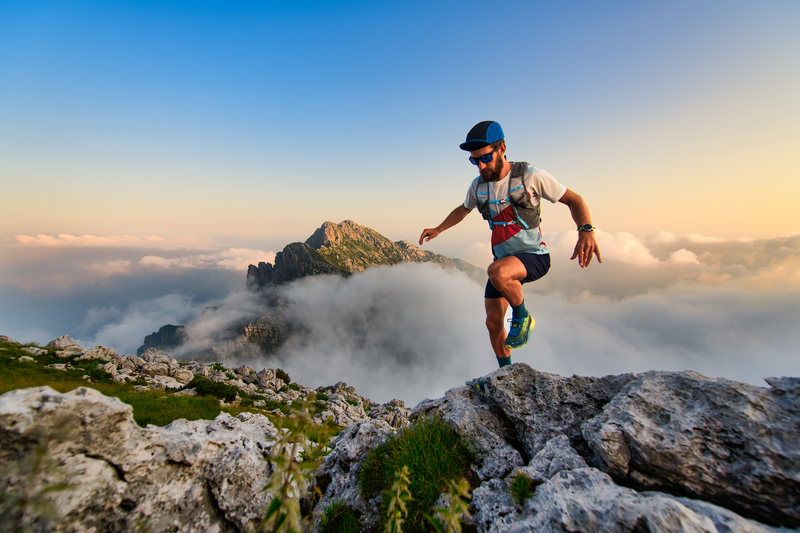
94% of researchers rate our articles as excellent or good
Learn more about the work of our research integrity team to safeguard the quality of each article we publish.
Find out more
ORIGINAL RESEARCH article
Front. ICT , 18 May 2015
Sec. Human-Media Interaction
Volume 2 - 2015 | https://doi.org/10.3389/fict.2015.00007
Communication is characterized by speakers’ dynamic adaptations and coordination of both linguistic and non-verbal behaviors. Understanding this phenomenon of alignment and its underlying mechanisms and processes in both human–human and human–computer interactions is of particular importance when building artificial interlocutors. In this paper, we contribute to further explorations of the still fragmentary alignment space with two controlled experiments on lexical and gestural alignment. Our results show, on the one hand, that human interlocutors align their lexical choice and gesture handedness in similar ways when interacting with real versus virtual humans. On the other hand, we found, however, also subtle differences. We discuss our findings in terms of a dynamic interplay of multiple components (automatic and strategic) and mechanisms (communicative and social).
Virtual humans or conversational agents are computer-generated characters with the abilities for using natural language, conducting dialog, expressing emotions, and showing non-verbal behaviors. Application areas of virtual humans are multifold, for instance, as pedagogical agents in education, in health intervention, or in entertainment. While these agents have advanced in both capability and applicability they, however, do not yet exploit their full potential and still lack much of the complexity and subtlety of human communicative behavior [cf. Krämer and Bente (2010) and Hartholt et al. (2013)]. In particular, human–agent interaction does not yet achieve the smooth, dynamic adaptations, and coordinations that are ubiquitous in human face-to-face communication.
A large body of evidence demonstrates that dialog partners mutually adapt or align with each other regarding both verbal and non-verbal behaviors – a phenomenon often termed as the “chameleon effect” (Chartrand and Bargh, 1999). Linguistically, interlocutors tend to align their lexical choices (Clark and Wilkes-Gibbs, 1986; Garrod and Anderson, 1987; Brennan, 1996; Brennan and Clark, 1996), acoustic–prosodic features (Nishimura et al., 2008; Kousidis and Dorran, 2009; Lee et al., 2010; Levitan and Hirschberg, 2011; Vaughan, 2011; Pardo et al., 2012; Truong and Heylen, 2012), or syntactic structures (Branigan et al., 2000; Gries, 2005; Messenger et al., 2012; Rowland et al., 2012; Reitter and Moore, 2014). Likewise, interaction partners have been found to align non-verbally, e.g., in their facial expressions (Dimberg, 1982; Likowski et al., 2012), manual gestures (Kimbara, 2006, 2008; Parrill and Kimbara, 2006; Holler and Wilkin, 2011; Bergmann and Kopp, 2012; Mol et al., 2012), or body postures (Chartrand and Bargh, 1999). Many of these features are aligned at the same time, as recently shown in an extensive study by Louwerse et al. (2012).
Terminologies describing this phenomenon are diverse, for instance, convergence (Leiser, 1989; Kousidis and Dorran, 2009; Pardo et al., 2012), accommodation (Giles et al., 1991; Staum Casasanto et al., 2010), interactional synchrony (Bernieri and Rosenthal, 1991), entrainment (Branigan et al., 2000; Lee et al., 2010; Levitan and Hirschberg, 2011), mimicry (Chartrand and Bargh, 1999; Van Baaren et al., 2004; Kimbara, 2006; Scissors et al., 2008; Holler and Wilkin, 2011), adaptation (Darves and Oviatt, 2002; Nilsenová and Nolting, 2010; Mol et al., 2012), repetition (Cleland and Pickering, 2003; McLean et al., 2004), or alignment (Pickering and Garrod, 2004; Branigan et al., 2010; Truong and Heylen, 2012; Reitter and Moore, 2014) are used. See Kopp (2010) for a detailed review of the terminology.
The mechanisms and processes underlying the phenomenon of alignment, however, are not yet fully understood. Basically, three different views have been proposed. The first one holds that alignment is the result of more or less controlled audience design and utilized by speakers to increase the probability of communicative success. Support for this view comes from evidence that alignment strength varies as a function of beliefs about the dialog partner (Brennan and Clark, 1996; Branigan et al., 2011). The second account views alignment as arising automatically and inevitably from direct perception–behavior links (Chartrand and Bargh, 1999) or automatic priming processes (Pickering and Garrod, 2004, 2006). Finally, alignment is discussed as a means of creating “social glue.” Many studies have demonstrated effective consequences in terms of increased rapport, more positive ratings of interlocutors (Maurer and Tindall, 1983; Bradac et al., 1988; Chartrand and Bargh, 1999; Lakin and Chartrand, 2003), higher tips for waitresses (Van Baaren et al., 2003), or greater prosocial behavior such as helpfulness and generosity (Van Baaren et al., 2004). Notably, these different accounts are not mutually exclusive (Branigan et al., 2010). The balance between the distinct components might vary according to the communicative context (Reitter and Moore, 2014), just as the different mechanisms might be at work simultaneously at different levels of processing (Bergmann and Kopp, 2012).
