- 1Institute of Cytology and Genetics, Siberian Branch of Russian Academy of Sciences, Novosibirsk, Russia
- 2Novosibirsk State University, Novosibirsk, Russia
- 3Children’s Hospital Los Angeles, Los Angeles, CA, USA
Some variations of human genome [for example, single nucleotide polymorphisms (SNPs)] are markers of hereditary diseases and drug responses. Analysis of them can help to improve treatment. Computer-based analysis of millions of SNPs in the 1000 Genomes project makes a search for SNP markers more targeted. Here, we combined two computer-based approaches: DNA sequence analysis and keyword search in databases. In the binding sites for TATA-binding protein (TBP) in human gene promoters, we found candidate SNP markers of gender-biased autoimmune diseases, including rs1143627 [cachexia in rheumatoid arthritis (double prevalence among women)]; rs11557611 [demyelinating diseases (thrice more prevalent among young white women than among non-white individuals)]; rs17231520 and rs569033466 [both: atherosclerosis comorbid with related diseases (double prevalence among women)]; rs563763767 [Hughes syndrome-related thrombosis (lethal during pregnancy)]; rs2814778 [autoimmune diseases (excluding multiple sclerosis and rheumatoid arthritis) underlying hypergammaglobulinemia in women]; rs72661131 and rs562962093 (both: preterm delivery in pregnant diabetic women); and rs35518301, rs34166473, rs34500389, rs33981098, rs33980857, rs397509430, rs34598529, rs33931746, rs281864525, and rs63750953 (all: autoimmune diseases underlying hypergammaglobulinemia in women). Validation of these predicted candidate SNP markers using the clinical standards may advance personalized medicine.
Introduction
Recent studies (1) showed that the imbalance between effectors and regulators of immune responses causes autoimmune diseases. Self-antigen tolerance characterizes a healthy immune system, whereas impairment of this tolerance leads to autoimmune diseases. Changes in the expression of self-antigens in response to inflammation, tissue lesions, viruses, free radicals, radiation, and pharmaceuticals may trigger autoimmune pathogenesis (2, 3). According to the concept of molecular mimicry, antibodies induced by an infection attack self-antigens that are similar to the pathogen’s epitopes. This concept is a common explanation for the development of autoimmune diseases, i.e., destruction of host tissues by the host immune system (4, 5). More than 100 autoimmune diseases are already known, among them, the 8 most prevalent disorders are psoriasis, rheumatoid arthritis, type I diabetes mellitus, multiple sclerosis, systemic lupus erythematosus, Crohn’s disease, ulcerative colitis, and systemic scleroderma (6). Genotyping of geographic subpopulations (7, 8) revealed genetic diversity of immune responses and the single nucleotide polymorphisms (SNPs) involved (9). Women have greater immune responsiveness than men do, and it manifests itself in the fourfold prevalence of autoimmune diseases among women (10). Sex hormones amplify this hyperimmune response as do adolescence, pregnancy, and menopause stress-related hormonal status of women (11–14).
Overall, sex differences in immune capabilities and autoimmune diseases are an established fact, and precise clinical observations in patients and experiments on animal models underlie the mainstream scientific research aimed at elucidating these phenomena in clinical practice. The gender-biased interactions between microorganisms and the human host (15, 16); the effects of diets (16), sex hormones (17), and the X chromosome inactivation (18) on the immune response; and activities of regulatory genes located on the X chromosome (19) are hot topics in this field of research. In addition to this mainstream research into gender-biased autoimmune diseases, the nascent postgenomic predictive preventive personalized medicine (20) offers hope of elucidation of the pathogenesis of such diseases. To this end, it would be worthwhile to use SNP markers of autoimmune diseases as additional genome-wide informative landmarks. This way, a physician may analyze these SNP markers in his/her patients to improve treatment; in addition, the patients can modify their lifestyle accordingly to reduce the risk of autoimmune complications of their illnesses. We conducted the present study in accordance with this new auxiliary strategy as an adjunctive treatment for prevention of autoimmune complications of monogenic diseases. For example, this kind of adjunctive modality improves survival in metastatic breast cancer (21).
Postgenomic SNP identification is a part of the 1000 Genomes project (22), whose results are available in the dbSNP database (23). The UCSC Genome Browser (24) visualizes the human reference genome (25, 26) as the ancestral variant for all SNPs. It allows clinical researchers to choose an appropriate set of SNPs for genotyping of patients in comparison with healthy volunteers in order to identify/validate disease-related SNP markers (27). Furthermore, these researchers can estimate the population frequencies (28), genetic drifts (29), expressivity, and penetrance (30) of these markers. The data from these clinical studies are available in many databases (31–34) designed for postgenomic predictive preventive personalized medicine (20).
Computer-based analysis of hundreds of millions of unannotated SNPs can make the search for SNP markers more targeted and less expensive (35). To this end, bioinformatics researchers (36–52) rate SNPs using genome-wide maps of genes, functional sites, nucleosomes, interchromosomal contacts, chromatin immunoprecipitation (ChIP) data, and transcriptomes in health (53), in disease (54), and after treatment (55). The Central Limit Theorem ensures an increase in the accuracy of these estimates with the increasing number, diversity, representativeness, and completeness of genome-wide maps (56). Due to this approach, thousands of SNP markers have been found within protein-coding regions of genes (32) [where SNPs alter gene products (57)] but only a few SNP markers among millions of SNPs in regulatory regions of genes (23, 25). The majority of the regulatory SNP markers are located in the [−70; −20] region relative to the transcription start site (58), where TATA-binding protein (TBP) binds to DNA (59). Among ~2600 human DNA-binding proteins (60), TBP is among the most important ones: a knockout (61) or knockdown (62) of the TBP gene is lethal because RNA polymerase II binds to the TBP–DNA complex to induce formation of the transcription preinitiation complex (58). Many experiments have shown that an increase in TBP’s affinity for the promoter of a gene manifests itself in overexpression of this gene and vice versa (63–65). Finally, data on high-throughput sequencing of immunoprecipitated chromatin (ChIP-Seq) validated the TBP-binding sites in most genes in yeast (66) and in mice (67). Similarly, in silico estimates that were verified by in vivo bioluminescence validated TBP-binding sites in humans (68).
Earlier, we developed a computer-based statistical estimate of SNP-caused alteration of TBP’s binding affinity for promoters (69); this estimate can predict a change in expression of the human genes associated with monogenic diseases (70). Then, we empirically verified such predictions using an electrophoretic mobility shift assay (EMSA) under equilibrium (71) and non-equilibrium (72) conditions in vitro as well as in real-time mode (73). Next, we conducted a comparison of these predictions with independent experimental data published by various authors (74–77). Finally, we developed the Web service SNP_TATA_Comparator1 (78) and showed how to use it in practice (79).
Recently, we expanded the applicability of our Web service (79) from the known SNP markers of monogenic diseases to candidate SNP markers of obesity-related complications of monogenic diseases (80). Here, we continued this extension in relation to autoimmune complications of monogenic diseases, and this work is expected to advance postgenomic predictive preventive personalized medicine (20).
Materials and Methods
DNA Sequences
We analyzed 90-bp DNA sequences {s−90 … s−1} of the proximal regions of core promoters in ancestral and minor variants (hereinafter: wt and mut variants, respectively) of the human genes from the default version of the reference human genome (where s0 is the transcription start site; si ∈ {a, c, g, t}); here, we used the current major assembly release GRCh38(NCBI)/hg38(UCSC) [in the terms used by the UCSC Genome Browser (24)]. Figure 1 shows examples of the ancestral (text box “Base sequence”) and minor variants (text box “Editable sequence”) of several biomedical and nearby candidate SNP markers in the promoters of some human genes. Arrows illustrate the process of retrieval of the ancestral DNA from Ensembl (25) on the basis of the list of transcripts for the reference human genome in GENCODE (26). The minor variants were compiled manually by introducing substitutions, deletions, and/or insertions into the ancestral variant.
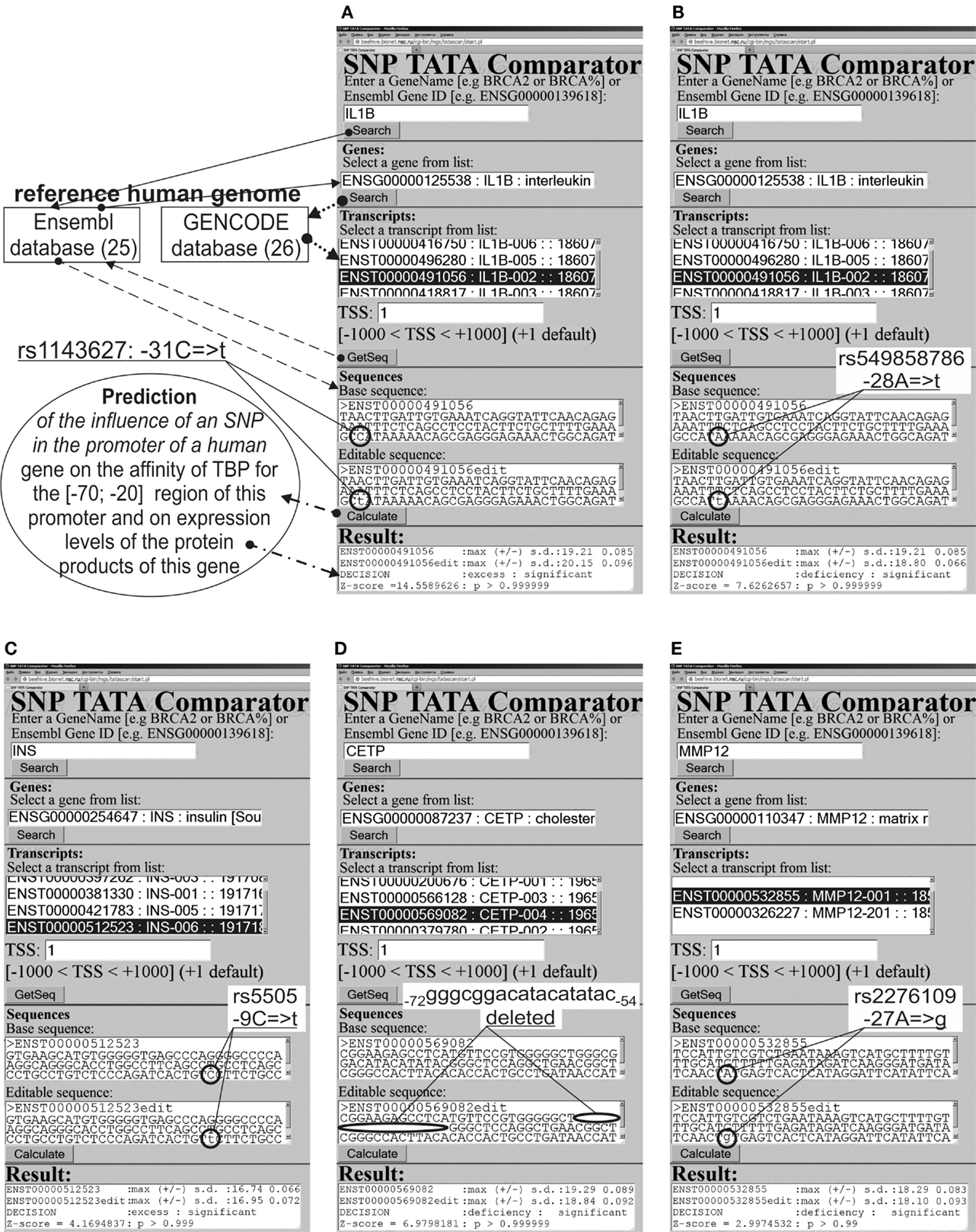
Figure 1. Examples of the predictions by SNP_TATA_Comparator (79) for statistically significant alterations in the expression of human genes. (A,C–E) Known biomedical SNP markers of autoimmune diseases; (B) the candidate SNP marker near the known SNP marker rs1143627 [see (A)].
One can find a brief description of our computer-based method for DNA sequence analysis (81–91) in Supplementary Material and a detailed description in our recent article (79).
Keyword Search
Here, we are expanding the range of applicability of our freely available Web-service (79) from monogenic diseases only to their autoimmune complications. For this purpose, we try to emulate the successful real-life expansion of diagnostic capabilities of the well-known SNP marker rs1143627 (Table 1: the first row). One can see that this marker of Graves’ disease (92, 93) was discovered in association with gastric cancer (94); after that, it was implicated in hepatocellular carcinoma (95), next in excess adiposity in older men (96), and then in non-small cell lung cancer (97), major recurrent depression (98), gastric ulcer, and chronic gastritis (99). Accordingly, we combined sequence analysis and keyword searches.
