- 1Department of Hematology and Medical Oncology, Icahn School of Medicine at Mount Sinai Hospital, New York, NY, United States
- 2Center for Computational Immunology, Tisch Cancer Institute, Icahn School of Medicine at Mount Sinai Hospital, New York, NY, United States
- 3Department of Pathology, Icahn School of Medicine at Mount Sinai Hospital, New York, NY, United States
- 4Department of Oncological Sciences, Icahn School of Medicine at Mount Sinai Hospital, New York, NY, United States
Tumor progression is typically accompanied by an accumulation of driver and passenger somatic mutations. A handful of those mutations occur in protein coding genes which introduce non-synonymous polymorphisms. Certain substitutions may give rise to novel, tumor-associated antigens or neoantigens, presentable by cancer cells to the host adaptive immune system. As antigen recognition is the core of an effective immune response, the identification of patient tumor specific antigens derived from transformed cells is of importance for immunotherapeutic approaches. Recent technological advances in DNA sequencing of tumor genomes, advances in gene expression analysis, algorithm development for antigen predictions and methods for T-cell receptor (TCR) repertoire sequencing have facilitated the selection of candidate immunogenic neoantigens. In this regard, multiple research groups have reported encouraging results of neoantigen-based cancer vaccines that generate tumor antigen specific immune responses, both in mouse models and clinical trials. Additionally, both the quantity and quality of neoantigens has been shown to have predictive value for clinical outcomes in checkpoint-blockade immunotherapy in certain tumor types. Neoantigen recognition by vaccination or through adoptive T cell therapy may have unprecedented potential to advance cancer immunotherapy in combination with other approaches. In our review we discuss three parameters regarding neoantigens: computational methods for epitope prediction, experimental methods for epitope immunogenicity validation and future directions for improvement of those methods. Within each section, we will describe the advantages and limitations of existing methods as well as highlight pressing fundamental problems to be addressed.
Introduction
Successful targeting of immune checkpoints including cytotoxic T lymphocyte-associated protein 4 (CTLA-4) and programmed cell death protein 1 (PD-1) has achieved durable regressions in a wide range of human cancers (referred to as checkpoint blockade). They include melanoma (1, 2), renal cell carcinoma (3), lung (4), bladder (5), and ovarian cancers (6), and microsatellite unstable malignancies (7, 8). Despite different mechanisms of action, both approaches have resulted in the activation and proliferation of tumor-reactive T cells (9). T cells recognize peptides presented on the major histocompatibility complex (MHC) of tumor cells. Tumor specific antigens which arise due to mutations in coding regions are collectively referred to as “neoantigens.” Neoantigens have a diversity of properties. They can differ from their wild type sequences by SNV, relative expression levels in the tumor, MHC affinity, differential recognition by T-cell receptors (TCRs) and elicitation of heightened cytotoxic and cytokine responses. Theoretically, T cells recognizing neoantigens may have not been deleted or tolerized so they have the potential to become primed. Moreover, unlike tumor-associated antigens (TAA) that are shared between tumor cells and normal tissue (e.g., Melan A/MART-1) neoantigens have a selective potential to elicit tumor exclusive T cell responses which makes them key elements for inclusion in cancer vaccines and as the basis for adoptive T cell transfer approaches (10–14). Indeed, initial attempts to target overexpressed TAA have met limited success in clinical trials potentially due to central and peripheral tolerance mechanisms which removes high-affinity TCRs that would otherwise potently recognize these TAA (15, 16). Unleashing immune responses against tumor-specific clonal mutations can achieve tumor regression through recognition by antigen-specific T cells (17–22). Furthermore, as a tumor's mutational landscape evolves with ongoing immunotherapy, the immune system may accommodate by changing the specificity of infiltrating T cell clones (23–25). Efficient approaches to identify and characterize immunogenic tumor neoantigens are central for these types of therapies.
Thus, far, MHC-I affinity is the only parameter which can be predicted with some reliability using neoantigen peptide and patient HLA allele sequences in silico, by using several computational tools. Our group recently proposed the concept of “neoantigen quality” (26, 27). This concept combines biophysical, chemical and computationally inferred properties of a neoantigen that make it more likely to induce a productive immune response against the tumor. These properties may include affinity of a neoantigen to MHC, avidity of the peptide-MHC complex to the recognizing TCR, type of T cells responding to the neoantigen and sequence similarity to known highly immunogenic epitopes (Figure 1). Recent studies from our group have shown that this parameter is a critical aspect in segregating responders to checkpoint therapy, but is not usually considered in algorithms of neoantigen prediction.
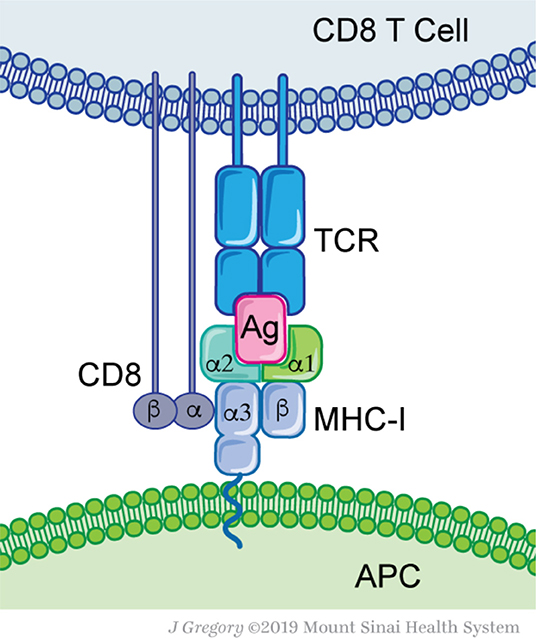
Figure 1. Molecular basis for antigen recognition. Antigen-presenting cells (APC) express MHC-I complex that contains an antigenic peptide (Ag) with its groove. MHC-I consist of two proteins, a conserved β2-microglobulin and a variable α-chain. The MHC-I-Ag complex is recognized by the T-cell receptor (TCR). Each TCR defines a clonal T cell population. Additional interactions, such as the CD8 protein—MHC-I, are not essential for Ag recognition, but are required for efficient T cell activation.
T cells are primed by antigen presenting cells (APC) that have taken up tumor antigens and processed them into smaller peptides that are eventually presented on MHC class I and II molecules (Figure 1). Intracellularly, antigens arise from proteins targeted for degradation by the 80S proteasome. Peptides of 9-12 amino acid residues in length are transported from the cytosol by specialized protein machinery (transporter associated with antigen presentation, TAP) and loaded on MHC-I molecules within the endoplasmic reticulum (28–30). Alternatively, antigens can arise from extracellular sources; captured necrotic or apoptotic cells and other vesicles that are cross-presented on professional APC such as dendritic cells (DC) (30, 31). As a tumor grows, tissue resident and migratory DC subsets capture tumor cell debris and convey them to draining lymph nodes (32–34). There, APC prime naïve T cells and educate them to recognize the harvested antigens (35, 36). Depending on the APC subset, nature of antigen and type of processing pathway, different responses can be achieved, either CD4+ T cell responses (Th1, Th2, Th17, and Treg) or cytotoxic CD8+ T cell responses (37, 38). The majority of APC prime naïve CD4+ T cells through MHC-II presented peptides, while the cross-presenting XCR1+ DC subset uniquely primes naïve CD8+ T cells (39, 40). The latter appear to be essential for successful immunotherapy regimens (41–43). After priming, reactive and expanded T cells can infiltrate the cancer site and eliminate these cells. Overall, proper antigen selection, processing, and T cell priming are at the heart of successful immune responses.
With the recognition that neoantigens can be a significant pool of tumor derived antigens depending upon the underlying mutational status of the tumor, the field has turned its attention to developing and optimizing neoantigen targeted immune therapies. There are generally two approaches: neoantigen vaccines and neoantigen targeted adoptive T cell therapies. Several clinical trials have been completed and/or are currently ongoing to enhance tumor-specific responses through neoantigen vaccination to induce expansion of neoantigen-specific CD4+ and CD8+ T cells. Vaccination techniques employ different neoantigen formulations such as peptides (13, 44, 45) combined with different adjuvants (46–49), mRNA (50, 51), DNA or as expressed in viral or bacterial vectors. Another interesting approach targets neoantigens to specific receptors expressed specifically by the cross-presenting APC cDC1. Here antigens are fused in frame to antibodies targeting the XCR1 receptor (52). Thus, far, vaccine-based approaches have demonstrated successful immunization of patients (53, 54), although CD4+ as opposed to CD8+ T cells are preferentially generated. Cell-based vaccination is also under investigation. In vitro expanded, neoantigen-pulsed dendritic cells have been evaluated for autologous injection in patients (46, 55–58) confirming immunogenicity (57, 58). Another approach focuses on the adoptive T cell transfer of expanded T cells purified from the patient's tumor or peripheral blood mononuclear cells (PBMC) either non-specifically or through selection in vitro. Some strategies have successfully utilized neoantigen-specific CD4+ and CD8+ cytotoxic T cells to eradicate solid tumors (59, 60). In another example, autologous T cell transfer of CD8+ T cells, specific to clonal neoantigens derived from cancer driver mutations, e.g., KRAS, has led to nearly complete tumor regression (61).
