- 1Centre for Robotics and Neural Systems, Faculty of Science and Engineering, Plymouth University, Plymouth, UK
- 2Lincoln Centre for Autonomous Systems, School of Computer Science, University of Lincoln, Lincoln, UK
- 3ID Lab, Department of Electronics and Information Systems, Ghent University, Ghent, Belgium
Several studies have indicated that interacting with social robots in educational contexts may lead to a greater learning than interactions with computers or virtual agents. As such, an increasing amount of social human–robot interaction research is being conducted in the learning domain, particularly with children. However, it is unclear precisely what social behavior a robot should employ in such interactions. Inspiration can be taken from human–human studies; this often leads to an assumption that the more social behavior an agent utilizes, the better the learning outcome will be. We apply a nonverbal behavior metric to a series of studies in which children are taught how to identify prime numbers by a robot with various behavioral manipulations. We find a trend, which generally agrees with the pedagogy literature, but also that overt nonverbal behavior does not account for all learning differences. We discuss the impact of novelty, child expectations, and responses to social cues to further the understanding of the relationship between robot social behavior and learning. We suggest that the combination of nonverbal behavior and social cue congruency is necessary to facilitate learning.
1. Introduction
The efficacy of robots in educational contexts has been demonstrated by several researchers when compared to not having a robot at all and when compared to other types of media, such as virtual characters (Han et al., 2005; Leyzberg et al., 2012; Tanaka and Matsuzoe, 2012; Alemi et al., 2014). One suggestion for why such differences are observed stems from the idea that humans see computers as social agents (Reeves and Nass, 1996) and that robots have increased social presence over other media as they are physically present in the world (Jung and Lee, 2004; Wainer et al., 2007). If the social behavior of an agent can be improved, then the social presence will increase and interaction outcomes should improve further (for example, through social facilitation effects (Zajonc, 1965)), but it is unclear how robot social behavior should be implemented to achieve such aims.
This has resulted in researchers exploring various aspects of robot social behavior and attempting to measure the outcomes of interactions in educational contexts, but a complex picture is emerging. While plenty of literature is available from pedagogical fields which describe teaching concepts, there are rarely examples of guidance for social behavior at the resolution required by social roboticists for designing robot behavior. The importance of social behavior in teaching and learning has been demonstrated between humans (Goldin-Meadow et al., 1992, 2001), but not enough is known for implementation in human–robot interaction (HRI) scenarios. This has led researchers to start exploring precisely how a robot should behave socially when information needs to be communicated to, and retained by, human learners (Huang and Mutlu, 2013; Kennedy et al., 2015d).
In this article, we seek to establish what constitutes appropriate social behavior for a robot with the aim of maximizing learning in educational interactions, as well as how such social behavior might be characterized across varied contexts. First, we review work conducted in the field of HRI between robots and children in learning environments, finding that the results are somewhat mixed and that it is difficult to draw comparisons between studies (Section 2.1). Following this, we consider how social behavior could be characterized, allowing for a better comparison between studies and highlighting immediacy as one potentially useful metric (Section 2). Immediacy literature is then used to generate a hypothesis for educational interactions between robots and children. In an evaluation to test this hypothesis, nonverbal immediacy scores are gathered for a variety of robot behaviors from the same context (Section 3). While the data broadly agrees with the predictions from the literature, there are important differences that are left unaccounted for. We discuss these differences and draw on the literature to hypothesize a possible model for the relationship between robot social cues and child learning (Section 2.5). The work contributes to the field by furthering our understanding of the impact of robot nonverbal social behavior on task outcomes, such as learning, and by proposing a model that generates predictions that can be objectively assessed through further empirical investigation.
2. Related Work
2.1. Robot Social Behavior and Child Learning in HRI
There are many examples of compelling results, which support the notion that the physical presence of a robot can have a positive impact on task performance and learning. Leyzberg et al. (2012) found that adults who were tutored by a physical robot significantly outperformed those who interacted with a virtual character when completing a logic puzzle. A controlled classroom-based study by Alemi et al. (2014) employed a robot to support learning English from a standard textbook over 5 weeks with a (human) teacher. In one condition, normal delivery was provided, and in the other, this delivery was augmented with a robot that was preprogrammed to explain words through speech and actions. It was found that using a robot to supplement teaching over this period led to significant child learning increases when compared to the same material being covered by the human teacher without a robot. This is strong evidence for the positive impact that robots can have in education, which has been supported in other scenarios. Tanaka and Matsuzoe (2012) also found that children learn significantly more when a robot is added to traditional teaching, both immediately after the experiment and after a delayed period (3–5 weeks later). Combined, these findings suggest that the use of a physically embodied robot can positively contribute to child learning.
Aspects of a robot’s nonverbal social behavior have been investigated in one-on-one tutoring scenarios with mixed results. Two studies in the same context by Kennedy et al. (2015c) and Kennedy et al. (2015d) have found that the nonverbal behavior of a robot does have an impact on learning, but that the effect is not always in agreement with predictions from the human–human interaction (HHI) literature. These studies will be considered in more detail in Section 3. Similarly, Herberg et al. (2015) found that the HHI literature would predict an increase in learning performance with increased gaze of a robot toward a pupil, but the opposite was observed: an Aldebaran NAO would look either toward or away from a child while they completed a worksheet based on material they had learnt from the robot, but this was not found to be the case. However, Saerbeck et al. (2010) varied socially supportive behaviors of a robot in a novel second language learning scenario. These behaviors included gestures, verbal utterances, and emotional expressions. Children learnt significantly more when the robot displayed these socially supportive behaviors.
The impact on child learning of verbal aspects of robot behavior has also been investigated. Gordon et al. (2015) developed robot behaviors to promote curiosity in children with the ultimate aim of increased learning. While the children were reciprocal in their curiosity, their learning did not increase as the HHI literature would predict. Kanda et al. (2012) compared a “social” robot to a “non-social” robot, operationalized through verbal utterances to children when they are completing a task. Children showed a preference for the social robot, but no learning differences were found.
Ultimately, it is a difficult task to present a coherent overview of the effect of robot social behavior on child learning, with many results appearing to contradict one another or not being comparable due to the difference in learning task or behavioral context. More researchers are now using the same robotic platforms and peripheral hardware than before (quite commonly the Aldebaran NAO with a large touchscreen, e.g., Baxter et al. (2012)), but there remain few other similarities between studies. Behavior of various elements of the system is reported alongside learning outcomes, but it is difficult to translate from these descriptions to something that can be compared between studies. As such, it becomes almost impossible to determine if differing results between studies (and discrepancies with HHI predictions) are due to differences in robot behavior, the study population, other contextual factors, or indeed a combination of all three. It is apparent that a characterization of the robot social behavior would help to clarify the differences between studies and provide a means by which certain factors could be accounted for in analysis; this will be explored in the following section.
2.2. Characterizing Social Behavior through Nonverbal Immediacy
To allow researchers to make clearer comparisons between studies and across contexts, a metric to characterize the social behavior of a robot is desirable. Various metrics have been used before in HRI. Retrospective video coding has been used in several HRI studies as a means of measuring differences in human behavioral responses to robots, for example, the studies by Tanaka and Matsuzoe (2012); Moshkina et al. (2014); Kennedy et al. (2015b). However, this method of characterizing social behavior is incredibly time consuming, particularly when the coding of multiple social cues is required. Furthermore, it provides data for social cues in isolation and does not easily provide a holistic characterization of the behavior. It is unclear what it means if the robot gazes for a certain number of seconds at the child in the interaction and also performs a certain number of gestures; this problem is exacerbated when a task context changes. The perception of the human directly interacting with the robot is also not accounted for. It is suggested that the direct perception of the human within the interaction is an important one, as they are the one being influenced by the robot behavior in the moment. This cannot be captured through post hoc video coding.
The Godspeed questionnaire series developed by Bartneck et al. (2009b) has been used in many HRI studies to measure users’ perception of robots (Bartneck et al., 2009a; Ham et al., 2011). The animacy and anthropomorphism elements of the scale in particular consider the social behavior and perception of the robot. However, it is not particularly suited to use with children due to the language level (i.e., use of words such as “stagnant,” “organic,” and “apathetic”). It may also be that the questionnaire would measure aspects of the robot not directly related to social behavior as it is asking about more general perceptions. While this could be of use in many studies, for the aim of characterizing social behavior in the case here, these aspects prevent suitable application.