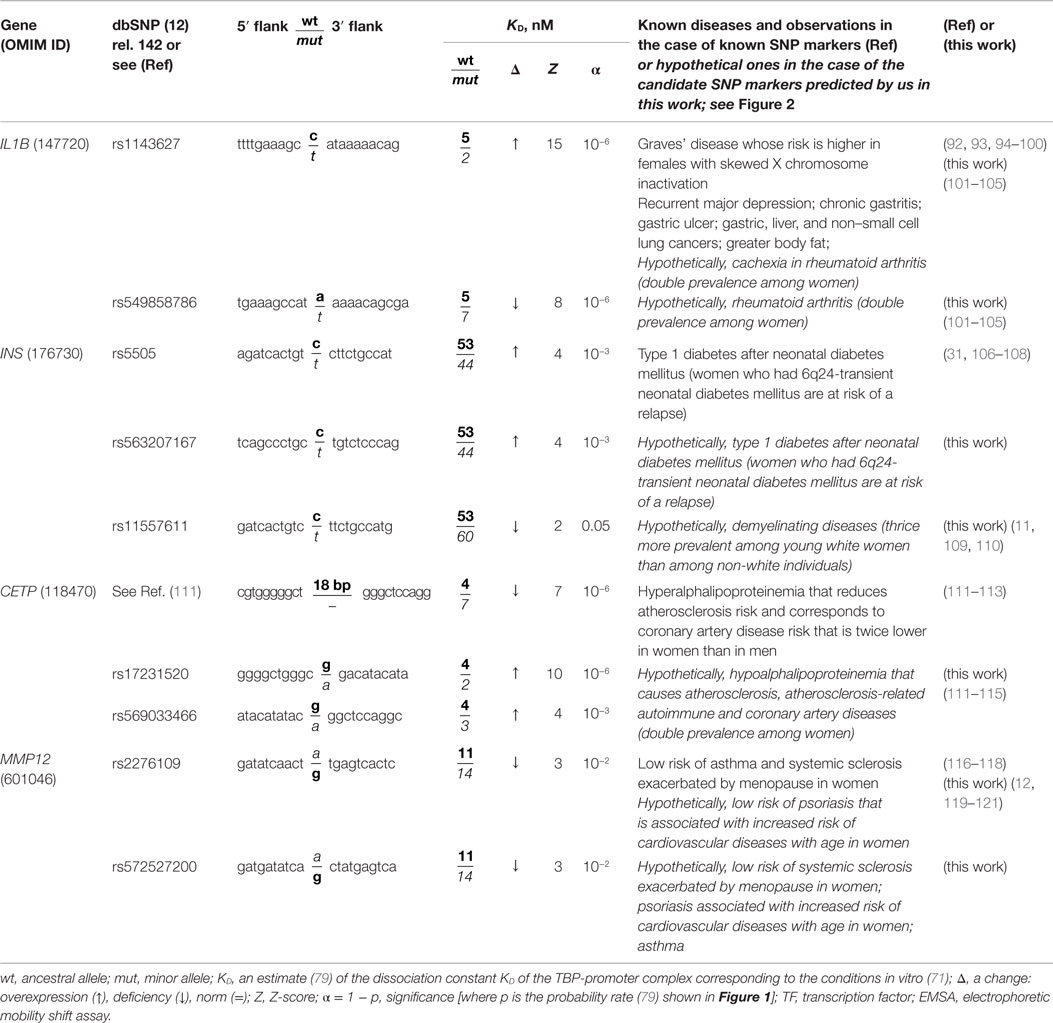
Table 1. Known and nearby candidate SNP markers (of autoimmune diseases) that can change affinity of TBP for a human gene promoter.
Figure 2 depicts a flow chart of our extension of the diagnostic potential of 68 known and candidate SNP markers (79) from monogenic diseases to gender-biased autoimmune diseases. To this end, for each SNP marker causing either significant overexpression or underexpression of the human gene containing this SNP, we manually performed a keyword search using proper combinations of the terms “overexpression,” “deficiency,” “knockout,” “women,” and many terms corresponding to various autoimmune diseases in public databases, as described in detail elsewhere (100). If we successfully found the autoimmune diseases whose biochemical marker corresponds to the expression change of the gene containing the SNP marker in question, then we did one more keyword search for co-occurrences of the found autoimmune diseases and the monogenic diseases whose SNP marker was being analyzed. This additional keyword search can serve as cross-validation of sorts at the level of a rough qualitative estimate without strict statistical criteria.
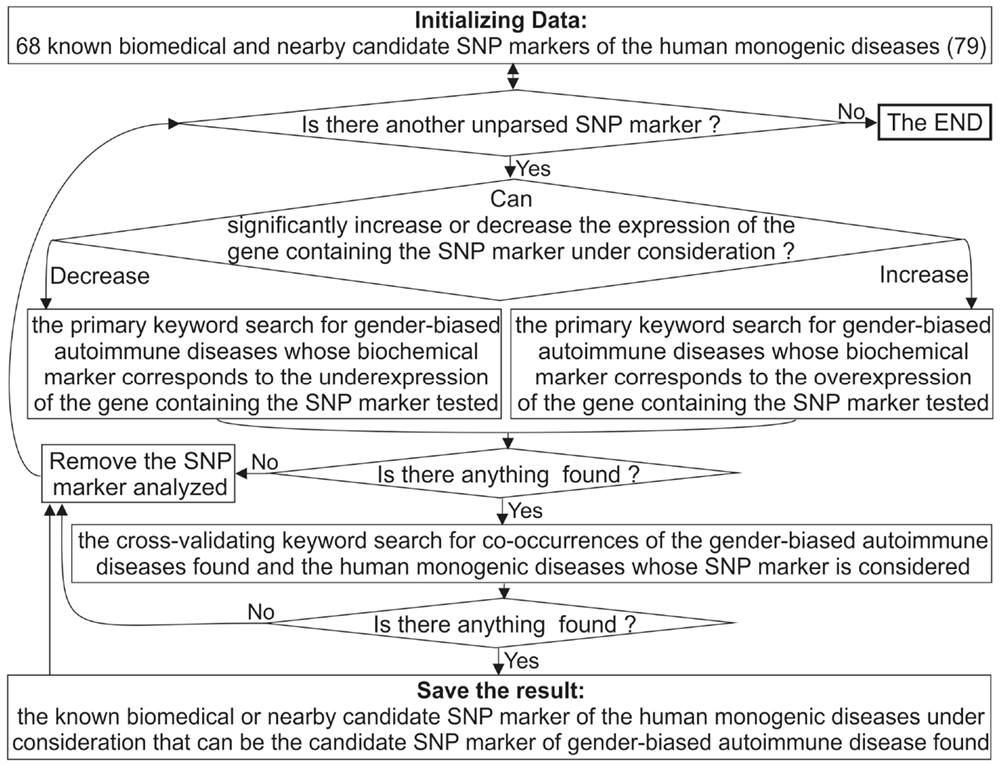
Figure 2. A flow chart showing extension of the diagnostic potential of 68 known and candidate SNP markers (79) from monogenic diseases to gender-biased autoimmune diseases.
Our heuristic interpretation of our predicted cases of significant overexpression or underexpression of the human genes is shown in italics in the second rightmost column of Tables 1–3, with the word “hypothetically” in front of these interpretations. These are clinical data found during our manual keyword search, with the corresponding references in the rightmost column of these tables [also shown in italics and marked with the phrase “(this work)”].
Results and Discussion
The Results on the Known SNP Markers of Gender-Biased Autoimmune Diseases
Table 1 shows the applicability of our Web service (79) to analysis of gender-biased autoimmune diseases. Let us consider only one example from these results in detail in order to briefly describe all the other results from Tables 1–3 in a similar way.
The Human IL1B Gene
The human IL1B gene (interleukin 1β) in its promoter contains a known SNP marker of intractable Graves’ disease (rs1143627) (92). This pathology has the highest prevalence among females with skewed X chromosome inactivation (93). This SNP is a substitution of a minor T for an ancestral C at position −31 (hereafter denoted as −31C → T) in the promoter of this gene. It converts a non-canonical variant of the TBP-binding site in DNA, C−31ATAAAA, to the canonical TATA box: T−31ATAAAA. In case of the minor allele −31T, the estimate of TBP’s affinity for the IL1B promoter (see “Materials and Methods”: Supplementary Material, Eqs. 1–4), equaling 20.15 ± 0.10 ln-units (2 nM, according to Table 1), is significantly stronger (Z = 14.56, α < 10−6) than the affinity corresponding to the ancestral allele (−31C, 19.21 ± 0.09 ln-units; 5 nM). This significant increase in affinity of TBP for the minor variant of the IL1B promoter corresponds (63–65) to overexpression of this gene (designated as “↑” in Table 1). This prediction is consistent with clinical studies showing overexpression of IL1B in patients with Graves’ disease (92).
Because clinical records of the patients with these diseases confirmed IL1B overexpression, we performed a primary keyword search for “IL1B overexpression” as a biochemical marker of “gender-biased autoimmune diseases” in various databases (hereafter, see Figure 2). The last column of Table 1 shows the result obtained. One study (101) showed an association of the biochemical marker “IL1B overexpression” with cachexia as a complication of rheumatoid arthritis (double prevalence among women). Therefore, we propose rs1143627 as a candidate SNP marker for this pathology (Table 1).
The 90-bp promoter that we studied here contains the candidate SNP marker rs549858786 reported in our recent work (79). This SNP can significantly decrease TBP’s affinity for the IL1B promoter (Figure 1B) and cause underexpression of IL1B. A primary keyword search yielded laboratory data (102) on a mouse model of human rheumatoid arthritis; these data showed that an IL1B deficiency elevates the risk of this autoimmune disease [its risk is twofold higher among women than among men (101)]. The final cross-validating keyword search (hereinafter: see Figure 2) yielded a retrospective study (103) showing significantly frequent co-occurrence of some pairs of rheumatic diseases and cancers. In addition, another research group (104) reported that rheumatoid arthritis can be a complication of gastric disease treatment that is based on non-steroidal anti-inflammatory drugs. One more paper (105) revealed that a high body-mass index is associated with a reduced risk of rheumatoid arthritis in men but not in women. These three independent findings mostly support our prediction of two rheumatoid arthritis-related candidate SNP rs1143627 and rs549858786 markers.
The Human INS Gene
The human INS gene (insulin) contains the known SNP marker of type 1 diabetes after neonatal diabetes mellitus (rs5505) (106); this mutation can increase the blood level of insulin (Figure 1C), promote the development of type 1 diabetes (107), and increase the risk of other autoimmune diseases (Table 1). In addition, it was reported that women who have 6q24-transient neonatal diabetes mellitus are at risk of a relapse (108). We predicted (79) insulin overexpression in the case of the unannotated SNP rs563207167 in the same promoter, as is the case for rs5505 (Table 1). Thus, we propose rs563207167 as a candidate SNP marker of the same gender-biased autoimmune disease (106–108).
Regarding another unannotated SNP rs11557611, we predicted (79) an insulin deficiency (Table 1), and we found (using the primary keyword search) a medical hypothesis that in vivo insulin deficiency is a possible cause of demyelinating disease (109), thrice more prevalent among young white women than among non-white individuals (11). The cross-validating keyword search pinpointed clinical cases of demyelinating diseases as a complication of type 1 diabetes in children (110). Thus, we propose rs11557611 as a candidate SNP marker of autoimmune demyelinating diseases.
The Human CETP Gene
The human CETP gene (plasma cholesteryl ester transfer protein) contains a minor variant of the promoter: the deletion G–72GGCGGACATACATATAC–54 between positions −72 and −54, which was implicated in hyperalphalipoproteinemia that reduces atherosclerosis risk (111). This effect is twofold greater in women than in men (112). This SNP significantly lowers expression of CETP (Figure 1D). Regarding two candidate SNP markers – rs17231520 and rs569033466 (located within the above-mentioned 18-bp deletion between positions −72 and −54) – we predicted (79) significant overexpression of CETP (Table 1) and linked them (by means of a primary keyword search) with hypoalphalipoproteinemia that increases the risk of premature atherosclerosis-related coronary disease and autoimmune diseases (113, 114). The cross-validating keyword search produced an article on atherosclerosis as a risk factor of coronary diseases (115). This finding may serve as a rationale for our prediction of rs17231520 and rs569033466 as candidate SNP markers of both atherosclerosis-related autoimmune disease and coronary diseases.
