- 1Department of Medical Genomics, GENYO, Center for Genomics and Oncological Research Pfizer, University of Granada, Granada, Spain
- 2Unit of Chronic Inflammation, Institute for Environmental Medicine, Karolinska Institute, Stockholm, Sweden
The importance of low frequency and rare variation in complex disease genetics is difficult to estimate in patient populations. Genome-wide association studies are therefore, underpowered to detect rare variation. We have used a combined approach of genome-wide-based imputation with a highly stringent sequence kernel association (SKAT) test and a case-control burden test. We identified 98 candidate genes containing rare variation that in aggregate show association with SLE many of which have recognized immunological function, but also function and expression related to relevant tissues such as the joints, skin, blood or central nervous system. In addition we also find that there is a significant enrichment of genes annotated for disease-causing mutations in the OMIM database, suggesting that in complex diseases such as SLE, such mutations may be involved in subtle or combined phenotypes or could accelerate specific organ abnormalities found in the disease. We here provide an important resource of candidate genes for SLE.
Introduction
Genome-wide association studies have been designed primarily to capture common variation and so far some 10,000 common genetic variants have been robustly associated with a wide range of complex diseases (1). Therefore, this methodology is underpowered to detect the effects of rare variants. There has been much debate as to the role of rare genetic variation on complex traits (2–4) and how rare variant studies complement GWASs (5). It is now accepted that rare variants located in different genes could in fact play a more important role in disease susceptibility than common variants (4). The challenge arises in measuring and statistically analyzing rare variation. It would be very unexpected to find rare variants that could have substantial effect sizes and therefore high penetrance contributing to complex traits, being more likely to have mutations with modest effects. For small effect sizes association testing may require composite tests of overall “mutational load,” pooling rare variants for analysis by addressing the question: do rare variants increase or decrease disease risk? (6). In-depth whole-genome sequencing is the most comprehensive approach for measuring rare and common variation in both coding and non-coding regions. However, nowadays, its application is limited by the costs and various computational challenges, especially for large-scale cohorts. Whole-exome sequencing is a cost-effective alternative, however one of its obvious drawback is the absence of variants in non-coding regions, which may be especially relevant in the context of complex disease genetics. Genotype imputation is likely to remain a valuable tool. At this point an interesting cost and a computationally effective alternative would be to combine genotype imputation with targeted sequencing in a gene-centered strategy. For dense genotyping arrays, imputation is able to predict nearly all missing common variation with high accuracy, but as the variant minor allele frequency (MAF) decreases, so does the accuracy of imputation, depending mainly on the size of the reference panel and the ancestry of the imputed samples, with the best efficiencies in European cohorts, mainly due to the sufficiently large size of the European reference panels (7–13).
We have previously tested the overall effect of imputed rare variation on particular genes in systemic autoimmunity (14). Recently, we have implemented and successfully applied a method based on genotype imputation of rare variation, on a set of genes detected by exome sequencing as possible candidates for association to systemic lupus erythematosus (SLE) by mutation in members of Icelandic SLE-multicase families (15).
In the present work the method has been brought to the genome level to scan for association of protein coding genes with SLE by rare variation in the European ancestry population. We executed stringent imputation in a densely genotyped set for analyzing association with SLE in a sample from the European population selecting the protein coding genes of the genome and then applying tests to detect those that significantly associated with the disease by rare-variation. This procedure provided a set of 98 genes as good candidates for association with SLE by mutation. Many of these genes showed immunological related functions or effects on other organs or tissues affected by the pathology, such as joints, skin, central nervous system or blood and some were involved in human energy metabolism and more specifically as part of the respiratory chain. Such a diversity of functions is to be expected because of the phenotypic heterogeneity of a complex disease as SLE.
Materials and Methods
Genome-Wide Association Analysis
We used GWAS data from 5,478 individuals of European ancestry including 4254 SLE patients and 1,224 controls genotyped using the Illumina HumanOmni1_Quad_v1-0_B chip. In order to increase the number of controls, additional data from European subjects were obtained from the dbGaP database (http://www.ncbi.nlm.nih.gov/gap) including the DCEG Imputation Reference Dataset (phs000396.v1.p1) with 1,175 individuals representing general population, and controls subjects from two case-control studies: 1,047 from the High Density SNP Association Analysis of Melanoma (phs000187.v1.p1) and 903 from GENIE UK-ROI Diabetic Nephropathy GWAS (phs000389.v1.p1). Note that only control from both case-control studies were included in our analysis. In total, the initial dataset consisted of 4,254 SLE patients and 4,349 controls.
In order to obtain a quality-controlled working dataset satisfying current state-of-the-art criteria for association studies, data filtering was conducted using PLINK v1.07 1 (16) applying the following criteria: minimum total call rate per sample of 90%, minimum call rate per marker of 98%, minor allele frequency (MAF) threshold of 1%, Hardy-Weinberg Equilibrium (HWE) p-value for cases and controls at a minimum of 0.0001, and in addition at 0.01 only for controls, and finally a cutoff p-value of 0.00001 for differential missingness in, the software REAP was used (17) applying a kinship coefficient threshold < 0.055. To correct for stratification, principal component analysis (PCA) was performed with smartpca, EIGENSOFT 4.0 beta package 2 (18). To confirm the European ancestry we ran a PCA with the set of independent markers (r2 < 0.1) that maximized differences in allelic frequencies between the four main 1000 Genomes subpopulations (EUR, AFR, AMR, and ASN). No samples were detected as “non-European” (Supplemental Figure 1). Next, the PCA was performed with the set of r2 < 0.1, that maximized differences in the allelic frequencies in 1000 Genomes EUR subpopulations (CEU, GBR, IBS, TSI, and FIN) and two additional subpopulations from our sample dataset, Greek and Turkish (GRK and TUR) representing the south-eastern European ancestry. The resulting PCs perfectly classified the individuals from reference populations by their geographical origin (Supplemental Figure 2). This last set of PCs was used for correcting for genetic stratification in the case-control association analysis (Supplemental Figure 3). This resulted in a λGC = 1.05 using the first 10 principal components. The final data set used for association analysis consisted of 4,212 cases and 4,065 controls.
Imputation
Release 19 (GRCh37.p13) was used as reference (https://www.encodeproject.org/files/gencode.v19.annotation/). Of 19,430 sequences annotated as “protein coding” in the gencode.v19.annotation.gtf file, 15,763 included in the final QC-filtered genotyping data set became our imputation working gene list. Each protein-coding gene region was extended 500,000 additional base pairs upstream and downstream, respectively, as it is known that large buffers may improve accuracy for low-frequency variants during imputation. Markers within each extended region were extracted from the GWAS data for imputation with IMPUTE2 (19) using the 1000 Genomes Project as reference panel. Specifically, we used 1000 Genomes Phase 3, b37 (October 2014), as these haplotypes have lower genotype discordance and improved imputation performance into downstream GWAS samples, especially for low frequency variants (20, 21). Genome-Wide Association Analysis of Imputed Rare Variants in complex diseases has been previously used as a gene-centered approach (7). In this paper imputation into a GWAS scaffold using the WTCCC European analysis cohort explicitly showed substantial gains in power to detect rare variant association within the gene where the extent of the increase in power depends crucially on the number of individuals in the reference panel. Therefore, power gains obtained from 500 to 4,000 samples in the reference panel were not as great as from 120 to 500 samples. Based on this, and for the objective of our study we considered as adequate the 2,504 samples present in the 1000 Genomes phase 3 reference panel (2014 release, http://mathgen.stats.ox.ac.uk/impute/1000GP_Phase3/) of which 503 are of European descent. Prior to imputation, each GWAS gene extended region was phased with SHAPEIT using the 1000 Genomes EUR subpopulation as reference (http://www.shapeit.fr/). A restrictive QC-filter was applied on the imputed genotypes (SNP genotyping rate ≥ 99%, sample genotyping rate ≥ 95%) without restriction of allele frequencies, in order to include both rare and low frequency variants. To ensure a highly reliable imputation, a conservative IMPUTE info_value threshold of ≥ 0.75 for each marker were applied as imputation quality score.
Functional Annotation of Genetic Variants
Annotation of analyzed genetic variants in their different functional categories was carried out using ANNOVAR (22).