Nonverbal immediacy (NVI) was introduced in the 1960s by Mehrabian (1968) and is defined as the “psychological availability” of an interaction partner. Immediacy is further introduced as being a measure that indicates “the attitude of a communicator toward his addressee” and in a general form “the extent to which communication behaviors enhance closeness to and nonverbal interaction with another” (Mehrabian, 1968). A number of specific social behaviors are listed (touching, distance, forward lean, eye contact, and body orientation) to form a part of this measure, which were later utilized by researchers that sought to create and validate measuring instruments for NVI. However, it is also this feature that makes NVI a particularly enticing prospect for designers of robot behavior, as the social cues used in the measure are explicit (which is often not the case in other measures of perception commonly used in the field, e.g., Bartneck et al. (2009b)). A reasonable volume of data also already exists for studies considering immediacy, with over 80 studies (and N nearly 25,000) from its inception to 2001 (Witt et al., 2004) and more since. This provides a context for NVI findings in HRI scenarios and a firm grounding in the human–human literature from which roboticists can draw.
Several versions of surveys have been developed and validated for measuring the nonverbal immediacy of adults (Richmond et al., 2003). Surveys have also been developed for verbal immediacy (Gorham, 1988), but their ability to measure precisely the concept of verbal immediacy remains the subject of debate (Robinson and Richmond, 1995). Both verbal and nonverbal measures consider observed overt behavior more than, but not excluding, perceptions. Immediacy has recently been used in HRI as a means of motivating robot behavior manipulations (Szafir and Mutlu, 2012) and characterizing social behavior (Kennedy et al., 2017).
There is a consensus on the instruments used to measure nonverbal immediacy (whereas this is less clear for verbal immediacy), and it is also transparent in terms of how participants are judging the robot. The Godspeed questionnaire is a useful tool for gathering perceptions, but nonverbal immediacy is clearly measuring overt social behavior, and so it is ideal given our scope of trying to characterize social behavior (often with children). Use of the NVI metric brings several other advantages to researchers in HRI and for robot behavior designers. The NVI metric can be used as a guideline for an explicit list of social cues available for manipulation as a part of robot behavior. Characterization of robot social behavior at this relatively low level is not readily available in other metrics. This provides a useful first step in designing robot behavior but also a means of evaluating and modifying future social behaviors. NVI constitutes part of an overall social behavior; hence NVI is treated as a characterization of the overall behavior, not a complete description or definition. Not all aspects of sociality or interaction are addressed through the measure, but to the knowledge of the authors, nor are these aspects fully covered by any other validated metric.
The NVI metric can be used with either the subjects themselves or with observers (during or after the interaction). This permits flexibility depending on the needs of the researcher. It is not always practical to collect such data from participants (for example, when they are young children or following an already lengthy interaction), so having the flexibility to gather these data post hoc is advantageous. Due to this mixture of practical and theoretical benefits, nonverbal immediacy (NVI) will be adopted as a social behavior characterization metric for this article.
Immediacy has been validated through physical manipulation of some of the social cues, specifically eye gaze and proximity, to ensure that the phenomenon indeed works in practice and is not a product of affect or bias in survey responses (Kelley and Gorham, 1988). It was indeed found that the physical manipulations that were made which would lead to a higher immediacy score (standing closer and providing more eye gaze) did lead to increased short-term recall of information. While there is clearly a difference between recall and learning, recall of information is a promising first step to acquiring new understanding and skills. These results were hypothesized to exist in the other immediacy behaviors (such as gestures) as well. Overall, the link between teacher immediacy and student learning is hypothesized to be a positive one, as reflected in the meta-review by Witt et al. (2004) and many studies (Comstock et al., 1995; McCroskey et al., 1996; Christensen and Menzel, 1998). Thus, this prediction can be tested in human–robot interaction, where the robot takes the role of the tutor. As a result, we generate the following hypothesis:
H1. A robot tutor perceived to have higher immediacy leads to greater learning than a robot perceived to have lower immediacy.
3. Applying Nonverbal Immediacy to HRI
In this section, an evaluation of nonverbal immediacy (NVI) in the context of cHRI is described. The aim is to explore whether the characterization that it provides can account for the differences between robot behaviors and learning outcomes of children. The wealth of literature that explores NVI in educational scenarios is generally in agreement that higher NVI of an instructor is positively correlated with learning outcomes of students. We evaluate 4 differently motivated robot behaviors and a human in a one-to-one maths-based educational interaction with children. The aim is to use these data to provide a comparison between behavioral manipulations to test predictions from the HHI immediacy literature regarding social behavior.
3.1. Task Design and Measures
All five behaviors under consideration use the same context and broader methodology. Children aged 8–9 years are taught how to identify prime numbers between 10 and 100 using a variation on the Sieve of Eratosthenes method. They interact with a tutor: in 4 conditions, this is an Aldebaran NAO robot, and in 1 condition, this is a human (Figure 1). Children complete pretests and posttests in prime number identification, as well as pretests and posttests for division by 2, 3, 5, and 7 (skills required by the Sieve of Eratosthenes method for numbers in the range used) on a large touchscreen. The tutor provides lessons on primes and dividing by 2, 3, 5, and 7 (Figure 2). In all cases, an experimenter briefs the child and introduces the child to the tutor. The experimenter remains in the room throughout the interaction, but out of view of the child. Two cameras record the interactions; one is directed toward the child and one toward the tutor. Interactions with the tutor would last for around 10–15 min, with an additional 5 min required afterward in conditions where nonverbal immediacy surveys were completed (details to follow).
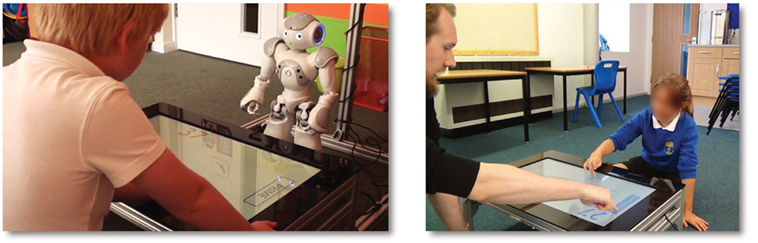
Figure 1. (Left) Still image from a human–robot interaction (specifically, the “social” condition), and (right) still image from the human–human condition. The tutor (either robot or human) teaches children how to identify prime numbers using the Sieve of Eratosthenes method using a large horizontal touchscreen as a shared workspace. The robot can “virtually” move numbers on screen (numbers move in correspondence with robot arm movements, but physical contact is not made with the screen).
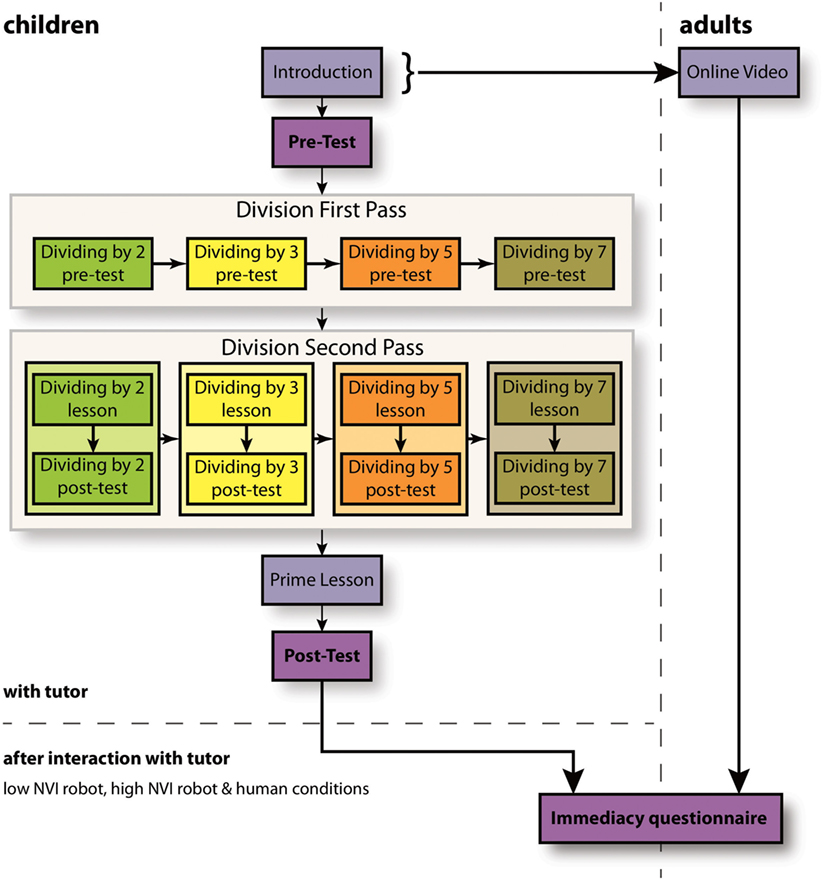
Figure 2. Task structure—the top section is led by the tutor and is aimed at teaching children how to calculate whether a number is prime. The bottom section consists of completing the nonverbal immediacy questionnaire—this is done after the interaction for 3 of the child conditions and via online videos to get adult responses. Dark purple boxes (pretest, posttest, and immediacy questionnaire) are the metrics under consideration in this article.
