- Media Psychology and Media Design Group, Department of Economic Sciences and Media, Institute for Media and Communication Science, TU Ilmenau, Ilmenau, Germany
Good public speaking skills are essential in many professions as well as everyday life, but speech anxiety is a common problem. While it is established that public speaking training in virtual reality (VR) is effective, comprehensive studies on the underlying factors that contribute to this success are rare. The “quality evaluation of user-system interaction in virtual reality” framework for evaluation of VR applications is presented that includes system features, user factors, and moderating variables. Based on this framework, variables that are postulated to influence the quality of a public speaking training application were selected for a first validation study. In a cross-sectional, repeated measures laboratory study [N = 36 undergraduate students; 36% men, 64% women, mean age = 26.42 years (SD = 3.42)], the effects of task difficulty (independent variable), ability to concentrate, fear of public speaking, and social presence (covariates) on public speaking performance (dependent variable) in a virtual training scenario were analyzed, using stereoscopic visualization on a screen. The results indicate that the covariates moderate the effect of task difficulty on speech performance, turning it into a non-significant effect. Further interrelations are explored. The presenter’s reaction to the virtual agents in the audience shows a tendency of overlap of explained variance with task difficulty. This underlines the need for more studies dedicated to the interaction of contributing factors for determining the quality of VR public speaking applications.
Introduction
Virtual reality (VR) technology as a tool offers great possibilities for training and therapy purposes. It provides a new and complex human–computer interaction paradigm (Nijholt, 2014), since users are no longer “external observers of images on a computer screen but are active participants in a computer-generated three-dimensional (3D) world” (Bowman and Hodges, 1999, p. 37). With VR applications, ecologically valid training and therapy scenarios can be presented that otherwise are hard to realize (for example, for training a presentation in front of a large audience or an audience with a different cultural background). Especially in comparison with traditional methods, they provide further advantages: stimulus presentation can be controlled and adapted to the clients’ progress, the scenarios are safe and minimize consequences of mistakes and are, therefore, often more acceptable, and virtual agents can be integrated into applications that aim at the training of social interactions (Wiederhold and Wiederhold, 2005b).
To date, a considerable amount of research was conducted on VR applications in a clinical context, investigating the use of virtual reality exposure therapy (VRET) for anxiety disorders. Meta-analyses show on the one hand that VRET leads to considerable reduction of negative affective symptoms for anxiety disorders and phobias like posttraumatic stress disorder (PTSD), social phobia, arachnophobia, acrophobia, panic disorder with agoraphobia, and aviophobia (Parsons and Rizzo, 2008). On the other hand, VRET also seems to be a promising intervention compared to classical evidence-based treatments for anxiety disorders. A meta-analysis by Opris et al. (2012) analyzed VRET outcomes for fear of flying, panic disorder/agoraphobia, social phobia, arachnophobia, acrophobia, and PTSD. The findings show that VRET leads to better outcomes than waiting list control. Further, VRET shows similar efficacy than classical interventions without VR exposure and comparable real-life impact with a good stability over time. Similar results have been obtained by another meta-analysis on VRET for specific phobias, social phobia, PTSD, and panic disorder, showing even a small effect size in favor of VRET over in vivo exposure (Powers and Emmelkamp, 2008). However, moderator analyses on these meta-analytic effects (e.g., influence of presence, immersion, or demographics) are often limited due to inconsistent reporting in the literature (Parsons and Rizzo, 2008).
One upcoming application context for VR social anxiety applications is public speaking therapy and training applications. Good public speaking skills are nowadays important for many professions and in everyday life. However, they require extensive training (Chollet et al., 2015). At the same time, fear of public speaking or public speaking anxiety is one of the most common social phobias in the world (Lee et al., 2002). It is characterized by anxiety even prior to or at the thought of having to communicate verbally with any group of people. For phobic people, it even leads to avoidance of such events that focus the group’s attention on themselves. Fear of public speaking can lead to physical distress and even panic (Rothwell, 2004) and lower speech performance (Menzel and Carrell, 1994). State anxiety needs to be distinguished from trait anxiety as a “personality trait,” though: state anxiety is “dependent upon both the person (trait anxiety) and the stressful situation” (Endler and Kocovski, 2001, p. 242). This means that the anxiety or fear experienced and triggered in a specific situation like giving a public speech should be considered and assessed as state anxiety (Menzel and Carrell, 1994). Treatment involves cognitive behavioral therapy (CBT), which includes exposure to fear-triggering stimuli (e.g., speaking in front of a group), reframing thoughts associated with the social scene, social skills training, and relaxation training (Wiederhold and Wiederhold, 2005a). Clinical VR public speaking applications are well researched. The findings are in line with state of research on anxiety disorders discussed above. Virtual audiences can induce anxiety in phobic and non-phobic people (Pertaub et al., 2002; Slater et al., 2006). Further, repeated exposure to a virtual audience can result in reduction of fear of public speaking symptoms (Wallach et al., 2009). In general, virtual fear of public speaking applications can be considered as an effective supplement in CBT, especially when compared to waiting list control conditions (Wallach et al., 2009). Recent findings suggest that VR public speaking applications might be a promising tool for training also: public speaking training applications with high simulation fidelity that depict realistic audiences do not only lead to higher presence (which is defined as the user’s psychological response to a VR system; Slater, 2003) and performance, but also to better transfer of gained skills into practice (Kothgassner et al., 2012).
Given the increasing distribution of VR public speaking applications, the need for systematic evaluation of their quality arises. High quality applications fulfill their expected purpose, with as few resources possible in a satisfying way (see Section on “Quality”). In the case of public speaking, training in VR should increase speech performance to be successful and effective. Various factors determine training success, which will be discussed in the following paragraphs.
Virtual reality training applications provide tasks to be fulfilled by the users. One of the most important task aspects is task difficulty (Sheridan, 1992). It is widely recognized and implemented in VR applications, especially in rehabilitation/training (Sveistrup, 2004) or assessment applications (Neguţ et al., 2016). A recent meta-analysis compared task difficulty of VR assessment tools for cognitive performance with paper-pencil and computerized measures. The findings suggest that tasks in VR have on the one hand high ecological validity, as high fidelity VR closely replicates “real world environments with stressors, distractors, and complex stimuli” (Neguţ et al., 2016; p. 418). On the other hand, they can also have an increased level of complexity compared to tasks in more traditional cognitive performance measures for the same reasons. They afford more cognitive resources, as a larger amount of information needs to be manipulated and processed while fulfilling assessment tasks (Neguţ et al., 2016). However, design of the virtual environment plays a role: poor display and/or interaction fidelity can decrease task performance, whereas good design might lower task difficulty and lead to better performance (Stickel et al., 2010; McMahan et al., 2012). This highlights the importance of guided design (Bowman and Hodges, 1999). Further, task difficulty is related not only to performance, but also to presence in VR, because all these variables depend on the allocation of cognitive resources. Given the limitation of human cognitive resources, those allocated to the task at hand cannot be invested, for example, in the experience of presence (Nash et al., 2000). State of research shows mixed results in this aspect: simple and highly automated tasks will probably not require a high level of presence in order to show high performance. More complicated tasks show a differentiated pattern: on the one hand, they seem to have a negative effect on presence (Riley, 2001; Slater et al., 1998), as more cognitive resources are allocated to the task and less to the environment (Nash et al., 2000). On the other hand, several findings suggest that tasks demanding many attentional resources may result in higher levels of presence in VR and maybe even performance (Nash et al., 2000). Transferred to the public speaking context, task difficulty is constituted of several dimensions, for example, the content of the speech (e.g., giving a talk on countries visited during a vacation vs. presenting the results of a scientific study), preparation (how much time was invested in preparing and rehearsing the talk; Menzel and Carrell, 1994), presentation (reading from a script vs. talking freely), and audience characteristics [e.g., formal or casual audience members; see also Morreale et al. (2007)]. Those specific task difficulty dimensions for public speaking and their role in VR applications have not been studied to date.