Mutation burden, neoantigen burden and quality have been demonstrated to be predictive for outcome of checkpoint blockade (26, 62–70). A few studies have highlighted the importance of neoantigens in shaping tumor evolution during immunotherapy with antibodies that target checkpoint molecules such as CTLA-4 and PD-1 (71, 72). However, neoantigen prediction approaches are not aligned with some utilizing solely gene expression (73, 74) or combining transcriptomics with genomics (75). The successful characterization of immunogenic neoantigens is critical to optimizing approaches that target these key epitopes. In this review we critically discuss current tools and methods for their selection (76).
The Landscape of Neoantigens
Neoantigens arise from multiple genetic and epigenetic aberrations (Figure 2). Well-characterized sources of neoantigens are somatic missense and indel mutations, or other genomic rearrangements, such as gene fusions. Frameshift neoantigens may prove to be more immunogenic than missense ones due to the lack of similarity to sequences in the human coding genome and are currently under active investigation (77, 78). Neoantigens derived from gene fusions have recently passed the immunogenicity test (12), and may be of special significance when mutational burden is low. Correct detection of somatic mutations is essential to identify neoantigens incorporated within these alterations (17, 79, 80). Neoantigens can also arise from transcriptome-based aberrations, including cancer-specific gene overexpression, alternative exon splicing, intron retention, premature transcription ending, readthrough the stop-codon by ribosomes and from upstream open reading frames (uORF) (Figure 2). Virus-induced cancers, e.g., HPV+, EBV+, generate strong immune responses due to presentation of viral antigens (81) and as such can be considered as cancer-specific antigens. Transcript-specific changes in exon usage (82, 83), intron retention (84), and transcription end usage were recently shown to produce cancer-specific neoantigens. Translation-based neoantigens, originating from uORF regions, cryptic short ORFs in non-coding RNAs still await their discovery on a pan-cancer level. Whole genome sequencing, deep RNAseq gene expression analysis, whole-cell and MHC-eluate mass-spectrometry will be necessary for a determination of the complete landscape of such neoantigens (85). Finally, cancer-specific post-translational protein modifications, e.g., phosphorylation, acetylation, methylation, citrullination, and etc., can be a potential source of neoantigens as well (86, 87). Aberrant over activity of protein kinases, histone acetylases, and methylases is well-known in multiple cancers. This can result in frequent modifications of non-natural protein targets or cancer-specific proteins, which may in turn produce immunogenic, tumor-specific neoantigens (88, 89) (Figure 2). It is important to point out that T cells with the capacity to recognize these modified antigens likely have escaped central tolerance and thus represent a large pool of T cell clones that could be harnessed to attack cancer cells. Technological advances in mass-spectrometry peptide detection from cancer MHC-I eluates will be essential for neoantigen discovery of this class (90).
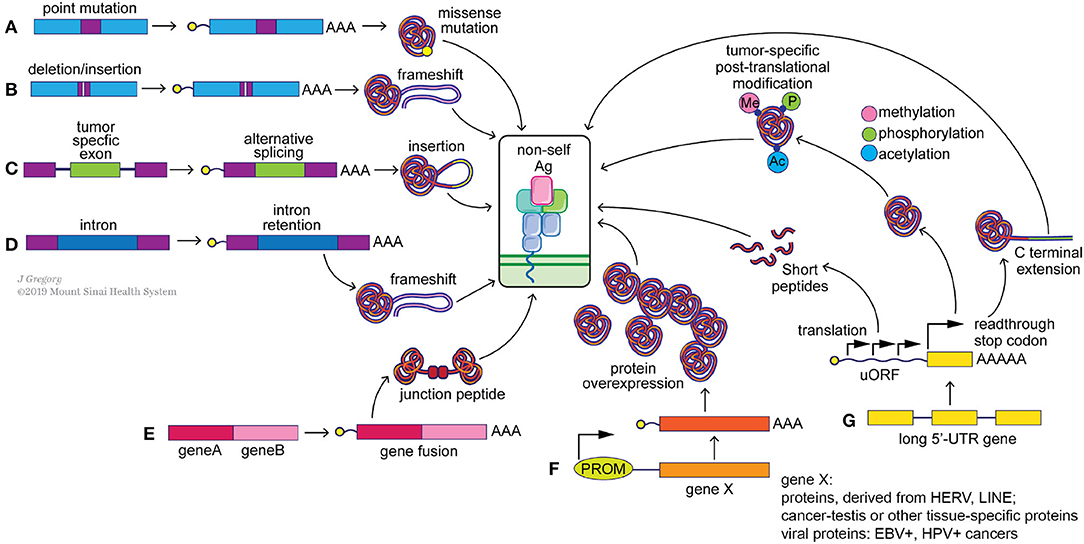
Figure 2. Potential sources of “non-self” tumor neoantigens. Genomic alterations such as point mutations (A), indels (B) and gene fusions (E) can result in the generation of missense and frameshift neoantigens. Splicing aberrations such as the retention of cancer-specific exons (C) and introns (D) can also lead to frameshift neoantigens. Epigenetic changes can alter the expression levels of immunogenic genomically-encoded proteins (F) including viral proteins from integrated chronically-infected cells (EBV, HPV), cancer-testis antigens (e.g., MAGE-A4) and proteins derived from LINE and HERV elements. Post-transcriptional changes (G), including translation of upstream open reading frames (uORF), stop codon readthrough and protein modifications, such as methylation, phosphorylation and acetylation, can generate tumor specific neoantigens as well.
On A Computational Hunt for Neoantigens
Somatic Mutation Calling
Despite the broad range of potential sources of neoantigens in cancer cells, the process of selection of genomically encoded antigens that are of immunological significance remains to be well-established. Many computational pipelines have been developed to predict neoantigens from cancer genomes (91, 92). A joint effort referred to as the Tumor Neoantigen Selection Alliance (TESLA; supported by the Parker Institute for Cancer Immunotherapy and the Cancer Research Institute) to find the right predictive algorithms for targeting neoantigens (based upon NSVs) through large scale validation is ongoing. At this time, a “typical” neoantigen pipeline includes the following steps:
• Whole exome or genome sequencing (WES or WGS) of tumor and matched normal DNA samples by Illumina short read sequencing platform.
• Quality control of sequencing reads.
• Alignment to the reference genome.
• Base quality recalibration and indel realignment.
• Comparison of normal and tumor alignments to call somatic mutations.
• Conversion of coding DNA somatic mutations to corresponding mutated peptide sequences.
• HLA-allele typing.
• Assessment of HLA-allele and mutated epitope (9–11mer) affinity to call neoantigens.
• Expression analysis of putative neoantigens, e.g., RNAseq, when possible.
Multiple tools exist to check the quality of sequencing reads with the most commonly used being FastQC (93, 94). Alternative tools are included in the Genome Analysis Toolkit (GATK) bundle. To perform read alignment, Novoalign (95), BWA (96), bowtie (97), STAR (98) are the most favored aligners. For a typical WES (or WGS) dataset BWA is a commonly used aligner. Base quality recalibration and indel realignment around clusters of putative somatic mutations are both integral tools of GATK and have been shown to reduce the false positive rates of mutation calling (99). Collectively, these “pre-processing” steps output aligned, cleaned, equilibrated *.bam files of tumor and matched normal samples. These matched datasets are fed to a combination of mutation callers to predict somatic mutations in tumor samples. A wide range of somatic mutation callers exist to date, such as Mutect (100), Varscan2 (101), VarDict (102), SomaticSniper (103), Strelka, and FastD (104). Many comparative studies have been performed to call mutations (105–108) (Figure 3A). Some key observations are noted below.
• A combination of multiple algorithms vs. a single mutation caller significantly lowers the false positive rate (108–110).
• Calling somatic mutations from additional sequencing such as of RNAseq of the same tumor sample and determining overlap may help to reduce the false positive rate. However, it may increase the rate of false negatives due to transient gene expression and variable read coverage (111).
• PCR-free WES protocols [KAPA HyperPrep Kit (112)] produce less bias in tumor allele frequencies but achieve it at the expense of reduced total genome loci coverage and of lowered total power of somatic mutation calling (109).
• Exome capture kits (Agilent SureSelect, NimbleGen SeqCap, Illumina TrueSeq, and Illumina Nextera) introduce sequencing coverage biases due to differences in capture probes. This makes it potentially hard to compare final mutation calls obtained from different WES kits of the same sample of DNA, resulting in increased false negative rates for somatic mutation calls (113–116).
• Sequencing read coverage drops significantly in GC-rich regions, decreasing the sensitivity of tumor allele detection in these loci. Correcting for GC-bias may help to rescue certain mutations and improve tumor allele frequency estimations (114).
• Maintaining high tumor purity of the sample before DNA sequencing is essential. High levels of normal DNA “contamination” decreases sensitivity of tumor mutation calling (105, 109, 114).
• The quality of the sample is important, e.g., fresh tissue samples are better than FFPE. It is highly advisable to avoid excessive sample handling known to introduce random DNA mutations, e.g., adenine/cytosine deamination, guanine oxidation, which can impact the results of Illumina sequencing. Otherwise the somatic mutation false positive rates increase (105, 109).
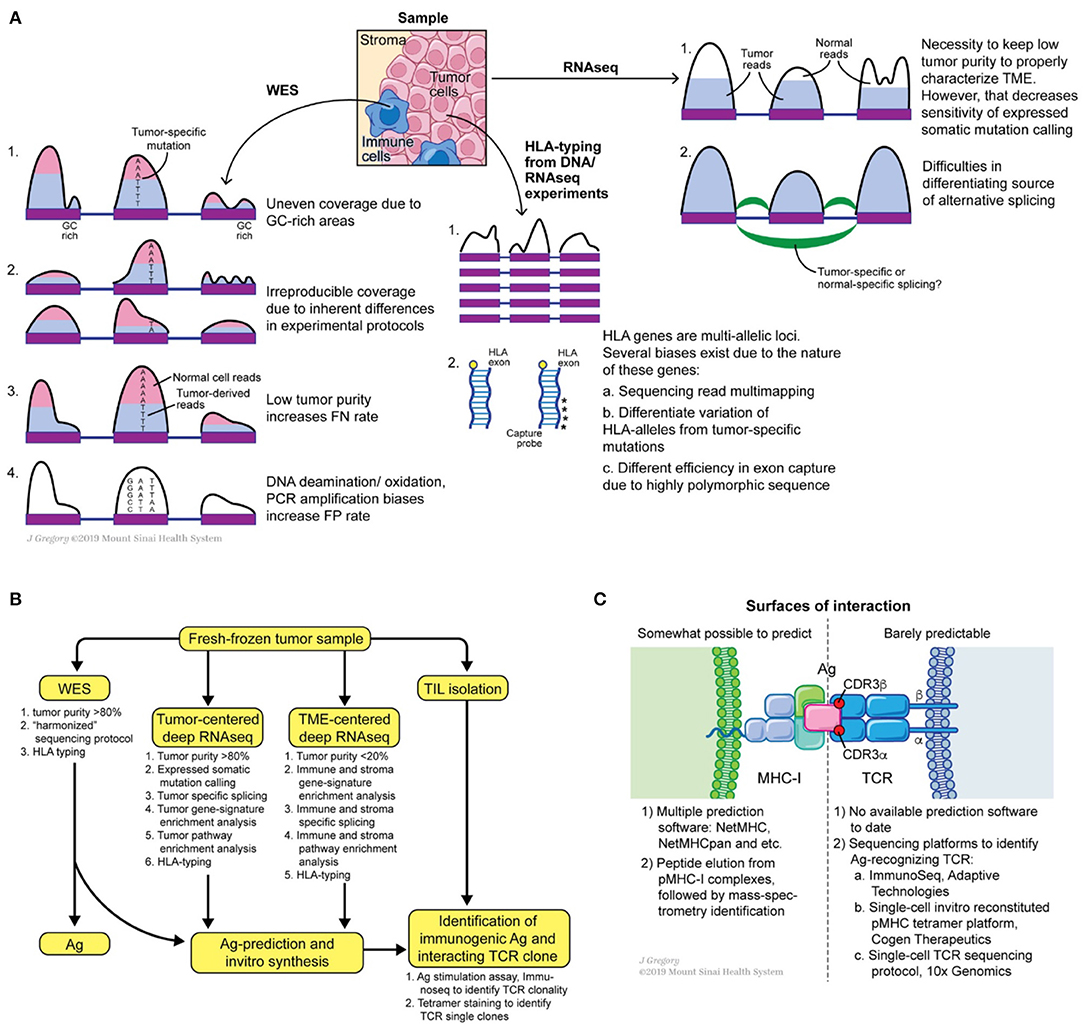
Figure 3. Potential sources of somatic mutation irreproducibility (A), suggested experimental design for comprehensive annotation of tumor mutational burden and tumor microenvironment, (B) and possible roads for innovation at the MHC-I antigen TCR interfaces (C).
Overall, using fresh or fresh-frozen samples with high tumor purity (>80%), sticking to one WES protocol, introducing a low number of PCR cycles, following GATK pre-processing recommendations, and applying several somatic mutation callers can benefit the generation of a reproducible, “harmonized” lists of somatic mutations (117). Calling somatic mutations from RNAseq of samples with high tumor content (>80%) can further refine the list of expressed mutations. Consensus on unified somatic calling pipelines will be essential to improve the overall prediction of neoantigens and detection of the shared ones.
HLA-Allele Typing
The next step in a neoantigen calling pipeline is HLA-allele typing. CD8+ T cells see antigens presented on the MHC-I complex, which is composed of conserved β2-microglobulin and a variable α-chain. The latter subunit is highly polymorphic and encoded within the HLA gene, which is represented by three loci on human chromosome 6: HLA-A, HLA-B, and HLA-C. Thus, HLA allele assignment consists of the gene name (A, B, or C) followed by a set of digits separated by colons: the first two digits specify serological activity (A*01, B*03, etc.) and the second two digits indicate protein sequence (A*01:05, B*03:05, etc.). Due to the high level of polymorphism of each gene, precise HLA-allele typing at protein level resolution from WES and RNAseq reads is a complicated task (118). Multiple tools were developed to address this problem such as PHLAT (119), seq2HLA (120), Optitype (121), Polysolver (122), HLAMatchmaker (123), HLAreporter (124), HLAforest (125), HLAminer (126), xHLA (127). Each tool differs in its performance, utilized set of input parameters and analyzed sequencing dataset (RNAseq or DNAseq). Comparative studies were performed and showed that Optitype has both greater specificity and selectivity (128). However, it is important to keep in mind that the quality of WES/RNAseq is critical for any successful HLA typing. Indeed, due to the highly polymorphic nature of HLA genes, WES capture kits vary in the capturing efficiency of DNA from those regions (Figure 3A). This technical variability in capturing clearly affects downstream results in allele determination. Thus, careful examination of WES/RNAseq read coverage in HLA gene regions is imperative for making optimal predictions.
Prediction of Neoantigen HLA-Allele Interactions
In the final step, the researcher performs predictions of tumor antigenic epitope- HLA-allele interactions to identify neoantigens from the total pool of mutated peptides. Several tools and programs which are undergoing constant modification, are dedicated to this problem; NetMHC-pan being the one most widely used. NetMHC utilizes a combination of several artificial neural-networks (ANNs) to predict peptide affinity to selected HLA alleles. Initially NetMHC was trained on viral antigens from IEDB [https://www.iedb.org, (129–134)], therefore rendering a bias toward the selection of viral-like epitopes. Despite its general popularity, users should always keep in mind the biases these classification methods can introduce. For example, viral epitopes were originally described for the most frequent HLA-alleles, e.g., HLA*A02:01, HLA*B07:02. Thus, netMHC based predictions for tumor epitopes are a priori better for highly frequent HLA-alleles than for low-frequency HLA-alleles. One way to overcome this issue is to improve predictions by training the algorithm on peptides eluted from MHC complexes of mono-allelic cancer cell lines and identified by mass-spectrometry analysis (135). However, mass-spectrometry itself has limited ability to detect all possible eluted antigens, thus the false negative rate can be high (90, 136, 137). Data from mass spectrometry analyses indicates that only a small fraction of neoepitopes is presented on the cell surface, likely due to a combination of such systematic biases and real biophysical effects in the processing machinery (138–140). Taken together, there is an urgent need for novel, unbiased methods to generate MHC-I complexes for every HLA-allele with broadly diversified antigen sequences in order to design novel classification tools (Figure 3C).
Apart from class I epitopes, class II restricted neoantigens are receiving increased interest. Class II neoantigens are those epitopes presented by the MHC-II complex and recognized by CD4+ T cells. Despite the recognition that MHC-II is significant for tumor neoantigen presentation and priming of CD4+ T cells (141) and for immunotherapy outcomes (142), the accuracy and precision of MHC-II epitope predictions are poor when compared to class I (143). The main difficulties with designing such classification tools are associated with the “openness” of the peptide-binding groove of HLA class II, which permits binding of a highly degenerate set of peptides, and therefore increasing the size of datasets needed for accurate machine learning-based model training. However, these obstacles provide an opportunity for more creative efforts to develop algorithms to predict such neoantigens.
Identification of Immunogenic Neoantigen-Reactive T Cells
Not every neoantigen presented on MHC-I complexes will have the capacity to induce CD8+ T cell responses (79). What defines neoantigen immunogenicity? Conventionally, an immunogenic neoantigen must prime and stimulate T cells efficiently. This occurs through (i) interaction of the neoantigen-MHC-I complex with a TCR on one or several T cell clones, and (ii) induction of T cell priming. This process generally results in either TNF-α, IFN-γ, or double TNF-α and IFN-γ cytokine responses, IL-2 release and T cell proliferation, and the acquisition of cytolytic activity in the case of CD8+ T cells. As reviewed above, vaccination has led to the priming and expansion of neoantigen-specific T cells in humans. These responses can be enumerated through assays which measure production of cytokines upon re-exposure to peptides (through ELISA type assays or intracellular staining) or binding to synthetic tetrameric or dextrameic complexes of peptide-MHC (pMHC) molecules. The latter method relies on in vitro folding of the MHC-I complex (144, 145) with peptide or UV-cleavable substrate (146) which is later exchanged for the peptide of interest (147).
Neoantigen-specific T cells with effector function have been identified within PBMC following vaccination or even after spontaneous induction (148), tumor infiltrating lymphocytes (149) and can even be differentiated from progenitors through in vitro priming approaches (150). A concerted effort is being made to expand potent neoantigen-reactive T cells for the purpose of adoptive cell therapy or to identify high avidity neoantigen-reactive TCRs which can be modified and transduced into a primary T cells. For example, to overcome thymic negative selection, which decreases TCR diversity in vivo (151), humanized mice can be used to select the most-optimal neoantigen-reactive TCRs (152). Tetramer-purified, neoantigen-reactive T cell clones can also be expanded from these sources or human blood or TILs in single-cell fashion and their TCRs sequenced. The selected TCRs can be used for recombinant TCR reconstitution (153) and characterization in vitro, for additional modification to improve TCR avidity and stability (129, 130) and then adapted for adoptive T cell transfer using a cancer patient's own T cells (131). In this regard tetramer staining can be applied to identify neoantigen-specific TCRs in a high-throughput manner (132). One discovery platform generates in vitro translated, DNA-barcoded pMHC complexes from a chemically synthesized DNA library (133). Once tetramer-positive T cells are purified, their interacting TCRs and DNA-barcoded antigens are identified through single-cell sequencing. Moreover, the same platform can be repurposed to characterize all possible peptide specificities for each HLA-allele of MHC-I and MHC-II complexes. Indeed, the ability to (i) start from a randomized DNA library of putative epitopes and (ii) characterize folding potential of produced pMHC complexes in large scale could yield invaluable information to train novel classification algorithms. Despite the obvious advantage of tetramer staining in identifying neoantigen-reactive T cells, this tool provides limited information on the functional status of purified T cells and their cytotoxic capacity (134). The recent development of T-scan screening technology holds promise to overcome this issue (154). Likewise, a recently developed method referred to as imPACT Isolation Technology identifies pre-existing T cell clones that recognize tumor neoantigens (155). Such approaches lay the foundation for multi-group collaborations to synthesize neoantigen-specific T cells for personalized adoptive T cell therapies (155).
Collectively, the identification of immunogenic neoantigens is a multi-step process that requires significant time, cost and labor to accomplish. Personalized neoantigen-based immunotherapies suffer from such drawbacks, sometimes requiring up to 3 months to manufacture the a short list of “best” candidates (156). A potential solution to this pipeline problem is to target shared neoantigens, that are highly recurrent, clonal, and broadly immunogenic across cancer patients. However, whether such immunogenic shared antigens are sufficiently available across broad cancer types remains to be determined. Prioritizing such antigens whenever possible is important, as any “off-the shelf” strategies that can be developed will significantly reduce the cost and increase the efficiency of neoantigen-specific cancer immunotherapies.
Concluding Remarks
We review the available tools for the computational prediction and experimental validation of tumor-associated neoantigens, discussing approaches for somatic mutation detection, HLA allele typing, and prediction of peptide-MHC interactions. We have made an effort to highlight the biases associated with particular approaches and suggest possible ways to minimize their influence. We also outline technologies for identifying immunogenic neoantigens. Future developments that could improve these strategies are suggested in Figure 3. Firstly, harmonization of somatic mutation calling can improve reproducibility across different platforms and sequencing centers. Secondly, in vitro assays for folding and characterization of pMHC complexes starting from randomized peptide libraries can improve existing prediction tools. Applying the same approach for peptide-MHC-II complexes may also improve MHC-II classification tools (157). Finally, single-cell identification of TCR-antigen interacting pairs will provide information on the principles of TCR-neoantigen interactions, making it possible to develop predictive methods for this type of interaction (158). The latter will be an invaluable tool for immunogenic neoantigen selection for vaccine designs, refining immunotherapy outcome predictions, or selecting the most avid TCR for adoptive recombinant T cell therapies. We believe the field of neoantigen-based immunotherapies of cancer is undergoing a major renaissance. Equipped with powerful sequencing technologies, sensitive computational tools for neoantigen discovery and efficient high-throughput platforms for characterization of their immunogenicity, scientists will have the potential to bring novel disruptive immunotherapies to the clinic to definitely improve outcomes of cancer patients.
Author Contributions
VR wrote the manuscript. VR, BG, and NB reviewed and revised the manuscript.
Funding
NB has National Institutes of Health grants R01CA201189, R01CA180913, and R01AI081848 and receives support from the Melanoma Research Alliance, Cancer Research Institute, Leukemia & Lymphoma Society and NYSTEM. BG has National Institutes of Health grants 7R01AI081848-04 and 1P30CA196521-01; BG has Stand Up To Cancer-National Science Foundation-Lustgarten Foundation Convergence Dream Team Grant sponsored by Stand Up to Cancer, the Lustgarten Foundation, the V Foundation and the National Science Foundation grant NSF 1545935; BG is the Pershing Square Sohn Prize-Mark Foundation Fellow supported by funding from The Mark Foundation for Cancer Research.
Conflict of Interest
NB receives research support or reagents from Novocure, Celldex, the Ludwig Institute for Cell Research, Genentech, Oncovir, and Regeneron, and is on the advisory boards of Neon, Tempest, Checkpoint Sciences, Curevac, Primevax, Novartis, Array BioPharma, Roche, and Avidea. NB receives grant support from and serves on the advisory board of the Parker Institute for Cancer Immunotherapy.
The remaining authors declare that the research was conducted in the absence of any commercial or financial relationships that could be construed as a potential conflict of interest.
Acknowledgments
We thank the members of NB and BG labs for critical assessment of the manuscript.
References
1. Hodi S, O'Day S, McDermott D, Weber R, Harris A, Johnson DW, et al. Improved survival with ipilimumab in patients with metastatic melanoma. N Engl J Med. (2010) 363:609–19. doi: 10.1056/NEJMoa1003466
2. Topalian S, Hodi S, Brahmer J, Gettinger S, Smith DC, Mcdermott DF, et al. Safety, Activity, and immune correlates of anti–PD-1 antibody in cancer. N Engl J Med. (2012) 366:2443–54. doi: 10.1056/NEJMoa1200690
3. Motzer RJ, Escudier B, McDermott DF, George S, Hammers HJ, Srinivas S, et al. Nivolumab versus everolimus in advanced renal-cell carcinoma. N Engl J Med. (2015) 373:1803–13. doi: 10.1056/NEJMoa1510665
4. Brahmer J, Reckamp KL, Baas P, Crinò L, Eberhardt WEE, Poddubskaya E, et al. Nivolumab versus docetaxel in advanced squamous-cell non-small-cell lung cancer. N Engl J Med. (2015) 373:123–35. doi: 10.1056/NEJMoa1504627
5. Powles T, Eder JP, Fine GD, Braiteh FS, Loriot Y, Cruz C, et al. MPDL3280A (anti-PD-L1) treatment leads to clinical activity in metastatic bladder cancer. Nature. (2014) 515:558–62. doi: 10.1038/nature13904
6. Hamanishi J, Mandai M, Ikeda T, Minami M, Kawaguchi A, Murayama T, et al. Safety and antitumor activity of anti-PD-1 antibody, nivolumab, in patients with platinum-resistant ovarian cancer. J Clin Oncol. (2015) 33:4015–22. doi: 10.1200/JCO.2015.62.3397
