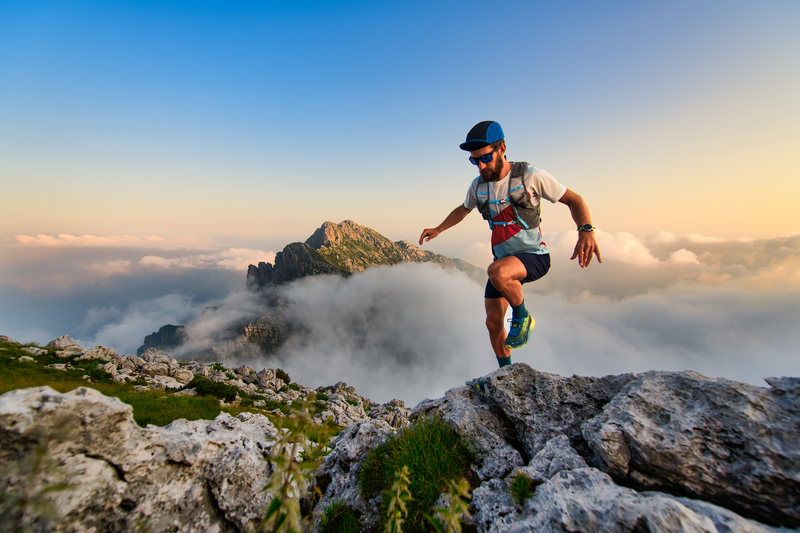
94% of researchers rate our articles as excellent or good
Learn more about the work of our research integrity team to safeguard the quality of each article we publish.
Find out more
REVIEW article
Front. Imaging , 14 February 2024
Sec. Image Capture
Volume 3 - 2024 | https://doi.org/10.3389/fimag.2024.1336829
This article is part of the Research Topic Horizons in Imaging View all 6 articles
Computational imaging technology (CIT), with its many variations, addresses the limitations of industrial design. CIT can effectively overcome the bottlenecks in physical information acquisition, model development, and resolution by being tightly coupled with mathematical calculations and signal processing in information acquisition, transmission, and interpretation. Qualitative improvements are achieved in the dimensions, scale, and resolution of the information. Therefore, in this review, the concepts and meaning of CIT are summarized before establishing a real CIT system. The basic common problems and relevant challenging technologies are analyzed, particularly the non-linear imaging model. The five typical imaging requirements–distance, resolution, applicability, field of view, and system size–are detailed. The corresponding key issues of super-large-aperture imaging systems, imaging beyond the diffraction limit, bionic optics, interpretation of light field information, computational optical system design, and computational detectors are also discussed. This review provides a global perspective for researchers to promote technological developments and applications.
Computational imaging technology (CIT) is a new imaging modality that has recently received considerable attention because of its novel physical characteristics. With the rapid development of optoelectronics (Zhang et al., 2017), information processing (Wickens and Carswell, 2021), photon integration (Fu et al., 2022), and other technical capabilities, photoelectric imaging technology is urgently required in various fields, such as remote sensing, biomedicine, deep space exploration, artificial intelligence, and resource exploration. However, owing to theoretical limitations, such as the Abbe diffraction limit, mutual restriction of a large field of view and high resolution, independent imaging links, and exponential attenuation of ballistic light intensity with an increase in propagation distance, the imaging effect of traditional imaging technology based on the object-image conjugation mode in photoelectric detection is limited by aspects such as imaging media, optical systems, and signal interpretation.
1) The imaging medium: Owing to the existence of strong scattering media due to meteorological conditions, such as haze, rain, and snow, photoelectric imaging systems cannot effectively receive information from the target directly. Sometimes, the low signal-to-noise ratio (SNR) causes a scattered light field information distribution, increasing the difficulty of SNR interpretation and reducing the effective imaging distance.
2) Optical system: The optical system cannot always simultaneously resolve the mutual conflict between the resolution and field of view, because the traditional imaging technology of the ray-tracing mode is affected by the principle and structure of the system. In addition, overcoming extremely small aberrations leads to increased complexity, volume, weight, and power consumption of the system.
3) Interpretation of the optical field information: the imaging mode with single-intensity information as the main means of detection is affected by considerable background noise that reduces the contrast between the target and the background, ease of aliasing, and difficulty in effective detection and interpretation.
Therefore, traditional imaging methods experience different degrees of information loss at the space, physical, and information levels, greatly limiting the application of photoelectric imaging systems in certain applications. Due to limitations in industrial design thinking, the development of traditional photoelectric imaging technology with “object-image conjugation” mode as the core has reached a plateau, and it is difficult to advance further. However, the emergence of CIT, which focuses on information acquisition and interpretation, lead to potential new opportunities (Lukac and Radha, 2011). CIT embraces many disciplines, such as optics, mathematics, and informatics, which makes imaging no longer solely reliant on optical hardware but also includes mathematical calculations and signal processing, breaking the limits of traditional photoelectric imaging technology. The development of optical CIT has led to the development of many new imaging technologies, such as ptychography imaging (Enders et al., 2014), lensless imaging (Monakhova et al., 2020), scattered light imaging (Cua et al., 2017), synthetic aperture imaging (Tian et al., 2023), and quantum imaging (Bogdanski et al., 2004), and has quickly become an important research direction in the imaging field globally. These developments play an important role in photoelectric detection.
Several publications analyze and condense computational imaging methods from varying standpoints. Ozcan provided a summary of recent research on emerging techniques in computational imaging, sensing, and diagnostics, as well as complementary non-computational methods that have the potential to revolutionize global healthcare delivery (Coskun and Ozcan, 2014). The IEEE even launched a journal, IEEE Transactions on Computational Imaging, in 2015 dedicated to the topic. Qionghai Dai investigated the most recent and most promising progress in computational imaging, considering the various dimensions of visual signals including spatial, temporal, angular, spectral, and phase dimensions (Hu et al., 2017). Ravindra A. Athale discusses the progress made in Computational Imaging since the mid-1990s and identified three motivations for using Computational Imaging: when a direct measurement of the desired parameter is physically impossible, when the dimensionality of the desired parameter is incompatible with present technology, and when making an indirect measurement is more advantageous than making a direct one (Mait et al., 2012).
The purpose of this paper is to investigate the potential advancements of computational imaging technology through five future application perspectives: “higher,” “farther,” “smaller,” “wider,” and “stronger,” for promoting the continuous and comprehensive development of CIT and rapid application transformation of the technology. In this study, the concept of computational imaging is described from broad and narrow perspectives, and its components are analyzed and key technologies existing in the process of technological development are summarized. This study provides a new perspective on the status quo, development, and future of CIT, which can help the development of corresponding imaging technology research and promote the further development of CIT.
CIT was born following the rapid development of information processing technology (Lee et al., 2022), micro-nano fabrication technology (Qian and Wang, 2010), artificial intelligence technology (Suo et al., 2021), and high-speed computing power (Ying et al., 2020), and is an innovation in photoelectric imaging technology. In a broad sense, all optical imaging methods introduced in the imaging process can be considered computational imaging. In addition, the use of the processing speed of powerful computers to assist or directly participate in the improvement of the imaging effect, such as image processing, belongs to computational imaging. In a narrow sense, CIT is driven by information, and the use of information acquisition, transmission, and interpretation to describe the optical imaging process, which is a multidisciplinary combination of new imaging technology, set optics, mathematics, and information technology.
Further, traditional optical imaging is “what you see is what you get, and what you get is what you see.” The information-centered computational imaging method combines a full-link imaging process with mathematical calculations and signal processing through information acquisition, transmission, and interpretation. In terms of the information dimension, scale, and resolution, information transmission is combined with mathematical analysis to overcome the bottleneck problems of difficult physical information acquisition, model development, and resolution in the imaging process, to achieve the imaging “get more than what you see, and get better than what you see” results.
CIT comprehensively considers the physical nature of the imaging process, promotes the movement of imaging system design from the traditional aberration-driven to information-driven methods, considers the full-link imaging process, and realizes the change in the information transmission mechanism. Its components can be divided into three aspects:
1) CIT designs imaging systems from the perspective of information transmission, improves the degrees of freedom of imaging, and fully excavates the light field information for the purpose of accurate information acquisition and transmission. This enables CIT to achieve a breakthrough in the “invisible,” “incomplete:” and other problems of traditional optical imaging technology.
2) From the perspective of the entire imaging process, CIT decomposes the traditional photoelectric imaging-independent optimization concept. The light field representation model migration to the transmission medium, and the imaging system into the imaging model driven by information transfer, give full play to the characteristics and advantages of the media optical system and information processing in the imaging link, breaking the limitations of traditional imaging.
3) CIT introduces the idea of information coding to broaden the information channel and increase the capacity of the information required for imaging in the form of active or passive coding. Through active coding methods such as light sources and optical system modulation, CIT expands the method of information acquisition and improves the efficiency of information collection. In addition, considering the encoding effect of the transmission medium on information, through the joint multiplexing of multi-dimensional physical quantities such as amplitude, phase, polarization, and spectrum, the interpretation ability of information is improved, and breakthroughs in imaging resolution, field of view, and action distance are achieved, turning “impossible” imaging into “possible.” However, CIT cannot process information that has not yet been acquired. Instead, it actively discards nonessential dimensional information and increases the amount of necessary dimensional information to improve the imaging performance and overcome the limitations of traditional imaging.
The concept of computational imaging has existed since the beginning of image processing; however, it was not until the 1990s that Athale first introduced it (Mait et al., 2012). Subsequently, Stanford University, Columbia University, the Massachusetts Institute of Technology, Boston University, and others formally initiated research on CIT. and established the Media Lab Camera Culture Group, Computational Imaging Lab, Hybrid Imaging Lab, Electrical and Computer Engineering Lab, and other laboratories that initiated research on CIT. In the USA, almost all top universities and research institutes have quickly established relevant laboratories and research centers. At the same time, many enterprises represented by the GelSight Company in the USA also quickly followed up and developed a series of products (Juyang et al., 1992). In the military field, the US Defense Advanced Research Projects Agency (DARPA) has set up several computational imaging related projects since 2007, such as “ARGUS-IS (Leninger et al., 2008),” “SCENICC (Sprague et al., 2012),” and “AWARE (Bar-Noy et al., 2011).” The North Atlantic Treaty Organization Science and Technology Agency also established a computational imaging task force in 2016, with defense units such as the US Army, Navy, Lockheed Martin, and the UK Ministry of Defense as the main members, and launched several projects such as SET-232 (Bosq et al., 2018).
In China, research on computational optical imaging is consistent with international activities. Corresponding laboratories and research centers were set up by Xidian University, Tsinghua University, Beijing Institute of Technology, and other universities, China Aerospace Science and Technology Corporation, Institute of Aerospace Information Research Institute, Xi'an Institute of Optics and Precision Mechanics, Changchun Institute of Optics, Fine Mechanics and Physics, and Chinese Academy of Sciences. To conduct research on computational optical imaging, the Computational Optical Imaging Technology Laboratory of the Institute of Aerospace Information Innovation, Chinese Academy of Sciences, has conducted extensive research in the fields of computational spectrum, light field, and active three dimensional (3D) imaging. The computational optical remote-sensor team at the Aerospace Information Research Institute developed and launched the world's first spaceborne computational spectral imaging payload (Liu et al., 2020). The National Information Laboratory and Institute of Optoelectronics Engineering at Tsinghua University have made important contributions (Cao et al., 2020). The Institute of Computational Imaging of Xidian University relies on the Key Laboratory of Computational Imaging of Xi'an to conduct research based on technologies such as scattered light imaging, polarization imaging, and wide area high-resolution computational imaging, and has obtained internationally recognized research results (Fei et al., 2019). The Optical Imaging and Computing Laboratory and the Measurement and Imaging Laboratory of the Beijing Institute of Technology have also proposed optimized solutions (Xinquan, 2007) for computational display and computational spectral imaging. The Intelligent Computational Imaging Laboratory of Nanjing University of Science and Technology has achieved excellent results in quantitative phase imaging, digital holographic imaging, and computational 3D imaging (Zhang et al., 2018). There are some notable research organizations in Europe that have been actively involved in computational imaging: Imperial College Computational Imaging Group (Imperial College London), Computational Imaging Group (University College London), Image and Video Analysis Group (Trinity College Dublin), Computer Vision Laboratory (ETH Zurich), Computer Graphics and Visualization Group (University of Zaragoza), Centre for Vision, Speech, and Signal Processing (University of Surrey), Max Planck Institute for Intelligent Systems (Germany), and Computer Vision and Image Processing Group (University of Verona).