Gene Case-Control Association Analysis by Rare Variation
While there is no universally accepted definition of “rare variant,” and a minor allele frequency (MAF) of 1% is the conventional definition of polymorphism, then a MAF < 1% would be understood as “rare variation.” We tested whether any of the N genes in the human genome had statistical evidence of association with SLE in the general European population due to the combined effect of all rare variation within each gene (MAF < 1%). Each gene was analyzed using two procedures: the sequence kernel association (SKAT) test (20, 21) and a case-control burden test by adjusting a logistic regression model with a “transformed” “genetic variable” equals to the sum of minor frequency alleles for all markers below the (< 1%) in the tested gene in each i individual (7). To note that in such case-control burden test, a result statistically significant indicates that the overall effect of rare variation on the gene goes in the same direction being either of risk (aggregate odd ratio > 1) or alternatively protective (aggregate odd ratio < 1). This feature of case-control burden analysis helps to interpret the effect of rare variation on the phenotype. In addition, running two association procedures, SKAT ⋂ case-control burden test would reduce the rate of false positives. Thus, we will consider as true positives those genes with significant association test for both procedures, SKAT and case-control burden test. However, in association tests that simultaneously include several markers, one effect of linkage disequilibrium (LD) between these markers could be collinearity. We have addressed the LD issue running the tests with a set of independent markers by applying a very restrictive LD threshold of r2 < 0.1. It could be argued that association signals would be lost by applying such a strict threshold of r2 but even so, if the signal remains it supports it as “true positive” (15). Supplemental Figure 4 summarizes the study workflow.
Correcting for Stratification in Rare Variant Association Analysis
We verified that the set of Principal Components computed with common variation was able to correct stratification for rare variant association analysis in our sample (15). To be as stringent as possible, the 10 first principal components (PC's) and genomic control (GC) were used to correct for stratification in both tests. For case-control burden 10 PC's corrected tests, the genomic inflation factor (λGC) was equal to 1.11 and for SKAT 10 PC's corrected tests it was equal to 1.24. These λGC values were used for correction of the resulting inflation on each type of association test (GC correction = Statistic / λGC). When no PC's correction was used, the λGC for case-control burden tests was equal to 1.44 and 2.97 for SKAT. Thus, the 10 PC's correction reduced the inflation by 33% in the case-control burden tests, and in 174% in the SKAT tests.
Correcting for Multiple Testing in Gene Case-Control Association Analysis of Rare Variation
Regarding the question of correcting for multiple testing in gene association by rare variants, a genomic association threshold of 106 is accepted (equivalent to Bonferroni correction for 19,000–20,000 protein encoding genes in the genome). It is also accepted that Bonferroni, although mathematically right would be very penalizing for biological data, therefore we opted for techniques based on permutation processes. Our multi-test correction procedure brings together the genotypes of all rare variants in all tested genes as columns into a single table. For each gene a number of markers equal to that of the gene was randomly extracted from this table and its association test calculated. By repeating the procedure for N times an empirical corrected P-value was calculated for each tested gene. It can be argued that when randomization is done, LD relations are abrogated affecting the empirical P-values computation in random tests, but this problem did not affect our multi-testing correction procedure since we use a working set of independent markers (r2 < 0.1) (15).
Enrichment in OMIM Annotations in the “Result-List” of Genes Associated to SLE by Rare Variation
Taking into account that pleiotropic effects on human complex traits was widespread (23), it would be expected that in a list of genes significantly enriched in rare variation there would also be an enrichment of diseases caused by mutations. To test this, we used three data sets. First, we downloaded the OMIM database (http://www.omim.org/downloads/; updated: March 23, 2015), and employed it to build a ‘gene-disease’ table with its 20,707 records (“gene-disease” pairs); second, the list of all GWAS imputed protein-coding genes in our final dataset; and thirdly, the list of N genes resulting as candidates to be SLE-associated from our rare variants association analysis. Then if our “result-list” of N associated genes provided annotations for X OMIM diseases, the procedure for testing enrichment in OMIM annotations was to randomly select a set of N genes from the list of GWAS imputed protein-coding genes, and count how many of them appeared on the OMIM “gene-disease” table. This procedure was repeated 1,000 times. The average number of OMIM disease and its standard deviation was calculated and then a Z-score test was performed providing the statistical significance of this enrichment.
Results
Imputation
A total of 13,956 genes passed the QC filter of the imputation process, summing a set of 5,305,811 markers, 2,595,206 variants with MAF > 1% (48.93%), and 2,709,605 variants (mutations) with MAF < 1% (51.07%). A set of 1,549,436 independent markers was obtained by applying a threshold of r2 < 0.1. As expected, rare variation was much less affected by the linkage disequilibrium than common variation, which resulted in 87,853 variants with MAF > 1% (5.56%) and 1,491,583 variants with MAF < 1% (94.44%) (Figure 1).
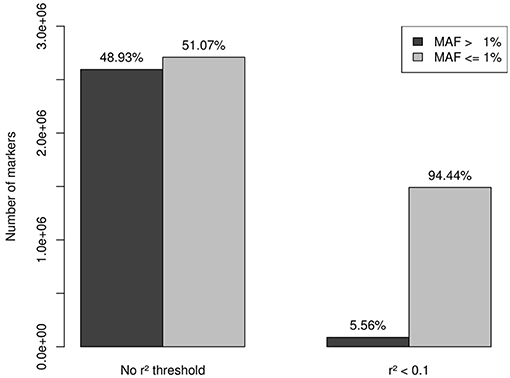
Figure 1. After QC filtering, imputation provided a set of 5,305,811 markers: 2,595,206 with MAF > 1% (48.93%) and 2,709,605 with MAF < 1% (51.07%). A set of 1,549,436 independent markers was obtained by applying a threshold of r2 < 0.1. Rare variation was less affected by the linkage disequilibrium than common variation, which resulted in 87,853 variants with MAF > 1% (5.56%) and 1,491,583 variants with MAF < 1% (94.44%). This last set of 1,491,583 independent rare-variants constituted our working set.
A working set of 1,306,324 independent (r2 < 0.1) rare variants (MAF < 0.1) was used to test genes for case-control association to the SLE phenotype (185,219 were non-polymorphic). The reliability of these tests depends on the accuracy of the imputation. When analyzing reliability of imputation in rare variants 3 intervals are usually differentiated: “singletons,” “very rare” variation and “rare” variation (4, 24). It is known that as the variant MAF decreases, so does the accuracy of imputation, improving with the size of the reference panel, and singletons, meaning that the minor allele is observed only in one chromosome, are not reliably imputed under any conditions. In our data set, 189,893 (14.5%) variants were singletons (in the 8,277 individuals sample this means a MAF = 0.006%) and if an additional threshold of MAF < 0.1% to distinguish rare variant from a category of “very” rare variant was applied, then 676,621 (51.8%) variants were classified as very rare-variants, while 439,810 (33.7%) had MAF between 0.1 and 1%. These results suggested that imputed genotype data used in the association analysis could be unreliable because of the predominance of markers belonging to the categories of very rare variants and singletons over the most reliable imputation category of rare variation, 0.1 < MAF ≤ 1%. However, in tests based on the combined effect of variants, the main factor is not the number of markers aggregated but more importantly the count of alleles of minor frequency in the sample of analyzed individuals. Thus, in our 8,277 individuals dataset the 3 categories of rare variation sum up 29,128,106 minor alleles. Singletons represented 14.5% of rare variation but only 0.65% of minor alleles. The 51.8% of the markers included in the very rare variation category add up to 4,671,537 minor frequency alleles, that is, 16.04%; while the remaining markers, sum up to 24,266,676 of minor frequency alleles, which represented the 83.33%, resulting in a 5 times greater ratio of “0.1% < MAF ≤ 1%” variation compared to the sum of the other two categories (Table 1, Figure 2). Therefore, despite its lower proportion, the expected effect of rare variation on the aggregate test would be greater than that of the “very rare” variation and singletons providing a higher reliability to the analysis.