Crucially, alignment is also an important phenomenon in human–computer interaction (HCI). On the one hand, computer systems that align to their users were found to be evaluated differently by their users. Especially, the social component of alignment seems to apply in HCI when systems are anthropomorphized in some way. Respective effects have been reported in terms of higher likeability ratings of speech-based dialog systems (Nass and Lee, 2001; Ward and Nakagawa, 2002) or as higher perceived social intelligence (André et al., 2004; Johnson et al., 2004), increased likability (Kühne et al., 2013), or increased persuasiveness (Bailenson and Yee, 2005) of virtual humans. The latter authors conclude that there is great potential for embodied agents to be effective “digital chameleons.”
On the other hand, people have also been shown to align to machines. Suzuki and Katagiri (2007) demonstrated alignment of loudness and reaction latency in response to computer-generated speech. Lexical alignment in human–computer interaction has been shown by Brennan (1996) as well as in a series of experiments by Branigan et al. (2011). The latter study has, in particular, addressed the question how much alignment occurs in HCI as compared to human–human dialog.
Participants were led to believe that they were interacting with either a computer or a human interlocutor, but, in fact, always interacted with a computer program that executed scripted utterances. In both text- and speech-based dialog, participants tended to repeat their partners choice of referring expression. Strikingly, they showed a stronger tendency to align with assumed “computer” versus “human” partners. Moreover, the tendency to align was strongest when the computer was presented as less capable. Branigan et al. (2011) conclude that users align lexically to machines to facilitate communicative success, based on beliefs about the communication partner. Along the same lines, Bell et al. (2003) found in a Wizard-of-Oz setting that speakers adapt their speech rate (fast versus slow) when interacting with an animated character in a simulated spoken dialog system. Speakers were found to align with the character, producing slow speech in response to the “slow computer” and fast speech in response to the “fast computer.” Oviatt et al. (2004) likewise showed that children’s speech aligns with that of an animated character, whose synthesized voices varied in their acoustic properties. Children were found to consistently align in amplitude and pause structure and such adaptations occurred bidirectionally and dynamically: children rapidly adapted their speech, for example, by inserting more pauses when interacting with an introverted interlocutor, while inserting fewer pauses when subsequently interacting with an extroverted interlocutor, even within the same conversation. More recently, Koulouria et al. (2014) looked at lexical alignment in dialogs between humans and humans-pretending-to-be-robots. They found increasing alignment as the interaction progressed (vocabulary range decreased), with higher error rates associated with less alignment, and higher user satisfaction associated with higher alignment.
Von der Pütten et al. (2011) demonstrated that participants, who were interviewed by a virtual agent in a Wizard-of-Oz setting, accommodate to the virtual interviewer with regard to wordiness. Kühne et al. (2013) in another study found that humans aligned linguistically to a virtual interlocutor that either spoke with a dialect or in High German. Investigating the lexical alignment toward a tutoring agent, Rosenthal-von der Pütten et al. (2013) varied the agent’s choice of words in terms of everyday language versus technical terms. Again, participants aligned to the agent’s use of language in both conditions. Finally, in a study addressing non-verbal alignment with virtual agents, Krämer et al. (2013) analyzed whether humans reciprocate an agent’s smile. Participants conducted a small-talk conversation with an agent that either did not smile, showed occasional smiles, or displayed frequent smiles. Results show that humans smiled longer when the agent was smiling.
In sum, this evidence proves the effectiveness of different components of alignment in human–agent interaction. However, a number of questions still remain open. First, previous studies did not put their results in relation to human–human interaction or “traditional” human–computer interaction. Thus, we do not know much about whether and to what extent the degree (or strength) of alignment differs across these interaction settings. This, however, can provide valuable hints to the possible components and functions (communicative versus social; automatic versus controlled) of alignment. Virtual humans bring anthropomorphic appearance and a strong social component to the interaction with computers. Consequently, they have been argued to be particularly effective social actors and “digital chameleons” (Bailenson and Yee, 2005). Thus, one would expect that alignment, if it is mainly socially driven, is stronger in interactions with embodied agents than in interaction with standard machine-like computers. At the same time, however, virtual humans that exhibit non-verbal behavior are perceived as more competent and skillful in communication (Bergmann et al., 2012, 2010). Hence, if alignment is mainly due to strategic audience design, one may expect to find less alignment in interactions with virtual humans as compared to disembodied systems.
Second, for the most part, alignment with virtual humans has been studied for linguistic aspects only. Non-verbal behaviors, however, are known to be a strong mediator of interpersonal alignment, in particular of automatic, sensorimotor-driven convergence (Kopp, 2010). Yet, it has not been looked at in human-agent interaction [Krämer et al. (2013) being a notable exception for smiling]. Non-verbal behaviors like facial expressions or body posture strongly effect phatic functions by, for instance, indicating sympathy/closeness or other social/emotional qualities. Here, with regard to alignment, hand–arm gestures are of particular interest: gestures play a special role in communication as they, in addition to phatic aspects, convey rich semantic–pragmatic information in tight coordination with speech. Alignment in co-verbal gesturing can hence very well serve both social and communicative functions. Moreover, there is a growing body of evidence from human–human interaction demonstrating that human interlocutors align their gestural behavior. Parrill and Kimbara (2006) demonstrated that observing gestural alignment (in this context often termed “mimicry”) in a video-recorded interaction affects people’s own gesture use in the way that they tended to reproduce the mimicked behavior in their own descriptions. In a similar setting, Mol et al. (2012) provided evidence for the alignment of handshapes in co-speech gestures: participants who saw a speaker in a video stimulus using gestures with a particular handshape were more likely to produce gestures with these handshapes later on, while retelling the story. Further, in studies of face-to-face communication, Holler and Wilkin (2011) showed that gesture mimicry occurs in repeated references to the same figure-like stimuli, and Kimbara (2008) provided evidence for gestural alignment in triadic interaction.