Although CIT research continues, and many new imaging technologies have been derived, fragmented research has led to the difficulty of global systematic consideration of CIT, weak theoretical foundational support, and unclear application requirements. At the same time, the rapid development of Graphics Processing Unit (GPU) technology and advancements in Artificial Intelligence (AI) (Sinha et al., 2017; Barbastathis et al., 2019) have significantly contributed to the progress and application of computational imaging. In addition, as a research field covering many individual technologies, the current development ideas of CIT are disorganized. The complexity and breadth of the system make it difficult to present a clear research context, and the common basic problems and key technologies lack in-depth thinking. CIT is a type of target-oriented research technology, and its related research serves to develop or improve specific performance indicators and improve the target imaging quality by sacrificing other non-essential dimensions.
In summary, based on the demand orientation of CIT, the five application perspectives of “higher,” “farther,” “smaller,” “wider,” and “stronger” in future development are analyzed in this study. The characteristics, application prospects, and mutual relations of CIT are clearly defined, and new theories and ideas of CIT are discussed from another perspective, to promote the development of CIT in an orderly, systematic, and continuous manner.
The optical resolution indicates the fineness of the images. Generally, the higher the resolution of an image, the more information it contains. This is an important performance indicator for imaging applications. It is difficult for photoelectric imaging systems to obtain an ideal image point from a point target in conventional optical imaging systems due to the diffraction effect of light waves, which results in a diffuse spot being obtained. The larger the size of the spot, the lower the resolution. The Abbe diffraction limit indicates that the resolution of an ideal optical system is determined by the angular radius of an Airy spot. When the diffraction limit is exceeded, the image cannot be clearly observed, limiting the resolution of the system. The resolution is defined by n sinϕ = 1.22 λ/D (Abbe, 1873) [Hole Diameter: D, wavelength: λ, n: refractive index of the working medium of the lens, ϕ half of the maximum cone angle (objective lens aperture angle) at which light enters the lens]. In diffraction limited systems, the larger the aperture of the optical system, the higher the imaging resolution. However, owing to the limitations of the production technology, cost, and application scenarios, the aperture of the optical system cannot be unlimited. To achieve effective resolution improvement, CIT must explore new oversized-aperture optical systems and novel imaging techniques that exceed diffraction limits and improve image reconstruction methods.
The finiteness of the aperture of a photoelectric imaging system limits the imaging resolution. Traditional large-aperture photoelectric imaging systems under industrial design suffer from long development cycles, difficult processing and installation, high development costs, and poor environmental adaptability. In addition, they are prone to deterioration of the imaging quality owing to a decrease in the surface accuracy of the optical system. To solve this problem, it is necessary to develop a new type of ultra-large aperture optical imaging system, which is mainly realized using two synthetic aperture imaging technologies: primary mirror splicing and array complementary imaging. Compared with the traditional single-aperture photoelectric imaging system, the imaging resolution is higher, the mirror processing difficulty is lower, and the system is lighter.
In a primary mirror splicing space-based telescope, the primary mirror of the single-aperture telescope is divided into small pieces, with the pieces of sub-mirror spliced into an equivalent primary mirror, and folded in the fairing of the launch vehicle. After launch, the pieces are unfolded and reassembled through precision confocal adjustment and the resolution of the equivalent large-aperture telescope is achieved. As shown in Figure 1, the James Webb Space Telescope, which is the successor to the Hubble Telescope, was designed to use 18 hexagonal sub-mirrors with a diagonal distance of 1.5 m (Sabelhaus, 2004). However, even if primary mirror splicing technology is adopted, it is difficult to support a space telescope larger than 8–10 m in the short term until the splicing and folding technology has been improved.
Figure 1. James Webb Space Telescope (Sabelhaus, 2004).
Array complementary image telescope technology is also known as distributed synthetic aperture interferometry imaging technology (Changsheng et al., 2017). It collects light based on multiple small-aperture telescopes and then performs complementary imaging on the optical imaging telescope through an optical path delay line, baseline matching, beam pointing, and other relay optical systems. An imaging baseline is formed to achieve equivalent large-aperture effects. The larger the baseline, the higher the image resolution. These distributed devices can be launched separately, and assembled into a common frame structure in space. In theory, the array complementary image method can realize a larger aperture than the primary mirror splicing technique.
In Xiang et al. (2021) proposed a coherent synthetic aperture imaging system. Figure 2 shows a schematic diagram of the system in a scene of two synchronous orbit satellites, one of which is equipped with a camera, and the other with a laser source for angle-changing illumination.
Figure 2. Array coherent synthetic aperture imaging (CSAI) system. (A) Schematic of CSAI system, (B) simplified CSAI system, and (C) photograph of CSAI system.
To achieve imaging beyond the diffraction limit, novel imaging methods, such as structure illumination microscopy (Gustafsson, 2000), stochastic optical reconstruction microscopy (Rust et al., 2006), negative refractive super lenses (Pendry, 2000), and ptychography imaging (Wang et al., 2022), have been proposed. The ptychography imaging concept was first proposed by HOPPE W in 1970s (Hegerl and Hoppe, 1972). The core of this method is to search for a unique complex solution to satisfy the constraints of multiple far-field diffracted intensity images in overlapping scanning modes. An iterative algorithm of phase restoration is used, which sacrifices the time dimension and thus achieves an imaging result with an ultra-diffractive resolution limit. In Zheng et al. (2013) proposed the Fourier ptychographic microscopy technique that uses an objective lens with lower magnification to simulate the performance of an objective lens with higher calculated magnification. Subsequently, a reconstruction algorithm was adopted to recover the complex amplitude information of the object and obtain high-resolution images. A conventional Fourier ptychographic imaging system uses a light emitting diode (LED) plate as an illumination source. Figure 3 shows a schematic of a conventional Fourier ptychographic imaging system (Jiasong et al., 2016) and the reconstructed images, which have a much higher imaging resolution than conventional optical microscopic imaging. Although imaging beyond the diffraction limit has been accomplished in the field of microscopic imaging, there has been no similar breakthrough in large-scale macroscopic imaging applications. This is also an urgent problem that needs to be solved using computational imaging in pursuit of higher imaging resolution.
Figure 3. Fourier ptychographic microscopy system based on light emitting diode (LED) array, its imaging reconstructed results (Jiasong et al., 2016). (A) Fourier ptychographic microscopy system based on LED array, and (B) reconstructed images.
In addition to the non-interference computational imaging microscopy techniques introduced above, interferometric computational imaging techniques (Park et al., 2018) such as synthetic aperture quantitative phase imaging (Cotte et al., 2013) have doubled the maximum spatial frequency as shown in Figure 4. Alexandrov et al. (2006) introduced a novel synthetic aperture optical microscopy technique that generates high-resolution, wide-field images in both amplitude and phase using Fourier holograms. The spatial and spectral qualities of the illumination field, as well as the collection and solid angles, determine the part of the complex two-dimensional spatial frequency spectrum of an object that is captured by each hologram. They showcased the use of synthetic microscopic imaging to capture spatial frequencies that are beyond the modulation transfer function of the collection optical system, all while maintaining a long working distance and wide field of view. While its capabilities are restricted by the numerical aperture of the objective lens.
Figure 4. (A) Reconstructed image for azimuthal angle 0°; (B, C) phase images of selected areas of synthesized image; (D) confocal microscope image.
Super-resolution reconstruction is a type of information processing technique that uses low-resolution image recovery to obtain high-resolution images, and was first proposed by Harris (1964). It depends on the number of raw low-resolution images that can be classified as single-image and multi-image super-resolution. In Dong et al. (2014) applied deep learning to a natural image super-resolution reconstruction procedure and proposed a super-resolution convolutional neural network (SRCNN) model, as shown in Figure 5. To address the problem of the weak learning ability of the SRCNN shallow model, Kim et al. (2016) proposed a very deep super-resolution network model that included 20 convolutional layers. The use of a deeper network model improved the reconstruction effect. However, a deeper network model results in slower convergence speed. Lim et al. (2017) proposed an enhanced deep super-resolution reconstruction network model with 69 convolutional layers. This method reduced the memory requirements by approximately 40% and improved the convergence speed by improving the residual. The restoration results are shown in Figure 6.
Figure 5. Comparison of super-resolution reconstruction effect of convolutional neural network. (A) Image super-resolution results, and (B) peak SNR (PSNR) curve.
However, because the super-resolution reconstruction technology relies only on subsequent data processing, the result of the super-resolution reconstruction is different from the real value. The next research direction is to organically combine super-resolution reconstruction with the imaging process to achieve real-time super-resolution.
The traditional industrial design of optoelectronic imaging relies on ballistic light. Owing to the exponential decay of the energy intensity of ballistic light in imaging environments, such as clouds, smoke, and haze, the traditional imaging distance is severely limited. Moreover, for long-distance imaging, the target information presents a low SNR. For signals below 1 dB current recovery methods cannot effectively extract the target information. To pursue longer detection distances, new imaging methods that are suitable for extreme imaging environments are needed to mine and interpret imaging information.
An optical imaging system that is exposed to bad weather conditions such as fog, haze, rain, or snow, or even to underwater imaging conditions, cannot obtain the target information directly owing to the random transmission of photons, which can only result in an irregular distribution of the scattered light field. Imaging through scattering technology is a mainstream imaging technology that can recover clear target information through the deep interpretation of scattered light images carrying hidden target information. Currently, imaging through scattering technology includes wavefront shaping (Katz et al., 2011), optical memory effect (OME)-based nonvisual imaging (Osnabrugge et al., 2017), and deep learning methods.
In Vellekoop and Mosk (2007) proposed a scattered light imaging technique based on wavefront shaping (Vellekoop, 2010). The experimental setup and imaging results are shown in Figure 7. When light passes through a strong scattering medium, the phase at each position behind the scattering layer is randomly distributed, forming a speckle image as shown in Figure 7B. However, when a feedback signal is introduced, as shown in Figure 7C, the brightness of the target behind the scattering layer is three times higher than that of the speckle image, and the focusing effect is improved beyond that of an optical lens. In Vellekoop et al. (2010) used feedback-based wavefront shaping imaging technology to focus on a thickness 6 μm behind a traditional optical system. It had a spot diameter 1/10 that of traditional imaging, which significantly improved the resolution of the optical system. Subsequently, Katz et al. (2012) used a feedback-based wavefront-shaping method to achieve real-time imaging through scattering media using incoherent light sources. The imaging effect is shown in Figures 7E, F, which significantly promotes the engineering application of feedback-based wavefront shaping technology.
Figure 7. Principle of wavefront shaping based on feedback and imaging results. (A, C) Experimental diagrams, (B) speckle image, and (D) single-point focusing result. (E) Camera image with incoherent light before correction, and (F) optimized phase pattern.
In Popoff et al. (2010a) first proposed a method based on transfer matrix (TM) measurement of the scattering medium as shown in Figure 8A. The core connects the incident light field to the outgoing light field through a complex matrix (Pendry, 2000). By measuring this complex matrix and combining it with optical phase conjugation (OPC) technology, focused imaging at any position or time can be achieved (Popoff et al., 2010b; Drémeau et al., 2015). In Liutkus et al. (2014) introduced compressed sensing in TM measurements, which significantly reduced the measurement difficulty of the transmission matrix. In the same year, Andreoli et al. (2015) proposed a method for measuring TM at different wavelengths, which solved the problem of wideband-focused imaging by establishing a 3D multispectral TM. In multispectral research, Dong et al. (2018) successfully achieved transparent scattering medium imaging using a multiplexing phase-inversion method, as shown in Figure 8B.
Figure 8. Optical TM of scattering imaging and experimental results. (A) Optical TM of scattering imaging, (B) initial speckle pattern and single point focus.
The imaging through scattering technology based on optical phase conjugation obtains the original incident light-field information from the reverse light path through the reciprocity of turbid media and the invariance of the time reversal path, as shown in Figure 9 (Feld et al., 2008). Compared with feedback-based wavefront shaping technology, the measurable number of channels in this method is not a single channel but multiple channels, making it suitable for real-time measurement. In Shen et al. (2016) successfully used the digital OPC (DOPC) technology to achieve light focusing through biological tissues with a thickness of 9.6 cm and imaging of chicken breast tissue in vitro with a thickness of 2.5 cm, effectively expanding the sample thickness in OPC technology. The imaging effect (shown in Figure 9C) has a huge advantage in non-invasive optical imaging, manipulation, and treatment of deep tissues. Subsequently, Ruan et al. (2017) achieved precise control of neurons through focused imaging of 2 mm thick live brain tissue. Wavefront shaping technology has great application potential in fields such as endoscopy, super-resolution imaging, nano positioning, and cryptography.