The Human MMP12 Gene
The human MMP12 gene (matrix metallopeptidase 12) contains the known SNP marker (rs2276109) of a reduced risk of chronic asthma in children and in smokers (116, 117) as well as with the reduced risk of systemic sclerosis (117) that is exacerbated by menopause in women (12). This SNP causes MMP12 underexpression (Figure 1E), in agreement with clinical data (118). A primary keyword search produced empirical data (119) that overexpression of this gene in human keratinocytes may be associated with psoriasis (12). After that, the cross-validating keyword search showed that asthma elevates the risk of psoriasis (120), whereas psoriasis and systemic sclerosis often co-occur (121). We also predicted another candidate SNP marker (rs572527200) (79), whose numerical values were identical to those in the case of the above-mentioned rs2276109. Thus, we propose rs2276109 and rs572527200 as candidate SNP markers of a low risk of psoriasis, asthma, and systemic sclerosis.
The Results on the Known SNP Markers of Monogenic Diseases That May Also Be Candidate SNP Markers of Gender-Biased Autoimmune Diseases
The Human HBB and HBD Genes
The human HBB and HBD genes (β- and δ-chains of hemoglobin, respectively) contain seven known SNP markers (rs34500389, rs33981098, rs33980857, rs34598529, rs33931746, rs397509430, and rs35518301) of resistance to malaria and thalassemia (Cooley’s anemia) (122). These SNPs cause underexpression of these genes (122) (Table 2). In addition, we predicted three candidate SNP markers (rs281864525, rs63750953, and rs34166473) of the same disorders (79) because these SNPs can also cause underexpression of HBB and HBD (Table 2). A primary keyword search revealed a retrospective analysis of autoimmune disease cases in children (123), where anemia is associated with a high risk of autoimmune diseases underlying hypergammaglobulinemia in women. One more cross-validating keyword search produced a review (124) showing an association between thalassemia and autoimmune diseases. Thus, we predicted ten candidate SNP markers of a gender-biased autoimmune complication of hypergammaglobulinemia (rs35518301, rs34166473, rs34500389, rs33981098, rs33980857, rs397509430, rs34598529, rs33931746, rs281864525, and rs63750953) (123).
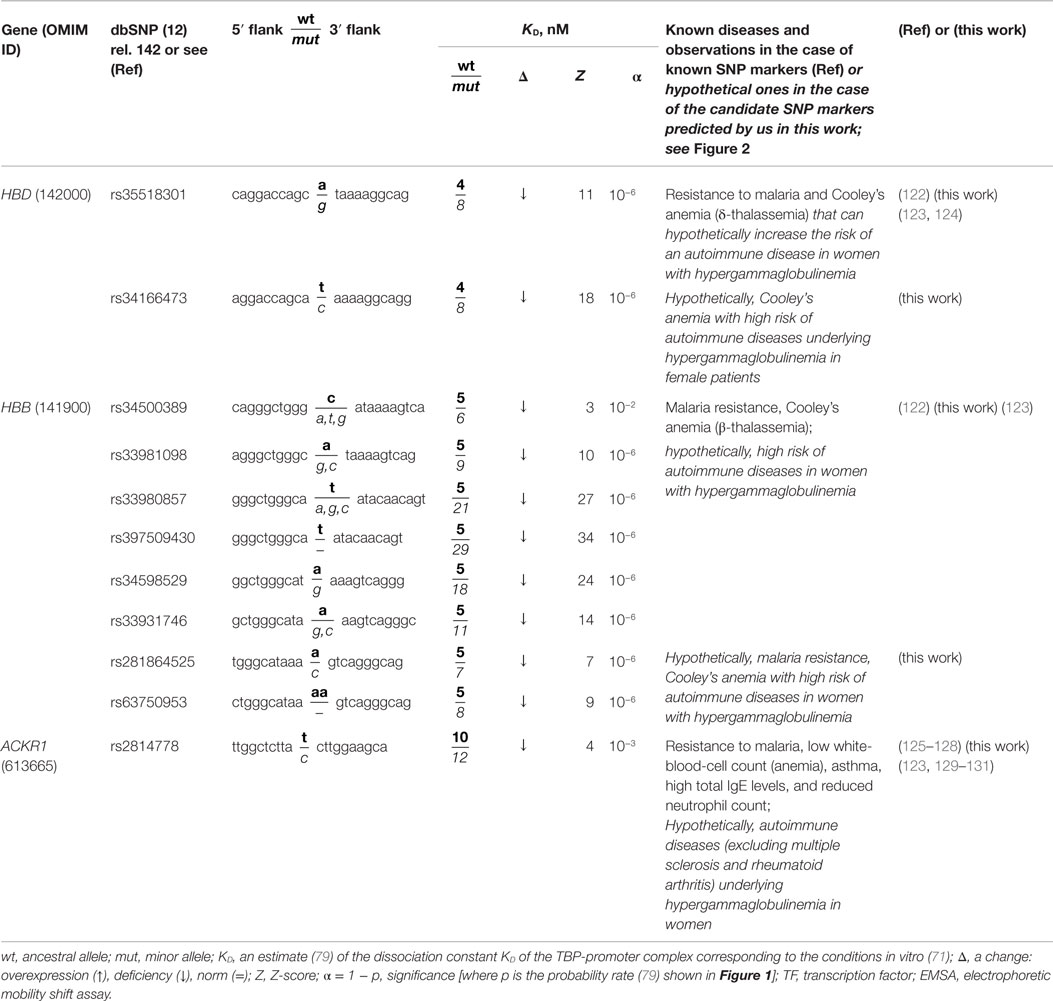
Table 2. Known and nearby candidate SNP markers (of anemias) that may be candidate SNP markers of underlying autoimmune diseases in women with hypergammaglobulinemia.
The Human ACKR1 Gene
The human ACKR1 gene (atypical chemokine receptor 1) contains the known SNP rs2814778 marker of malaria resistance (125) and of a lower white-blood cell count (126), a reduced neutrophil count (127), asthma, and high total IgE levels (128). This SNP can reduce the expression of this gene (79), in line with other studies (125–128), as shown in Table 2. A primary keyword search allowed us to propose rs2814778 as a candidate SNP marker of a lower risk of multiple sclerosis (129) and rheumatoid arthritis (130) and a candidate marker of a higher risk of other autoimmune diseases underlying hypergammaglobulinemia in women (123), as shown in Table 2. Indeed, the final cross-validating keyword search uncovered a retrospective association between asthma and subsequent autoimmune diseases diagnosed at least 5 years after asthma (131).
The Human StAR Gene
The human StAR gene (steroidogenic acute regulatory protein) contains a biomedical SNP marker of hypertension in diabetes (rs16887226) (132), with the highest risk at the waist circumference >87 cm in women and >99 cm in men (133). The EMSA showed that this SNP disrupts a tissue-specific unknown transcription factor-binding site rather than the ubiquitous TBP-binding site (132) and reduces this gene’s expression. Table 3 shows that our prediction (79) is supported by these EMSA data (132). Near this known rs16887226 marker, we predicted a candidate SNP marker of hypertension in diabetes (rs544850971) (79) because it can damage the TBP-binding site and thus reduce StAR expression, as rs16887226 does. Using a primary keywords search, we further predicted that during a deficiency in StAR as a mediator between the circadian and immune systems, both rs16887226 and rs544850971 can serve as separate candidate SNP markers of low resistance to endotoxins (134) and of a good chance for partial restoration of this resistance by training in postmenopausal women (135). Lastly, the cross-validating keyword search pinpointed a clinical association (136) between the endothelial dysfunction and the hypertension, diabetes, and endotoxemia pathologies whose candidate SNP rs16887226 and rs544850971 markers were predicted here (Table 3).
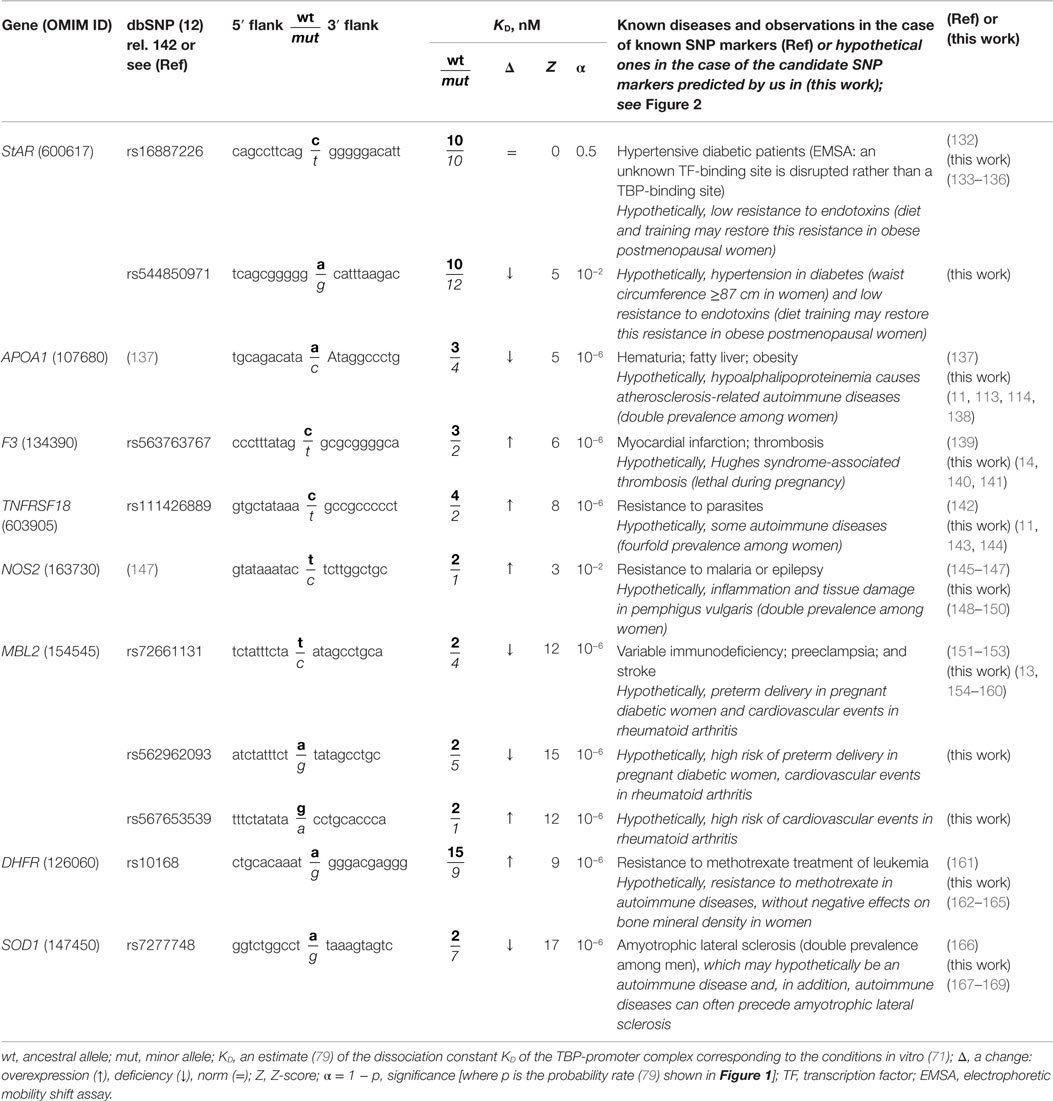
Table 3. Known and nearby candidate SNP markers (of monogenic diseases) that may also be candidate SNP markers of autoimmune diseases.
The Human APOA1 Gene
The human APOA1 gene (apolipoprotein A-I) contains the −35A → C substitution inside a proven TATA box (the canonical form of the TBP-binding sites). This substitution reduces the expression of this gene and thus is the SNP marker of hematuria, fatty liver, and obesity (137). A primary keyword search revealed a knockout APOA1−/− mouse model of human hypoalphalipoproteinemia (113) characterized by an elevated risk of atherosclerosis-related autoimmune diseases (118) [double prevalence among females (112)]. After that, the cross-validating keyword search yielded a review showing obesity-induced development of atherosclerosis in children and in adolescents (138). Thus, we predicted this known SNP marker of obesity to be a candidate SNP marker of atherosclerosis-related autoimmune diseases (Table 3).
The Human F3 Gene
The human F3 gene (coagulation factor F3) contains the known SNP rs563763767 marker of the high risk of myocardial infarction and thromboembolism whose molecular cause is F3 overexpression (139) as we predicted in silico and confirmed in our experiments in vitro (72). A primary keyword search revealed that F3 overexpression is a biochemical marker of Hughes syndrome-associated thrombosis (140), which is lethal during pregnancy (14). The cross-validating keyword search produced a clinical practice report on Hughes syndrome as an earlier easily detectable and preventable cause of myocardial ischemia (141). Thus, we predicted (Table 3) the known SNP marker of myocardial infarction (rs563763767) to be a candidate SNP marker of Hughes syndrome whose early detection is easy and can prevent (141) Hughes syndrome-associated thrombosis (140), which is lethal during pregnancy (14).
The Human TNFRSF18 Gene
The human TNFRSF18 gene (glucocorticoid-induced TNFR-related protein) contains the known SNP marker of resistance to parasites (rs111426889) (142) due to overexpression of this gene (79). A primary keyword search yielded a minireview (143) showing that TNFRSF18 overexpression can cause development of some autoimmune diseases with fourfold prevalence among women (10). Then, the cross-validating keyword search produced laboratory data showing that diabetic mice are resistant to mycobacteria, whereas a mycobacterial infection prevents this autoimmune disease (144). Thus, we predicted that the known SNP marker of resistance to parasites (rs111426889) can additionally be a candidate SNP marker of autoimmune diseases (Table 3).
The Human NOS2 Gene
The human NOS2 gene (inducible nitric oxide synthase 2) contains the −51T → C substitution as a known SNP marker of epilepsy (145) and resistance to malaria (146, 147) due to overexpression of this gene (79) (Table 3). A primary keyword search pointed to an empirical study on a mouse model of human pemphigus vulgaris (148) where NOS2 overexpression as a biochemical marker was found to be associated with inflammation and tissue damage as two complications of this autoimmune disease (148). This disease is twofold more prevalent among women than among men (149). Using the cross-validating keyword search, we found a clinical case report of pemphigus vulgaris after antiepileptic therapy (150). On this basis, we predicted that this known SNP marker of epilepsy can be a candidate SNP marker of inflammation and tissue damage as complications of pemphigus vulgaris (Table 3).
The Human MBL2 Gene
The human MBL2 gene (soluble mannose-binding lectin 2) contains a known SNP marker (rs72661131) of variable immunodeficiency (151), preeclampsia (152), and stroke (153). This SNP impairs expression of this gene, as we predicted (79) and proved in experiments under both equilibrium (71) and non-equilibrium (72) conditions in vitro. A primary keyword search produced clinical findings of a high risk of preterm delivery in pregnant diabetic women (13) and a report about cardiovascular events in rheumatoid arthritis (154); the latter is twice more frequent in women than in men (101). Near this SNP rs72661131, we found two unannotated SNPs (rs562962093 and rs567653539), which can cause the MBL2 underexpression and overexpression, respectively (Table 3). The cross-validating keyword search yielded six articles (155–160) showing that the variable immunodeficiency, preeclampsia, stroke disorders, and autoimmune diseases are clinically associated. Thus, we predicted three candidate SNP markers of preterm delivery in pregnant diabetic women (13) and cardiovascular events in rheumatoid arthritis (154) (rs72661131, rs562962093, and rs567653539; Table 3).
The Human DHFR Gene
The human DHFR gene (dihydrofolate reductase) contains the known SNP marker of resistance to methotrexate treatment in children with acute lymphoblastic leukemia (rs10168) (161). This SNP causes overexpression of DHFR (79). A primary keyword search pointed to autoimmune diseases that are commonly treated with this drug (162) because it has no negative effects on bone mineral density in women (163). Next, the cross-validating keyword search produced two clinical reports (164, 165) showing that autoimmune diseases elevate the risk of leukemia. These data favor our prediction that the known SNP marker of resistance to methotrexate treatment in leukemia (rs10168) can additionally be a candidate SNP marker of the same drug resistance in autoimmune diseases (162, 163).
The Human SOD1 Gene
The human SOD1 gene (soluble superoxide dismutase 1) contains the known SNP marker of familial amyotrophic lateral sclerosis (rs1143627) (166) caused by underexpression of this gene, as we predicted in silico (79) and proved in in vitro experiments (72). Although this degenerative disorder of the central nervous system is not generally considered an autoimmune disease, our primary keyword search revealed a relevant empirical study on a mouse model of human multiple sclerosis (167). It shows an association of amyotrophic lateral sclerosis with autoimmune diseases (167). It is worth mentioning that amyotrophic lateral sclerosis occurs twice as often in men (168); this situation is not characteristic of autoimmune diseases. The cross-validating keyword search yielded an epidemiologic review (169) of the autoimmune diseases preceding amyotrophic lateral sclerosis. Thus, we predicted the known SNP marker of amyotrophic lateral sclerosis (rs1143627) to be a candidate SNP marker of autoimmune diseases.
The Results of the Statistical Comparison between the Computationally Predicted and Experimentally Measured –ln(KD) Values of the TBP-Promoter Affinity
As a final cross-validation test, we conducted a statistical comparison between the −ln(KD) values of the TBP-promoter affinity that were predicted in silico (Tables 1–3) and measured by the EMSA in vitro (72). On an absolute natural logarithmic scale, Figure 3A shows a significant correlation, namely: linear (r = 0.75; α < 0.0025), Goodman–Kruskal’s generalized (γ = 0.53; α < 0.01), Spearman’s rank correlation (R = 0.76, α < 0.0025), and Kendall’s rank correlation (τ = 0.52; α < 0.01). On the other hand, Figure 3B shows this correlation on a relative natural logarithmic scale: r = 0.77 (α < 0.0025), γ = 0.65 (α < 0.0025), R = 0.81 (α < 0.0025), and τ = 0.65 (α < 0.0025). Thus, eight statistical tests indicated the robustness of the correlation between our predicted values (Tables 1–3) and empirical −ln(KD) values (72). This robustness can cross-validate our predictions that known and candidate SNP markers of monogenic diseases can be candidate SNP markers of autoimmune complications of these diseases.
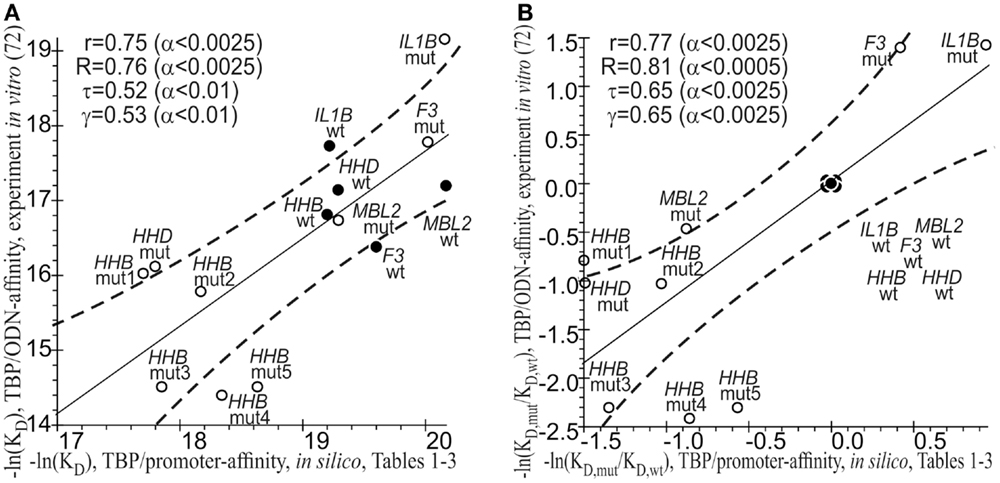
Figure 3. The significant correlations between the −ln(KD) values predicted (Tables 1–3, the x-axis) and measured by an electrophoretic mobility shift assay (EMSA) in vitro (the y-axis) from our previous article (72). (A) Absolute scales; (B) relative scales. Solid and dashed lines denote the linear regression and boundaries of its 95% confidence interval, respectively; ● and ○ are the ancestral and minor alleles, respectively; r, R, τ, γ, and α are coefficients of Pearson’s linear correlation, Spearman’s rank correlation, Kendall’s rank correlation, and Goodman–Kruskal’s generalized correlation and their significance, respectively; mut1 = rs33980857:a, mut2 = rs33980857:c, mut3 = rs34598529:g, mut4 = rs3393174:g, and mut5 = rs3393174:c; ODN, oligodeoxyribonucleotide.
How to Use Candidate SNP Markers of Autoimmune Complications of Monogenic Diseases
This study is focused on the TBP-binding site because it is the best-studied site upstream of the transcriptional start of any mRNA in the genome, where RNA polymerase II binds to the anchoring TBP–DNA complex at the stage of preinitiation complex formation (58). As continuation of our previous analysis of monogenic diseases (74–79), here, in addition to the genetic susceptibility to diseases – previously the only known SNP manifestation – we identified associations with complications of autoimmune diseases (e.g., rs1143627: autoimmune diseases often precede amyotrophic lateral sclerosis), gender specificity of such complications (e.g., rs72661131 and rs562962093: preterm delivery in pregnant diabetic women), autoimmune complications of non-autoimmune diseases (e.g., Table 2: autoimmune diseases underlying hypergammaglobulinemia in women), gender-biased epigenetic regulation of gene expression (e.g., rs1143627: skewed X chromosome inactivation), drug resistance (e.g., rs10168: resistance to methotrexate treatment of autoimmune diseases), and the effects of a lifestyle in women (e.g., rs16887226 and rs544850971: diet and training can restore resistance to endotoxins). These findings extend the field of practical applications of our Web service due to the keyword searches (100).
Be that as it may, known SNP markers of monogenic diseases are known to cause these disease, whereas the questions “What is the cause?” and “What is the consequence?” in relation to the pathogenesis of autoimmune diseases are still the focus of active biomedical research (15–19). Each candidate SNP marker predicted here is only a genome-wide informative landmark in a patient with the minor allele of this SNP; this situation can help the patient and his/her doctor to improve the lifestyle and treatment, respectively, to prevent autoimmune complications of the illness in question. As an example, here we predicted a candidate SNP marker of Hughes syndrome-associated thrombosis (rs563763767), which is lethal during pregnancy (140), whereas Hughes syndrome is easy to diagnose early and is a preventable cause of myocardial ischemia (141). Keeping this additional information in mind, a pregnant woman with the minor allele of this SNP and her physician can arrange additional diagnostic tests to monitor emergence and development of the symptoms of the relevant autoimmune complications, in addition to an adjunctive treatment during her pregnancy. Similarly, parents of the obese children or adolescents with the –35C allele of the APOA1 gene, when obesity was caused by their accelerated development and maturation, can modify the diet and lifestyle of their children to reduce the excess body fat before an imbalance of the immune system causes atherosclerosis. Moreover, two candidate SNP markers predicted by us (rs16887226 and rs544850971) would be interesting to obese postmenopausal women with the minor alleles of these SNPs who developed low resistance to endotoxins; these women can resort to training and dietary changes in order to restore this resistance (135). By the same token, all the other candidate SNP markers predicted here (Tables 1–3) may help both patients and clinicians to improve quality of life and efficiency of health care.
With this auxiliary bioinformatic approach, here we could perform only something like cross-validation with rough qualitative estimates and limitations of a keyword search in databases without exact statistical tests. Consequently, biomedical standardization of the SNP-disease association data available today (100) may advance postgenomic predictive preventive personalized medicine (20).
It should be noted that there are known problems with the computational prediction of the TBP-binding site because this site may shift depending on whether TBP interacts with an ancestral or minor allele of a human gene promoter (170). To address this problem, instead of computational prediction of the exact location of this 15-bp site within human gene promoters, we estimated the maximal value of TBP’s binding affinity for the whole 50-bp region where TBP binds to DNA of these promoters (59). In addition to the commonly accepted prediction criterion of the TBP-binding site [i.e., Bucher’s position-weight matrix score (86)], we took into account both prior and subsequent molecular events, such as TBP’s sliding along DNA (83) and stabilization of the TBP-promoter complex by bending of the DNA axis at a right angle (87), respectively (see Materials and Methods: Supplementary Material). In Figure 3, one can see the statistically significant correlations between our estimates in silico (this work) and empirical in vitro values (72) of TBP’s binding affinity for the human gene promoters. Moreover, these correlations are robust, i.e., they persist despite variations of linear, rank, or generalized correlation criteria. This robustness supports our results on the candidate SNP markers of autoimmune complications of monogenic diseases.
