- 1Department of Physics and Astronomy, University of Pittsburgh, Pittsburgh, PA, United States
- 2Department of Physics, West Virginia University, Morgantown, WV, United States
With advances in digital technology, research-validated self-paced learning tools can play an increasingly important role in helping students with diverse backgrounds become good problem solvers and independent learners. Thus, it is important to ensure that all students engage with self-paced learning tools effectively in order to learn the content deeply, develop good problem-solving skills, and transfer their learning from one context to another. Here, we first provide an overview of a holistic framework for engaging students with self-paced learning tools so that they can transfer their learning to solve novel problems. The framework not only takes into account the features of the self-paced learning tools but also how those tools are implemented, the extent to which the tools take into account student characteristics, and whether factors related to students’ social environments are accounted for appropriately in the implementation of those tools. We then describe an investigation in which we interpret the findings using the framework. In this study, a research-validated self-paced physics tutorial was implemented in both controlled one-on-one interviews and in large enrollment, introductory calculus-based physics courses as a self-paced learning tool. We find that students who used the tutorial in a controlled one-on-one interview situation performed significantly better on transfer problems than those who used it as a self-paced learning tool in the large-scale implementation. The findings suggest that critically examining and taking into account how the self-paced tools are implemented and incentivized, student characteristics including their self-regulation and time-management skills, and social and environmental factors can greatly impact the extent and manner in which students engage with these learning tools. Getting buy in from students about the value of these tools and providing appropriate support while implementing them is critical for ensuring that students, who otherwise may be constrained by motivational, social, and environmental factors, engage effectively with the tools in order to learn deeply and transfer their learning.
Introduction
Background: Self-Paced Learning Tools
Research-validated self-paced learning tools provide a valuable opportunity for personalized learning and can supplement learning even in brick and mortar classrooms (Kulik and Kulik, 1991; Azevedo et al., 2004, 2005; Azevedo, 2005; Allen and Seaman, 2013; Breslow et al., 2013; Colvin et al., 2014; Seaton et al., 2014; Alraimi et al., 2015; Bower et al., 2015; Margaryan et al., 2015). Adaptive self-paced learning tools can allow students with diverse prior preparations to obtain feedback and support based upon their needs, and students can work through them at their own pace and receive help as needed (Reif, 1987; Lenaerts et al., 2002; Chen et al., 2010; Chandra and Watters, 2012; Debowska et al., 2012; Chen and Gladding, 2014). Appropriate use of research-validated self-paced adaptive learning tools can be particularly beneficial for under-prepared students and provide a variety of students an opportunity to learn. These tools can play a central role in scaffolding student learning, helping them gain a deep understanding of the content (Yalcinalp et al., 1995; McDermott, 1996; Korkmaz and Harwood, 2004; Singh, 2008a; Kohnle et al., 2010; Marshman and Singh, 2015; Sayer et al., 2017), develop their problem-solving, reasoning, and meta-cognitive skills (Reif and Scott, 1999; Singh, 2004; Demetriadis et al., 2008; Singh and Haileselassie, 2010), and facilitate transfer of learning from one context to another (Chi et al., 1994).
However, even in a brick-and-mortar class, ensuring that students engage effectively with available self-paced learning tools to learn is challenging, especially among students who are struggling with the course material and are in need of out of class help to learn. For example, students may lack the motivation, self-regulation, and time-management skills necessary for effective engagement with self-paced learning tools (Bandura, 1997; Zimmerman and Schunk, 2001; Wigfield et al., 2008; Moos and Azevedo, 2008, 2009; Marshman et al., 2017), and their environments may not be conducive to effective engagement with these tools without explicit additional support. Thus, without a critical focus on effective implementation of these tools and sufficient help and incentives to ensure effective engagement with the tools, students may not follow the guidelines for using these tools even if they are research-validated and continuously available via the internet. The ineffective use of these self-paced learning tools can significantly reduce their efficacy and impede transfer of learning to new situations.
It is therefore important to investigate how students engage with self-paced learning tools (e.g., in a controlled environment where their interactions with the tool are monitored vs. when they are not monitored) and contemplate strategies that can provide additional support and incentives to students who otherwise may not engage with them as intended. We have been investigating how students engage with optional, web-based tutorials outside of class in introductory physics courses when told that engaging with them would help them with their homework and quizzes (DeVore et al., 2017). These investigations suggest that students often do not engage with the research-validated self-paced tutorials in a manner in which the researchers intended. In particular, many students skimmed through the tutorials or tried to memorize procedures from them without developing a functional understanding of the concepts and a large fraction of students did not engage with them at all. The findings of these investigations motivated us to develop a theoretical framework that holistically takes into account the characteristics of the students and the self-paced learning tools, as well as the environments in which the tools are implemented. The framework can be used as a guide in the development and implementation of self-paced learning tools that encourage all students to engage with them effectively in order to learn.
Goal
The goal of this paper is to first provide an overview of the theoretical framework that focuses on the factors that can impact effective student engagement with the self-paced learning tools and the extent to which students learn from those tools and are able to transfer their learning to new contexts. Then, we report on an investigation in an introductory physics course involving a self-paced learning tutorial on angular momentum and how the findings were interpreted using the framework. In particular, we discuss how introductory physics students who were asked to engage with a research-validated tutorial on the conservation of angular momentum as a self-paced learning tool did not benefit as much as those who used the same tool in a controlled environment and they especially struggled to transfer their learning to a new situation. Then, we summarize the findings of the investigation vis-à-vis the framework that shed light on the aspects of the implementation of research-validated self-paced learning tools that should be critically considered in order to improve their effectiveness.
Overview of the Strategies for Engaged Learning Framework (SELF)
The SELF (see Figure 1), is a holistic framework which suggests that, for effective learning from self-paced learning tools, the instructional design and learning tools, their implementation, student characteristics, and social and environmental factors collectively play a role and determine how effectively a majority of students will engage with them (DeVore et al., 2017). The framework consists of four quadrants and posits that all of them must be considered holistically to help students learn effectively. The horizontal dimension involves the characteristics of learning tools and students, both of which should be taken into account when developing and implementing learning tools effectively. The vertical dimension involves internal and external characteristics of the learning tools and the learners. This dimension focuses on how the characteristics of the learning tools and students as well as the environments in which the tools are implemented are important to consider ensuring that students will engage with them effectively and learn from them.
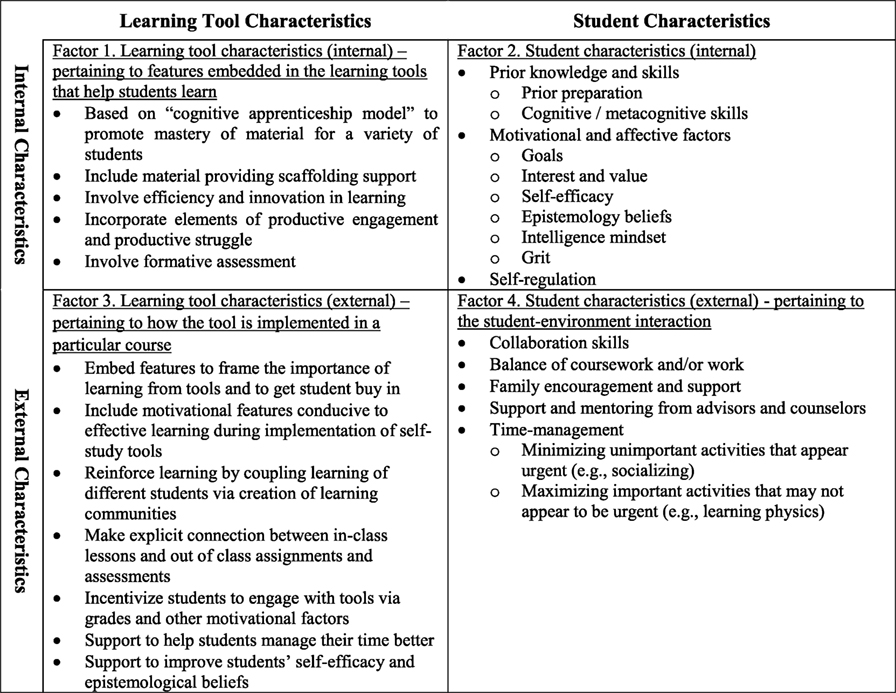
Figure 1. Strategies for Engaged Learning Framework (SELF). This figure was first presented in DeVore et al. (2017).
The internal characteristics of the tool pertain to the tool itself (e.g., whether it includes formative assessment). The external characteristics of the tool pertain to how the tool is implemented and incentivized (e.g., whether the tool is framed appropriately to ensure student buy in). The internal characteristics of the students pertain to, e.g., students’ prior preparation, motivation, goals, and epistemological beliefs about learning in a particular discipline that can impact their level of engagement with the tools. The external characteristics of the students pertain to social and environmental factors such as support from mentors and balance of coursework. These four factors should be taken into account holistically to develop and implement self-paced learning tools effectively in order to help students learn content, develop problem-solving skills, and transfer their learning to new contexts.
