistasis network analysis and functional interactions into genotype-phenotype association studies have provided important insights into smallpox vaccine-induced immunity and specifically the role of variants in RXRA (the gene encoding a vitamin A receptor) in immune responses to smallpox and other viral vaccines (96–98). The most thorough and efficient study for such purposes is a two-stage (discovery–replication) genome-scale analysis (99), followed by functional studies to (1) validate which specific gene polymorphisms and pathways/gene sets have the biggest or most critical effect on inter-individual variations in immune responses among immunized subjects, and (2) identify the mechanism(s) by which these effects occur. Significant work delineating the effect of gene polymorphisms on hepatitis B, measles, mumps, rubella, influenza, smallpox, and anthrax vaccine-induced immune responses has been published (98, 100–107). Examples include the identification and replication of a CD46 measles virus receptor variant coding for a 53% reduction in Ab response to measles vaccine, which is a finding that could be used to reverse engineer a vaccine to circumvent this viral receptor genetic restriction (108). Our studies identified a SNP (rs2064479, p = 8.6 × 10−8) in the class II HLA-DPB1 gene region associated with variations in rubella-specific Ab titers after rubella vaccine (106). Additional SNPs (p ≤ 1.0 × 10−7) in high linkage disequilibrium (LD, r2 ≥ 0.8) of rs2064479 were also positioned near the genetic region of HLA-DPB1. Some of these polymorphisms were predicted to be located in miRNA binding sites. These data validate the previous findings of HLA-DPB1 genotypes (i.e., HLA-DPB1*04:01 and HLA-DPB1*03:01) linked with rubella vaccine-specific immune response (109, 110). It has been previously demonstrated that the DPB1*04:01 and DPB1*03:01 alleles are associated with significantly higher and lower Ab responses, respectively (109, 110). It is highly likely that the DPB1*04:01 molecule presents an array of processed epitopes to CD4+ T cells different from that of the DPB1*03:01 allele and is therefore able to stimulate more robust rubella-specific T cell responses, which in turn elicit robust humoral immune responses. Indeed, earlier work revealed that HLA genes/proteins are critical elements for immune responses to rubella vaccination, accounting for ~20% of the total genetic inter-individual variation in Ab response to rubella (109).
Multiple GWAS studies have shown that allele-specific HLA class I and class II genetic polymorphisms play a fundamental function in the differential generation of viral vaccine-induced immune responses (109, 111–116). Identifying which specific HLA alleles are associated with protective immune responses through vaccination is critical for population health and for a deeper understanding of vaccine-induced immunology and vaccine development. We have leveraged such knowledge to identify naturally processed and HLA-presented viral-derived epitopes using mass spectrometry techniques (117). Peptide identification using this approach provides the framework for the selection and use of these immunodominant pathogen epitopes as candidate vaccine targets (118). Studies have illustrated several regulatory and common SNPs in the different regions of HLA genes associated with immune responses to childhood immunization, such as the capsular group C meningococcal (MenC); Haemophilus influenza type B (Hib); tetanus toxoid (TT); hepatitis B (HBV); 7-valent pneumococcal conjugate (PCV7); and the diphtheria, tetanus, and acellular pertussis (DTaP) vaccines (119, 120). Through studies such as these, GWAS can be used to identify critical genetic determinants of vaccine-specific immunity and assist in the development of novel vaccines that overcome these genetic restrictions.
GWAS studies also demonstrate that multigenic effects (121, 122) including HLA and a variety of immune, innate, and adaptive gene SNPs significantly affect immune responses to vaccines (114, 123–126). Likewise, synergistic effects of tapasin gene polymorphisms and specific HLA class I alleles to generate stronger anti-viral CD8+ T cell responses have been observed. In a study of subjects with resolved or chronic hepatitis C virus (HCV) infection in UK, Germany and US, tapasin G alleles in a combination with specific HLA class IB alleles with an aspartate (Asp) at residues 114 and 156 have been associated with stronger anti-viral CD8+ T cell responses against HCV and with the outcome of HCV infection (127). This suggests that tapasin gene polymorphisms maybe important for antigen processing and HLA class I peptide loading mechanisms (128). A large number of other genes and gene families (e.g., interferon response factors, pattern recognition receptors, cytokines, chemokines) have been implicated in the control of immune responses to vaccines (129), and the literature is full of disease susceptibility studies that highlight additional genes and pathways contributing to immune responses to pathogens (130). Many of these study results are available in online databases such as this one: https://www.ebi.ac.uk/gwas/. Genetic studies of vaccine responses have revealed effects that are both quantifiable and predictable (7, 13, 109, 114, 121, 122, 131–135). Informed by such studies, the development of novel vaccines and adjuvants that specifically target innate receptors and their signaling pathways (e.g., TLR pathway), leading to higher protection rates and enhanced immune responses, is possible.
Barrier #3: Pathogen Variability
Pathogen genetic sequence variability is a major impediment to vaccine development (136–138). This can manifest in multiple ways: (1) tremendous sequence diversity among viral strains—as an example, a major challenge in the development of an effective rhinovirus vaccine is that it must elicit cross-protective immunity across over 160 different circulating rhinovirus strains (139); (2) antigenic drift or shift, as demonstrated by influenza viruses, which necessitates a yearly reformulation of influenza vaccines; (3) a complicated lifecycle during which large segments of the genome are turned on and off, as is the case with Plasmodium falciparum (Plasmodium life-cycle stages also affect the type of immune response that is required to combat the pathogen); (4) pathogens with large, complex genomes, such as Mycobacterium tuberculosis, where effective immunologic targets or immunomodulatory molecules are difficult to identify, and therefore have not been effectively dealt with; (5) vaccine-induced pressure leading to changes in serotype prevalence, as has been demonstrated with the heptavalent pneumococcal vaccine (140); (6) pathogens with rapid mutation rates, such as HIV and HCV, also complicate the issue as the antigenic targets of the immune response rapidly shift during an infection forcing the immune response to chase an ever-changing target; (7) zoonoses that cross the species barrier to infect humans (e.g., SARS-CoV, MERS-CoV, H5, SARS-CoV-2).
The host immune response typically recognizes and responds to a small set of immunodominant epitopes (141). For humoral responses, these epitopes are typically the linear or conformational areas that are readily accessible to antibodies. Unfortunately, these areas of the pathogen genome are often hot spots for mutation or recombination events, enabling the pathogen to evade immune responses by displaying modified surface proteins that are no longer recognized by existing antibodies, forcing the immune system to start over—an effect repeatedly demonstrated by influenza virus and HIV (142–145). Sequence differences between viral, bacterial, and parasite strains are often found at these locations; therefore, a neutralizing antibody specific to an epitope on the HA protein of one influenza strain will not necessarily bind to or neutralize that same site on another influenza strain. An analogous situation exists for bacteria, where a second strain may possess entirely different virulence factors than the first. The new strain may be effectively invisible to the immune response specific for the first strain. In this manner, strain diversity contributes to antigenic differences that determine whether or not immune responses are cross-protective. Understanding the factors controlling immunodominance and how pathogens exploit this is of critical importance (146, 147).
By identifying genetically conserved regions, investigators can target epitopes more likely to be present across multiple strains, thereby creating immune responses that are cross-protective. For example, the use of conserved stalk regions of the influenza hemagglutinin (HA) protein to develop universal influenza vaccines is an excellent example of this type of work. Another example is the Plasmodium falciparum Reticulocyte Binding Protein Homolog 5 (PfRh5), which facilitates parasite entry into human red blood cells through binding to the Ok blood group antigen (148). Because the PfRh5 protein is targeted by broadly acting, parasite-neutralizing antibodies that transcend different strains, PfRh5-based vaccines have shown promise as vaccine immunogens (149).
Genome-sequence data is used to do the following: determine pathogen strain diversity; identify virulence factors; select conserved regions; construct vectors; create recombinant proteins, attenuate vaccine strains (149–153); and create nucleic acid-based vaccines (154, 155), which contain specific gene sequences necessary for the in vivo expression of selected antigens. Additionally, the identification of such virulence factors enables researchers to selectively remove regions of the pathogen genome and create safer, attenuated strains for use as live-attenuated vaccines. For example, bubonic plague is caused by Yersinia pestis and is one of the deadliest diseases known. A variety of killed, whole-cell vaccines have been available since before 1900, but none are currently licensed (156). A number of live, attenuated vaccines have been produced, but concerns regarding reversion to virulence have precluded their widespread use (156). Current efforts have focused on subunit vaccines, with the subunits (typically virulence factors such as the F1 and V proteins, although other such as NlpD, Caf1 have been used) (157) being identified through genomic approaches. These vaccines have several advantages, including increased safety profiles, rapid induction of protective immunity, and a requirement for fewer vaccine doses (158). Unfortunately, Caf1 deletion does not always prevent lethal infection (159), which suggests that it is not essential for virulence. Similarly, although it is widely assumed that LcrV antibodies are necessary for protection, some primate models indicate that this may not be true for pneumonic plague (160). Further work needs to be done to clarify these issues. Another example is Rift Valley fever virus. The Rift Valley fever non-structural protein NSs was identified as a component that could be removed from the Rift Valley fever veterinary vaccine in order to differentiate infected from vaccinated animals (161). Studies found that the NSs protein was a virulence factor and that removal of the protein increased animal survival from 50 to 95% (162). Virulence proteins can also be used as components of protein-based vaccines. Excellent examples of this are the diphtheria and tetanus toxoid vaccines that contain formaldehyde-detoxified toxins (163), which enable recipients to develop antibodies that recognize and neutralize the native toxins, thus eliminating the major cause of pathogenesis during infection. Sequencing studies have also identified the role of gene sequences in meningococcal antigen expression (164), have identified meningococcal genotypes associated with increase virulence or invasion (165, 166), and have provided insight into immune evasion mechanisms (167).
For a complex pathogen such as plasmodium, in which multiple life-cycle stages occur with very different genes (and proteins) expressed at each stage, it is important to identify the proper sets of immune targets for vaccine development. Genomic technologies have allowed investigators to “mine” the plasmodium genome for antigen discovery. In a recent study, investigators identified the UIS3 gene as essential for parasite development in the liver. UIS3-deficient sporozoites were created and found to infect hepatocytes but were unable to establish a blood-stage infection (168). Vaccination with these modified sporozoites could protect immunized animals from an infectious challenge. In another study, scientists identified genes preferentially expressed by parasites capable of infecting the placenta through the CSA receptor (169). Just like the UIS3 example, these genes may serve as useful targets for a vaccine against malaria in pregnant women. Fortunately, these approaches can also be applied to less complex pathogens. A similar microarray-analysis approach identified Neisseria serogroup B genes that were upregulated during infection and were subsequently demonstrated to encode proteins targeted by protective immune responses (170).