At the start of the interaction, the children complete a pretest in prime numbers on the touchscreen without any feedback from the screen or the tutor. A posttest is completed by the children at the end of the interaction; again no feedback is provided to the child so as not to influence their categorizations. Two tests are used in a cross-testing strategy, so children have a different pretest and posttest, and the tests are varied as to whether they are used as a pretest or posttest. The tests require the children to categorize numbers as “prime” or “not prime” by dragging and dropping numbers on screen into the category labels. Each test has 12 numbers, so by chance, a score of 6 would be expected (given 2 possible categories 50% is chance). Learning is measured through the improvement in child score from the prime number pretest to posttest. By considering the improvement, any prior knowledge (correct or otherwise) or deviation in division skill is factored in to the learning measure. The mean and SD score (of 12) for the pretests are compared to those of the posttest to calculate the learning effect size (Cohen’s d) for each condition.
The prime number task was selected in consultation with education professionals to ensure that it was appropriate for the capabilities of children of this age. Children of this age have not yet learnt prime number concepts in school, but do have sufficient (but imperfect) skills for dividing by 2, 3, 5, and 7 as required by the technique for calculating whether numbers are prime. During the division sections of the interaction, the tutor provides feedback on child categorizations.
Nonverbal immediacy (NVI) scores are collected through questionnaires. For children, this was done after the interaction with the tutor had been completed, for adults, this was online (details in Section 3.4). A standard nonverbal immediacy questionnaire was adapted for use with children by modifying some of the language; the original and modified versions alongside the score formula can be seen online.1 Both the Robot Nonverbal Immediacy Questionnaire (RNIQ) and Child-Friendly Nonverbal Immediacy Questionnaire (CNIQ) were used depending on condition for children. Adults had the same questionnaire but with “the child” in place of “you” as they were observing the interaction, rather than participating in it. The questionnaire consists of 16 questions about overt nonverbal behavior of the tutor. Each question is answered on a 5-point Likert scale, and a final immediacy score is calculated by combining these answers. Some count positively toward the nonverbal immediacy score, whereas some count negatively, depending on the wording of the question. The version in the Appendix shows the questionnaire used for this study when a robot (as opposed to a human) tutor was used as this has been validated for use in HRI (Kennedy et al., 2017) and corresponds to the validated version from prior human-based literature (Witt et al., 2004).
Existing immediacy literature extensively uses adults (often students) as subjects; studies with children are rare. Prior work has been conducted with the adapted nonverbal immediacy scale for use with robots and children (Kennedy et al., 2017); however, the task in this article is novel in this context (one-to-one interactions instead of group instruction). Children present unique challenges when using questionnaire scales, such as providing different answers for negatively worded questions to positively worded ones (Borgers et al., 2004) or trying to please experimenters (Belpaeme et al., 2013), which can consequently make it difficult to detect differences in responses (Kennedy et al., 2017). As children are not well represented in immediacy literature, using adults for NVI scores more tightly grounds our hypotheses and assumptions to the existing literature. However, NVI ratings are collected from children in robot conditions in which NVI is intentionally manipulated. As the nonverbal immediacy was intentionally manipulated between these conditions, and the adult results can provide some context, we can observe whether children do perceive the manipulation on this scale, potentially broadening the applicability of our findings.
3.2. Conditions
A total of 5 conditions are used in this evaluation.2 As described in the introduction, an often adopted approach to social behavioral design is to consider how a human behaves and reproduce that (insofar as is possible) on the robot. As such, we use 2 conditions, seeking to follow and also invert this approach. We additionally use 2 conditions derived from the NVI literature, again seeking to maximize and minimize the behaviors along this scale. The final condition is a human benchmark. Further details for each can be seen in Table 1 and below:
1. “Social” robot (SR)—this condition is derived from observations of an expert human–human tutor completing this task with 6 different children. This condition reflects a human model-based approach to designing the behavior. The social behavior of the tutor was analyzed through video coding, and these behaviors were implemented on the robot where possible.
2. “Asocial” robot (AR)—this condition considers the behavior generated for the SR condition and seeks to “invert” it. That is, the behavior is intentionally manipulated such that an opposite implementation is produced, for example, the SR condition seeks to maximize mutual gaze, whereas this condition actively minimizes mutual gaze. The quantity of social cues used in this condition is exactly the same as the SR condition above; however, the placement of these cues is varied (for example, a wave would occur during the greeting in SR, but during an explanation in AR).
3. High NVI robot (HNVI)—this condition uses the literature to drive the behavioral design. The behavior is derived from considering how the social cues within the nonverbal immediacy scale can be maximized. For example, the robot will seek to maximize gaze toward the child and make frequent gestures.
4. Low NVI robot (LNVI)—this condition is intended to be the opposite to the HNVI condition. Again, the nonverbal immediacy literature is used to drive the design, but in this case, all of the social cues are minimized. For example, the robot avoids gazing at the child and makes no gestures.
5. Human (HU)—this is a human benchmark. The human follows the same script for the lessons as the robot, but they are not constrained in their social behavior. The intention here is that we can then acquire data for a “natural”, non-robot interaction where the social behavior is not being manipulated; this can then be used to provide context for the robot conditions.
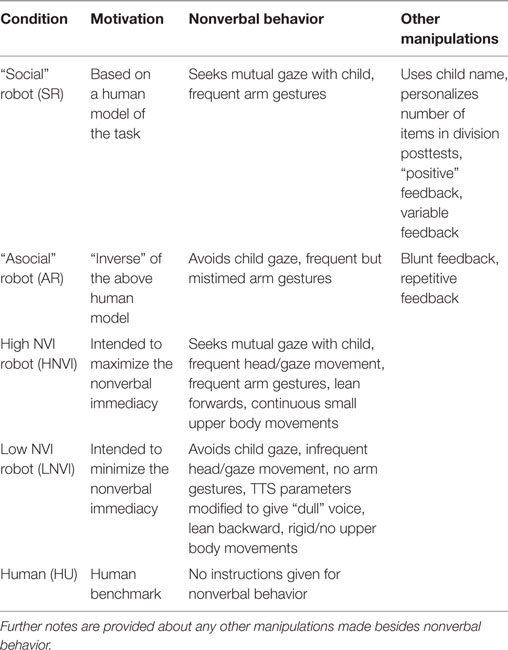
Table 1. Operationalization of the differences in nonverbal behavior between the conditions considered in the study presented in this article.
A summary of the motivations for the conditions and the operationalization of the differences between conditions can be seen in Table 1. Further implementation details can be seen in “Robot Behavior.” While the Aldebaran NAO platform cannot be manipulated for some of the cues involved in the nonverbal immediacy measure given the physical setup and modalities of the robot (i.e., smiling and touching), it has been manipulated on all of the other cues possible. This leaves only 4 of the 16 questions (2 of 8 cues) not manipulated in the metric. Specifically, these are questions 4, 8, 9, and 13, as seen in the Appendix, pertaining to frowning/smiling and touching.
3.2.1. Robot Behavior
Throughout the division sections of the interaction, the tutor (human or robot) would provide feedback on child categorizations and could also suggest numbers for the child to look at next. This was done through moving a number to the center of the screen and making a comment such as “why don’t you try this one next?” The tutor would also provide some prescripted lessons (Figure 2) that would include 2 example categorizations on screen. These aspects are central to the delivery of the learning content, so are maintained across all conditions to prevent a confound in learning content.