Figure 9. Optical phase conjugation (OPC) of imaging through scattering. (A) Tissue turbidity information, and (B) OPC light field reconstruction. (C) Result of 2.5 cm isolated chicken breast tissue.
In Feng et al. (1988) and Freund et al. (1988) first proposed the OME. Specifically, the intensity of the speckle pattern obtained after scattering in the medium does not change significantly; it just changes by a small amount when the incident angle of the light wave changes within a small range. By using the OME of scattering media, Bertolotti et al. (2012) proposed a scanning-based speckle correlation imaging technique based on OME. The incident light scans the imaging target within the OME range to obtain speckle images at different angles. The target is reconstructed using a phase-recovery algorithm, as shown in Figure 10A. Although this method can achieve single-frame imaging, the complex data collection process cannot meet the requirements of real-time detection.
Figure 10. (A) Schematic of scanning-based speckle correlation imaging principle. (B) Rotation tracking results of different objects.
In addition, Katz et al. (2014) proposed a non-invasive imaging method based on single-shot speckle correlation (SSC) that does not need to consider spatial scanning and is suitable for non-invasive imaging through scattering. Through the long-term efforts of researchers, the SSC technology has emerged in multiple fields. In Stasio et al. (2015) proposed a light manipulation method for multi-core fibers by combining DOPC and OME in fiber imaging. The following year, Porat et al. (2016) also introduced SSC into fiber optic imaging, breaking the constraint that the input and output ends of traditional fiber optic endoscopes need to fit with the imaging object and image plane. In Qiao et al. (2017) proposed a non-invasive 3D light field manipulation method based on OME. The following year, Chengfei et al. (2018) tracked 3D targets hidden behind scattering media by studying the correlation of imaging objects through different positions and postures, as shown in Figure 10B.
The size of the image object in the scattered light imaging technique based on the OME is constrained by the OME range, which limits its field of view. In actual scene imaging, OME is not applicable when the scattering medium is thick and the imaging object is too large.
In a specific combat environment, such as urban street combat and military counterterrorism, it is necessary to be able to observe terrorist activities and master the initiative of counterterrorism by circumnavigating obstacles, such as streets and walls, over a long distance. Therefore, there is a need to view objects that are hidden by obstacles and that cannot be achieved using traditional optics. In the non-visual field scenario, the information carried in back-scattered light can be interpreted to realize real-time monitoring of multiple targets bypassing obstacles.
In Ramesh and Davis (2008) proposed the non-line-of-sight (NLOS) imaging technique. Based on in-depth exploration, current NLOS imaging is divided mainly into active and passive NLOS imaging. The difference between the two methods is that active NLOS imaging uses actively modulated lasers, captures photons after three scattering cycles, and completes the reconstruction of the target object by calculating the time-of-flight information of the photons. Passive NLOS imaging light sources use natural ambient light with no need for modulation to achieve the reconstruction of the hidden object.
In Raskar (2012) first used a streak tube camera to realize the 3D imaging of hidden targets. The experimental setup is shown in Figure 11. It can reconstruct images of targets at different depths with a resolution of up to centimeters and high temporal and spatial resolutions. In Gariepy et al. (2016), and then in Chan et al. (2017) achieved NLOS detection within a few seconds and realized dynamic tracking of moving targets as shown in Figure 12. In Wu et al. (2021) increased the imaging distance by three orders of magnitude for the first time, achieving 1.43 km of NLOS detection imaging and real-time tracking of hidden target objects. They designed a near-infrared, high-efficiency NLOS imaging system and improved algorithm models to solve the problems of optical attenuation and spatiotemporal information mixing caused by diffuse reflection. This system is expected to be used in real scenarios such as daily transportation, national defense, and security.
Figure 11. Active NLOS imaging technique based on striped-tube camera. (A) Imaging light path, (B) striped image, and (C) reconstruction result.
Figure 12. NLOS imaging principle and reconstruction result based on occlusion. (A) Experimental optical path, (B) raw data without occlusion, (C) original data with occlusion, (D) reconstruction result without occlusion, and (E) reconstruction result with occlusion.
Active NLOS imaging has good resolution and high accuracy. Ambient light has a small influence, and can be used for 3D reconstruction with the help of a modulated light source. However, passive NLOS imaging can achieve the reconstruction of hidden targets using natural ambient light, which makes it more suitable for practical applications. Currently, passive NLOS imaging is mainly based on spatial coherence or intensity coherence. In Batarseh et al. (2018) used a dual-phase Sagnac interferometer to reconstruct and estimate the position of hidden objects; the imaging results are shown in Figure 13A. In Saunders et al. (2019) achieved passive NLOS imaging of two-dimensional scenes based on the intensity coherence theory by incorporating occlusions of estimated positions in their experiments to obtain the spatial information of photons. The image reconstruction results are shown in Figure 13B. Although passive NLOS imaging is suitable for practical scenarios, because its light source does not require modulation, the detector receives less photon information, resulting in a low SNR and imaging resolution in Batarseh et al. (2018).
Figure 13. (A, B) The intensity distribution across the DuPSaI field of view corresponding to the square and equilateral triangle objects, respectively. (C, D) Plots of real and imaginary components of SCF measured for the square and equilateral triangle objects, respectively. The imaginary component is color coded and superposed on the 3D representation of the real part of SCF. (E, F) Variations of real and imaginary SCF components at y = 0. The corresponding apodizing function ΓA(s) is also indicated by dashed lines. (G, H) The 1D projection of the intensity distributions recovered from SCF measurements (solid lines) together with the actual intensity profiles evaluated across the targets (dotted lines).
In general, non-visual imaging can effectively detect scenes outside the visual domain, which is of great significance for applications such as military operations, public transportation safety, hostage rescue, anti-terrorism street fighting, and biomedical imaging.
With the continuous development of computer technology, many studies report on the use of deep learning to solve relevant problems in imaging through scattering. If traditional scattered light imaging is regarded as a forward propagation process, scattered light imaging based on deep learning is a reverse solution process in which the input light field information is obtained by building a suitable neural network based on the output light field intensity. In Ando et al. (2015) introduced deep learning in imaging through scattering for the first time and used a support vector machine to scatter the collected face data; non-face data intensity maps were classified. In Lyu et al. (2019) established a hybrid neural network (HNN) model to recover hidden targets in strongly scattering media, as shown in Figure 14. Although the HNN reconstruction results are similar to those of the original image, the reconstruction results based on OME do not recover the image under the same conditions. The recovery range of scattered light imaging based on deep learning is wider than that of OME. Subsequently, Li et al. (2018) trained scattering maps with different scattering media, and their network structure autonomously used the statistical features in the training data to realize the image recovery of different types of objects under different scattering media. In Lai et al. (2021) introduced the idea of transferring learning to the problem of recovering different types of objects by training images from multimode fibers (MMFs) and scattering media. MMF data was migrated to a scattering medium to achieve image recovery for different objects and scattering media.
Figure 14. Character reconstruction results. (A) Speckle pattern, (B) hybrid neural network (HNN) reconstruction results, (C) original image, and (D) reconstruction results of optical memory effect.
Scattered light imaging based on deep learning has several advantages compared with traditional scattered light imaging. For example, scattered light imaging can be realized through intensity measurement, and for strong scattering media, imaging can obtain a larger field of view. Nevertheless, it is undeniable that the deep learning method still has shortcomings such as a heavy computing burden, long computing time, high cost, and weak flexibility. Moreover, it cannot explain the physical laws of light propagation in the scattering medium. In addition, the better trained network is not well adapted to other systems, and the network structure cannot automatically adjust the parameters according to the changes in the imaging environment.
Polarization plays an irreplaceable role in the study of descattering. The current research shows that the polarization distribution characteristics of a scattered light field are closely related to the imaging distance. The polarization statistical characteristics of a scattering medium, such as water, were studied, and the intensity and polarization characteristic distribution of the scattered light field were considered globally to address the long-distance imaging problem affected by the medium.
In Tyo et al. (1996) investigated the ability of polarization difference images for recovering target information at different scattering levels, and in Tyo (2000) analyzed the point spread functions (PSFs) of polarization difference and summation images, and investigated the PSFs in single-scattering and multiple-scattering media using the Monte Carlo algorithm. The PSFs of the polarization difference images were found to be much narrower than those of the polarization summation images, as shown in Figure 15A, implying that the use of the polarization difference technique in transmission scattered light imaging can acquire target images with more high-frequency information and better imaging results.
Figure 15. (A) PSF comparison between polarization difference and polarization summing images. (B) De-hazing results in a real scene (Liu et al., 2015).
In Schechner et al. (2003) proposed a polarization haze imaging model that showed that the two types of spectral intensities received by the detector (background-scattered light and target-information light) exhibited obvious polarization differences. The distribution of target information light can be effectively resolved by the extraction and interpretation of the polarization difference information. In Panigrahi et al. (2015) obtained polarization images from snapshot polarization cameras and proposed a linear representation method for optimizing the polarization image contrast, which effectively enhanced the visual effect of long-distance imaging over 1 km. To date, polarization differential imaging technology has developed relatively maturely; however, the use of this method is limited when the haze concentration is high. To reduce the complexity of the algorithm, this method assumes that the reflected light of the target is unpolarized, which is not applicable in many scenarios. In addition, this technique is highly dependent on the sky region and is not universal.
To address these problems, Fang et al. (2014) proposed a haze-removal algorithm. For no-sky background imaging, Zhang et al. (2016) combined polarization imaging technology with dark channel prior technology and proposed a new haze removal method. Although the above methods can achieve image defogging, they do not consider the difference in the frequency domain between the target and haze. Liu et al. (2015) analyzed the characteristics of haze images based on the frequency domain distribution of the target information and haze information, and proposed a multi-scale polarization defogging technology, greatly improving the details of the image shown in Figure 15B.
In Schechner and Karpel (2006) proposed an underwater polarized imaging model based on a polarized differential imaging defogging technique combined with an image processing defogging technique that can achieve clear underwater imaging. In Liu et al. (2015) achieved clear transmission scattering imaging. They combined the differences in the spatial frequency distribution of the scattering medium and atmospheric molecules with multiscale image processing based on polarized differential imaging. This was followed by further studies on underwater long-range polarization extended-range imaging (Han et al., 2017; Liu et al., 2018), as shown in Figure 16. In Hu et al. (2021) proposed a polarization differential underwater imaging technique with three degrees of freedom.
Figure 16. Underwater imaging results of polarization differential imaging technology. (A) CP (correlation peak) value curve, (B) traditional and polarization imaging results, (C) passive polarization imaging simulated results, and (D) underwater reconstructed image.
Although polarization imaging technology can effectively remove the adverse effects of scattering media, the use of polarizers and other components leads to the loss of light intensity energy, which is not conducive to remote imaging; therefore, it is necessary to develop a high-efficiency polarization detection method. In addition, the precise interpretation of the polarization information is a topic for future research focusing on polarization imaging technology.
To achieve clear imaging and target detection under harsh conditions at long or even at very long distances, signal processing needs to be optimized. According to the results of signal attenuation quantification, the signal-to-background ratio interval of the current detected signal has been analyzed and studies conducted on the extraction and recovery of weak signals from chaotic systems. Imaging targets with low SNRs at long distances usually requires a long time for information accumulation and processing. In Li et al. (2020) designed a single-photon light detection and ranging (LIDAR) imaging system for the detection of very weak single-photon signals. They designed a single-photon LIDAR imaging system to achieve 3D imaging of targets at a distance of 45 km. In 2021, this imaging system was further optimized to achieve 3D imaging of mountain targets at a distance of 200 km (Li J. Y. et al., 2021; Li X. et al., 2021), as shown in Figure 17.
Figure 17. Illustration of single photon long-range imaging over 200 km. (A) Photograph of mountains, (B) experimental setup, (C) experimental hardware, (D) experimental environment, and (E) 3D profile of mountains.
The traditional optical system design, under the guidance of industrial design ideas, is driven by aberrations. To satisfy the requirements of the imaging field of view, focal length, and image quality, multiple lens combinations made of different materials are required to eliminate aberrations. Such systems are often complex in structure, large in size, and heavy, and an increase in the number of lenses causes difficulties in processing technology. It is difficult to realize miniaturized and lightweight photoelectric imaging systems.