Against the background of public speaking training applications, ability to concentrate on the task at hand in VR is relevant (Schuemie et al., 2001; Sacau et al., 2008). Given the relation to cognitive resource allocation for attention and concentration, ability to concentrate is a highly relevant user state for task performance, and can influence presence (Draper et al., 1998; MacEdonio et al., 2007). VR applications represent tools for diagnosis and therapy with high ecological validity and effectiveness for disorders related to attention and concentration, like attention deficit disorder (Cho et al., 2002; Anton et al., 2009) as well as memory training (Optale et al., 2010). Studies with non-clinical samples on ability to concentrate are uncommon, though.
Further, presence is one of the most researched constructs in VR applications. Besides its function in training applications (Kothgassner et al., 2012), presence is regarded to have a key role in VR therapy for anxiety disorders (Wiederhold and Wiederhold, 2005b). As already briefly defined above, presence can be described as a user’s subjective psychological response to a VR system or the sense of “being there” (Reeves, 1991; Slater, 2003). Researchers agree that presence should trigger the experience of fear and anxiety in virtual environments for phobia treatment and training (Ling et al., 2014). Inducing these emotional states is crucial for clients to confront them and train certain skills to overcome their fear (Wiederhold and Wiederhold, 1998). However, recent research revealed that the correlation between presence measures and anxiety showed mixed results and differed between phobias (Ling et al., 2014). A recent meta-analysis (Ling et al., 2014) even showed a null-effect for social anxiety [see also Felnhofer et al. (2014)]. Ling et al. (2014) argue that “one might conclude that subjective presence measures do not capture the essential sense of presence that is responsible for activating fear related to social anxiety in individuals” (p. 8f.), but rather virtual presence or place illusion (Slater, 2009). In the case of fear of public speaking as a sub-form of social anxiety, “a simulation should include virtual human behavior actions that can be used as indicators for positive or negative human evaluation” (Poeschl and Doering, 2015, p. 59). These aspects then have to be acknowledged in presence measures as well. The concept of social presence (SP) (Nowak and Biocca, 2003) meets this requirement. Youngblut (2003) defines SP as follows: “Social presence occurs when users feel that a form, behavior, or sensory experience indicates the presence of another individual. The amount of social presence is the degree to which a user feels access to the intelligence, intentions, and sensory impressions of another” (p. 4). The “other” named in the definition not only addresses other human beings, but also computer-generated agents (Youngblut, 2003). SP acknowledges personal interaction, including the sub-dimensions of co-presence (as a prerequisite), psychological involvement, and behavioral engagement (Biocca et al., 2001).
As can be seen from state of research, the quality of a VR public speaking application is a function of various factors. Given the well-established research and development as well as the increasing implementation of such public speaking applications, there is a need for integrative approaches to evaluate VR social anxiety treatments or trainings. This paper tries to take into account the factors discussed above. In order to integrate determinants that influence the quality and thereby the success of VR training applications, the “quality evaluation of user-system interaction in virtual reality” (QUEST-VR; see Section “QUEST-VR Framework”) framework was developed and validated in parts by the presented study. For example, fidelity aspects and user traits are not regarded further due to research economic reasons. Based on the framework, four factors discussed above that contribute to public speaking performance (outcome) were selected for evaluation of a VR public speaking training environment. Task difficulty (system factor) and ability to concentrate (user state) were selected, because state of research shows that these aspects affect performance in real-life, and (social) presence (moderating factor) is claimed to be a key factor in VR scenarios. State fear of public speaking (moderating factor) is a further control variable as such an environment is prone to induce this emotional state during the interaction, which lowers speech performance. Speech performance is considered the outcome variable and used as an indicator for training effectiveness and thereby the application’s quality.
The following hypotheses were derived based on the current state of research and the QUEST-VR framework:
H1: SP, fear of public speaking, and ability to concentrate correlate with speech-giving performance in VR.
H2: High task difficulty (speech-giving without preparation) leads to lower public speaking performance in VR than low task difficulty (speech-giving with preparation).
H3: SP, fear of public speaking, and ability to concentrate influence the relation between task difficulty and speech-giving performance in VR.
Materials and Methods
QUEST-VR Framework
Given the increasing application of clinical and non-clinical VR social anxiety training applications, there is a need for comprehensive evaluation approaches. The QUEST-VR1 framework was developed in order to systematically include various determinants that influence the quality and thereby the success of VR training applications.
The framework includes system and user characteristics as well as the system-user interaction and moderating factors (factors that result from the actual use situation) as determinants of a VR application’s quality (see Figure 1). The factors selected for the empirical study that validated the framework are also provided in Figure 1.
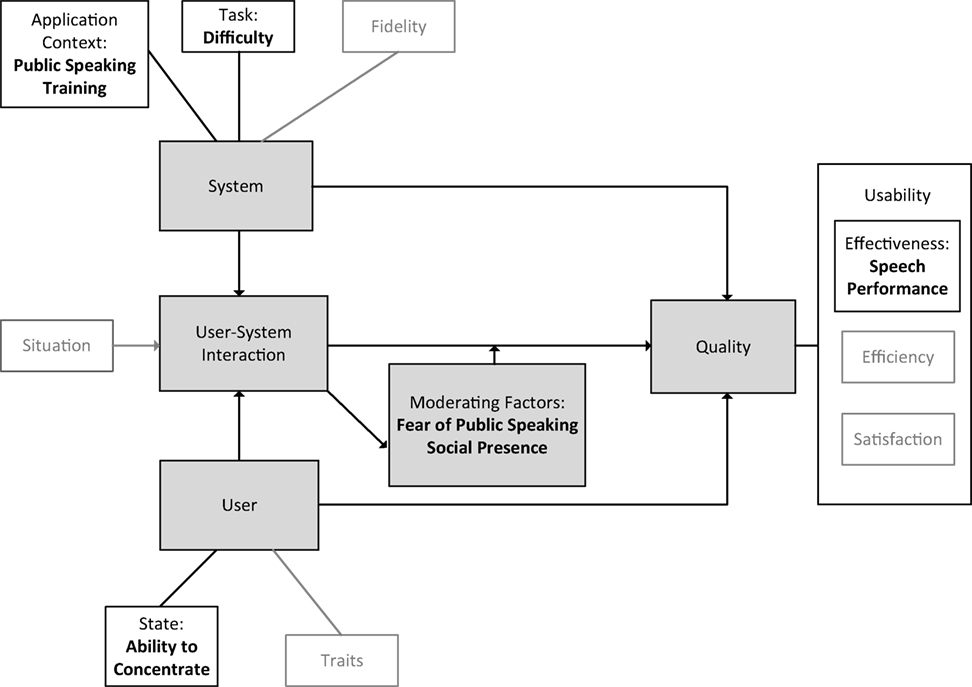
Figure 1. The QUEST-VR (quality evaluation of user-system interaction in virtual reality) framework (see text footnote 1), including variables selected for the validation study. Main concepts of the framework are highlighted in grey.