In this paper, we report two controlled experiments to investigate these two issues. In the first experiment, we looked at lexical alignment with human and artificial interaction partners. To this end, we adopted and extended the experimental paradigm applied in Branigan et al. (2011) by adding a condition of interaction with a virtual human. Moreover, the experiment was carried out in German language so that we gain data on how lexical alignment in human–human or human–machine settings generalizes across languages. The second experiment focused on gestural alignment in human–human versus human–agent interaction. Participants engaged in a multimodal interaction with a real versus a virtual human whose gestures were systematically manipulated in one particular, communicatively irrelevant feature, namely handedness (one- versus two-handed gestures). This allows for measuring how participants follow and align in their own gesturing to different kinds of interaction partner. Table 1 gives an overview of how the two experiments address different aspects of alignment with real and virtual humans as interlocutors.
Table 1. Experimental investigation of different aspects of alignment (gestural and linguistic) in combination with partner type (real versus virtual humans) in experiments 1 and 2.
The design of experiment 1 was based on the lexical alignment paradigm developed and applied in several experiments by Branigan et al. (2011). Participants were engaged in a pairwise, screen-based game. The game involved two alternating tasks: participants alternated between choosing a picture in response to a name produced by the partner (participant-matching turn) and naming a picture for their partner (participant-naming turn). An experimental trial consisted of a participant-matching turn followed by a participant-naming turn. We manipulated the name that the partner used to label the picture on the participant-matching turn, so that the partner had either used a strongly preferred or a strongly dispreferred (but acceptable) prime name, and examined how this affected participants target description for the same picture on the subsequent participant-naming turn. Specifically, we tested whether participants used the same name to describe the picture that their partner had previously used to describe that picture.
We used a 2 × 2 × 2 mixed design in which we independently manipulated the name used by the partner (preferred prime versus dispreferred prime) as a within-subjects variable, and level of partner belief [human partner (H) versus computer partner (C)] as well as the level of virtual human presence [virtual human present (VH) versus not present (NVH)] as a between-subjects variables. In the VH conditions, we employed the virtual character “Billie” with the Articulated Communicator Engine [ACE; Kopp and Wachsmuth (2004)] for facial animation. To synthesize the virtual human’s speech, we employed MaryTTS (Schröder and Trouvain, 2003) in version 4.3.1 with the German voice bits1-hsmm. The same synthetic voice was also employed for the partners’ utterances in the NVH conditions. In all conditions, the actual behavior of the partner was identical and generated automatically.
A total of 16 experimental items were prepared in two consecutive steps of pretesting. Each experimental item consisted of (i) a prime picture along with a distractor picture, (ii) a preferred as well as a dispreferred term for the prime picture, and (iii) a target picture (identical to the prime picture) along with another distractor picture (see Figure 1A). For example, one item comprised a prime picture of a bus/coach with distractor picture of an elephant, the prime names “bus” and “coach,” and the target picture with a distractor picture of a sock. For the experimental items, we chose prime/target pictures that have both a highly favored name and a fully acceptable, but dispreferred alternative name (see Supplementary Material).
Figure 1. Picture stimuli (A) from experiment 1 with preferred name “Bus” (Engl.: “bus”), dispreferred name “Reisebus” (Engl.: “coach”) and (B) examples of tangram figures employed in experiment 2.
To identify such items, we conducted a two-part pretest. First, we selected 131 pictures that could be labeled using more than one name. Then, in the first pretest, 20 participants rated how acceptable each name was for that picture on a Likert scale from 1 (completely unacceptable) to 7 (completely acceptable). Based on the results, we then selected 94 pictures with two alternative names that each had a rating of more than 5 (M = 6.08). In the second pretest, another 20 participants were provided with those 94 pictures. In a forced-choice task, they indicated which of the two names they would use to name that picture. Finally, we selected 16 pictures for which one name was preferred by more than 85% of participants. The remaining pictures were used as distractor pictures in the experimental items and as filler pictures. We constructed two lists, such that each list contained eight items in each condition and one version of each item appeared in each list. We used a fixed randomized order of filler items; the order of the experimental items was randomized individually for each participant.
Participants were randomly assigned to the experimental conditions. Gender was distributed equally across conditions. All participants were informed that they were to play a picture-naming and -matching game with a partner. Those in the condition NVH + H (no virtual human; believed human partner) were told that they were to play with a person sitting in another room; those in the condition NVH + C (no virtual human; believed computer partner) were told that they were to play with a computer (see Figure 2A). Those in the condition VH + H (virtual human; believed human partner) played with a virtual character whom they were told was controlled by a human player next door; those in the condition VH + C (virtual human; believed computer partner) were told that they would play against an autonomous virtual human (see Figure 2B).
Figure 2. Experimental conditions of experiment 1: (A) conditions without virtual human (NVH) and (B) with virtual human as game partner (VH); beliefs about the partner (human versus computer) were varied independently.
In all conditions, participants were seated in front of a screen. All participants believed that their partner saw the same pictures as they did. Participants’ task alternated between picking a picture that matched the name given by the partner, and naming a picture for the partner to select in the presence of a distractor picture. In total, participants experienced 140 trials, each consisting of a participant-matching turn and a subsequent participant-naming turn.
On participant-matching turns, a matching (prime) and a mismatching (distractor) picture appeared side by side. A name “produced by the partner” was generated with the synthetic voice after a random delay of 1000–2000 ms. Variable delays were used in order to maintain the appearance of a partner producing a response in realtime. Synthetic speech was identical in all four experimental conditions. In conditions with the virtual human being present, speech was synchronized with the virtual agent’s lip movements. Participants pressed “1” on a keyboard to select the left picture or “2” to select the right picture. The matching picture appeared on the left on half the trials and on the right on the other half.
On participant-naming turns, two pictures appeared side by side, the target picture (identical to the original prime picture) and another distractor picture. After 2000 ms, a yellow box surrounded one of the pictures to be named by the participant. The highlighted picture was on the left for half of the trials and on the right for the other half. Participants were told that their partner would select which one of two pictures matched the name they said. After 1500 ms (on half of trials) or 2000 ms (on the remaining half), a red box surrounded the already highlighted picture; participants were told that this indicated which picture their partner had selected (and hence it followed that the partner always appeared to choose the correct picture).