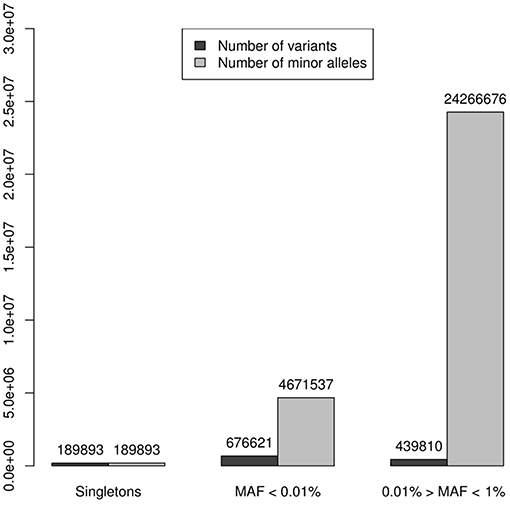
Figure 2. Numbers of variants were represented by dark columns: in the set of 1,306,324 independent (r2 < 0.1) polymorphic rare variants (MAF < 0.1), 189,893 (14.5%) were singletons (in the 8,277 individuals sample this means a MAF = 0.006%), 676,621 (51.8%) were classified as “very rare-variants” (0.006% < MAF < 0.1%), and 439,810 (33.7%) considered as a rare variation in a “strict sense” (0.1% < MAF ≤ 1%); these numbers of variants were represented by dark columns. Lighter columns represented the sums of alleles of minor frequency in each of the 3 categories of rare variation, these 3 categories sum up 29,128,106 minor alleles: the 189,893 singletons represented only 0.65% of minor alleles; the 676,621 the markers, which were very rare variation, add up to 4,671,537 minor frequency alleles, it was the 16.04%; while the remaining markers, sum up to 24,266,676 of minor frequency alleles representing the 83.33%.
Note that the proportions of the different functional categories in the rare variation (MAF < 1% with and without r2 filtering was similar (Table 2, Figure 3), being the intronic the most abundant category, 85% of the total, while the exonic rare variants represented only 2%, of which more than 98% were synonymous (Figure 4).
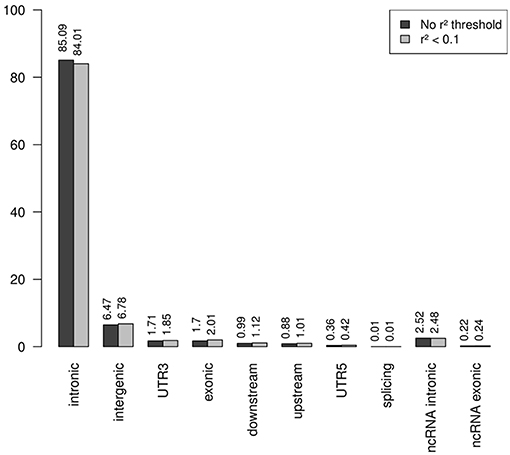
Figure 3. Functional annotation of rare variation. Note that the percentages of the different functional categories in rare variation with and without r2 filtering was similar, being the intronic the most abundant category, 85% of the total.
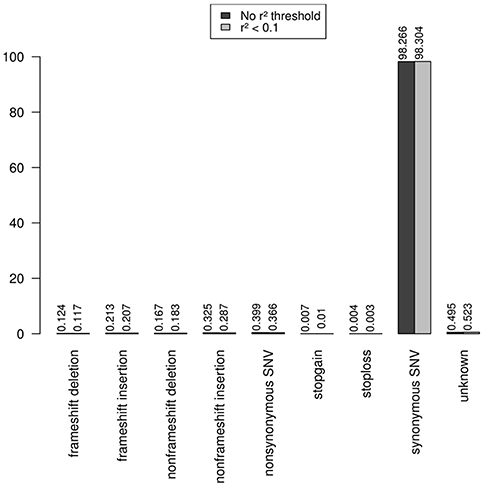
Figure 4. Functional annotation of exonic rare variation. The exonic rare variants represented only 2%, and more than 98% of these exonic mutations were synonymous.
Rare-Variant Association Gene-Centered Analysis, and OMIM Annotation Enrichment
Under these conditions a set of 281 genes showed SKAT test with Genomic Control and multi-testing corrected P < 0.05 (Supplemental Table 1). Noted that 441 genes also presented Genomic Control and multi-testing corrected significant tests for enrichment in rare variation (Supplemental Table 2). When the OMIM annotation enrichment analysis were executed, the list of SKAT associated genes was significantly enriched with 119 OMIM diseases (Supplemental Table 3) instead of the 81 expected at random, which gave a value of P = 3E-03. Of these 281 genes, 139 were enriched in mutations in cases vs. controls and the remaining 142 were depleted. Note that the list of 139 genes enriched in mutations had 80 OMIM diseases annotations when expected was just 40, which gave a P-value of 6E-05. Remark that the 140 depleted genes showed no significant enrichment in OMIM diseases annotations (39 vs. 41 expected, P = 0.59).
As best candidates for SLE association by rare variation, we selected the set of 98 genes which simultaneously showed Genomic Control and multi-testing corrected P < 0.05 in both SKAT test and case-control burden test, with the purpose of reducing the proportion of possible spurious associations. These are shown in Table 3. Some of these are discussed as excellent candidates for the identification of individuals with particular clinical phenotypes that may be directly targeted for sequencing. ANNOVAR annotation of the independent mutations mapped on these 98 genes are shown in Supplemental Table 5.
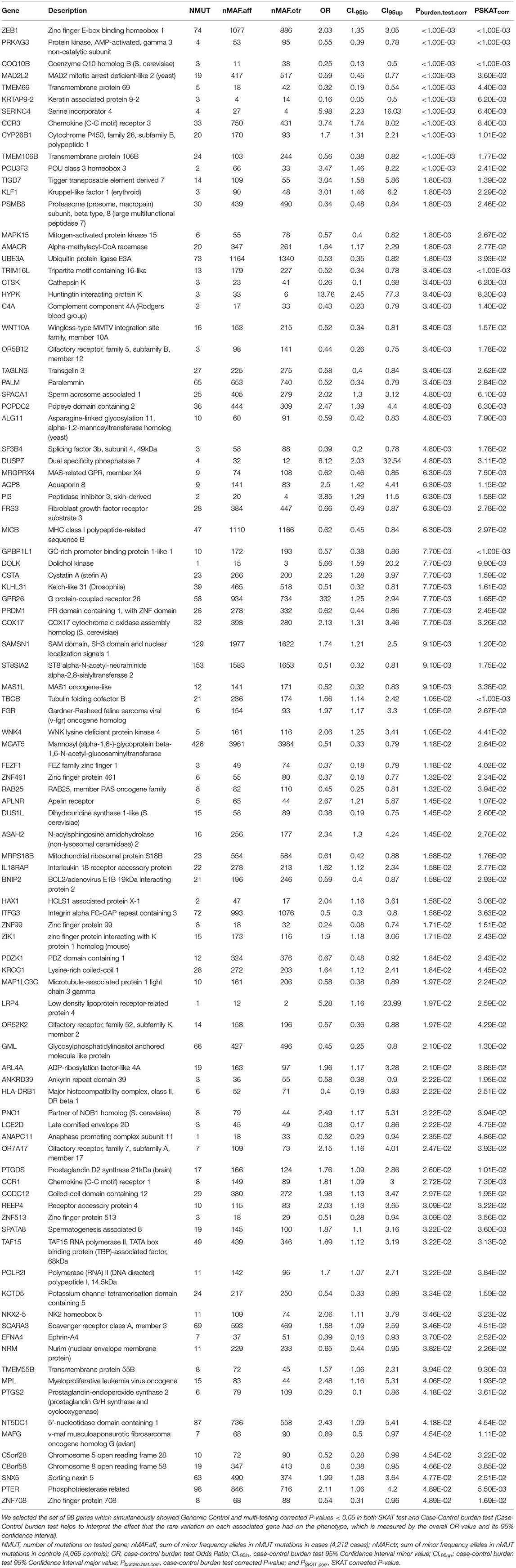
Table 3. Best gene candidates for SLE association through rare variation in European ancestry population.
Discussion
We have described a strategy to identify the association of rare variation with a complex disease based on densely genotyped data and stringent imputation. Our study provides a first list of genes potentially involved in SLE through rare mutations that may have an impact on the clinical presentation of the disease.