Insect compound eyes are small, have a large field-of-view angle, and are sensitive to high-speed moving objects. Inspired by their unique imaging modes, the compound eye-like optical system achieves wide-area high-resolution imaging by mimicking biological visual mechanisms, which significantly improves the imaging performance of optoelectronic imaging and detection equipment. In Brady and Hagen (2009) proposed the TOMBO compound eye imaging system, which was designed using the juxtaposed compound eye structure of dragonflies as a reference, as shown in Figure 18. Each aperture images the full field of view. Compared with traditional single-aperture imaging, the multi-aperture imaging method effectively improves the information capacity of the system. Although multi-scale imaging is obviously different from the multi-aperture imaging approach, the multi-scale system adopts a multi-stage system cascade to achieve high-performance imaging, and most of the more mature imaging systems currently contain only two stages (Figure 18C), large-scale primary optics and small-scale secondary optics. The large-scale primary optics are used mainly to collect as much light energy as possible and to perform the initial aberration correction, whereas small-scale secondary optics are used mainly to secondarily transmit the light passing through the primary optics and image it onto the detector behind the secondary optics. Overall, however, the compound eye-like optical imaging technology not only effectively solves the problem of constraints between a large field of view and high resolution but also significantly reduces the size, weight, and power consumption of the imaging system.
Figure 18. Principle of multi-aperture imaging. (A) Traditional imaging, (B) 3 × 3 multi-aperture imaging, and (C) 5 × 5 multi-aperture imaging.
Since 2012, the Swiss Federal Institute of Technology in Lausanne has successively developed Panoptic (Afshari et al., 2012; Popovic et al., 2014), OMNI-R (Akin et al., 2013), GigaEye-1 (Cogal et al., 2014), GigaEye-2 (Popovic, 2016), and other multi-aperture imaging systems, among which, the OMNI-R has a full-field-of-view angle as high as 360° × 100°, and its structure is similar to that of GigaEye-1. However, GigaEye-1 supports two imaging modes and exhibits good imaging effects for both static and dynamic scenes. In 2017, an ultra-compact high-definition imitation compound eye system was developed (Cogal and Leblebici, 2016), which has a pixel count of up to 1.1 million pixels while achieving full-field-of-view of 180° × 180° imaging, and the radius of the whole system is only 5 mm. The system is equipped with a distributed illumination system, which is able to achieve dark-environmental imaging. In 2014, a new astronomical telescope was designed by Law et al. (2014, 2015) that can image an area of 384 square degrees and detect up to 16 magnitudes, which greatly improves the telescope's imaging range and detection capability.
In a traditional optical system design, the imaging link has a one-way design and independent optimization. This means that the optical design and image-processing algorithms are independent of each other, and the imaging link cannot be considered as a whole. Hence, it is easy to miss the optimal scheme for the joint design of an optical system and image processing. The minimalist optical system comprehensively considers the entire imaging link and achieves the purpose of simplifying the optical system structure and reducing costs based on the idea of global optimization to better promote the engineering application of the optical system. A comparison of the two design processes is shown in Figure 19A (Stork and Robinson, 2008).
Figure 19. (A) Traditional and global designs compared. (B) Restoration images of traditional and joint designs compared. (C) Image quality of three lens joint design and six lens traditional design compared.
In Robinson and Stork (2006) proposed a method for the joint design of an optical system, detector, and image processing for document scanners and other instruments. The method uses the mean square error (MSE) of the predicted recovered image and the optically blurred image as an evaluation index and combines it with the Wiener filtering-based image recovery algorithm to recover optically blurred images. The effect of the recovered image was better than that of the traditional design (Robinson and Stork, 2007, 2008), as shown in Figure 19B. In 2008, Robinson and Stork proposed the idea of co-designing an optical design with image restoration, which achieved end-to-end optimization by combining an optical transfer function with an image processing system using the MSE between the restored and original target images. In Li J. Y. et al. (2021) combined Zemax software and image processing through data exchange dynamic link communication technology to form a closed-loop link for optical-algorithmic joint design optimization, which reduced the difficulty of the optical system design with the system volume, weight, and cost, as shown in Figure 19C. The joint design of a simple lens can be comparable to the imaging quality of a traditionally designed complex lens.
Computational optical system design technology effectively solves the shortcomings of the traditional optical system with complex internal structure, large volume, and high cost through the whole link integration optimization design and provides strong technical support for the miniaturization, light weight, and portability of photoelectric imaging equipment.
Traditional photodetectors are based on photosensitive semiconductors. The thermal sensitivity, negative resistivity, temperature characteristics of semiconductors, and quantum effects generated for detectors of small sizes affect the detection efficiency, thus affecting the photoelectric imaging ability. Therefore, it is necessary to find new photosensitive materials and create new photosensitive components to overcome the material limitations of silicon-based semiconductors to improve the detection sensitivity and reduce the detection threshold. At present, photodetectors can only respond to light intensity. With the attenuation of light waves through long-distance transmission, the light intensity information reaching the detector is limited, and more is needed to retain the physical quantity information of other dimensions. The development of a detector with multi-dimensional physical quantity response is of great significance for achieving remote imaging.
A traditional photodetector has a fixed plane structure and depends on the correction compensation of the optical system to obtain the ability of the focusing plane target surface. This can be achieved by using different lens combinations, resulting in a complex system, image distortion, and quality degradation problems. The retinal structure of the human eye is concave, allowing imaging to be realized by relying only on the relatively simple optical structure of the lens. Inspired by this, if an imaging detector with a flexible curved surface is used, as shown in Figure 20, and the adaptive layout is conducted according to the focal plane shape of the optical system, the correction pressure of the optical system's imaging distortion can be reduced, the optical system design and complexity can be simplified, and high-resolution performance can be achieved (Zhang et al., 2017). However, current processing technology limits the preparation of curved surface detectors, and improving the process is major difficulty.
Figure 20. Curved computational detector. (A) Photograph of hemispherical FPA based on original silicon optoelectronics, (B) ray patterns traced, (C) imaging setup, and (D) image result of “W” on curved detector.
In the process of converting incident photons into discrete digital signals that can be processed by a computer, it is necessary to perform signal sampling; Nyquist sampling used by traditional detectors causes considerable data redundancy and time-consuming data processing. Therefore, it is necessary to design a new non-uniform sampling computing detector and establish a matching computational optical system design scheme so that the significance of detection and sampling focuses on the target information to improve the imaging resolution and signal processing speed. In addition, it is necessary to develop an optical processing method that can transfer the information calculated from the electrical signal processing at the back end of the detector to the front end of the detector so that the detector itself can preprocess the imaging information.
The amount of information obtained by an optical imaging system is determined by its field of view and the resolution of the optical system. A large field of view can cover a larger observation range, and a high resolution can provide more detailed information. The amount of information focused through the lens and collected by the imaging equipment is always limited because the spatial bandwidth product cannot be improved, similar to that used to determine the performance of a traditional imaging system. This results in a pair of irreconcilable contradictions between the spatial resolution and the imaging field of view. Therefore, through computational imaging, the system coding mode is used to upgrade the dimensions of the information, improve the utilization rate of the light-field information, and achieve a large field of view and high resolution.
Single-scale multi-aperture imaging is a technique used to improve and realize the function of an imaging system by mimicking biological visual mechanisms, such as the widely used fisheye lens. To achieve a large field of view and high-resolution imaging, a biomimetic multi-aperture imaging system was developed by drawing on the structure of the compound eye of arthropods, which was first proposed by Brady and Hagen (2009), to some extent solving the problem of the incompatibility of a large field of view and high resolution.
In Akin et al. (2013) developed a high-resolution imaging system inspired by the panoramic optics approach, capable of omnidirectional video recording at 30 frames per second and a resolution of 9,000 × 2,400. The imaging field of view of the system reached 360° × 100°, and the physical and photographic samples of the system are shown in Figure 21A.
Figure 21. (A) High resolution omni-directional light field imaging system and results. (B) ARGUS-IS system and imaging renderings. (C) Multi-aperture system prototype and imaging results.
ARGUS-IS (Leninger et al., 2008), an aerial camera system jointly developed by DARPA in the USA and Aerospace Systems in the UK in 2013, has a strong imaging reconnaissance capability. With 1.8 billion pixels, the system can monitor an area of more than 24 square kilometers from an altitude of 5.4 km, and detect more than 40,000 moving objects, including the identification and calibration of moving people and cars. It also has powerful information storage capabilities, as shown in Figure 21B. Its imaging resolution is sufficient to identify and track vehicles and pedestrians from an altitude of 6,500 m, ground resolution of 0.15 m, instantaneous field of view angle of 23 μrad, and can simultaneously track at least 65 targets.
In Fu et al. (2015) designed the first generation of a bionic compound eye optical system with a large field of view and low edge aperture resolution. However, the central aperture had the opposite effect (Kitamura et al., 2004). The 31 components detected the target using the edge aperture and accurately identified the target using the center aperture with a full field of view of 53.9°. In Shao et al. (2020) research group designed a multi-aperture system with a full field of view of 123.5° × 38.5°. The system also supported real-time viewing and other functions, such as images, videos, and other information. The prototype and imaging results are shown in Figure 21C.
Since Brady and Hagen (2009) proposed the theory of multi-scale imaging in 2009, this imaging method has received extensive attention from researchers worldwide. Since 2012, the AWARE-2 (Golish et al., 2012; Youn et al., 2014), AWARE-10 (Nakamura et al., 2013; Marks et al., 2014), and AWARE-40 (Nakamura et al., 2013) were developed. It takes only 18 s to actually shoot a single photograph, which effectively realizes high-resolution imaging with a large field of view. As an improved version of AWARE-2, AWARE-10 has a field of view of 100° × 60°, two billion pixels, and a resolution of 12.5 cm @ 5 km, representing a significant improvement in the number of pixels and resolution compared to AWARE-2.
In Shao et al. (2020)'s team developed a prototype multi-scale wide-area high-resolution computational optical imaging system based on the design principle of the secondary imaging system, as shown in Figure 22A (Fei et al., 2019). It had an imaging field of view of 120° × 90°, a system pixel count of 3.2 billion, and a resolution of 5 cm @ 5 km, which was capable of clearly resolving target objects within a range of 5 km. It was suitable for applications such as key area defense, border patrol, long-distance detection, and social activity surveillance. In the future, such systems can also play an important role in airborne, ground-based, and super-converged real-view reconnaissance. In the same year, Shao Xiaopeng's team designed a multi-scale system in the infrared band with a range of 8–12 μm. The system had a magnification ratio of 2 × and a focal length of 68–136 mm. The system resolution was 0.179 mrad in the telephoto mode, and 0.36 mrad in the short focal length, which was capable of effectively realizing the acquisition of targets in a large field of view and the identification of targets in a small field of view with high precision. The following year, the team miniaturized the multi-scale computational optics system, taking advantage of Galileo's compact structure to reduce the volume and complexity of the computational optics system. This resulted in a significant reduction in cost and energy consumption, and greater applicability when the system was engineered for application.
Figure 22. (A) Multi-scale computational optical imaging system and its imaging effect. (B) Optical imaging system and imaging effect of AWARE-40.
On the basis of AWARE-2 (Golish et al., 2012; Youn et al., 2014) and AWARE-10 (Nakamura et al., 2013), Brady developed an AWARE-40 (Nakamura et al., 2013; Marks et al., 2014) imaging system. Unlike AWARE-2 and AWARE-10, the primary mirror in AWARE-40 adopted a double Gaussian-like structure instead of a spherical lens, and its pixel count was up to 3.6 billion, with a resolution of up to 5.4 cm @ 5 km. The system had a superior imaging performance and could clearly detect and identify long-distance targets. The prototype and the imaging results are shown in Figure 22B. In Wu et al. (2016) comprehensively considered light, light field information, sensors, and image reconstruction in the computational imaging process and used 4D deconvolution algorithms based on a multi-scale imaging design scheme to obtain a large-field-of-view high-definition image suitable for biomedical applications.
Extreme imaging conditions are an urgent problem in photoelectric imaging technology and are also the key to improving the universality of the system. However, traditional imaging methods are limited by single-intensity detection and information interpretation. It is difficult to obtain and calculate the target information effectively under the interference of a strong background and noise, resulting in imaging failure. CIT fully mines the polarization, spectrum, and other information of the light field, conducts high-dimensional constraints through upgraded light field information, and effectively solves the target information in extreme scenes to achieve high-universality photoelectric imaging.