To make participants familiar with the procedure, the first experimental trial was preceded with 14 filler items. Then, participants encountered 16 experimental trials, each consisting of a participant-matching turn and a participant-naming turn. We exemplify this procedure in Figure 3, which shows a dispreferred-prime trial involving the object in Figure 1A. Its preferred name (established by pretests; see below) is “bus”; a dispreferred name is “coach.” In the participant-matching turn, the participant saw a picture of this object and a distractor picture of another object (the cooking pot). The partner labeled the prime object using the dispreferred name “coach,” and the participant responded by selecting the appropriate picture (their choice is indicated by the red frame). After two filler trials (each comprising a participant-matching turn and a participant-naming turn), the participant had to describe the same object back to the partner in the participant-naming trial. We tested whether participants aligned with the partner on the dispreferred name (“coach”) against the basic tendency to use the preferred name (“bus”) that we would normally expect them to use. Thus, we tested whether participants’ target names were aligned with the partner’s prime names.
Figure 3. Procedure for experimental trials in experiment 1: participant-matching turn consisting of (1a) partner naming for the prime picture of a bus/coach and (1b) participant matching; subsequent participant-naming turn consisting of (2a) participant naming for the target picture of a bus/coach and (2b) partner matching.
For all experiments and pretests, participants were recruited at Bielefeld University. They were native speakers of German and none of them took part in more than one experiment or pretest. In this experiment, a total of 90 subjects, aged from 18 to 61 (M = 27.9, SD = 6.68), participated. Forty-seven participants were female and 43 participants were male.
The second study engaged participants in a game with a partner, in which they alternately matched and described tangram pictures. Descriptions were given multimodally with speech and gestures. A 2 × 3 mixed design was employed to investigate participants gesture handedness as a function of the between-subject factor partner type [human partner (H) versus virtual human partner (VH)] and the within-subject factor handedness (one- versus two-handed gestures versus no gesture as a control condition) of the partners gesturing. As in the first experiment, we employed the virtual human “Billie” in the VH condition with behavior specified in the Behavior Markup Language [BML; Vilhjálmsson et al. (2007)] and realized with the AsapRealizer (Reidsma and van Welbergen, 2013; van Welbergen et al., 2014), a framework for multimodal behavior realization for artificial agents. The virtual human’s gestures were modeled on the basis of gestures produced by humans when describing tangrams in a previous study (Bergmann et al., 2014). In the VH condition, we employed a Wizard-of-Oz setting to control the agent’s behavior. That is, an experimenter initiated the agent’s utterances based on audio and video data of the participant. In the H condition, a confederate acted as partner and produced gestures according to the handedness condition. The confederate was not informed about the aim of the experiment and its particular research questions.
The materials comprised a set of 30 tangram figures as picture stimuli (see Figure 1B for some examples; for the full set of stimuli see Supplementary Material). These either had to be described by the participants or were described by their virtual/real human partner.
Participants were randomly assigned to one of the two between-subject conditions (VH and H, respectively). All participants were informed that they were to play a tangram-description and -matching game with a partner. Those in the H condition were interacting directly with a human confederate, those in the VH condition were interacting with the virtual human whose upper body was displayed in almost life-size on a 46″ screen. Participants sat opposite their respective partner at a table and had a view on a screen that displayed the tangram pictures to be described. Moreover, they had a set of tangram cards in front of them on the table for the matching task. Participants alternated between selecting a tangram figure that matched the description given by their partner, and themselves describing tangram figures displayed on the screen. They were provided with blocks of 10 consecutive items in each of the three handedness conditions, respectively. The order of these blocks was counterbalanced.
A total of 54 participants (26 in each between-subject condition), aged from 19 to 54 years (M = 26.41, SD = 7.21), took part in the study. Thirty-two participants were female and 22 were male. All of them were recruited at Bielefeld University and received 6€ for participating. Gender was distributed equally across conditions.
Table 2 shows for each condition the proportions of dispreferred target names used by participants after the partner had used a preferred or dispreferred-prime name. Following Branigan et al. (2011), we calculate alignment for dispreferred names as the proportion of dispreferred targets following dispreferred primes minus the proportion of dispreferred targets following preferred primes. To analyze this alignment effect in the different experimental conditions, we used a non-parametric Wilcoxon signed-rank test (because variables were not normally distributed). In all conditions, the proportion of dispreferred target names was significantly higher after the partner used a dispreferred-prime name than after the partner used a preferred prime name. This holds for participants who interacted with the virtual human (z = −5.57, p < 0.001, r = −0.83), for participants in the conditions without a virtual human (z = −5.23, p < 0.001, r = −0.83), for participants believing themselves to be interacting with a human partner (z = −5.05, p < 0.001, r = −0.75), as well as for participants believing to interact with a computer partner (z = −5.66, p < 0.001, r = −0.84).
Table 2. Mean proportion of dispreferred target names following partners use of preferred or dispreferred-prime name by condition.
In order to assess the influence of experimental conditions on the alignment effect, a two-way ANOVA was conducted that examined the effect of partner belief and virtual human presence on the alignment effect. There was a significant main effect of partner belief on alignment strength [F(1, 86) = 8. 84, p = 0. 004, ] in the way that participants aligned stronger in interaction with a computer partner as compared to interacting with a human interlocutor. The main effect of virtual human presence was non-significant [F(1, 86) = 0. 46, p = 0. 5, ]. However, there was a significant interaction effect between the type of partner belief and virtual human presence [F(1, 86) = 6. 92, p = 0. 01, ], see Figure 4. We further conducted separate analyses with one-way ANOVA analyses for participants who interacted with the virtual human as opposed to participants in the no-agent conditions. For participants who were not opposed to the virtual human, there was a significant main effect of partner belief [F(1, 44) = 13. 76, p < 0. 001, ] such that alignment was stronger when participants believed to be interacting with a computer partner. By contrast, for participants who were interacting with the virtual human, there was no significant main effect [F(1, 44) = 0. 07, p = 0. 80, ].