Although it could seem difficult to justify the role of non-coding rare gene variation as causal, there are numerous examples that support it. Efforts to identify risk alleles usually are focused on exploring coding mutation by exome sequencing, noting Pullabhatla et al. (25), as a recent example in SLE, but analogous works for non-coding variants are scant. As examples supporting the causative role of rare non-coding gene variation in these complex phenotypes, it has been recently reported that non-noding mutation affected plasma lipid traits in a founder population (26), or in a more generalized perspective, it has been demonstrated that rare variants contribute to large gene expression changes across tissues and provide an integrative method for interpretation of rare variants effects (27).
It is important to point out that a significant test for aggregated case-control burden test would indicate that in the set of individuals forming the sample, the overall effect of the rare variation on the gene goes in the same direction being either of risk or protective. This feature of case-control burden analysis helps to interpret the effect of rare variation on the phenotype, which is measured by the overall OR values, OR > 1 or OR < 1, risk or protection, respectively. Mutations associated with diseases were usually considered to be detrimental to health, increasing the risk of disease. However, there are a growing number of reported mutations shown to be protective, lowering the risk of certain diseases and conditions (28–31). In this context we can explain why the gene STAT4 associated to SLE by common variation (32–45) and our 20th SKAT best-hit, failed the burden test (Supplemental Tables 1, 4). There were two sets of rare variation mapping on the gene with opposite join effects and therefore reducing the power of the overall burden test. The same rationale could be applied to the other SLE associated genes by common variation also detected as targets by rare variation with SKAT but not by burden test in our study (Supplemental Tables 1, 4): GTF2IRD1 (39, 42), DAB2 (41), NOTCH4 (37), CLEC16A (32, 42, 44), TNFSF4 (32, 40–46), and C2 (36). The same can be argued for DOCK8 (ORburden.test = 2.33, P SKAT.corr = 0.03 and Pburden.test.corr = 0.204) the cause of Hyper-IgE recurrent infection syndrome (HIES) autosomal recessive by homozygous or compound heterozygous mutation (OMIM #243700). Note that it has been reported a case of DOCK8 deficiency caused by a truncating mutation, associated with SLE (47).
Focusing on the best-hits which meet the criteria of simultaneous significant association in both rare variation tests, SKAT and burden test, we found some genes described as associated with SLE by common variation such as TMEM55B (46), SPATA8 (36), PRDM1 (32, 40, 42, 44, 48), and HLA-DRB1 (36, 40, 42, 43, 45) (Table 3). Note IRF7 also associated to SLE through common variants (32, 38, 44) had association values through rare variation close to statistical significance (PSKAT.corr = 0.082 and Pburden.test.corr = 0.036) (Supplemental Table 4).
In this kind of studies it is usual to obtain lists of genes with a difficult functional justification. However, in this case we can relate many of the best hits in Table 3 directly to immunological functions or with effects on other organs or tissues affected by SLE, such as skin, central nervous system or blood. For example our list contained C4A, PSKAT.corr = 0.014 and Pburden.test.corr = 0.0034, ORburden.test = 0.43 and CI95%.burden.test = (0.23, 0.79), which was early related to SLE through mutation [OMIM: 152700; (49)]. The zinc finger E-box binding homeobox 1 gene, ZEB1, P SKAT.corr < 1.00E-03 and Pburden.test.corr < 1.00E-03, ORenrich = 2.03 and CI 95%.enrich = (1.35, 3.05), acts as a transcriptional repressor inhibiting interleukin-2 (IL-2) gene expression. Note that IL-2 plays a critical role in immune tolerance, and insufficient IL-2 production upon stimulation has been recognized in SLE pathogenesis, particularly it has been described a new epigenetic pathway in the control of IL-2 production in SLE whereby low levels of miR-200a-3p accumulate the binding of the ZEB1-CtBP2 complex to the IL-2 promoter and suppresses IL-2 production (50). The role of CCR3 [P SKAT.corr = 0.00838 and Pburden.test.corr < 1.00E-03, ORburden.test = 3.74 and CI 95%.burden.test = (1.74, 8.02)] in inflammation is widely known (OMIM *601268).
The protein encoded by CYP26B1, P SKAT.corr = 0.01 and Pburden.test.corr < 1.00E-03, ORburden.test = 1.7 and CI 95%.burden.test = (1.31, 2.21), functions as a critical regulator of all-trans retinoic acid levels by the specific inactivation of all-trans retinoic acid to hydroxylated forms. Mast cells (MCs) are known to be regulators of inflammation. It has been reported that the ATP-P2X7 pathway induces MCs activation and consequently exacerbates inflammation. P2X7 expression on MCs was reduced by fibroblasts in the skin. Cyp26b1 was highly expressed in skin fibroblasts. Cyp26b1 inhibition resulted in upregulation of P2X7 on MCs and the presence of excessive amounts of retinoic acid correlated with the increased expression of P2X7 on skin MCs and consequent P2X7- and MC-dependent dermatitis (so-called retinoid dermatitis) (51).
The protein encoded by TIGD7 belongs to the “tigger subfamily of the pogo superfamily of DNA-mediated transposons” in humans, P SKAT.corr = 0.0139 and Pburden.test.corr = 0.00179, ORburden.test = 3.04 and CI95%.burden.test = (1.58, 5.86). The exact function of this gene is not known, but it is very similar to CENPB considered a major centromere auto-antigen recognized by sera from patients with anti-cetromere-antibodies (ACA), which occur in some autoimmune diseases, frequently in limited systemic scleroderma and occasionally in its diffuse form (52, 53).
AQP8, P SKAT.corr = 0.0115 and Pburden.test.corr = 0.0063, ORburden.test = 2.5 and CI 95%.burden.test = (1.42, 4.41). It has been reported that efficient induction of B cell activation and differentiation requires H2O2 fluxes across the plasma membrane for signal amplification. NADPH-oxidase 2 is the main source of H2O2 and AQP8 is the transport facilitator across the plasma membrane. AQP8 silencing inducible B lymphoma cells responded poorly to TLR and BCR stimulation. Conversely a silencing-resistant AQP8 rescued responsiveness (54). In addition AQP8 was the major antibody target on human salivary glands in patients with primary Sjögren's syndrome (55).
It was known that SAMSN1 (=HACS1), P SKAT.corr = 0.012 and Pburden.test.corr = 0.0091, ORburden.test = 1.74 and CI95%.burden.test = (1.21, 2.5), is up-regulated by B cell activation signals and it participates in B cell activation and differentiation (56).
MICB, P SKAT.corr = 0.0297 and Pburden.test.corr = 0.0062, ORburden.test = 0.62 and CI 95%.burden.test = (0.45, 0.84), acts as a stress-induced self-antigen that is recognized by gamma delta T cells. MICB might play a role in both SLE and cutaneous LE (CLE) in european population (57). In addition MICB has been associated with susceptibility to SLE in Han Chinese Population (58, 59).
CTSK, P SKAT.corr = 0.0062 and Pburden.test.corr = 0.0034, ORburden.test = 0.28 and CI95%.burden.test = (0.1, 0.68), is highly expressed by rheumatoid synovial fibroblasts (RSF) that are activated by toll-like receptor signaling pathways in rheumatoid arthritis and is known to play a key role in the degradation of type Iand type II collagen. Thus, cathepsin K is implicated in the degradation of bone and cartilage in RA (60). In addition it has been suggested that CTSK is involved in development of psoriasis-like skin lesions through TLR-dependent Th17 activation (61).
Autoinflammation, lipodystrophy, and dermatosis syndrome (ALDD) can be caused by homozygous mutations in the PSMB8 gene (OMIM: # 256040), P SKAT.corr = 0.0246 and Pburden.test.corr = 0.00179, ORburden.test = 0.64 and CI 95%.burden.test = (0.48, 0.84). This autosomal recessive systemic autoinflammatory disorder is characterized by early childhood onset of annular erythematous plaques on the face and extremities with subsequent development of partial lipodystrophy and laboratory evidence of immune dysregulation. More variable features include recurrent fever, severe joint contractures, muscle weakness and atrophy, hepatosplenomegaly, basal ganglia calcifications, and microcytic anemia (62–64). This disorder encompasses Nakajo-Nishimura syndrome (NKJO); joint In contractures, muscular atrophy, microcytic anemia, and panniculitis-induced lipodystrophy (JMP syndrome); and chronic atypical neutrophilic dermatosis with lipodystrophy and elevated temperature syndrome (CANDLE). Furthermore, mutations in PSMB8 and other proteasome unit genes were shown to lead to an increased type I interferon signature (65), a characteristic of SLE.