Factors 1 and 2: Internal Characteristics of Learning Tools and Students
Factors 1 and 2 of the framework (the internal characteristics of the learning tool and students) are informed by several cognitive theories that point to the importance of knowing students’ prior knowledge and difficulties in order to develop effective instructional tools. For example, Hammer’s “resource” model suggests that students’ prior knowledge and learning difficulties can be used as a resource to help students learn better (Hammer, 1994a,b). Similarly, the Piagetian model of learning emphasizes an “optimal mismatch” between what the student knows and is able to do and the instructional design (Piaget, 1978). In particular, this model focuses on the importance of knowing students’ prior knowledge, skills, and difficulties and using this knowledge to design instruction to help them assimilate and accommodate new ideas and build a good knowledge structure. Vygotsky’s “zone of proximal development” (ZPD) refers to the zone defined by the difference between what a student can do on his/her own and what a student can do with the help of an instructor who is familiar with his/her prior knowledge and skills (Posner et al., 1982). Scaffolding is a crucial component of this learning model and can be used to stretch students’ learning beyond their current knowledge by carefully crafted instruction. Bransford and Schwartz’s “preparation for future learning” (PFL) framework suggests that instructional design should include elements of both innovation and efficiency to help students transfer their learning from one context to another (Schwartz et al., 2005). Transfer of learning involves applying knowledge flexibly to new situations other than those in which the knowledge was initially learned and is a hallmark of expertise (Gick and Holyoak, 1983, 1987; Singh, 2008c,d; Nokes-Malach and Mestre, 2013). One interpretation of the PFL model posits that efficiency and innovation can be considered to be two orthogonal dimensions in the instructional design. If instruction only focuses on efficiently transmitting information, cognitive engagement will be diminished and learning will not be effective. On the other hand, if the instruction is solely focused on innovation, students will struggle to connect what they are learning with their prior knowledge and learning and transfer will be inhibited. An appropriate balance of efficiency and innovation builds on students’ prior knowledge and difficulties appropriately and helps them decontextualize their learning (i.e., apply their learning in many different contexts), which can facilitate transfer of learning. All of these cognitive theories (“resources,” “optimal mismatch,” “ZPD,” and “PFL” learning models) point to the fact that one must determine the prior knowledge, motivation, and self-regulation of students (Kulik, 1994; Mangels et al., 2006; Hsieh et al., 2007; Sungur, 2007; Fryer and Elliott, 2008; Hulleman et al., 2008; Greene et al., 2010; Song et al., 2016) in order to design effective instruction commensurate with students’ current knowledge and skills.
Moreover, instructional design that conforms to the field tested cognitive apprenticeship framework (Collins et al., 1989) can help students learn effectively (see Factor 1). The cognitive apprenticeship model involves three major components: modeling, coaching and scaffolding, and weaning. In this approach, “modeling” means that the instructor demonstrates and exemplifies the skills that students should learn. “Coaching and scaffolding” refer to providing students suitable practice, guidance, and feedback so that they learn the skills necessary for good performance. “Weaning” means gradually fading the support and feedback with a focus on helping students develop self-reliance. Much research in physics education has focused on the cognitive factors in developing effective pedagogical tools and assessment. For example, in physics and other related disciplines, tutorials (Chang, 2001; Singh et al., 2006; Wagner et al., 2006; Singh, 2008b; Zhu and Singh, 2011, 2012a,b, 2013; Brown and Singh, 2015; DeVore and Singh, 2015; Sayer et al., 2015; Singh and Marshman, 2015; DeVore et al., 2016a,b; Marshman and Singh, 2016, 2017a,b,c), peer instruction (clicker questions with peer discussion) (Mazur, 1997), collaborative group problem solving with context-rich physics problems (Heller and Hollabaugh, 1992), POGIL (process-oriented guided-inquiry learning) activities (Farrell et al., 1999), etc. have been found effective in helping students learn (Shaffer and McDermott, 1992; Singh, 2009; Yerushalmi et al., 2012a,b; Stewart et al., 2016; Wood et al., 2016).
Factors 3 and 4: External Characteristics of Learning Tools and Students
We note that instructional tools and student characteristics (Factors 1 and 2) do not exist in a “vacuum.” One must also take into account the environment, which includes the setting in which the learning tools are implemented and students’ social environments. Factors 3 and 4 of the SELF framework focus on how learning tools are implemented in a particular course and students’ environments, respectively. In particular, Factor 4 (the student-environment interaction) can either encourage or discourage effective engagement with learning tools. For example, having supportive parents, teachers, and mentors can be beneficial in fostering students’ motivation and engagement with learning tools (Grolnick et al., 2002). Students’ time management skills have also been shown to correlate with performance in college (Britton and Tesser, 1991). Students’ self-regulation can also either hinder or enhance engagement with self-paced learning tools. In addition, Factor 3 (how learning tools are implemented and incentivized in a course) can affect the extent and manner in which students engage with the learning tools. For example, “framing” instruction to achieve student “buy-in” (e.g., why students should deliberately engage with self-paced learning tools) can help in motivating students to engage with them. Studies have shown that providing to students rationales for why a particular learning activity is worth the effort and why it is useful for them both in the short and long run can help them engage with it more constructively (Deci et al., 1994; Jang, 2008). Motivational researchers also posit that providing stimulating and interesting tasks that are personally meaningful, interesting, relevant, and/or useful to students can increase their interest and value in a subject, increasing their motivation and engagement in learning (Pintrich, 2003). For example, physics education researchers have developed “context-rich” physics problems, i.e., problems that involve “real-world” applications of physics principles and are complex and ill-defined (Heller and Hollabaugh, 1992). These types of problems can often increase students’ interest and value associated with physics. Furthermore, instruction that fosters a community of learners can also encourage productive engagement in learning. Within this community of learners, students are encouraged to construct their own knowledge while being held accountable to others, which can, in part, encourage them to engage deeply with the content (Brown, 1997; Engle and Conant, 2002). These types of peer collaborations can be exploited and incentivized when students are learning by engaging with self-paced learning tools.
In sum, the four factors of the SELF Framework can be considered holistically when designing instruction to help students engage effectively with learning tools, including in a self-paced learning environment. We note that each of the factors can interact with other factors. For example, the way in which instructional tools are implemented (Factor 3) is impacted by the characteristics of the learning tool (Factor 1), the characteristics of the students (Factor 2), and the way in which students interact with their social environments (Factor 4). Furthermore, the student characteristics in Factor 2 can inform the learning tool characteristics (Factor 1), the implementation of learning tools (Factor 3), as well as how the student interacts with the environment (Factor 4). Below, we describe a study in which we investigated how students engaged with a self-paced (web-based) tutorial in an introductory physics course and describe the findings of the study in light of the SELF framework.
Research Objectives and Questions
In this study, we investigated how students engage with a research-validated, self-paced introductory physics tutorial on angular momentum conservation in (1) one-on-one interview settings and (2) a large-scale implementation as a self-paced learning tool in a calculus-based introductory physics course at a large research university in the US and interpreted the findings using the framework described in the preceding section. The self-paced tool used in this study was a research-validated web-based tutorial that focused on quantitative problem solving involving angular momentum conservation principle and was designed to aid students via a guided inquiry-based approach to learning. In the interview setting, the researchers assured student engagement by requiring them to work through the tutorial in a deliberate manner as prescribed. Students in the large-scale implementation of the tutorial were encouraged to use the self-paced tutorial as preparation for homework and quizzes and had the option to use it as a self-study tool outside of class, so that the researchers did not have control over how the students engaged with it. Student learning was evaluated by their performance on a pre-quiz problem that was identical to the tutorial problem (that either provided scaffolding support or did not—the scaffolding support provided will be detailed later) and a transfer quiz problem (called paired problem) that was comparable to the tutorial problem in that it was an angular momentum conservation problem for introductory physics but was posed in a different context. We compared the performance of students in the large-scale implementation and one-on-one interview settings on the paired quiz problem that required transfer of learning. In particular, the researchers focused on four research questions:
1. In the large scale-implementation of the self-paced tutorial, how does the performance of students who worked through the tutorial compare to the performance of students who did not work through the tutorial on a “pre-quiz” problem that is identical to the tutorial problem?
2. In the large scale-implementation of the self-paced tutorial, how does the performance of students who only worked through an “unscaffolded pre-quiz” problem compare with the performance of the students who worked through the tutorial on a “paired” quiz problem (i.e., is there any difference in the performance of students who worked on the tutorial vs. those who only worked through an “unscaffolded pre-quiz” problem on a follow up transfer problem)?
3. In the large-scale implementation of the self-paced tutorial, how does the performance of students who only worked through a “scaffolded pre-quiz” problem compare with the performance of the students who worked through the tutorial on a “paired” quiz problem (i.e., is there any difference in the performance of students who worked on the tutorial vs. those who only worked through a scaffolded “pre-quiz” problem on a follow up transfer problem)?
4. How does the performance of students who worked through the tutorial in a monitored, one-on-one interview setting compare to the performance of students who worked through the tutorial as a self-paced learning tool in the large-scale implementation on a “paired quiz” problem that involved transfer of learning?
We discuss the findings of these research questions vis-à-vis the holistic framework. The answers to these research questions and their interpretation using the holistic framework can shed light on the characteristics and implementation of the self-paced learning tutorial that resulted in effective or ineffective student engagement and transfer of learning.
Methodology
Overview
In this investigation involving introductory student engagement with a self-paced tutorial on angular momentum conservation, students were asked to work through a research-validated tutorial in a one-on-one interview situation in which the researchers monitored them and required that they work through the tutorial deliberately while thinking aloud. The same tutorial was also implemented in large, introductory calculus-based physics courses in which students were given the option to use it as a self-paced learning tool outside of class in order to prepare for their homework and quiz on the same content. The tutorial focused on a quantitative problem involving angular momentum conservation and was designed to aid students via a guided approach to learning. Student learning was evaluated by their performance on in-class “scaffolded or unscaffolded pre-quiz” problems. The “scaffolded pre-quiz” consisted of the same problem as in the tutorial and broke the problem down into sub-problems in a multiple-choice format. The “unscaffolded pre-quiz” consisted of an open-ended problem identical to the tutorial problem and did not break the problem into sub-problems. In addition, students were assessed on their ability to transfer their learning from the tutorial problem to a “paired” quiz problem, which was similar to the tutorial problem in the underlying physics principles but had different “surface” features (the paired quiz problem was given immediately after the pre-quiz problem).
This study was carried out in accordance with the recommendations of the Human Research Protection Office. The research study and protocols used in the study were reviewed and approved by the University of Pittsburgh Institutional Review Board committee. All interviewed subjects gave written informed consent.
Learning Tools and Assessments Used
The details of the development of the web-based tutorial were reported in a prior study (DeVore et al., 2017). It was guided by the cognitive apprenticeship learning framework (Collins et al., 1989). In this approach, “modeling” implies that the instructor demonstrates and exemplifies the skills that students should learn (e.g., how to solve physics problems systematically). “Coaching” involves providing students opportunities for practice and guidance so that they are actively engaged in learning the skills necessary for good performance. “Weaning” consists of reducing the support and feedback gradually so as to help students develop self-reliance. The web-based tutorial includes modeling via breaking the tutorial problem down into sub-problems and including a systematic approach to problem solving. It also involves coaching by providing immediate feedback and support based on students’ difficulties. The coaching and scaffolding are adaptive in that the help and guidance provided to students after they answer each multiple-choice sub-problem are tailored to the student’s specific difficulty. The adaptive web-based tutorial also involves weaning by gradually providing less scaffolding as student understanding improves and they become more confident in solving the problem on their own. In addition, the tutorial also includes reflection sub-problems that require students to transfer their learning to different contexts and develop self-reliance.
As described in DeVore et al. (2017), similar to other self-paced introductory physics tutorials, the angular momentum conservation tutorial starts with an overarching problem, which is quantitative in nature. Figure 2 shows the overarching problem for this tutorial. Before working through the tutorial, students are asked to attempt the problem to the best of their ability. The tutorial then divides this overarching problem into a series of sub-problems, which take the form of research-guided, conceptual, multiple-choice questions. These sub-problems help students learn effective approaches for successfully solving a physics problem, e.g., analyzing the problem conceptually, planning and implementing the solution, and reflecting on the final answer and the entire problem-solving process. The alternative choices in these multiple-choice questions bring out common difficulties students have with the concepts. Incorrect responses direct students to appropriate help sessions in which students are provided suitable feedback and conceptual explanations with diagrams and/or appropriate equations to learn relevant physics concepts. The correct responses to the multiple-choice questions advance students to a brief statement affirming their selection followed by the next sub-problem.
Figure 3 shows an example of a sub-problem in the conservation of angular momentum web-based tutorial and the adaptive feedback provided to students. The top image in Figure 3 shows the sub-problem in which students are provided an opportunity to determine the magnitude of the initial angular momentum of a particular system. If the students select answer option A (which is incorrect), the adaptive web-based tutorial provides feedback that helps students think about the angular momentum associated with the bullet (middle image in Figure 3). If the student selects answer option C (which is correct), the adaptive feedback confirms that the students’ answer is correct and gives a reason for why it is correct (bottom image in Figure 3).
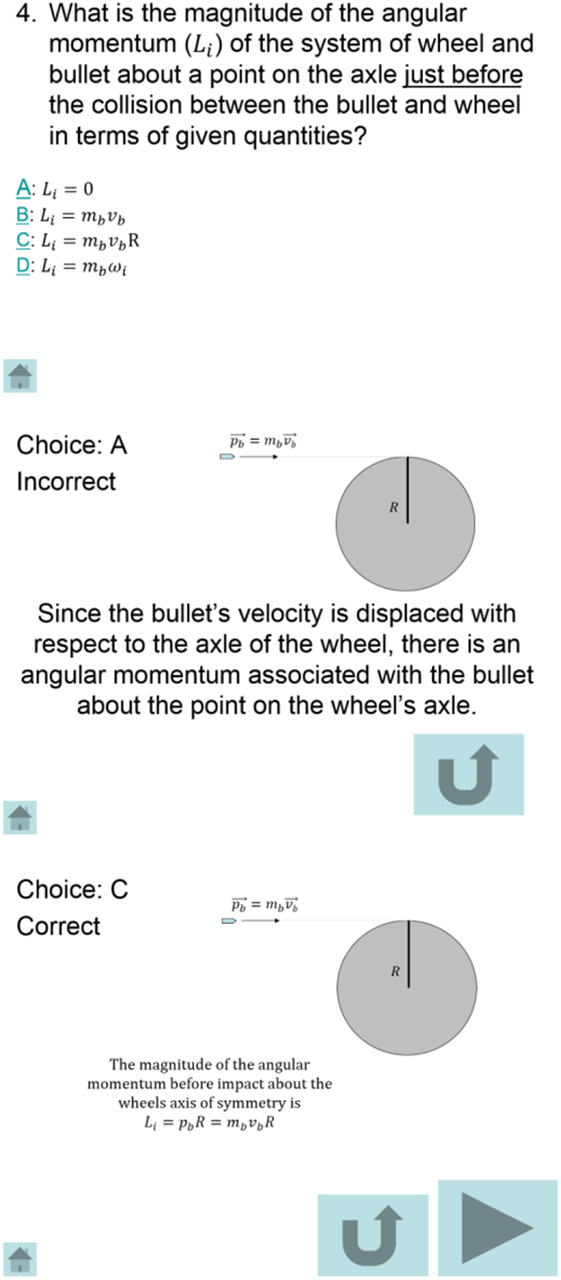
Figure 3. Example of a sub-problem from the tutorial focusing on conservation of angular momentum. Appropriate adaptive support is provided, depending on whether the student selects a certain incorrect or a correct response.
After students work on other sub-problems, they answer several reflection sub-problems. These reflection sub-problems focus on helping students reflect upon what they have learned and apply the concepts learned in different contexts (to help them decontextualize their learning and promote transfer). If students have difficulty answering the reflection sub-problems, the tutorial again provides adaptive feedback that caters to student difficulties.
The development of the tutorials, of which the tutorial on angular momentum conservation described here is a subset, went through a cyclic, iterative process detailed elsewhere (DeVore et al., 2017). For the angular momentum conservation tutorial problem, three graduate student researchers and one professor (all physics education researchers) performed a cognitive task analysis (Wieman, 2015) to decompose it into a series of sub-problems dealing with different stages of problem solving. Each sub-problem was posed as a multiple-choice question. The incorrect options for each multiple-choice question included common difficulties that were discovered by having introductory physics students solve similar problems in an open-ended format in think-aloud interviews. Explanations for each multiple choice option were written and refined based on one-on-one student interviews to aid students in repairing and extending their knowledge structure when they select an incorrect option. Using this approach, the initial draft of the web-based tutorial was created. The initial draft of the tutorial was revised many times based on interviews with introductory physics students and feedback from graduate students and several professors who worked through it to ensure that they agreed with the wording of the sub-problems and progression of the tutorial. During this revision process, the fine-tuned version of the tutorial was implemented in one-on-one think aloud (Ericsson and Simon, 1993; Chi, 1994) interviews with introductory physics students and were shown to improve student performance on the paired problem that was developed in parallel with the tutorial to assess transfer of learning to a new context (see Box 1 for the paired problem for the tutorial focusing on conservation of angular momentum discussed here).
Box 1 Paired problem for the conservation of angular momentum tutorial.
Suppose that a merry-go-round, which can be approximated as a disk, has no one on it, but it is rotating about a central vertical axis at 0.2 revolutions per second. If a 100kg man quickly sits down on the edge of it, what will be its new speed? (A disk of mass m and radius R has a moment of inertia I = (1/2)mR2, mass of merry-go-round = 200kg, radius of merry-go-round = 6m).
The paired quiz problem associated with the tutorial requires the same underlying physics concepts to solve it but is posed in a different context, i.e., it focuses on assessing transfer of learning (Gick and Holyoak, 1983, 1987; Singh, 2002, 2008c,d; Nokes-Malach and Mestre, 2013) from the tutorial problem to a new context (application of the conservation of angular momentum principle is required in order to solve the paired problem-see Box 1). The paired problem assesses whether students have learned to de-contextualize the problem solving approach and concepts learned via the tutorial. The paired quiz problem is an open-ended problem that is not broken up into sub-problems. This type of a problem can play an important role in the weaning part of the learning model and can assess whether students have developed self-reliance and are able to solve other problems based upon the same underlying concepts as the tutorial problem without any guidance or support.
After students had the opportunity to use the tutorial as a self-paced learning tool as a part of the introductory physics class, a pre-quiz problem was administered immediately followed by a paired quiz problem. While the paired quiz problem was the same for all students, those in some recitation classes were randomly administered the scaffolded version of the pre-quiz problem while those in the other recitation classes were administered unscaffolded (US) version of the pre-quiz problem. The scaffolded pre-quiz consists of multiple-choice questions, structured in the same way as the associated tutorial. In other words, the multiple-choice questions that students answer as part of the scaffolded pre-quiz involve the same questions as the tutorial sub-problems (in the same order as in the tutorial, but students are not provided feedback on whether their choices are correct, unlike the immediate feedback that is available for the sub-problems in the tutorial). Thus, the difference between the tutorial and the scaffolded pre-quiz is that the tutorial provides feedback to students after they choose an answer. On the other hand, the scaffolded pre-quiz offers no such feedback or reinforcement when an answer is selected for each multiple-choice question. The US pre-quiz is identical to the tutorial problem except that it is open-ended—students are provided no additional scaffolding (the problem is not broken into sub-problems).
Student Demographics and Implementation Approach
Below, we describe the student demographics and methodology for the implementation of the angular momentum tutorial in one-on-one implementation to student volunteers and as a self-paced learning tool in a calculus-based introductory physics course (taken primarily by freshman undergraduate students interested in pursuing engineering or physical science majors) at the University of Pittsburgh, which is a large, typical state-affiliated university in the US.
We first determined whether the tutorial was effective in one-on-one interview settings before implementing and assessing its impact in large introductory physics course as a self-paced learning tool. Twenty 2–3 h long, one-on-one, think-aloud interviews were conducted with students who were enrolled in either an algebra or calculus-based introductory physics course. Approximately half of the students were enrolled in an algebra-based physics course and the other half were enrolled in a calculus-based physics course. These students were paid volunteers who responded to a flyer distributed in the introductory physics classes. They had traditional, lecture-based classroom instruction related to physics concepts covered in the tutorial. The interview data were de-identified so it is not possible to match students’ interview data with whether they were enrolled in an algebra-based or calculus-based course. In this deliberate one-on-one interview implementation, students were observed and audio-recorded by a researcher as they worked on the tutorial. The researcher required that the students follow the instructions for working through the tutorial. For example, students were first asked to outline the solution to the tutorial problem to the best of their ability before they started the tutorial. They were required to answer each sub-problem in the appropriate order. Throughout this one-on-one implementation process, each student was asked to think aloud so that the researcher could understand their thought processes and the researcher recorded observations of each student’s interaction with the web-based tool. The researcher remained silent while the students worked and only prompted them to keep talking if they remained silent for a long time. After working through the tutorial, the students worked on the paired problem. Students in the one-on-one interview situation spent between 15 and 30 min on the tutorial. All students had enough time to finish working through the tutorial and the paired problem.
After we found that the tutorial was effective in one-on-one interview settings, it was then implemented as a self-paced learning tool as part of a large, calculus-based introductory physics course. The course was a first semester physics course with 220 students (split into two sections). These students came from varied backgrounds with a majority of them pursuing engineering or physical science degrees. Approximately 60% of the students had taken a high school calculus course and were concurrently enrolled in a college level calculus course. On average, the students were between 18 and 19 years of age. The course was comprised of ~30% females. We note that none of the students in the course had visual disabilities. This implementation allowed the researchers to determine the effectiveness of the tutorial for students in a typical introductory physics course at a research university in the US in which researchers had no control over how the tutorial was used by the students as a self-paced learning tool. Table 1 shows the sequence of the self-study tool activities and recitation quizzes in the introductory physics course.
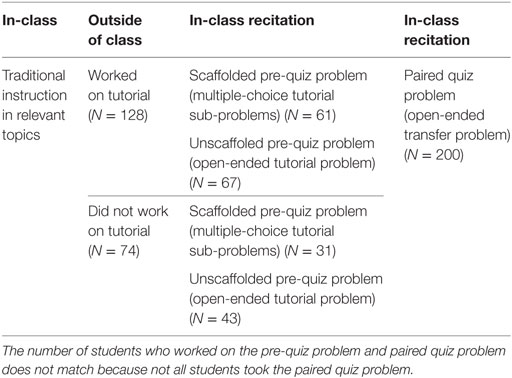
Table 1. Sequence of activities involving the self-study tool in a calculus-based introductory physics course and number of students (N) in each group.
The tutorial was posted on the course website after students had received classroom instruction in relevant concepts. It could be used at a time convenient to each student, but the amount of time each student spent working through it could not be tracked. The tutorial and associated homework problems were assigned in the same week. Instructors incentivized the self-study tutorial by telling students that the tutorial would be helpful for solving assigned homework problems and in-class quiz problems (scaffolded and US pre-quiz problems and paired quiz problems) for that week. Although students were made aware that no points would be awarded simply for completing the web-based tutorial, announcements were made in class, posted on the course website, and sent via email informing students that the tutorial was available after relevant concepts were covered in class.
The scaffolded or US pre-quiz and paired problem were administered in a recitation class in the following week after students had access to the associated web-based tutorial to use as a self-paced learning tool for an entire week. Students in different recitation classes were randomly assigned to either an US or scaffolded pre-quiz condition. Immediately after students submitted the solution to the pre-quiz problem (that was identical to the tutorial problem), they were given the corresponding paired quiz problem (a problem that involves the same physics principles as the tutorial problem shown in Box 1). All students had sufficient time to complete the tutorial and quizzes. Students were given a grade based on their performance on the pre-quiz and paired quiz problems as their weekly quiz grade. On top of each sheet with the paired problem quizzes that were administered in the recitation classes, students were asked questions such as whether they had worked through the online tutorial, whether they thought the tutorial was effective at helping them solve the problem, and how much time they spent on the tutorial (they were told that their answers to these questions would not influence their score on the quiz). These questions allowed us to separate students into “tutorial” or “non-tutorial” groups and determine the performance of students who engaged with the tutorial on the pre-quizzes and paired quizzes.
The purpose of administering the pre-quiz was twofold. First, we wanted to examine whether students who worked through the tutorial as a self-paced learning tool were able to solve the same tutorial problem successfully in a quiz setting without the adaptive support of the tutorial (catering to specific student difficulties). We note that the scaffolded pre-quiz involved breaking the tutorial problem down into sub-problems, but there was no adaptive feature in the pre-quiz and students were not given any feedback or support if they selected an incorrect response to any of the sub-problems on the pre-quiz. The US pre-quiz consisted of an open-ended problem identical to the tutorial and did not break the problem into sub-problems and gave no adaptive support to students. Thus, the pre-quizzes allowed us to examine, in part, how effectively the students engaged with the self-study tutorial (but not necessarily the extent to which they could transfer their learning) by evaluating their performance on the pre-quiz problems that were identical to the tutorial problem with and without scaffolding (and without adaptive support that the tutorial provided). The second purpose of giving the pre-quiz was to compare the performance of students who worked through the tutorial with those who only worked on the corresponding scaffolded pre-quiz (but did not work through the tutorial) on the paired quiz problems. The pre-quizzes enabled us to evaluate whether students who worked on only the pre-quizzes performed better or worse than those who engaged with the tutorial as a self-study tool on paired problems (which were transfer problems involving the same underlying concepts). In this way, we were able to investigate, in part, whether students who worked through the self-paced tutorial engaged with it effectively and were able to transfer their learning to a new context (as opposed to students who only worked through a scaffolded pre-quiz that did not include adaptive learning support).
To compare the performance of students who worked on the tutorial in a one-on-one interview setting with those who used it as a self-study tool, we examined student performance on the paired problem in these two settings. Three graduate students and a professor who do research in physics education iteratively developed a rubric for the paired problem. Once the final version of the rubric was agreed upon, 10% of the paired problem quizzes were graded independently. When the scores were compared, the inter-rater agreement was better than 90% across the three graduate students and professor. In this way, we were able to examine, in part, the level of student engagement with the self-study tutorial in a one-on-one implementation and a large-scale, self-study implementation.
Results
In this section, we refer to students who worked through the tutorial as group “T” and students who did not work through the tutorial as group “NT.” For the different scaffolding pre-quiz conditions, students who worked through a scaffolded pre-quiz are referred to as the “S” group and students who worked through an US pre-quiz are referred to as the “NS” group. Students who were in the one-on-one interview condition are referred to as the “INT” group.
In regards to research question 1 (In the large scale-implementation of the self-paced tutorial, how does the performance of students who worked through the tutorial compare to the performance of students who did not work through the tutorial on “pre-quiz” problems that are identical to the tutorial problem?), we focus on students’ performance on the pre-quiz problems shown in Table 2. For the scaffolded and US pre-quiz in the large-scale implementation of the tutorial in calculus-based physics courses, Table 2 shows that the T group performed better than the NT group on the pre-quiz that involved the same problem as the tutorial problem. A t-test indicated that the T group performed significantly better than the NT group on the scaffolded pre-quiz (p < 0.001) and the US pre-quiz (p < 0.001). This finding suggests that the tutorial was beneficial for helping students be able to at least reproduce the solution to the same problem as the tutorial problem, whether the problem was broken into multiple-choice sub-problems (scaffolded pre-quiz) or an open-ended format (US pre-quiz).
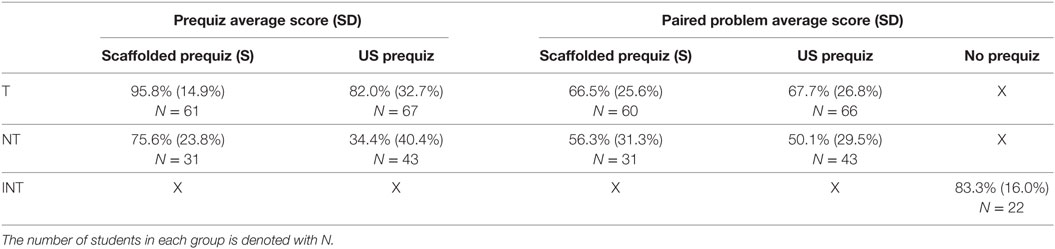
Table 2. Student performance on scaffolded (S) and unscaffolded (US) pre-quizzes and paired quizzes for the conservation of angular momentum tutorial with SDs for the tutorial group (T), non-tutorial group (NT), and one-on-one interview group (INT).
Regarding research question 2 (In the large-scale implementation of the self-paced tutorial, how does the performance of students who only worked through an unscaffolded “pre-quiz” problem compare with the performance of the students who worked through the tutorial on a “paired” quiz problem?), we discuss the students’ performance on the paired problems that required transfer of the learning from the tutorial. Table 2 shows that in the large-scale implementation of the tutorial in calculus-based physics courses, students in the T + US group had an average score of 67.7% on the paired problem that required transfer of learning. On the other hand, students in the NT + US group had an average score of 50.1% on the paired problem. A t-test revealed that students in the T + US group performed significantly better than students in the NT + US group on the paired problem (p = 0.002). This finding suggests that students who worked through the tutorial that included scaffolding support performed better on the paired quiz transfer problem than students who had not been given any scaffolding support via the tutorial or pre-quiz problem.
In regards to research question 3 (In the large-scale implementation of the self-paced tutorial, how does the performance of students who only worked through a scaffolded “pre-quiz” problem compare with the performance of the students who worked through the tutorial on a “paired” quiz problem?), Table 2 shows that students in the NT + S group had an average of 56.3% on the paired quiz problem involving transfer of learning. Students in the T + S group had an average of 66.5% on the paired quiz problem involving transfer of learning. Students in the T + US group had an average of 67.7% on the paired quiz involving transfer of learning, which was not statistically significantly different from the average score of the students in the T + S group on the paired problem (66.5%). A t-test revealed that the average score of the students in the NT + S group on the paired problem (56.3%) is not statistically significantly different from the average score of the students in the T + S group (66.5%) (p = 0.124), nor is it statistically significantly different from the average score of the students in the T + US group (67.7%) (p = 0.798). It appears that students who worked through the tutorial that included both scaffolding support and adaptive features did not perform significantly better on the paired problem that required transfer of learning than students who had only worked through a pre-quiz that included scaffolding support. This finding suggests that students who stated that they worked through the tutorial may not have taken advantage of the adaptive features of the tutorial to help them transfer their learning to new contexts.
We now discuss findings related to research question 4 (How does the performance of students who worked through the tutorial in a monitored, one-on-one interview setting compare to the performance of students who worked through the tutorial as a self-paced learning tool in the large-scale implementation on “paired quiz” problems that involved transfer of learning?). We found that students in the INT group performed significantly better on the paired problem than the students in the T group. Table 2 shows that the students in the INT group had an average of 83% on the paired problem (compared to ~67% for the students in the T group). We note that the INT group was comprised of students in algebra-based and calculus-based physics courses, but we were unable to separate out the scores of students in algebra-based and calculus-based physics courses because the data were de-identified without separating them. However, the SDs of the scores of the INT group on the paired problems are small compared to the SDs of the scores of the T group (the SD on the paired problem for the INT group is 16%, compared to 25.6% in the T group). Thus, it appears that students in the INT group (i.e., students in both algebra-based and calculus-based physics courses) had comparable scores on the paired problems, and so a comparison between the INT group and the calculus-based T group is appropriate. Moreover, prior research suggests that students in the algebra-based introductory physics courses on average perform worse than those in the calculus-based introductory physics (DeVore et al., 2017). Therefore, if we had not de-identified the data and could separate the interview group into two sub-groups (algebra-based vs. calculus-based), the average scores of the calculus-based group would most likely be higher than 83%. In sum, students who worked through the tutorial as a self-study tool in the large-scale implementation of the tutorial performed significantly worse compared to the students who engaged with the tutorial in one-on-one interview settings on the paired quiz problem that required transfer of learning.
Discussion and Interpretation of Findings in Terms of the Self Framework
Our findings suggest that introductory physics students who reported that they worked through the self-paced tutorial on angular momentum conservation performed better than those who did not on pre-quiz problems (even in the unscaffolded version when no support was provided) that were identical to the tutorial problem. This implies that students who reported working through the tutorial were better at reproducing the solution of the tutorial problem than those who did not work through the tutorial. Furthermore, students who worked through the tutorial performed better than those who only worked through an unscaffolded pre-quiz but did not work through the tutorial on the paired problem involving transfer of learning. However, overall, students who worked through the tutorial struggled on the transfer problem and did not perform significantly better than those who only worked through a scaffolded pre-quiz on the paired problem. We also found that students who worked on the tutorial in a one-on-one setting performed significantly better on the paired quiz problem that required transfer of learning than those who worked on the tutorial in the large-scale implementation as a self-paced learning tool.
Our findings suggest that many students who worked through the tutorial without supervision in the large-scale implementation may not have engaged with it in an effective manner. While the students who took advantage of the tutorial performed better on the paired problem than those who worked only through an unscaffolded pre-quiz, the students in the tutorial group did not perform significantly better on the paired problem than those who worked only through a scaffolded pre-quiz. This finding indicates that students may have benefited somewhat from the scaffolding support from the tutorial (i.e., when the tutorial problem was broken down into sub-problems) on the paired problems, but they may not have taken full advantage of the adaptive features of the tutorial that were meant to help them repair their knowledge structure and transfer their learning to new contexts. Furthermore, students who engaged with the tutorial as a self-study tool in the large-scale implementation of the tutorial performed significantly worse than those who worked through the tutorial in the one-on-one interview setting on the paired problem. The students in the one-on-one interview setting were required to work through the tutorial in a deliberate and engaged manner, and they performed well on the paired quiz that involved transfer of learning from the tutorial. This finding indicates that the tutorial was effective in helping students learn physics concepts and transfer their learning to new situations when students engaged with it in a deliberate manner. However, students who used the tutorial as a self-study tool in a large-scale implementation without supervision did not perform as well as those in the one-on-one interview settings on the paired transfer problems, indicating that they may not have taken advantage of the adaptive features of the tutorial in a deliberate and engaged manner. This dichotomy between the performance of the self-study group and one-on-one implementation group on the paired problem suggests that a carefully designed tutorial, when used as intended, can be a powerful learning tool for introductory physics students across diverse levels of prior preparation. However, ensuring that students engage with it effectively as a self-paced learning tool can be challenging.
The significantly worse performance of the tutorial group on the paired quiz problem in the large-scale, self-study implementation (compared to the one-on-one implementation group) may be due to the fact that students engaged superficially with the tutorial. Although these students were given explicit instructions on how to work through the tutorial effectively, they could have taken short cuts and skipped sub-problems if they decided not to adopt a deliberate learning approach while using the web-based tool. Indeed, based upon student comments and other data gathered with their responses to the paired problem in the self-study group, some students explicitly commented that they “skimmed” or “looked over” the tutorial but that type of engagement with the adaptive web-based tool may not help them learn deeply and transfer their learning in order to apply the concepts learned to new situations. Additionally, they may not have attempted to first solve the tutorial problem on their own without the scaffolding provided by the web-based tutorial (even though explicitly told to do so), even though this step would have allowed them to productively struggle with the problem and prime them to learn from the tutorial (Kapur, 2008; Clark and Bjork, 2014). We note that even some of the students in one-on-one interviews needed to be prompted several times to make a prediction for each sub-problem rather than randomly guessing an answer. Furthermore, the written responses of students who used the tutorial as a self-study tool on the paired problem suggest that many of them may have memorized a few equations by skimming through the tutorial. These students may have expected that those equations would help them in solving the paired quiz problem instead of engaging with the self-paced tool in a systematic manner. Interestingly, in a survey given at the end of the course, a majority of students who claimed that they had used the self-paced tutorial stated that they thought that it was helpful. However, their performance on the paired problem reflected that they had not learned effectively from it.
The findings of this study can be interpreted in terms of the SELF framework. We note that the development of the self-paced tutorial discussed here was based upon a cognitive task analysis of the underlying concepts, built on students’ prior knowledge and difficulties, and drew upon cognitive learning theories (i.e., Factors 1 and 2 of the SELF framework). However, we found that the tutorial was not as effective in helping students transfer their learning when implemented as a self-paced learning tool in a large physics course (in which students’ engagement with the tutorial was unsupervised) compared to supervised, one-on-one interview situations, as measured by their performance on a transfer problem. This dichotomy in students’ performance on the problem involving transfer of learning supports the notion in the SELF framework that a major challenge in effectively implementing a research-validated interactive tutorial as a self-study tool is likely to be related to how it was implemented and incentivized (whether students had sufficient incentives to effectively engage with it in a self-paced learning environment), whether students had the motivation, self-regulation, and time-management skills to engage with it and how the constraints of the social environments impacted their engagement (i.e., Factor 4 of the SELF framework) (Ericsson et al., 1993; Winne, 1996; Pintrich, 2003; Narciss et al., 2007; Mason and Singh, 2010; Brown et al., 2016). It appears that without sufficient support to help students develop self-management and time-management skills and providing incentives to motivate students to engage with the self-paced tutorial, many students may not have effectively engaged with it. In particular, the SELF framework supports that haphazard use of a research-validated self-paced tool can reduce its effectiveness significantly and inhibit transfer of learning to new contexts as we found in this investigation. Therefore, it is important for educators and education researchers to contemplate how to provide appropriate incentives and support in order for students to engage effectively and benefit from self-paced learning tools.
While students’ environments are challenging to account for when implementing self-paced learning tools, Factor 3 of the SELF framework focuses on incentives and support students can be provided during the implementation of the self-paced learning tools to improve their level of engagement. Factor 3 posits that the external characteristics of the tools (i.e., how the tools are implemented in classes) may improve student engagement with the tools by taking into account students’ characteristics and environments. In our study, students may have engaged more effectively with the self-paced tutorial if elements from Factor 3 were included in the implementation of the self-study tools. For example, it may be helpful to get student buy-in by having students think carefully about why they should engage deliberately with a self-paced learning tool. Students who struggle with managing their time can be provided guidance in making a daily schedule that includes enough time for learning from self-paced tools. Additionally, the instructor can strive to make connections between self-paced learning assignments and other in-class lessons or out of class activities and assessments to help students engage with the self-paced learning tools more effectively.
Moreover, some students may not have engaged effectively with the self-paced tutorial in our study due to self-efficacy issues or unproductive beliefs about learning as suggested in the SELF framework. In particular, students who have low self-efficacy (Bandura, 1997; Moos and Azevedo, 2009) and/or unproductive epistemological beliefs about learning in a particular discipline such as physics (e.g., physics is just a collection of facts and formulas, only a few smart people can do physics, and that learning physics involves memorizing physics formulas and reiterating them on exams) (Hammer, 1994a,b; Redish et al., 1998; Maries et al., 2016) are unlikely to productively engage with the self-paced learning tools. Therefore, students who have difficulty engaging with the self-study tools due to lack of self-efficacy or unproductive epistemological beliefs can be helped to improve their self-efficacy and develop productive epistemological beliefs. For example, a short online intervention has been shown to improve student self-efficacy (Mangels et al., 2006). These issues are important to address in order to ensure that students who are most in need of learning from self-paced learning tools benefit from them and can flexibly transfer their learning to new situations (Tinto, 1993, 1997; Braxton, 2000; Braxton et al., 2004; Herzog, 2005; Diaz and Cartnal, 2006; Anderson, 2011; Boston and Ice, 2011; Boston et al., 2011; DeAngelo et al., 2011; Campbell and Mislevy, 2013).
Moreover, the SELF framework proposes that another factor that may help students effectively engage with self-paced learning tools is encouraging them to engage in learning communities. In these learning communities, all students would be expected to learn from the self-study tools and then engage in some follow up activities in a group environment (either electronically or physically, depending on the class). Thus, individual students are accountable to their group members and are encouraged to engage with self-study assignments and activities deliberately to prepare for the group activities. For example, in the study discussed here, if students were assigned to work in a learning community on a complex physics problem after engaging with the self-paced learning tool, they may have had more incentive to engage deeply with the tutorial individually in order to prepare for the group work.
Furthermore, incorporating grade incentives (Morrison et al., 1995; Li et al., 2015) to engage with the self-study tool is another factor that can increase student engagement (see Factor 3 of the framework). For example, instructors can give course credit to students based on their answers to each sub-problem with decreasing scores if they answer the same sub-problem multiple times. This strategy might encourage students to answer each sub-problem carefully instead of guessing the answers. In addition, if students in the study described here were asked to submit a copy of the correct answer to each sub-problem and explain their reasoning, this practice may have increased their motivation to deliberately engage with the self-study tool and help them transfer their learning to new contexts.
It is also important to note that we cannot disentangle any of the factors in the SELF framework and how they impact student engagement with a self-paced learning tool. For example, students who are lacking prior preparation (factor 2 of the framework) may also have difficulty with time-management (Factor 4 of the framework). Furthermore, when students work in learning communities that keep each student accountable while providing mutual support (see Factor 3 of the framework), they may manage their time better (see Factor 4 of the framework). To help students learn effectively from the self-study tools and transfer their learning to new contexts, the characteristics of the learning tools (Factors 1 and 3) should take into account the characteristics of the students and their environments (Factors 2 and 4). In particular, Factor 3, which is often ignored by educators who develop and/or implement self-study tools, is a critical aspect of ensuring that students effectively engage with self-paced learning tools and learn to transfer their learning to new situations.
Our investigation suggests that, despite the ease and convenience of accessing adaptive, self-paced tutorials, there are challenges in ensuring that students, especially those who in need of out-of-class scaffolding support, engage with them effectively. Even well-designed self-study tools that take into account students’ prior knowledge and difficulties and are based on cognitive learning theories may not necessarily help students transfer their learning if students do not engage with them effectively due to motivational or environmental factors. Thus, if a learning tool is aimed at improving students’ transfer of learning, deep engagement is necessary and it is crucial that the developers and implementers of the self-paced tool appropriately take into account factors such as students’ motivation, self-regulation and time-management skills, and social environments (i.e., Factors 2 and 4 of the SELF framework). It is possible that if students are provided supports and incentives such as those in Factor 3 of the SELF framework, they may engage with research-validated self-study tool such as the tutorial described here more effectively and their transfer of learning to new contexts will be improved.
Ethics Statement
We obtained IRB approval to work with human subjects.
Author Contributions
Each other contributed equally to the article.
Conflict of Interest Statement
The authors declare that the research was conducted in the absence of any commercial or financial relationships that could be construed as a potential conflict of interest.
Acknowledgments
We thank the US National Science Foundation for financial support (grant numbers PHY-1505460 and DUE-1524575) and members of the University of Pittsburgh physics education research group for their help with the rubric development and grading to establish inter-rater reliability.
Funding
US National Science Foundation for financial support (grant numbers PHY-1505460 and DUE-1524575).
References
Allen, I., and Seaman, J. (2013). Changing Course: Ten Years of Tracking Online Education in the U.S. Sloan Consortium. Available at: http://www.onlinelearningsurvey.com/reports/changingcourse.pdf
Alraimi, K., Zo, H., and Ciganek, A. (2015). Understanding the MOOCs continuance: the role of openness and reputation. Comput. Educ. 80, 28. doi: 10.1016/j.compedu.2014.08.006
Azevedo, R. (2005). Special issue on computers as metacognitive tools for enhancing student learning, computer environments as metacognitive tools for enhancing learning. Educ. Psychol. 40, 193. doi:10.1207/s15326985ep4004_1
Azevedo, R., Cromley, J., Winters, F., Moos, D., and Greene, J. (2005). Special issue on scaffolding self-regulated learning and metacognition: implications for the design of computer-based scaffolds, adaptive human scaffolding facilitates adolescents’ self-regulated learning with hypermedia. Instr. Sci. 33, 381. doi:10.1007/s11251-005-1273-8
Azevedo, R., Guthrie, J., and Seibert, D. (2004). The role of self-regulated learning in fostering students’ conceptual understanding of complex systems with hypermedia. J. Educ. Comput. Res. 30, 87. doi:10.2190/DVWX-GM1T-6THQ-5WC7
Boston, W., and Ice, P. (2011). Assessing retention in online learning: an administrative perspective. Online J. Dist. Learn. Admin. 14, 2.
Boston, W., Ice, P., and Gibson, A. (2011). Comprehensive assessment of student retention in online learning environments. Online J. Dist. Learn. Admin. 14, 1.
Bower, M., Dalgarno, B., Kennedy, G., Lee, M., and Kenney, J. (2015). Design and implementation factors in blended synchronous learning environments: outcomes from a cross-case analysis. Comput. Educ. 86, 1. doi:10.1016/j.compedu.2015.03.006
Braxton, J. (2000). Reworking the Student Departure Puzzle. Nashville, TN: Vanderbilt University Press.
Braxton, J., Hirschy, A., and McClendon, S. (2004). Understanding and Reducing College Student Departure, ASHE-ERIC Higher Education Report, Vol. 30. San Francisco, CA: Jossey-Bass.
Breslow, L., Pritchard, D., DeBoer, J., Stump, G., Ho, A., and Seaton, D. (2013). Studying learning in the worldwide classroom research into edX’s first MOOC. Res. Pract. Assess. 8, 13.
Britton, B., and Tesser, A. (1991). Effects of time-management practices on college grades. J. Educ. Psychol. 83, 405–410. doi:10.1037/0022-0663.83.3.405
Brown, A. (1997). Transforming schools into communities of thinking and learning about serious matters. Am. Psychol. 52, 399–413. doi:10.1037/0003-066X.52.4.399
Brown, B., Mason, A., and Singh, C. (2016). Improving performance in quantum mechanics with explicit incentives to correct mistakes. Phys. Rev. Phys. Educ. Res. 12, 010121. doi:10.1103/PhysRevPhysEducRes.12.010121
Brown, B., and Singh, C. (2015). “Development and evaluation of a quantum interactive learning tutorial on Larmor precession of spin,” in Proceedings of the Physics Education Research Conference 2014, eds P. Engelhardt, A. Churukian, and D. Jones (Minneapolis, MN). doi:10.1119/perc.2014.pr.008
Campbell, C., and Mislevy, J. (2013). Students’ perceptions matter: early signs of undergraduate student retention/attrition. J. Coll. Stud. Ret. 14, 467. doi:10.2190/CS.14.4.c
Chandra, V., and Watters, J. (2012). Re-thinking physics teaching with web-based learning. Comput. Educ. 58, 631. doi:10.1016/j.compedu.2011.09.010
Chang, C. (2001). A problem-solving based computer-assisted tutorial for the earth sciences. J. Comput. Assist. Learn. 17, 263–274. doi:10.1046/j.0266-4909.2001.00181.x
Chen, Z., and Gladding, G. (2014). How to make a good animation: a grounded cognition model of how visual representation design affects the construction of abstract physics knowledge. Phys. Rev. ST Phys. Educ. Res. 10, 010111. doi:10.1103/PhysRevSTPER.10.010111
Chen, Z., Stelzer, T., and Gladding, G. (2010). Using multi-media modules to better prepare students for introductory physics lecture. Phys. Rev. ST Phys. Educ. Res. 6, 010108. doi:10.1103/PhysRevSTPER.6.010108
Chi, M. (1994). “Thinking aloud,” in The Think Aloud Method, eds M. W. van Someren, Y. F. Barnard, and J. A. C. Sandberg (London: Academic Press).
Chi, M., Slotta, J., and de Leeuw, N. (1994). From things to processes: a theory of conceptual change for learning science concepts. Learn. Instruct. 4, 27–43. doi:10.1016/0959-4752(94)90017-5
Clark, C. M., and Bjork, R. A. (2014). “When and why introducing difficulties and errors can enhance instruction,” in Applying the Science of Learning in Education: Infusing Psychological Science into the Curriculum, eds V. A. Benassi, C. E. Overson, and C. M. Hakala. Available at: www.teachpsych.org/ebooks/asle2014/index.php
Collins, A., Brown, J., and Newman, S. (1989). “Cognitive apprenticeship: teaching the crafts of reading, writing and mathematics,” in Knowing, learning, and instruction: Essays in honor of Robert Glaser, ed. L. B. Resnick (Hillsdale, NJ: Lawrence Erlbaum), 453–494.
Colvin, K., Champaign, J., Liu, A., Zhou, Q., Fredericks, C., and Pritchard, D. (2014). Learning in an introductory physics MOOC: all cohorts learn equally, including an on-campus class. Int. Rev. Res. Open Dist. Learn. 15, 263.
DeAngelo, L., Franke, R., Hurtado, S., Pryor, J., and Tran, S. (2011). Completing College: Assessing Graduation Rates at Four-Year Institutions. Los Angeles, CA: UCLA Higher Education Research Institute.
Debowska, E., Girwidz, R., Greczyło, T., Kohnle, A., Mason, B., Mathelitsch, L., et al. (2012). Report and recommendations on multi-media materials for teaching and learning electricity and magnetism. Eur. J. Phys 34, L47–L54. doi:10.1088/0143-0807/34/3/L47
Deci, E., Eghrari, H., Patrick, B., and Leone, D. (1994). Facilitating internalization: the self-determination theory perspective. J. Pers. 62, 119–142. doi:10.1111/j.1467-6494.1994.tb00797.x
Demetriadis, S., Papadopoulos, P., Stamelos, I., and Fischer, F. (2008). The effect of scaffolding students’ context-generating cognitive activity in technology-enhanced case-based learning. Comput. Educ. 51, 939. doi:10.1016/j.compedu.2007.09.012
DeVore, S., Gauthier, A., Levy, J., and Singh, C. (2016a). Development and evaluation of a tutorial to improve students’ understanding of a lock-in amplifier. Phys. Rev. Phys. Educ. Res. 12, 020127. doi:10.1103/PhysRevPhysEducRes.12.020127
DeVore, S., Gauthier, A., Levy, J., and Singh, C. (2016b). Improving student understanding of lock-in amplifiers. Am. J. Phys. 84, 52. doi:10.1119/1.4934957
DeVore, S., Marshman, E., and Singh, C. (2017). Challenge of engaging all students via self-paced interactive electronic learning tutorials for introductory physics. Phys. Rev. PER 13, 010127. doi:10.1103/PhysRevPhysEducRes.13.010127
DeVore, S., and Singh, C. (2015). “Development of an interactive tutorial on quantum key distribution,” in Proceedings of the Physics Education Research Conference 2014, eds P. Engelhardt, A. Churukian, and D. Jones (Minneapolis, MN). doi:10.1119/perc.2014.pr.011
Diaz, D., and Cartnal, R. (2006). Term length as an indicator of attrition in online learning. Innov. J. Online Educ. 2, 5.
Engle, R., and Conant, F. (2002). Guiding principles for fostering productive disciplinary engagement: explaining an emergent argument in a community of learning classroom. Cogn. Instruct. 20, 399–483. doi:10.1207/S1532690XCI2004_1
Ericsson, A., Krampe, R., and Tesch-Romer, C. (1993). The role of deliberate practice in the acquisition of expert performance. Psychol. Rev. 100, 363. doi:10.1037/0033-295X.100.3.363
Ericsson, A., and Simon, H. (1993). Protocol Analysis: Verbal Reports as Data, revised ed. Cambridge, MA: MIT Press.
Farrell, J., Moog, R., and Spencer, J. (1999). A guided inquiry chemistry course. J. Chem. Educ. 76, 570–574. doi:10.1021/ed076p570
Fryer, J., and Elliott, A. (2008). “Self-regulation of achievement goal pursuit,” in Motivation and Self-Regulated Learning: Theory, Research and Applications, eds D. H. Schunk, and B. J. Zimmerman (New York, NY: Lawrence Erlbaum), 53.
Gick, M., and Holyoak, K. (1983). Schema induction and analogical transfer. Cog. Psy. 15, 1–38. doi:10.1016/0010-0285(83)90002-6
Gick, M., and Holyoak, K. (1987). “The cognitive basis of knowledge transfer,” in Transfer of Learning: Contemporary Research and Applications, eds S. Cormier, and J. Hagman (San Diego, CA: Academic Press), 9–46.
Greene, J., Costa, I., Robertson, J., Pan, Y., and Deekens, V. (2010). Exploring relations among college students’ prior knowledge, implicit theories of intelligence, and self-regulated learning in a hypermedia environment. Comput. Educ. 55, 1027. doi:10.1016/j.compedu.2010.04.013
Grolnick, W., Gurland, S., Jacob, K., and Decourcey, W. (2002). “The development of self-determination in middle childhood and adolescence,” in Development of Achievement Motivation, eds A. Wigfield, and J. Eccles (San Diego, CA: Academic Press), 147–171.
Hammer, D. (1994a). Epistemological beliefs in introductory physics. Cogn. Instruct. 12, 151. doi:10.1207/s1532690xci1202_4
Hammer, D. (1994b). Students’ beliefs about conceptual knowledge in introductory physics. Int. J. Sci. Educ. 16, 385. doi:10.1080/0950069940160402
Heller, P., and Hollabaugh, M. (1992). Teaching problem solving through cooperative grouping. Part 2: designing problems and structuring groups. Am. J. Phys. 60, 637. doi:10.1119/1.17118
Herzog, S. (2005). Measuring determinants of student return vs. dropout/stopout vs. transfer: a first-to-second year analysis of new freshmen. Res. High. Educ. 46, 883. doi:10.1007/s11162-005-6933-7
Hsieh, P., Sullivan, J., and Guerra, N. (2007). A closer look at college students: self-efficacy and goal orientation. J. Adv. Acad. 18, 454. doi:10.4219/jaa-2007-500
Hulleman, C., Durik, A., Schweigert, S., and Harachiewicz, J. (2008). Task values, achievement goals, and interest: an integrative analysis. J. Educ. Psychol. 100, 398. doi:10.1037/0022-0663.100.2.398
Jang, H. (2008). Supporting students’ motivation, engagement, and learning during an uninteresting activity. J. Educ. Psychol. 100, 798–811. doi:10.1037/a0012841
Kohnle, A., Douglass, M., Edwards, T. J., Gillies, A. D., Hooley, C. A., and Sinclair, S. D. (2010). Developing and evaluating animations for teaching quantum mechanics concepts. Eur. J. Phys. 31, 1441. doi:10.1088/0143-0807/31/6/010
Korkmaz, A., and Harwood, W. (2004). Web-supported chemistry education: design of an online tutorial for learning molecular symmetry. J. Sci. Educ. Technol. 13, 243–253. doi:10.1023/B:JOST.0000031263.82327.6e
Kulik, C., and Kulik, J. (1991). Effectiveness of computer-based instruction: an updated analysis. Comput. Hum. Behav. 7, 75. doi:10.1016/0747-5632(91)90030-5
Kulik, J. (1994). “Meta-analytic studies of findings on computer-based instruction,” in Technology Assessment in Education, and Training, eds E. Baker, and H. Jr. O’Neil (New York, NY: Routledge), 9.
Lenaerts, J., Wieme, W., Janssens, F., and Van Hoecke, T. (2002). Designing digital resources for a physics course. Eur. J. Phys. 23, 175–182. doi:10.1088/0143-0807/23/2/311
Li, L., Li, B., and Luo, Y. (2015). Using a dual safeguard web-based interactive teaching approach in an introductory physics class. Phys. Rev. ST Phys. Educ. Res. 11, 010106. doi:10.1103/PhysRevSTPER.11.010106
Mangels, J., Butterfield, B., Lamb, J., Good, C., and Dweck, C. (2006). Why do beliefs about intelligence influence learning success? A social cognitive neuroscience model. Soc. Cogn. Affect. Neurosci. 1, 75. doi:10.1093/scan/nsl013
Margaryan, A., Bianco, M., and Littlejohn, A. (2015). Instructional quality of massive open online courses. Comput. Educ. 80, 77. doi:10.1016/j.compedu.2014.08.005
Maries, A., Lin, S., and Singh, C. (2016). “The impact of students’ epistemological framing on a task requiring representational consistency,” in Proceedings of the Physics Education Research Conference 2016 (Sacramento, CA). doi:10.1119/perc.2016.pr.048
Marshman, E., Kalender, Z., Schunn, C., Nokes-Malach, T., and Singh, C. (2017). A longitudinal analysis of students’ motivational characteristics in introductory physics courses: gender differences. Can. J. Phys. doi:10.1139/cjp-2017-0185
Marshman, E., and Singh, C. (2016). Interactive tutorial to improve student understanding of single photon experiments involving a Mach-Zehnder Interferometer. Eur. J. Phys. 37, 024001. doi:10.1088/0143-0807/37/2/024001
Marshman, E., and Singh, C. (2017a). Investigating and improving student understanding of quantum mechanics in the context of single photon interference. Phys. Rev. Phys. Educ. Res. 13, 010117. doi:10.1103/PhysRevPhysEducRes.13.010117
Marshman, E., and Singh, C. (2017b). Investigating and improving student understanding of the probability distributions for measuring physical observables in quantum mechanics. Eur. J. Phys. 38, 025705. doi:10.1088/1361-6404/aa57d1
Marshman, E., and Singh, C. (2017c). Investigating and improving student understanding of the expectation values of observables in quantum mechanics. Eur. J. Phys. 38, 045701. doi:10.1088/1361-6404/aa6d34
Marshman, E., and Singh, D. (2015). “Developing an interactive tutorial on a quantum eraser,” in Proceedings of the Physics Education Research Conference 2014, eds P. Engelhardt, A. Churukian, and D. Jones (Minneapolis, MN). doi:10.1119/perc.2014.pr.040
Mason, A., and Singh, C. (2010). Do advanced students learn from their mistakes without explicit intervention? Am. J. Phys. 78, 760. doi:10.1119/1.3318805
McDermott, L. (1996). Development of a computer-based tutorial on the photoelectric effect. Am. J. Phys. 64, 1370–1379. doi:10.1119/1.18360
Moos, D., and Azevedo, R. (2008). Exploring the fluctuation of motivation and use of self-regulatory processes during learning with hypermedia. Instr. Sci. 36, 203. doi:10.1007/s11251-007-9028-3
Moos, D., and Azevedo, R. (2009). Learning with computer-based learning environments: a literature review of computer self-efficacy. Rev. Educ. Res. 79, 576. doi:10.3102/0034654308326083
Morrison, G., Ross, S., Gopalakrishnan, M., and Casey, J. (1995). The effects of feedback and incentives on achievement in computer-based instruction. Contemp. Educ. Psychol. 20, 32. doi:10.1006/ceps.1995.1002
Narciss, S., Proske, A., and Koerndle, H. (2007). Promoting self-regulated learning in web-based learning environments. Comput. Hum. Behav. 23, 1126. doi:10.1016/j.chb.2006.10.006
Nokes-Malach, T., and Mestre, J. (2013). Toward a model of transfer as sense-making. Educ. Psy. 48, 184–207. doi:10.1080/00461520.2013.807556
Pintrich, P. (2003). A motivational science perspective on the role of student motivation in learning and teaching contexts. J. Educ. Psychol. 95, 667–686. doi:10.1037/0022-0663.95.4.667
Posner, G., Strike, K., Hewson, W., and Gertzog, W. (1982). Accommodation of a scientific conception: toward a theory of conceptual change. Sci. Educ. 66, 211. doi:10.1002/sce.3730660207
Redish, E., Saul, J., and Steinberg, R. (1998). Student expectations in introductory physics. Am. J. Phys. 66, 212. doi:10.1119/1.18847
Reif, F. (1987). Instructional design, cognition, and technology: applications to the teaching of scientific concepts. J. Res. Sci. Teach. 24, 309. doi:10.1002/tea.3660240405
Reif, F., and Scott, L. (1999). Teaching scientific thinking skills: students and computers coaching each other. Am. J. Phys. 67, 819l–883l. doi:10.1119/1.19130
Sayer, R., Maries, A., and Singh, C. (2015). “Developing and evaluating a tutorial on the double-slit experiment,” in Proceedings of the Physics Education Research Conference 2015, eds A. Churukian, D. Jones, and L. Ding (College Park, MD). doi:10.1119/perc.2015.pr.070
Sayer, R., Maries, A., and Singh, C. (2017). A quantum interactive learning tutorial on the double-slit experiment to improve student understanding of quantum mechanics. Phys. Rev. PER 13, 010123. doi:10.1103/PhysRevPhysEducRes.13.010123
Schwartz, D., Bransford, J., and Sears, D. (2005). “Efficiency and innovation in transfer,” in Transfer of Learning from a Modern Multidisciplinary Perspective, ed. J. Mestre (Greenwich, CT: Information Age), 1.
Seaton, D., Bergner, Y., Chuang, I., Mitros, P., and Pritchard, D. (2014). Who does what in a massive open online course? Commun. ACM 57, 58. doi:10.1145/2500876
Shaffer, P., and McDermott, L. (1992). Research as a guide for curriculum development: an example from introductory electricity. Part II: design of instructional strategies. Am. J. Phys 60, 1003. doi:10.1119/1.16979
Singh, C. (2004). Interactive video tutorials for enhancing problem-solving reasoning, and meta-cognitive skills of introductory physics students. AIP Conf. Proc. 720, 177. doi:10.1063/1.1807283
Singh, C. (2008a). Computer-Based Tutorials to Develop Expertise in Introductory Physics Students, American Physical Society (APS) Forum on Education Newsletter, Summer, 13. Available at: https://www.aps.org/units/fed/newsletters/summer2008/singh.cfm
Singh, C. (2008b). Interactive learning tutorials on quantum mechanics. Am. J. Phys. 76, 400. doi:10.1119/1.2837812
Singh, C. (2008c). Assessing student expertise in introductory physics with isomorphic problems. I. Performance on a non-intuitive problem pair from introductory physics. Phys. Rev. ST Phys. Educ. Res. 4, 010104. doi:10.1103/PhysRevSTPER.4.010104
Singh, C. (2008d). Assessing student expertise in introductory physics with isomorphic problems. II. Effect of some potential factors on problem solving and transfer. Phys. Rev. ST Phys. Educ. Res. 4, 010105. doi:10.1103/physrevstper.4.010105
Singh, C., Belloni, M., and Christian, W. (2006). Improving students’ understanding of quantum mechanics. Phys. Today 59, 43. doi:10.1063/1.2349732
Singh, C., and Haileselassie, D. (2010). Developing problem solving skills of students taking introductory physics via web-based tutorials. J. Coll. Sci. Teach. 39, 34. Available at: https://www.learntechlib.org/p/108402/
Singh, C., and Marshman, E. (2015). “Developing an interactive tutorial on a Mach-Zehnder interferometer with single photons,” in Proceedings of the Physics Education Research Conference 2014, eds P. Engelhardt, A. Churukian, and D. Jones (Minneapolis, MN). doi:10.1119/perc.2014.pr.056
Song, H., Kalett, A., and Plass, J. (2016). Interplay of prior knowledge, self-regulation and motivation in complex multimedia learning environments. J. Comput. Assist. Learn. 32, 31. doi:10.1111/jcal.12117
Stewart, J., DeVore, S., Stewart, G., and Michaluk, L. (2016). Behavioral self-regulation in a physics class. Phys. Rev. PER 12, 010125.
Sungur, S. (2007). Contribution of motivational beliefs and metacognition to students’ performance under consequential and nonconsequential test conditions. Educ. Res. Eval. 13, 127. doi:10.1080/13803610701234898
Tinto, V. (1993). Leaving College: Rethinking the Causes and Cures of Student Attrition. Chicago, IL: University of Chicago Press.
Tinto, V. (1997). Classrooms as communities: exploring the educational character of student persistence. J. Higher Educ. 68, 599. doi:10.2307/2959965
Wagner, A., Altherr, S., Eckert, B., and Jorg Jodl, H. (2006). Multimedia in physics education: two teaching videos on the absorption and emission spectrum of sodium. Eur. J. Phys. 27, L31–L35. doi:10.1088/0143-0807/27/6/L01
Wieman, C. (2015). Comparative cognitive task analyses of experimental science and instructional laboratory courses. Phys. Teach. 53, 349. doi:10.1119/1.4928349
Wigfield, A., Hoa, L., and Klauda, S. (2008). “The role of achievement values in the regulation of achievement behaviors,” in Motivation and Self-Regulated Learning: Theory, Research and Applications, eds D. Schunk, and B. Zimmerman (New York, NY: Lawrence Erlbaum), 169.
Winne, P. (1996). A metacognitive view of individual differences in self-regulated learning. Learn. Individ. Differ. 8, 327. doi:10.1016/S1041-6080(96)90022-9
Wood, A., Galloway, R., Donnelly, R., and Hardy, J. (2016). Characterizing interactive engagement activities in a flipped introductory physics class. Phys. Rev. PER 12, 010140.
Yalcinalp, S., Geban, O., and Ozkan, I. (1995). Effectiveness of using computer assisted supplementary instruction for teaching the mole concept. J. Res. Sci. Teach. 32, 1083–1095. doi:10.1002/tea.3660321007
Yerushalmi, E., Cohen, E., Mason, A., and Singh, C. (2012a). What do students do when asked to diagnose their mistakes? Does it help them? I. An atypical quiz context. Phys. Rev. ST Phys. Educ. Res. 8, 020109. doi:10.1103/PhysRevSTPER.8.020109
Yerushalmi, E., Cohen, E., Mason, A., and Singh, C. (2012b). What do students do when asked to diagnose their mistakes? Does it help them? II. A more typical quiz context. Phys. Rev. ST PER 8, 020110. doi:10.1103/physrevstper.8.020110
Zhu, G., and Singh, C. (2011). Improving students’ understanding of quantum mechanics via the Stern-Gerlach experiment. Am. J. Phys. 79, 499. doi:10.1119/1.3546093
Zhu, G., and Singh, C. (2012a). Improving students’ understanding of quantum measurement: I. Investigation of difficulties. Phys. Rev. ST Phys. Educ. Res. 8, 010117. doi:10.1103/PhysRevSTPER.8.010117
Zhu, G., and Singh, C. (2012b). Improving students’ understanding of quantum measurement: II. Development of research-based learning tools. Phys. Rev. ST Phys. Educ. Res. 8, 010118. doi:10.1103/PhysRevSTPER.8.010118
Zhu, G., and Singh, C. (2013). Improving student understanding of addition of angular momentum in quantum mechanics. Phys. Rev. ST Phys. Educ. Res. 9, 010101. doi:10.1103/PhysRevSTPER.9.010101
Keywords: self-paced learning, adaptive learning, personalized learning, transfer of learning, physics education research
Citation: Marshman EM, DeVore S and Singh C (2018) Challenge of Helping Introductory Physics Students Transfer Their Learning by Engaging with a Self-Paced Learning Tutorial. Front. ICT 5:3. doi: 10.3389/fict.2018.00003
Received: 24 October 2017; Accepted: 12 February 2018;
Published: 07 March 2018
Edited by:
James Davis Slotta, Boston College, United StatesReviewed by:
S. Raj Chaudhury, University of South Alabama, United StatesLicia Maria Rita Montagna, Humanitas Research Hospital, Italy
Copyright: © 2018 Marshman, DeVore and Singh. This is an open-access article distributed under the terms of the Creative Commons Attribution License (CC BY). The use, distribution or reproduction in other forums is permitted, provided the original author(s) and the copyright owner are credited and that the original publication in this journal is cited, in accordance with accepted academic practice. No use, distribution or reproduction is permitted which does not comply with these terms.
*Correspondence: Emily Megan Marshman, ZW1tMTAxQHBpdHQuZWR1