Table 3 shows the mean proportions of participants’ one-handed versus two-handed gestures after seeing their (virtual or real) partner doing gestures with one hand, both hands, or no gesture at all.
Table 3. Mean proportion of participants’ 1-handed versus 2-handed gesture use per experimental condition [i.e., in response to either one-handed, two-handed, or no-gesture stimuli provided either by the virtual agent (VA) or the human confederate (H)].
A mixed-design ANOVA with partner handedness (1-handed gestures, 2-handed gestures, no gestures) as a within-subject factor and partner type (H, VA) as a between-subject factor revealed a significant main effect of the factor partner handedness [F(2, 88) = 8. 28, p = 0. 001, ]. There was no main effect of partner type on the proportion of participants’ 1-handed versus 2-handed response gestures [F(1, 44) = 0. 46, p = 0. 55, ], nor was there an interaction effect of partner handedness and partner type [F(1, 44) = 0. 94, p = 0. 34, ].
Further, we conducted separate analyses for participants who interacted with the virtual agent as opposed to participants who interacted with the human partner. Results are visualized in Figure 5. For participants who had a human partner, a repeated-measures ANOVA showed a significant main effect of the factor partner handedness [F(2, 36) = 4. 15, p = 0. 024, ]. Post hoc analyses with Tukey’s HSD indicated that this main effect was driven by significantly increased proportions of matching versus non-matching gestures. The proportion of one-handed participant gestures was significantly higher in response to one-handed partner gestures than in response to two-handed partner gestures (p = 0.012) or the no-gesture control condition (p = 0.012). Likewise, the proportion of two-handed participant gestures in response to two-handed partner gestures was significantly increased in comparison with the proportion of two-handed participant gestures in response to one-handed gestures performed by the human partner (p = 0.024). In addition, the proportion of two-handed participant gestures in response to one-handed partner gestures was significantly lower than in the control condition (p = 0.024). In sum, participants’ handedness in human–human interaction was characterized by an increase of one-handed gestures (and a decrease of two-handed ones) in response to one-handed stimulus gestures, and an increase of two-handed gestures (and a decrease of one-handed ones) in response to two-handed stimulus gestures.
Figure 5. Results of experiment 2: proportions of one-handed gestures (left) and two-handed gestures (right) for the different blocks of interaction during which the partner gestures differently.
For participants who interacted with the virtual human, a repeated-measures ANOVA also showed a significant main effect of the factor partner handedness [F(2, 52) = 5. 08, p = 0. 01, ]. Post hoc analyses employing Tukey’s HSD indicated that this main effect was driven by significantly increased proportions of matching versus non-matching gestures. Again, the proportion of one-handed participant gestures was significantly higher in response to one-handed agent gestures than in response to two-handed agent gestures (p = 0.012). Likewise, the proportion of two-handed participant gestures in response to two-handed agent gestures was significantly higher than in response to one-handed agent gestures (p = 0.012). Moreover, the proportion of one-handed participant gestures in response to two-handed agent gestures was significantly decreased in comparison with the no-gesture control condition (p = 0.009). Similarly, the proportion of matching two-handed response gestures was significantly higher than the proportion of two-handed response gestures in the control condition (p = 0.009). In all, participants’ handedness in interaction with the virtual human was characterized by an increase of two-handed gestures in response to two-handed stimuli gestures, and a decrease of two-handed gestures in response to one-handed stimulus gestures.
Our first experiment was meant to elucidate whether the presence of a virtual human as interaction partner, as well as the beliefs about the interaction partner affect linguistic alignment. The results, first of all, provide strong support for the phenomenon of lexical alignment in both human–human and human–computer interactions. Lexical alignment was found in all experimental conditions: when interacting with a virtual human, when interacting via speech only, when participants were believing themselves to be interacting with a human partner or with a computer partner. In accordance with Rosenthal-von der Pütten et al. (2013), these results demonstrate that lexical alignment does occur in interaction with a virtual character. Moreover, and also in line with previous findings by Branigan et al. (2011), we identified a main effect of partner type: participants showed a stronger tendency to align lexically with their interaction partner when they believed to be interacting with a computer as opposed to a human.
Having conducted the same experimental paradigm with similar stimulus material, but in German instead of English, our results thus corroborate Branigan et al.’s outcome that lexical alignment with computers is stronger than with human interlocutors. However, we found this effect to crucially interact with the visual presence of a virtual human as interaction partner. When a virtual human was present, the effect disappeared and lexical alignment was similar regardless of whom participants believed themselves to be interacting with (cf. Figure 4).
How can we explain this finding? One way to interpret this is within the scope of the agent-versus-avatar discussion in social psychology [cf. Von der Pütten et al. (2010) and Fox et al. (2014)]. Agents are distinguished from avatars by how they are controlled: avatars are controlled by humans, whereas agents are controlled by computational algorithms. In numerous studies, researchers investigated whether agents and avatars differ with regard to their social effects – tested with a multitude of measures. Von der Pütten et al. (2010), for instance, analyzed differences between avatars and agents in terms of social evaluation or social behavior, and came to a clear conclusion that interacting with an avatar or an agent barely resulted in any differences. This provides support for the concept of Ethopoeia, which states that, as humans are inherently social, human-like social rules apply automatically and unconsciously also in interactions with computers (Nass et al., 1997; Nass and Moon, 2000). Accordingly, there is no difference between agents and avatars as long as the artificial characters provide a sufficient quantity of social cues such as natural language or non-verbal behaviors. By contrast, other studies employing different measures of social affect came to the result that perceived avatars produce stronger social responses than perceived agents (Fox et al., 2014) as predicted by the model of social influence in virtual environments (Blascovich, 2002).
Our lexical alignment results lend support to the Ethopoeia theory, implying that the character’s appearance and behavior created such a strong social presence for the participants that their beliefs about how the character was controlled and corresponding audience design decisions would be rendered almost non-effective. Indeed, the strength of lexical alignment seems to decrease the more human-like social cues from the interlocutor are present. That is, lexical alignment is among those dynamic adaptations of linguistic behavior that probably have a strategic component, but that are also affected by the social cues provided by a virtual human. So, while Branigan et al. (2011) suggest that people stick with rather persistent interlocutor models in HCI (Pearson et al., 2006), the present results suggest that virtual humans are treated more like real humans in that observed appearance and behavior rapidly override or update initial beliefs – a phenomenon known from human–human interactions (Von der Pütten et al., 2010; Bergmann et al., 2012). Further research is required at this point addressing the question in how far alignment strength might alter dynamically and which factors might affect these dynamics.