The roles of TRIM16L, P SKAT.corr = 0.0033 and Pburden.test.corr < 1.00E-03, ORburden.test = 0.52 and CI95%.burden.test = (0.34, 0.78), in immune response are unknown, however it has been reported that in fish models TRIM16L exerted negative regulation of the interferon immune response against DNA virus infection (66). The early events that facilitate viral persistence in chronic viral infections have been linked to the activity of the immunoregulatory cytokine IL-10. It has been reported that IL-10 induced the expression of MGAT5, a glycosyltransferase that enhances N-glycan branching on surface glyco- proteins, P SKAT.corr = 0.0264 and Pburden.test.corr = 0.0118, ORburden.test = 0.51 and CI 95%.burden.test = (0.33, 0.79). Increased N-glycan branching on CD8+ T cells promoted the formation of a galectin 3-mediated membrane lattice, which restricted the interaction of key glycoproteins, ultimately increasing the antigenic threshold required for T cell activation allowing the establishment of chronic infection (67).
The serine incorporator 4, SERINC4, P SKAT.corr = 0.0064 and Pburden.test.corr < 1.00E-03, ORburden.test = 5.98 and CI95%.burden.test = (2.23, 16.03), incorporates amino acid serine into membranes and facilitates the synthesis of two serine-derived lipids, phosphatidylserine and sphingolipids (68).
Gene KLF1, PSKAT.corr = 0.00179 and Pburden.test.corr = 0.0229, ORburden.test = 3.01 and CI95%.burden.test = (1.46, 6.20), encodes a hematopoietic-specific transcription factor that induces high-level expression of adult beta-globin and other erythroid genes. Heterozygous loss-of-function mutations in this gene result in the dominant In(Lu) blood phenotype (69). Compound heterozygosity for KLF1 mutations is associated with microcytic hypochromic anemia and increased fetal hemoglobin (70). Mutations in KLF1 cause dyserythropoietic anemia congenital type IV (OMIM: 613673).
TMEM106B [P SKAT.corr < 1.00E-03 and Pburden.test.corr = 0.0177, ORburden.test = 0.56 and CI95%.burden.test = (0.38, 0.82)] was associated with frontotemporal dementia (71, 72). In addition TMEM106B has been associated with inflammation, neuronal loss, and cognitive deficits, even in the absence of known brain disease, and their impact is highly selective for the frontal cerebral cortex of older individuals (73).
Mutations affecting the gene ALG11 [PSKAT.corr = 0.0079 and Pburden.test.corr = 0.0048, ORburden.test = 0.56 and CI95%.burden.test = (0.42, 0.83)] cause congenital disorder of glycosylation 1P (CDG1P) [OMIM: 613661], a multisystem disorder caused by a defect in glycoprotein biosynthesis and characterized by under-glycosylated serum glycoproteins. Congenital disorders of glycosylation result in a wide variety of clinical features, such as defects in the nervous system development, psychomotor retardation, dysmorphic features, hypotonia, coagulation disorders, and immunodeficiency (74, 75).
MAD2L2, PSKAT.corr < 0.001 and Pburden.test.corr = 0.0036, ORburden.test = 0.59 and CI95%.burden.test = (0.45, 0.77), controls DNA repair at telomeres and DNA breaks by inhibiting 5' end resection (76). Note that a role for MAPK15 (=ERK8), P SKAT.corr = 0.0018 and P enrich.corr = 0.0267, OR enrich = 0.57 and CI 95%.enrich = (0.40,0.77), in the response to, or repair of, DNA single strand breaks has been proposed (77), and it is annotated as “positive regulation of telomerase activity,” biological process (GO:0051973). MRGPRX4 (= MrgX4) [P SKAT.corr = 0.0063 and Pburden.test.corr = 0.00748, ORburden.test = 0.62 and CI95%.burden.test = (0.46, 0.85)] is a Mas-related G-protein coupled receptor X (MrgXs). It was described as an oncogene in human colorectal cancers (78), however, it has recently been linked to immunological functions. AG-30/5C is an angiogenic host defense peptide (HDP) that activates various functions of fibroblasts and endothelial cells, including cytokine/chemokine production and wound healing. It has been shown that AG-30/5C enhanced the production of cytokines/chemokines and facilitated keratinocyte migration and proliferation mainly via MrgX3 and MrgX4 receptors constitutively expressed in keratinocytes and up-regulated upon stimulation with TLR ligands. AG-30/5C-induced activation of keratinocytes was controlled by MAPK and NF-κB pathways (79).
In addition other genes associated to human energy metabolism and more specifically in the mitochondrion, as part of the respiratory chain, the best hit associated to SLE by rare variation was COQ10B, PSKAT.corr < 0.001 and Pburden.test.corr < 0.001, ORburden.test = 0.25 and CI95%.burden.test = (0.13, 0.50). It encodes coenzyme Q, an essential component of the electron transport chain. The copper metallochaperone COX17, P SKAT.corr = 0.0077 and Pburden.test.corr = 0.0326, ORburden.test = 2.13 and CI95%.burden.test = (1.31, 3.46), is essential for the assembly and activation of the cytochrome c oxidase complex (80), the terminal component of the mitochondrial respiratory chain that catalyzes the electron transfer from reduced cytochrome c to oxygen. Null mutations in COX17 elicit a loss of cytochrome oxidase due to the failure of the mutants to complete assembly of the complex [OMIM: *604813]. It has been reported that SLE T-cells have persistently hyperpolarized mitochondria associated with increased mitochondrial mass, high levels of reactive oxygen species (ROS) and low levels of ATP. These hyperpolarized mitochondria resist the depolarization required for activation-induced apoptosis and predispose T cells for necrosis, thus stimulating inflammation in SLE (81, 82). Necrotic cell lysates activate dendriticcells and might account for increased interferon a production and inflammation in lupus patients (83). Additionally, the mitochondrial ATP deficits also reduce the macrophage energy that is needed to clear apoptotic bodies (84). The mitochondrial transmembrane potential is result of the respiratory electron transport chain that drives the flow of electrons from NADH to molecular oxygen by its last enzyme the cytochrome c oxidadase. Note that it has been reported that COX17 is essential for activation of cytochrome C oxidase (80) linking the COX17 function with the SLE phenotype.
Conclusions
Here we present a set of 98 genes as good candidates for association with SLE by mutation affecting a diversity of functions in different organs and tissues. Considering that complex phenotypes involves the intervention of multiple genes associated by common variation, the same scheme could be expected for genes associated by rare variation. Thus, each gene or set of genes would influence in a small group of affected carriers explaining the clinical heterogeneity or complexity of this pathology. However, it is necessary to remark that these results are preliminary and would need to be confirmed by sequencing in the best candidate carriers in our sample data set.
Author Contributions
MM-B contributed to the conception and design of the study, organized the database, performed the statistical analysis, wrote the first draft of the manuscript, and participated in the manuscript revision. MA-R provided the raw genotype data sets, contributed to the conception and design of the study, interpretation of results, manuscript revision and wrote the final draft of the manuscript.
Conflict of Interest Statement
The authors declare that the research was conducted in the absence of any commercial or financial relationships that could be construed as a potential conflict of interest.