Barrier #4: New Vaccines and Vaccine Safety
Drivers for the use of genomics in vaccinology include not only the public health need for new vaccines, but also the need to ensure vaccine safety and the need to develop directed approaches to de-risk the costs and time involved in vaccine development. The recognition that human genetic diversity leads to variations in infectious disease expression, severity, and disease outcomes, as well as variations in vaccine response, means that immune responses to vaccines are, at some level, predictable (7). In 2007, we developed and published the immune response network theory, which stated that immune responses to a vaccine are the “cumulative result of non-random interactions with host genes, epigenetic phenomena, metagenomics and the microbiome, gene dominance, complementarity, epistasis, co-infections, and other factors occurring within the system as a whole” (7, 92). Critical to our understanding of how vaccines induce protective (or aberrant) immune responses are the ideas that such responses are not random (and hence can be predictable) and occur at the systems level (92). In turn, this led to the development of vaccinomics and systems vaccinology (7, 13, 171–178). This emerging paradigm is an approach that utilizes the tools and insights derived from systems biology; high-dimensional, high-throughput “omics” technologies; and genomics (7, 13, 59, 66, 79, 175, 179–183). Vaccinomics leverages high-resolution data, such as transcriptomics, proteomics, and metabolomics/lipidomics/glycomics, epigenetics, etc., to derive holistic (systems-level) and mechanistic models of both protective and aberrant immune system responses (i.e., “immune signatures”). Such high-dimensional data are utilized in a new, directed four-step vaccine-development paradigm we have described as, “discover, characterize, validate and apply” (13). The idea is to discover new vaccine targets through the use of genomic technologies, characterize these targets in terms of their ability to generate protective immune responses, validate the findings in genetically diverse populations, and apply such findings to new vaccine development and vaccine safety studies.
The increased public scrutiny of vaccine safety has led to several large-scale initiatives designed to enhance our understanding of what drives adverse events after vaccination. One such effort, the BIoVacSafe Project (http://www.biovacsafe.eu/), began in 2012 with an overall goal to improve vaccine safety monitoring and understand what drives adverse reactions to vaccines. The effort had several objectives: (1) to understand early inflammatory responses after vaccination; (2) to develop biomarkers of autoimmunity; and (3) to capture the incidence of autoimmune disease in the population in order to identify those at higher risk of severe adverse events such as anaphylactic shock. A key to this endeavor has been the use of high-dimensional systems vaccinology approaches (184).
Conventional vaccines to prevent infectious diseases typically consist of killed or attenuated pathogens or of proteins from those microorganisms. In contrast, new vaccines being developed, which are poised to make major inroads in medicine, take advantage of genomic technologies to understand which host genes are activated/silenced, which host proteins or metabolites are involved, and what leads to a long duration (durability) of the immune response in vaccinated individuals (O'Connor et al., 119). The second genomic revolution in the vaccine field has to do with the vaccine constructs themselves. Specifically, genomic universal influenza virus vaccines can take the form of DNA or RNA that encode desired hemagglutinins or domains thereof. On administration, the genes enter cells, which then produce the proteins/components of proteins of interest. Compared with manufacturing proteins in cell cultures or whole viruses in embryonated eggs, producing just DNA/RNA is possibly simpler and less expensive. The latter approach is also amenable to making combinations of different epitopes and antigens for complex novel influenza virus vaccines. Checkpoint inhibitors may be used to enhance the immune responses of immunosubdominant epitopes. Finally, genomic vaccines may express antibodies for passive immunization instead of antigens to allow for rapid protection in the case of an emerging pandemic.
ADITEC Project (https://www.aditecproject.eu/) is a European initiative to organize the use of systems biology, adjuvant discovery, immunization routes, novel vaccine vectors and formulations, information about host factors, and results from animal models in order to develop novel immunization technologies and drive vaccine discovery. This consortium has published dozens of papers every year since its inception in 2011 and holds seminars and advanced courses in fields related to vaccinology. This project has resulted in nearly three dozen new immunization technologies, over 20 new animal and in vitro models being developed, multiple patents, and at least a dozen clinical trials. In the United States, the Human Vaccines Project is using systems biology, artificial intelligence (AI), and cutting-edge technologies to understand how the immune system functions and responds to vaccines (44). Addressing this fundamental gap in our knowledge will enable us to decode the human immune system, develop predictive markers of vaccine response, and create AI models of the immune system. These and other similar initiatives demonstrate the power of sustained collaborative partnerships between academia, industry, and governmental agencies. Increasingly, sophisticated computational modeling and machine learning approaches will be leveraged to understand immune function (185), identify optimal epitopes (186), as well as design and test new vaccines (187–189).
While the idea of personalized medicine is making progress, very little is known why some humans are more resistant to a pathogen and others are more susceptible. Combined with a better understanding of who responds well to a particular vaccine, this knowledge will be crucial to provide adequate protection and to design novel vaccines/gene sequences for an individual.
Vaccine safety is also being addressed using genetic approaches—termed “adversomics”—using the tools of immunogenomics, systems biology, computational modeling, and bioinformatics in order to better understand both genetic and non-genetic drivers of aberrant vaccine responses at the molecular level (7, 171, 172, 176). This is similar to the use of “omic” technologies in the field of toxicology (190, 191).
Adversomics presupposes that vaccine adverse reactions and events are not random and are predetermined genetically and in other ways. Immune-mediated vaccine adverse events are the primary outcomes of interest for the field of adversomics (184, 192). New biologic understandings, and the necessity of preventing serious adverse vaccine events, are critical to enhancing and—in some population groups—restoring public trust in vaccine safety, and for creating new knowledge applied to developing new vaccines that are both safe and effective. The pathway to accomplishing these goals is to understand the genetic and molecular mechanisms that determine inter-individual variations in vaccine response and reactivity. In turn, mechanistic knowledge of underlying vaccine adverse events could allow the ability to predict serious adverse events, and to design new vaccines that reduce or even eliminate harmful vaccine-related reactions. This endeavor is likely to be complementary to a more individualized approach to vaccine practice.
Examples of the value of genomics in vaccine safety have been published. McKinney et al. identified an association between specific cytokines after smallpox vaccination and the development of fever (193). Stanley et al. identified the influence of specific SNP haplotypes in the IL-1A, IL4, and IL18 gene complex in the development of fever after smallpox vaccination (194). Feenstra and colleagues identified a variety of genes and SNPs [IFI44L, CD46, SCN1A, 2A, TMEM16 (ANO3)] in the etiology of fever and febrile seizures after MMR vaccination (195). We and others have published on the association of myopericarditis after smallpox vaccine (196–200). This has resulted in studies attempting to determine possible genetic associations (176, 201, 202).
Barrier #5: Non-heritable Factors (e.g., Environment and Geography)
In addition to host genetics, non-heritable or environmental factors (e.g., pathogenic and symbiotic microorganisms, infections, diet, smoking, geographic, and other factors) play a role in shaping biological post-vaccination responses; however, the contribution of environmental factors to vaccine-induced immune responses is less understood (93). It is possible that inter-individual variation in immune responses induced by environmental factors would be significant in shaping adaptive post-vaccination responses. As an example, by using a systems vaccinology approach to assess immune responses stimulated by trivalent inactivated influenza vaccination (TIV), the gene expression of TLR5 at day 3 after vaccination was found to correlate with influenza vaccination response (HAI titers) 28 days after vaccination (203). While TLR5 mediates the sensing of flagellin on bacteria, it has been shown that it is also necessary to generate B cell responses and Ab production to viral vaccines (e.g., inactivated influenza and inactivated polio vaccines) (203, 204). Vaccination of TLR5−/− mice with TIV has caused a substantial reduction in Ab levels and frequencies of short-lived plasma cells confirming the gut microbiota can influence the heterogeneity in vaccine responses. Hence, there is close interaction among the components of the human immune system and the host microbiota, and this interface may influence vaccine-induced immune response and affect vaccine efficacy. Such findings require systems-level omics technologies to dissect the contributions and inter-relationships between multiple factors.
The effect of genetic contribution (heritability) on vaccine-induced immunity has mainly been estimated through monozygotic and dizygotic twin studies, which provided an approach to control for common environmental factors. Most of these studies have found that immune responses to many vaccines are heritable (205–207). For example, the estimated heritability for anti-HBs Ab concentrations after receipt of hepatitis B vaccine ranged between 61 and 91% in different studies (205, 206, 208). With respect to MMR vaccinations, the estimated heritability for Ab responses to measles, mumps and rubella virus vaccines has been found to be 88.5, 38.8, and 45.7%, respectively (209). Using the frequency of the human immune cell repertoire by FACS, a large genetic study of 1,629 individuals (14–102 years old) from Sardinia, Italy, found many cell populations (that are positive for the CD93 marker) with very high heritability (>60%), including Tregs and their subsets (mean 55%) (210). Thus, circulating immune-cell phenotypes may have measurable heritable components. In contrast, a recent systems-level influenza-vaccine twin study by Brodin et al. used 210 healthy twins (8–82 years old) to examine 204 different parameters of the immune system, and immune response outcomes found that non-heritable factors had a greater influence than heritable factors (211). Given that many earlier vaccine investigations in twins have studied infants and young children, the authors proposed that “many if not most of the less heritable traits that we measured in our mostly adult population may be much more heritable if measured in young children” (211). Similar environments may thus falsely suggest heritable traits in vaccinations of twins. It was suggested that variation in the human immune response increases with age and is driven by non-heritable factors, such as frequent environmental contact with various pathogens (e.g., CMV, influenza) and microbes. This hypothesis illustrates one of the challenges in translating findings from genetic studies (e.g., genetic variants that underline heritable immune response traits) to new vaccine development without accounting for continuously changing, non-heritable influences.
Conclusions and Perspective
Vaccine development in the twenty-first century is enabled by increasingly sophisticated genetic and high-dimensional assays, aided by bioinformatics approaches (212–214). This has allowed unprecedented resolution, at the whole-systems level, of how innate, adaptive, and cellular immune responses are generated, interact, and are maintained after vaccination. These technologies are being further leveraged in understanding adverse (aberrant) vaccine responses and the durability of immunity to vaccines, which represent areas of intense investigation due to their importance to human health. Taken together, genetic technologies and approaches have led to a new era of genetic design of vaccines and have provided solutions to the barriers currently impeding progress in this area (Table 1).