System
System features are factors that can be directly designed and manipulated. They comprise a system’s application context (Bowman et al., 2005), task characteristics (Nash et al., 2000), and the system’s fidelity (Bowman and McMahan, 2007, see Figure 1). A suitable design is a necessary requirement for satisfactory use and outcomes of a specific system, as for example, poor user interaction in VR can decrease performance (Stickel et al., 2010; McMahan et al., 2012). Therefore, these factors are usually already considered in the design process (Bowman and Hodges, 1999).
The application context determines what the specific application serves for. The specific tasks that have to be fulfilled by the users (Bowman et al., 2008) as well as the task characteristics (e.g., several levels of difficulty) are deducted from the specific context.
Fidelity or immersion is defined as “the objective level of sensory fidelity a VR system provides” (Slater, 2003). Fidelity can be further divided into display fidelity (Bowman and McMahan, 2007), interaction fidelity (McMahan et al., 2012), and simulation fidelity (Lee et al., 2013). Fidelity aspects can affect user experience (e.g., presence) as well as user performance (Nash et al., 2000).
For the validation study, public speaking training served as the application context and preparation as an aspect of task difficulty (see Figure 1). All participants trained in the same virtual environment that consisted of a virtual audience only (without prompting for example), in order to investigate this aspect of task difficulty without further influences.
User
The user component (see Figure 1) covers biological, physical, psychological, and social characteristics of users and is based on the human factors definitions by Chapanis (1991) and Stramler (1993). It includes human capabilities as well as human limitations that are relevant for safe, comfortable, and effective design, operation, or use of products or systems. These variables can be further categorized as traits (enduring personal qualities or attributes that influence behavior across situations) and states (temporary internal characteristics; Chaplin et al., 1988).
Relevant user traits are adaptability, prior experience with VR, susceptibility to immersion, and socio-demographic variables like gender or age (Nash et al., 2000; Youngblut, 2003). Several states have been researched in relation to VR applications: relevant are, for example, motivation to interact with VR, attention resources, and identification with an avatar [for an overview, see Nash et al. (2000) and Youngblut (2003)].
For the validation study, ability to concentrate (see Figure 1) on the task at hand in VR was chosen as a user state.
User-System Interaction
The third component of the QUEST-VR framework is the user-system interaction (see Figure 1), representing the actual use of the system by a user. Within the use situation, users experience the system and, as a result, display certain behavioral actions that are related to performance. The displayed behavior is the result of the interaction between dispositional and situational variables in the specific use situation (Larsen and Buss, 2013).
Moderating Factors
The effect the user-system interaction has on quality measures of a VR application can be influenced by moderating or mediating factors. These factors directly result from the interaction. User-system interaction can lead to “side effects,” which can be intended (e.g., presence) or not (e.g., cyber-sickness). Those effects play either a moderating or a mediating role and influence the effects of user-system interaction on the quality measures (see Figure 1).
In this study, state fear of public speaking and SP were analyzed as moderating factors that resulted directly from the use situation.
Quality
The quality of a VR application represents the outcome in the QUEST-VR framework (see Figure 1). Quality is defined by the International Organization for Standardization (ISO) as the “degree to which a set of inherent characteristics fulfills requirements” (International Organization for Standardization, 2015). The degree represents the level to which a product or service satisfies, which can be deemed, for example, as good or poor quality of a product. For VR applications, this can be broken down into further aspects of quality that are also known from a usability context (effectiveness, efficiency, and satisfaction; ISO 9241-11, Part 11; International Organization for Standardization, 1998). A VR training system shows high quality when the expected purpose of the application is fulfilled (increase in performance, see Figure 1) with as few resources as possible, and when system usage is satisfying for the users. In the validation study, public speaking performance as a measure of training effectiveness was selected as outcome variable.
Research Design
A cross-sectional repeated measures laboratory study was conducted. Task difficulty (low vs. high, within-subject factor) constituted the independent variable. A within-subject design was chosen in order to reduce participant based error variance and, therefore, to increase test power. Observed speech performance behavior served as the dependent variable; SP, state fear of public speaking, and ability to concentrate were acknowledged as control variables. The study was designed, implemented, and conducted according to the guidelines of the APA research ethics committee.
Low task difficulty (first exposure) was implemented as speech-giving with preparation: participants received an article about the town where the study took place and the participants lived. The article was based on the respective Wikipedia article. The material handed out to participants is provided as Supplementary Material. They were given 10 min to prepare a short speech about the town based on this article and were allowed to take notes that they could also use during the speech. They then delivered a speech of a maximum of 5 min.
High task difficulty (second exposure) was constituted as speech-giving without preparation: directly after the first task, participants were asked to deliver a speech of again 5 min about their hometown. Subjects received a guideline consisting of bullet points comparable to the content of the article for the first task, which is also provided as Supplementary Material. The task had to be fulfilled immediately without further preparation or notes.
In order to control for variability of prior special knowledge about the residential town (low difficulty) and hometown (high difficulty) as best as possible, the article as well as the bullet points covered a wide range of information (geography and demographics, schools and institutions, history, tourism and sights, and museums).
Sequence of tasks was not counter-balanced, because fulfilling the hard task before the easy task would have made the low task difficulty condition even easier due to practice effects. However, this means that learning effects from the easy task condition to the hard task condition are possible. Therefore, further statistical differentiation of these effects from task difficulty effects is needed (see Section “Results”).
Participants
An ad hoc sample with a total of N = 37 undergraduate students at a mid-sized university in Germany were recruited by personal invitations, email, and a Facebook fan page. One participant was excluded due to a damaged video recording. The final sample consisted of N = 36 participants [36% men, 64% women, mean age = 26.42 years (SD = 3.42)].
Measures
The participants’ speech-giving performance was video-recorded and rated by four independent coders. The speech evaluation form (Lucas, 2016), which is a standardized behavioral observation system, was used to rate speech behavior. The coders were trained in using the system to ensure sufficient reliability. Due to the study design, only the following categories that could be referred to speech-giving with a predefined topic and the use of prepared materials were used (three-point observation rating scale from 1 = poor to 3 = excellent performance, mean Spearman’s ρ = 0.76). Introduction of the speech was rated by clear introduction of the topic, if credibility was established and if the body of the speech was previewed. For the body of the speech, making points clear and accurate and clear language was evaluated. For the delivery, maintaining of eye contact was rated as well as if enthusiasm for the topic was communicated. Rating of the conclusion comprised of preparation of the audience for the ending and if the central idea was reinforced. For overall evaluation the coders rated if the topic was challenging and narrowed and if the speech met the assignment. The coders rated every of the 13 categories per exposure, using a single close-up video of the participants delivering the speech for each condition respectively. The videos were distributed to the coders, therefore one video was rated by a single coder. For the general speech performance score, the means of the 13 ratings was calculated.
Social presence was measured by the questionnaire developed by Poeschl and Doering (2015), because it specifically covers presence aspects in virtual public speaking environments. The questionnaire consists of four five-point Likert scales (from 1 = strongly disagree to 5 = strongly agree) on sub-dimensions of SP (see Table 1).
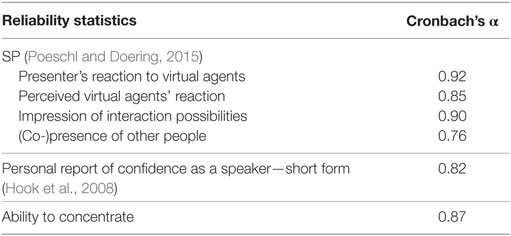
Table 1. Reliability statistics for social presence (SP) subscales, fear of public speaking, and ability to concentrate questionnaires.