In the second experiment, we investigated whether humans also align gesturally to a visually present partner, and whether this also holds for virtual humans as interaction partners. We found clear evidence for “inducable” alignment of gesture handedness, i.e., when the interaction partner changed handedness of gesture use, human participants tended to follow within a certain period of time. This finding, first of all, provides support for the existence of gestural alignment.
However, while previous studies have looked at human–human alignment of overall gesture forms (Kimbara, 2006; Parrill and Kimbara, 2006; Holler and Wilkin, 2011) or handshape use (Kimbara, 2008; Mol et al., 2012), the present study supplements these findings with respect to handedness. This is important as handedness, in contrast to other gestural features like handshape, conveys no or little meaning in the experimental setting. In general, handedness is often communicatively less relevant. Drawing gestures, for instance, in which the index finger is used as a “pen” to draw, e.g., the shape of an object in the air, convey meaning mainly through the movement trajectory of the hand (or index finger). It is irrelevant whether such a drawing is performed either with one hand or by two hands. The results from our experiment 2, hence, are unlikely to be driven by some form of grounding or audience design to foster communicative success. Our findings instead show that alignment is not restricted to meaning-related aspects of verbal or non-verbal communication, and indicate a multicomponential nature of interpersonal alignment.
A second major finding from experiment 2 is that gestural alignment strength did not differ significantly in interactions with a real versus a virtual human. This may lend further support to the Ethopoeia view that virtual humans can create human-like social presence that triggers the same adaptation mechanisms as in human–human interaction. But we can shed even further light on the nature of these mechanisms. In a recent analysis of a large corpus of human–human dialog data, Bergmann and Kopp (2012) have shown that alignment of communicatively less relevant gesture features is actually more prevalent than alignment of communicatively relevant ones. A possible explanation for this could be that multiple adaptation mechanisms are simultaneously at work when we interact – from high-level strategic mechanisms driven by grounding, to lower-level mechanisms driven by priming of the sensorimotor system. The former should primarily affect alignment in communicatively relevant gestural features, while the latter could more strongly affect features that are less constrained communicatively [see Kopp and Bergmann (2013) for a more detailed proposal of such a model].
In our experimental setting, handedness may belong to the latter category and may thus be influenced by automatic “motor resonance” processes, which may also be triggered by virtual humans’ gesturing. Neuroscientific research has suggested a neurological basis for this – a frontoparietal action–observation network that supports automatic behavior imitation by mapping observed action into motor codes also involved in execution (Cross et al., 2009). Recent evidence, in particular, showed that automatic imitation even occurs in a strategic context (Cook et al., 2012). Crucially, the action–observation network has been shown to be biologically tuned: responses are stronger when observing human bodily behavior as compared to artificial bodily behavior (Kilner et al., 2003; Tai et al., 2004; Engel et al., 2009). Following these considerations, alignment in handedness should be stronger in interaction with real human interlocutors as opposed to an artificial dialog partner. Although it might be the case, however, that our virtual human’s gesturing (synthesized by way of model-based computer animation) might have fallen short of fully natural human gesturing in some way, the fact that there was no difference in the VH versus H conditions implies that whatever aspects the synthesis fell short in, they were not crucial for distinguishing virtual versus real human in relevant ways for behavior adaptation. In other words, the gesturing synthesis may not have been fully human-like, but it was human-like enough to induce human-like gestural alignment.
Finally, our results do show a difference in how participants aligned their gesturing in response to human versus agent stimuli. In human–human interaction, there was an increase of one-handed gestures in the matching condition, while the two-handed gesture rate remained unaffected and on the level of the control condition. By contrast, in human–agent interaction, the alignment effect was due to an increase of two-handed gestures in the matching condition, while the one-handed gestures remained largely unaffected. Here, the multicomponential nature of alignment might be manifesting again. Assuming that two-handed gesturing is more effortful than one-handed gesturing, participants were found to be willing to invest more effort when communicating with a virtual human. This may be, just like lexical alignment, due to the beliefs about the artificial partner as not being so capable of recognizing gestures. A more systematic experiment is needed to reveal whether this adaption effect is really only uni-directional (from one- to two-handed gesturing).
The current picture of alignment is very fragmented, with various phenomena, mechanisms, and effects being discussed. We would argue that it makes more sense to speak of an “alignment space” that needs to be systematically explored. The present findings complement this fragmentary picture in several ways.
First, we provided first evidence for both lexical and gestural alignments with virtual humans. This shows that adaptation takes place in interaction with artificial characters regarding communicative features (lexical alignment) as well as features without obvious communicative function (handedness alignment). Second, our results from the lexical alignment experiment suggest that people take initial beliefs about their interlocutor into account when adapting their choice of words to their addressees’ – but only to a certain degree. When social cues and presence as created by a virtual human come into play, automatic social reactions appear to override initial beliefs in shaping lexical alignment. Finally, our evidence from handedness alignment in gestures suggests that automatic motor resonance is (at least) complemented by audience design in affecting how people align with their interaction partners.
Overall, we interpret our findings such that alignment of communicative behavior is the result of a dynamic interplay of multiple mechanisms. There are, on the one hand, mechanisms aiming at communicative success with both, strategic (audience design) and automatic (motor resonance) components. On the other hand, there are social mechanisms, which may also divide into rather strategic components and rather automatic components. Strategically, speakers might align to express their affiliation with an interlocutor and to enhance their interpersonal relationship. Automatically, speakers respond to social cues provided by their dialog partner. Further research is needed to elucidate how different mechanisms and driving forces compete and interact with each other. As the current work has shown, research with virtual humans can be a valuable means to address and distinguish the different kinds of processes and mechanisms identified so far.