These novel approaches have been driven by public health urgency, demand for vaccine safety, cost considerations, and the inability of past vaccine-development paradigms to lead to viable vaccine candidates against complex and hyper-variable pathogens quickly enough to meet public health needs at an affordable cost. As a result, vaccine development is being accelerated by genetic and bioinformatics approaches (186). In the last decade, new vaccines against influenza have been developed and licensed, as have vaccines against meningococcus group B, hepatitis B, and herpes zoster using genomics-based approaches. Many more vaccines are in development.
Genetic approaches have enabled the identification of relationships/networks between individual genetic variants and specific aspects of vaccine-induced innate, adaptive, or cellular immune responses. The promise of vaccinomics is to identify specific immune response profiles that may serve as signatures or biomarkers that accurately predict vaccine immunogenicity, efficacy, and/or safety. Furthermore, it has the potential to identify genetic variants or antigens that lead to newer and safer vaccine candidates. We believe that the development of very large and detailed genotype:phenotype databases will eventually lead to a new model of personalized vaccine practice (i.e., the delivery of the right vaccine to the right person at the right time) that utilizes genetic and immune signatures to do the following: develop new vaccine candidates; predict the need for a vaccine and the dose needed to induce protective immunity; and to predict whether a significant adverse effect is likely to occur—in other words, personalized vaccinology.
Yet, barriers remain. Issues of high costs for genetic-based assays, including the cost of analysis and the complexity of such data exist, as well as inertia on the part of current vaccine developers conspiring to delay the full use of these rapidly advancing new paradigms. Funders of research must realize not only the promise of such vaccine development approaches but also the costs. For example, the standard allowable budget for the most common NIH research funding mechanism in the USA, the R01, has not changed in the past 30 years despite massive advances in science and the cost of experiments and statistical analysis over this time period.
Infectious diseases have always been—and always will be—a threat to human health. An excellent example of this is the current COVID-19 pandemic. This demonstrates how easily and repeatedly pathogens can emerge and affect humanity on a global scale. We had ample warning that novel coronaviruses can and do jump species and cause widespread and serious disease in humans. Our efforts to create vaccines against SARS and MERS resulted in products that reached clinical trials but no licensed vaccines. Fortunately, what we learned from those outbreaks has been rapidly applied to the SARS-CoV2 and we have seen clinical trials begin within 5 months of the first reported cases. This is a tremendous achievement. We have no choice but to continue to accelerate our ability to protect ourselves against pathogens that harm and kill. We are poised to do so, and the future is bright. Novel tools and paradigms allow highly directed study at levels of genetics and biology unimaginable just a handful of years ago. An example is that of the CRISPR/Cas9 technology that is revolutionizing genome editing of cells and pathogens; this technology has been used to excise virulence genes and create Pseudorabies virus vaccines (215) and to create duck enteritis virus (DEV) recombinants expressing avian influenza (highly pathogenic H5N1) and duck tembusu virus (DTMUV) antigens. The resulting trivalent vaccine elicits protection against all three duck pathogens (216).
Perhaps Albert Camus said it best in his book The Plague:
Everybody knows that pestilences have a way of recurring in the world; yet somehow we find it hard to believe in ones that crash down on our heads from a blue sky. There have been as many plagues as wars in history; yet always plagues and wars take people equally by surprise (217).
Author Contributions
RK, IO, and GP conceived of the topic. RK drafted the manuscript and organized the figures and tables. IO, PP, and GP revised the manuscript. RK, IO, PP, and GP approved the final manuscript. All authors contributed to the article and approved the submitted version.
Funding
Research reported in this publication was supported by the National Institute of Allergy and Infectious Diseases of the National Institutes of Health under award numbers U01AI089859, R01AI132348, R37AI048793, R01AI033144, R01AI121054, R01AI138965, R01AI127365, 75N93019C00052 (NIAID), R01AI145870, 75N93019C0051, P01AI097092, and 75D30119C06088 (Centers for Disease Control and Prevention). The content is solely the responsibility of the authors and does not necessarily represent the official views of the National Institutes of Health.
Conflict of Interest
GP is the chair of a Safety Evaluation Committee for novel investigational vaccine trials being conducted by Merck Research Laboratories. GP offers consultative advice on vaccine development to Merck & Co. Inc., Avianax, Valneva, Medicago, Sanofi Pasteur, GlaxoSmithKline, Emergent Biosolutions, and Dynavax. GP and IO hold four patents related to vaccinia and measles peptide research. RK has received funding from Merck Research Laboratories to study waning immunity to mumps vaccine. GP, RK, and IO have received grant funding from ICW Ventures for preclinical studies on a peptide-based COVID-19 vaccine. These activities have been reviewed by the Mayo Clinic Conflict of Interest Review Board and are conducted in compliance with Mayo Clinic Conflict of Interest policies. The Icahn School of Medicine has filed patents on the development of universal influenza virus vaccines (PP).
Acknowledgments
We thank Caroline L. Vitse for editorial assistance.
References
1. MMWR CDC. Centers for Disease Control and Prevention (CDC). Ten great public health achievements–United States, 1900-1999. MMWR. (1999) 48:241–3.
2. Ozawa S, Portnoy A, Getaneh H, Clark S, Knoll M, Bishai D, et al. Modeling the economic burden of adult vaccine-preventable diseases in the United States. Health Affairs. (2016) 35:2124–32. doi: 10.1377/hlthaff.2016.0462
3. Lee LA, Franzel L, Atwell J, Datta SD, Friberg IK, Goldie SJ, et al. The estimated mortality impact of vaccinations forecast to be administered during 2011-2020 in 73 countries supported by the GAVI Alliance. Vaccine. (2013) 31(Suppl 2):B61–72. doi: 10.1016/j.vaccine.2012.11.035
4. Rolfes MA, Flannery B, Chung J, O'halloran A, Garg S, Belongia EA, et al. Effects of influenza vaccination in the United States during the 2017-2018 influenza season. Clin Infect Dis. (2019) 69:1845–53. doi: 10.1093/cid/ciz075
5. Perry RT, Murray JS, Gacic-Dobo M, Dabbagh A, Mulders MN, Strebel PM, et al. Progress toward regional measles elimination - worldwide, 2000-2014. MMWR Morb Mortal Weekly Rep. (2015) 64:1246–51. doi: 10.15585/mmwr.6444a4
7. Poland GA, Kennedy RB, Mckinney BA, Ovsyannikova IG, Lambert ND, Jacobson RM, et al. Vaccinomics, adversomics, and the immune response network theory: individualized vaccinology in the 21st century. Semin Immunol. (2013) 25:89–103. doi: 10.1016/j.smim.2013.04.007
8. Bragazzi NL, Gianfredi V, Villarini M, Rosselli R, Nasr A, Hussein A, et al. Vaccines meet big data: state-of-the-art and future prospects. From the classical 3Is (“isolate-inactivate-inject”) vaccinology 1.0 to vaccinology 3.0, vaccinomics, and beyond: a historical overview. Front Public Health. (2018) 6:62. doi: 10.3389/fpubh.2018.00062
9. Wallis J, Shenton DP, Carlisle RC. Novel approaches for the design, delivery and administration of vaccine technologies. Clin Exp Immunol. (2019) 196:189–204. doi: 10.1111/cei.13287
10. Halstead SB. Dengvaxia sensitizes seronegatives to vaccine enhanced disease regardless of age. Vaccine. (2017) 35:6355–8. doi: 10.1016/j.vaccine.2017.09.089
11. Rappuoli R. Reverse vaccinology. CurrOpinMicrobiol. (2000) 3:445–50. doi: 10.1016/S1369-5274(00)00119-3
12. Poland GA, Ovsyannikova IG, Jacobson RM. Vaccinomics and Personalized Vaccinology. The Jordan Report. U.S. Department of Health and Human Services, Washington, DC, United States. (2012).
13. Poland GA, Ovsyannikova IG, Kennedy RB. Personalized vaccinology: a review. Vaccine. (2017) 36:5350–7. doi: 10.1016/j.vaccine.2017.07.062
14. Gasparini R, Panatto D, Bragazzi NL, Lai PL, Bechini A, Levi M, et al. How the knowledge of interactions between meningococcus and the human immune system has been used to prepare effective Neisseria meningitidis vaccines. J Immunol Res. (2015) 2015:189153. doi: 10.1155/2015/189153
15. Maiden MCJ. The impact of nucleotide sequence analysis on meningococcal vaccine development and assessment. Front Immunol. (2018) 9:3151. doi: 10.3389/fimmu.2018.03151
16. Catalanotto C, Cogoni C, Zardo G. MicroRNA in control of gene expression: an overview of nuclear functions. Int J Mol Sci. (2016) 17:1712. doi: 10.3390/ijms17101712
17. Lopez-Pajares V. Long non-coding RNA regulation of gene expression during differentiation. Pflugers Arch. (2016) 468:971–81. doi: 10.1007/s00424-016-1809-6
18. Li X, Gong L, Gu H. Regulation of immune system development and function by Cbl-mediated ubiquitination. Immunol Rev. (2019) 291:123–33. doi: 10.1111/imr.12789
19. Chen J, Wang Y, Wang C, Hu JF, Li W. LncRNA functions as a new emerging epigenetic factor in determining the fate of stem cells. Front Genet. (2020) 11:277. doi: 10.3389/fgene.2020.00277
20. Niehrs C, Luke B. Regulatory R-loops as facilitators of gene expression and genome stability. Nat Rev Mol Cell Biol. (2020) 21:167–78. doi: 10.1038/s41580-019-0206-3
21. Noordermeer D, Feil R. Differential 3D chromatin organization and gene activity in genomic imprinting. Curr Opin Genet Dev. (2020) 61:17–24. doi: 10.1016/j.gde.2020.03.004
22. Stewart-Morgan KR, Petryk N, Groth A. Chromatin replication and epigenetic cell memory. Nat Cell Biol. (2020) 22:361–71. doi: 10.1038/s41556-020-0487-y
23. Giani AM, Gallo GR, Gianfranceschi L, Formenti G. Long walk to genomics: history and current approaches to genome sequencing and assembly. Comput Struct Biotechnol J. (2020) 18:9–19. doi: 10.1016/j.csbj.2019.11.002
24. Brodie TM, Tosevski V. Broad immune monitoring and profiling of T cell subsets with mass cytometry. Methods Mol Biol. (2018) 1745:67–82. doi: 10.1007/978-1-4939-7680-5_4
25. Reeves PM, Sluder AE, Paul SR, Scholzen A, Kashiwagi S, Poznansky MC. Application and utility of mass cytometry in vaccine development. FASEB J. (2018) 32:5–15. doi: 10.1096/fj.201700325r
26. Johnson KL, Ovsyannikova IG, Poland G, Muddiman DC. Identification of class II HLA-DRB1*03-bound measles virus peptides by 2D-liquid chromatography tandem mass spectrometry. J Proteome Res. (2005) 4:2243–9. doi: 10.1021/pr0501416
27. Johnson KL, Ovsyannikova IG, Mason CJ, Bergen HR 3rd, Poland GA. Discovery of naturally processed and HLA-presented class I peptides from vaccinia virus infection using mass spectrometry for vaccine development. Vaccine. (2009) 28:38–47. doi: 10.1016/j.vaccine.2009.09.126
28. Araiza-Villanueva M, Avila-Calderon ED, Flores-Romo L, Calderon-Amador J, Sriranganathan N, Qublan HA, et al. Proteomic analysis of membrane blebs of brucella abortus 2308 and rb51 and their evaluation as an acellular vaccine. Front Microbiol. (2019) 10:2714. doi: 10.3389/fmicb.2019.02714
29. Chen R, Fulton KM, Twine SM, Li J. Identification of Mhc peptides using mass spectrometry for neoantigen discovery and cancer vaccine development. Mass Spectrom Rev. (2019). doi: 10.1002/mas.21616. [Epub ahead of print].