All robot behavior was autonomous, apart from the experimenter clicking a button to start the system once the child was sat in front of the touchscreen. The touchscreen and a Microsoft Kinect were used to provide input for the robot to act in an autonomous manner. The touchscreen would provide information to the robot about the images being displayed and the child moves on screen, the Kinect would provide the vector of head gaze for the child and whether this was toward the robot. Through these inputs, the robot behavior could be made contingent on child actions, for example, by providing verbal feedback after child moves (in all conditions), or manipulating mutual gaze. In all robot conditions, the robot gaze was contingent on the child’s gaze, but with differing strategies depending on the motivation of the condition. The AR and LNVI conditions would actively minimize mutual gaze by intentionally avoiding looking at the child, whereas the SR and HNVI conditions would actively maximize mutual gaze by looking at the child when data from the Kinect indicated that the child was looking at the robot. Robot speech manipulation executed in the LNVI condition to make the robot voice “dull” was achieved through lowering the vocal shaping parameter of the TTS engine (provided by Acapela).
Due to the human model-based approach, some personalization aspects such as use of child name were included as part of the social behavior in the SR condition. This was not done in the NVI conditions as these manipulations are not motivated through the NVI metric. The HNVI condition also addresses more of the NVI questionnaire items (leaning forward and continuous “relaxed” upper body movements) than the SR condition due to this difference in motivation. The AR condition has the same quantity of behavior as the SR condition, whereas the LNVI has a lack of behavior. As a concrete example, the AR condition includes inappropriately placed gestures, whereas the LNVI condition includes no gestures. Consequently, the LNVI and HNVI conditions provide useful comparisons both to one another and to the SR and AR conditions.
3.3. Participants
To provide NVI scores for all 5 conditions, video clips of the conditions were rated by adults. Nonverbal immediacy scores were also acquired at the time of running the experiments for 3 of the 5 conditions (high and low NVI robot and human) from children through paper questionnaires (Table 2). These scores allow a check that the NVI manipulation between the robot conditions could be perceived by the children, with the adult data provided context for these ratings. Written informed consent from parents/guardians was received for the children to take part in the study, and they additionally provided verbal assent themselves, in accordance with the Declaration of Helsinki. Written informed consent from parents/guardians and verbal assent from children were also received for the publication of identifiable images. The protocol was reviewed and approved by the Plymouth University ethics board. Table 2 shows numbers of participants per condition and average ages for the adult conditions; all children were aged 8 or 9 years old and were recruited through a visit to their school, where the experiment took place.
3.4. Adult Nonverbal Immediacy Score Procedure
Videos shown to adults to acquire nonverbal immediacy scores were each 47 s long. The videos contained both the interaction video (42 s) and a verification code (5 s; details in the following paragraph). The length of video was selected to be 42 s as the literature suggests that at least around 6 s are required to form a judgment of social behavior (Ambady and Rosenthal, 1993), and there was a natural pause at 42 s in the speech in all conditions so that it would not cut part-way through a sentence. The interaction clips were all from the start of an interaction, so the same information was being provided by the tutor to the child in the clip.
To provide sufficient subject numbers for all of the conditions, an online crowdsourcing service3 was used. The participants were restricted to the USA and could only take part if they had a reliable record within the crowdsourcing platform. A test question was put in place whereby participants had to enter a 4 digit number into a text box. This number was shown at the end of the video for 5 s (the video controls were disabled so it could not be paused and the number would disappear after the video had finished). A different number was used for each video. If the participants did not enter this number correctly, then their response was discarded. The crowdsourcing platform did not allow the prevention of users completing multiple conditions, so any duplicates were removed, i.e., only those seeing a video for the first time were kept as valid responses. A total of 366 responses were collected, but 209 were discarded as they did not answer the test question correctly, the user had completed another condition,4 or the response was clearly spam (for example, all answers were “1”). This left 157 responses across 5 conditions; 90M/67F (Table 2).
4. Results
When performing a one-way ANOVA, a significant effect is found for condition seen, showing that the robot behavior influences perceived nonverbal immediacy; F(4,152) = 14.057, p < 0.001. Post hoc pairwise comparisons with Bonferroni correction reveal that the adult-judged NVI of the LNVI condition is significantly different to all other conditions (p < 0.001 in all cases), but no other pairwise comparisons are statistically significant at p < 0.05. The nonverbal immediacy score means and learning effect sizes for each condition can be seen in Table 3. Children learning occurs in all conditions. Generally, it can be seen that the conditions with higher rated nonverbal immediacy lead to greater child improvement in identifying prime numbers.
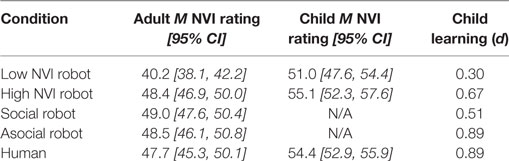
Table 3. Adult and child nonverbal immediacy ratings and child learning (as measured through effect size between pretests and posttests for prime numbers) by tutor condition.
While significance testing provides an indication that most of the conditions are similar (at least statistically) in terms of NVI, additional information for addressing the hypothesis can be gleaned by considering the trend that these data suggest (Figure 3). A strong positive correlation is found between the (adult) NVI score of the conditions and the learning effect sizes (Cohen’s d) of children who interacted in those conditions (r(3) = 0.70, p = 0.188). This correlation is not significant, likely due to the small number of conditions under consideration, but the strength of the correlation suggests that a relationship could be present.
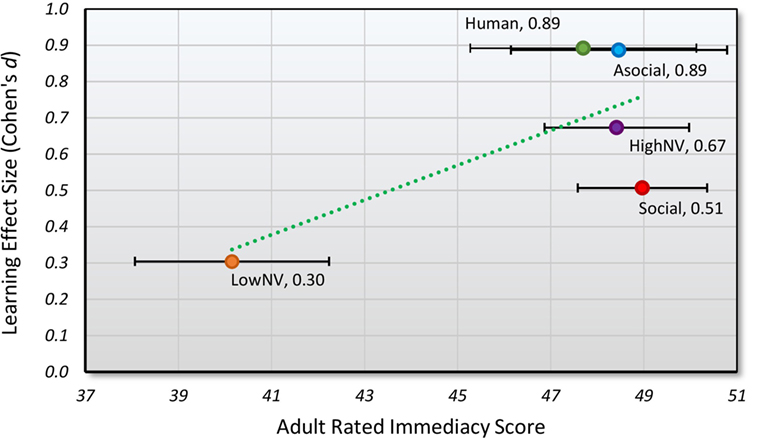
Figure 3. Nonverbal immediacy scores as judged by adults and learning effect sizes for the prime number task. The dotted green line indicates a trend toward greater nonverbal immediacy of the tutor leading to increased learning. Error bars show 95% confidence interval.
When the immediacy scores provided by the children who interacted with the robot are also considered, a similar pattern can be seen (Figure 4). The adult and child immediacy ratings correlate well, with a strong positive correlation (r(1) = 1.00, p < 0.001). There is also a strong positive correlation for the children between immediacy score of the conditions and the learning effect sizes (Cohen’s d) in those conditions (r(1) = 0.86, p = 0.341). Again, significance is not observed, but the power of the test is low due to the number of data points available for comparison. The strong positive correlations between child immediacy scores and learning and adult immediacy scores and learning provide some support for hypothesis H1 (that higher tutor NVI leads to greater learning), but further data points would be desired to explore this relationship further. It should be noted that we consider the results of 57 children and 157 adults across 5 conditions; acquiring further data points for more behaviors (and deciding what these behaviors should be) would be a time-consuming task.
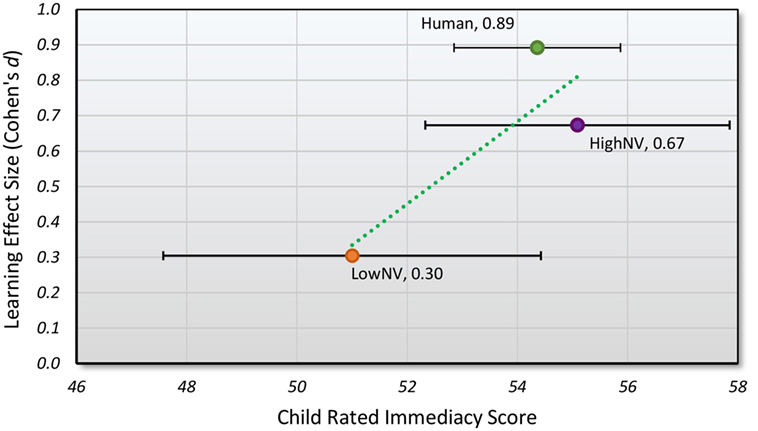
Figure 4. Nonverbal immediacy scores as judged by the children in the interaction and learning effect sizes for the prime number task. The dotted green line indicates a trend toward greater perceived nonverbal immediacy of the tutor leading to increased learning. Error bars show 95% confidence interval.