Supplementary Material
The Supplementary Material for this article can be found online at: https://www.frontiersin.org/articles/10.3389/fimmu.2019.00258/full#supplementary-material
References
1. MacArthur J, Bowler E, Cerezo M, Gil L, Hall P, Hastings E, et al. The new NHGRI-EBI Catalog of published genome-wide association studies (GWAS Catalog). Nucleic Acids Res. (2017) 45:D896–901. doi: 10.1093/nar/gkw1133
2. Dickson SP, Wang K, Krantz I, Hakonarson H, Goldstein DB. Rare variants create synthetic genome-wide associations. PLoS Biol. (2010) 8:e1000294. doi: 10.1371/journal.pbio.1000294
3. Frazer KA, Murray SS, Schork NJ, Topol EJ. Human genetic variation and its contribution to complex traits. Nat Rev Genet. (2009) 10:241–51. doi: 10.1038/nrg2554
4. Saint Pierre A, Génin E. How important are rare variants in common disease? Brief Funct Genomics (2014) 13:353–61. doi: 10.1093/bfgp/elu025
5. Sazonovs A, Barrett JC. (2018). Rare-variant studies to complement genome-wide association studies. Annu Rev Genomics Hum Genet. (2018) 19:97–112. doi: 10.1146/annurev-genom-083117-021641
6. Manolio TA, Collins FS, Cox NJ, Goldstein DB, Hindorff LA, Hunter DJ, et al. Finding the missing heritability of complex diseases. Nature (2009) 461:747–53. doi: 10.1038/nature08494
7. Mägi R, Asimit JL, Day-Williams AG, Zeggini E, Morris AP. Genome-wide association analysis of imputed rare variants: application to seven common complex diseases. Genet Epidemiol. (2012) 36:785–96. doi: 10.1002/gepi.21675
8. Asimit JL, Zeggini E. Imputation of rare variants in next-generation association studies. Hum Hered. (2012) 74:196–204. doi: 10.1159/000345602
9. Zheng HF, Rong JJ, Liu M, Han F, Zhang XW, Richards JB, et al. Performance of genotype imputation for low frequency and rare variants from the 1000 genomes. PLoS ONE (2015) 10:e0116487. doi: 10.1371/journal.pone.0116487
10. Huang J, Howie B, McCarthy S, Memari Y, Walter K, Min JL, et al. Improved imputation of low-frequency and rare variants using the UK10K haplotype reference panel. Nat Commun. (2015) 6:8111. doi: 10.1038/ncomms9111
11. Mitt M, Kals M, Pärn K, Gabriel SB, Lander ES, Palotie A, et al. Improved imputation accuracy of rare and low-frequency variants using population-specific high-coverage WGS-based imputation reference panel. Eur J Hum Genet. (2017) 25:869–76. doi: 10.1038/ejhg.2017.51
12. Iglesias AI, van der Lee SJ, Bonnemaijer PWM, Höhn R, Nag A, Gharahkhani P, et al. Haplotype reference consortium panel: practical implications of imputations with large reference panels. Hum Mutat. (2017) 38:1025–32. doi: 10.1002/humu.23247
13. McCarthy S, Das S, Kretzschmar W, Delaneau O, Wood AR, Teumer A, et al. Haplotype reference consortium. A reference panel of 64,976 haplotypes for genotype imputation. Nat Genet. (2016) 48:1279–83. doi: 10.1038/ng.3643
14. Günther C, Kind B, Reijns MA, Berndt N, Martinez-Bueno M, Wolf C, et al. Defective removal of ribonucleotides from DNA promotes systemic autoimmunity. J Clin Invest. (2015) 125:413–24. doi: 10.1172/JCI78001
15. Delgado-Vega AM, Martínez-Bueno M, Oparina NY, López Herráez D, Kristjansdottir H, Steinsson K, et al. Whole exome sequencing of patients from multicase families with systemic lupus Erythematosus identifies multiple rare variants. Sci Rep. (2018) 8:8775. doi: 10.1038/s41598-018-26274-y
16. Purcell S, Neale B, Todd-Brown K, Thomas L, Ferreira MAR, Bender D, et al. PLINK: a toolset for whole-genome association and population-based linkage analysis. Am J Human Genet. (2007) 81:559–75. doi: 10.1086/519795
17. Thornton T, Tang H, Hoffman TJ, Ochs-Balcom HM, Baan BJ, Risch N. Estimating kinship in admixed populations. Am J Human Genet. (2012) 91:122–38. doi: 10.1016/j.ajhg.2012.05.024
18. Price AL, Patterson NJ, Plenge RM, Weinblatt ME, Shadick NA, Reich D. Principal components analysis corrects for stratification in genome-wide association studies. Nat Genet. (2006) 38:904–9. doi: 10.1038/ng1847
19. Howie BN, Donnelly P, Marchini J. A flexible and accurate genotype imputation method for the next generation of genome-wide association studies. PLoS Genet. (2009) 5:e1000529. doi: 10.1371/journal.pgen.1000529
20. Timbers TA, Garland SJ, Mohan S, Flibotte S, Edgley M, Muncaster Q, et al. Accelerating gene discovery by phenotyping whole-genome sequenced multi-mutation strains and using the Sequence Kernel Association Test (SKAT). PLoS Genet. (2016) 12:e1006235. doi: 10.1371/journal.pgen.1006235
21. Ward LD, Kellis M. HaploReg: a resource for exploring chromatin states, conservation, and regulatory motif alterations within sets of genetically linked variants. Nucleic Acids Res. (2012) 40:D930–4. doi: 10.1093/nar/gkr917
22. Wang K, Li M, Hakonarson H. ANNOVAR: functional annotation of genetic variants from next-generation sequencing data. Nucleic Acids Res. (2010) 38:e164. doi: 10.1093/nar/gkq603
23. Solovieff N, Cotsapas C, Lee PH, Purcell SM, Smoller JW. Pleiotropy in complex traits: challenges and strategies. Nat Rev Genet. (2013) 14:483–95. doi: 10.1038/nrg3461
24. Bodmer W, Bonilla C. Common and rare variants in multifactorial susceptibility to common diseases. Nat Genet. (2008) 40:695–701. doi: 10.1038/ng.f.136
25. Pullabhatla V, Roberts AL, Lewis MJ, Mauro D, Morris DL, Odhams CA, et al. De novo mutations implicate novel genes in systemic lupus erythematosus. Hum Mol Genet. (2018) 27:421–9. doi: 10.1093/hmg/ddx407
26. Igartua C, Mozaffari SV, Nicolae DL, Ober C. Rare non-coding variants are associated with plasma lipid traits in a founder population. Sci Rep. (2017) 7:16415. doi: 10.1038/s41598-017-16550-8
27. Li X, Kim Y, Tsang EK, Davis JR, Damani FN, Chiang C, et al. The impact of rare variation on gene expression across tissues. Nature (2017) 550:239–43. doi: 10.1038/nature24267
28. Butler JM, Hall N, Narendran N, Yang YC, Paraoan L. Identification of candidate protective variants for common diseases and evaluation of their protective potential. BMC Genomics (2017) 18:575. doi: 10.1186/s12864–017-3964–3
29. Steinthorsdottir V, Thorleifsson G, Sulem P, Helgason H, Grarup N, Sigurdsson A, et al. Identification of low-frequency and rare sequence variants associated with elevated or reduced risk of type 2 diabetes. Nat Genet. (2014) 46:294–8. doi: 10.1038/ng.2882
30. Cohen J, Pertsemlidis A, Kotowski IK, Graham R, Garcia CK, Hobbs HH. Low LDL cholesterol in individuals of African descent resulting from frequent nonsense mutations in PCSK9. Nat Genet. (2005) 37:161–5. doi: 10.1038/ng1509
31. Costford SR, Kavaslar N, Ahituv N, Chaudhry SN, Schackwitz WS, Dent R, et al. Gain-of-function R225W mutation in human AMPKgamma(3) causing increased glycogen and decreased triglyceride in skeletal muscle. PLoS ONE (2007) 2:e903. doi: 10.1371/journal.pone.0000903
32. Bentham J, Morris DL, Graham DSC, Pinder CL, Tombleson P, Behrens TW, et al. Genetic association analyses implicate aberrant regulation of innate and adaptive immunity genes in the pathogenesis of systemic lupus erythematosus. Nat Genet. (2015) 47:1457–64. doi: 10.1038/ng.3434