7. Le DT, Uram JN, Wang H, Bartlett BR, Kemberling H, Eyring AD, et al. PD-1 Blockade in tumors with mismatch-repair deficiency. N Engl J Med. (2015) 372:2509–20. doi: 10.1200/jco.2015.33.15_suppl.lba100
8. Le DT, Durham JN, Smith KN, Wang H, Bartlett BR, Aulakh LK, et al. Mismatch repair deficiency predicts response of solid tumors to PD-1 blockade. Science. (2017) 413:409–13. doi: 10.1126/science.aan6733
9. Tumeh PC, Harview CL, Yearley JH, Shintaku IP, Taylor EJM, Robert L, et al. PD-1 blockade induces responses by inhibiting adaptive immune resistance. Nature. (2014) 515:568–71. doi: 10.1038/nature13954
10. Schumacher TN, Schreiber RD. Neoantigens in cancer immunotherapy. Science. (2015) 348:69–74. doi: 10.1126/science.aaa4971
11. De Vries J, Figdor C. Immunotherapy: cancer vaccine triggers antiviral-type defences. Nature. (2016) 534:329–31. doi: 10.1038/nature18443
12. Yang W, Lee K, Srivastava RM, Kuo F, Krishna C, Chowell D, et al. Immunogenic neoantigens derived from gene fusions stimulate T cell responses. Nat Med. (2019) 25:1–9. doi: 10.1038/s41591-019-0434-2
13. Keskin DB, Anandappa AJ, Sun J, Tirosh I, Mathewson ND, Li S, et al. Neoantigen vaccine generates intratumoral T cell responses in phase Ib glioblastoma trial. Nature. (2019) 565:234–9. doi: 10.1038/s41586-018-0792-9
14. Tran E, Robbins PF, Rosenberg SA. Final “common pathway” of human cancer immunotherapy: targeting random somatic mutations. Nat Immunol. (2017) 18:255–62. doi: 10.1038/ni.3682
15. Coulie PG, Van Den Eynde BJ, Van Der Bruggen P, Boon T. Tumour antigens recognized by T lymphocytes: at the core of cancer immunotherapy. Nat Rev Cancer. (2014) 14:135–46. doi: 10.1038/nrc3670
16. Stone JD, Harris DT, Kranz DM. TCR affinity for p/MHC formed by tumor antigens that are self-proteins: impact on efficacy and toxicity. Curr Opin Immunol. (2015) 33:16–22. doi: 10.1016/j.coi.2015.01.003
17. Efremova M, Finotello F, Rieder D, Trajanoski Z, Gutenberg-universität J, Gutenberg-universität J. Neoantigens generated by individual mutations and their role in cancer immunity and immunotherapy. Front Immunol. (2017) 8:1679. doi: 10.3389/fimmu.2017.01679
18. Mcgranahan N, Furness AJS, Rosenthal R, Ramskov S, Lyngaa R, Saini SK, et al. Clonal neoantigens elicit T cell immunoreactivity and sensitivity to immune checkpoint blockade. Science. (2016) 351:1463–9. doi: 10.1126/science.aaf1490
19. Hugo W, Zaretsky JM, Sun L, Song C, Moreno BH, Hu-Lieskovan S, et al. Genomic and transcriptomic features of response to anti-PD-1 therapy in metastatic melanoma. Cell. (2016) 165:35–44. doi: 10.1016/j.cell.2016.02.065
20. Van Allen EM, Miao D, Schilling B, Shukla SA, Blank C, Zimmer L, et al. Genomic correlates of response to CTLA-4 blockade in metastatic melanoma. Science. (2015) 350:207–11. doi: 10.1126/science.aad0095
21. Rizvi NA, Hellmann MD, Snyder A, Kvistborg P, Makarov V, Havel JJ, et al. Mutational landscape determines sensitivity to PD-1 blockade in non-small cell lung cancer. Science. (2015) 348:124–8. doi: 10.1126/science.aaa1348
22. Giannakis M, Mu XJ, Shukla SA, Qian ZR, Cohen O, Nishihara R, et al. Genomic correlates of immune-cell infiltrates in colorectal carcinoma. Cell Rep. (2016) 15:857–65. doi: 10.1016/j.celrep.2016.03.075
23. Riaz N, Havel JJ, Makarov V, Desrichard A, Urba WJ, Sims JS, et al. Tumor and microenvironment evolution during immunotherapy with nivolumab. Cell. (2017) 171:934–49.e15. doi: 10.1016/j.cell.2017.09.028
24. Havel JJ, Chowell D, Chan TA. The evolving landscape of biomarkers for checkpoint inhibitor immunotherapy. Nat Rev Cancer. (2019) 19:133–50. doi: 10.1038/s41568-019-0116-x
25. Yost KE, Satpathy AT, Wells DK, Qi Y, Wang C, Kageyama R, et al. Clonal replacement of tumor-specific T cells following PD-1 blockade. Nat Med. (2019) 25:1251–9. doi: 10.1101/648899
26. Luksza M, Riaz N, Makarov V, Balachandran VP, Hellmann MD, Solovyov A, et al. A neoantigen fitness model predicts tumour response to checkpoint blockade immunotherapy. Nature. (2017) 551:517–20. doi: 10.1038/nature24473
27. Richman LP, Vonderheide RH, Rech AJ. Neoantigen dissimilarity to the self-proteome predicts immunogenicity and response to immune checkpoint blockade. Cell Syst. (2019) 9:375–82.e4. doi: 10.1016/j.cels.2019.08.009
28. Santambrogio L, Berendam SJ, Engelhard VH. The antigen processing and presentation machinery in lymphatic endothelial cells. Front Immunol. (2019) 10:1033. doi: 10.3389/fimmu.2019.01033
29. Mellman I, Steinman RM. Dendritic cells: specialized and regulated antigen processing machines. Cell. (2001) 106:255–8. doi: 10.1016/S0092-8674(01)00449-4
30. Blum JS, Wearsch PA, Cresswell P. Pathways of antigen processing. Annu Rev Immunol. (2013) 31:443–73. doi: 10.1146/annurev-immunol-032712-095910
31. Cruz FM, Colbert JD, Merino E, Kriegsman BA, Rock KL. The biology and underlying mechanisms of cross-presentation of exogenous antigens on MHC-I molecules. Annu Rev Immunol. (2017) 35:149–76. doi: 10.1146/annurev-immunol-041015-055254
32. Boltjes A, van Wijk F. Human dendritic cell functional specialization in steady-state and inflammation. Front Immunol. (2014) 5:131. doi: 10.3389/fimmu.2014.00131
33. Dalod M, Chelbi R, Malissen B, Lawrence T. Dendritic cell maturation: functional specialization through signaling specificity and transcriptional programming. EMBO J. (2014) 33:1104–16. doi: 10.1002/embj.201488027
34. Worah K, Mathan TSM, Vu Manh TP, Keerthikumar S, Schreibelt G, Tel J, et al. Proteomics of human dendritic cell subsets reveals subset-specific surface markers and differential inflammasome function. Cell Rep. (2016) 16:2953–66. doi: 10.1016/j.celrep.2016.08.023
35. Stockwin LH, McGonagle D, Martin IG, Blair GE. Dendritic cells: immunological sentinels with a central role in health and disease. Immunol Cell Biol. (2000) 78:91–102. doi: 10.1046/j.1440-1711.2000.00888.x
36. Hancock DG, Guy TV, Shklovskaya E, Fazekas de St Groth B. Experimental models to investigate the function of dendritic cell subsets: challenges and implications. Clin Exp Immunol. (2013) 171:147–54. doi: 10.1111/cei.12027
37. Gutiérrez-Martínez E, Planès R, Anselmi G, Reynolds M, Menezes S, Adiko AC, et al. Cross-presentation of cell-associated antigens by MHC class I in dendritic cell subsets. Front Immunol. (2015) 6:363. doi: 10.3389/fimmu.2015.00363
38. Merad M, Sathe P, Helft J, Miller J, Mortha A. The dendritic cell lineage: ontogeny and function of dendritic cells and their subsets in the steady state and the inflamed setting. Annu Rev Immunol. (2013) 31:563–604. doi: 10.1146/annurev-immunol-020711-074950
39. Jongbloed SL, Kassianos AJ, McDonald KJ, Clark GJ, Ju X, Angel CE, et al. Human CD141+ (BDCA-3)+ dendritic cells (DCs) represent a unique myeloid DC subset that cross-presents necrotic cell antigens. J Exp Med. (2010) 207:1247–60. doi: 10.1084/jem.20092140
40. Xu MM, Pu Y, Han D, Shi Y, Cao X, Liang H, et al. Dendritic cells but not macrophages sense tumor mitochondrial DNA for cross-priming through signal regulatory protein α signaling. Immunity. (2017) 47:363–73.e5. doi: 10.1016/j.immuni.2017.07.016
41. Broz ML, Binnewies M, Boldajipour B, Nelson AE, Pollack JL, Erle DJ, et al. Dissecting the tumor myeloid compartment reveals rare activating antigen-presenting cells critical for T cell immunity. Cancer Cell. (2014) 26:638–52. doi: 10.1016/j.ccell.2014.09.007
42. Hammerich L, Marron TU, Upadhyay R, Svensson-arvelund J, Dhainaut M, Hussein S, et al. Systemic clinical tumor regressions and potentiation of PD1 blockade with in situ vaccination. Nat Med. (2019) 25:814–24. doi: 10.1038/s41591-019-0410-x
43. Salmon H, Remark R, Gnjatic S, Merad M. Host tissue determinants of tumour immunity. Nat Rev Cancer. (2019) 19:215–27.