30. Sarkizova S, Klaeger S, Le PM, Li LW, Oliveira G, Keshishian H, et al. A large peptidome dataset improves HLA class I epitope prediction across most of the human population. Nat Biotechnol. (2020) 38:199–209. doi: 10.1038/s41587-019-0322-9
31. Li S, Sullivan NL, Rouphael N, Yu T, Banton S, Maddur MS, et al. Metabolic phenotypes of response to vaccination in humans. Cell. (2017) 169:862–77 e817. doi: 10.1016/j.cell.2017.04.026
32. Manchester M, Anand A. Metabolomics: strategies to define the role of metabolism in virus infection and pathogenesis. Adv Virus Res. (2017) 98:57–81. doi: 10.1016/bs.aivir.2017.02.001
33. Hagan T, Cortese M, Rouphael N, Boudreau C, Linde C, Maddur MS, et al. Antibiotics-driven gut microbiome perturbation alters immunity to vaccines in humans. Cell. (2019) 178:1313–28 e1313. doi: 10.1016/j.cell.2019.08.010
34. Breuer K, Foroushani AK, Laird MR, Chen C, Sribnaia A, Lo R, et al. InnateDB: systems biology of innate immunity and beyond–recent updates and continuing curation. Nucleic Acids Res. (2013) 41:D1228–33. doi: 10.1093/nar/gks1147
35. Behbehani GK. Immunophenotyping by mass cytometry. Methods Mol Biol. (2019) 2032:31–51. doi: 10.1007/978-1-4939-9650-6_2
36. Mahajan S, Vita R, Shackelford D, Lane J, Schulten V, Zarebski L, et al. Epitope specific antibodies and t cell receptors in the immune epitope database. Front Immunol. (2018) 9:2688. doi: 10.3389/fimmu.2018.02688
37. Biancotto A, Mccoy JP. Studying the human immunome: the complexity of comprehensive leukocyte immunophenotyping. Curr Top Microbiol Immunol. (2014) 377:23–60. doi: 10.1007/82_2013_336
38. Bhattacharya S, Dunn P, Thomas CG, Smith B, Schaefer H, Chen J, et al. ImmPort, toward repurposing of open access immunological assay data for translational and clinical research. Sci Data. (2018) 5:180015. doi: 10.1038/sdata.2018.15
39. Hurley CK. Naming HLA diversity: a review of HLA nomenclature. Hum Immunol. (2020) S0198-8859(20)30065-3. doi: 10.1016/j.humimm.2020.03.005
40. What can immunologists learn from systems approaches? Trends Immunol. (2018) 39:163–6. doi: 10.1016/j.it.2018.01.002
41. How can systems biology test principles and tools using immune cells as a model? Cell Syst. (2018) 6:146–8. doi: 10.1016/j.cels.2018.02.005
42. Cady N, Peterson SR, Freedman SN, Mangalam AK. Beyond metabolism: the complex interplay between dietary phytoestrogens, gut bacteria, and cells of nervous and immune systems. Front Neurol. (2020) 11:150. doi: 10.3389/fneur.2020.00150
43. Harandi AM. Systems analysis of human vaccine adjuvants. Semin Immunol. (2018) 39:30–4. doi: 10.1016/j.smim.2018.08.001
44. Wooden SL, Koff WC. The human vaccines project: towards a comprehensive understanding of the human immune response to immunization. Hum Vaccin Immunother. (2018) 14:2214–6. doi: 10.1080/21645515.2018.1476813
45. Singer M, Anderson AC. Revolutionizing cancer immunology: the power of next-generation sequencing technologies. Cancer Immunol Res. (2019) 7:168–73. doi: 10.1158/2326-6066.CIR-18-0281
46. Minervini CF, Cumbo C, Orsini P, Anelli L, Zagaria A, Specchia G, et al. Nanopore sequencing in blood diseases: a wide range of opportunities. Front Genet. (2020) 11:76. doi: 10.3389/fgene.2020.00076
47. Hasin Y, Seldin M, Lusis A. Multi-omics approaches to disease. Genome Biol. (2017) 18:83. doi: 10.1186/s13059-017-1215-1
48. Weiner J 3rd, Mohney RP, Kaufmann SHE. The potential of metabolic profiling for vaccine development. Semin Immunol. (2018) 39:44–51. doi: 10.1016/j.smim.2018.07.002
49. Scarno G, Pietropaolo G, Di Censo C, Gadina M, Santoni A, Sciume G. Transcriptional, epigenetic and pharmacological control of JAK/STAT pathway in NK cells. Front Immunol. (2019) 10:2456. doi: 10.3389/fimmu.2019.02456
50. Baharlou H, Canete NP, Cunningham AL, Harman AN, Patrick E. Mass cytometry imaging for the study of human diseases-applications and data analysis strategies. Front Immunol. (2019) 10:2657. doi: 10.3389/fimmu.2019.02657
51. Hartmann FJ, Bendall SC. Immune monitoring using mass cytometry and related high-dimensional imaging approaches. Nat Rev Rheumatol. (2020) 16:87–99. doi: 10.1038/s41584-019-0338-z
52. Lun XK, Szklarczyk D, Gabor A, Dobberstein N, Zanotelli VRT, Saez-Rodriguez J, et al. Analysis of the human kinome and phosphatome by mass cytometry reveals overexpression-induced effects on cancer-related signaling. Mol Cell. (2019) 74:1086–102 e1085. doi: 10.1016/j.molcel.2019.04.021
53. Claassen M. Shooting movies of signaling network dynamics with multiparametric cytometry. Curr Top Microbiol Immunol. (2014) 377:177–89. doi: 10.1007/82_2013_350
54. Sen N, Arvin AM. Dissecting the molecular mechanisms of the tropism of varicella-zoster virus for human T cells. J Virol. (2016) 90:3284–7. doi: 10.1128/JVI.03375-14
55. Schulz C, Hammerschmidt S. Exploitation of physiology and metabolomics to identify pneumococcal vaccine candidates. Expert Rev Vaccines. (2013) 12:1061–75. doi: 10.1586/14760584.2013.824708
56. De Figueiredo P, Ficht TA, Rice-Ficht A, Rossetti CA, Adams LG. Pathogenesis and immunobiology of brucellosis: review of Brucella-host interactions. Am J Pathol. (2015) 185:1505–17. doi: 10.1016/j.ajpath.2015.03.003
57. Davey L, Valdivia RH. Bacterial genetics and molecular pathogenesis in the age of high throughput DNA sequencing. Curr Opin Microbiol. (2020) 54:59–66. doi: 10.1016/j.mib.2020.01.007
58. Valli RXE, Lyng M, Kirkpatrick CL. There is no hiding if you Seq: recent breakthroughs in Pseudomonas aeruginosa research revealed by genomic and transcriptomic next-generation sequencing. J Med Microbiol. (2020) 69:162–75. doi: 10.1099/jmm.0.001135
59. Querec TD, Akondy RS, Lee EK, Cao W, Nakaya HI, Teuwen D, et al. Systems biology approach predicts immunogenicity of the yellow fever vaccine in humans. Nat Immunol. (2009) 10:116–25. doi: 10.1038/ni.1688
60. Dunachie S, Berthoud T, Hill AV, Fletcher HA. Transcriptional changes induced by candidate malaria vaccines and correlation with protection against malaria in a human challenge model. Vaccine. (2015) 33:5321–31. doi: 10.1016/j.vaccine.2015.07.087
61. Bordignon J, Probst CM, Mosimann AL, Pavoni DP, Stella V, Buck GA, et al. Expression profile of interferon stimulated genes in central nervous system of mice infected with dengue virus Type-1. Virology. (2008) 377:319–29. doi: 10.1016/j.virol.2008.04.033
62. Sun P, Garcia J, Comach G, Vahey MT, Wang Z, Forshey BM, et al. Sequential waves of gene expression in patients with clinically defined dengue illnesses reveal subtle disease phases and predict disease severity. PLoS Negl Trop Dis. (2013) 7:e2298. doi: 10.1371/journal.pntd.0002298
63. Yen JY, Garamszegi S, Geisbert JB, Rubins KH, Geisbert TW, Honko A, et al. Therapeutics of Ebola hemorrhagic fever: whole-genome transcriptional analysis of successful disease mitigation. J Infect Dis. (2011) 204(Suppl 3):S1043–52. doi: 10.1093/infdis/jir345
64. Van Den Berg RA, Coccia M, Ballou WR, Kester KE, Ockenhouse CF, Vekemans J, et al. Predicting RTS,S vaccine-mediated protection from transcriptomes in a malaria-challenge clinical trial. Front Immunol. (2017) 8:557. doi: 10.3389/fimmu.2017.00557
65. Rao S, Ghosh D, Asturias EJ, Weinberg A. What can we learn about influenza infection and vaccination from transcriptomics? Hum Vaccin Immunother. (2019) 15:1–9. doi: 10.1080/21645515.2019.1608744
66. Li S, Rouphael N, Duraisingham S, Romero-Steiner S, Presnell S, Davis C, et al. Molecular signatures of antibody responses derived from a systems biology study of five human vaccines. Nat Immunol. (2014) 15:195–204. doi: 10.1038/ni.2789
67. Nakaya HI, Clutterbuck E, Kazmin D, Wang L, Cortese M, Bosinger SE, et al. Systems biology of immunity to MF59-adjuvanted versus nonadjuvanted trivalent seasonal influenza vaccines in early childhood. Proc Natl Acad Sci USA. (2016) 113:1853–8. doi: 10.1073/pnas.1519690113
68. Ovsyannikova IG, Salk HM, Kennedy RB, Haralambieva IH, Zimmermann MT, Grill DE, et al. Gene signatures associated with adaptive humoral immunity following seasonal influenza A/H1N1 vaccination. Genes Immun.(2016) 17:371–9. doi: 10.1038/gene.2016.34
69. Kazmin D, Nakaya HI, Lee EK, Johnson MJ, Van Der Most R, Van Den Berg RA, et al. Systems analysis of protective immune responses to RTS,S malaria vaccination in humans. Proc Natl Acad Sci USA. (2017) 114:2425–30. doi: 10.1073/pnas.1621489114
70. Zimmermann MT, Kennedy RB, Grill DE, Oberg AL, Goergen KM, Ovsyannikova IG, et al. Integration of immune cell populations, mRNA-Seq, and CpG methylation to better predict humoral immunity to influenza vaccination: dependence of mRNA-Seq/CpG methylation on immune cell populations. Front Immunol. (2017) 8:445. doi: 10.3389/fimmu.2017.00445
71. A two-dose hepatitis B vaccine for adults (Heplisav-B). JAMA. (2018) 319:822–3. doi: 10.1001/jama.2018.1097
73. Khurana S, Verma N, Yewdell JW, Hilbert AK, Castellino F, Lattanzi M, et al. MF59 adjuvant enhances diversity and affinity of antibody-mediated immune response to pandemic influenza vaccines. Sci Transl Med. (2011) 3:85ra48. doi: 10.1126/scitranslmed.3002336
74. Scheifele DW, Mcneil SA, Ward BJ, Dionne M, Cooper C, Coleman B, et al. Safety, immunogenicity, and tolerability of three influenza vaccines in older adults: results of a randomized, controlled comparison. Hum Vaccines Immunother. (2013) 9:2460–73. doi: 10.4161/hv.25580
75. Frey SE, Reyes MR, Reynales H, Bermal NN, Nicolay U, Narasimhan V, et al. Comparison of the safety and immunogenicity of an MF59(R)-adjuvanted with a non-adjuvanted seasonal influenza vaccine in elderly subjects. Vaccine. (2014) 32:5027–34. doi: 10.1016/j.vaccine.2014.07.013
76. Sullivan NL, Eberhardt CS, Wieland A, Vora KA, Pulendran B, Ahmed R. Understanding the immunology of the Zostavax shingles vaccine. Curr Opin Immunol. (2019) 59:25–30. doi: 10.1016/j.coi.2019.02.005
77. Weinberg A, Pang L, Johnson MJ, Caldas Y, Cho A, Tovar-Salazar A, et al. The effect of age on the immunogenicity of the live attenuated zoster vaccine is predicted by baseline regulatory T cells and VZV-specific T cell immunity. J Virol. (2019) 93:e00305-19. doi: 10.1128/JVI.00305-19
78. Lambert ND, Ovsyannikova IG, Pankratz VS, Jacobson RM, Poland GA. Understanding the immune response to seasonal influenza vaccination in older adults: a systems biology approach. Expert Rev Vaccines. (2012) 11:985–94. doi: 10.1586/erv.12.61
79. Haralambieva IH, Kennedy RB, Ovsyannikova IG, Whitaker JA, Poland GA. Variability in humoral immunity to measles vaccine: new developments. Trends Mol Med. (2015) 21:789–801. doi: 10.1016/j.molmed.2015.10.005
80. Fiebelkorn AP, Coleman LA, Belongia EA, Freeman SK, York D, Bi D, et al. Measles virus neutralizing antibody response, cell-mediated immunity, and immunoglobulin G antibody avidity before and after receipt of a third dose of measles, mumps, and rubella vaccine in young adults. J Infect Dis. (2016) 213:1115–23. doi: 10.1093/infdis/jiv555
81. Antia A, Ahmed H, Handel A, Carlson NE, Amanna IJ, Antia R, et al. Heterogeneity and longevity of antibody memory to viruses and vaccines. PLoS Biol. (2018) 16:e2006601. doi: 10.1371/journal.pbio.2006601
82. Haralambieva IH, Kennedy RB, Simon WL, Goergen KM, Grill DE, Ovsyannikova IG, et al. Differential miRNA expression in B cells is associated with inter-individual differences in humoral immune response to measles vaccination. PLoS ONE. (2018) 13:e0191812. doi: 10.1371/journal.pone.0191812
83. Crooke SN, Haralambieva IH, Grill DE, Ovsyannikova IG, Kennedy RB, Poland GA. Seroprevalence and durability of rubella virus antibodies in a highly immunized population. Vaccine. (2019) 37:3876–82. doi: 10.1016/j.vaccine.2019.05.049
84. Kennedy RB, Ovsyannikova IG, Thomas A, Larrabee BR, Rubin S, Poland GA. Differential durability of immune responses to measles and mumps following MMR vaccination. Vaccine. (2019) 37:1775–84. doi: 10.1016/j.vaccine.2019.02.030
85. Anasir MI, Poh CL. Structural vaccinology for viral vaccine design. Front Microbiol. (2019) 10:738. doi: 10.3389/fmicb.2019.00738
86. Graham BS, Gilman MSA, Mclellan JS. Structure-based vaccine antigen design. Annu Rev Med. (2019) 70:91–104. doi: 10.1146/annurev-med-121217-094234
87. Pierce BG, Boucher EN, Piepenbrink KH, Ejemel M, Rapp CA, Thomas WD Jr, et al. Structure-based design of hepatitis C virus vaccines that elicit neutralizing antibody responses to a conserved epitope. J Virol. (2017) 91:e01032-17. doi: 10.1128/JVI.01032-17
88. Crank MC, Ruckwardt TJ, Chen M, Morabito KM, Phung E, Costner PJ, et al. A proof of concept for structure-based vaccine design targeting RSV in humans. Science. (2019) 365:505–9. doi: 10.1126/science.aav9033
89. Sesterhenn F, Bonet J, Correia BE. Structure-based immunogen design-leading the way to the new age of precision vaccines. Curr Opin Struct Biol. (2018) 51:163–9. doi: 10.1016/j.sbi.2018.06.002
90. Yan D, Wei YQ, Guo HC, Sun SQ. The application of virus-like particles as vaccines and biological vehicles. Appl Microbiol Biotechnol. (2015) 99:10415–32. doi: 10.1007/s00253-015-7000-8
91. Charlton Hume HK, Vidigal J, Carrondo MJT, Middelberg APJ, Roldao A, Lua LHL. Synthetic biology for bioengineering virus-like particle vaccines. Biotechnol Bioeng. (2019) 116:919–35. doi: 10.1002/bit.26890
92. Poland GA, Ovsyannikova IG, Jacobson RM, Smith DI. Heterogeneity in vaccine immune response: the role of immunogenetics and the emerging field of vaccinomics. Clin PharmacolTher. (2007) 82:653–64. doi: 10.1038/sj.clpt.6100415
93. Brodin P, Davis MM. Human immune system variation. Nat Rev Immunol. (2017) 17:21–9. doi: 10.1038/nri.2016.125
95. Poland GA. Pharmacology, vaccinomics, and the second golden age of vaccinology. Clin PharmacolTher. (2007) 82:623–6. doi: 10.1038/sj.clpt.6100379
96. Ovsyannikova IG, Haralambieva IH, Vierkant RA, O'byrne MM, Jacobson RM, Poland GA. Effects of vitamin A and D receptor gene polymorphisms/haplotypes on immune responses to measles vaccine. Pharmacogenet Genomics. (2012) 22:20–31. doi: 10.1097/FPC.0b013e32834df186
97. Grzegorzewska AE, Jodlowska E, Mostowska A, Sowinska A, Jagodzinski PP. Single nucleotide polymorphisms of vitamin D binding protein, vitamin D receptor and retinoid X receptor alpha genes and response to hepatitis B vaccination in renal replacement therapy patients. Expert Rev Vaccines. (2014) 13:1–9. doi: 10.1586/14760584.2014.962521
98. Mckinney BA, Lareau C, Oberg AL, Kennedy RB, Ovsyannikova IG, Poland GA. The integration of epistasis network and functional interactions in a GWAS implicates RXR pathway genes in the immune response to smallpox vaccine. PLoS ONE. (2016) 11:e0158016. doi: 10.1371/journal.pone.0158016
99. Paltoo DN, Laura RL, Feolo M, Gillanders E, Ramos EM, Rutter JL, et al. Data use under the NIH GWAS data sharing policy and future directions. Nat Genet. (2014) 46:934–8. doi: 10.1038/ng.3062
100. Png E, Thalamuthu A, Ong RT, Snippe H, Boland GJ, Seielstad M. A genome-wide association study of hepatitis B vaccine response in an Indonesian population reveals multiple independent risk variants in the HLA region. Hum Mol Genet. (2011) 20:3893–8. doi: 10.1093/hmg/ddr302
101. Ovsyannikova IG, Kennedy RB, O'byrne M, Jacobson RM, Pankratz VS, Poland GA. Genome-wide association study of antibody response to smallpox vaccine. Vaccine. (2012) 30:4182–9. doi: 10.1016/j.vaccine.2012.04.055
102. Pajewski NM, Shrestha S, Quinn CP, Parker SD, Wiener H, Aissani B, et al. A genome-wide association study of host genetic determinants of the antibody response to anthrax vaccine adsorbed. Vaccine. (2012) 30:4778–84. doi: 10.1016/j.vaccine.2012.05.032
103. Franco LM, Bucasas KL, Wells JM, Nino D, Wang X, Zapata GE, et al. Integrative genomic analysis of the human immune response to influenza vaccination. eLife. (2013) 2:e00299. doi: 10.7554/eLife.00299
104. Kennedy RB, Ovsyannikova IG, Haralambieva IH, Lambert ND, Pankratz VS, Poland GA. Genome-wide SNP associations with rubella-specific cytokine responses in measles-mumps-rubella vaccine recipients. Immunogenetics. (2014) 66:493–9. doi: 10.1007/s00251-014-0776-3
105. Pan L, Zhang L, Zhang W, Wu X, Li Y, Yan B, et al. A genome-wide association study identifies polymorphisms in the HLA-DR region associated with non-response to hepatitis B vaccination in Chinese Han populations. Hum Mol Genet. (2014) 23:2210–9. doi: 10.1093/hmg/ddt586
106. Lambert ND, Haralambieva IH, Kennedy RB, Ovsyannikova IG, Pankrantz VS, Poland GA. Polymorphisms in HLA-DPB1 are associated with differences in rubella-specific humoral immunity after vaccination. J Infect Dis. (2015) 211:898–905. doi: 10.1093/infdis/jiu553
107. Haralambieva IH, Ovsyannikova IG, Kennedy RB, Larrabee BR, Zimmermann MT, Grill DE, et al. Genome-wide associations of CD46 and IFI44L genetic variants with neutralizing antibody response to measles vaccine. Human Genet. (2017) 136:421–35. doi: 10.1007/s00439-017-1768-9
108. Ovsyannikova IG, Haralambieva IH, Vierkant RA, O'byrne MM, Jacobson RM, Poland GA. The association of CD46, SLAM, and CD209 cellular receptor gene SNPs with variations in measles vaccine-induced immune responses–a replication study and examination of novel polymorphisms. Human Heredity. (2011) 72:206–23. doi: 10.1159/000331585
109. Ovsyannikova IG, Jacobson RM, Vierkant RA, O'byrne MM, Poland GA. Replication of rubella vaccine population genetic studies: validation of HLA genotype and humoral response associations. Vaccine. (2009) 27:6926–31. doi: 10.1016/j.vaccine.2009.08.109