Hyperspectral imaging uses narrow and continuous spectra for the continuous remote sensing imaging of targets, which has great advantages in the fine classification, matching identification, and analysis of distant targets. However, the global scanning of multiple spectral bands results in tens or even hundreds of gigabytes of data per spectral data cube. Hence, rapidly locating the target information of interest from a large amount of data is a problem that needs to be solved by hyperspectral imaging technology in the future. In addition, the traditional scanning imaging method of hyperspectral imaging results in poor real-time imaging.
In Kumar et al. (2017) proposed a single-exposure multispectral imaging technique using a single camera. It sacrificed spatial dimensional information using spatial correlation and spectral decorrelation of scattered images to achieve multispectral imaging of simple structures, as shown in Figure 23.
Figure 23. (A) Schematic of multispectral imaging technique with scattering medium and monochromatic camera, (B) snapshot hyperspectral imaging.
In Monakhova et al. (2020) presented a system comprising an array of tiled spectral filters placed directly on an image sensor and a diffusion plate placed close to the sensor. Each point on the diffusion plate plane was mapped to a unique pseudorandom pattern on the spectrum filter array, which encoded multiplexed spatial spectral information. By solving the sparse constraint inverse problem, hyperspectral images were recovered at a sub-super-pixel resolution.
A spectral detector based on a monochrome camera can achieve spectral imaging with a compact and simple structure, and its cost is significantly less than that of a traditional spectral imaging system. However, the trade-off between spectral resolution and spectral range and the energy transmittance problem still exists, and the related theoretical supplement is the key research direction of spectral imaging technology in the next step.
The existing 3D imaging methods are limited by the means of interpretation and imaging equipment, and cannot satisfy the increasing demand for 3D imaging in many different application scenarios. It is difficult to achieve the universal high-precision imaging of long-range targets. Polarized 3D imaging technology, through the study of the object surface morphology and polarization characteristics of the reflected light, interprets the multi-physical information of the optical field and achieves high-precision reconstruction of the target (Miyazaki et al., 2016). The separation of the specular-diffuse reflection and the multi-value of the azimuth angle in the polarized 3D imaging method have been the core problems restricting its development in Figure 24A. In Miyazaki et al. (2002a) proposed a rotational measurement method to eliminate the multi-value of the incidence angle in specular reflection and then proposed visible and infrared wavelength measurement methods (Miyazaki et al., 2002b), which effectively avoided the multi-value of the incidence angle. With the help of the polarization of far-infrared wavelengths and high-precision measurement of the angle of incidence, they solved the multi-value problem of the angle of incidence. However, methods using far-infrared and visible light band measurement are complex and expensive. Moreover, different bands must be resolved under image matching and other issues, making the process cumbersome and complex. Many studies have proposed effective solutions to the multi-value problem of the incidence angle. On the basis of accurately obtaining the target angle of incidence, eliminating the multi-value problem of azimuth has become another challenge for researchers. Morel et al. (2006) proposed an active illumination method for the multi-value azimuth. This method solves the multi-value azimuth problem by modulating the light source in different directions; however, it cannot measure the azimuth of a moving target. Zhou et al. (2013) proposed a method to diffuse the azimuth information from the high-frequency region to the low-frequency region to solve the multi-value problem in the low-frequency region, which can achieve high-precision 3D reconstruction of the target on complex surfaces.
Figure 24. (A) Results of specular-diffuse reflection separation and 3D reconstruction of different targets. (B) 3D reconstruction results in different environments. (C) 3D reconstruction results with millimeter-level 3D imaging accuracy at a long distance.
In Kadambi et al. (2017) acquired a surface depth map of a target object using Kinect and then fused it with the polarized 3D imaging technique. They effectively recovered the detailed information of the object's surface depth map while solving the problem of a non-unique azimuthal angle and realized high-precision 3D imaging under different target conditions. The reconstruction results are shown in Figure 24B. However, this method is limited by the distance of the Kinect device and it is difficult to achieve high-precision imaging over long distances. Subsequently, Han et al. (2022) combined a deep learning approach with a monocular polarization camera to simultaneously achieve specular-diffuse reflection separation and azimuthal correction to achieve millimeter-level 3D imaging accuracy at a long distance, as shown in Figure 24C. In addition, Li X. et al. (2021) proposed a near-infrared monocular 3D computational polarization imaging method to improve the material universality. They introduced a reference gradient field in the weight constraints to globally correct the surface normal blurring of the target with inhomogeneous reflectivity, which realized the direct shape reconstruction of inhomogeneous surfaces with inhomogeneous reflectivity. This method is simple, robust, and effectively avoids the influence of changes in the reflectivity.
By increasing the dimensions of the light field information, polarization 3D imaging technology solves the problem of mutual restriction between the imaging distance and 3D accuracy in 3D imaging and effectively improves the universality of 3D imaging technology.
This improvement in the physical dimensions can relax, and achieve a universal expansion of, the imaging conditions. For extremely low-SNR decoding technology under extreme imaging conditions, an increase in the mathematical dimension is also a major development idea. A typical example is the sparse low-rank decomposition of signals, and its core introduces a sparse low-rank matrix decomposition model in mathematics for signal detection. The slow-varying background term is regarded as a low-rank term, and the weak man-made target term is regarded as a sparse term. Accordingly, with the help of the optimization model the SNR of the target signal can be greatly enhanced. Then, through the separation of the information and inverse transformation, the detection of the weak target and optical field disturbance signal in a specific domain can be achieved, which greatly improves the imaging universality. This principle is illustrated in Figure 25A (Zutao et al., 2016).
Figure 25. (A) Decomposition principle of low-rank and sparse matrix. (B) Recovery result of low-rank sparse decomposition model based on the truncation norm (LRSD-TNN). (C) Face restoration results of LRSD-TNN. (D) Reconstruction results of original image under different concentration conditions. (E) Defogging results in different scenes.
Because the solution of the sparse low-rank problem is pathological, in Cao et al. (2017) proposed a low-rank sparse decomposition model based on the truncation norm to detect the background and foreground of datasets from different video databases. The recovered background and foreground contain no noise, which meets practical requirements. Part of the processing effect is shown in Figure 25B. This idea was then used to process the face image to effectively remove shadows and reflections from the image, and the processing effect is shown in Figure 25C.
Subsequently, Fei et al. (2021) applied sparse-low-rank decomposition to underwater imaging for the first time and proposed an underwater polarimetric imaging technique based on sparse-low-rank characteristics. They established a sparse low-rank decomposition model for underwater images in the polarimetric domain, effectively separated the target and background information, and reconstructed a high-definition target image to achieve high-quality recovery of the image under the conditions of a low SNR. The imaging results are shown in Figure 25D. In the same year, Daubechies et al. (2004) and Berman et al. (2016) proposed a low-rank and dictionary expression decomposition method for haze removal in dense fog scenes, by constructing a low-rank and dictionary expression decomposition model to obtain a low-rank “haze” map. They then recovered a clear image using double and triple interpolation, producing a haze removal effect in different scenes, as shown in Figure 25E. This proves that the proposed method yields satisfactory results for hazy images in different scenes.
“Higher,” “farther,” “smaller,” “wider,” and “stronger” are the urgent needs of the next development of photoelectric imaging technology, as well as the core of the development of CIT. In many cases, CIT allows for several skills to be achieved simultaneously. For example, computational microscopy techniques (McLeod and Ozcan, 2016; Aidukas et al., 2019) able to guarantee compact, portable and low-cost imaging systems with very large field of view and super-resolution capabilities have recently demonstrated as shown in Figure 26. Polarized 3D imaging (Han et al., 2022) not only obtains target polarization information for high contrast imaging, but also achieves high-precision 3D imaging and restores the true 3D information of the target.
Figure 26. (A) Experimental setup of the low-cost computational microscopy, (B) Bayer color filter array, (C) FPM imaging scheme.
In CIT imaging, the imaging process was comprehensively considered in the entire link, and the encoding effect of the transmission medium on the imaging information was analyzed. The joint multiplexing of multi-dimensional physical quantities such as amplitude, phase, polarization, and spectrum can improve the interpretation ability of information, improve imaging resolution, imaging field of view, imaging distance, imaging equipment, and imaging universality, and finally achieve a breakthrough in imaging limits.
In the age of information technology, the emergence of powerful computational capabilities, constantly innovating information theories, new detector structures, and new technologies, such as quantum optics, have brought broader development space for optoelectronic imaging and promoted the emergence of CIT that combines traditional optics and signal processing technology. The contribution of GPU and AI (Kellman et al., 2020) to computational imaging is profound and has played a crucial role in advancing various aspects of image processing, computer vision, and related fields. GPU contribute through their parallel processing power, enabling accelerated image processing algorithms, supporting deep learning applications for image recognition and reconstruction, facilitating real-time image and video processing, and efficiently handling large datasets. Researchers have found that AI algorithms (Schmidhuber, 2015; Horisaki et al., 2016) can provide new perspectives and significant enhancements compared to traditional CIT methods, while in certain cases, specific difficulties in CIT have spurred advancements in AI architectures themselves. The symbiotic relationship between these two disciplines is expected to yield mutual benefits, particularly due to their close ties to optimization theory and application.
CIT introduces mathematical computation into the imaging physical process, is driven by imaging information transfer, integrates the design of the entire link, enhances information utilization and interpretation, and achieves revolutionary advantages that are difficult to obtain using traditional optical imaging technology. The advantages include improvement in imaging resolution, extension of imaging distance, increased imaging field of view, and reduction in the size of the optical system. CIT is expected to realize imaging of clouds and fog, living organisms, tissue imaging, NLOS imaging, and other subversive imaging applications.
The development of CIT is not only a reliable way to overcome the limitations of traditional photoelectric imaging but also an inevitable choice for the future development of photoelectric imaging technology. However, as an emerging cutting-edge crosscutting technology, many challenges remain in the development of CIT. On the one hand, the lack of basic theories leads to a lack of guidance for the interpretation of information and system design. In addition, the direction of development is unclear, resulting in fragmentation of studies and technology, and independence. Table 1 lists the advantages and disadvantages of the typical CITs.
The core of the future development of CIT is the efficient deciphering of high-dimensional light field information, which cannot be separated from the promotion of the following technologies: (1) The development of high-performance system components is the foundation of computational imaging technology, such as free-form surface optical systems (Li and Gu, 2004; Ye et al., 2017), high-performance detectors (Tan and Mohseni, 2018; Wang et al., 2020), and related fields. (2) Light field control devices such as meta-surface (Zhao et al., 2021; Yu et al., 2022; Arbabi and Faraon, 2023) technology will utilize nanostructures to introduce more physical quantities into the imaging process, achieving higher performance imaging while also solving the problem of large system volume and mass. (3) The improvement of computing power [hardware and compressive sensing theory (Qaisar et al., 2013; Rani et al., 2018), new models such as deep learning (LeCun et al., 2015; Kelleher, 2019), quantum computing methods (Hidary and Hidary, 2019; Rawat et al., 2022), photon computing (Antonik et al., 2019; Pammi et al., 2019), etc.] is still a necessary condition for CIT to further enhance. The above methods will be conducive to achieving the ambitious goals of computational imaging development, including higher, farther, smaller, wider, and stronger. In addition, CIT will offer a more systematic and integrated solution to meet imaging needs for future development. By combining the respective advantages of a variety of imaging methods, it can achieve imaging effects and application scenarios that are not possible for traditional photoelectric imaging technology, realizing imaging applications such as remote sensing imaging in space, biomedical imaging, underwater imaging, and military counter-imaging. We believe that with the continuous development of CIT and theory, computational imaging systems will be richer, more 3D, and more effective; thus, CIT can become a future-oriented imaging technology to support forward-looking, strategic scientific, and technological research areas.
MX: Conceptualization, Writing – original draft, Writing – review & editing. FL: Writing – review & editing. JL: Investigation, Writing – review & editing. XD: Investigation, Writing – review & editing. QL: Writing – original draft. XS: Conceptualization, Funding acquisition, Validation, Writing – review & editing.
The author(s) declare financial support was received for the research, authorship, and/or publication of this article. This research was funded by National Natural Science Foundation of China, grant numbers 62205259, 62075175, 61975254, and 62105254, the Open Research Fund of CAS Key Laboratory of Space Precision Measurement Technology, grant number B022420004, and National Key Laboratory of Infrared Detection Technologies grant number IRDT-23-06.