Finally, the present study indicates that alignment has a role to play in the design of dialog systems and virtual humans. It has often been reported that system-to-user alignment can foster acceptance, user experience and interaction quality (Brockmann et al., 2005; Buschmeier et al., 2009). The opposite effect of user-to-system alignment can also be exploited, e.g., in order to affect users to use particular behaviors that can be better processed by the system. For instance, predictions of users’ choice of words might speed up and improve the results of automatic speech recognition. Similarly, predictions about users’ non-verbal behavior like gestures or facial expressions might enhance recognition rates for those social signals. Furthermore, the alignment effect may be exploited for application goals. For one thing, alignment may promote learning and could be investigated in the development of e-learning systems and pedagogical agents. For example, lexical alignment might help to extend learners’ vocabulary with respect to technical terms (Rosenthal-von der Pütten et al., 2013), syntactic alignment might help to learn language (Messenger et al., 2012; Rowland et al., 2012) or a foreign language (McDonough, 2006), and gestural alignment might support teaching in different ways (Cui et al., 2014). First steps into this direction have already been taken with respect to math education (Alibali et al., 2013) or vocabulary acquisition in a foreign language (Bergmann and Macedonia, 2013). Understanding human learners’ alignment in response to virtual teachers or tutors might help to improve and optimize human–agent interaction with respect to learning outcome, motivation, and/or teacher acceptance.
The authors declare that the research was conducted in the absence of any commercial or financial relationships that could be construed as a potential conflict of interest.
The authors are grateful to Volkan Aksu and Katharina Klein. We acknowledge support by the Collaborative Research Centre SFB 673 “Alignment in Communication” and the Cluster of Excellence in “Cognitive Interaction Technology” (CITEC), both funded by the German Research Foundation (DFG). HB was supported by a British Academy/Leverhulme Trust Senior Research Fellowship.
The Supplementary Material for this article can be found online at http://journal.frontiersin.org/article/10.3389/fict.2015.00007
Alibali, M. W., Young, A. G., Crooks, N. M., Yeo, A., Wolfgram, M. S., Ledesma, I. M., et al. (2013). Students learn more when their teacher has learned to gesture effectively. Gesture 13, 210–233. doi: 10.3758/BRM.40.4.1106
André, E., Rehm, M., Minker, W., and Buhler, D. (2004). “Endowing spoken language dialogue systems with emotional intelligence,” in Affective Dialogue Systems, eds E. André, L. Dybkjaer, W. Minker, and P. Heisterkamp (Berlin: Springer), 178–187.
Bailenson, J., and Yee, N. (2005). Digital chameleons: automatic assimilation of nonverbal gestures in immersive virtual environments. Psychol. Sci. 16, 814–819. doi:10.1111/j.1467-9280.2005.01619.x
Bell, L., Gustafson, J., and Heldner, M. (2003). “Prosodic adaptation in human–computer interaction,” in Proceedings of ICPhS-03. Barcelona, 2453–2456.
Bergmann, K., Böck, R., and Jaecks, P. (2014). “Emogest: investigating the impact of emotions on spontaneous co-speech gestures,” in Proceedings of the LREC Workshop on Multimodal Corpora, eds J. Edlund, D. Heylen, and P. Paggio (Reykjavik).
Bergmann, K., Eyssel, F. A., and Kopp, S. (2012). “A second chance to make a first impression? How appearance and nonverbal behavior affect perceived warmth and competence of virtual agents over time,” in Proceedings of the 12th International Conference on Intelligent Virtual Agents (Berlin: Springer), 126–138.
Bergmann, K., and Kopp, S. (2012). “Gestural alignment in natural dialogue,” in Proceedings of the 34th Annual Conference of the Cognitive Science Society (Austin, TX: Cognitive Science Society), 1326–1331.
Bergmann, K., Kopp, S., and Eyssel, F. (2010). “Individualized gesturing outperforms average gesturing–evaluating gesture production in virtual humans,” in Proceedings of the 10th Conference on Intelligent Virtual Agents (Berlin: Springer), 104–117.
Bergmann, K., and Macedonia, M. (2013). “A virtual agent as vocabulary trainer: iconic gestures help to improve learners’ memory performance,” in Proceedings of the 13th International Conference on Intelligent Virtual Agents (Berlin: Springer), 139–148.
Bernieri, F., and Rosenthal, R. (1991). “Interpersonal coordination: behavior matching and interactional synchrony,” in Fundamentals of Nonverbal Behavior. Studies in Emotion and Social Interaction, eds R. Feldman and B. Rime (New York, NY: Cambridge University Press), 401–432.
Blascovich, J. (2002). “A theoretical model of social influence for increasing the utility of collaborative virtual environments,” in Proceedings of the 4th International Conference on Collaborative Virtual Environments (Bonn: ACM), 25–30.
Bradac, J. J., Mulac, A., and House, A. (1988). Lexical diversity and magnitude of convergent versus divergent style shifting perceptual and evaluative consequences. Lang. Commun. 8, 213–228. doi:10.1016/0271-5309(88)90019-5
Branigan, H., Pickering, M., and Cleland, A. (2000). Syntactic co-ordination in dialogue. Cognition 75, B13–B25. doi:10.1016/S0010-0277(99)00081-5
Branigan, H. P., Pickering, M. J., Pearson, J., and Mclean, J. F. (2010). Linguistic alignment between people and computers. J. Pragmat. 9, 2355–2368. doi:10.1016/j.pragma.2009.12.012
Branigan, H. P., Pickering, M. J., Pearson, J., McLean, J. F., and Brown, A. (2011). The role of beliefs in lexical alignment: evidence from dialogs with humans and computers. Cognition 121, 41–57. doi:10.1016/j.cognition.2011.05.011
Brennan, S. (1996). “Lexical entrainment in spontaneous dialog,” in Proceedings of the International Symposium on Spoken Dialogue. Philadelphia, PA, 41–44.
Brennan, S. E., and Clark, H. H. (1996). Lexical choice and conceptual pacts in conversation. J. Exp. Psychol. Learn. Mem. Cogn. 22, 1482–1493.
Brockmann, C., Isard, A., Oberlander, J., and White, M. (2005). “Modelling alignment for affective dialogue,” in Proceedings of the Workshop on Adapting the Interaction Style to Affective Factors at the 10th International Conference on User Modeling. Edinburgh.