110. Ovsyannikova IG, Pankratz VS, Larrabee BR, Jacobson RM, Poland GA. HLA genotypes and rubella vaccine immune response: additional evidence. Vaccine. (2014) 32:4206–13. doi: 10.1016/j.vaccine.2014.04.091
111. Desombere I, Willems A, Leroux-Roels G. Response to hepatitis B vaccine: multiple HLA genes are involved. Tissue Antigens. (1998) 51:593–604. doi: 10.1111/j.1399-0039.1998.tb03001.x
112. Mcdermott AB, Cohen SBA, Zuckerman JN, Madrigal JA. Human leukocyte antigens influence the immune response to a pre-S/S hepatitis B vaccine. Vaccine. (1999) 17:330–9. doi: 10.1016/S0264-410X(98)00203-5
113. Gelder CM, Lambkin R, Hart KW, Fleming D, Williams OM, Bunce M, et al. Associations between human leukocyte antigens and nonresponsiveness to influenza vaccine. J Infect Dis. (2002) 185:114–7. doi: 10.1086/338014
114. Ovsyannikova IG, Pankratz VS, Vierkant RA, Jacobson RM, Poland GA. Human leukocyte antigen haplotypes in the genetic control of immune response to measles-mumps-rubella vaccine. J Infect Dis. (2006) 193:655–63. doi: 10.1086/500144
115. Ovsyannikova IG, Pankratz VS, Vierkant RA, Jacobson RM, Poland GA. Consistency of HLA associations between two independent measles vaccine cohorts: a replication study. Vaccine. (2012) 30:2146–52. doi: 10.1016/j.vaccine.2012.01.038
116. Ovsyannikova IG, Pankratz VS, Salk HM, Kennedy RB, Poland GA. HLA alleles associated with the adaptive immune response to smallpox vaccine: a replication study. Human Genet. (2014) 133:1083–92. doi: 10.1007/s00439-014-1449-x
117. Ovsyannikova IG, Johnson KL, Bergen HR 2nd, Poland GA. Mass spectrometry and peptide-based vaccine development. Clin Pharmacol. Ther. (2007) 82:644–52. doi: 10.1038/sj.clpt.6100389
118. Hunt DF, Henderson RA, Shabanowitz J, Sakaguchi K, Michel H, Sevilir N, et al. Pillars article: characterization of peptides bound to the class I MHC molecule HLA-A2.1 by mass spectrometry. Science 1992. 255: 1261-1263. Science. J Immunol. (2007) 179:2669–71. doi: 10.1126/science.1546328
119. Yucesoy B, Talzhanov Y, Johnson VJ, Wilson NW, Biagini RE, Wang W, et al. Genetic variants within the MHC region are associated with immune responsiveness to childhood vaccinations. Vaccine. (2013) 31:5381–91. doi: 10.1016/j.vaccine.2013.09.026
120. O'connor D, Png E, Khor CC, Snape MD, Hill AVS, Van Der Klis F, et al. Common genetic variations associated with the persistence of immunity following childhood immunization. Cell Rep. (2019) 27:3241–53 e3244. doi: 10.1016/j.celrep.2019.05.053
121. Pankratz VS, Vierkant RA, O'byrne MM, Ovsyannikova IG, Poland GA. Associations between SNPs in candidate immune-relevant genes and rubella antibody levels: a multigenic assessment. BMC Immunol. (2010) 11:48. doi: 10.1186/1471-2172-11-48
122. Kennedy RB, Ovsyannikova IG, Haralambieva IH, O'byrne MM, Jacobson RM, Pankratz VS, et al. Multigenic control of measles vaccine immunity mediated by polymorphisms in measles receptor, innate pathway, and cytokine genes. Vaccine. (2012) 30:2159–67. doi: 10.1016/j.vaccine.2012.01.025
123. Ovsyannikova IG, Dhiman N, Haralambieva IH, Vierkant RA, O'byrne MM, Jacobson RM, et al. Rubella vaccine-induced cellular immunity: evidence of associations with polymorphisms in the Toll-like, vitamin A and D receptors, and innate immune response genes. Human Genet. (2010) 127:207–21. doi: 10.1007/s00439-009-0763-1
124. Ovsyannikova IG, Haralambieva IH, Kennedy RB, O'byrne MM, Pankratz VS, Poland GA. Genetic variation in IL18R1 and IL18 genes and inteferon gamma ELISPOT response to smallpox vaccination: an unexpected relationship. J Infect Dis. (2013) 208:1422–30. doi: 10.1093/infdis/jit341
125. Ovsyannikova IG, Pankratz VS, Vierkant RA, Pajewski NM, Quinn CP, Kaslow RA, et al. Human leukocyte antigens and cellular immune responses to anthrax vaccine adsorbed. Infect Immun. (2013) 81:2584–91. doi: 10.1128/IAI.00269-13
126. Kennedy RB, Ovsyannikova IG, Haralambieva IH, Lambert ND, Pankratz VS, Poland GA. Genetic polymorphisms associated with rubella virus-specific cellular immunity following MMR vaccination. Human Genet. (2014) 133:1407–17. doi: 10.1007/s00439-014-1471-z
127. Ashraf S, Nitschke K, Warshow UM, Brooks CR, Kim AY, Lauer GM, et al. Synergism of tapasin and human leukocyte antigens in resolving hepatitis C virus infection. Hepatology. (2013) 58:881–9. doi: 10.1002/hep.26415
128. Williams AP, Bevan S, Bunce M, Houlston R, Welsh KI, Elliott T. Identification of novel Tapasin polymorphisms and linkage disequilibrium to MHC class I alleles. Immunogenetics. (2000) 52:9–11. doi: 10.1007/s002510000244
129. Linnik JE, Egli A. Impact of host genetic polymorphisms on vaccine induced antibody response. Hum Vaccin Immunother. (2016) 12:907–15. doi: 10.1080/21645515.2015.1119345
130. Mozzi A, Pontremoli C, Sironi M. Genetic susceptibility to infectious diseases: current status and future perspectives from genome-wide approaches. Infect Genet Evol. (2018) 66:286–307. doi: 10.1016/j.meegid.2017.09.028
131. Ovsyannikova IG, Jacobson RM, Vierkant RA, Pankratz VS, Poland GA. HLA supertypes and immune responses to measles-mumps-rubella viral vaccine: findings and implications for vaccine design. Vaccine. (2007) 25:3090–100. doi: 10.1016/j.vaccine.2007.01.020
132. Ovsyannikova IG, Vierkant RA, Pankratz VS, O'byrne MM, Jacobson RM, Poland GA. HLA haplotype and supertype associations with cellular immune responses and cytokine production in healthy children after rubella vaccine. Vaccine. (2009) 27:3349–58. doi: 10.1016/j.vaccine.2009.01.080
133. Dhiman N, Haralambieva IH, Kennedy RB, Vierkant RA, O'byrne MM, Ovsyannikova IG, et al. SNP/haplotype associations in cytokine and cytokine receptor genes and immunity to rubella vaccine. Immunogenetics. (2010) 62:197–210. doi: 10.1007/s00251-010-0423-6
134. Haralambieva IH, Dhiman N, Ovsyannikova IG, Vierkant RA, Pankratz VS, Jacobson RM, et al. 2′-5′-Oligoadenylate synthetase single-nucleotide polymorphisms and haplotypes are associated with variations in immune responses to rubella vaccine. HumImmunol. (2010) 71:383–91. doi: 10.1016/j.humimm.2010.01.004
135. Ovsyannikova IG, Haralambieva IH, Dhiman N, O'byrne MM, Pankratz VS, Jacobson RM, et al. Polymorphisms in the vitamin A receptor and innate immunity genes influence the antibody response to rubella vaccination. J Infect Dis. (2010) 201:207–13. doi: 10.1086/649588
136. Cifuente JO, Moratorio G. Evolutionary and structural overview of human picornavirus capsid antibody evasion. Front Cell Infect Microbiol. (2019) 9:283. doi: 10.3389/fcimb.2019.00283
137. Lapa D, Garbuglia AR, Capobianchi MR, Del Porto P. Hepatitis C virus genetic variability, human immune response, and genome polymorphisms: which is the interplay? Cells. (2019) 8:305. doi: 10.3390/cells8040305
138. Green LR, Al-Rubaiawi AA, Al-Maeni M, Harrison OB, Blades M, Oldfield NJ, et al. Localized hypermutation is the major driver of meningococcal genetic variability during persistent asymptomatic carriage. mBio. (2020) 11:e03068-19. doi: 10.1128/mBio.03068-19
139. Poland GA, Barry MA. Common cold, uncommon variation. N Engl J Med. (2009) 360:2245–6. doi: 10.1056/NEJMcibr0902053
140. Hanage WP, Finkelstein JA, Huang SS, Pelton SI, Stevenson AE, Kleinman K, et al. Evidence that pneumococcal serotype replacement in Massachusetts following conjugate vaccination is now complete. Epidemics. (2010) 2:80–4. doi: 10.1016/j.epidem.2010.03.005
141. Angeletti D, Gibbs JS, Angel M, Kosik I, Hickman HD, Frank GM, et al. Defining B cell immunodominance to viruses. Nat Immunol. (2017) 18:456–63. doi: 10.1038/ni.3680
142. Dinter J, Duong E, Lai NY, Berberich MJ, Kourjian G, Bracho-Sanchez E, et al. Variable processing and cross-presentation of HIV by dendritic cells and macrophages shapes CTL immunodominance and immune escape. PLoS Pathog. (2015) 11:e1004725. doi: 10.1371/journal.ppat.1004725
143. Havenar-Daughton C, Lee JH, Crotty S. Tfh cells and HIV bnAbs, an immunodominance model of the HIV neutralizing antibody generation problem. Immunol Rev. (2017) 275:49–61. doi: 10.1111/imr.12512
144. Altman MO, Angeletti D, Yewdell JW. Antibody immunodominance: the key to understanding influenza virus antigenic drift. Viral Immunol. (2018) 31:142–9. doi: 10.1089/vim.2017.0129
145. Zost SJ, Wu NC, Hensley SE, Wilson IA. Immunodominance and antigenic variation of influenza virus hemagglutinin: implications for design of universal vaccine Immunogens. J Infect Dis. (2019) 219:S38–S45. doi: 10.1093/infdis/jiy696
146. Angeletti D, Yewdell JW. Understanding and manipulating viral immunity: antibody immunodominance enters center stage. Trends Immunol. (2018) 39:549–61. doi: 10.1016/j.it.2018.04.008