State fear of public speaking was measured by an adapted short form of the Personal Report of Confidence as a Speaker (Hook et al., 2008). Questions are answered in a true-false format (with the scores ranging from 0 = no fear of public speaking to 12 = highest level of fear of public speaking). Items were adapted to speech-giving in a VR environment.
For measuring ability to concentrate in VR, a 10-item questionnaire (six-point Likert scale from 1 = not at all to 6 = very much) was developed, based on the Wender Utah Rating Scale (Ward et al., 1993), and adapted to a virtual public speaking scenario.
Reliability statistics of the measures are provided in Table 1.
Study Environment
The hardware setup for the study consisted of a workstation that provided the virtual environment (VE). The VE was created on a DELL Workstation with an Intel(R) Xeon(R) CPU X5650 @ 2.67 GHz, 12 GB of RAM, and a NVIDIA GeForce GTX 560 graphics card with 2 GB of RAM. The stereoscopic visualization was displayed with rear projection on screen (2,800 mm × 2,100 mm) by two DLP projectors with a native SXGA + (1,400 × 1,050) resolution. The incorporated software setup was based on the CryEngine3 (Version PC v3.4.0 3696 freeSDK) as a 3D engine for real time rendering. The screen setup is presented in Figure 2. The visualization was projected on the middle screen.
The application was a prototype at the time of the study with a basic visualization of audience behavior. The virtual scene (5 min’ length) was seen from a first person perspective and consisted of a male audience with eight members sitting in a lecture room (see Figure 3). The agents showed random behavior like leaning forward or talking to each other. Due to research economic reasons, no real interaction with the presenter was implemented (e.g., audience reactions to a boring style of presentation). A video of the visualization is provided as Supplementary Material. Further, audience behavior was specifically designed as neutral behavior (neither explicitly positive nor negative; Slater et al., 2006) and treated as a constant. For the same reason, no head tracking was implemented (rendering was not updated to the participants’ eye point). Instead, participants were asked to stand in a sweet spot for the stereoscopic visualization.
Procedure
The experiment took place in June 2015. After an oral briefing, subjects completed a questionnaire on socio-demographic data. Afterward, they completed the two tasks. Subsequently, participants filled out questionnaires on SP, fear of public speaking, and ability to concentrate, before being debriefed. As the tasks were fulfilled in direct succession, the covariates were measured with regard to the whole experience in VR. This also served to keep strain on participants to a minimum, because it reduced the time for their participation in the study. In accordance to prior studies (see “Introduction”), no training session in front of the virtual audience was conducted and participants had no prior experience with the system. This prevented unplanned habituation effects which would have had an influence on task difficulty.
Results
In general, participants performed rather well in the low (first exposure) as well as the high task difficulty condition (second exposure). However, mean performance showed higher descriptive statistics for the low difficulty condition (see Figure 4). Fear of public speaking was also from low to medium, however, it showed a rather wide range (see Figure 5).
Social presence was medium for all sub-constructs, although co-presence of other people was a bit higher than the other dimensions (see Figure 6).
Finally, ability to concentrate in VR was rather low (see Figure 7), maybe due to the novel experience of using a VR public speaking system, and participants were rather excited about the study.
As a first step for hypothesis testing, it was examined if the covariates (SP, fear of public speaking, and ability to concentrate) correlate with speech-giving performance in VR (Hypothesis 1). Bivariate Pearson correlations were computed (see Table 2), while all requirements for the data analysis were met.
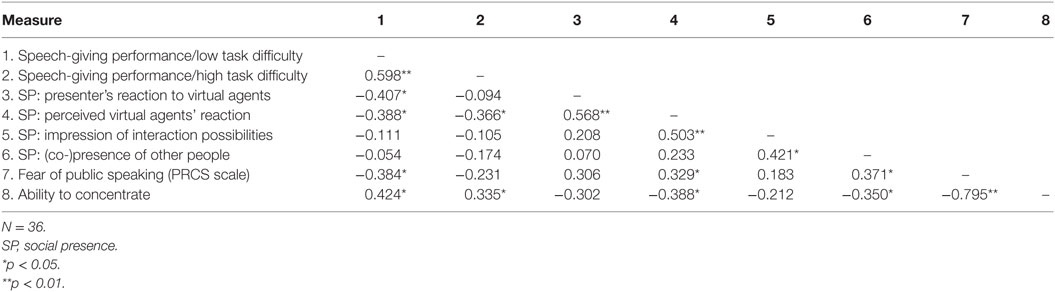
Table 2. Intercorrelations between speech-giving performance for low and high task difficulty (experimental condition), SP dimensions, fear of public speaking, and ability to concentrate.
As Table 2 shows, SP dimensions do not correlate in general with speech-giving performance. Further, they show a tendency to correlate negatively. This is in line with state of research that states that especially for unfamiliar tasks, presence can have a negative effect on performance (Nash et al., 2000). Speaking in front of a virtual audience could have been a novelty for participants.
Further, low and high task difficulty (first vs. second exposure) showed different patterns: for the low difficulty condition, only the presenter’s reaction to the virtual agents as well as their reactions as perceived by the presenter revealed significant correlations, with medium effect sizes. For the high difficulty condition, only the perceived virtual agents’ reaction presented a medium effect (see Table 2). It seems that in the easy condition, where participants could prepare the speech, they might have had enough cognitive resources left to acknowledge their own reactions toward the audience. For both conditions, subjects seem to consider if the audience reacted toward them. This is also in line with previous research, because part of a public speaking scenario is anticipated human evaluation by the audience (Ling et al., 2014). The impression of interaction possibilities as well as co-presence only show small and insignificant effects. Giving a frontal speech is not a very interactive task (a discussion, for example, was not simulated), therefore, the respective presence dimension might have not played an important role in the study. Concerning co-presence, it could be assumed that participants were aware that the virtual agents were not other human beings, as the agents in the visualization were clearly models. Further, maybe participants’ related co-presence to the experimenters. Those retreated during the speeches, but did not leave the room due to the quick sequence of tasks to be administered to the subjects.
In line with the theoretical background, fear of public speaking showed a significant medium negative correlation with performance for the easy task and a negative tendency for the hard task (see Table 2). The smaller effect for the hard task could be explained by the procedure sequence of the experiment, as the hard task was implemented in the second exposure: maybe the participants got used to the environment and the task. State of research shows that within CBT, fear decreases over time during an exposure (Wiederhold and Wiederhold, 2005a).
Finally, and unsurprisingly, higher ability to concentrate in VR showed positive and medium correlations with performance for both task conditions (see Table 2). In light of the complex intercorrelation patterns, Hypothesis 1 could only be partially confirmed, i.e., for the dimensions showing significant effects.
For testing Hypothesis 2, a repeated measures ANOVA was conducted (requirements for data analysis were fulfilled). Task difficulty revealed a large effect on speech-giving performance [F(1, 35) = 8.55; p = 0.006; ]; high difficulty (M = 2.13, SD = 0.41) resulted in lower speech performance scores than low difficulty (M = 2.30, SD = 0.35). However, learning effects from the low difficulty condition (first exposure) could be possible. Still, a learning effect would probably lead to better speech performance in the second (high difficulty) condition. Therefore, the effect of task difficulty could be even larger. Although this seems to still support Hypothesis 2, a final statement concerning task difficulty effects cannot be derived due to the chosen study design.
In order to test Hypothesis 3, an ANCOVA with the SP dimensions that showed significant correlations with speech performance, fear of public speaking, and ability to concentrate, was conducted (Table 3). Requirements for data analysis were fulfilled.