Finally, it is worth noting that our analysis of the candidate SNP markers of autoimmune diseases (Tables 1–3) will merely inform physicians about the degree of the molecular (e.g., KD values, Z-score, and α value) and biomedical evidence (two rightmost columns in Tables 1–3) as a rationale for expensive and labor-consuming validation of a particular SNP in a particular disease. The decisive proof would be the significantly higher frequency in patients than in healthy people, and this frequency can be confounded by climate, environmental conditions, lifestyles, and the ethnic, social, age, and gender composition of cohorts (171). Because statistical significance of the predicted SNP markers varied from high confidence (α < 10−6) to borderline significance (α < 0.05), the proposed markers should be tested according to proper biomedical standards and protocols prior to application to clinical practice. For the best targeting of our analysis, we arranged the ancestral and minor alleles of each candidate SNP marker of autoimmune diseases by KD values expressed as affinity of TBP’s binding to synthetic aptamers of double-stranded DNA 26 bp long, as we predicted for in vitro conditions (71). We found that these KD values vary from 1 to 60 nM, whereas their variation among alleles of a certain SNP is within 1 nM, which is less than 2% of the KD range. Thus, the allelic variation is too small for accurate experimental determination of differences in KD without consideration of additional data on the expected range of the values to be measured. That is why the predicted KD values (Tables 1–3) require empirical verification with sophisticated equipment (71–73).
Conclusion
Here we predicted candidate SNP markers of gender-biased autoimmune complications of monogenic diseases (Tables 1–3). They are located within TBP-binding sites of human gene promoters. Validation of these markers in accordance with clinical standards can bridge the gap between the best-studied SNPs (within protein-coding regions of genes) and the worst-studied SNPs (in regulatory regions of genes). After that, the validated SNP markers can allow physicians to select the best treatment for each patient and may help patients to choose a lifestyle reducing the risk of autoimmune complications.
Author Contributions
MP, manuscript writing; LS, manuscript editing; DR, software development and application; OA, data compilation; PP, data analysis; and NK, conceived of and supervised the study.
Conflict of Interest Statement
The authors declare that the research was conducted in the absence of any commercial or financial relationships that could be construed as a potential conflict of interest.
Acknowledgments
The authors thank Victor V. Gulevich for English translation. The English text was edited by Shevchuk Editing2 (Brooklyn, NY, USA). MP thanks Dr. Alena Zolotarenko for useful ideas.
Funding
Manuscript writing was supported by project #14-04-00485 from the Russian Foundation for Basic Research (for LS). The software development was supported by project #14-24-00123 from the Russian Scientific Foundation (for DR and NK). The data analysis and keyword search were supported by project #0324-2015-0003 (for MP and OA) from the Russian Government Budget.
Supplementary Material
The Supplementary Material for this article can be found online at http://journal.frontiersin.org/article/10.3389/fimmu.2016.00130
Footnotes
References
1. Rosenblum MD, Remedios KA, Abbas AK. Mechanisms of human autoimmunity. J Clin Invest (2015) 125:2228–33. doi: 10.1172/JCI78088
2. Zimmer M, Medcalf RL, Fink TM, Mattmann C, Lichter P, Jenne DE. Three human elastase-like genes coordinately expressed in the myelomonocyte lineage are organized as a single genetic locus on 19pter. Proc Natl Acad Sci U S A (1992) 89:8215–9. doi:10.1073/pnas.89.17.8215
3. Perricone C, Ceccarelli F, Valesini G. An overview on the genetic of rheumatoid arthritis: a never-ending story. Autoimmun Rev (2011) 10:599–608. doi:10.1016/j.autrev.2011.04.021
4. Terato K, Do CT, Shionoya H. Slipping through the cracks: linking low immune function and intestinal bacterial imbalance to the etiology of rheumatoid arthritis. Autoimmune Dis (2015) 2015:636207. doi:10.1155/2015/636207
5. Korczowska I. Rheumatoid arthritis susceptibility genes: an overview. World J Orthop (2014) 5:544–9. doi:10.5312/wjo.v5.i4.544
6. Kim HY, Kim HR, Lee SH. Advances in systems biology approaches for autoimmune diseases. Immune Netw (2014) 14:73–80. doi:10.4110/in.2014.14.2.73
7. Esteves LM, Bulhoes SM, Brilhante MJ, Mota-Vieira L. Three multiplex snapshot assays for SNP genotyping in candidate innate immune genes. BMC Res Notes (2013) 6:54. doi:10.1186/1756-0500-6-54
8. Goeldner I, Skare TL, Utiyama SR, Nisihara RM, Tong HV, Messias-Reason IJ, et al. Mannose binding lectin and susceptibility to rheumatoid arthritis in Brazilian patients and their relatives. PLoS One (2014) 9:e95519. doi:10.1371/journal.pone.0095519
9. Mohan VK, Ganesan N, Gopalakrishnan R. Association of susceptible genetic markers and autoantibodies in rheumatoid arthritis. J Genet (2014) 93:597–605. doi:10.1007/s12041-014-0380-1
10. Fairweather D, Rose NR. Women and autoimmune diseases. Emerg Infect Dis (2004) 10:2005–11. doi:10.3201/eid1011.040367
11. Papais-Alvarenga RM, Vasconcelos CC, Carra A, Castillo IS, Florentin S, Diaz de Bedoya FH, et al. Central nervous system idiopathic inflammatory demyelinating disorders in south americans: a descriptive, multicenter, cross-sectional study. PLoS One (2015) 10:e0127757. doi:10.1371/journal.pone.0127757
12. Pietrzak A, Czuczwar P, Mosiewicz J, Paszkowski T, Chodorowska G, Bartosinska J, et al. Cardiovascular disease in psoriatic post-menopausal women. J Eur Acad Dermatol Venereol (2015) 29:1231–4. doi:10.1111/jdv.12620
13. Annells MF, Hart PH, Mullighan CG, Heatley SL, Robinson JS, Bardy P, et al. Interleukins-1, -4, -6, -10, tumor necrosis factor, transforming growth factor-beta, FAS, and mannose-binding protein C gene polymorphisms in Australian women: risk of preterm birth. Am J Obstet Gynecol (2004) 191:2056–67. doi:10.1016/j.ajog.2004.04.021
14. Sun Y, Cui M, Zhu W, Xu W, Li N. A case report of a pregnancy-related death caused by primary antiphospholipid antibody syndrome. Int Med Case Rep J (2014) 7:159–63. doi:10.2147/IMCRJ.S71321
15. Sanchez B, Hevia A, González S, Margolles A. Interaction of intestinal microorganisms with the human host in the framework of autoimmune diseases. Front Immunol (2015) 6:594. doi:10.3389/fimmu.2015.00594
16. Mu Q, Zhang H, Luo XM. SLE: another autoimmune disorder influenced by microbes and diet? Front Immunol (2015) 6:608. doi:10.3389/fimmu.2015.00608
17. Keselman A, Heller NM. Estrogen signaling modulates allergic inflammation and contributes to sex differences in asthma. Front Immunol (2015) 6:568. doi:10.3389/fimmu.2015.00568
18. McDonald G, Cabal N, Vannier A, Umiker B, Yin RH, Orjalo AV Jr, et al. Female bias in systemic lupus erythematosus is associated with the differential expression of X-linked toll-like receptor 8. Front Immunol (2015) 6:457. doi:10.3389/fimmu.2015.00457
19. Nie J, Li YY, Zheng SG, Tsun A, Li B. FOXP3+ Treg cells and gender bias in autoimmune diseases. Front Immunol (2015) 6:493. doi:10.3389/fimmu.2015.00493
20. Trovato GM. Sustainable medical research by effective and comprehensive medical skills: overcoming the frontiers by predictive, preventive and personalized medicine. EPMA J (2014) 5:14. doi:10.1186/1878-5085-5-14
21. Eniu A. Integrating biological agents into systemic therapy of breast cancer: trastuzumab, lapatinib, bevacizumab. J BUON (2007) 12:S119–26.
22. Colonna V, Ayub Q, Chen Y, Pagani L, Luisi P, Pybus M, et al. Human genomic regions with exceptionally high levels of population differentiation identified from 911 whole-genome sequences. Genome Biol (2014) 15:R88. doi:10.1186/gb-2014-15-6-r88
23. Sherry ST, Ward MH, Kholodov M, Baker J, Phan L, Smigielski EM, et al. dbSNP: the NCBI database of genetic variation. Nucleic Acids Res (2001) 29:308–11. doi:10.1093/nar/29.1.308
24. Haeussler M, Raney BJ, Hinrichs AS, Clawson H, Zweig AS, Karolchik D, et al. Navigating protected genomics data with UCSC Genome Browser in a Box. Bioinformatics (2015) 31:764–6. doi:10.1093/bioinformatics/btu712
25. Zerbino DR, Wilder SP, Johnson N, Juettemann T, Flicek PR. The ensembl regulatory build. Genome Biol (2015) 16:56. doi:10.1186/s13059-015-0621-5
26. Harrow J, Frankish A, Gonzalez JM, Tapanari E, Diekhans M, Kokocinski F, et al. GENCODE: the reference human genome annotation for The ENCODE Project. Genome Res (2012) 22:1760–74. doi:10.1101/gr.135350.111
27. Abbas A, Lechevrel M, Sichel F. Identification of new single nucleotid polymorphisms (SNP) in alcohol dehydrogenase class IV ADH7 gene within a French population. Arch Toxicol (2006) 80:201–5. doi:10.1007/s00204-005-0031-7
28. Willems P, Verhagen O, Segeren C, Veenhuizen P, Guikema J, Wiemer E, et al. Consensus strategy to quantitate malignant cells in myeloma patients is validated in a multicenter study. Belgium-Dutch Hematology-Oncology Group. Blood (2000) 96:63–70.
29. Pardo LM, MacKay I, Oostra B, van Duijn CM, Aulchenko YS. The effect of genetic drift in a young genetically isolated population. Ann Hum Genet (2005) 69:288–95. doi:10.1046/J.1469-1809.2005.00162.x
30. Ou QS, Cheng ZJ, Yang B, Jiang L, Chen J. Analysis of the ratio of mitchondrial DNA with A1555G mutant to wild type in deaf patients of Fujian province in China by a new method and its relationship with the severity of hearing loss. Chin Med J (Engl) (2011) 124:3347–52.