44. Ott PA, Hu Z, Keskin DB, Shukla SA, Sun J, Bozym DJ, et al. An immunogenic personal neoantigen vaccine for patients with melanoma. Nature. (2017) 547:217–21. doi: 10.1038/nature22991
45. Hilf N, Kuttruff-Coqui S, Frenzel K, Bukur V, Stevanović S, Gouttefangeas C, et al. Actively personalized vaccination trial for newly diagnosed glioblastoma. Nature. (2019) 565:240–5. doi: 10.1038/s41586-018-0810-y
46. Temizoz B, Kuroda E, Ishii KJ. Vaccine adjuvants as potential cancer immunotherapeutics. Int Immunol. (2016) 28:329–38. doi: 10.1093/intimm/dxw015
47. Kranz LM, Diken M, Haas H, Kreiter S, Loquai C, Reuter KC, et al. Systemic RNA delivery to dendritic cells exploits antiviral defence for cancer immunotherapy. Nature. (2016) 534:396–401. doi: 10.1038/nature18300
48. Klinman DM. Immunotherapeutic uses of CpG oligodeoxynucleotides. Nat Rev Immunol. (2004) 4:249–59. doi: 10.1038/nri1329
49. Kinkead HL, Jaffee EM, Zaidi N, Kinkead HL, Hopkins A, Lutz E, et al. Combining STING-based neoantigen-targeted vaccine with checkpoint modulators enhances antitumor immunity in murine pancreatic cancer. JCI Insight. (2018) 3:122857. doi: 10.1172/jci.insight.122857
50. Scheel B, Teufel R, Probst J, Carralot J-P, Geginat J, Radsak M, et al. Toll-like receptor-dependent activation of several human blood cell types by protamine-condensed mRNA. Eur J Immunol. (2005) 35:1557–66. doi: 10.1002/eji.200425656
51. Sahin U, Derhovanessian E, Miller M, Kloke B-P, Simon P, Löwer M, et al. Personalized RNA mutanome vaccines mobilize poly-specific therapeutic immunity against cancer. Nature. (2017) 547:222–6. doi: 10.1038/nature23003
52. Tullett KM, Leal Rojas IM, Minoda Y, Tan PS, Zhang J-G, Smith C, et al. Targeting CLEC9A delivers antigen to human CD141(+) DC for CD4(+) and CD8(+) T cell recognition. JCI Insight. (2016) 1:e87102. doi: 10.1172/jci.insight.87102
53. Linette GP, Carreno BM. Neoantigen vaccines pass the immunogenicity test. Trends Mol Med. (2017) 23:869–71. doi: 10.1016/j.molmed.2017.08.007
55. Boudreau JE, Bonehill A, Thielemans K, Wan Y. Engineering dendritic cells to enhance cancer immunotherapy. Mol Ther. (2011) 19:841–53. doi: 10.1038/mt.2011.57
56. Selmi A, Vascotto F, Kautz-Neu K, Türeci Ö, Sahin U, von Stebut E, et al. Uptake of synthetic naked RNA by skin-resident dendritic cells via macropinocytosis allows antigen expression and induction of T-cell responses in mice. Cancer Immunol Immunother. (2016) 65:1075–83. doi: 10.1007/s00262-016-1869-7
57. Binnewies M, Mujal AM, Pollack JL, Ye CJ, Roberts EW, Krummel MF, et al. Unleashing type-2 dendritic cells to drive protective antitumor CD4+ T cell immunity. Cell. (2019) 177:556–71.e16. doi: 10.1016/j.cell.2019.02.005
58. Carreno BM, Magrini V, Becker-Hapak M, Hundal J, Ly A, Linette GP. A dendritic cell vaccine increases the breadth and diversity of melanoma neoantigen-specific T cells. Science. (2015) 348:803–8. doi: 10.1126/science.aaa3828
59. Emerson R, Chapuis AG, Desmarais C, Lai IP, Roberts IM, Yee C, et al. Tracking the fate and origin of clinically relevant adoptively transferred CD8 + T cells in vivo. Sci Immunol. (2017) 2:eaal2568. doi: 10.1126/sciimmunol.aal2568
60. Yamaguchi N, Winter CM, Wu MF, Kanno Y, Yamaguchi A, Seo M, et al. Cancer immunotherapy based on mutation-specific CD4+ T cells in a patient with epithelial cancer. Science. (2014) 9:641–6. doi: 10.1126/science.1251102
61. Tran E, Robbins PF, Lu Y-C, Prickett TD, Gartner JJ, Jia L, et al. T-cell transfer therapy targeting mutant KRAS in cancer. N Engl J Med. (2016) 375:2255–62. doi: 10.1056/NEJMoa1609279
62. Balachandran VP, Luksza M, Zhao JN, Makarov V, Moral JA, Remark R, et al. Identification of unique neoantigen qualities in long-term survivors of pancreatic cancer. Nature. (2017) 551:S12–6. doi: 10.1038/nature24462
63. Zhang J, Caruso FP, Sa JK, Justesen S, Nam D, Sims P, et al. The combination of neoantigen quality and T lymphocyte infiltrates identifies glioblastomas with the longest survival. Commun Biol. (2019) 2:135. doi: 10.1038/s42003-019-0369-7
64. Wood MA, Paralkar M, Paralkar MP, Nguyen A, Struck AJ, Ellrott K, et al. Population-level distribution and putative immunogenicity of cancer neoepitopes. BMC Cancer. (2018) 18:414. doi: 10.1186/s12885-018-4325-6
65. Turajlic S, Litchfield K, Xu H, Rosenthal R, McGranahan N, Reading JL, et al. Insertion-and-deletion-derived tumour-specific neoantigens and the immunogenic phenotype: a pan-cancer analysis. Lancet Oncol. (2017) 18:1009–21. doi: 10.1016/S1470-2045(17)30516-8
66. Spranger S, Luke JJ, Bao R, Zha Y, Hernandez KM, Li Y, et al. Density of immunogenic antigens does not explain the presence or absence of the T-cell-inflamed tumor microenvironment in melanoma. Proc Natl Acad Sci USA. (2016) 113:E7759–68. doi: 10.1073/pnas.1609376113
67. Hellmann M. Mutation burden, neoantigens, and response to T cell checkpoint blockade. TAT Conf Proc. (2016).
68. Charoentong P, Angelova M, Charoentong P, Finotello F, Angelova M, Mayer C, et al. Pan-cancer immunogenomic analyses reveal genotype-immunophenotype relationships and predictors of response to checkpoint blockade: cell reports. Cell Rep. (2017) 18:248–62. doi: 10.1016/j.celrep.2016.12.019
69. Blank C, Haanen J, Ribas A, Schumacher T. The cancer immunogram. Science. (2016) 352:658–60. doi: 10.1126/science.aaf2834
70. Bräunlein E, Krackhardt AM. Identification and characterization of neoantigens as well as respective immune responses in cancer patients. Front Immunol. (2017) 8:1702. doi: 10.3389/fimmu.2017.01702
71. Rosenthal R, Cadieux EL, Salgado R, Bakir M Al, Moore DA, Hiley CT, et al. Neoantigen-directed immune escape in lung cancer evolution. Nature. (2019) 567:479–85. doi: 10.1038/s41586-019-1032-7
72. Marty R, Kaabinejadian S, Rossell D, Slifker MJ, Haar J van de, Engin HB, et al. MHC-I genotype restricts the oncogenic mutational landscape. Cell. (2017) 171:1–12.e15. doi: 10.1016/j.cell.2017.09.050
73. Auslander N, Zhang G, Lee JS, Frederick DT, Miao B, Moll T, et al. Robust prediction of response to immune checkpoint blockade therapy in metastatic melanoma. Nat Med. (2018) 24:1545–9. doi: 10.1038/s41591-018-0247-8
74. Mlecnik B, Bindea G, Angell HK, Maby P, Angelova M, Tougeron D, et al. Integrative analyses of colorectal cancer show immunoscore is a stronger predictor of patient survival than microsatellite instability. Immunity. (2016) 44:698–711. doi: 10.1016/j.immuni.2016.02.025
75. Senft D, Leiserson MDM, Ruppin E, Ronai ZA. Precision oncology: the road ahead. Trends Mol Med. (2017) 23:874–98. doi: 10.1016/j.molmed.2017.08.003
76. Snyder A, Chan TA. Immunogenic peptide discovery in cancer genomes. Curr Opin Genet Dev. (2015) 30:7–16. doi: 10.1016/j.gde.2014.12.003
77. Roudko V, Bozkus CC, Orfanelli T, Blank SV. Widespread immunogenic poly-epitope frameshift mutations in microsatellite unstable tumors. bioRvix. (2019) 1–53. doi: 10.1101/662262
78. Schwitalle Y, Kloor M, Eiermann S, Linnebacher M, Kienle P, Knaebel HP, et al. Immune response against frameshift-induced neopeptides in HNPCC patients and healthy HNPCC mutation carriers. Gastroenterology. (2008) 134:988–97. doi: 10.1053/j.gastro.2008.01.015
79. Editorial. The problem with neoantigen prediction. Nat Biotechnol. (2017) 35:97. doi: 10.1038/nbt.3800
80. Robbins PF, Lu YC, El-Gamil M, Li YF, Gross C, Gartner J, et al. Mining exomic sequencing data to identify mutated antigens recognized by adoptively transferred tumor-reactive T cells. Nat Med. (2013) 19:747–52. doi: 10.1038/nm.3161
81. Rosato PC, Wijeyesinghe S, Stolley JM, Nelson CE, Davis RL, Manlove LS, et al. Virus-specific memory T cells populate tumors and can be repurposed for tumor immunotherapy. Nat Commun. (2019) 10:567. doi: 10.1038/s41467-019-08534-1
82. Hoyos LE, Abdel-Wahab O. Cancer-specific splicing changes and the potential for splicing-derived neoantigens. Cancer Cell. (2018) 34:181–3. doi: 10.1016/j.ccell.2018.07.008