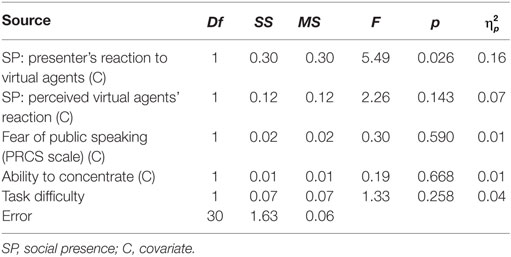
Table 3. Analysis of covariance of public speaking performance as a function of task difficulty with SP dimensions, fear of public speaking, and ability to concentrate as covariates.
The introduction of the covariates decreased the effect of task difficulty from small to medium, and it turned insignificant. There seems to be an overlap between task difficulty and SP for explained variance for performance (see Table 3). The presenter’s reaction to the virtual agents in the application seems to be especially relevant (it shows a medium effect size). This SP factor is constituted of items stating that the audience behavior influenced the presenters’ style of presentation and had an influence on their mood, as well as that the presenters reacted to the people in the audience, and that they got distracted by them (Poeschl and Doering, 2015). Reacting to the virtual audience could have afforded cognitive resources that could not at the same time be allocated to the task at hand. Therefore, this could have decreased performance.
However, due to the non-randomized presentation of the tasks (the low difficulty task in the first exposure, the high difficulty task in the second exposure), learning effects cannot be ruled out. Further, the covariates showed complex correlation patterns with speech performance (see Table 2). Therefore, conclusions on Hypothesis 3 cannot be drawn on the basis of this study. Although the ANCOVA revealed an overlap of explained variance of the covariates and task difficulty, there is no indication whether this overlap is partialed out from explained variance of either the low or high task difficulty condition.
In order to gain more insight into possible influences of SP, fear of public speaking, and ability to concentrate on the difference of speech performance between the task difficulty conditions, the data were further analyzed in an explorative way.
Due to the possibility of practice effects in the given design, the difference between the two conditions is of interest, when the effect of the low task difficulty condition is partialed out of the high task difficulty condition. A new dependent variable was calculated from the standardized residuals that were determined by means of a linear regression analysis of the speech performance in the low difficulty condition (predictor) on the speech performance in the high difficulty condition (criterion). This ensured that the new dependent variable represented the difference in speech performance between the task conditions that is independent of the performance in the easy task [for this procedure, see also Schumann and Schultheiss (2009)].
A linear multiple regression analysis was conducted to predict the residual performance difference (criterion) based on presenter’s reaction to virtual agents, perceived virtual agents’ reaction (both SP dimensions), fear of public speaking, and ability to concentrate (predictors). The requirements for this analysis were met. The descriptive statistics for the regression analysis are presented in Table 4, and the summary of the regression analysis in Table 5.
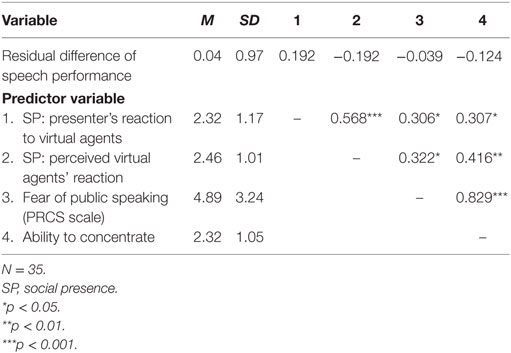
Table 4. Means, SDs, and intercorrelations for residual difference of speech performance between task difficulty conditions and SP, fear of public speaking, and ability to concentrate predictor variables.
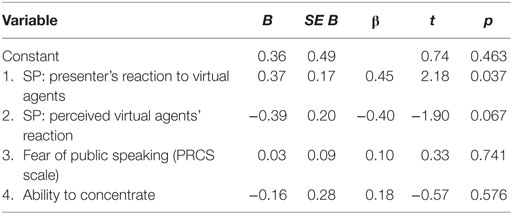
Table 5. Regression analysis summary for social presence (SP), fear of public speaking, and ability to concentrate predicting residual difference of speech performance between task difficulty conditions.
A non-significant regression equation was found [F(4, 30) = 1.65, p = 0.187], with an R2 of 0.181, which represents a medium effect. However, test power was too low (1 − β = 0.51) due to the small sample size. Still, the presenter’s reaction to virtual agents was a significant predictor of residual difference of speech performance between task difficulty conditions. This analysis shows a similar pattern as the ANCOVA results. It seems that only the presenter’s reaction to virtual agents as a SP dimensions explains variance of speech performance after controlling for learning effects due to the prior speech performance in the easy task. The other covariates do not contribute significantly to the regression equation. However, this result can only be interpreted as a tendency due to the non-significant effect of the whole regression model.
Discussion
Although VR training applications and VR public speaking applications in particular are a successful asset, comprehensive studies that analyze what determinants contribute to this success are still scarce today. This study introduces the QUEST-VR framework that can be used as a heuristic tool to evaluate interactive VR setups. The framework includes system and user characteristics as well as the system-user interaction and moderating factors as determinants of a VR application’s quality. A first partial validation of the framework was implemented by evaluating the quality of a VR public speaking training application. A within-subject laboratory study was conducted. The influence of task difficulty (system factor), ability to concentrate (user state), state fear of public speaking, and SP (moderating factors) on public speaking performance in VR was analyzed.
Concerning Hypothesis 1, intercorrelations of ability to concentrate, fear of public speaking, and SP with speech-giving performance in VR were examined. They revealed complex patterns: in line with state of research, ability to concentrate showed positive and medium correlations with speech-giving performance independently for task difficulty conditions.
However, task difficulty showed different patterns for the other covariates. Fear of public speaking correlated negatively with performance and with a medium effect for the easy task, but for the hard task only showed a negative tendency. However, this can be explained with a sequence effect, as the hard task followed the easy task and fear decreases over time (Wiederhold and Wiederhold, 2005a). Controlling for a sequence effect was not feasible as it would have made the easy task even easier if it had followed the hard task due to practice effects.
Social presence dimensions also revealed interesting patterns. Impression of interaction possibilities and co-presence as SP dimensions showed very small and insignificant effect sizes. Participants were probably fully aware that the audience did not consist of real people and the VR application was not interactive (for example, no questions and answers were implemented). Still, SP dimensions reflecting reactions of the presenters toward the audience and vice versa appear to be relevant: for the easy as well as the hard task, subjects seemed to consider how the audience reacted toward them with medium effect sizes. This can be explained by the fact that anticipated human evaluation is an integral part of public speaking tasks (Ling et al., 2014). For the low difficulty condition, the presenters’ reaction to the audience correlated with speech performance, again with a medium effect. Participants had the opportunity to prepare the speech and might, therefore, have had enough cognitive resources left to notice their own reactions. In general, SP dimensions showed negative correlations with speech performance, which is common with unfamiliar tasks (Nash et al., 2000). Using a new VR training environment probably presented a novelty for the participants.
Therefore, the findings supported Hypothesis 1 only for ability to concentrate in VR and the SP dimension that covers how presenters experience the audience’s reaction toward them. The complex pattern of correlations hints at underlying interrelations that are just as complex and highlight the need for comprehensive approaches when evaluating VR applications. However, as the tasks were administered directly after another without a break, ability to concentrate, fear of public speaking, and SP were measured with regard to the whole experience. Measuring the covariates after each task (directly related to the task difficulty condition) could show different effects. Therefore, generalizability of the effects is clearly limited. The findings should be replicated with a different research design.
In the next step, the effect of task difficulty on speech-giving performance in VR was examined. Unsurprisingly, high task difficulty led to lower performance than low task difficulty with a large effect. However, as the sequence of tasks was not randomized, a learning effect between the tasks cannot be ruled out. It should lead to better speech performance in the second (high difficulty) condition. Therefore, the effect of task difficulty could be even larger. Due to the study design, a final statement concerning Hypothesis 2 cannot be given. However, including ability to concentrate, fear of public speaking, and SP dimensions (these that correlated with performance) as covariates in the analysis led to a reduction of the effect of task difficulty, considerably reducing its effect size to a small to medium effect. The presenter’s reaction to the virtual agents in the application (as SP dimension) seems to have a significant contribution, showing a medium effect size. This factor shows an overlap of explained variance by task difficulty on performance. The SP factor concerned covers that speakers feel that the audience influences their mood, style of presentation and even distracts them (Poeschl and Doering, 2015). These reactions could have blocked cognitive resources that would have otherwise been allocated to the task and then led to higher performance.
Still, due to possible sequence effects and the complex intercorrelations of covariates with the speech performance, Hypothesis 3 cannot be confirmed on the basis of this study. In order to gain more insight into the interrelations, further exploratory analyses were conducted. Possible learning effects between the task difficulty conditions were taken into account. A new dependent variable was calculated from the standardized residuals that were determined by means of a linear regression analysis of the speech performance in the low difficulty condition on the speech performance in the high difficulty condition. This variable represented the difference in speech performance between the task conditions that is independent of the performance in the easy task. A linear multiple regression analysis was conducted to predict the residual performance difference based on the covariates included in the ANCOVA. Although the presenter’s reaction to virtual agents (SP dimension) was a significant predictor (showing a similar effect as obtained in the ANCOVA), the regression model was insignificant. However, variance explained of the regression model revealed a medium effect. The test power was too low, possibly because of the small sample size. Therefore, the impact of this specific SP dimension of speech performance can only be interpreted as a tendency.
The hypotheses in this study were only supported in parts. The interplay of determinants that was shown in the results should be explored more thoroughly. Especially the effects of the covariates on performance and their respective interrelations should be analyzed in future studies with more rigorous designs. This will help to gain a better understanding on causal relationships on what exactly determines an application’s quality and thereby its success.
This study has several limitations. First, the sample consisted of undergraduate students with a majority of women. Although the VR training application targets students, other target groups (e.g., lecturers, politicians, and business people) would also be possible. However, the findings cannot be generalized toward these groups or even other use cases like job interviews.
Second, several design aspects should be improved in future studies. The covariates were measured with regard to the whole experience of delivering speeches in VR and not for the single task conditions, respectively, as the tasks were fulfilled in direct succession. This had the benefit of reducing strain on participants by keeping the time for their participation in the study short. However, measuring the covariates explicitly for each condition could lead to different and more reliable results. Also, sequence effects of the task conditions could not be controlled: fulfilling the hard task before the easy task could have led to practice effects and, therefore, further lowered difficulty for the easy task. Still, future studies should use designs that avoid entanglement of task difficulty and sequence. However, the dependent variable speech performance was measured by means of a behavior observation system. Therefore, the study combined objective and subjective measures.
Third, the application is still a prototype. It only included male virtual agents and a very limited number of displayed non-verbal behavior actions. Further, no real interaction between presenters and the audience (like questions and answers) and no head tracking was implemented. Therefore, realism of the scenario was clearly limited. This could lead to lower presence experienced and higher performance scores, if participants did not take the audience seriously. A more realistic visualization (e.g., based on video data of audience members) should reveal different and maybe larger effects. Additionally, the prototype did not include a self-avatar. Recent research shows that including self-avatars in VR could be very beneficial for public speaking training systems. First, gesturing seems to lighten cognitive load in explanation tasks (Goldin-Meadow et al., 2001), which are very similar to public speaking. Loading off mental tasks by gestures during speaking tasks, therefore, has an impact on task difficulty. A recent study showed that implementing an active self-avatar and allowing gestures in VR during a recall task significantly increased performance compared to no self-avatar and no gestures allowed (Steed et al., 2016). Second, people with communication anxiety might prefer to “become someone else” in a public speaking situation. Aymerich-Franch et al. (2014) could show on the one hand that social anxiety correlated significantly with a preference for embodying a dissimilar avatar in VR. On the other hand, participants with an assigned self-avatar experienced more self-presence and higher levels of anxiety. In order to reduce anxiety, which can represent an important inhibition threshold for training sessions in VR, clients could be offered a choice of avatars for initial training, including dissimilar avatars. In further sessions, the self-avatar could be gradually adapted to a realistic self-avatar with models based on photographs of the participants’ faces (Aymerich-Franch et al., 2014). In this way, self-presence could be gradually increased while being matched to the clients’ training progress and their decrease in anxiety until conditions similar to real public speaking scenarios are reached. This procedure would be comparable to confronting increasingly frightening stimuli in conventional CBT.
Lastly, only a small selection of determinants could be acknowledged in the present study due to research economic reasons. For example, different technological setups (head mounted display, desktop, and projection screens) were not compared in the study. It would be interesting to learn if this would lead to the same effects and if a specific setup would prove to be the most efficient. Further, other user factors like prior experience with VR or motivation to interact with VR could have an impact on performance and could not be considered in this study. Last but not least, only a single training session (containing two tasks) was conducted. Training programs usually consist of several sessions and task difficulty can be adapted to the trainee’s progress. Evaluating the application’s quality in a whole training program still needs to be done.
However, using the QUEST-VR framework as a tool to derive variables for evaluation of a VR public speaking training application proved feasible and fruitful. The comprehensive analysis of system features, user factors and moderating variables on speech performance revealed interesting and complex patterns of findings that can serve as a basis for future studies. Still, the feasibility of the framework as a heuristic tool for evaluation should also be tested for VR applications with a different context, for example other phobias. Using comprehensive studies will not only increase the understanding of human-computer interaction in VR, but can also help to improve an application’s quality and successful implementation.
Ethics Statement
As the university the study was conducted at does not have an ethics board, the study was designed, implemented, and conducted according to the guidelines of the APA research ethics committee. Upon arriving at the lab, the participants were briefed orally and signed a consent form that included all the following points in writing: participants were informed that the study was voluntary and all data were collected confidentially and processed anonymously. They were informed that they could withdraw their consent on any given point in time without negative consequences. They were further informed that they would be video-recorded during the experiment, but all data would be erased at their request. No vulnerable populations were involved.
Author Contributions
SP: the author’s contributions to the paper are developing the theoretical background and state of research for the study and the paper, study design, data analysis, and interpretation for the study as well as writing and revising the manuscript. The QUEST-VR framework was developed in collaboration with Doug A. Bowman, Virginia Polytechnic Institute and State University. A publication of the framework in itself is in preparation (see text footnote 1). Doug A. Bowman has agreed that the author uses and presents the framework in this paper.
Conflict of Interest Statement
The author declares that the research was conducted in the absence of any commercial or financial relationships that could be construed as a potential conflict of interest.
Acknowledgments
The author would like to thank to Annika Mann and Darja Schuetz for their help with the preparation of the experiment and the data collection. I acknowledge support for the Article Processing Charge by the German Research Foundation and the Open Access Publication Fund of the Technische Universität Ilmenau.
Funding
The research described in the paper was not funded.
Supplementary Material
The Supplementary Material for this article can be found online at http://journal.frontiersin.org/article/10.3389/fict.2017.00013/full#supplementary-material.
Video. Visualization of the training application.
Data Sheet. Material handed out to participants.
Footnote
- ^The QUEST-VR framework was developed in collaboration with Doug A. Bowman, Virginia Polytechnic Institute and State University. A publication of the framework in itself is in preparation [Poeschl, S., Bowman, D. A., and Doering, N. Determining quality for virtual reality application design and evaluation – a survey on the role of application areas (in preparation)].
References
Anton, R., Opris, D., Dobrean, A., David, D., and Rizzo, A. (2009). “Virtual reality in rehabilitation of attention deficit/hyperactivity disorder. The instrument construction principles,” in Proceedings of the 2009 Virtual Rehabilitation International Conference, Haifa.
Aymerich-Franch, L., Kizilcec, R. F., and Bailenson, J. N. (2014). The relationship between virtual self similarity and social anxiety. Front. Hum. Neurosci. 8:944. doi: 10.3389/fnhum.2014.00944
Biocca, F. A., Harms, C., and Gregg, J. (2001). “The networked minds measure of social presence: pilot test of the factor structure and concurrent validity,” in Proceedings of the 4th Annual International Workshop on Presence, (Valencia: International Society for Presence Research).
Bowman, D. A., Coquillart, S., Fröhlich, B., Hirose, M., Kitamura, Y., Kiyokawa, K., et al. (2008). 3D user interfaces: new directions and perspectives. IEEE Comput. Graph. Appl. 28, 20–36. doi:10.1109/MCG.2008.109
Bowman, D. A., and Hodges, L. F. (1999). Formalizing the design, evaluation, and application of interaction techniques for immersive virtual environments. J. Vis. Lang. Comput. 10, 37–53. doi:10.1006/jvlc.1998.0111
Bowman, D. A., Kruijff, E., LaViola, J. J., and Poupyrev, I. (2005). 3D User Interfaces: Theory and Practice. Boston: Addison-Wesley Professional.
Bowman, D. A., and McMahan, R. P. (2007). Virtual reality: how much immersion is enough? Computer 40, 36–43. doi:10.1109/MC.2007.257
Chapanis, A. (1991). To communicate the human factors message, you have to know what the message is and how to communicate it. Hum. Factors Soc. Bull. 34, 1–4.
Chaplin, W. F., John, O. P., and Goldberg, L. R. (1988). Conceptions of states and traits: dimensional attributes with ideals as prototypes. J. Pers. Soc. Psychol. 54, 541–557. doi:10.1037/0022-3514.54.4.541
Cho, B. H., Lee, J. M., Ku, J. H., Jang, D. P., Kim, J. S., Kim, I. Y., et al. (2002). “Attention enhancement system using virtual reality and EEG biofeedback,” in Proceedings of the 2002 Virtual Reality Conference, (Orlando, FL).
Chollet, M., Wörtwein, T., Morency, L.-P., Shapiro, A., and Scherer, S. (2015). “Exploring feedback strategies to improve public speaking,” in The 2015 ACM International Joint Conference, eds K. Mase, M. Langheinrich, D. Gatica-Perez, H. Gellersen, T. Choudhury, and K. Yatani, (Osaka), 1143–1154.
Draper, V. D., Kaber, D. B., and Usher, J. M. (1998). Telepresence. Hum. Factors 40, 354–375. doi:10.1518/001872098779591386
Endler, N. S., and Kocovski, N. L. (2001). State and trait anxiety revisited. J. Anxiety Disord. 15, 231–245. doi:10.1016/S0887-6185(01)00060-3
Felnhofer, A., Kothgassner, O. D., Hetterle, T., Beutl, L., Hlavacs, H., and Kryspin-Exner, I. (2014). Afraid to be there? Evaluating the relation between presence, self-reported anxiety, and heart rate in a virtual public speaking task: cyberpsychology, behavior, and social networking. Cyberpsychol. Behav. Soc. Netw. 17, 310–316. doi:10.1089/cyber.2013.0472
Goldin-Meadow, S., Nusbaum, H., Kelly, S. D., and Wagner, S. (2001). Explaining math: gesturing lightens the load. Psychol. Sci. 12, 516–522. doi:10.1111/1467-9280.00395
Hook, J. N., Smith, C. A., and Valentiner, D. P. (2008). A short-form of the personal report of confidence as a speaker. Pers. Individ. Dif. 44, 1306–1313. doi:10.1016/j.paid.2007.11.021
International Organization for Standardization. (1998). Ergonomic Requirements for Office Work with Visual Display Terminals (VDTs) – Part 11: Guidance on Usability 13.180; 35.180. ISO 9241-11:1998. International Organization for Standardization. Available at: http://www.iso.org/iso/home/store/catalogue_tc/catalogue_detail.htm?csnumber=16883
International Organization for Standardization. (2015). Quality Management Systems – Fundamentals and Vocabulary 9000:2015. EN ISO 9000:2015-11. Berlin: Beuth Verlag.
Kothgassner, O. D., Felnhofer, A., Beutl, L., Hlavacs, H., Lehenbauer, M., and Stetina, B. (2012). A virtual training tool for giving talks. Lect. Notes Comput. Sci. 7522, 53–66. doi:10.1007/978-3-642-33542-6_5
Larsen, R. J., and Buss, D. M. (2013). Personality Psychology: Domains of Knowledge about Human Nature. Maidenhead: McGraw Hill.
Lee, C., Rincon, G. A., Meyer, G., Hoellerer, T., and Bowman, D. A. (2013). The effects of visual realism on search tasks in mixed reality simulation. IEEE Trans. Vis. Comput. Graph 19, 547–556. doi:10.1109/tvcg.2013.41
Lee, J. M., Ku, J., Jang, D. P., Kim, D. H., Choi, Y. H., Kim, I. Y., et al. (2002). Virtual reality system for treatment of the fear of public speaking using image-based rendering and moving pictures: cyberpsychology & behavior. Cyberpsychol. Behav. 5, 191–195. doi:10.1089/109493102760147169
Ling, Y., Nefs, H. T., Morina, N., Heynderickx, I., and Brinkman, W.-P. (2014). A meta-analysis on the relationship between self-reported presence and anxiety in virtual reality exposure therapy for anxiety disorders. PLoS ONE 9:e96144. doi:10.1371/journal.pone.0096144
Lucas, S. E. (2016). Speech Evaluation Form. Available at: http://highered.mheducation.com/sites/007313564x/student_view0/speech_evaluation_forms.html
MacEdonio, M. F., Parsons, T. D., Digiuseppe, R. A., Weiderhold, B. K., and Rizzo, A. A. (2007). Immersiveness and physiological arousal within panoramic video-based virtual reality. Cyberpsychol. Behav. 10, 508–515. doi:10.1089/cpb.2007.9997
McMahan, R. P., Bowman, D. A., Zielinski, D. J., and Brady, R. B. (2012). Evaluating display fidelity and interaction fidelity in a virtual reality game. IEEE Trans. Vis. Comput. Graph 18, 626–633. doi:10.1109/tvcg.2012.43
Menzel, K. E., and Carrell, L. J. (1994). The relationship between preparation and performance in public speaking. Commun. Educ. 43, 17–26. doi:10.1080/03634529409378958
Morreale, S., Moore, M., Surges-Tatum, D., and Webster, L. (2007). The Competent Speaker Speech Evaluation Form. Available at: http://www.une.edu/sites/default/files/Public-Speaking2013.pdf
Nash, E. B., Edwards, G. W., Thompson, J. A., and Barfield, W. (2000). A review of presence and performance in virtual environments. Int. J. Hum. Comput. Interact. 12, 1–41. doi:10.1207/S15327590IJHC1201_1