31. Landrum MJ, Lee JM, Riley GR, Jang W, Rubinstein WS, Church DM, et al. ClinVar: public archive of relationships among sequence variation and human phenotype. Nucleic Acids Res (2014) 42:D980–5. doi:10.1093/nar/gkt1113
32. Amberger JS, Bocchini CA, Schiettecatte F, Scott AF, Hamosh A. OMIM.org: online mendelian inheritance in man (OMIM®), an online catalog of human genes and genetic disorders. Nucleic Acids Res (2015) 43:D789–98. doi:10.1093/nar/gku1205
33. International HapMap 3 Consortium, Altshuler DM, Gibbs RA, Peltonen L, Altshuler DM, Gibbs RA, et al. Integrating common and rare genetic variation in diverse human populations. Nature (2010) 467:52–8. doi:10.1038/nature09298
34. Welter D, MacArthur J, Morales J, Burdett T, Hall P, Junkins H, et al. The NHGRI GWAS Catalog, a curated resource of SNP-trait associations. Nucleic Acids Res (2014) 42:D1001–6. doi:10.1093/nar/gkt1229
35. Vasiliev GV, Merkulov VM, Kobzev VF, Merkulova TI, Ponomarenko MP, Kolchanov NA. Point mutations within 663-666 bp of intron 6 of the human TDO2 gene, associated with a number of psychiatric disorders, damage the YY-1 transcription factor binding site. FEBS Lett (1999) 462:85–8. doi:10.1016/S0014-5793(99)01513-6
36. Chen CY, Chang IS, Hsiung CA, Wasserman WW. On the identification of potential regulatory variants within genome wide association candidate SNP sets. BMC Med Genomics (2014) 7:34. doi:10.1186/1755-8794-7-34
37. Deyneko IV, Weiss S, Leschner S. An integrative computational approach to effectively guide experimental identification of regulatory elements in promoters. BMC Bioinformatics (2012) 13:202. doi:10.1186/1471-2105-13-202
38. Andersen MC, Engstrom PG, Lithwick S, Arenillas D, Eriksson P, Lenhard B, et al. In silico detection of sequence variations modifying transcriptional regulation. PLoS Comput Biol (2008) 4:e5. doi:10.1371/journal.pcbi.0040005
39. Macintyre G, Bailey J, Haviv I, Kowalczyk A. is-rSNP: a novel technique for in silico regulatory SNP detection. Bioinformatics (2010) 26:i524–30. doi:10.1093/bioinformatics/btq378
40. Deyneko IV, Kel AE, Kel-Margoulis OV, Deineko EV, Wingender E, Weiss S. MatrixCatch – a novel tool for the recognition of composite regulatory elements in promoters. BMC Bioinformatics (2013) 14:241. doi:10.1186/1471-2105-14-241
41. Boyle AP, Hong EL, Hariharan M, Cheng Y, Schaub MA, Kasowski M, et al. Annotation of functional variation in personal genomes using RegulomeDB. Genome Res (2012) 22:1790–7. doi:10.1101/gr.137323.112
42. Riva A. Large-scale computational identification of regulatory SNPs with rSNP-MAPPER. BMC Genomics (2012) 13:S7. doi:10.1186/1471-2164-13-S4-S7
43. Leschner S, Deyneko IV, Lienenklaus S, Wolf K, Bloecker H, Bumann D, et al. Identification of tumor-specific Salmonella typhimurium promoters and their regulatory logic. Nucleic Acids Res (2012) 40:2984–94. doi:10.1093/nar/gkr1041
44. Fu Y, Liu Z, Lou S, Bedford J, Mu XJ, Yip KY, et al. FunSeq2: a framework for prioritizing noncoding regulatory variants in cancer. Genome Biol (2014) 15:480. doi:10.1186/s13059-014-0480-5
45. Chen CC, Xiao S, Xie D, Cao X, Song CX, Wang T, et al. Understanding variation in transcription factor binding by modeling transcription factor genome-epigenome interactions. PLoS Comput Biol (2013) 9:e1003367. doi:10.1371/journal.pcbi.1003367
46. Deyneko IV, Bredohl B, Wesely D, Kalybaeva YM, Kel AE, Blocker H, et al. FeatureScan: revealing property-dependent similarity of nucleotide sequences. Nucleic Acids Res (2006) 34:W591–5. doi:10.1093/nar/gkl337
47. Bryzgalov LO, Antontseva EV, Matveeva MY, Shilov AG, Kashina EV, Mordvinov VA, et al. Detection of regulatory SNPs in human genome using ChIP-seq ENCODE data. PLoS One (2013) 8:e78833. doi:10.1371/journal.pone.0078833
48. Johnson AD, Handsaker RE, Pulit SL, Nizzari MM, O’Donnell CJ, de Bakker PI. SNAP: a web-based tool for identification and annotation of proxy SNPs using HapMap. Bioinformatics (2008) 24:2938–9. doi:10.1093/bioinformatics/btn564
49. Deyneko IV, Kalybaeva YM, Kel AE, Blocker H. Human-chimpanzee promoter comparisons: property-conserved evolution? Genomics (2010) 96:129–33. doi:10.1016/j.ygeno.2010.06.003
50. Coetzee SG, Rhie SK, Berman BP, Coetzee GA, Noushmehr H. FunciSNP: an R/bioconductor tool integrating functional non-coding data sets with genetic association studies to identify candidate regulatory SNPs. Nucleic Acids Res (2012) 40:e139. doi:10.1093/nar/gks542
51. Saccone SF, Bolze R, Thomas P, Quan J, Mehta G, Deelman E, et al. SPOT: a web-based tool for using biological databases to prioritize SNPs after a genome-wide association study. Nucleic Acids Res (2010) 38:W201–9. doi:10.1093/nar/gkq513
52. Barenboim M, Manke T. ChroMoS: an integrated web tool for SNP classification, prioritization and functional interpretation. Bioinformatics (2013) 29:2197–8. doi:10.1093/bioinformatics/btt356
53. Ni Y, Hall AW, Battenhouse A, Iyer VR. Simultaneous SNP identification and assessment of allele-specific bias from ChIP-seq data. BMC Genet (2012) 13:46. doi:10.1186/1471-2156-13-46
54. Hu J, Locasale JW, Bielas JH, O’Sullivan J, Sheahan K, Cantley LC, et al. Heterogeneity of tumor-induced gene expression changes in the human metabolic network. Nat Biotechnol (2013) 31:522–9. doi:10.1038/nbt.2530
55. Hein M, Graver S. Tumor cell response to bevacizumab single agent therapy in vitro. Cancer Cell Int (2013) 13:94. doi:10.1186/1475-2867-13-94
56. Ponomarenko MP, Ponomarenko JV, Frolov AS, Podkolodnaya OA, Vorobyev DG, Kolchanov NA, et al. Oligonucleotide frequency matrices addressed to recognizing functional DNA sites. Bioinformatics (1999) 15:631–43. doi:10.1093/bioinformatics/15.7.631
57. Mitsuyasu H, Izuhara K, Mao XQ, Gao PS, Arinobu Y, Enomoto T, et al. Ile50Val variant of IL4R alpha upregulates IgE synthesis and associates with atopic asthma. Nat Genet (1998) 19:119–20. doi:10.1038/472
58. Ponomarenko M, Mironova V, Gunbin K, Savinkova L. Hogness box. 2nd ed. In: Maloy S, Hughes K, editors. Brenner’s Encyclopedia of Genetics. Vol. 3, San Diego: Academic Press; Elsevier (2013). p. 491–4.
59. Savinkova LK, Ponomarenko MP, Ponomarenko PM, Drachkova IA, Lysova MV, Arshinova TV, et al. TATA box polymorphisms in human gene promoters and associated hereditary pathologies. Biochemistry (Mosc) (2009) 74:117–29. doi:10.1134/S0006297909020011
60. Babu MM, Luscombe NM, Aravind L, Gerstein M, Teichmann SA. Structure and evolution of transcriptional regulatory networks. Curr Opin Struct Biol (2004) 14:283–91. doi:10.1016/j.sbi.2004.05.004
61. Martianov I, Viville S, Davidson I. RNA polymerase II transcription in murine cells lacking the TATA binding protein. Science (2002) 298:1036–9. doi:10.1126/science.1076327
62. Muller F, Lakatos L, Dantonel J, Strahle U, Tora L. TBP is not universally required for zygotic RNA polymerase II transcription in zebrafish. Curr Biol (2001) 11:282–7. doi:10.1016/S0960-9822(01)00076-8
63. Pugh BF. Control of gene expression through regulation of the TATA-binding protein. Gene (2000) 255:1–14. doi:10.1016/S0378-1119(00)00288-2
64. Mogno I, Vallania F, Mitra RD, Cohen BA. TATA is a modular component of synthetic promoters. Genome Res (2010) 20:1391–7. doi:10.1101/gr.106732.110
65. Ponomarenko PM, Suslov VV, Savinkova LK, Ponomarenko MP, Kolchanov NA. A precise equation of equilibrium of four steps of TBP binding with the TATA box for prognosis of phenotypic manifestation of mutations. Biophysics (2010) 55:358–69. doi:10.1134/S0006350910030036
66. Rhee HS, Pugh BF. Genome-wide structure and organization of eukaryotic pre-initiation complexes. Nature (2012) 483:295–301. doi:10.1038/nature10799
67. Choukrallah MA, Kobi D, Martianov I, Pijnappel WW, Mischerikow N, Ye T, et al. Interconversion between active and inactive TATA-binding protein transcription complexes in the mouse genome. Nucleic Acids Res (2012) 40:1446–59. doi:10.1093/nar/gkr802
68. Yang MQ, Laflamme K, Gotea V, Joiner CH, Seidel NE, Wong C, et al. Genome-wide detection of a TFIID localization element from an initial human disease mutation. Nucleic Acids Res (2011) 39:2175–87. doi:10.1093/nar/gkq1035
69. Ponomarenko PM, Savinkova LK, Drachkova IA, Lysova MV, Arshinova TV, Ponomarenko MP, et al. A step-by-step model of TBP/TATA box binding allows predicting human hereditary diseases by single nucleotide polymorphism. Dokl Biochem Biophys (2008) 419:88–92. doi:10.1134/S1607672908020117
70. Ponomarenko PM, Ponomarenko MP, Drachkova IA, Lysova MV, Arshinova TV, Savinkova LK, et al. Prediction of the affinity of the TATA-binding protein to TATA boxes with single nucleotide polymorphisms. Mol Biol (2009) 43:472–9. doi:10.1134/S0026893309030157
71. Savinkova L, Drachkova I, Arshinova T, Ponomarenko P, Ponomarenko M, Kolchanov N. An experimental verification of the predicted effects of promoter TATA-box polymorphisms associated with human diseases on interactions between the TATA boxes and TATA-binding protein. PLoS One (2013) 8:e54626. doi:10.1371/journal.pone.0054626
72. Drachkova I, Savinkova L, Arshinova T, Ponomarenko M, Peltek S, Kolchanov N. The mechanism by which TATA-box polymorphisms associated with human hereditary diseases influence interactions with the TATA-binding protein. Hum Mutat (2014) 35:601–8. doi:10.1002/humu.22535
73. Arkova OV, Kuznetsov NA, Fedorova OS, Kolchanov NA, Savinkova LK. Real-time interaction between TBP and the TATA box of the human triosephosphate isomerase gene promoter in the norm and pathology. Acta Naturae (2014) 6:36–40.
74. Suslov VV, Ponomarenko PM, Efimov VM, Savinkova LK, Ponomarenko MP, Kolchanov NA. SNPs in the HIV-1 TATA box and the AIDS pandemic. J Bioinform Comput Biol (2010) 8:607–25. doi:10.1142/S0219720010004677
75. Suslov VV, Ponomarenko PM, Ponomarenko MP, Drachkova IA, Arshinova TV, Savinkova LK, et al. TATA box polymorphisms in genes of commercial and laboratory animals and plants associated with selectively valuable traits. Russ J Genet (2010) 46:394–403. doi:10.1134/S1022795410040022
76. Mironova VV, Omelyanchuk NA, Ponomarenko PM, Ponomarenko MP, Kolchanov NA. Specific/nonspecific binding of TBP to promoter DNA of the auxin response factor genes in plants correlated with ARFs function on gene transcription (activator/repressor). Dokl Biochem Biophys (2010) 433:191–6. doi:10.1134/S1607672910040125
77. Ponomarenko MP, Suslov VV, Gunbin KV, Ponomarenko PM, Vishnevsky OV, Kolchanov NA. Identification of the relationship between the variability of the expression of signaling pathway genes in the human brain and the affinity of TATA-binding protein to their promoters. Russ J Genet Appl Res (2015) 5:626–34. doi:10.1134/S2079059715060088
78. Rasskazov DA, Gunbin KV, Ponomarenko PM, Vishnevsky OV, Ponomarenko MP, Afonnikov DA. SNP_TATA_Comparator: web-service for comparison of SNPs within gene promoters associated with human diseases using the equilibrium equation of the TBP/TATA complex. Vavilov J Genet Breed (2013) 17:599–606; (in Russian).
79. Ponomarenko M, Rasskazov D, Arkova O, Ponomarenko P, Suslov V, Savinkova L, et al. How to use SNP_TATA_Comparator to find a significant change in gene expression caused by the regulatory SNP of this gene’s promoter via a change in affinity of the TATA-binding protein for this promoter. Biomed Res Int (2015) 2015:359835. doi:10.1155/2015/359835
80. Arkova OV, Ponomarenko MP, Rasskazov DA, Drachkova IA, Arshinova TV, Ponomarenko PM, et al. Obesity-related known and candidate SNP markers can significantly change affinity of TATA-binding protein for human gene promoters. BMC Genomics (2015) 16:S5. doi:10.1186/1471-2164-16-S13-S5
81. Delgadillo RF, Whittington JE, Parkhurst LK, Parkhurst LJ. The TATA-binding protein core domain in solution variably bends TATA sequences via a three-step binding mechanism. Biochemistry (2009) 48:1801–9. doi:10.1021/bi8018724
82. Hahn S, Buratowski S, Sharp PA, Guarente L. Yeast TATA-binding protein TFIID binds to TATA elements with both consensus and nonconsensus DNA sequences. Proc Natl Acad Sci U S A (1989) 86:5718–22. doi:10.1073/pnas.86.15.5718
83. Coleman RA, Pugh BF. Evidence for functional binding and stable sliding of the TATA binding protein on nonspecific DNA. J Biol Chem (1995) 270:13850–9. doi:10.1074/jbc.270.23.13850
84. Karas H, Knuppel R, Schulz W, Sklenar H, Wingender E. Combining structural analysis of DNA with search routines for the detection of transcription regulatory elements. Comput Appl Biosci (1996) 12:441–6.
85. Ponomarenko MP, Ponomarenko JV, Frolov AS, Podkolodny NL, Savinkova LK, Kolchanov NA, et al. Identification of sequence-dependent DNA features correlating to activity of DNA sites interacting with proteins. Bioinformatics (1999) 15:687–703. doi:10.1093/bioinformatics/15.7.687
86. Bucher P. Weight matrix descriptions of four eukaryotic RNA polymerase II promoter elements derived from 502 unrelated promoter sequences. J Mol Biol (1990) 212:563–78. doi:10.1016/0022-2836(90)90223-9
87. Flatters D, Lavery R. Sequence-dependent dynamics of TATA-box binding sites. Biophys J (1998) 75:372–81. doi:10.1016/S0006-3495(98)77521-6
88. Ponomarenko MP, Savinkova LK, Ponomarenko YV, Kel’ AE, Titov I, Kolchanov NA. Simulation of TATA box sequences in eukaryotes. Mol Biol (1997) 31:616–22.