5. Discussion
There is a clear trend in support of hypothesis H1: that a tutor perceived to have higher immediacy leads to greater learning. As such, increasing the nonverbal immediacy behaviors used by a social robot would likely be an effective way of improving child learning in educational interactions. However, nonverbal immediacy does not account for all of the differences in learning. Three of the conditions have near identical NVI scores as judged by adults, but quite varied learning results (high NVI robot: M = 48.4 NVI score/d = 0.67 pre–post test improvement, asocial robot: NVI M = 48.5/d = 0.89, social robot: NVI M = 49.0/d = 0.51). This partially reflects the slightly mixed picture of immediacy that the pedagogy literature presents; for example, the disagreement as to whether NVI has a linear (Christensen and Menzel, 1998) or curvilinear (Comstock et al., 1995) relationship with learning. Nonetheless, there are further factors that may be introduced by the use of a robot that may have had an influence on the results. Nonverbal immediacy only considers overt observed social behaviors, so by design does not cover all possible aspects of effective social behavior for teaching. While this seems to be enough in HHI (Witt et al., 2004), it may not be for HRI since various inherent facets of human behavior cannot be assumed for robots. Several possible explanations as to why this learning variation is present will now be discussed. From this, a possible model (suggested to be more accurate) of the relationship between social behavior and learning is proposed. Such a model may be useful in describing (and testing) the relationship between social behavior and child learning for future research.
5.1. Timing of Social Cues
The quantity of social cues used in both the social robot and the asocial robot conditions is exactly the same; however, the timing is varied. Timing is not considered as part of the nonverbal immediacy metric—the scale measures whether cues have, or have not, been used, rather than whether their timing was appropriate. The cues used in the asocial robot condition were intentionally placed at inappropriate times (for example, waving part-way through the introduction, instead of when saying hello). This is not factored into the nonverbal immediacy measure, but could impact the learning (Nussbaum, 1992).
The timing of social cues in the human condition may also explain why the learning in this condition was higher than the others. The robot conditions are contingent on aspects of child behavior, such as gaze and touchscreen moves, but are not adapted to individual children (for example, the number of feedback instances the robot provides would not be based on how well the child was performing). However, the human is presumably adaptive in both the number of social cues used and the timing of these cues. Again, this would not be directly revealed by the immediacy metric, but could account for some of the learning difference. Indeed, the nonverbal immediacy metric comes from HHI studies and has been validated in such environments. In HHI, there is a reasonable assumption that the timing of social cues will be appropriate, and so it may not be necessary to include it as part of a behavioral metric for HHI. However, when applied to social robotics, the assumption of appropriate timing no longer applies, and so to fully account for learning differences in HRI, timing may need more explicit incorporation into characterizations of social behavior. This constitutes a limitation of the NVI metric, but also an opportunity for expansion in future work to capture timing aspects.
5.2. Relative Importance of Social Cues
One substantial difference between the robot conditions and the human condition is the possibility of using facial expressions. The robotic platform used for the studies was the Aldebaran NAO. This platform has limited ability to generate facial expressions as none of the elements of the face can move, only the eye color can be changed. On the other hand, the human has a rich set of facial expressions to draw upon.
While the overall nonverbal immediacy scores for the asocial, social, and human conditions are tightly bunched, the make-up of the scores is not. For example, the robot scores (asocial and social combined) are higher for gesturing, averaging M = 4.3 (95% CI 4.1, 4.5) out of 5 for the nonverbal immediacy question about gesturing (the robot uses its hands and arms to gesture while talking to you), compared to M = 3.1 (95% CI 2.7, 3.5) for the human. However, the human is perceived to smile more (M = 2.5, 95% CI 2.1, 2.8) than the robot (M = 1.8, 95% CI 1.5, 2.0). Through principle component analysis, Wilson and Locker (2007) found that different elements of nonverbal behavior do not contribute equally to either the nonverbal immediacy construct or instructor effectiveness. Facial expressions (specifically smiles) have a large impact on both the nonverbal immediacy construct and the instructor effectiveness, whereas gestures do not have such a large effect (although still a meaningful contribution; smiles: 0.54, gestures: 0.30 component contribution from Wilson and Locker (2007)).
In the nonverbal immediacy metric, all social cues are given equal weighting. However, this may not always be the most appropriate method for combining the cues given the evidence, which suggests that some cues may contribute more than others to various outcomes (McCroskey et al., 1996; Wilson and Locker, 2007). This could be a further explanation as to why several of the conditions in the study conducted here have near identical overall nonverbal immediacy scores, but very different learning outcomes.
5.3. Novelty of Character and Behavior
The novelty of both the character (i.e., robot or human) and the behavior itself could have had an impact on the learning results found in the study. Novelty is often highlighted as a potential issue in experiments conducted in the field (Kanda et al., 2004; Sung et al., 2009). The novelty of the robot behavior could override the differences between the conditions and subsequently influence the learning of the child. In the social robot condition here, novel behavior (such as new gestures) was often introduced when providing lessons to the child. Between humans, this would likely result in a positive effect (Goldin-Meadow et al., 2001), but when done by a robot, the novelty of the behavior may counteract the intended positive effect.
There may also be a difference in the novelty effect for the children seeing the robot when compared to the human. Although the human is not one that they are familiar with, they are still “just” a human, whereas the robot is likely to be more exciting and novel as child interaction with robots is more limited than with humans. The additional novelty of the robot could have been a distraction from the learning, explaining why the learning in the human condition is higher.
Finally, the novelty may have impacted the nonverbal immediacy scores themselves. It is possible that observers (be they children or adults) score immediacy on a relative scale. It is reasonable to suggest that the immediacy of the characters is judged not as a standalone piece of behavior, but in the context of an observer’s prior experience, or expectations for what that character may be capable of. Clear expectations will likely exist for human behavior, but not for robot behavior, which may lead to an overestimation of robot immediacy. This would impact on the ability of considering the human and robots on the same nonverbal immediacy scale and drawing correlations with learning and cannot be ruled out as a factor in the results.
5.4. (In)Congruency of Social Cues
As previously discussed, the robot is limited in the social cues that it can produce (for example, it cannot produce facial expressions). This meant that the conditions all manipulated the available robot social cues, but if social cues are interpreted as a single percept by the human (as suggested by the literature (Zaki, 2013)), then this could lead to complications.
In the case of the social robot, many social cues are used to try and maximize the “sociality” of the robot. This means that there is a lot of gaze from the robot to the child, and the robot uses a lot of gestures. However, it still cannot produce facial expressions. This incongruency between the social cues could produce an adverse effect in terms of perception on the part of the child and subsequently diminish the learning outcome. There are clear parallels here with the concept of the Uncanny Valley (Mori et al., 2012), with models for the Uncanny Valley based on category boundaries in perception indicating issues arising from these mismatches (Moore, 2012).
The expectation the child has for the robot social behavior is suggested to be of great importance (Kennedy et al., 2015a). If their expectations are formed early on through high quantities of gaze and gestures, then there would be a discrepancy when facial expressions do not match this expectation. Again, this expectation discrepancy may lead to adverse effects on learning outcomes, as in the case of perceptual issues due to cue incongruence. These issues may become exacerbated as the overall level of sociality of behavior of the robot increases as any incongruencies then become more pronounced. As stated in the study by Richmond et al. (1987), higher immediacy generally leads to more communication, which can create misperceptions (of liking, or expected behavior).
As the nonverbal immediacy scale has been rigorously validated (McCroskey et al., 1996; Richmond et al., 2003), it is known that it does indeed provide a reliable metric for immediacy in humans (Cronbach’s alpha is typically between 0.70 and 0.85 (McCroskey et al., 1996)). Typically, internal consistency measures of a scale would be used to evaluate the ability of items in a scale to measure a unidimensional construct, i.e., how congruent the items are with one another. As such, a consistency measure could be used as an indicator of the congruency between the cues. The robot lacks a number of capabilities when compared to humans, and there are several scale items that are known to be impaired on the robot, such as smiling/frowning. Using an internal consistency measure across all NVI questionnaire items (with the negatively worded question responses reversed) can reveal cases in which the cues are relatively more or less congruent. Greater internal consistency indicates lower variability between questionnaire items (the social cues) and, therefore, more congruence between the social cues. Lower internal consistency indicates larger variability between scale items and thus greater incongruency between the cues.