147. Ritmahan W, Kesmir C, Vroomans RMA. Revealing factors determining immunodominant responses against dominant epitopes. Immunogenetics. (2020) 72:109–18. doi: 10.1007/s00251-019-01134-9
148. Baum J, Chen L, Healer J, Lopaticki S, Boyle M, Triglia T, et al. Reticulocyte-binding protein homologue 5 - an essential adhesin involved in invasion of human erythrocytes by Plasmodium falciparum. Int J Parasitol. (2009) 39:371–80. doi: 10.1016/j.ijpara.2008.10.006
149. Douglas AD, Williams AR, Knuepfer E, Illingworth JJ, Furze JM, Crosnier C, et al. Neutralization of Plasmodium falciparum merozoites by antibodies against PfRH5. J Immunol. (2014) 192:245–58. doi: 10.4049/jimmunol.1302045
150. Bocker MT, Hellwig I, Breiling A, Eckstein V, Ho AD, Lyko F. Genome-wide promoter DNA methylation dynamics of human hematopoietic progenitor cells during differentiation and aging. Blood. (2011) 117:e182–9. doi: 10.1182/blood-2011-01-331926
151. Crosnier C, Bustamante LY, Bartholdson SJ, Bei AK, Theron M, Uchikawa M, et al. Basigin is a receptor essential for erythrocyte invasion by Plasmodium falciparum. Nature. (2011) 480:534–7. doi: 10.1038/nature10606
152. Zhang H, Tang X, Zhu C, Song Y, Yin J, Xu J, et al. Adenovirus-mediated artificial MicroRNAs targeting matrix or nucleoprotein genes protect mice against lethal influenza virus challenge. Gene Ther. (2015) 22:653–62. doi: 10.1038/gt.2015.31
153. Tang X, Zhang H, Song Y, Zhou D, Wang J. Hemagglutinin-targeting artificial microRNAs expressed by adenovirus protect mice from different clades of H5N1 infection. Mol Ther Nucl Acids. (2016) 5:e311. doi: 10.1038/mtna.2016.25
154. Campeotto I, Goldenzweig A, Davey J, Barfod L, Marshall JM, Silk SE, et al. One-step design of a stable variant of the malaria invasion protein RH5 for use as a vaccine immunogen. Proc Natl Acad Sci USA. (2017) 114:998–1002. doi: 10.1073/pnas.1616903114
155. Jin J, Tarrant RD, Bolam EJ, Angell-Manning P, Soegaard M, Pattinson DJ, et al. Production, quality control, stability, and potency of cGMP-produced Plasmodium falciparum RH5.1 protein vaccine expressed in Drosophila S2 cells. NPJ Vaccines. (2018) 3:32. doi: 10.1038/s41541-018-0071-7
156. Williamson ED, Oyston PCF. Plague vaccines. In: Plokin SA, Orenstein W, Offit P, editors. Vaccines. 6th ed Philadelphia, PA: Elsevier (2013). p. 493–503.
157. Sun W, Singh AK. Plague vaccine: recent progress and prospects. NPJ Vaccines. (2019) 4:11. doi: 10.1038/s41541-019-0105-9
158. Chu K, Hu J, Meng F, Li J, Luo L, Xu J, et al. Immunogenicity and safety of subunit plague vaccine: a randomized phase 2a clinical trial. Hum Vaccin Immunother. (2016) 12:2334–40. doi: 10.1080/21645515.2016.1175261
159. Friedlander AM, Welkos SL, Worsham PL, Andrews GP, Heath DG, Anderson GW Jr, et al. Relationship between virulence and immunity as revealed in recent studies of the F1 capsule of Yersinia pestis. Clin Infect Dis. (1995) 21(Suppl 2):S178–81. doi: 10.1093/clinids/21.Supplement_2.S178
160. Titball RW, Williamson ED. Vaccination against bubonic and pneumonic plague. Vaccine. (2001) 19:4175–84. doi: 10.1016/S0264-410X(01)00163-3
161. Lihoradova OA, Indran SV, Kalveram B, Lokugamage N, Head JA, Gong B, et al. Characterization of Rift Valley fever virus MP-12 strain encoding NSs of Punta Toro virus or sandfly fever Sicilian virus. PLoS Negl Trop Dis. (2013) 7:e2181. doi: 10.1371/journal.pntd.0002181
162. Nishiyama S, Slack OA, Lokugamage N, Hill TE, Juelich TL, Zhang L, et al. Attenuation of pathogenic Rift Valley fever virus strain through the chimeric S-segment encoding sandfly fever phlebovirus NSs or a dominant-negative PKR. Virulence. (2016) 7:871–81. doi: 10.1080/21505594.2016.1195528
163. Alsarraf H, Dedic E, Bjerrum MJ, Ostergaard O, Kristensen MP, Petersen JW, et al. Biophysical comparison of diphtheria and tetanus toxins with the formaldehyde-detoxified toxoids, the main components of diphtheria and tetanus vaccines. Virulence. (2017) 8:1880–9. doi: 10.1080/21505594.2017.1321726
164. Moxon ER, Rainey PB, Nowak MA, Lenski RE. Adaptive evolution of highly mutable loci in pathogenic bacteria. Curr Biol. (1994) 4:24–33. doi: 10.1016/S0960-9822(00)00005-1
165. Maiden MCJ, Caugant DA. The population biology of Neisseria meningitidis: implications for meningococcal disease, epidemiology and control. In: Frosch M, Maiden Weinheim MCJ, editors. Handbook of Meningococcal Disease. Weinheim: Wiley-VCH Verlag GmbH & Co KGaA. (2006) p. 17–35.
166. Dyet K, Devoy A, Mcdowell R, Martin D. New Zealand's epidemic of meningococcal disease described using molecular analysis: implications for vaccine delivery. Vaccine. (2005) 23:2228–30. doi: 10.1016/j.vaccine.2005.01.050
167. Pizza M, Rappuoli R. Neisseria meningitidis: pathogenesis and immunity. Curr Opin Microbiol. (2015) 23:68–72. doi: 10.1016/j.mib.2014.11.006
168. Mueller AK, Labaied M, Kappe SH, Matuschewski K. Genetically modified Plasmodium parasites as a protective experimental malaria vaccine. Nature. (2005) 433:164–7. doi: 10.1038/nature03188
169. Francis SE, Malkov VA, Oleinikov AV, Rossnagle E, Wendler JP, Mutabingwa TK, et al. Six genes are preferentially transcribed by the circulating and sequestered forms of Plasmodium falciparum parasites that infect pregnant women. Infect Immun. (2007) 75:4838–50. doi: 10.1128/IAI.00635-07
170. Grifantini R, Bartolini E, Muzzi A, Draghi M, Frigimelica E, Berger J, et al. Previously unrecognized vaccine candidates against group B meningococcus identified by DNA microarrays. Nat Biotechnol. (2002) 20:914–21. doi: 10.1038/nbt728
171. Poland GA, Ovsyannikova IG, Jacobson RM. Adversomics: the emerging field of vaccine adverse event immunogenetics. Pediatr Infect Dis J. (2009) 28:431–2. doi: 10.1097/INF.0b013e3181a6a511
172. Poland GA. Vaccidents and adversomics. Vaccine. (2010) 28:6549–50. doi: 10.1016/j.vaccine.2010.08.032
173. Furman D, Davis MM. New approaches to understanding the immune response to vaccination and infection. Vaccine. (2015) 33:5271–81. doi: 10.1016/j.vaccine.2015.06.117
174. Hagan T, Nakaya HI, Subramaniam S, Pulendran B. Systems vaccinology: enabling rational vaccine design with systems biological approaches. Vaccine. (2015) 33:5294–301. doi: 10.1016/j.vaccine.2015.03.072
175. Oberg AL, Mckinney BA, Schaid DJ, Pankratz VS, Kennedy RB, Poland GA. Lessons learned in the analysis of high-dimensional data in vaccinomics. Vaccine. (2015) 33:5262–70. doi: 10.1016/j.vaccine.2015.04.088
176. Whitaker JA, Ovsyannikova IG, Poland GA. Adversomics: a new paradigm for vaccine safety and design. Expert Rev Vaccines. (2015) 14:1–13. doi: 10.1586/14760584.2015.1038249
177. Bae JM. Introduction of vaccinomics to develop personalized vaccines in light of changes in the usage of hantaan virus vaccine [Hantavax(R)] in Korea. J Prev Med Public Health. (2019) 52:277–80. doi: 10.3961/jpmph.19.018
178. Raeven RHM, Van Riet E, Meiring HD, Metz B, Kersten GFA. Systems vaccinology and big data in the vaccine development chain. Immunology. (2019) 156:33–46. doi: 10.1111/imm.13012
179. Kennedy RB, Poland GA. The top five “game changers” in vaccinology: toward rational and directed vaccine development. Omics. (2011) 15:533–7. doi: 10.1089/omi.2011.0012
180. Ovsyannikova IG, Poland GA. Vaccinomics: current findings, challenges and novel approaches for vaccine development. Am Assoc Pharm Sci. (2011) 13:438–44. doi: 10.1208/s12248-011-9281-x
181. Poland GA, Kennedy RB, Ovsyannikova IG. Vaccinomics and personalized vaccinology: Is science leading us toward a new path of directed vaccine development and discovery? PLoS Pathogens. (2011) 7:e1002344. doi: 10.1371/journal.ppat.1002344
182. Poland GA, Ovsyannikova IG, Kennedy RB, Haralambieva IH, Jacobson RM. Vaccinomics and a new paradigm for the development of preventive vaccines against viral infections. Omics. (2011) 15:625–36. doi: 10.1089/omi.2011.0032
183. Natrajan MS, Rouphael N, Lai L, Kazmin D, Jensen TL, Weiss DS, et al. Systems vaccinology for a live attenuated tularemia vaccine reveals unique transcriptional signatures that predict humoral and cellular immune responses. Vaccines. (2019) 8:4. doi: 10.3390/vaccines8010004
184. Lewis DJ, Lythgoe MP. Application of “systems vaccinology” to evaluate inflammation and reactogenicity of adjuvanted preventative vaccines. J Immunol Res. (2015) 2015:909406. doi: 10.1155/2015/909406
185. Miho E, Yermanos A, Weber CR, Berger CT, Reddy ST, Greiff V. Computational strategies for dissecting the high-dimensional complexity of adaptive immune repertoires. Front Immunol. (2018) 9:224. doi: 10.3389/fimmu.2018.00224
186. Sun P, Guo S, Sun J, Tan L, Lu C, Ma Z. Advances in in-silico B-cell epitope prediction. Curr Top Med Chem. (2019) 19:105–15. doi: 10.2174/1568026619666181130111827