89. IUPAC-IUB commission on biochemical nomenclature (CBN). Abbreviations and symbols for nucleic acids, polynucleotides and their constituents. J Mol Biol (1971) 55:299–310.
90. Ponomarenko JV, Furman DP, Frolov AS, Podkolodny NL, Orlova GV, Ponomarenko MP, et al. ACTIVITY: a database on DNA/RNA sites activity adapted to apply sequence-activity relationships from one system to another. Nucleic Acids Res (2001) 29:284–7. doi:10.1093/nar/29.1.284
91. Waardenberg AJ, Basset SD, Bouveret R, Harvey RP. CompGO: an R package for comparing and visualizing gene ontology enrichment differences between DNA binding experiments. BMC Bioinformatics (2015) 16:275. doi:10.1186/s12859-015-0701-2
92. Hayashi F, Watanabe M, Nanba T, Inoue N, Akamizu T, Iwatani Y. Association of the -31C/T functional polymorphism in the interleukin-1beta gene with the intractability of Graves’ disease and the proportion of T helper type 17 cells. Clin Exp Immunol (2009) 158:281–6. doi:10.1111/j.1365-2249.2009.04034.x
93. Simmonds MJ, Kavvoura FK, Brand OJ, Newby PR, Jackson LE, Hargreaves CE, et al. Skewed X chromosome inactivation and female preponderance in autoimmune thyroid disease: an association study and meta-analysis. J Clin Endocrinol Metab (2014) 99:E127–31. doi:10.1210/jc.2013-2667
94. El-Omar EM, Carrington M, Chow WH, McColl KE, Bream JH, Young HA, et al. Interleukin-1 polymorphisms associated with increased risk of gastric cancer. Nature (2000) 404:398–402. doi:10.1038/35006081
95. Wang Y, Kato N, Hoshida Y, Yoshida H, Taniguchi H, Goto T, et al. Interleukin-1beta gene polymorphisms associated with hepatocellular carcinoma in hepatitis C virus infection. Hepatology (2003) 37:65–71. doi:10.1053/jhep.2003.50017
96. Strandberg L, Mellstrom D, Ljunggren O, Grundberg E, Karlsson MK, Holmberg AH, et al. IL6 and IL1B polymorphisms are associated with fat mass in older men: the MrOS Study Sweden. Obesity (Silver Spring) (2008) 16:710–3. doi:10.1038/oby.2007.95
97. Wu KS, Zhou X, Zheng F, Xu XQ, Lin YH, Yang J. Influence of interleukin-1 beta genetic polymorphism, smoking and alcohol drinking on the risk of non-small cell lung cancer. Clin Chim Acta (2010) 411:1441–6. doi:10.1016/j.cca.2010.05.035
98. Borkowska P, Kucia K, Rzezniczek S, Paul-Samojedny M, Kowalczyk M, Owczarek A, et al. Interleukin-1beta promoter (-31T/C and -511C/T) polymorphisms in major recurrent depression. J Mol Neurosci (2011) 44:12–6. doi:10.1007/s12031-011-9507-5
99. Martinez-Carrillo DN, Garza-Gonzalez E, Betancourt-Linares R, Monico-Manzano T, Antunez-Rivera C, Roman-Roman A, et al. Association of IL1B -511C/-31T haplotype and Helicobacter pylori vacA genotypes with gastric ulcer and chronic gastritis. BMC Gastroenterol (2010) 10:126. doi:10.1186/1471-230X-10-126
100. Missala I, Kassner U, Steinhagen-Thiessen E. A systematic literature review of the association of lipoprotein(a) and autoimmune diseases and atherosclerosis. Int J Rheumatol (2012) 2012:480784. doi:10.1155/2012/480784
101. Walsmith J, Roubenoff R. Cachexia in rheumatoid arthritis. Int J Cardiol (2002) 85:89–99. doi:10.1016/S0167-5273(02)00237-1
102. Yamazaki H, Takeoka M, Kitazawa M, Ehara T, Itano N, Kato H, et al. ASC plays a role in the priming phase of the immune response to type II collagen in collagen-induced arthritis. Rheumatol Int (2012) 32:1625–32. doi:10.1007/s00296-011-1825-y
103. Chang SH, Park JK, Lee YJ, Yang JA, Lee EY, Song YW, et al. Comparison of cancer incidence among patients with rheumatic disease: a retrospective cohort study. Arthritis Res Ther (2014) 16:428. doi:10.1186/s13075-014-0428-x
104. Voutilainen M, Sokka T, Juhola M, Farkkila M, Hannonen P. Nonsteroidal anti-inflammatory drug-associated upper gastrointestinal lesions in rheumatoid arthritis patients. Relationships to gastric histology, Helicobacter pylori infection, and other risk factors for peptic ulcer. Scand J Gastroenterol (1998) 33:811–6. doi:10.1080/00365529850171459
105. Turesson C, Bergstrom U, Pikwer M, Nilsson JA, Jacobsson LT. A high body mass index is associated with reduced risk of rheumatoid arthritis in men, but not in women. Rheumatology (Oxford) (2015) 55:307–14. doi:10.1093/rheumatology/kev313
106. Jones AG, Hattersley AT. Reevaluation of a case of type 1 diabetes mellitus diagnosed before 6 months of age. Nat Rev Endocrinol (2010) 6:347–51. doi:10.1038/nrendo.2010.42
107. Nakayama M. Insulin as a key autoantigen in the development of type 1 diabetes. Diabetes Metab Res Rev (2011) 27:773–7. doi:10.1002/dmrr.1250
108. Temple IK, Mackay DJG, Docherty LE. Diabetes mellitus, 6q24-related transient neonatal. In: Pagon RA, Adam MP, Ardinger HH, Wallace SE, Amemiya A, Bean LJH, et al., editors. GeneReviews® [Internet]. Seattle, WA: University of Washington (2005). 135 p.
109. Gong X, Xie Z, Zuo H. In vivo insulin deficiency as a potential etiology for demyelinating disease. Med Hypotheses (2008) 71:399–403. doi:10.1016/j.mehy.2008.04.006
110. Petzold S, Kapellen T, Siekmeyer M, Hirsch W, Bartelt H, Siekmeyer W, et al. Acute cerebral infarction and extra pontine myelinolysis in children with new onset type 1 diabetes mellitus. Pediatr Diabetes (2011) 12:513–7. doi:10.1111/j.1399-5448.2010.00732.x
111. Plengpanich W, Le Goff W, Poolsuk S, Julia Z, Guerin M, Khovidhunkit W. CETP deficiency due to a novel mutation in the CETP gene promoter and its effect on cholesterol efflux and selective uptake into hepatocytes. Atherosclerosis (2011) 216:370–3. doi:10.1016/j.atherosclerosis.2011.01.051
112. Boes E, Coassin S, Kollerits B, Heid IM, Kronenberg F. Genetic-epidemiological evidence on genes associated with HDL cholesterol levels: a systematic in-depth review. Exp Gerontol (2009) 44:136–60. doi:10.1016/j.exger.2008.11.003
113. Oka K, Belalcazar LM, Dieker C, Nour EA, Nuno-Gonzalez P, Paul A, et al. Sustained phenotypic correction in a mouse model of hypoalphalipoproteinemia with a helper-dependent adenovirus vector. Gene Ther (2007) 14:191–202. doi:10.1038/sj.gt.3302819
114. Wick G, Xu Q. Atherosclerosis – an autoimmune disease. Exp Gerontol (1999) 34:559–66. doi:10.1016/S0531-5565(99)00035-2
115. Mamudu HM, Paul T, Veeranki SP, Wang L, Panchal HB, Budoff M. Subclinical atherosclerosis and relationship with risk factors of coronary artery disease in a rural population. Am J Med Sci (2015) 350:257–62. doi:10.1097/MAJ.0000000000000548
116. Hunninghake GM, Cho MH, Tesfaigzi Y, Soto-Quiros ME, Avila L, Lasky-Su J, et al. MMP12, lung function, and COPD in high-risk populations. N Engl J Med (2009) 361:2599–608. doi:10.1056/NEJMoa0904006
117. Manetti M, Ibba-Manneschi L, Fatini C, Guiducci S, Cuomo G, Bonino C, et al. Association of a functional polymorphism in the matrix metalloproteinase-12 promoter region with systemic sclerosis in an Italian population. J Rheumatol (2010) 37:1852–7. doi:10.3899/jrheum.100237
118. Woodruff PG, Koth LL, Yang YH, Rodriguez MW, Favoreto S, Dolganov GM, et al. A distinctive alveolar macrophage activation state induced by cigarette smoking. Am J Respir Crit Care Med (2005) 172:1383–92. doi:10.1164/rccm.200505-686OC
119. Starodubtseva NL, Sobolev VV, Soboleva AG, Nikolaev AA, Bruskin SA. Genes expression of metalloproteinases (MMP-1, MMP-2, MMP-9, and MMP-12) associated with psoriasis. Russ J Genet (2011) 47:1117–23. doi:10.1134/S102279541109016X
120. Egeberg A, Khalid U, Gislason GH, Mallbris L, Skov L, Hansen PR. Risk of psoriasis in patients with childhood asthma: a Danish nationwide cohort study. Br J Dermatol (2015) 173:159–64. doi:10.1111/bjd.13781
121. Walls AC, Qureshi AA. Psoriasis and concomitant fibrosing disorders: lichen sclerosus, morphea, and systemic sclerosis. J Am Acad Dermatol (2012) 67:1079–83. doi:10.1016/j.jaad.2012.04.031
122. Martiney JA, Cerami A, Slater AF. Inhibition of hemozoin formation in Plasmodium falciparum trophozoite extracts by heme analogs: possible implication in the resistance to malaria conferred by the beta-thalassemia trait. Mol Med (1996) 2:236–46.
123. Lo MS, Zurakowski D, Son MB, Sundel RP. Hypergammaglobulinemia in the pediatric population as a marker for underlying autoimmune disease: a retrospective cohort study. Pediatr Rheumatol Online J (2013) 11:42. doi:10.1186/1546-0096-11-42
124. Altinoz MA, Gedikoglu G, Deniz G. β-Thalassemia trait association with autoimmune diseases: β-globin locus proximity to the immunity genes or role of hemorphins? Immunopharmacol Immunotoxicol (2012) 34:181–90. doi:10.3109/08923973.2011.599391
125. Michon P, Woolley I, Wood EM, Kastens W, Zimmerman PA, Adams JH. Duffy-null promoter heterozygosity reduces DARC expression and abrogates adhesion of the P. vivax ligand required for blood-stage infection. FEBS Lett (2001) 495:111–4. doi:10.1016/S0014-5793(01)02370-5
126. Nalls MA, Wilson JG, Patterson NJ, Tandon A, Zmuda JM, Huntsman S, et al. Admixture mapping of white cell count: genetic locus responsible for lower white blood cell count in the Health ABC and Jackson Heart studies. Am J Hum Genet (2008) 82:81–7. doi:10.1016/j.ajhg.2007.09.003
127. Reich D, Nalls MA, Kao WH, Akylbekova EL, Tandon A, Patterson N, et al. Reduced neutrophil count in people of African descent is due to a regulatory variant in the Duffy antigen receptor for chemokines gene. PLoS Genet (2009) 5:e1000360. doi:10.1371/journal.pgen.1000360
128. Vergara C, Tsai YJ, Grant AV, Rafaels N, Gao L, Hand T, et al. Gene encoding Duffy antigen/receptor for chemokines is associated with asthma and IgE in three populations. Am J Respir Crit Care Med (2008) 178:1017–22. doi:10.1164/rccm.200801-182OC
129. Minten C, Alt C, Gentner M, Frei E, Deutsch U, Lyck R, et al. DARC shuttles inflammatory chemokines across the blood-brain barrier during autoimmune central nervous system inflammation. Brain (2014) 137:1454–69. doi:10.1093/brain/awu045
130. Smith E, McGettrick HM, Stone MA, Shaw JS, Middleton J, Nash GB, et al. Duffy antigen receptor for chemokines and CXCL5 are essential for the recruitment of neutrophils in a multicellular model of rheumatoid arthritis synovium. Arthritis Rheum (2008) 58:1968–73. doi:10.1002/art.23545
131. Hemminki K, Li X, Sundquist J, Sundquist K. Subsequent autoimmune or related disease in asthma patients: clustering of diseases or medical care? Ann Epidemiol (2010) 20:217–22. doi:10.1016/j.annepidem.2009.11.007
132. Casal AJ, Sinclair VJ, Capponi AM, Nicod J, Huynh-Do U, Ferrari P. A novel mutation in the steroidogenic acute regulatory protein gene promoter leading to reduced promoter activity. J Mol Endocrinol (2006) 37:71–80. doi:10.1677/jme.1.02082
133. Gouveia LA, Marucci Mde F, Lebrao ML, Duarte YA. Association between waist circumference (WC) values and hypertension, heart disease (HD) and diabetes, reported by the elderly – SABE Survey: health, wellness and aging, 2000 and 2006. Arch Gerontol Geriatr (2014) 59:62–8. doi:10.1016/j.archger.2014.02.005
134. Wang J, Luo Y, Wang K, Wang Y, Zhang X, Teng H, et al. Clock-controlled StAR’s expression and corticosterone production contribute to the endotoxemia immune response. Chronobiol Int (2015) 32:358–67. doi:10.3109/07420528.2014.982284
135. Phillips MD, Patrizi RM, Cheek DJ, Wooten JS, Barbee JJ, Mitchell JB. Resistance training reduces subclinical inflammation in obese, postmenopausal women. Med Sci Sports Exerc (2012) 44:2099–110. doi:10.1249/MSS.0b013e3182644984
136. Briner VA, Luscher TF. Role of vascular endothelial abnormalities in clinical medicine: atherosclerosis, hypertension, diabetes, and endotoxemia. Adv Intern Med (1994) 39:1–22.