Guttman’s λ6 (or G6) for each condition has been calculated,5 revealing that indeed there are differences in how congruent the cues could be considered to be (Table 4; Figure 5). All of the NVI questionnaire items are included in the λ6 calculation. The behavioral conditions used here are restricted in such a way that a lower reliability would be expected (as several cues of the scale are not utilized) for some conditions. Indeed, these values fall in line with predictions that could be made based on the social behavior in each of the conditions. The human reliability score provides a “sanity check” as it is assumed that human behavior would have a certain degree of internal consistency between social cues, which is reflected by it having the highest value. In addition, the LNVI robot condition has intentionally low NVI behavior, so the lack of smiling or touching (high NVI behaviors) does not cause incongruency (signified by a lower λ6 score), whereas the HNVI robot condition has intentionally high NVI behavior where possible on the robot, so the lack of smiling and touching cause greater overall incongruency, resulting in a considerably lower λ6 score.
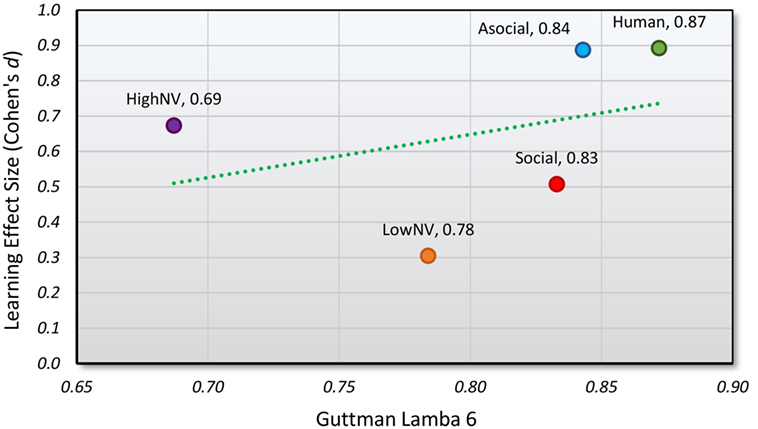
Figure 5. Guttman’s λ6 against learning effect size for each of the prime tutoring conditions. The dotted line indicates a trend toward greater internal consistency (measured through λ6) leading to greater learning.
5.5. A Hypothesis: Social Cue Congruency and Learning
Taking Guttman’s λ6 to provide an indication of the congruency of social cues, then it is clear that this alone would not provide a strong predictor of learning (Figure 5). However, these data can be combined with the social behavior (as measured through immediacy) to be compared to learning outcomes. In the resulting space, both congruency and social behavior could have an impact on learning, as hypothesized in the previous section (Figure 6).
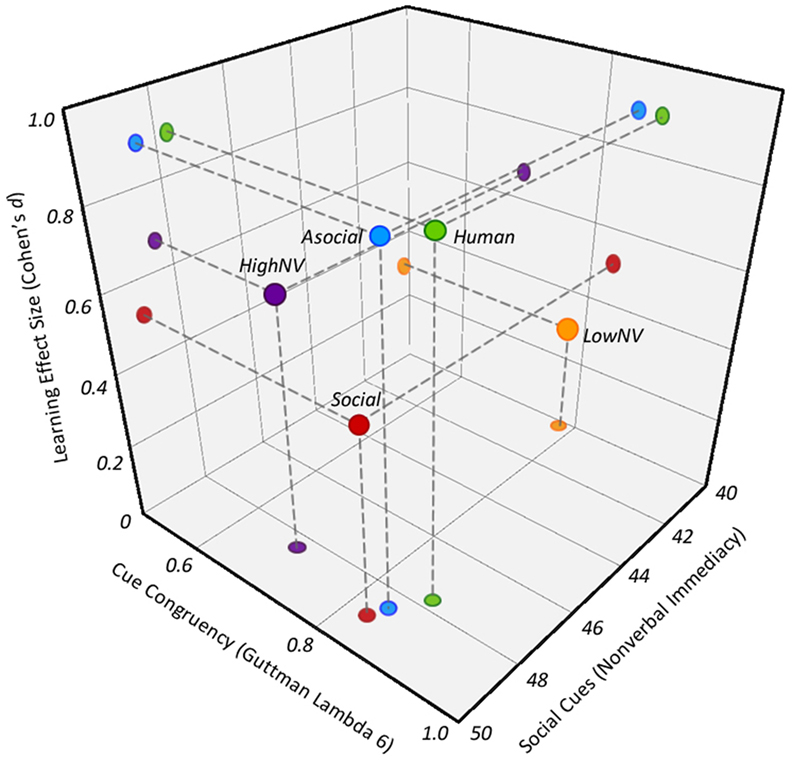
Figure 6. Learning, congruency, and social behavior for each of the 5 conditions. Learning is measured in effect size between pretest and posttest for children. Congruency is indicated through Guttman’s λ6 of the adult nonverbal immediacy scores. Social behavior is characterized through nonverbal immediacy ratings from adults. An interactive version of this figure is available online to provide different perspectives of the space: https://goo.gl/ZNPxc8.
Our data show that learning is best with human behavior, which is shown to be highly social and reasonably congruent. When the social behavior used is congruent, but not highly social, then the learning drops to a low level. The general trend of our data shows that when the congruency of the cues increases (indicated by Guttman’s λ6), learning also increases, and the same is true for social cues. The combination of congruency and social behavior as characterized by nonverbal immediacy provides a basis for learning predictions, where the combination of high social behavior and social cue congruency is necessary to maximize potential learning.
Such a hypothesis is supported by the view of social cues being perceived as a single percept, as suggested by Zaki (2013). Experimental evidence with perception of emotions would seem to provide additional weight to such a perspective (Nook et al., 2015). This has clear implications for designers of social robot behavior when human perceptions or outcomes are of any degree of importance. The combination of all social cues in context must be considered alongside the expectations of the human to generate appropriate behavior. Not only does this give rise to a number of challenges, such as identifying combinatorial contextual expectations for social cues, but it could also have implications for how social cues should be examined experimentally. The isolation of specific social cues in experimental scenarios would not describe the role of that social cue, but the role of that social cue, given the context of all other cues. This is an important distinction that leads to a great deal more complexity in “solving” behavioral design for social robots, but that would also contribute to explanations of why a complex picture is emerging in terms of the effect of robot behavior on learning, as discussed in Section 2.1. The NVI metric and the predictions (that can be objectively examined) we put forward below provide a means through which robot behavior designers can iteratively implement and evaluate holistic social behaviors in an efficient manner, contributing to a more coherent framework in this regard. In particular, three predictions can be derived from the extremities of the space that is presented:
P1. Highly social behavior of a tutor robot (as characterized by nonverbal immediacy) with high congruency will lead to maximum potential learning.
P2. Low social behavior of a tutor robot with low congruency will lead to minimal potential learning.
P3. A mismatch in the social behavior of a tutor robot and the social cue congruency will lead to less than maximum potential learning.
Guttman’s Lambda, as providing a measure of consistency, is used here as a proxy for the congruency of cues as observed by the study participants. We argue that this provides the necessary insight into cue congruency; however, the mapping between this metric and overtly judged congruency remains to be characterized. This would not necessarily be something that would be straightforward to achieve due to the potentially complex interactions between large numbers of social cues. For these predictions, use of the NVI metric as the characterization of social behavior would still suffer from some of the issues outlined earlier in this discussion: lack of timing information, relative cue importance, and novelty of behavior. The predictions are based on the general trends observed here, and it is noted that NVI is not a comprehensive measure of social behavior; indeed the SR condition in particular would not be fully explained using this means alone when compared to other results such as the AR condition. In addition, the data used for the learning axis were collected with relatively few samples (just over 10 per condition) in a specific experimental setup. Ideally, many further samples would be collected in both short and long term. The data collected here are over the short term and with children unfamiliar with robots. As longer term interactions take place, or as robots become more commonplace in society, expectations may change.