Neguţ, A., Matu, S.-A., Sava, F. A., and David, D. (2016). Task difficulty of virtual reality-based assessment tools compared to classical paper-and-pencil or computerized measures: a meta-analytic approach. Comput. Human Behav. 54, 414–424. doi:10.1016/j.chb.2015.08.029
Nijholt, A. (2014). Breaking fresh ground in human-media interaction research. Front. ICT 1:41. doi:10.3389/fict.2014.00004
Nowak, K. L., and Biocca, F. A. (2003). The effect of the agency and anthropomorphism on users’ sense of telepresence, copresence, and social presence in virtual environments. Presence 12, 481–494. doi:10.1162/105474603322761289
Opris, D., Pintea, S., Garcia-Palacios, A., Botella, C., Szamoskozi, S., and David, D. (2012). Virtual reality exposure therapy in anxiety disorders: a quantitative meta-analysis. Depress. Anxiety 29, 85–93. doi:10.1002/da.20910
Optale, G., Urgesi, C., Busato, V., Marin, S., Piron, L., Priftis, K., et al. (2010). Controlling memory impairment in elderly adults using virtual reality memory training: a randomized controlled pilot study. Neurorehabil. Neural Repair 24, 348–357. doi:10.1177/1545968309353328
Parsons, T. D., and Rizzo, A. A. (2008). Affective outcomes of virtual reality exposure therapy for anxiety and specific phobias: a meta-analysis. J. Behav. Ther. Exp. Psychiatry 39, 250–261. doi:10.1016/j.jbtep.2007.07.007
Pertaub, D.-P., Slater, M., and Barker, C. (2002). An experiment on public speaking anxiety in response to three different types of virtual audience. Presence 11, 68–78. doi:10.1162/105474602317343668
Poeschl, S., and Doering, N. (2015). Measuring co-presence and social presence in virtual environments – psychometric construction of a german scale for a fear of public speaking scenario. Ann. Rev. Cyberther. Telemed. 13, 58–63. doi:10.3233/978-1-61499-595-1-58
Powers, M. B., and Emmelkamp, P. M. G. (2008). Virtual reality exposure therapy for anxiety disorders: a meta-analysis. J. Anxiety Disord. 22, 561–569. doi:10.1016/j.janxdis.2007.04.006
Reeves, B. R. (1991). “Being There”: Television as Symbolic Versus Natural Experience. Standford, CA: Stanford University Institute for Communication Research.
Riley, J. M. (2001). The Utility of Measures of Attention and Spatial Awareness for Quantifying Telepresence [Ph.D. Dissertation]. Mississippi: Missisippi State University.
Rothwell, J. D. (2004). In the Company of Others: An Introduction to Communication. New York: McGraw Hill.
Sacau, A., Laarni, J., and Hartmann, T. (2008). Influence of individual factors on presence. Comput. Human Behav. 24, 2255–2273. doi:10.1016/j.chb.2007.11.001
Schuemie, M. J., van der Straaten, P., Krijn, M., and van der Mast, C. A. (2001). Research on presence in virtual reality: a survey. Cyberpsychol. Behav. 4, 183–201. doi:10.1089/109493101300117884
Schumann, C., and Schultheiss, D. (2009). Power and nerves of steel or thrill of adventure and patience? An empirical study on the use of different video game genres. J. Gaming Virtual Worlds 1, 39–56. doi:10.1386/jgvw.1.1.39_1
Sheridan, T. B. (1992). Musings on telepresence and virtual presence. Presence 1, 120–126. doi:10.1162/pres.1992.1.1.120
Slater, M. (2009). Place illusion and plausibility can lead to realistic behaviour in immersive virtual environments. Philos. Trans. R. Soc. Lond. B Biol. Sci. 364, 3549–3557. doi:10.1098/rstb.2009.0138
Slater, M., Pertaub, D.-P., Barker, C., and Clark, D. M. (2006). An experimental study on fear of public speaking using a virtual environment. Cyberpsychol. Behav. 9, 627–633. doi:10.1089/cpb.2006.9.627
Slater, M., Steed, A., McCarthy, J., and Maringelli, F. (1998). The influence of body movements on presence in virtual environments. Hum. Factors 40, 469–477. doi:10.1518/001872098779591368
Steed, A., Pan, Y., Zisch, F., and Steptoe, W. (2016). “The impact of a self-avatar on cognitive load in immersive virtual reality,” in 2016 IEEE Virtual Reality (VR), (Greenville, SC), 67–76.
Stickel, C., Ebner, M., and Holzinger, A. (2010). “The XAOS metric – understanding visual complexity as measure of usability,” in HCI in Work and Learning, Life and Leisure, eds G. Leitner, M. Hitz, and A. Holzinger (Berlin, Heidelberg: Springer), 278–290.
Sveistrup, H. (2004). Motor rehabilitation using virtual reality. J. Neuroeng. Rehabil. 1, 1–8. doi:10.1186/1743-0003-1-10
Wallach, H. S., Safir, M. P., and Bar-Zvi, M. (2009). Virtual reality cognitive behavior therapy for public speaking anxiety: a randomized clinical trial. Behav. Modif. 33, 314–338. doi:10.1177/0145445509331926
Ward, M. F., Wender, P. H., and Reimherr, F. W. (1993). The Wender Utah Rating Scale: an aid in the retrospective diagnosis of childhood attention deficit hyperactivity disorder. Am. J. Psychiatry 150, 885–890.
Wiederhold, B. K., and Wiederhold, M. D. (eds) (2005b). Virtual Reality Therapy for Anxiety Disorders: Advances in Evaluation and Treatment. Washington, DC: American Psychological Association.
Wiederhold, B. K., and Wiederhold, M. D. (1998). A review of virtual reality as a psychotherapeutic tool. Cyberpsychol. Behav. 1, 45–52. doi:10.1089/cpb.1998.1.45
Wiederhold, B. K., and Wiederhold, M. D. (2005a). “Anxiety disorders and their treatment,” in Virtual Reality Therapy for Anxiety Disorders: Advances in Evaluation and Treatment, eds B. K. Wiederhold and M. D. Wiederhold (Washington, DC: American Psychological Association), 31–45.
Youngblut, C. (2003). Experience of Presence in Virtual Environments. Alexandria, VA. Available at: www.dtic.mil/cgi-bin/GetTRDoc?AD=ADA427495
Keywords: virtual reality, training, task difficulty, social presence, ability to concentrate, fear of public speaking, speech performance
Citation: Poeschl S (2017) Virtual Reality Training for Public Speaking—A QUEST-VR Framework Validation. Front. ICT 4:13. doi: 10.3389/fict.2017.00013
Received: 23 June 2016; Accepted: 03 May 2017;
Published: 19 June 2017
Edited by:
John Quarles, University of Texas at San Antonio, United StatesReviewed by:
Joseph Gabbard, Virginia Tech, United StatesMar Gonzalez-Franco, Microsoft Research, United States
Bruno Herbelin, École Polytechnique Fédérale de Lausanne, Switzerland
Copyright: © 2017 Poeschl. This is an open-access article distributed under the terms of the Creative Commons Attribution License (CC BY). The use, distribution or reproduction in other forums is permitted, provided the original author(s) or licensor are credited and that the original publication in this journal is cited, in accordance with accepted academic practice. No use, distribution or reproduction is permitted which does not comply with these terms.
*Correspondence: Sandra Poeschl, c2FuZHJhLnBvZXNjaGxAdHUtaWxtZW5hdS5kZQ==