187. Bowick GC, Barrett AD. Comparative pathogenesis and systems biology for biodefense virus vaccine development. J Biomed Biotechnol. (2010) 2010:236528. doi: 10.1155/2010/236528
188. Scheuermann RH, Sinkovits RS, Schenkelberg T, Koff WC. A bioinformatics roadmap for the human vaccines project. Expert Rev Vaccines. (2017) 16:535–44. doi: 10.1080/14760584.2017.1322752
189. Benkeser D, Gilbert PB, Carone M. Estimating and testing vaccine sieve effects using machine learning. J Am Stat Assoc. (2019) 114:1038–49. doi: 10.1080/01621459.2018.1529594
190. Heijne WH, Kienhuis AS, Van Ommen B, Stierum RH, Groten JP. Systems toxicology: applications of toxicogenomics, transcriptomics, proteomics and metabolomics in toxicology. Expert Rev Proteomics. (2005) 2:767–80. doi: 10.1586/14789450.2.5.767
191. Brockmeier EK, Hodges G, Hutchinson TH, Butler E, Hecker M, Tollefsen KE, et al. The role of omics in the application of adverse outcome pathways for chemical risk assessment. Toxicol Sci. (2017) 158:252–62. doi: 10.1093/toxsci/kfx097
192. Campbell-Tofte J, Vrahatis A, Josefsen K, Mehlsen J, Winther K. Investigating the aetiology of adverse events following HPV vaccination with systems vaccinology. Cell Mol Life Sci. (2019) 76:67–87. doi: 10.1007/s00018-018-2925-6
193. Mckinney BA, Reif DM, Rock MT, Edwards KM, Kingsmore SF, Moore JH, et al. Cytokine expression patterns associated with systemic adverse events following smallpox immunization. J Infect Dis. (2006) 194:444–53. doi: 10.1086/505503
194. Stanley SL Jr, Frey SE, Taillon-Miller P, Guo J, Miller RD, Koboldt DC, et al. The immunogenetics of smallpox vaccination. J InfectDis. (2007) 196:212–9. doi: 10.1086/518794
195. Feenstra B, Pasternak B, Geller F, Carstensen L, Wang T, Huang F, et al. Common variants associated with general and MMR vaccine-related febrile seizures. Nat Genet. (2014) 46:1274–82. doi: 10.1038/ng.3129
196. Poland GA, Grabenstein JD, Neff JM. The US smallpox vaccination program: a review of a large modern era smallpox vaccination implementation program. Vaccine. (2005) 23:2078–81. doi: 10.1016/j.vaccine.2005.01.012
197. Chapman LE, Iskander JK, Chen RT, Neff J, Birkhead GS, Poland G, et al. A process for sentinel case review to assess causal relationships between smallpox vaccination and adverse outcomes, 2003-2004. Clin Infect Dis. (2008) 46(Suppl 3):S271–93. doi: 10.1086/524750
198. Neff J, Modlin J, Birkhead GS, Poland G, Robertson RM, Sepkowitz K, et al. Monitoring the safety of a smallpox vaccination program in the United States: report of the joint Smallpox Vaccine Safety Working Group of the advisory committee on immunization practices and the Armed Forces Epidemiological Board. Clin Infect Dis. (2008) 46(Suppl 3):S258–70. doi: 10.1086/524749
199. Bruner DI, Butler BS. Smallpox vaccination-associated myopericarditis is more common with the newest smallpox vaccine. J Emerg Med. (2014) 46:e85–7. doi: 10.1016/j.jemermed.2013.06.001
200. Engler RJ, Nelson MR, Collins LC Jr, Spooner C, Hemann BA, Gibbs BT, et al. A prospective study of the incidence of myocarditis/pericarditis and new onset cardiac symptoms following smallpox and influenza vaccination. PLoS ONE. (2015) 10:e0118283. doi: 10.1371/journal.pone.0118283
201. Wilson CB, Marcuse EK. Vaccine safety–vaccine benefits: science and the public's perception. Nat Rev Immunol. (2001) 1:160–5. doi: 10.1038/35100585
202. Halsell JS, Riddle JR, Atwood JE, Gardner P, Shope R, Poland GA, et al. Myopericarditis following smallpox vaccination among vaccinia-naive US military personnel. J Am Med Assoc. (2003) 289:3283–9. doi: 10.1001/jama.289.24.3283
203. Oh JZ, Ravindran R, Chassaing B, Carvalho FA, Maddur MS, Bower M, et al. TLR5-mediated sensing of gut microbiota is necessary for antibody responses to seasonal influenza vaccination. Immunity. (2014) 41:478–92. doi: 10.1016/j.immuni.2014.08.009
204. Pasare C, Medzhitov R. Control of B-cell responses by Toll-like receptors. Nature. (2005) 438:364–8. doi: 10.1038/nature04267
205. Hohler T, Reuss E, Evers N, Dietrich E, Rittner C, Freitag CM, et al. Differential genetic determination of immune responsiveness to hepatitis B surface antigen and to hepatitis A virus: a vaccination study in twins. Lancet. (2002) 360:991–5. doi: 10.1016/S0140-6736(02)11083-X
206. Newport MJ, Goetghebuer T, Weiss HA, The MRCGTSG, Whittle H, Siegrist CA, et al. Genetic regulation of immune responses to vaccines in early life. Genes Immun. (2004) 5:122–9. doi: 10.1038/sj.gene.6364051
207. Klein NP, Fireman B, Enright A, Ray P, Black S, Dekker CL. A role for genetics in the immune response to the varicella vaccine. Pediatr Infect Dis J. (2007) 26:300–5. doi: 10.1097/01.inf.0000257454.74513.07
208. Yan K, Cai W, Cao F, Sun H, Chen S, Xu R, et al. Genetic effects have a dominant role on poor responses to infant vaccination to hepatitis B virus. J Hum Genet. (2013) 58:293–7. doi: 10.1038/jhg.2013.18
209. Tan PL, Jacobson RM, Poland GA, Jacobsen SJ, Pankratz VS. Twin studies of immunogenicity–determining the genetic contribution to vaccine failure. Vaccine. (2001) 19:2434–9. doi: 10.1016/S0264-410X(00)00468-0
210. Orru V, Steri M, Sole G, Sidore C, Virdis F, Dei M, et al. Genetic variants regulating immune cell levels in health and disease. Cell. (2013) 155:242–56. doi: 10.1016/j.cell.2013.08.041
211. Brodin P, Jojic V, Gao T, Bhattacharya S, Angel CJ, Furman D, et al. Variation in the human immune system is largely driven by non-heritable influences. Cell. (2015) 160:37–47. doi: 10.1016/j.cell.2014.12.020
212. Fong LE, Munoz-Rojas AR, Miller-Jensen K. Advancing systems immunology through data-driven statistical analysis. Curr Opin Biotechnol. (2018) 52:109–15. doi: 10.1016/j.copbio.2018.03.009
213. Guest JD, Pierce BG. Computational modeling of hepatitis C virus envelope glycoprotein structure and recognition. Front Immunol. (2018) 9:1117. doi: 10.3389/fimmu.2018.01117
214. Sousa SA, Seixas AMM, Leitao JH. Postgenomic approaches and bioinformatics tools to advance the development of vaccines against bacteria of the burkholderia cepacia complex. Vaccines. (2018) 6:34. doi: 10.3390/vaccines6020034
215. Liang X, Sun L, Yu T, Pan Y, Wang D, Hu X, et al. A CRISPR/Cas9 and Cre/Lox system-based express vaccine development strategy against re-emerging Pseudorabies virus. Sci Rep. (2016) 6:19176. doi: 10.1038/srep19176
216. Zou Z, Huang K, Wei Y, Chen H, Liu Z, Jin M. Construction of a highly efficient CRISPR/Cas9-mediated duck enteritis virus-based vaccine against H5N1 avian influenza virus and duck Tembusu virus infection. Sci Rep. (2017) 7:1478. doi: 10.1038/s41598-017-01554-1
Glossary
Vaccinomics
The integration of immunogenetics and immunogenomics with systems biology, immune profiling, and bioinformatics approaches used in the development and study of new vaccines.
Adversomics
The study of vaccine adverse reactions using immunogenetics and systems biology approaches to better understand the genetic and non-genetic drivers of vaccine adverse reactions at the molecular level.
Epigenomics
The study of the complete set of epigenetic modifications on the genetic material of a cell (i.e., the epigenome).
Genome-wide association studies (GWAS)
The observational study of a genome-wide set of genetic variants in different subjects to examine if any one variant is associated with a trait.
Systems biology
The study of complex systems in order to understand the networks of interactions and effects of those interactions.
CRISPR–Cas-9 technology
A gene-editing technology that can be used in the development of vaccines.
Keywords: vaccine, genomics, transcriptomics, vaccine development, genetics
Citation: Kennedy RB, Ovsyannikova IG, Palese P and Poland GA (2020) Current Challenges in Vaccinology. Front. Immunol. 11:1181. doi: 10.3389/fimmu.2020.01181
Received: 09 March 2020; Accepted: 13 May 2020;
Published: 25 June 2020.
Edited by:
Ursula Wiedermann, Medical University of Vienna, AustriaReviewed by:
Denise Doolan, James Cook University, AustraliaWayne Koff, Human Vaccines Project, United States
Dominique Missiakas, University of Chicago, United States
Daniel E. Zak, Seattle Biomedical Research Institute, United States
Copyright © 2020 Kennedy, Ovsyannikova, Palese and Poland. This is an open-access article distributed under the terms of the Creative Commons Attribution License (CC BY). The use, distribution or reproduction in other forums is permitted, provided the original author(s) and the copyright owner(s) are credited and that the original publication in this journal is cited, in accordance with accepted academic practice. No use, distribution or reproduction is permitted which does not comply with these terms.
*Correspondence: Richard B. Kennedy, a2VubmVkeS5yaWNrQG1heW8uZWR1