The authors declare that the research was conducted in the absence of any commercial or financial relationships that could be construed as a potential conflict of interest.
All claims expressed in this article are solely those of the authors and do not necessarily represent those of their affiliated organizations, or those of the publisher, the editors and the reviewers. Any product that may be evaluated in this article, or claim that may be made by its manufacturer, is not guaranteed or endorsed by the publisher.
Abbe, E. (1873). Beiträge zur theorie des mikroskops und der mikroskopischen wahrnehmung. Arch. Für. Mikrosk. Anat. 9, 413–468. doi: 10.1007/BF02956173
Afshari, H., Popovic, V., Tasci, T., Schmid, A., and Leblebici, Y. (2012). A spherical multi-camera system with real-time omnidirectional video acquisition capability. IEEE Trans. Consum. Electron. 58, 1110–1118. doi: 10.1109/TCE.2012.6414975
Aidukas, T., Eckert, R., Harvey, A. R., Waller, L., and Konda, P. C. (2019). Low-cost, sub-micron resolution, wide-field computational microscopy using opensource hardware. Sci. Rep. 9, 1–12. doi: 10.1038/s41598-019-43845-9
Akin, A., Cogal, O., Seyid, K., Afshari, H., Schmid, A., and Leblebici, Y. (2013). Hemispherical multiple camera system for high resolution omni-directional light field imaging. Emerg. Sel. Top Circ. Syst. IEEE J. 3, 137–144. doi: 10.1109/JETCAS.2013.2256831
Alexandrov, S. A., Hillman, T. R., Gutzler, T., and Sampson, D. D. (2006). Synthetic aperture fourier holographic optical microscopy. Phys. Rev. Lett. 97:168102. doi: 10.1103/PhysRevLett.97.168102
Ando, T., Horisaki, R., and Tanida, J. (2015). Speckle-learning-based object recognition through scattering media. Opt. Expr. 23:33902. doi: 10.1364/OE.23.033902
Andreoli, D., Volpe, G., Popoff, S., Katz, O., Grésillon, S., Gigan, S., et al. (2015). Deterministic control of broadband light through a multiply scattering medium via the multispectral transmission matrix. Sci. Rep. 5:10347. doi: 10.1038/srep10347
Antonik, P., Marsal, N., Brunner, D., and Rontani, D. (2019). Human action recognition with a large-scale brain-inspired photonic computer. Nat. Mach. Intell. 1, 530–537. doi: 10.1038/s42256-019-0110-8
Arbabi, A., and Faraon, A. (2023). Advances in optical metalenses. Nat. Photon. 17, 16–25. doi: 10.1038/s41566-022-01108-6
Barbastathis, G., Ozcan, A., and Situ, G. (2019). On the use of deep learning for computational imaging. Optica 6, 921–943. doi: 10.1364/OPTICA.6.000921
Bar-Noy, A., Cirincione, G., Govindan, R., Krishnamurthy, S., and Yener, A. (2011). “Quality-of-information aware networking for tactical military networks,” in 2011 IEEE International Conference on Pervasive Computing and Communications Workshops (PERCOM Workshops) (IEEE), 2–7. Available online at: https://xueshu.baidu.com/u/citation?type=bibandpaperid=1j4304j0ad080jh0q41d0t50qk380364 (accessed December 26, 2023).
Batarseh, M., Sukhov, S., Shen, Z., Gemar, H., Rezvani, R., Dogariu, A., et al. (2018). Passive sensing around the corner using spatial coherence. Nat. Commun. 9, 3629. doi: 10.1038/s41467-018-05985-w
Berman, D., Treibitz, T., and Avidan, S. (2016). “Non-local image dehazing,” in 2016 IEEE Conference on Computer Vision and Pattern Recognition (CVPR) (Las Vegas, NV, USA: IEEE), 1674–1682. doi: 10.1109/CVPR.2016.185
Bertolotti, J., van Putten, E. G., Blum, C., Lagendijk, A., Vos, W. L., Mosk, A. P., et al. (2012). Non-invasive imaging through opaque scattering layers. Nature 491, 232–234. doi: 10.1038/nature11578
Bogdanski, J., Bjork, G., and Karlsson, A. (2004). Quantum and classical correlated imaging. Available online at: http://arxiv.org/abs/quant-ph/0407127 (accessed June 26, 2023).
Bosq, T. W. D., Agarwal, S., Dijk, J., Gungor, A., and Leonard, K. (2018). An overview of joint activities on computational imaging and compressive sensing systems by NATO SET-232. Computat. Imag. III 10669, 54–72. doi: 10.1117/12.2307852
Brady, D. J., and Hagen, N. (2009). Multiscale lens design. Opt. Expr. 17, 10659–10674. doi: 10.1364/OE.17.010659
Cao, F., Chen, J., Ye, H., Zhao, J., and Zhou, Z. (2017). Recovering low-rank and sparse matrix based on the truncated nuclear norm. Neural Netw. 85, 10–20. doi: 10.1016/j.neunet.2016.09.005
Cao, L., Zhang, H., Brady, D. J., and Liu, S. (2020). Noise suppression for ballistic-photons based compressive in-line holographic imaging through inhomogeneous medium. Opt. Expr. 28, 10337–10349. doi: 10.1364/OE.385992
Chan, S., Warburton, R. E., Gariepy, G., Leach, J., and Faccio, D. (2017). Non-line-of-sight tracking of people at long range. Opt. Expr. 25, 10109–10117. doi: 10.1364/OE.25.010109
Changsheng, S., Yongtian, Z., Zhongwen, H., Teng, X., and Zhen, W. (2017). Astronomy optical interferometric telescope array optimizationbased on modified UV sampling method. J. Appl. Opt. 38, 532–536. doi: 10.5768/JAO201738.0401007
Chengfei, G., Jietao, L., Tengfei, W., Lei, Z., and Xiaopeng, S. (2018). Tracking moving targets behind a scattering medium via speckle correlation. Appl. Opt. 57:905. doi: 10.1364/AO.57.000905
Cogal, O., Akin, A., Seyid, K., Popovic, V., Schmid, A., Ott, B., et al. (2014). “A new omni-directional multi-camera system for high resolution surveillance,” in Mobile Multimedia/Image Processing, Security, and Applications 2014 (SPIE), 179–187. doi: 10.1117/12.2049698
Cogal, O., and Leblebici, Y. (2016). An insect eye inspired miniaturized multi-camera system for endoscopic imaging. IEEE Trans. Biomed. Circ. Syst. 11, 212–224. doi: 10.1109/TBCAS.2016.2547388
Coskun, A. F., and Ozcan, A. (2014). Computational imaging, sensing and diagnostics for global health applications. Curr. Opin. Biotechnol. 25, 8–16. doi: 10.1016/j.copbio.2013.08.008
Cotte, Y., Toy, F., Jourdain, P., Pavillon, N., Boss, D., Magistretti, P., et al. (2013). Marker-free phase nanoscopy. Nat. Photon. 7, 113–117. doi: 10.1038/nphoton.2012.329
Cua, M., Zhou, E., and Yang, C. (2017). Imaging moving targets through scattering media. Opt. Expr. 25:3935. doi: 10.1364/OE.25.003935
Daubechies, I., Defrise, M., and De Mol, C. (2004). An iterative thresholding algorithm for linear inverse problems with a sparsity constraint. Commun. Pure Appl. Mathem. 57, 1413–1457. doi: 10.1002/cpa.20042
Dong, C., Loy, C. C., He, K., and Tang, X. (2014). “Learning a deep convolutional network for image super-resolution,” in Computer Vision–ECCV 2014: 13th European Conference, Zurich, Switzerland, September 6-12, 2014, Proceedings, Part IV 13 (Cham: Springer International Publishing), 184–199. doi: 10.1007/978-3-319-10593-2_13
Dong, J., Krzakala, F., and Gigan, S. (2018). “Spectral method for multiplexed phase retrieval and application in optical imaging in complex media,” in ICASSP 2019-2019 IEEE International Conference on Acoustics, Speech and Signal Processing (ICASSP) (IEEE), 4963–4967. doi: 10.1109/ICASSP.2019.8682329
Drémeau, A., Liutkus, A., Martina, D., Katz, O., Schülke, C., Krzakala, F., et al. (2015). Reference-less measurement of the transmission matrix of a highly scattering material using a DMD and phase retrieval techniques. Opt. Expr. 23, 11898–11911. doi: 10.1364/OE.23.011898
Enders, B., Dierolf, M., Cloetens, P., Stockmar, M., Pfeiffer, F., Thibault, P., et al. (2014). Ptychography with broad-bandwidth radiation. Appl. Phys. Lett. 104:171104. doi: 10.1063/1.4874304
Fang, S., Xia, X., Huo, X., and Chen, C. (2014). Image dehazing using polarization effects of objects and airlight. Opt. Expr. 22, 19523–19537. doi: 10.1364/OE.22.019523
Fei, L., Shao-Jie, S., Ping-Li, H., Lin, Z., and Xiao-Peng, S. (2021). Clear underwater vision in non-uniform scattering field by low-rank-and-sparse-decomposition-based olarization imaging. Acta Phys. Sinica 70:385. doi: 10.7498/aps.70.20210314
Fei, L., Yazhe, W., Pingli, H., Jiawei, L., and Xiaopeng, S. (2019). Design of monocentric wide field-of-view and high-resolution computational imaging system. Acta Phys. Sin. 68:1447. doi: 10.7498/aps.68.20182229
Feld, M. S., Yang, C., Psaltis, D., and Yaqoob, Z. (2008). Optical phase conjugation for turbidity suppression in biological samples. Nat. Photon. 2, 110–115. doi: 10.1038/nphoton.2007.297
Feng, S., Kane, C., Lee, P. A., and Stone, A. D. (1988). Correlations and fluctuations of coherent wave transmission through disordered media. Phys. Rev. Lett. 61, 834–837. doi: 10.1103/PhysRevLett.61.834
Freund, I., Rosenbluh, M., and Feng, S. (1988). Memory effects in propagation of optical waves through disordered media. Phys. Rev. Lett. 61, 2328–2331. doi: 10.1103/PhysRevLett.61.2328
Fu, W., Zhao, D., Li, Z., Liu, S., Tian, C., Huang, K., et al. (2022). Ultracompact meta-imagers for arbitrary all-optical convolution. Light Sci. Appl. 11:62. doi: 10.1038/s41377-022-00752-5
Fu, Y., Zhao, Y., Liu, Z., and Dong, Z. (2015). Design of bionic compound eye optical system based on field of view stitching method. China J. Sci. Instrum. 36, 422–429.
Gariepy, G., Tonolini, F., Henderson, R., Leach, J., and Faccio, D. (2016). Detection and tracking of moving objects hidden from view. Nat. Photon. 10, 23–26. doi: 10.1038/nphoton.2015.234
Golish, D. R., Vera, E. M., Kelly, K. J., Gong, Q., Jansen, P. A., Hughes, J. M., et al. (2012). Development of a scalable image formation pipeline for multiscale gigapixel photography. Opt. Expr. 20:22048. doi: 10.1364/OE.20.022048
Gustafsson, M. G. L. (2000). Surpassing the lateral resolution limit by a factor of two using structured illumination microscopy. J. Microsc. 198, 82–87. doi: 10.1046/j.1365-2818.2000.00710.x
Han, P., Cai, Y., Liu, F., Li, X., Liang, R., Yan, M., et al. (2022). Computational polarization 3D: new solution for monocular shape recovery in natural conditions. Opt. Lasers Eng. 151:106925. doi: 10.1016/j.optlaseng.2021.106925
Han, P., Liu, F., Yang, K., Ma, J., Li, J., Shao, X., et al. (2017). Active underwater descattering and image recovery. Appl. Opt. 56:6631. doi: 10.1364/AO.56.006631
Harris, J. L. (1964). Diffraction and resolving power. J. Opt. Soc. Am. 54:931. doi: 10.1364/JOSA.54.000931
Hegerl, R., and Hoppe, W. (1972). Phase evaluation in generalized diffraction (ptychography). Available online at: https://xueshu.baidu.com/u/citation?type=bibandpaperid=1b9f8cfe7b9b3ac2bac3983574af9864 (accessed December 26, 2023).