6. Conclusion
In this article, we have considered the use of nonverbal immediacy as a means of characterizing nonverbal social behavior in human–robot interactions. In a one-to-one maths tutoring task with humans and robots, it was shown that children and adults provide strong positively correlated ratings of tutor nonverbal immediacy. In addition, in agreement with the human–human literature, a positive correlation between tutor nonverbal immediacy and child learning was found. However, nonverbal immediacy alone could not account for all of the learning differences between tutoring conditions. This discrepancy led to the consideration of social cue congruency as an additional factor to social behavior in learning outcomes. Guttman’s λ6 was used to provide an indication of congruency between social cues. The combination of social behavior (as measured through nonverbal immediacy) and cue congruency (as indicated by Guttman’s λ6) provided an explanation of the learning data. It is suggested that if we are to achieve desirable outcomes with, and reactions to, social robots, greater consideration must be given to all cues in the context of multimodal social behavior and their possible perception as a unified construct. The hypotheses we have generated predict that the combination of high social behavior, and social cue congruency is necessary to maximize learning. The Robot Nonverbal Immediacy Questionnaire (RNIQ) developed for use here is offered as a means of gathering data for such characterizations.
Ethics Statement
This study was carried out in accordance with the recommendations of Plymouth University ethics board with written informed consent from all adult subjects. Child subjects gave verbal informed consent themselves, and written informed consent was provided by a parent or guardian. All subjects gave informed consent in accordance with the Declaration of Helsinki. The protocol was approved by the Plymouth University ethics board.
Author Contributions
Conception and design of the work, interpretation and analysis of the data, and draft and critical revisions of the work: JK, PB, and TB. Acquisition of the data: JK.
Conflict of Interest Statement
The authors declare that the research was conducted in the absence of any commercial or financial relationships that could be construed as a potential conflict of interest.
Funding
This work is partially funded by the EU H2020 L2TOR project (grant 688014), the EU FP7 DREAM project (grant 611391), and the School of Computing, Electronics and Maths, Plymouth University, UK.
Footnotes
- ^http://goo.gl/UoL5QM, also included as an Appendix.
- ^Please note that while some data have previously been published for all of these conditions (Kennedy et al., 2015c,d, 2016), this article presents both novel data collection and different analysis perspectives in a new context to the prior work.
- ^http://www.crowdflower.com/.
- ^The majority of exclusions were due to users having completed another condition, thereby impairing the independence of the results.
- ^Cronbach’s alpha tends to be the de facto standard for evaluating internal consistency and reliability; however, its use as such a measure has been called into question (Revelle and Zinbarg, 2009)—including by its own creator (Cronbach and Shavelson, 2004). Instead λ6 is used, which considers the amount of variance in each item that can be accounted for by the linear regression of all other items (the squared multiple correlation) (Guttman, 1945). This provides a lower bound for item communality, becoming a better estimate with increased numbers of items. This would appear to provide a logical (but likely imperfect) indicator for the congruency of cues as required here.
References
Alemi, M., Meghdari, A., and Ghazisaedy, M. (2014). Employing humanoid robots for teaching English language in Iranian junior high-schools. Int. J. Hum. Robot. 11, 1450022-1–1450022-25. doi: 10.1142/S0219843614500224
Ambady, N., and Rosenthal, R. (1993). Half a minute: predicting teacher evaluations from thin slices of nonverbal behavior and physical attractiveness. J. Pers. Soc. Psychol. 64, 431. doi:10.1037/0022-3514.64.3.431
Bartneck, C., Kanda, T., Mubin, O., and Al Mahmud, A. (2009a). Does the design of a robot influence its animacy and perceived intelligence? Int. J. Soc. Robot. 1, 195–204. doi:10.1007/s12369-009-0013-7
Bartneck, C., Kuli, D., Croft, E., and Zoghbi, S. (2009b). Measurement instruments for the anthropomorphism, animacy, likeability, perceived intelligence, and perceived safety of robots. Int. J. Soc. Robot. 1, 71–81. doi:10.1007/s12369-008-0001-3
Baxter, P., Wood, R., and Belpaeme, T. (2012). “A touchscreen-based ’sandtray’ to facilitate, mediate and contextualise human-robot social interaction,” in Proceedings of the 7th Annual ACM/IEEE International Conference on Human-Robot Interaction (New York, NY: ACM), 105–106.
Belpaeme, T., Baxter, P., De Greeff, J., Kennedy, J., Read, R., Looije, R., et al. (2013). “Child-robot interaction: perspectives and challenges,” in Proceedings of the 5th International Conference on Social Robotics ICSR’, Vol. 13 (Cham, Switzerland: Springer), 452–459.
Borgers, N., Sikkel, D., and Hox, J. (2004). Response effects in surveys on children and adolescents: the effect of number of response options, negative wording, and neutral mid-point. Qual. Quant. 38, 17–33. doi:10.1023/B:QUQU.0000013236.29205.a6
Christensen, L. J., and Menzel, K. E. (1998). The linear relationship between student reports of teacher immediacy behaviors and perceptions of state motivation, and of cognitive, affective, and behavioral learning. Commun. Educ. 47, 82–90. doi:10.1080/03634529809379112
Comstock, J., Rowell, E., and Bowers, J. W. (1995). Food for thought: teacher nonverbal immediacy, student learning, and curvilinearity. Commun. Educ. 44, 251–266. doi:10.1080/03634529509379015
Cronbach, L. J., and Shavelson, R. J. (2004). My current thoughts on coefficient alpha and successor procedures. Educ. Psychol. Meas. 64, 391–418. doi:10.1177/0013164404266386
Goldin-Meadow, S., Nusbaum, H., Kelly, S. D., and Wagner, S. (2001). Explaining math: gesturing lightens the load. Psychol. Sci. 12, 516–522. doi:10.1111/1467-9280.00395
Goldin-Meadow, S., Wein, D., and Chang, C. (1992). Assessing knowledge through gesture: using children’s hands to read their minds. Cogn. Instr. 9, 201–219. doi:10.1207/s1532690xci0903_2
Gordon, G., Breazeal, C., and Engel, S. (2015). “Can children catch curiosity from a social robot?” in Proceedings of the 10th ACM/IEEE International Conference on Human-Robot Interaction (New York, NY: ACM), 91–98.
Gorham, J. (1988). The relationship between verbal teacher immediacy behaviors and student learning. Commun. Educ. 37, 40–53. doi:10.1080/03634528809378702
Guttman, L. (1945). A basis for analyzing test-retest reliability. Psychometrika 10, 255–282. doi:10.1007/BF02288892
Ham, J., Bokhorst, R., and Cabibihan, J. (2011). “The influence of gazing and gestures of a storytelling robot on its persuasive power,” in International Conference on Social Robotics (Cham, Switzerland).
Han, J., Jo, M., Park, S., and Kim, S. (2005). “The educational use of home robots for children,” in Proceedings of the IEEE International Symposium on Robots and Human Interactive Communications RO-MAN, Vol. 2005 (Piscataway, NJ: IEEE), 378–383.
Herberg, J., Feller, S., Yengin, I., and Saerbeck, M. (2015). “Robot watchfulness hinders learning performance,” in Proceedings of the 24th IEEE International Symposium on Robot and Human Interactive Communication, RO-MAN, Vol. 2015 (Piscataway, NJ), 153–160.
Huang, C.-M., and Mutlu, B. (2013). “Modeling and evaluating narrative gestures for humanlike robots,” in Proceedings of the Robotics: Science and Systems Conference, RSS’ (Berlin), 13.
Jung, Y., and Lee, K. M. (2004). “Effects of physical embodiment on social presence of social robots,” in Proceedings of the 7th Annual International Workshop on Presence (Valencia, Spain), 80–87.
Kanda, T., Hirano, T., Eaton, D., and Ishiguro, H. (2004). Interactive robots as social partners and peer tutors for children: a field trial. Hum. Comput. Interact. 19, 61–84. doi:10.1207/s15327051hci1901&2_4
Kanda, T., Shimada, M., and Koizumi, S. (2012). “Children learning with a social robot,” in Proceedings of the 7th ACM/IEEE International Conference on Human-Robot Interaction (New York, NY: ACM), 351–358.
Kelley, D. H., and Gorham, J. (1988). Effects of immediacy on recall of information. Commun. Educ. 37, 198–207. doi:10.1080/03634528809378719
Kennedy, J., Baxter, P., and Belpaeme, T. (2015a). “Can less be more? The impact of robot social behaviour on human learning,” in Proceedings of the 4th International Symposium on New Frontiers in HRI at AISB 2015 (Canterbury).