137. Matsunaga A, Sasaki J, Han H, Huang W, Kugi M, Koga T, et al. Compound heterozygosity for an apolipoprotein A1 gene promoter mutation and a structural nonsense mutation with apolipoprotein A1 deficiency. Arterioscler Thromb Vasc Biol (1999) 19:348–55. doi:10.1161/01.ATV.19.2.348
138. Barton M. Obesity and aging: determinants of endothelial cell dysfunction and atherosclerosis. Pflugers Arch (2010) 460:825–37. doi:10.1007/s00424-010-0860-y
139. Arnaud E, Barbalat V, Nicaud V, Cambien F, Evans A, Morrison C, et al. Polymorphisms in the 5’ regulatory region of the tissue factor gene and the risk of myocardial infarction and venous thromboembolism: the ECTIM and PATHROS studies. Etude Cas-Temoins de l’Infarctus du Myocarde. Paris Thrombosis case-control Study. Arterioscler Thromb Vasc Biol (2000) 20:892–8. doi:10.1161/01.ATV.20.3.892
140. Lopez-Pedrera C, Buendia P, Barbarroja N, Siendones E, Velasco F, Cuadrado MJ. Antiphospholipid-mediated thrombosis: interplay between anticardiolipin antibodies and vascular cells. Clin Appl Thromb Hemost (2006) 12:41–5. doi:10.1177/107602960601200107
141. Hughes G. Hughes syndrome (antiphospholipid syndrome) and myocardial infarction. Int J Clin Pract (2010) 64:1183–4. doi:10.1111/j.1742-1241.2010.02340.x
142. Velavan TP, Bechlars S, Huang X, Kremsner PG, Kun JF. Novel regulatory SNPs in the promoter region of the TNFRSF18 gene in a Gabonese population. Braz J Med Biol Res (2011) 44:418–20. doi:10.1590/S0100-879X2011007500036
143. Nocentini G, Riccardi C. GITR: a multifaceted regulator of immunity belonging to the tumor necrosis factor receptor superfamily. Eur J Immunol (2005) 35:1016–22. doi:10.1002/eji.200425818
144. Bras A, Aguas AP. Diabetes-prone NOD mice are resistant to Mycobacterium avium and the infection prevents autoimmune disease. Immunology (1996) 89:20–5. doi:10.1046/j.1365-2567.1996.d01-717.x
145. Gonzalez-Martinez JA, Moddel G, Ying Z, Prayson RA, Bingaman WE, Najm IM. Neuronal nitric oxide synthase expression in resected epileptic dysplastic neocortex. J Neurosurg (2009) 110:343–9. doi:10.3171/2008.6.17608
146. Clark IA, Rockett KA, Burgner D. Genes, nitric oxide and malaria in African children. Trends Parasitol (2003) 19:335–7. doi:10.1016/S1471-4922(03)00147-8
147. Burgner D, Rockett K, Ackerman H, Hull J, Usen S, Pinder M, et al. Haplotypic relationship between SNP and microsatellite markers at the NOS2A locus in two populations. Genes Immun (2003) 4:506–14. doi:10.1038/sj.gene.6364022
148. Marquina M, Espana A, Fernandez-Galar M, Lopez-Zabalza MJ. The role of nitric oxide synthases in pemphigus vulgaris in a mouse model. Br J Dermatol (2008) 159:68–76. doi:10.1111/j.1365-2133.2008.08582.x
149. Pires CA, Viana VB, Araújo FC, Muller SF, Oliveira MS, Carneiro FR. Evaluation of cases of pemphigus vulgaris and pemphigus foliaceus from a reference service in Para state, Brazil. An Bras Dermatol (2014) 89:556–61. doi:10.1590/abd1806-4841.20142679
150. Sehgal VN, Srivastava G. Corticosteroid-unresponsive pemphigus vulgaris following antiepileptic therapy. Int J Dermatol (1988) 27:258. doi:10.1111/j.1365-4362.1988.tb03223.x
151. Boldt AB, Culpi L, Tsuneto LT, de Souza IR, Kun JF, Petzl-Erler ML. Diversity of the MBL2 gene in various Brazilian populations and the case of selection at the mannose-binding lectin locus. Hum Immunol (2006) 67:722–34. doi:10.1016/j.humimm.2006.05.009
152. Sziller I, Babula O, Hupuczi P, Nagy B, Rigo B, Szabo G, et al. Mannose-binding lectin (MBL) codon 54 gene polymorphism protects against development of pre-eclampsia, HELLP syndrome and pre-eclampsia-associated intrauterine growth restriction. Mol Hum Reprod (2007) 13:281–5. doi:10.1093/molehr/gam003
153. Cervera A, Planas AM, Justicia C, Urra X, Jensenius JC, Torres F, et al. Genetically-defined deficiency of mannose-binding lectin is associated with protection after experimental stroke in mice and outcome in human stroke. PLoS One (2010) 5:e8433. doi:10.1371/journal.pone.0008433
154. Troelsen LN, Garred P, Christiansen B, Torp-Pedersen C, Christensen IJ, Narvestad E, et al. Double role of mannose-binding lectin in relation to carotid intima-media thickness in patients with rheumatoid arthritis. Mol Immunol (2010) 47:713–8. doi:10.1016/j.molimm.2009.10.021
155. Filipovic B, Sporcic Z, Randjelovic T, Nikolic G. Common variable immunodeficiency associated with inflammatory bowel disease and type I diabetes. Clin Med Case Rep (2009) 2:67–71.
156. Swierkot J, Lewandowicz-Uszynska A, Chlebicki A, Szmyrka-Kaczmarek M, Polanska B, Jankowski A, et al. Rheumatoid arthritis in a patient with common variable immunodeficiency: difficulty in diagnosis and therapy. Clin Rheumatol (2006) 25:92–4. doi:10.1007/s10067-005-1141-6
157. Wolfberg AJ, Lee-Parritz A, Peller AJ, Lieberman ES. Association of rheumatologic disease with preeclampsia. Obstet Gynecol (2004) 103:1190–3. doi:10.1097/01.AOG.0000126279.87151.e1
158. Zha AM, Di Napoli M, Behrouz R. Prevention of stroke in rheumatoid arthritis. Curr Neurol Neurosci Rep (2015) 15:77. doi:10.1007/s11910-015-0600-y
159. Policardo L, Seghieri G, Francesconi P, Anichini R, Franconi F, Seghieri C, et al. Gender difference in diabetes-associated risk of first-ever and recurrent ischemic stroke. J Diabetes Complications (2015) 29:713–7. doi:10.1016/j.jdiacomp.2014.12.008
160. Basu A, Yu JY, Jenkins AJ, Nankervis AJ, Hanssen KF, Henriksen T, et al. Trace elements as predictors of preeclampsia in type 1 diabetic pregnancy. Nutr Res (2015) 35:421–30. doi:10.1016/j.nutres.2015.04.004
161. Al-Shakfa F, Dulucq S, Brukner I, Milacic I, Ansari M, Beaulieu P, et al. DNA variants in region for noncoding interfering transcript of dihydrofolate reductase gene and outcome in childhood acute lymphoblastic leukemia. Clin Cancer Res (2009) 15:6931–8. doi:10.1158/1078-0432.CCR-09-0641
162. Counihan TJ, Feighery C. Immunosuppressive therapy in autoimmune disease – a review. Ir J Med Sci (1991) 160:199–205. doi:10.1007/BF02957312
163. di Munno O, Mazzantini M, Sinigaglia L, Bianchi G, Minisola G, Muratore M, et al. Effect of low dose methotrexate on bone density in women with rheumatoid arthritis: results from a multicenter cross-sectional study. J Rheumatol (2004) 31:1305–9.
164. Anderson LA, Pfeiffer RM, Landgren O, Gadalla S, Berndt SI, Engels EA. Risks of myeloid malignancies in patients with autoimmune conditions. Br J Cancer (2009) 100:822–8. doi:10.1038/sj.bjc.6604935
165. Kristinsson SY, Bjorkholm M, Hultcrantz M, Derolf AR, Landgren O, Goldin LR. Chronic immune stimulation might act as a trigger for the development of acute myeloid leukemia or myelodysplastic syndromes. J Clin Oncol (2011) 29:2897–903. doi:10.1200/JCO.2011.34.8540
166. Niemann S, Broom WJ, Brown RH Jr. Analysis of a genetic defect in the TATA box of the SOD1 gene in a patient with familial amyotrophic lateral sclerosis. Muscle Nerve (2007) 36:704–7. doi:10.1002/mus.20855
167. Ende N, Weinstein F, Chen R, Ende M. Human umbilical cord blood effect on sod mice (amyotrophic lateral sclerosis). Life Sci (2000) 67:53–9. doi:10.1016/S0024-3205(00)00602-0
168. Sun Y, Lu CJ, Chen RC, Hou WH, Li CY. Risk of amyotrophic lateral sclerosis in patients with diabetes: a nationwide population-based cohort study. J Epidemiol (2015) 25:445–51. doi:10.2188/jea.JE20140176
169. Turner MR, Goldacre R, Ramagopalan S, Talbot K, Goldacre MJ. Autoimmune disease preceding amyotrophic lateral sclerosis: an epidemiologic study. Neurology (2013) 81:1222–5. doi:10.1212/WNL.0b013e3182a6cc13
170. Zukunft J, Lang T, Richter T, Hirsch-Ernst KI, Nussler AK, Klein K, et al. A natural CYP2B6 TATA box polymorphism (-82T – > C) leading to enhanced transcription and relocation of the transcriptional start site. Mol Pharmacol (2005) 67:1772–82. doi:10.1124/mol.104.008086
Keywords: gene, promoter, TATA-binding protein, TBP-binding site, single nucleotide polymorphism, expression change, gender-biased autoimmune disease, SNP marker
Citation: Ponomarenko MP, Arkova O, Rasskazov D, Ponomarenko P, Savinkova L and Kolchanov N (2016) Candidate SNP Markers of Gender-Biased Autoimmune Complications of Monogenic Diseases Are Predicted by a Significant Change in the Affinity of TATA-Binding Protein for Human Gene Promoters. Front. Immunol. 7:130. doi: 10.3389/fimmu.2016.00130
Received: 25 September 2015; Accepted: 21 March 2016;
Published: 04 April 2016
Edited by:
Xin M. Luo, Virginia Tech, USAReviewed by:
Igor B. Rogozin, National Institutes of Health, USAAlexander V. Favorov, Johns Hopkins University, USA
Copyright: © 2016 Ponomarenko, Arkova, Rasskazov, Ponomarenko, Savinkova and Kolchanov. This is an open-access article distributed under the terms of the Creative Commons Attribution License (CC BY). The use, distribution or reproduction in other forums is permitted, provided the original author(s) or licensor are credited and that the original publication in this journal is cited, in accordance with accepted academic practice. No use, distribution or reproduction is permitted which does not comply with these terms.
*Correspondence: Mikhail P. Ponomarenko, cG9uQGJpb25ldC5uc2MucnU=