Hidary, J. D., and Hidary, J. D. (2019). Quantum Computing: An Applied Approach. New York: Springer. doi: 10.1007/978-3-030-23922-0
Horisaki, R., Takagi, R., and Tanida, J. (2016). Learning-based imaging through scattering media. Opt. Expr. 24, 13738–13743. doi: 10.1364/OE.24.013738
Hu, H. F., Li, J. Q., and Li, X. B. (2021). Underwater polarization difference imaging with three degrees of freedom. Acta Opt. Sin. 41:0329001. doi: 10.3788/AOS202141.0329001
Hu, X., Wu, J., Suo, J., and Dai, Q. (2017). Emerging theories and technologies on computational imaging. Front. Inf. Technol. Electron. Eng. 18, 1207–1221. doi: 10.1631/FITEE.1700211
Jiasong, S., Yuzhen, Z., Qian, C., and Chao, Z. (2016). Fourier ptychographic microscopy: theory, advances, and applications. Acta Opt. Sin. 36:1011005. doi: 10.3788/AOS201636.1011005
Juyang, W., Paul, C., and Marc, H. (1992). Camera calibration with distortion models and accuracy evaluation. IEEE Trans. Pattern. Anal. Mach. Intell. 14, 965–980. doi: 10.1109/34.159901
Kadambi, A., Taamazyan, V. A., Shi, B., and Raskar, R. (2017). Depth sensing using geometrically constrained polarization normals. Int. J. Comput. Vis. 125, 34–51. doi: 10.1007/s11263-017-1025-7
Katz, O., Heidmann, P., Fink, M., and Gigan, S. (2014). Non-invasive single-shot imaging through scattering layers and around corners via speckle correlations. Nat. Photon. 8, 784–790. doi: 10.1038/nphoton.2014.189
Katz, O., Small, E., Bromberg, Y., and Silberberg, Y. (2011). Focusing and compression of ultrashort pulses through scattering media. Nat. Photon. 5, 372–377. doi: 10.1038/nphoton.2011.72
Katz, O., Small, E., and Silberberg, Y. (2012). Looking around corners and through thin turbid layers in real time with scattered incoherent light. Nat. Photon. 6, 549–553. doi: 10.1038/nphoton.2012.150
Kellman, M., Zhang, K., Markley, E., Tamir, J., Bostan, E., Lustig, M., et al. (2020). Memory-efficient learning for large-scale computational imaging. IEEE Trans. Comput. Imag. 6, 1403–1414. doi: 10.1109/TCI.2020.3025735
Kim, J., Lee, J. K., and Lee, K. M. (2016). “Accurate image super-resolution using very deep convolutional networks,” in Proceedings of the IEEE Conference on Computer Vision and Pattern Recognition, 1646–1654. doi: 10.1109/CVPR.2016.182
Kitamura, Y., Shogenji, R., Yamada, K., Miyatake, S., Miyamoto, M., Morimoto, T., et al. (2004). Reconstruction of a high-resolution image on a compound-eye image-capturing system. Appl. Opt. 43, 1719–1727. doi: 10.1364/AO.43.001719
Kumar, S. S., Dongliang, T., and Cuong, D. (2017). Single-shot multispectral imaging with a monochromatic camera. Optica 4:1209. doi: 10.1364/OPTICA.4.001209
Lai, X., Li, Q., Wu, X., Liu, G., and Pu, J. (2021). Mutual transfer learning of reconstructing images through a multimode fiber or a scattering medium. IEEE Access 9, 68387–68395. doi: 10.1109/ACCESS.2021.3077560
Law, N. M., Fors, O., Ratzloff, J., Wulfken, P., Kavanaugh, D., Sitar, D. J., et al. (2015). Evryscope science: exploring the potential of all-sky gigapixel-scale telescopes. Publ. Astron. Soc. Pac. 127, 234–249. doi: 10.1086/680521
Law, N. M., Fors, O., Wulfken, P., Ratzloff, J., and Kavanaugh, D. (2014). “The Evryscope: the first full-sky gigapixel-scale telescope,” in Ground-based and Airborne Telescopes V (SPIE), 357–365. doi: 10.1117/12.2057031
LeCun, Y., Bengio, Y., and Hinton, G. (2015). Deep learning. Nature 521, 436–444. doi: 10.1038/nature14539
Lee, D. D., Pham, P., Largman, Y., and Ng, A. (2022). “Advances in neural information processing systems 22,” in Proceedings of the 26th Annual Conference on Neural Information Processing Systems 2012 (NIPS).
Leninger, B., Edwards, J., Antoniades, J., Chester, D., Haas, D., Liu, E., et al. (2008). Autonomous real-time ground ubiquitous surveillance-imaging system (ARGUS-IS). Proc. SPIE – Int. Soc. Opt. Eng. 6981, 69810H−11. doi: 10.1117/12.784724
Li, J. Y., Feng, W. X., Liu, F., Wei, Y. Z., and Shao, X. P. (2021). Design of airborne multi-scale wide-field-of-view and high-resolution imaging system. Acta Opt. Sin. 41, 50–60. doi: 10.3788/AOS202141.0208002
Li, X., Liu, F., Han, P., Zhang, S., and Shao, X. (2021). Near-infrared monocular 3D computational polarization imaging of surfaces exhibiting nonuniform reflectance. Opt. Expr. 29, 15616–15630. doi: 10.1364/OE.423790
Li, Y., and Gu, P. (2004). Free-form surface inspection techniques state of the art review. Comput.-Aided Des. 36, 1395–1417. doi: 10.1016/j.cad.2004.02.009
Li, Y., Xue, Y., and Tian, L. (2018). Deep speckle correlation: a deep learning approach toward scalable imaging through scattering media. Optica 5, 1181–1190. doi: 10.1364/OPTICA.5.001181
Li, Z. P., Huang, X., Cao, Y., Wang, B., Li, Y. H., Jin, W., et al. (2020). Single-photon computational 3D imaging at 45 km. Photon. Res. 8, 1532–1540. doi: 10.1364/PRJ.390091
Lim, B., Son, S., Kim, H., Nah, S., and Mu Lee, K. (2017). “Enhanced deep residual networks for single image super-resolution,” in Proceedings of the IEEE Conference on Computer Vision and Pattern Recognition Workshops, 136–144. doi: 10.1109/CVPRW.2017.151
Liu, F., Cao, L., Shao, X., Han, P., and Bin, X. (2015). Polarimetric dehazing utilizing spatial frequency segregation of images. Appl. Opt. 54:8116. doi: 10.1364/AO.54.008116
Liu, F., Han, P., Wei, Y., Yang, K., Huang, S., Li, X., et al. (2018). Deeply seeing through highly turbid water by active polarization imaging. Opt. Lett. 43, 4903–4906. doi: 10.1364/OL.43.004903
Liu, Y., Zhao, H., Shen, D., Xu, J., and Yu, X. (2020). “Research on snapshot infrared computational spectral imaging technology,” in Conference on Infrared Device and Infrared Technology Available online at: https://xueshu.baidu.com/u/citation?type=bibandpaperid=1w120am0pa3n08609d6d0rh0vx586617 (accessed December 26, 2023).
Liutkus, A., Martina, D., Popoff, S., Chardon, G., Katz, O., Lerosey, G., et al. (2014). Imaging with nature: compressive imaging using a multiply scattering medium. Rep. 4:5552. doi: 10.1038/srep05552
Lukac, R., and Radha, H. (2011). Computational photography: methods and applications. J. Electron Imaging. Available online at: https://xueshu.baidu.com/u/citation?type=bibandpaperid=2f3dad1aea4b8e3aac3614987d4abc97 (accessed December 26, 2023).
Lyu, M., Wang, H., Li, G., Zheng, S., and Situ, G. (2019). Learning-based lensless imaging through optically thick scattering media. Adv. Photon. 1:10. doi: 10.1117/1.AP.1.3.036002
Mait, J. N., Euliss, G. W., and Athale, R. A. (2012). Computational imaging. IEEE. Available online at: https://xueshu.baidu.com/u/citation?type=bibandpaperid=ec226e2abc1ca0a55496cbe565da0209 (accessed December 26, 2023).
Marks, D. L., Llull, P. R., Phillips, Z., Anderson, J. G., Feller, S. D., Vera, E. M., et al. (2014). Characterization of the AWARE 10 two-gigapixel wide-field-of-view visible imager. Appl. Opt. 53, C54–C63. doi: 10.1364/AO.53.000C54
McLeod, E., and Ozcan, A. (2016). Unconventional methods of imaging: computational microscopy and compact implementations. Rep. Prog. Phys. 79:076001. doi: 10.1088/0034-4885/79/7/076001
Miyazaki, D., Kagesawa, M., and Ikeuchi, K. (2002b). “Determining shapes of transparent objects from two polarization images,” in MVA, 26–31.
Miyazaki, D., Saito, M., Sato, Y., and Ikeuchi, K. (2002a). Determining surface orientations of transparent objects based on polarization degrees in visible and infrared wavelengths. JOSA A 19, 687–694. doi: 10.1364/JOSAA.19.000687
Miyazaki, D., Shigetomi, T., Baba, M., Furukawa, R., Hiura, S., Asada, N., et al. (2016). Surface normal estimation of black specular objects from multiview polarization images. Opt. Eng. 56:041303. doi: 10.1117/1.OE.56.4.041303
Monakhova, K., Yanny, K., Aggarwal, N., and Waller, L. (2020). Spectral DiffuserCam: lensless snapshot hyperspectral imaging with a spectral filter array. Optica 7, 1298–1307. doi: 10.1364/OPTICA.397214
Morel, O., Stolz, C., Meriaudeau, F., and Gorria, P. (2006). Active lighting applied to three-dimensional reconstruction of specular metallic surfaces by polarization imaging. Appl. Opt. 45, 4062–4068. doi: 10.1364/AO.45.004062
Nakamura, T., Kittle, D. S., Youn, S. H., Feller, S. D., Tanida, J., Brady, D. J., et al. (2013). Autofocus for a multiscale gigapixel camera. Appl. Opt. 52:8146. doi: 10.1364/AO.52.008146
Osnabrugge, G., Horstmeyer, R., Papadopoulos, I. N., Judkewitz, B., and Vellekoop, I. M. (2017). Generalized optical memory effect. Optica 4:886. doi: 10.1364/OPTICA.4.000886
Pammi, V. A., Alfaro-Bittner, K., Clerc, M. G., and Barbay, S. (2019). Photonic computing with single and coupled spiking micropillar lasers. IEEE J. Sel. Top. Quantum Electron. 26, 1–7. doi: 10.1109/JSTQE.2019.2929187
Panigrahi, S., Fade, J., and Alouini, M. (2015). Adaptive polarimetric image representation for contrast optimization of a polarized beacon through fog. J. Opt. 17:252. doi: 10.1088/2040-8978/17/6/065703
Park, Y., Depeursinge, C., and Popescu, G. (2018). Quantitative phase imaging in biomedicine. Nat. Photon. 12, 578–589. doi: 10.1038/s41566-018-0253-x
Pendry, J. B. (2000). Negative refraction makes a perfect lens. Phys. Rev. Lett. 85:3966. doi: 10.1103/PhysRevLett.85.3966
Popoff, S., Lerosey, G., Fink, M., Boccara, A. C., and Gigan, S. (2010b). Image transmission through an opaque material. Nat. Commun. 1:81. doi: 10.1038/ncomms1078
Popoff, S. M., Lerosey, G., Carminati, R., Fink, M., Boccara, A. C., Gigan, S., et al. (2010a). Measuring the transmission matrix in optics: an approach to the study and control of light propagation in disordered media. Phys. Rev. Lett. 104:100601. doi: 10.1103/PhysRevLett.104.100601
Popovic, V. (2016). Real-time computational gigapixel multi-camera systems. Thesis, EPFL. doi: 10.1007/978-3-319-59057-8_4
Popovic, V., Seyid, K., Akin, A., Cogal, Ö., Afshari, H., Schmid, A., et al. (2014). Image blending in a high frame rate FPGA-based multi-camera system. J. Sign. Proc. Syst. 76, 169–184. doi: 10.1007/s11265-013-0858-8
Porat, A., Andresen, E. R., Rigneault, H., Oron, D., Gigan, S., and Katz, O. (2016). Widefield lensless imaging through a fiber bundle via speckle correlations. Opt. Expr. 24, 16835–16855. doi: 10.1364/OE.24.016835
Qaisar, S., Bilal, R. M., Iqbal, W., Naureen, M., and Lee, S. (2013). Compressive sensing: from theory to applications, a survey. J. Commun. Netw. 15, 443–456. doi: 10.1109/JCN.2013.000083
Qian, T., and Wang, Y. (2010). Micro/nano-fabrication technologies for cell biology. Med. Biol. Eng. Comput. 48, 1023–1032. doi: 10.1007/s11517-010-0632-z
Qiao, M., Liu, H., Pang, G., and Han, S. (2017). Non-invasive three-dimension control of light between turbid layers using a surface quasi-point light source for precorrection. Rep. 7:9792. doi: 10.1038/s41598-017-10450-7
Ramesh, R., and Davis, J. (2008). 5d time-light transport matrix: what can we reason about scene properties? Acta Leprol. Available online at: https://dspace.mit.edu/handle/1721.1/67888?show=full
Rani, M., Dhok, S. B., and Deshmukh, R. B. A. (2018). systematic review of compressive sensing: concepts, implementations and applications. IEEE Access 6, 4875–4894. doi: 10.1109/ACCESS.2018.2793851
Raskar, R. (2012). Recovering three-dimensional shape around a corner using ultrafast time-of-fiight imaging. Nature 3:745. doi: 10.1038/ncomms1747
Rawat, B., Mehra, N., Bist, A. S., Yusup, M., and Sanjaya, Y. P. A. (2022). Quantum computing and AI: impacts and possibilities. ADI J. Recent Innov. 3, 202–207. doi: 10.34306/ajri.v3i2.656
Robinson, M. D., and Stork, D. G. (2006). “Joint design of lens systems and digital image processing,” in International Optical Design Conference (Optica Publishing Group), WB4. doi: 10.1364/IODC.2006.WB4
Robinson, M. D., and Stork, D. G. (2007). “Joint digital-optical design of multi-frame imaging systems,” in Computational Optical Sensing and Imaging (Optica Publishing Group), CMB2. doi: 10.1364/COSI.2007.CMB2
Robinson, M. D., and Stork, D. G. (2008). Joint digital-optical design of superresolution multiframe imaging systems. Appl. Opt. 47, 11–20. doi: 10.1364/AO.47.000B11
Ruan, H., Brake, J., Robinson, J. E., Liu, Y., Jang, M., Xiao, C., et al. (2017). Deep tissue optical focusing and optogenetic modulation with time-reversed ultrasonically encoded light. Sci. Adv. 3:eaao5520. doi: 10.1126/sciadv.aao5520
Rust, M. J., Bates, M., and Zhuang, X. (2006). Sub-diffraction-limit imaging by stochastic optical reconstruction microscopy (STORM). Nat. Methods 3, 793–796. doi: 10.1038/nmeth929
Sabelhaus, P. A. (2004). An Overview of the James Webb Space Telescope %28JWST%29 Project. Available online at: https://xueshu.baidu.com/u/citation?type=bibandpaperid=19d39339a8725c71611fc5e00befc675 (accessed December 26, 2023).
Saunders, C., Murray-Bruce, J., and Goyal, V. K. (2019). Computational periscopy with an ordinary digital camera. Nature 565:472. doi: 10.1038/s41586-018-0868-6
Schechner, Y. Y., and Karpel, N. (2006). Recovery of underwater visibility and structure by polarization analysis. IEEE J. Ocean Eng. 30, 570–587. doi: 10.1109/JOE.2005.850871
Schechner, Y. Y., Narasimhan, S. G., and Nayar, S. K. (2003). Polarization-based vision through haze. Appl. Opt. 42, 511–525. doi: 10.1364/AO.42.000511
Schmidhuber, J. (2015). Deep learning in neural networks: an overview. Neural Netw. 61, 85–117. doi: 10.1016/j.neunet.2014.09.003
Shao, X. P., Liu, F., Li, W., Yang, L., Yang, S., and Liu, J. (2020). Latest progress in computational imaging technology and application. Laser Optoelectr. Progr. 57:020001. doi: 10.3788/LOP57.020001
Shen, Y., Liu, Y., Ma, C., and Wang, L. V. (2016). Focusing light through biological tissue and tissue-mimicking phantoms up to 9.6cm in thickness with digital optical phase conjugation. J. Biomed. Opt. 21:85001. doi: 10.1117/1.JBO.21.8.085001
Sinha, A., Lee, J., Li, S., and Barbastathis, G. (2017). Lensless computational imaging through deep learning. Optica 4, 1117–1125. doi: 10.1364/OPTICA.4.001117
Sprague, R., Zhang, A., Hendricks, L., O'Brien, T., and Rutherford, T. (2012). Novel HMD concepts from the DARPA SCENICC program. Proc. Spie. 8383:1. doi: 10.1117/12.922475
Stasio, N., Conkey, D. B., Moser, C., and Psaltis, D. (2015). Light control in a multicore fiber using the memory effect. Opt. Expr. 23:30532. doi: 10.1364/OE.23.030532
Stork, D. G., and Robinson, M. D. (2008). Theoretical foundations for joint digital-optical analysis of electro-optical imaging systems. Appl. Opt. 47:B64. doi: 10.1364/AO.47.000B64
Suo, J., Zhang, W., Gong, J., Yuan, X., Brady, D. J., Dai, Q., et al. (2021). Computational Imaging and Artificial Intelligence: The Next Revolution of Mobile Vision. Available online at: http://arxiv.org/abs/2109.08880 (accessed June 27, 2023).
Tan, C. L., and Mohseni, H. (2018). Emerging technologies for high performance infrared detectors. Nanophotonics 7, 169–197. doi: 10.1515/nanoph-2017-0061
Tian, Z., Zhao, M., Yang, D., Wang, S., and Pan, A. (2023). Optical remote imaging via Fourier ptychography. Photon. Res. 11:2072. doi: 10.1364/PRJ.493938
Tyo, J. S. (2000). Enhancement of the point-spread function for imaging in scattering media by use of polarization-difference imaging. JOSA A 17, 1–10. doi: 10.1364/JOSAA.17.000001
Tyo, J. S., Rowe, M. P., Pugh, E. N., and Engheta, N. (1996). Target detection in optically scattering media by polarization-difference imaging. Appl. Opt. 35, 1855–1870. doi: 10.1364/AO.35.001855
Vellekoop, I. M. (2010). “Focusing light through living tissue,” in Optical Coherence Tomography and Coherence Domain Optical Methods in Biomedicine XIV (SPIE), 406–415. Available online at: https://xueshu.baidu.com/u/citation?type=bibandpaperid=5b655643a3c68d28adc47dbb8b905a3c (accessed December 26, 2023).
Vellekoop, I. M., Lagendijk, A., and Mosk, A. P. (2010). Exploiting disorder for perfect focusing. Nat. Photon. 4, 320–322. doi: 10.1038/nphoton.2010.3
Vellekoop, I. M., and Mosk, A. P. (2007). Focusing coherent light through opaque strongly scattering media. Opt. Lett. 32:2309. doi: 10.1364/OL.32.002309
Wang, B., Zhang, Z. B., Zhong, S. P., Zheng, Z. Q., Xu, P., Zhang, H., et al. (2020). Recent progress in high-performance photo-detectors enabled by the pulsed laser deposition technology. J. Mater. Chem. C 8, 4988–5014. doi: 10.1039/C9TC07098B
Wang, C., Hu, M., Takashima, Y., Schulz, T. J., and Brady, D. J. (2022). Snapshot ptychography on array cameras. Opt. Expr. 30:2585. doi: 10.1364/OE.447499
Wickens, C. D., and Carswell, C. M. (2021). Information processing. Handb. Hum. Factors Ergon. 5, 114–158. doi: 10.1002/9781119636113.ch5
Wu, C., Liu, J., Huang, X., Li, Z. P., and Pan, J. W. (2021). Non-line-of-sight imaging over 1.43 km. Proc. Natl. Acad. Sci. 118:e2024468118. doi: 10.1073/pnas.2024468118
Wu, J., Xiong, B., Lin, X., He, J., Suo, J., and Dai, Q. (2016). Snapshot hyperspectral volumetric microscopy. Sci. Rep. 6:24624. doi: 10.1038/srep24624
Xiang, M., Pan, A., Zhao, Y., Fan, X., Zhao, H., Li, C., et al. (2021). Coherent synthetic aperture imaging for visible remote sensing via reflective Fourier ptychography. Opt. Soc. Am. 46, 29–32. doi: 10.1364/OL.409258
Xinquan, W. (2007). Image reconstruction for the computed-tomography imaging interferometer. Acta Opt. Sin. 27, 1600–1604.
Ye, J., Chen, L., Li, X., Yuan, Q., and Gao, Z. (2017). Review of optical freeform surface representation technique and its application. Opt. Eng. 56, 110901–110901. doi: 10.1117/1.OE.56.11.110901
Ying, Z., Feng, C., Zhao, Z., Dhar, S., Dalir, H., Gu, J., et al. (2020). Electronic-photonic arithmetic logic unit for high-speed computing. Nat. Commun. 11:2154. doi: 10.1038/s41467-020-16057-3
Youn, S. H., Son, H. S., Marks, D. L., Shaw, J. M., McLaughlin, P. O., Feller, S. D., et al. (2014). Optical performance test and validation of microcameras in multiscale, gigapixel imagers. Opt. Expr. 22, 3712–3723. doi: 10.1364/OE.22.003712
Yu, F., Chen, J., Huang, L., Zhao, Z., Wang, J., Jin, R., et al. (2022). Photonic slide rule with metasurfaces. Light Sci. Appl. 11:77. doi: 10.1038/s41377-022-00765-0
Zhang, J., Chen, Q., Li, J., Sun, J., and Zuo, C. (2018). Lensfree dynamic super-resolved phase imaging based on active micro-scanning. Opt. Lett. 43:3714. doi: 10.1364/OL.43.003714
Zhang, K., Jung, Y. H., Mikael, S., Seo, J. H., Kim, M., Mi, H., et al. (2017). Origami silicon optoelectronics for hemispherical electronic eye systems. Nat. Commun. 8:1782. doi: 10.1038/s41467-017-01926-1
Zhang, W., Liang, J., Ju, H., Ren, L., Qu, E., and Wu, Z. (2016). A robust haze-removal scheme in polarimetric dehazing imaging based on automatic identification of sky region. Opt. Laser Technol. 86, 145–151. doi: 10.1016/j.optlastec.2016.07.015
Zhao, F., Shen, Z., Wang, D., Xu, B., Chen, X., Yang, Y., et al. (2021). Synthetic aperture metalens. Photon. Res. 9:2388. doi: 10.1364/PRJ.440185
Zheng, G., Horstmeyer, R., and Yang, C. (2013). Wide-field, high-resolution Fourier ptychographic microscopy. Nat. Photon. 7, 739–745. doi: 10.1038/nphoton.2013.187
Zhou, Z., Wu, Z., and Tan, P. (2013). Multi-view photometric stereo with spatially varying isotropic materials. Digit Signal Proc. 21, 391–403. doi: 10.1109/CVPR.2013.195
Keywords: computational imaging interpretation, imaging model, computational imaging system, optical system design, information transfer
Citation: Xiang M, Liu F, Liu J, Dong X, Liu Q and Shao X (2024) Computational optical imaging: challenges, opportunities, new trends, and emerging applications. Front. Imaging. 3:1336829. doi: 10.3389/fimag.2024.1336829
Received: 11 November 2023; Accepted: 24 January 2024;
Published: 14 February 2024.
Edited by:
Pasquale Imperatore, National Research Council (CNR), ItalyReviewed by:
Pasquale Memmolo, National Research Council (CNR), ItalyCopyright © 2024 Xiang, Liu, Liu, Dong, Liu and Shao. This is an open-access article distributed under the terms of the Creative Commons Attribution License (CC BY). The use, distribution or reproduction in other forums is permitted, provided the original author(s) and the copyright owner(s) are credited and that the original publication in this journal is cited, in accordance with accepted academic practice. No use, distribution or reproduction is permitted which does not comply with these terms.
*Correspondence: Xiaopeng Shao, eHBzaGFvQHhpZGlhbi5lZHUuY24=
Disclaimer: All claims expressed in this article are solely those of the authors and do not necessarily represent those of their affiliated organizations, or those of the publisher, the editors and the reviewers. Any product that may be evaluated in this article or claim that may be made by its manufacturer is not guaranteed or endorsed by the publisher.
Research integrity at Frontiers
Learn more about the work of our research integrity team to safeguard the quality of each article we publish.