Kennedy, J., Baxter, P., and Belpaeme, T. (2015b). Comparing robot embodiments in a guided discovery learning interaction with children. Int. J. Soc. Robot. 7, 293–308. doi:10.1007/s12369-014-0277-4
Kennedy, J., Baxter, P., and Belpaeme, T. (2015c). “The robot who tried too hard: social behaviour of a robot tutor can negatively affect child learning,” in Proceedings of the 10th ACM/IEEE International Conference on Human-Robot Interaction (New York, NY: ACM), 67–74.
Kennedy, J., Baxter, P., Senft, E., and Belpaeme, T. (2015d). “Higher nonverbal immediacy leads to greater learning gains in child-robot tutoring interactions,” in Proceedings of the International Conference on Social Robotics (Cham, Switzerland).
Kennedy, J., Baxter, P., and Belpaeme, T. (2017). Nonverbal immediacy as a characterisation of social behaviour for human-robot interaction. Int. J. Soc. Robot. 9, 109–128. doi:10.1007/s12369-016-0378-3
Kennedy, J., Baxter, P., Senft, E., and Belpaeme, T. (2016). “Heart vs hard drive: children learn more from a human tutor than a social robot,” in Proceedings of the 11th ACM/IEEE Conference on Human-Robot Interaction (Piscataway, NJ: ACM), 451–452.
Leyzberg, D., Spaulding, S., Toneva, M., and Scassellati, B. (2012). “The physical presence of a robot tutor increases cognitive learning gains,” in Proceedings of the 34th Annual Conference of the Cognitive Science Society, CogSci, Vol. 2012 (Austin, TX), 1882–1887.
McCroskey, J. C., Sallinen, A., Fayer, J. M., Richmond, V. P., and Barraclough, R. A. (1996). Nonverbal immediacy and cognitive learning: a cross-cultural investigation. Commun. Educ. 45, 200–211. doi:10.1080/03634529609379049
Mehrabian, A. (1968). Some referents and measures of nonverbal behavior. Behav. Res. Methods Instrum. 1, 203–207. doi:10.3758/BF03208096
Moore, R. K. (2012). A Bayesian explanation of the ‘Uncanny Valley’ effect and related psychological phenomena. Nat. Sci. Rep. 2:864. doi:10.1038/srep00864
Mori, M., MacDorman, K. F., and Kageki, N. (2012). The uncanny valley [from the field]. IEEE Robot. Autom. Mag. 19, 98–100. doi:10.1109/MRA.2012.2192811
Moshkina, L., Trickett, S., and Trafton, J. G. (2014). “Social engagement in public places: a tale of one robot,” in Proceedings of the 2014 ACM/IEEE International Conference on Human-Robot Interaction (New York, NY: ACM), 382–389.
Nook, E. C., Lindquist, K. A., and Zaki, J. (2015). A new look at emotion perception: concepts speed and shape facial emotion recognition. Emotion 15, 569–578. doi:10.1037/a0039166
Nussbaum, J. F. (1992). Effective teacher behaviors. Commun. Educ. 41, 167–180. doi:10.1080/03634529209378878
Reeves, B., and Nass, C. (1996). How People Treat Computers, Television, and New Media like Real People and Places. New York, NY: CSLI Publications, Cambridge University press.
Revelle, W., and Zinbarg, R. E. (2009). Coefficients alpha, beta, omega, and the glb: comments on Sijtsma. Psychometrika 74, 145–154. doi:10.1007/s11336-008-9102-z
Richmond, V., McCroskey, J., and Payne, S. (1987). Nonverbal Behavior in Interpersonal Relations. Englewood Cliffs, NJ: Prentice-Hall.
Richmond, V. P., McCroskey, J. C., and Johnson, A. D. (2003). Development of the Nonverbal Immediacy Scale (NIS): measures of self- and other-perceived nonverbal immediacy. Commun. Q. 51, 504–517. doi:10.1080/01463370309370170
Robinson, R. Y., and Richmond, V. P. (1995). Validity of the verbal immediacy scale. Commun. Res. Rep. 12, 80–84. doi:10.1080/08824099509362042
Saerbeck, M., Schut, T., Bartneck, C., and Janse, M. D. (2010). “Expressive robots in education: varying the degree of social supportive behavior of a robotic tutor,” in Proceedings of the SIGCHI Conference on Human Factors in Computing Systems, CHI’10 (New York, NY: ACM), 1613–1622.
Sung, J., Christensen, H. I., and Grinter, R. E. (2009). “Robots in the wild: understanding long-term use,” in Prcoeedings of the 4th ACM/IEEE International Conference on Human-Robot Interaction (New York, NY: IEEE), 45–52.
Szafir, D., and Mutlu, B. (2012). “Pay attention! Designing adaptive agents that monitor and improve user engagement,” in Proceedings of the SIGCHI Conference on Human Factors in Computing Systems, CHI’12 (New York, NY: ACM), 11–20.
Tanaka, F., and Matsuzoe, S. (2012). Children teach a care-receiving robot to promote their learning: field experiments in a classroom for vocabulary learning. J. Hum. Robot Interact. 1, 78–95. doi:10.5898/JHRI.1.1.Tanaka
Wainer, J., Feil-Seifer, D. J., Shell, D. A., and Mataric, M. J. (2007). “Embodiment and human-robot interaction: a task-based perspective,” in Proceedings of the 16th IEEE International Symposium on Robot and Human interactive Communication (IEEE), RO-MAN, Vol. 2007 (Piscataway, NJ), 872–877.
Wilson, J. H., and Locker, L. Jr. (2007). Immediacy scale represents four factors: nonverbal and verbal components predict student outcomes. J. Classroom Interact. 42, 4–10.
Witt, P. L., Wheeless, L. R., and Allen, M. (2004). A meta-analytical review of the relationship between teacher immediacy and student learning. Commun. Monogr. 71, 184–207. doi:10.1080/036452042000228054
Zaki, J. (2013). Cue integration a common framework for social cognition and physical perception. Perspect. Psychol. Sci. 8, 296–312. doi:10.1177/1745691613475454
Appendix
A. Robot Nonverbal Immediacy Questionnaire (RNIQ)
The following is the questionnaire used by participants in the evaluation to rate the nonverbal immediacy of the robot, based on the short-form nonverbal immediacy scale-observer report. Options are provided in equally sized boxes below each question (or equally spaced radio buttons in the online version). The options are: 1 = Never; 2 = Rarely; 3 = Sometimes; 4 = Often; 5 = Very Often. The questions are as follows:
1. The robot uses its hands and arms to gesture while talking to you
2. The robot uses a dull voice while talking to you
3. The robot looks at you while talking to you
4. The robot frowns while talking to you
5. The robot has a very tense body position while talking to you
6. The robot moves away from you while talking to you
7. The robot changes how it speaks while talking to you
8. The robot touches you on the shoulder or arm while talking to you
9. The robot smiles while talking to you
10. The robot looks away from you while talking to you
11. The robot has a relaxed body position while talking to you
12. The robot stays still while talking to you
13. The robot avoids touching you while talking to you
14. The robot moves closer to you while talking to you
15. The robot looks keen while talking to you
16. The robot is bored while talking to you
Scoring:
Step 1. Add the scores from the following items:
1, 3, 7, 8, 9, 11, 14, and 15.
Step 2. Add the scores from the following items:
2, 4, 5, 6, 10, 12, 13, and 16.
Total Score = 48 plus Step 1 minus Step 2.
Keywords: human–robot interaction, robot tutors, social behavior, child learning, nonverbal immediacy
Citation: Kennedy J, Baxter P and Belpaeme T (2017) The Impact of Robot Tutor Nonverbal Social Behavior on Child Learning. Front. ICT 4:6. doi: 10.3389/fict.2017.00006
Received: 31 December 2016; Accepted: 29 March 2017;
Published: 24 April 2017
Edited by:
Hatice Gunes, University of Cambridge, UKReviewed by:
Shin’Ichi Konomi, University of Tokyo, JapanKhiet Phuong Truong, University of Twente, Netherlands
Copyright: © 2017 Kennedy, Baxter and Belpaeme. This is an open-access article distributed under the terms of the Creative Commons Attribution License (CC BY). The use, distribution or reproduction in other forums is permitted, provided the original author(s) or licensor are credited and that the original publication in this journal is cited, in accordance with accepted academic practice. No use, distribution or reproduction is permitted which does not comply with these terms.
*Correspondence: James Kennedy, amFtZXMua2VubmVkeUBwbHltb3V0aC5hYy51aw==