83. Kahles A, Lehmann K Van, Toussaint NC, Hüser M, Stark SG, Sachsenberg T, et al. Comprehensive analysis of alternative splicing across tumors from 8,705 patients. Cancer Cell. (2018) 34:211–24.e6. doi: 10.1016/j.ccell.2018.07.001
84. Smart A, Margolis C, Pimentel H, He MX, Miao D, Adeegbe D, et al. Intron retention as a novel source of cancer neoantigens. bioRxiv. (2018). doi: 10.1101/309450
85. Yadav M, Jhunjhunwala S, Phung QT, Lupardus P, Tanguay J, Bumbaca S, et al. Predicting immunogenic tumour mutations by combining mass spectrometry and exome sequencing. Nature. (2014) 515:572–6. doi: 10.1038/nature14001
86. Doyle HA, Mamula MJ. Post-translational protein modifications in antigen recognition and autoimmunity. Trends Immunol. (2001) 22:443–9. doi: 10.1016/S1471-4906(01)01976-7
87. Engelhard VH, Altrich-Vanlith M, Ostankovitch M, Zarling AL. Post-translational modifications of naturally processed MHC-binding epitopes. Curr Opin Immunol. (2006) 18:92–7. doi: 10.1016/j.coi.2005.11.015
88. Malaker SA, Penny SA, Steadman LG, Myers PT, Loke JC, Raghavan M, et al. Identification of glycopeptides as posttranslationally modified neoantigens in leukemia. Cancer Immunol. Res. (2017) 5:376–84. doi: 10.1158/2326-6066.CIR-16-0280
89. Raposo B, Merky P, Lundqvist C, Yamada H, Urbonaviciute V, Niaudet C, et al. T cells specific for post-translational modifications escape intrathymic tolerance induction. Nat Commun. (2018) 9:353. doi: 10.1038/s41467-017-02763-y
90. Purcell AW. Mass spectrometry – based identification of MHC-bound peptides for immunopeptidomics. Nat Protoc. (2019) 14:1687–707. doi: 10.1038/s41596-019-0133-y
91. Meena N, Mathur P, Medicherla KM, Suravakhala P. A bioinformatics pipeline for whole exome sequencing: overview of the processing and steps from raw data to downstream analysis. bioRvix. (2017). doi: 10.1101/201145
92. Hintzsche JD, Robinson WA, Tan AC. A survey of computational tools to analyze and interpret whole exome sequencing data. Int J Genomics. (2016) 2016:7983236. doi: 10.1155/2016/7983236
93. Leggett RM, Ramirez-Gonzalez RH, Clavijo BJ, Waite D, Davey RP. Sequencing quality assessment tools to enable data-driven informatics for high throughput genomics. Front Genet. (2013) 4:288. doi: 10.3389/fgene.2013.00288
94. Wingett SW, Andrews S. FastQ screen: a tool for multi-genome mapping and quality control. F1000Research. (2018) 7:1338. doi: 10.12688/f1000research.15931.1
96. Li H, Durbin R. Fast and accurate long-read alignment with Burrows-Wheeler transform. Bioinformatics. (2010) 26:589–95. doi: 10.1093/bioinformatics/btp698
97. Langmead B. Aligning short sequencing reads with Bowtie. Curr Protoc Bioinforma. (2010) Chapter 11:Unit 11.7. doi: 10.1002/0471250953.bi1107s32
98. Dobin A, Davis CA, Schlesinger F, Drenkow J, Zaleski C, Jha S, et al. STAR: ultrafast universal RNA-seq aligner. Bioinformatics. (2013) 29:15–21. doi: 10.1093/bioinformatics/bts635
99. DePristo MA, Banks E, Poplin R, Garimella KV, Maguire JR, Hartl C, et al. A framework for variation discovery and genotyping using next-generation DNA sequencing data. Nat Genet. (2011) 43:491–8. doi: 10.1038/ng.806
100. Cibulskis K, Lawrence MS, Carter SL, Sivachenko A, Jaffe D, Sougnez C, et al. Sensitive detection of somatic point mutations in impure and heterogeneous cancer samples. Nat Biotechnol. (2013) 31:213–9. doi: 10.1038/nbt.2514
101. Koboldt DC, Larson DE, Wilson RK. Using varscan 2 for germline variant calling and somatic mutation detection. Curr Protoc Bioinforma. (2013) 44:15.4.1–17. doi: 10.1002/0471250953.bi1504s44
102. Lai Z, Markovets A, Ahdesmaki M, Chapman B, Hofmann O, McEwen R, et al. VarDict: a novel and versatile variant caller for next-generation sequencing in cancer research. Nucleic Acids Res. (2016) 75:4864. doi: 10.1158/1538-7445.AM2015-4864
103. Larson DE, Harris CC, Chen K, Koboldt DC, Abbott TE, Dooling DJ, et al. Somaticsniper: identification of somatic point mutations in whole genome sequencing data. Bioinformatics. (2012) 28:311–7. doi: 10.1093/bioinformatics/btr665
104. Xu F, Wang W, Wang P, Jun Li M, Chung Sham P, Wang J. A fast and accurate SNP detection algorithm for next-generation sequencing data. Nat Commun. (2012) 3:1258. doi: 10.1038/ncomms2256
105. Jennings LJ, Arcila ME, Corless C, Kamel-reid S, Lubin IM, Pfeifer J, et al. Guidelines for validation of next-generation sequencing e based oncology panels A joint consensus recommendation of the association for molecular pathology and college of american pathologists. J Mol Diagnostics. (2017) 19:341–65. doi: 10.1016/j.jmoldx.2017.01.011
106. Clark M, Chen R, Lam H, Karczewski K, Chen R, Euskirchen G, et al. Performance comparison of exome DNA sequencing technologies. Nat Biotechnol. (2014) 29:908–14. doi: 10.1038/nbt.1975
107. Denroche RE, Mullen L, Timms L, Beck T, Yung CK, Stein L, et al. A cancer cell-line titration series for evaluating somatic classification. BMC Res Notes. (2015) 8:823. doi: 10.1186/s13104-015-1803-7
108. Warden CD, Adamson AW, Neuhausen SL, Wu X. Detailed comparison of two popular variant calling packages for exome and targeted exon studies. PeerJ. (2014) 2:e600. doi: 10.7717/peerj.600
109. Alioto TS, Buchhalter I, Derdak S, Hutter B, Eldridge MD, Hovig E, et al. A comprehensive assessment of somatic mutation detection in cancer using whole-genome sequencing. Nat Commun. (2015) 6:10001. doi: 10.1038/ncomms10001
112. Meyer J, Whitehorn H, Kits H, Adapters KD, Beads KP. KAPA HyperPrep Kits offer a flexible, high-efficiency library preparation solution for PCR-free human whole-genome sequencing. Roche (2018).
113. Wang VG, Kim H, Chuang JH. Whole-exome sequencing capture kit biases yield false negative mutation calls in TCGA cohorts. PLoS ONE. (2018) 13:e0204912. doi: 10.1371/journal.pone.0204912
114. Wang Q, Shashikant CS, Jensen M, Altman NS, Girirajan S. Novel metrics to measure coverage in whole exome sequencing datasets reveal local and global non-uniformity. Sci Rep. (2017) 7:885. doi: 10.1038/s41598-017-01005-x
115. Chilamakuri CSR, Lorenz S, Madoui M, Vodák D, Sun J, Hovig E, et al. Performance comparison of four exome capture systems for deep sequencing. BMC Genomics. (2014) 15:449. doi: 10.1186/1471-2164-15-449
116. Shigemizu D, Momozawa Y, Abe T, Morizono T, Boroevich KA, Takata S, et al. Performance comparison of four commercial human whole-exome capture platforms. Sci Rep. (2015) 5:1–8. doi: 10.1038/srep12742
117. Warr A, Robert C, Hume D, Archibald A, Deeb N, Watson M. Exome sequencing : current and future perspectives. G3. (2015) 5:1543–50. doi: 10.1534/g3.115.018564
118. Lazaro A, Tu B, Yang R, Xiao Y, Kariyawasam K, Ng J, et al. Human leukocyte antigen (HLA) typing by DNA sequencing. Methods Mol Biol. (2013) 1034:161–95. doi: 10.1007/978-1-62703-493-7_9
119. Bai Y, Ni M, Cooper B, Wei Y, Fury W. Inference of high resolution HLA types using genome-wide RNA or DNA sequencing reads. BMC Genomics. (2014) 15:1–16. doi: 10.1186/1471-2164-15-325
120. Boegel S, Löwer M, Schäfer M, Bukur T, Graaf J De, Boisguérin V, et al. HLA typing from RNA-Seq sequence reads. Genome Med. (2013) 4:102. doi: 10.1186/gm403
121. Schubert B, Mohr C, Sturm M, Feldhahn M, Kohlbacher O. OptiType : precision HLA typing from next-generation sequencing data. Bioinformatics. (2014) 30:3310–6. doi: 10.1093/bioinformatics/btu548
122. Shukla SA, Rooney MS, Rajasagi M, Tiao G, Philip M, Steelman S, et al. Comprehensive analysis of cancer-associated somatic mutations in class I HLA genes. Nat Biotechnol. (2016) 33:1152–8. doi: 10.1038/nbt.3344
123. Duquesnoy J. HLAMatchmaker : a molecularly based algorithm for histocompatibility determination. Hum Immunol. (2002) 63:339–52. doi: 10.1016/S0198-8859(02)00382-8
124. Huang Y, Yang J, Ying D, Zhang Y, Shotelersuk V, Hirankarn N, et al. HLAreporter: a tool for HLA typing from next generation sequencing data. Genome Med. (2015) 7:1–12. doi: 10.1186/s13073-015-0145-3
125. Kim HJ, Pourmand N. HLA haplotyping from RNA-seq data using hierarchical read weighting. PLoS ONE. (2013) 8:e67885. doi: 10.1371/journal.pone.0067885