Buschmeier, H., Bergmann, K., and Kopp, S. (2009). “An alignment-capable microplanner for natural language generation,” in Proceedings of the 12th European Workshop on Natural Language Generation (Association for Computational Linguistics). Athens, 82–89.
Chartrand, T., and Bargh, J. (1999). The chameleon effect: the perception-behavior link and social interaction. J. Pers. Soc. Psychol. 76, 893–910. doi:10.1037/0022-3514.76.6.893
Clark, H., and Wilkes-Gibbs, D. (1986). Referring as a collaborative process. Cognition 22, 1–39. doi:10.1016/0010-0277(86)90010-7
Cleland, A., and Pickering, M. (2003). The use of lexical and syntactic information in language production: evidence from the priming of noun-phrase structure. J. Mem. Lang. 49, 214–230. doi:10.1016/S0749-596X(03)00060-3
Cook, R., Bird, G., Lünser, G., Huck, S., and Heyes, C. (2012). Automatic imitation in a strategic context: players of rock–paper–scissors imitate opponents’ gestures. Proc. Biol. Sci. 279, 780–786. doi:10.1098/rspb.2011.1024
Cross, E. S., de, C., Hamilton, A. F., Kraemer, D. J. M., Kelley, W. M., and Grafton, S. T. (2009). Dissociable substrates for body motion and physical experience in the human action observation network. Eur. J. Neurosci. 30, 1383–1392. doi:10.1111/j.1460-9568.2009.06941.x
Cui, J., Wu, M.-L., Rajasekaran, S. D., Adamo-Villani, N., and Popescu, V. (2014). “Avatar-based research on gesture in instruction: opportunities, challenges, and solutions,” in Proceedings of the 6th Conference of the International Society for Gesture Studies. San Diego, CA.
Darves, C., and Oviatt, S. (2002). “Adaptation of users’ spoken dialogue patterns in a conversational interface,” in Proceedings of the International Conference on Spoken Language Processing, eds J. Hansen and B. Pellom (Denver, CO), 561–564.
Dimberg, U. (1982). Facial reactions to facial expressions. Psychophysiology 19, 643–647. doi:10.1111/j.1469-8986.1982.tb02516.x
Engel, L., Frum, C., Puce, A., Walker, N. A., and Lewis, J. (2009). Different categories of living and non-living sound-sources activate distinct cortical networks. Neuroimage 47, 1778–1791. doi:10.1016/j.neuroimage.2009.05.041
Fox, J., Ahn, S., Janssen, J., Yeykelis, L., Segovia, K., and Bailenson, J. N. (2014). Avatars versus agents: a meta-analysis quantifying the effect of agency. Hum. Comput. Interact. doi:10.1080/07370024.2014.921494
Garrod, S., and Anderson, A. (1987). Saying what you mean in dialogue: a study in conceptual and semantic co-ordination. Cognition 27, 181–218. doi:10.1016/0010-0277(87)90018-7
Giles, H., Coupland, N., and Coupland, J. (1991). “Accomodation theory: communication, context and consequence,” in Contexts of Accomodation: Developments in Applied Sociolinguistics, eds H. Giles, N. Coupland, and J. Coupland (Cambridge: Cambridge University Press), 1–68.
Gries, S. (2005). Syntactic priming: a corpus-based approach. J. Psycholinguist. Res. 34, 365–399. doi:10.1007/s10936-005-6139-3
Hartholt, A., Traum, D., Marsella, S., Shapiro, A., Stratou, G., Leuski, A., et al. (2013). “All together now, introducing the virtual human toolkit,” in Proceedings of the 13th International Conference on Intelligent Virtual Agents (Berlin: Springer), 368–381.
Holler, J., and Wilkin, K. (2011). Co-speech gesture mimicry in the process of collaborative referring during face-to-face dialogue. J. Nonverbal Behav. 35, 133–153. doi:10.1007/s10919-011-0105-6
Johnson, L., Rizzo, P., Bosma, W., Ghijsen, M., and van Welbergen, H. (2004). “Generating socially appropriate tutorial dialog,” in Affective Dialogue Systems, eds E. André, L. Dybkjaer, W. Minker, and P. Heisterkamp (Berlin: Springer), 254–264.
Kilner, J. M., Paulignan, Y., and Blakemore, S.-J. (2003). An interference effect of observed biological movement on action. Curr. Biol. 13, 522–525. doi:10.1016/S0960-9822(03)00165-9
Kimbara, I. (2008). Gesture form convergence in joint description. J. Nonverbal Behav. 32, 123–131. doi:10.1007/s10919-007-0044-4
Kopp, S. (2010). Social resonance and embodied coordination in face-to-face conversation with artificial interlocutors. Speech Commun. 52, 587–597. doi:10.1016/j.specom.2010.02.007
Kopp, S., and Bergmann, K. (2013). “Automatic and strategic alignment of co-verbal gestures in dialogue,” in Alignment in Communication: Towards a New Theory of Communication, eds I. Wachsmuth, J. de Ruiter, P. Jaecks, and S. Kopp (Amsterdam: John Benjamins Publishing Company), 87–107.
Kopp, S., and Wachsmuth, I. (2004). Synthesizing multimodal utterances for conversational agents. Comput. Animat. Virtual Worlds 15, 39–52. doi:10.1002/cav.6
Koulouria, T., Lauria, S., and Macredie, R. D. (2014). Do (and say) as i say: linguistic adaptation in human-computer dialogs. Hum. Comput. Interact. doi:10.1080/07370024.2014.934180
Kousidis, S., and Dorran, D. (2009). “Monitoring convergence of temporal features in spontaneous dialogue speech,” in Proceedings of the 1st Young Researchers Workshop on Speech Technology. Dublin.
Krämer, N., and Bente, G. (2010). Personalizing e-learning. The social effects of pedagogical agents. Educ. Psychol. Rev. 22, 71–87. doi:10.1007/s10648-010-9123-x
Krämer, N., Kopp, S., Becker-Asano, C., and Sommer, N. (2013). Smile and the world will smile with you – the effects of a virtual agent’s smile on users’ evaluation and behavior. