- Virtual Environments and Computer Graphics Group, Department of Computer Science, University College London, London, UK
One potential application for virtual environments (VEs) is the training of spatial knowledge. A critical question is what features the VE should have in order to facilitate this training. Previous research has shown that people rely on environmental features, such as sockets and wall decorations, when learning object locations. The aim of this study is to explore the effect of varied environmental feature fidelity of VEs, the use of self-avatars, and the level of immersion on object location learning and recall. Following a between-subjects experimental design, participants were asked to learn the location of three identical objects by navigating one of the three environments: a physical laboratory or low and high detail VE replicas of this laboratory. Participants who experienced the VEs could use either a head-mounted display (HMD) or a desktop computer. Half of the participants learning in the HMD and desktop systems were assigned a virtual body. Participants were then asked to place physical versions of the three objects in the physical laboratory in the same configuration. We tracked participant movement, measured object placement, and administered a questionnaire related to aspects of the experience. HMD learning resulted in statistically significant higher performance than desktop learning. Results indicate that, when learning in low detail VEs, there is no difference in performance between participants using HMD and desktop systems. Overall, providing the participant with a virtual body had a negative impact on performance. Preliminary inspection of navigation data indicates that spatial learning strategies are different in systems with varying levels of immersion.
1. Introduction
1.1. Human Spatial Memory and Navigation
If virtual environments (VEs) are going to be used for spatial training, it is critical to understand how people explore and perceive surrounding space and the objects contained in it. However, the question of how humans learn and recall locations within an environment remains unanswered, since contrasting results have been reported. Previous findings suggest that the human brain could combine mechanisms based on geometric properties of the environment as well as self-motion. Moreover, it is not clear whether these strategies are the same when encountering real and virtual environments.
Previous research has looked at the effect of landmark configuration on search behavior. Spetch et al. (1996, 1997) analyzed the effect of expansions of an array of landmarks on the locus of search for an object presented in a location equidistant to the landmarks. Their results indicated that humans focus on locations that preserve all angles to the landmarks, preserving ratios of distances between landmarks, rather than absolute distances.
Waller et al. (2000) reported contrasting results when exploring the role of metric distances and angular information of landmarks on location learning in virtual reality (VR). Participants observed a cued location in relation to three landmarks in an immersive VE. They were then asked to return to that location during testing. Landmark configuration was modified between learning and testing to differentiate the effects of distance and inter-landmark angular information. They found that, overall, participants relied more on distance information than angular information.
Hartley et al. (2004) used a desktop VE to investigate the effect of manipulations to the boundaries of an environment on object location learning and recall in humans. Participants were presented an object in a rectangular arena, with distant features to help orient themselves. After a brief delay, they reentered the arena and were asked to mark the location where the object had been. The geometry of the environment was altered between the stages of learning and recall on some of the trials. Response data was compared with a series of spatial distributions predicted by various geometric models. They found that responses that maintained fixed distances from nearby walls were more common after expansions of the arena and for locations nearer to the boundaries, whereas responses that preserved fixed ratios between opposing walls were more common after contractions of the arena and for locations nearer to the center. A model derived from response properties of place cells in the rat hippocampus, which matches distances of the cue to the four boundaries of the arena was the best fit for their results. Hartley et al. (2000, 2004) concluded that their results were consistent with the neural representation of location in the hippocampus.
There is also evidence for spatial updating of egocentric representations (Wang and Simons, 1999; Wang and Spelke, 2002; Mou et al., 2004). Wang and Simons (1999) showed that locations of objects on a circular table can be better remembered if participants navigate around it, rather than using the equivalent rotation of the table. These results highlight the role of proprioceptive and vestibular inputs during self-motion.
If humans generate a cognitive map based on experience, awareness of the syntheticness of a computer-generated environment and the way it is explored may have an impact on spatial encoding and recall. Considering object location learning and recall as the crucial and most elementary form of training, this study explores the impact of environmental features, self-avatar, and immersion on spatial memory by asking participants to learn and recall the location of three identical objects.
1.2. Environmental Fidelity
When training in a VE, it is important to have an understanding of the technological variables that can be sacrificed without degrading learning effectiveness transfer to the real world (Waller et al., 1998; Ragan et al., 2015). One of these variables is environmental fidelity, which can be understood as the fidelity of mapping from a real-world space to a computer-generated virtual replica. A distinction can be made between two broad types of environmental cues: geometric, cues provided by environmental surfaces such as walls, and featural, non-geometric cues provided by the environment, such as color (Cheng, 1986; Gallistel, 1990; Kelly et al., 2009). Previous research has demonstrated an inclination for spatial localization to be based mainly on geometric properties of an environment, rather than featural cues (Cheng, 1986).
Although geometric fidelity of a space can be reproduced using basic 3D objects, such as planes, spheres, or cubes, high feature fidelity is not always achievable or may result in the development of computationally expensive systems. Previous studies have assessed the impact of rendering style on distance perception accuracy in virtual replicas of concurrently occupied VEs (Interrante et al., 2006; Phillips et al., 2009). These studies suggest that there are no indications of perceived compressed distances in immersive VEs where participants can be certain of them being faithful representations of their occupied space. Slater (2009) explored the effect visual realism on sense of presence in immersive VEs. Participants were exposed to a VE rendered in two levels of visual realism. They found that subjective presence was higher for the version of the VE with higher visual realism. However, Masahiro Mori’s “Uncanny Valley” hypothesis remains unanswered, since it is not clear whether higher environmental fidelity might result in training enhancement up to a point after which there might be a decrease in performance due to defect magnification.
Based on previous results, in our study, we directly compare performance resulting from learning object locations in concurrently occupied virtual and real environments. We explore learning and recall of multiple external object locations as subjective measures of spatial perception. Our research focuses on understanding which cues are necessary for the design of virtual spaces that will ensure the optimal transfer of spatial knowledge to the real world. It is the purpose of this study to explore object location learning and recall in VEs with varying levels of feature fidelity.
1.3. Self-Avatar
Slater and Usoh (1994) have suggested that the sense of presence in VEs can be enhanced by providing users with a virtual self-avatar. Related work has found that fully tracked, high fidelity virtual avatars can improve distance estimation accuracy in non-photorealistic virtual replica environments (Phillips et al., 2010). Similarly, self-embodiment in highly realistic VEs has been reported to increase accuracy in distance judgments (Ries et al., 2008). However, performance enhancement seems to be compromised when using low geometry avatar representation or single point tracking (Ries et al., 2009). Our experiment looks at the use of single point tracking virtual avatars based on head tracking in an object location memory task. The aim is to explore if a low fidelity virtual avatar can enhance performance in an object memory location task, where there is no interaction with the environment and the virtual objects in it, other than unguided, exploratory navigation. Results from this study could inform the design of future training systems in which robust avatar motion fidelity involving full body tracking is not available.
1.4. Level of Immersion
The term immersion can be understood as the objective fidelity of sensorial stimuli offered by a virtual reality (VR) system. While many applications of VR in training have used desktop environments, suitable immersive VR systems are now becoming widely available. Highly immersive VR technology potentially increases experimental and environment realism, gives researchers the ability to perform manipulations to an environment, and provides new data sources, such as body tracking, amongst other benefits (Loomis et al., 1999; Ragan et al., 2015). When being presented with a stereoscopic view and given access to self-motion cues, participants can respond realistically to situations and events (Usoh et al., 1999; Slater, 2009). Previous research has shown that display and interaction fidelity has a strong effect on strategy and performance in a VR first-person shooter game (McMahan et al., 2012). As technology moves toward augmentation of real world learning by the use of virtual tools, performance in systems with different levels of immersion must be analyzed and compared with real world learning. In the context of this research, we use the concept of level of immersion to refer to the different widely available consumer displays and navigation techniques used by the participants to explore the experimental environment. In this study, we consider real world learning the highest level of immersion, followed by head-mounted display (HMD) learning and then desktop learning. We also consider the navigation technique associated with each learning system as an inherent and crucial element of level of immersion. All learning systems as well as the corresponding navigation techniques are further detailed in Section “Experimental Design and Hypotheses.” We expect the level of immersion to have an effect on learning and recall performance (Waller et al., 1998; Bowman et al., 2009).
1.5. Experimental Design and Hypotheses
In this study, we aimed to explore the effect of level of immersion, the presence or absence of a virtual body, and the role of environmental features on object location memory. We compared placement accuracy when object locations were learnt in the real world and object locations were learnt in two distinct virtual replicas of the environment: a high detail 3D scan, where color, environmental, and geometric features are available, and a low-detail non-photorealistic replica of the shape of the room, where only geometric features were accessible. Participants learnt the position of three identical objects in one of the three environments as shown in Figure 2. Once learning was complete and after a short period of time, participants were asked to place the three objects in the real room in their original positions (see Figure 1).
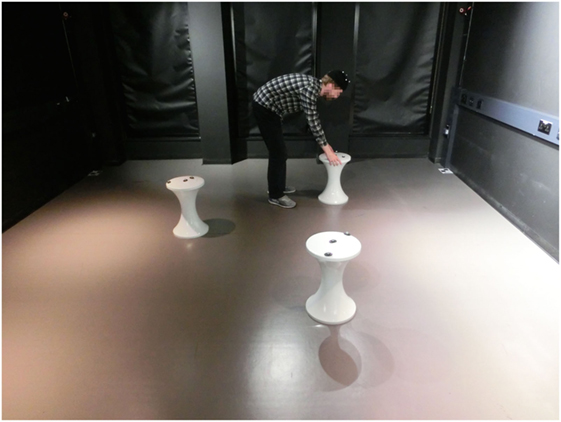
Figure 1. Participant placing the three objects in the recall stage. Plastic stools were used as objects for the study. Three retroreflective markers were attached to each stool for optical tracking.
Participants observed the VEs and learnt objects positions in different systems following a 2 × 2 × 2 design, with fidelity (high detail, low detail) as a within-subjects factor and avatar (body, no body) and level of immersion (HMD, desktop learning system) as between-subjects factors. Real world learning in the real environment with physical objects was treated as an additional learning system. Table 1 contains a summary of the mixed design experimental conditions.
Participants in the learning system conditions with a virtual body were assigned a single point tracking avatar model based on head tracking. In other words, a fixed mannequin was placed underneath the participant’s head position, with no other reference points or animated movements. Participants learning in the real world and in the HMD learning system conditions were able to explore the space by physically walking around the room. Participants learning in the desktop system condition were able to navigate the room by using keyboard and mouse control, to change position and view, respectively. All participants completed the learning stage in one of the three learning systems and then placed the physical objects in the real world (see “Procedure”). In addition to the between-subjects learning system variable, one variable was manipulated within participants learning in the desktop and HMD systems: VE fidelity. Participants in the desktop and HMD learning conditions repeated the task two times, one time in the low detail VE and one time in the high detail VE. The order in which participants experienced the low detail and high detail VEs was altered, ensuring that the two possible combinations were tested equally. Participants learning in the real world repeated the same task two times, always in the real environment. The dependent variable was placement error, or the absolute distance between participant response and original object position, based on x- and y-coordinates, in meters. We also recorded the navigation paths of all participants when learning and recalling object locations.
We hypothesized that providing optic flow information, natural locomotion, and access to idiothetic cues in an HMD would promote higher similarity with real world learning in terms of placement accuracy and navigation. Previous results have indicated that training in a virtual environment of relatively low fidelity allows people to develop useful representations of large-scale navigable space (Waller et al., 1998), contrary to the thought that increasing overall fidelity of a simulator will lead to increases in transfer (Hays and Singer, 1989). Regarding the presence and absence of a single point tracked avatar, we intent to further replicate and verify the results of previous studies in which this type of low motion fidelity virtual body has degraded performance (Ries et al., 2009). Because of the availability of geometric as well as environmental cues, we expected learning in the high detail VE to result in greater accuracy than learning in the low detail VE when placing the objects in their original positions. We predicted that spatial learning and recall in systems with higher level of immersion would result in comparable resemblance with real world learning.
2. Materials and Methods
2.1. Materials
The experiment was conducted in a lab at University College London. The laboratory consisted of a 6 m long × 4 m wide × 3 m high open space. The high detail VE was comprised of a high fidelity 3D laser scan point cloud of the room with textures derived from photographs, rendered with a GPU-based point cloud renderer. 3D scanning was performed with a Faro Focus 3D S120 laser scanner. The low detail VE was modeled using diffuse shaded planes to reproduce the geometric shape of the laboratory. Figure 2 shows screen captures of the low detail VE, high detail VE, and real room from the same viewport. All environments were rendered at scale 1:1 in Unity at 60FPS without VSync and a vertical FOV of 60° for the desktop system and 60FPS in each eye for the HMD on an Intel Xeon E7 CPU, with 16 GB RAM and Nvidia GTX 680 GPU running Windows 7. The physical room contained a table and a computer that was not included in the model. Previous research has found no effect of presence and absence of rendered furniture on participant distance estimation (Interrante et al., 2008).
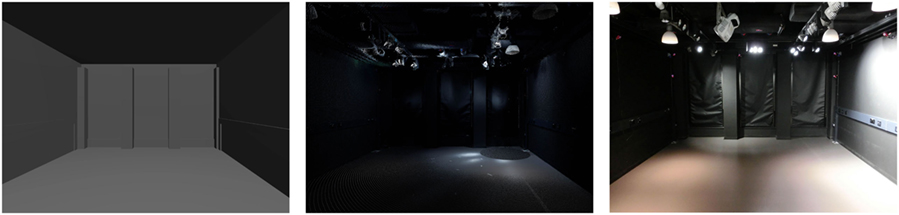
Figure 2. Screen captures of the low detail VE (left), high detail VE (middle), and photograph of the real environment (right). The photograph of the real environment was taken under lighting conditions that were different to the experimental lighting conditions.
Head tracking and object positional data were logged with a NaturalPoint OptiTrack motion capture system using twelve Flex 3 cameras and retroreflective markers, at a sampling rate of 60 Hz. The measured mean tracking error was 3 mm. A 27″ Dell U2713HM monitor and an Oculus Rift Development Kit 2 (DK2) were used as displays for the desktop and HMD learning conditions, respectively. High fidelity single point tracking virtual avatars, based on head tracking, were used in the corresponding desktop body and HMD body conditions. A female and male avatar model were obtained from the Rocketbox® Library (Havok, 2014). These were preprocessed to remove the heads before being included in the virtual scene. The avatars were not animated and remained in an idle position throughout the task. An Epson EB-585Wi projector was mounted in the ceiling of the laboratory, aligned with the room, and projecting onto the ground. It was used to place the physical versions of the objects in their corresponding positions for real-world learning. The objects used in the study were three identical white Tam Tam plastic stools from Habitat. These stools are lightweight, easy to carry, and rotationally symmetrical along the vertical axis, providing no orientation cues to the participant. The stools have a diameter of 31 cm at the widest section and a height of 45 cm.
2.2. Participants
A total of 20 participants (9 females, 11 males; average age 26 years, SD = 5.3) were recruited from the student and staff population at University College London. All participants signed a consent form and the study was approved by the University College London Research Ethics Committee (project ID: 6708/002). Participants were paid £10 for participation. They were assigned to the different experimental conditions based on individual results for a standard spatial ability test to avoid any possible bias between groups (Bodner and Guay, 1997).
2.3. Procedure
The experimental task consisted of two phases, before and during the lab session. Table 2 shows an outline of the experimental task. Participants performed all their trials in the same learning system condition. Before the lab session, participants were asked to read an online information sheet that introduced the experimental task. They were asked to read and sign an online informed consent form and asked to complete a digital version of a standard spatial ability test as well as a background questionnaire.
During the lab session, participants were asked to sign a paper copy of the consent form and asked to read an information sheet with written instructions describing the experimental task. Participants were asked to switch off their mobile phones and were introduced into the lab. No practice trials were done, and participants were not given feedback on their performance throughout the experiment.
The experimental task consisted of two trials, each with a learning and a recall stage. The learning stage involved viewing the three virtual objects in the real room or one of the low and high detail VEs in one of the three learning system conditions: real world, desktop, or HMD. In the recall stage, participants were asked to place the three physical objects as they remembered them from the learning stage into the real room. No further information was given, and participants were asked to try their best if they were in doubt as to where the object’s original position was. There was no time limit for the learning and recall stages, and participants were able to freely navigate the environment. Participants could navigate through all objects of the environment, but not through the environment boundaries. An experimenter was present at all times during the experimental task to manage cables and provide guidance on the different experimental stages.
Participants learning in the HMD and desktop learning systems (16 participants) performed the two trials, each corresponding to one of the two versions of the VE in the learning stage: high detail and low detail. Participants experienced the two VEs in different orders, ensuring that the two possible combinations were tested equally. Participants learning in the real world (4 participants) performed the same trial twice, always learning in the real room. In each trial, and for each participant, all three objects were randomly arranged on a conceptual 5 × 5 grid, avoiding straight line configurations. Subjects could not see the grid in the environment and were asked to ignore retroreflective markers on the stools, which were used to track and identify the stools for data collection.
After each trial, participants were asked to complete a short online questionnaire measuring examination, confidence, difficulty, movement, application, and observation (see Table 3). After the two trials were completed, they were interviewed regarding individual strategies used throughout the experimental task.
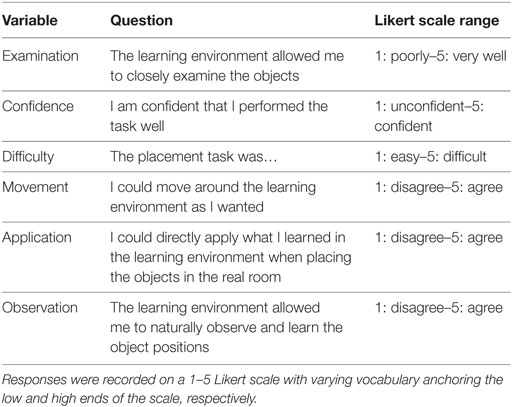
Table 3. Post-trial questionnaire related to several aspects of the experience: examination, confidence, difficulty, movement, application, and observation.
3. Results
3.1. Object Placement
Tracked object placement data were used to calculate the Euclidean distance, referred to as placement error, between object positions as placed by participants in the recall stage and original object positions. Figure 3 shows mean placement errors for all learning system conditions. For statistical analysis, the mean placement error was calculated from the error of each of the three objects for all trials.
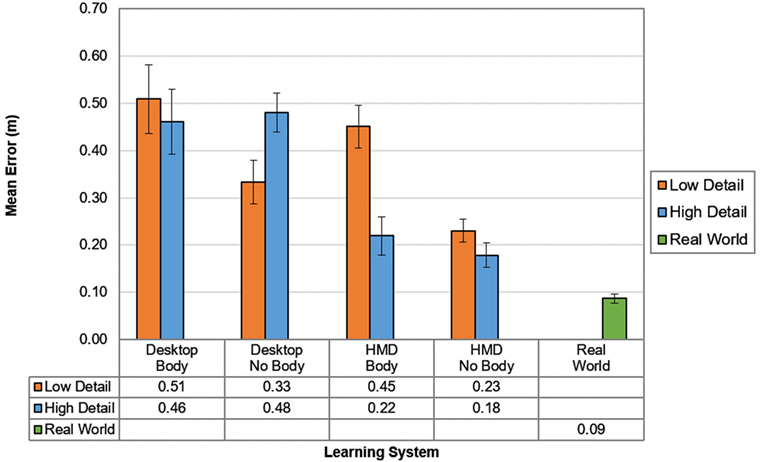
Figure 3. Mean placement errors in all learning system conditions for real world (green), high detail (blue), and low detail (orange) VEs in meters. Error bars show SEs.
A three-way mixed ANOVA with fidelity (high detail, low detail) as a within-subjects factor and avatar (body, no body) and level of immersion (HMD, desktop learning system) as between-subjects factors was run. There were no outliers in the data, as assessed by inspection of a box plot. There was homogeneity of variances for both high detail placement errors (p = 0.257) and low detail placement errors (p = 0.143), as assessed by Levene’s test for equality of variances. Results showed a statistically significant two-way interaction between fidelity and level of immersion, F(1, 12) = 6.3, p = 0.027, and fidelity and avatar, F(1, 12) = 6.3, p = 0.027.
Statistical significance of simple main effects was accepted at a Bonferroni-adjusted alpha level of.025. There was a statistically significant simple main effect of avatar for the low detail environment, F(1, 12) = 6.453, p = 0.026, but not for the high detail environment, F(1, 12) = 0.017, p = 0.899. All pairwise comparisons were performed for statistically significant simple main effects. Bonferroni corrections were made with comparisons within each simple main effect considered a family of comparisons. Adjusted p-values are reported. Mean placement error was lower when an avatar was present than when an avatar was absent when learning in the low detail environment, with a mean difference of −0.20 (95% CI, −0.372 to −0.028), p = 0.026. There was a statistically significant simple main effect of learning system for the high detail environment, F(1, 12) = 16.423, p = 0.002, but not for the low detail environment, F(1, 12) = 1.098, p = 0.315. Mean placement error was lower when learning with an HMD system than with a desktop system, when learning in the high detail environment, with a mean difference of −0.083 (95% CI, −0.254 to −0.089), p = 0.002.
A Kruskal–Wallis H test showed that there was an overall statistically significant difference in placement error between the different learning systems, χ2(2) = 56.452, p < 0.001, with a mean rank placement error score of 84.15 for desktop learning, 57.53 for HMD learning, and 19.15 for real-world learning. When comparing the three system conditions, real-world learning resulted in statistically significant lower placement error (M = 0.09, SD = 0.04), followed by HMD learning (M = 0.27, SD = 0.16), and desktop learning (M = 0.45, SD = 0.21), respectively. No statistically significant differences were found between the two trials.
3.2. Questionnaire
A one-way between-subjects ANOVA was performed on questionnaire responses for desktop body, desktop no body, HMD body, and HMD no body learning system conditions, for high and low detail VEs. Results show a large number of mixed significant interactions with no overarching trend due to the limited number of repetitions.
3.3. Navigation
Tracking results, shown in Figure 4, indicate contrasting movement patterns in real world, HMD, and desktop learning system conditions. Qualitative inspection of data suggests that participants learning in the real world and HMD systems primarily navigated areas within the boundaries of the conceptual 5 × 5 object grid whereas participants learning in the desktop computer mainly navigated areas outside the boundaries of the conceptual 5 × 5 object grid. The mean percentage of time spent navigating inside and outside the conceptual 5 × 5 object grid was calculated for each learning system and is shown in Figure 5.
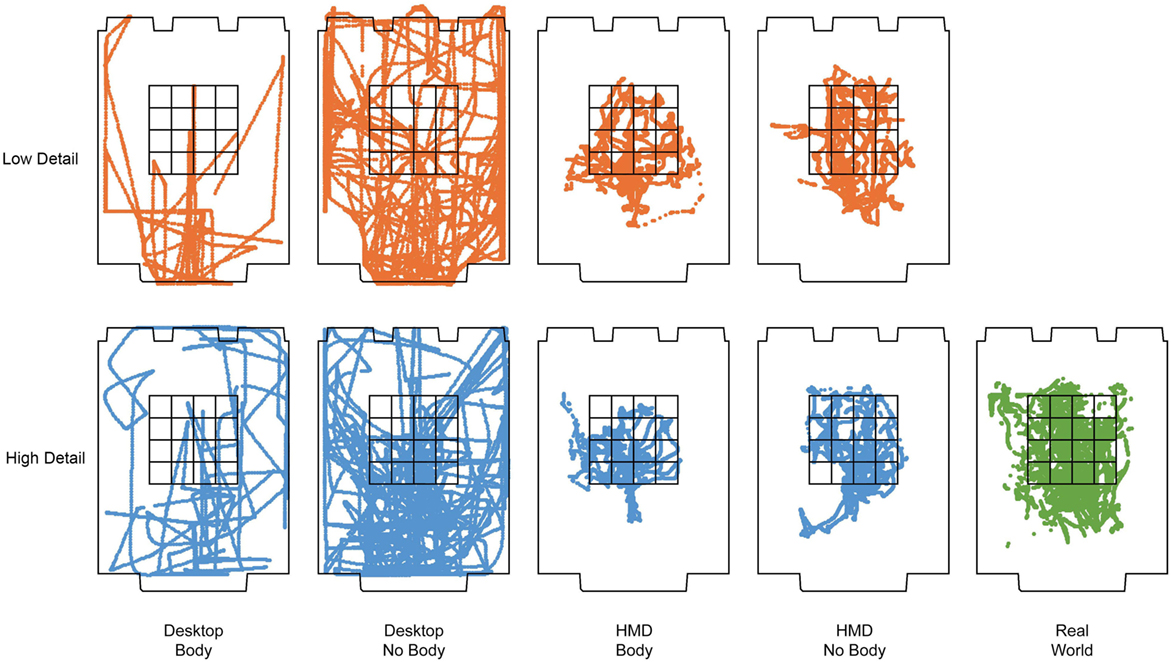
Figure 4. Learning stage 2D (XY plane) tracked navigation trajectories for all participants in each learning condition for real world (green), high detail (blue), and low detail (orange) VEs. Each point represents an XY position at a sampling rate of 60 Hz. All intersections in the 5 × 5 conceptual grid represent a possible object position. The conceptual 5 × 5 object grid was invisible to participants.
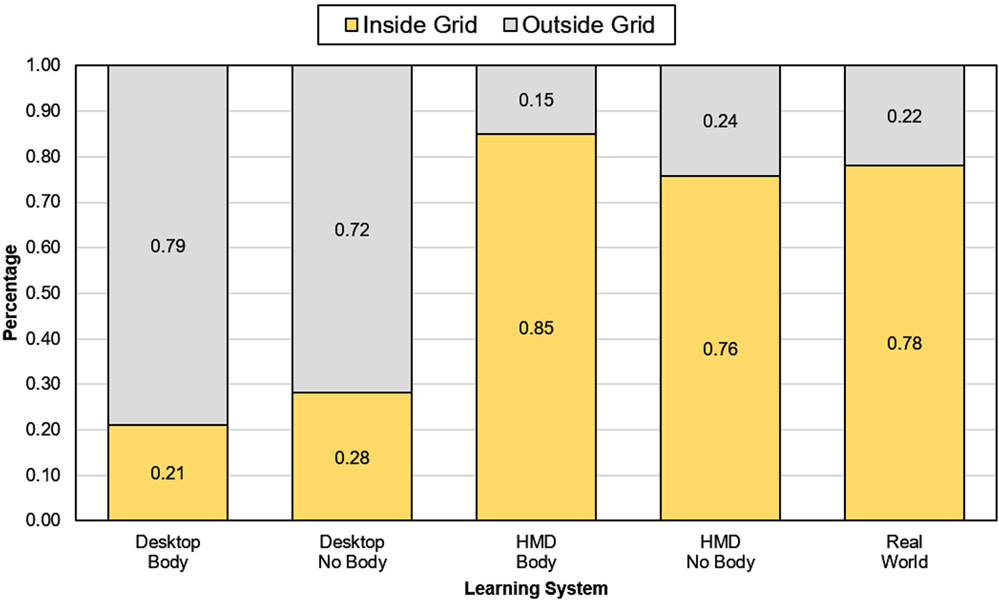
Figure 5. Mean percentage of navigation time spent outside (gray) and inside (yellow) the conceptual 5 × 5 object grid for all participants in each learning condition during the learning stage.
A one-way between-subjects ANOVA was conducted to compare the effect of learning system on the percentage of time spent navigating inside the conceptual 5 × 5 object grid in desktop, HMD, and real-world learning system conditions. There was a significant effect of learning system on percentage time spent navigating inside the conceptual 5 × 5 object grid at the p < 0.05 level [F(2, 39) = 371.991, p < 0.001]. Post hoc comparisons using the Tukey HSD test indicated that the mean percentage spent navigating inside the conceptual 5 × 5 object grid for desktop learning (M = 0.24, SD = 0.06) was significantly lower than the mean percentage spent navigating inside the conceptual 5 × 5 object grid for HMD learning (M = 0.80, SD = 0.08) and real world learning (M = 0.78, SD = 0.04). No significant difference was found between HMD and real-world learning.
To further illustrate differences in navigation strategies, we created cluster heat maps of the time spent in each region of the room for each of the system conditions: Desktop (left), HMD (middle), and real world (right), shown in Figure 6. These results show different spatial navigation strategies between desktop and HMD learning strategies, where the former tended to access areas toward the far end of the room and the latter tended to navigate areas clustered within the object grid along the Y axis. Along the X axis, the range of positions accessed by participants learning in the desktop system was wider than the range of movement performed by participants learning in the HMD system. HMD navigation was not only different from desktop navigation but also qualitatively very similar to real world navigation during learning.
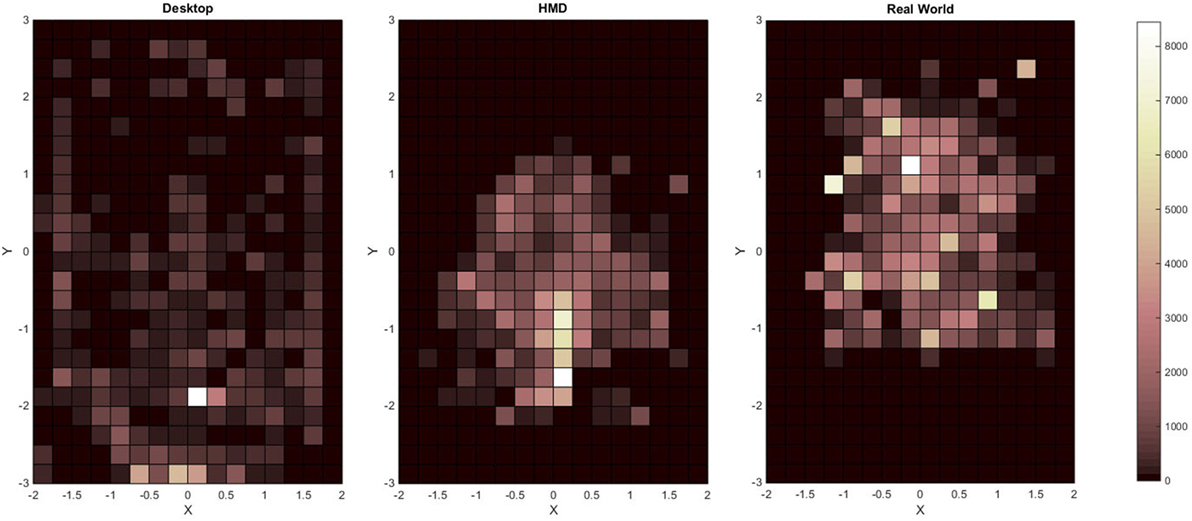
Figure 6. Cluster heat maps of the time spent in each region of the room for each of the system conditions: desktop (left), HMD (middle), and real world (right). The columns represent X-axis, and the rows represent Y-axis positions (in meters). Each cell is colorized based on the level of counts of the head location (for all participants) in each region during learning.
4. Discussion
This study analyses object location memory transfer from VR to the real world. It extends previous work on spatial perception in VEs (Ellis and Menges, 1998; Waller et al., 1998; Loomis and Knapp, 2003; Thompson et al., 2004; Interrante et al., 2008; Ries et al., 2008; Phillips et al., 2009, 2010; Mohler et al., 2010; Lin et al., 2011) by suggesting an experimental task in which participants are asked to learn and recall a series of object configurations in concurrently occupied virtual and real environments.
Our results illustrate that HMD learning resulted in statistically significant higher performance followed by desktop learning. Our analysis suggests that availability of environmental features in VEs can enhance object location memory under certain setups. The overall negative effect of the self-avatar indicates that single point tracked virtual bodies may not be sufficient to increase performance in this experimental task. Specifically, the use of self-avatar in HMD body learning impaired placement accuracy. Single point tracking caused the virtual self-avatar to appear in front of the participant’s real body if they leaned forward, partially occluding some of the available environmental features. The degradation in performance might have been because the virtual body occluded features in the environment that the participant could have attended to. This might then have forced a change to a different strategy for learning one or more object placements. Moreover, the lack of motion fidelity provided by single point virtual bodies might interfere with presence in VEs.
The results on navigation strategies seem promising. Similar to participants learning in the real world, participants learning in the HMD system mainly navigated areas within the boundaries of the conceptual 5 × 5 object grid, whereas participants learning in the desktop system primarily explored areas outside the boundaries of the conceptual 5 × 5 object grid. This may suggest that, when learning object locations in less immersive systems, users navigate toward the environment boundaries to obtain more global views of the scene. In addition, the range of areas of the room accessed by participants learning in the desktop system was wider than the range of areas of the room participants learning in the real world and HMD system in the X and Y axis. Although differences in navigation in systems with varying levels of immersion have been reported (Ruddle et al., 1999), further exploration is required to understand the trajectories selected by users when learning object locations.
One of the limitations of the work presented here is the relatively low number of participants. A larger population sample is needed to further validate our results as well as to explore the effect of more complex self-avatars with higher motion fidelity on spatial memory. It would also allow us to analyze navigation trajectories in more detail, exploring the regions visited by participants in relation to the object locations and features of the environment. Other experimental tasks comparing object location memory in systems with varying levels of immersion are required to confirm whether our results are generalizable.
5. Conclusion
In this paper, we present a study on object location memory. The experimental task involves several judgments, including distance estimation, and it is not clear exactly what strategies participants use to learn object locations (Hartley et al., 2004). Previous work has shown that distance estimation is impaired within immersive VR, although including a self-avatar and increasing confidence in fidelity can reduce this impairment (Loomis and Knapp, 2003; Thompson et al., 2004; Interrante et al., 2006, 2008; Ries et al., 2008, 2009; Phillips et al., 2009, 2010; Mohler et al., 2010; Lin et al., 2011). Our results suggest that the level of immersion is extremely important for accurate object location learning and recall, and that higher environmental fidelity may reinforce learning transfer from VEs to the real world. However, most importantly, they indicate that providing users with a virtual body can interfere with successful completion of the task. This motivates studies of more complex self-representations.
We believe that the main outcomes of this study could be generalized to other spatial learning scenarios and assist experts in the design of training simulations related to spatial memory, where trainees are required to remember component or tool locations as part of the task. Overall, our results denote that HMD training resembles real world training more than desktop learning, related to higher object location memory accuracy. However, desktop training applications can be suitable and offer acceptable results when precise location learning accuracy is not required. Regarding self-avatars, our results suggest that a low fidelity avatar representation can degrade object location memory. In our experimental task, this observation is particularly important when the training transfer takes place from a low fidelity VE, where only basic geometric cues are available, to the real world equivalent.
Author Contributions
MM-L wrote the code, ran the study, analyzed the data, and wrote the paper. AS supervised MM-L through each stage of the work including planning and running the study, analyzing the data, and writing the paper.
Conflict of Interest Statement
The authors declare that the research was conducted in the absence of any commercial or financial relationships that could be construed as a potential conflict of interest.
References
Bodner, G. M., and Guay, R. B. (1997). The purdue visualization of rotations test. Chem. Educ. 2, 1–17. doi: 10.1007/s00897970138a
Bowman, D. A., Sowndararajan, A., Ragan, E. D., and Kopper, R. (2009). “Higher levels of immersion improve procedure memorization performance,” in Proceedings of the 15th Joint Virtual Reality Eurographics Conference on Virtual Environments, Lyon.
Cheng, K. (1986). A purely geometric module in the rat’s spatial representation. Cognition 23, 149–178. doi:10.1016/0010-0277(86)90041-7
Ellis, S. R., and Menges, B. M. (1998). Localization of virtual objects in the near visual field. Hum. Factors 40, 415–431. doi:10.1518/001872098779591278
Hartley, T., Burgess, N., Lever, C., Cacucci, F., and O’Keefe, J. (2000). Modeling place fields in terms of the cortical inputs to the hippocampus. Hippocampus 10, 369–379. doi:10.1002/1098-1063(2000)10:4<369::AID-HIPO3>3.0.CO;2-0
Hartley, T., Trinkler, I., and Burgess, N. (2004). Geometric determinants of human spatial memory. Cognition 94, 39–75. doi:10.1016/j.cognition.2003.12.001
Havok. (2014). Rocketbox® Libraries. Available at: http://rocketbox.de/
Hays, R., and Singer, M. (1989). Simulation Fidelity in Training System Design: Bridging the Gap between Reality and Training. New York, NY: Springer-Verlag.
Interrante, V., Ries, B., and Anderson, L. (2006). “Distance perception in immersive virtual environments, revisited,” in Virtual Reality Conference, 2006 (Alexandria, VA: IEEE), 3–10.
Interrante, V., Ries, B., Lindquist, J., Kaeding, M., and Anderson, L. (2008). Elucidating factors that can facilitate veridical spatial perception in immersive virtual environments. Presence (Camb) 17, 176–198. doi:10.1162/pres.17.2.176
Kelly, J. W., McNamara, T. P., Bodenheimer, B., Carr, T. H., and Rieser, J. J. (2009). Individual differences in using geometric and featural cues to maintain spatial orientation: cue quantity and cue ambiguity are more important than cue type. Psychon. Bull. Rev. 16, 176–181. doi:10.3758/PBR.16.1.176
Lin, Q., Xie, X., Erdemir, A., Narasimham, G., McNamara, T. P., Rieser, J., et al. (2011). “Egocentric distance perception in real and HMD-based virtual environments: the effect of limited scanning method,” in Proceedings of the ACM SIGGRAPH Symposium on Applied Perception in Graphics and Visualization, APGV ’11 (New York, NY: ACM), 75–82.
Loomis, J. M., Blascovich, J. J., and Beall, A. C. (1999). Immersive virtual environment technology as a basic research tool in psychology. Behav. Res. Methods Instrum. Comput. 31, 557–564. doi:10.3758/BF03200735
Loomis, J. M., and Knapp, J. M. (2003). Visual perception of egocentric distance in real and virtual environments. Virtual Adapt. Environ. 11, 21–46. doi:10.1201/9781410608888.pt1
McMahan, R. P., Bowman, D. A., Zielinski, D. J., and Brady, R. B. (2012). Evaluating display fidelity and interaction fidelity in a virtual reality game. IEEE Trans. Vis. Comput. Graph. 18, 626–633. doi:10.1109/TVCG.2012.43
Mohler, B. J., Creem-Regehr, S. H., Thompson, W. B., and Bulthoff, H. H. (2010). The effect of viewing a self-avatar on distance judgments in an HMD-based virtual environment. Presence (Camb) 19, 230–242. doi:10.1162/pres.19.3.230
Mou, W., McNamara, T. P., Valiquette, C. M., and Rump, B. (2004). Allocentric and egocentric updating of spatial memories. J. Exp. Psychol. Learn. Mem. Cogn. 30, 142. doi:10.1037/0278-7393.30.1.142
Phillips, L., Ries, B., Interrante, V., Kaeding, M., and Anderson, L. (2009). “Distance perception in NPR immersive virtual environments, revisited,” in Proceedings of the 6th Symposium on Applied Perception in Graphics and Visualization (Chania: ACM), 11–14.
Phillips, L., Ries, B., Kaeding, M., and Interrante, V. (2010). “Avatar self-embodiment enhances distance perception accuracy in non-photorealistic immersive virtual environments,” in Virtual Reality Conference (VR), 2010 IEEE (Boston, MA: IEEE), 115–1148.
Ragan, E. D., Bowman, D. A., Kopper, R., Stinson, C., Scerbo, S., and McMahan, R. P. (2015). Effects of field of view and visual complexity on virtual reality training effectiveness for a visual scanning task. IEEE Trans. Vis. Comput. Graph. 21, 794–807. doi:10.1109/TVCG.2015.2403312
Ries, B., Interrante, V., Kaeding, M., and Anderson, L. (2008). “The effect of self-embodiment on distance perception in immersive virtual environments,” in Proceedings of the 2008 ACM Symposium on Virtual Reality Software and Technology (Bordeaux: ACM), 167–170.
Ries, B., Interrante, V., Kaeding, M., and Phillips, L. (2009). “Analyzing the effect of a virtual avatar’s geometric and motion fidelity on ego-centric spatial perception in immersive virtual environments,” in Proceedings of the 16th ACM Symposium on Virtual Reality Software and Technology (ACM), 59–66.
Ruddle, R. A., Payne, S. J., and Jones, D. M. (1999). Navigating large-scale virtual environments: what differences occur between helmet-mounted and desk-top displays? Presence (Camb) 8, 157–168. doi:10.1162/105474699566143
Slater, M. (2009). Place illusion and plausibility can lead to realistic behaviour in immersive virtual environments. Philos. Trans. R Soc. B Biol. Sci. 364, 3549–3557. doi:10.1098/rstb.2009.0138
Slater, M., and Usoh, M. (1994). Body centred interaction in immersive virtual environments. Artif. Life. Virtual Real. 1, 125–148.
Spetch, M. L., Cheng, K., and MacDonald, S. E. (1996). Learning the configuration of a landmark array: I. touch-screen studies with pigeons and humans. J. Comp. Psychol. 110, 55. doi:10.1037/0735-7036.110.1.55
Spetch, M. L., Cheng, K., MacDonald, S. E., Linkenhoker, B. A., Kelly, D. M., and Doerkson, S. R. (1997). Use of landmark configuration in pigeons and humans: II. generality across search tasks. J. Comp. Psychol. 111, 14. doi:10.1037/0735-7036.111.1.14
Thompson, W. K., Willemsen, P., Gooch, A., Creem-Regehr, S. H., Loomis, J. M., Beall, A. C., et al. (2004). Does the quality of the computer graphics matter when judging distances in visually immersive environments? Presence 13, 560–571. doi:10.1162/1054746042545292
Usoh, M., Arthur, K., Whitton, M. C., Bastos, R., Steed, A., Slater, M., et al. (1999). “Walking, walking-in-place, flying, in virtual environments,” in Proceedings of the 26th Annual Conference on Computer Graphics and Interactive Techniques, SIGGRAPH ’99 (New York, NY: ACM Press/Addison-Wesley Publishing Co.), 359–364. doi:10.1145/311535.311589
Waller, D., Hunt, E., and Knapp, D. (1998). The transfer of spatial knowledge in virtual environment training. Presence Teleop. Virt. Environ. 7, 129–143. doi:10.1162/105474698565631
Waller, D., Loomis, J. M., Golledge, R. G., and Beall, A. C. (2000). Place learning in humans: the role of distance and direction information. Spat. Cogn. Comput. 2, 333–354. doi:10.1023/A:1015514424931
Wang, R. F., and Simons, D. J. (1999). Active and passive scene recognition across views. Cognition 70, 191–210. doi:10.1016/S0010-0277(99)00012-8
Keywords: virtual environments, spatial memory, immersion, self-avatar, environmental fidelity
Citation: Murcia-López M and Steed A (2016) The Effect of Environmental Features, Self-Avatar, and Immersion on Object Location Memory in Virtual Environments. Front. ICT 3:24. doi: 10.3389/fict.2016.00024
Received: 23 May 2016; Accepted: 17 October 2016;
Published: 03 November 2016
Edited by:
Eric D. Ragan, Texas A&M University, USACopyright: © 2016 Murcia-López and Steed. This is an open-access article distributed under the terms of the Creative Commons Attribution License (CC BY). The use, distribution or reproduction in other forums is permitted, provided the original author(s) or licensor are credited and that the original publication in this journal is cited, in accordance with accepted academic practice. No use, distribution or reproduction is permitted which does not comply with these terms.
*Correspondence: María Murcia-López, bWFyaWEubXVyY2lhLjEzQHVjbC5hYy51aw==