126. Warren RL, Choe G, Freeman DJ, Castellarin M, Munro S, Moore R, et al. Derivation of HLA types from shotgun sequence datasets. Genome Med. (2012) 4:95. doi: 10.1186/gm396
127. Xie C, Xuan Z, Wong M, Piper J, Long T, Kirkness EF, et al. Fast and accurate HLA typing from short-read next-generation sequence data with xHLA. PNAS. (2017) 114:8059–64. doi: 10.1073/pnas.1707945114
128. Kiyotani K, Mai TH, Nakamura Y. Comparison of exome-based HLA class I genotyping tools: identification of platform-specific genotyping errors. J Hum Genet. (2016) 62:1–9. doi: 10.1038/jhg.2016.141
129. Kuball J, Hauptrock B, Malina V, Antunes E, Voss R-H, Wolfl M, et al. Increasing functional avidity of TCR-redirected T cells by removing defined N -glycosylation sites in the TCR constant domain. J Exp Med. (2009) 206:463–75. doi: 10.1084/jem.20082487
130. Schmitt TM, Stromnes IM, Chapuis AG, Greenberg PD. New strategies in engineering T-cell receptor gene-modified T cells to more effectively target malignancies. Clin Cancer Res. (2015) 21:5191–7. doi: 10.1158/1078-0432.CCR-15-0860
131. Klebanoff CA, Rosenberg SA, Restifo NP. Prospects for gene-engineered T cell immunotherapy for solid cancers. Nat Med. (2016) 22:26–36. doi: 10.1038/nm.4015
132. Zhang S-Q, Ma K-Y, Schonnesen AA, Zhang M, He C, Sun E, et al. High-throughput determination of the antigen specificities of T cell receptors in single cells. bioRxiv. (2018) 457069. doi: 10.1101/457069
133. Cogen Therapeutics. Available online at: http://cogentherapeutics.com
134. Sibener LV, Fernandes RA, Kolawole EM, Carbone CB, Liu F, McAffee D, et al. Isolation of a structural mechanism for uncoupling T cell receptor signaling from peptide-MHC binding. Cell. (2018) 174:672–87.e27. doi: 10.1016/j.cell.2018.06.017
135. Abelin JG, Keskin DB, Sarkizova S, Hacohen N, Rooney MS, Carr SA, et al. Mass spectrometry profiling of HLA-associated peptidomes in mono-allelic cells enables more accurate epitope prediction. Immunity. (2017) 46:315–26. doi: 10.1016/j.immuni.2017.02.007
136. Olaf R, Falk K, Deres K, Schild H, Norda M, Metzger J, et al. Isolation and analysis of naturally processed viral peptides as recognized by cytotoxic T cells. Nature. (1990) 348:252–4. doi: 10.1038/348252a0
137. Hunt DF, Henderson RA, Shabanowitz J, Sakaguchi K, Michel H, Sevilir N, et al. Characterization of peptides bound to the Class I MHC molecule HLA-A2.1 by Mass Spectrometry. Science. (1992) 255:1–4. doi: 10.1126/science.1546328
138. Bassani-Sternberg M, Bräunlein E, Klar R, Engleitner T, Sinitcyn P, Audehm S, et al. Direct identification of clinically relevant neoepitopes presented on native human melanoma tissue by mass spectrometry. Nat Commun. (2016) 7:13404. doi: 10.1038/ncomms13404
139. Newey A, Griffiths B, Michaux J, Pak HS, Stevenson BJ, Woolston A, et al. Immunopeptidomics of colorectal cancer organoids reveals a sparse HLA class I neoantigen landscape and no increase in neoantigens with interferon or MEK- inhibitor treatment. J Immunother Cancer. (2019) 8:1–15. doi: 10.1186/s40425-019-0769-8
140. Chong C, Müller M, Pak H, Harnett D, Huber F, Grun D, et al. Integrated proteogenomic deep sequencing and analytics accurately identify non-canonical peptides in tumor immunopeptidomes. bioRxiv. (2019) 758680.
141. Axelrod ML, Cook RS, Johnson DB, Balko JM. Biological consequences of MHC-II expression by tumor cells in cancer. Clin Cancer Res. (2019) 25:2392–402. doi: 10.1158/1078-0432.CCR-18-3200
142. Alspach E, Lussier DM, Miceli AP, Kizhvatov I, DuPage M, Luoma AM, et al. MHC-II neoantigens shape tumour immunity and response to immunotherapy. Nature. (2019) 574:696–701. doi: 10.1038/s41586-019-1671-8
143. Nielsen M, Lund O, Buus S, Lundegaard C. MHC Class II epitope predictive algorithms. Immunology. (2010) 130:319–28. doi: 10.1111/j.1365-2567.2010.03268.x
144. Garboczi DN, Hung DT, Wiley DONC. HLA-A2-peptide complexes: Refolding and crystallization of molecules expressed in Eschericlhia coli and complexed with single antigenic peptides. PNAS. (1992) 89:3429–33. doi: 10.1073/pnas.89.8.3429
145. Fahnestock ML, Tamir I, Narhi L, Bjorkman PJ. Thermal stability comparison of purified empty and peptide-filled forms of a class I MHC molecule. Science. (1992) 258:1658–62. doi: 10.1126/science.1360705
146. Toebes M, Coccoris M, Bins A, Rodenko B, Gomez R, Nieuwkoop NJ, et al. Design and use of conditional MHC class I ligands. Nat Med. (2006) 12:246–51. doi: 10.1038/nm1360
147. Rodenko B, Toebes M, Hadrup SR, Esch WJE Van, Molenaar AM, Schumacher TNM, et al. Generation of peptide-MHC class I complexes through UV-mediated ligand exchange. Nat Protoc. (2006) 1:1120–32. doi: 10.1038/nprot.2006.121
148. Ali M, Giannakopoulou E, Böschen M, Strønen E, Yang W, Toebes M, et al. Induction of neoantigen-reactive T cells from healthy donors. Nat Protoc. (2019) 14:1926–43 doi: 10.1038/s41596-019-0170-6
149. Gee MH, Han A, Lofgren SM, Beausang JF, Mendoza JL, Birnbaum ME, et al. Antigen identification for orphan T cell receptors expressed on tumor-infiltrating lymphocytes. Cell. (2017) 172:549–56.e16. doi: 10.1016/j.cell.2017.11.043
150. Schmitt TM, Aggen DH, Ishida-Tsubota K, Ochsenreither S, Kranz DM, Greenberg PD. Generation of higher affinity T cell receptors by antigen-driven differentiation of progenitor t cells in vitro. Nat Biotechnol. (2017) 35:1188–95. doi: 10.1038/nbt.4004
151. Klein L, Kyewski B, Allen PM, Hogquist KA. Positive and negative selection of the T cell repertoire: what thymocytes see and don't see. Nat Rev Immunol. (2014) 14:377–91. doi: 10.1038/nri3667
152. Obenaus M, Leitão C, Leisegang M, Chen X, Gavvovidis I, Van Der Bruggen P, et al. Identification of human T-cell receptors with optimal affinity to cancer antigens using antigen-negative humanized mice. Nat Biotechnol. (2015) 33:402–7. doi: 10.1038/nbt.3147
153. Hu Z, Anandappa AJ, Sun J, Kim J, Leet DE, David J, et al. A cloning and expression system to probe T cell receptor specificity and assess functional avidity to neoantigens. Blood. (2018) 132:1911–21. doi: 10.1182/blood-2018-04-843763
154. Wang CI, Wucherpfennig KW, Lyerly HK, Elledge SJ, Kula T, Dezfulian MH, et al. T-scan: a genome-wide method for the systematic discovery of T cell epitopes. Cell. (2019) 178:1016–28.e13. doi: 10.1016/j.cell.2019.07.009
155. Baker RG, Hoos AX, Adam SJ, Wholley D, Doroshow J, Lowy D, et al. The partnership for accelerating cancer therapies. Cancer J. (2018) 24:111–4. doi: 10.1097/PPO.0000000000000321
156. Yamamoto TN, Kishton RJ, Restifo NP. Developing neoantigen-targeted T cell–based treatments for solid tumors. Nat Med. (2019) 25:1488–99. doi: 10.1038/s41591-019-0596-y
157. Vollers SS, Stern LJ. Class II major histocompatibility complex tetramer staining: progress, problems, and prospects. Immunology. (2008) 123:305–13. doi: 10.1111/j.1365-2567.2007.02801.x
Keywords: neoantigen, TCR, WES, HLA-allele, MHC-I epitope
Citation: Roudko V, Greenbaum B and Bhardwaj N (2020) Computational Prediction and Validation of Tumor-Associated Neoantigens. Front. Immunol. 11:27. doi: 10.3389/fimmu.2020.00027
Received: 23 August 2019; Accepted: 08 January 2020;
Published: 24 January 2020.
Edited by:
Peter Brossart, University of Bonn, GermanyReviewed by:
Zsolt Sebestyen, University Medical Center Utrecht, NetherlandsJuliane Sarah Walz, Tübingen University Hospital, Germany
Copyright © 2020 Roudko, Greenbaum and Bhardwaj. This is an open-access article distributed under the terms of the Creative Commons Attribution License (CC BY). The use, distribution or reproduction in other forums is permitted, provided the original author(s) and the copyright owner(s) are credited and that the original publication in this journal is cited, in accordance with accepted academic practice. No use, distribution or reproduction is permitted which does not comply with these terms.
*Correspondence: Nina Bhardwaj, nina.bhardwaj@mssm.edu