33. Lee YH, Bae SC, Choi SJ, Ji JD, Song GG. Genome-wide pathway analysis of genome-wide association studies on systemic lupus erythematosus and rheumatoid arthritis. Mol Biol Rep. (2012) 39:10627–35. doi: 10.1007/s11033-012-1952-x
34. Graham RR, Cotsapas C, Davies L, Hackett R, Lessard CJ, Leon JM, et al. Genetic variants near TNFAIP3 on 6q23 are associated with systemic lupus erythematosus. Nat Genet. (2008) 40:1059–61. doi: 10.1038/ng.200
35. Demirci FY, Wang X, Kelly JA, Morris DL, Barmada MM, Feingold E, et al. Identification of a new susceptibility locus for systemic lupus erythematosus on chromosome 12 in individuals of European ancestry. Arthritis Rheumatol. (2016) 68:174–83. doi: 10.1002/art.39403
36. Armstrong DL, Zidovetzki R, Alarcón-Riquelme ME, Tsao BP, Criswell LA, Kimberly RP, et al. GWAS identifies novel SLE susceptibility genes and explains the association of the HLA region. Genes Immun. (2014) 15:347–54. doi: 10.1038/gene.2014.23
37. Hom G, Graham RR, Modrek B, Taylor KE, Ortmann W, Garnier S, et al. Association of systemic lupus erythematosus with C8orf13-BLK and ITGAM-ITGAX. N Engl J Med. (2008) 358:900–9. doi: 10.1056/NEJMoa0707865
38. Martin JE, Assassi S, Diaz-Gallo LM, Broen JC, Simeon CP, Castellvi I, et al. A systemic sclerosis and systemic lupus erythematosus pan-meta-GWAS reveals new shared susceptibility loci. Hum Mol Genet. (2013) 22:4021–9. doi: 10.1093/hmg/ddt248
39. Chung SA, Taylor KE, Graham RR, Nititham J, Lee AT, Ortmann WA, et al. Differential genetic associations for systemic lupus erythematosus based on anti-dsDNA autoantibody production. PLoS Genet. (2011) 7:e1001323. doi: 10.1371/journal.pgen.1001323
40. Han JW, Zheng HF, Cui Y, Sun LD, Ye DQ, Hu Z, et al. Genome-wide association study in a Chinese Han population identifies nine new susceptibility loci for systemic lupus erythematosus. Nat Genet. (2009) 41:1234–7. doi: 10.1038/ng.472
41. Lessard CJ, Sajuthi S, Zhao J, Kim K, Ice JA, Li H, et al. Identification of a systemic lupus erythematosus risk locus spanning ATG16L2, FCHSD2, and P2RY2 in Koreans. Arthritis Rheumatol. (2016) 68:1197–209. doi: 10.1002/art.39548
42. Yang W, Tang H, Zhang Y, Tang X, Zhang J, Sun L, et al. Meta-analysis followed by replication identifies loci in or near CDKN1B, TET3, CD80, DRAM1, and ARID5B as associated with systemic lupus erythematosus in Asians. Am J Hum Genet. (2013) 92:41–51. doi: 10.1016/j.ajhg.2012.11.018
43. Gateva V, Sandling JK, Hom G, Taylor KE, Chung SA, Sun X, et al. A large-scale replication study identifies TNIP1, PRDM1, JAZF1, UHRF1BP1 and IL10 as risk loci for systemic lupus erythematosus. Nat Genet. (2009) 41:1228–33. doi: 10.1038/ng.468
44. Morris DL, Sheng Y, Zhang Y, Wang YF, Zhu Z, Tombleson P, et al. Genome-wide association meta-analysis in Chinese and European individuals identifies ten new loci associated with systemic lupus erythematosus. Nat Genet. (2016) 48:940–6. doi: 10.1038/ng.3603
45. Alarcón-Riquelme ME, Ziegler JT, Molineros J, Howard TD, Moreno-Estrada A, Sánchez-Rodríguez E, et al. Genome-wide association study in an amerindian ancestry population reveals novel systemic lupus erythematosus risk loci and the role of european admixture. Arthritis Rheumatol. (2016) 68:932–43. doi: 10.1002/art.39504
46. International Consortium for Systemic Lupus Erythematosus Genetics (SLEGEN), Harley JB, Alarcón-Riquelme ME, Criswell LA, Jacob CO, Kimberly RP, et al. Genome-wide association scan in women with systemic lupus erythematosus identifies susceptibility variants in ITGAM, PXK, KIAA1542 and other loci. Nat Genet. (2008) 40:204–10. doi: 10.1038/ng.81
47. Jouhadi Z, Khadir K, Ailal F, Bouayad K, Nadifi S, Engelhardt KR, et al. Ten-year follow-up of a DOCK8-deficient child with features of systemic lupus erythematosus. Pediatrics (2014) 134:e1458–63. doi: 10.1542/peds.2013-1383
48. Márquez A, Vidal-Bralo L, Rodríguez-Rodríguez L, González-Gay MA, Balsa A, González-Álvaro I, et al. A combined large-scale meta-analysis identifies COG6 as a novel shared risk locus for rheumatoid arthritis and systemic lupus erythematosus. Ann Rheum Dis. (2017) 76:286–94. doi: 10.1136/annrheumdis-2016-209436
49. Rupert KL, Moulds JM, Yang Y, Arnett FC, Warren RW, Reveille JD, et al. The molecular basis of complete complement C4A and C4B deficiencies in a systemic lupus erythematosus patient with homozygous C4A and C4B mutant genes. J Immunol. (2002) 169:1570–8. doi: 10.4049/jimmunol.169.3.1570
50. Katsuyama E, Yan M, Watanabe KS, Matsushima S, Yamamura Y, Hiramatsu S, et al. Downregulation of miR-200a-3p, targeting CtBP2 complex, is involved in the hypoproduction of IL-2 in systemic lupus erythematosus-derived T cells. J Immunol. (2017) 198:4268–76. doi: 10.4049/jimmunol.1601705
51. Kurashima Y, Amiya T, Fujisawa K, Shibata N, Suzuki Y, Kogure Y, et al. The enzyme Cyp26b1 mediates inhibition of mast cell activation by fibroblasts to maintain skin-barrier homeostasis. Immunity (2014) 40:530–41. doi: 10.1016/j.immuni.2014.01.014
52. Hudson M, Mahler M, Pope J, You D, Tatibouet S, Steele R, et al. Clinical correlates of CENP-A and CENP-B antibodies in a large cohort of patients with systemic sclerosis. J Rheumatol. (2012) 39:787–94. doi: 10.3899/rheum.111133
53. Mahler M, Mierau R, Genth E, Blüthner M. Development of a CENP-A/CENP-B-specific immune response in a patient with systemic sclerosis. Arthritis Rheum. (2002) 46:1866–72. doi: 10.1002/art.10330
54. Bertolotti M, Farinelli G, Galli M, Aiuti A, Sitia R. AQP8 transports NOX2-generated H2O2 across the plasma membrane to promote signaling in B cells. J Leukoc Biol. (2016) 100:1071–9. doi: 10.1189/jlb.2AB0116-045R
55. Tzartos JS, Stergiou C, Daoussis D, Zisimopoulou P, Andonopoulos AP, Zolota V, et al. Antibodies to aquaporins are frequent in patients with primary Sjögren's syndrome. Rheumatology (2017) 56:2114–22. doi: 10.1093/rheumatology/kex328
56. Zhu YX, Benn S, Li ZH, Wei E, Masih-Khan E, Trieu Y, et al. The SH3-SAM adaptor HACS1 is up-regulated in B cell activation signaling cascades. J Exp Med. (2004) 200:737–47. doi: 10.1084/jem.20031816
57. Kunz M, König IR, Schillert A, Kruppa J, Ziegler A, Grallert H, et al. Genome-wide association study identifies new susceptibility loci for cutaneous lupus erythematosus. Exp Dermatol. (2015) 24:510–5. doi: 10.1111/exd.12708
58. Zhang Y-M, Zhou X-J, Cheng FJ, Qi Y-Y, Hou P, Zhao M-H, et al. Polymorphism rs(3828903) within MICB is associated with susceptibility to systemic lupus erythematosus in a northern han chinese population. J Immunol Res. (2016) 2016:1343760. doi: 10.1155/2016/1343760
59. Yu P, Zhu Q, Chen C, Fu X, Li Y, Liu L, et al. Association between major histocompatibility complex class i chain-related gene polymorphisms and susceptibility of systemic lupus erythematosus. Am J Med Sci. (2017) 354:430–5. doi: 10.1016/j.amjms.2017.06.003
60. Hirabara S, Kojima T, Takahashi N, Hanabayashi M, Ishiguro N. Hyaluronan inhibits TLR-4 dependent cathepsin K and matrix metalloproteinase 1 expression in human fibroblasts. Biochem Biophys Res Commun. (2013) 430:519–22. doi: 10.1016/j.bbrc.2012.12.003
61. Hirai T, Kanda T, Sato K, Takaishi M, Nakajima K, Yamamoto M, et al. Cathepsin K is involved in development of psoriasis-like skin lesions through TLR-dependent Th17 activation. J Immunol. (2013) 190:4805–11. doi: 10.4049/jimmunol.1200901
62. Agarwal AK, Xing C, DeMartino GN, Mizrachi D, Hernandez MD, Sousa AB, et al. PSMB8 encoding the β5i proteasome subunit is mutated in joint contractures, muscle atrophy, microcytic anemia, and panniculitis-induced lipodystrophy syndrome. Am J Hum Genet. (2010) 87:866–72. doi: 10.1016/j.ajhg.2010.10.031
63. Kitamura A, Maekawa Y, Uehara H, Izumi K, Kawachi I, Nishizawa M, et al. A mutation in the immunoproteasome subunit PSMB8 causes autoinflammation and lipodystrophy in humans. J Clin Invest. (2011) 121:4150–60. doi: 10.1172/JCI58414
64. Arima K, Kinoshita A, Mishima H, Kanazawa N, Kaneko T, Mizushima T, et al. Proteasome assembly defect due to a proteasome subunit beta type 8 (PSMB8) mutation causes the autoinflammatory disorder, Nakajo-Nishimura syndrome. Proc Natl Acad Sci USA. (2011) 108:14914–9. doi: 10.1073/pnas.1106015108
65. Kariuki SN, Ghodke-Puranik Y, Dorschner JM, Chrabot BS, Kelly JA, Tsao BP, et al. Genetic analysis of the pathogenic molecular sub-phenotype interferon-alpha identifies multiple novel loci involved in systemic lupus erythematosus. Genes Immun. (2015) 16:15–23. doi: 10.1038/gene.2014.57
66. Yu Y, Huang X, Zhang J, Liu J, Hu Y, Yang Y, et al. Fish TRIM16L exerts negative regulation on antiviral immune response against grouper iridoviruses. Fish Shellfish Immunol. (2016) 59:256–67. doi: 10.1016/j.fsi.2016.10.044
67. Smith LK, Boukhaled GM, Condotta SA, Mazouz S, Guthmiller JJ, Vijay R, et al. Interleukin-10 directly inhibits CD8+ T cell function by enhancing N-glycan branching to decrease antigen Sensitivity. Immunity (2018) 48:299–312.e5. doi: 10.1142/10755
68. Inuzuka M, Hayakawa M, Ingi T. Serinc, an activity-regulated protein family, incorporates serine into membrane lipid synthesis. J Biol Chem. (2005) 280:35776–83. doi: 10.1074/jbc.M505712200
69. Kawai M, Obara K, Onodera T, Enomoto T, Ogasawara K, Tsuneyama H, et al. Mutations of the KLF1 gene detected in Japanese with the In(Lu) phenotype. Transfusion (2017) 57:1072–7. doi: 10.1111/trf.13990
70. Huang J, Zhang X, Liu D, Wei X, Shang X, Xiong F, et al. Compound heterozygosity for KLF1 mutations is associated with microcytic hypochromic anemia and increased fetal hemoglobin. Eur J Hum Genet. (2015) 23:1341–8. doi: 10.1038/ejhg.2014.291
71. Premi E, Grassi M, van Swieten J, Galimberti D, Graff C, Masellis M, et al. Cognitive reserve and TMEM106B genotype modulate brain damage in presymptomatic frontotemporal dementia: a GENFI study. Brain (2017) 140:1784–91. doi: 10.1093/brain/awx103
72. Gallagher MD, Posavi M, Huang P, Unger TL, Berlyand Y, Gruenewald AL, et al. A dementia-associated risk variant near TMEM106B alters chromatin architecture and gene expression. Am J Hum Genet. (2017) 101:643–63. doi: 10.1016/j.ajhg.2017.09.004
73. Rhinn H, Abeliovich A. Differential aging analysis in human cerebral cortex identifies variants in TMEM106B and GRN that regulate aging phenotypes. Cell Syst. (2017) 4:404–15.e5. doi: 10.1016/j.cels.2017.02.009
74. Thiel C, Rind N, Popovici D, Hoffmann GF, Hanson K, Conway RL, et al. Improved diagnostics lead to identification of three new patients with congenital disorder of glycosylation-Ip. Hum Mutat. (2012) 33:485–7. doi: 10.1002/humu.22019
75. Rind N, Schmeiser V, Thiel C, Absmanner B, Lübbehusen J, Hocks J, et al. A severe human metabolic disease caused by deficiency of the endoplasmatic mannosyltransferase hALG11 leads to congenital disorder of glycosylation-Ip. Hum Mol Genet. (2010) 19:1413–24. doi: 10.1093/hmg/ddq016
76. Boersma V, Moatti N, Segura-Bayona S, Peuscher MH, van der Torre J, Wevers BA, et al. MAD2L2 controls DNA repair at telomeres and DNA breaks by inhibiting 5' end resection. Nature (2015) 521:537–40. doi: 10.1038/nature14216
77. Klevernic IV, Martin NM, Cohen P. Regulation of the activity and expression of ERK8 by DNA damage. FEBS Lett. (2009) 583:680–4. doi: 10.1016/j.febslet.2009.01.011
78. Gylfe AE, Kondelin J, Turunen M, Ristolainen H, Katainen R, Pitkänen E, et al. Identification of candidate oncogenes in human colorectal cancers with microsatellite instability. Gastroenterology (2013) 145:540–3.e22. doi: 10.1053/j.gastro.2013.05.015
79. Kiatsurayanon C, Niyonsaba F, Chieosilapatham P, Okumura K, Ikeda S, Ogawa H. Angiogenic peptide (AG)-30/5C activates human keratinocytes to produce cytokines/chemokines and to migrate and proliferate via MrgX receptors. J Dermatol Sci. (2016) 83:190–9. doi: 10.1016/j.jdermsci.2016.05.006
80. Takahashi Y, Kako K, Kashiwabara S, Takehara A, Inada Y, Arai H, et al. Mammalian copper chaperone Cox17p has an essential role in activation of cytochrome C oxidase and embryonic development. Mol Cell Biol. (2002) 22:7614–21. doi: 10.1128/MCB.22.21.7614-7621.2002
81. McGrath H Jr. Ultraviolet-A1 irradiation therapy for systemic lupus erythematosus. Lupus (2017) 26:1239–51. doi: 10.1177/0961203317707064
82. Gergely P Jr, Grossman C, Niland B, Puskas F, Neupane H, Allam F, et al. Mitochondrial hyperpolarization and ATP depletion in patients with systemic lupus erythematosus. Arthritis Rheum. (2002) 46:175–90. doi: 10.1002/1529-0131(200201)46:1<175::AID-ART10015>3.0.CO;2-H
83. Fernandez D, Perl A. Metabolic control of T cell activation and death in SLE. Autoimmun Rev. (2009) 8:184–9. doi: 10.1016/j.autrev.2008.07.041
Keywords: SLE, systemic lupus erythematosus, imputated rare variation, GWAS—genome-wide association study, sequence kernel association test, aggregated case-control enrichment
Citation: Martínez-Bueno M and Alarcón-Riquelme ME (2019) Exploring Impact of Rare Variation in Systemic Lupus Erythematosus by a Genome Wide Imputation Approach. Front. Immunol. 10:258. doi: 10.3389/fimmu.2019.00258
Received: 27 July 2018; Accepted: 29 January 2019;
Published: 26 February 2019.
Edited by:
Laurence Morel, University of Florida, United StatesReviewed by:
Jason Weinstein, Rutgers Biomedical and Health Sciences, United StatesCeline Berthier, University of Michigan, United States
Wentian Li, Feinstein Institute for Medical Research, United States
Copyright © 2019 Martínez-Bueno and Alarcón-Riquelme. This is an open-access article distributed under the terms of the Creative Commons Attribution License (CC BY). The use, distribution or reproduction in other forums is permitted, provided the original author(s) and the copyright owner(s) are credited and that the original publication in this journal is cited, in accordance with accepted academic practice. No use, distribution or reproduction is permitted which does not comply with these terms.
*Correspondence: Manuel Martínez-Bueno, bWFudWVsLm1hcnRpbmV6QGdlbnlvLmVz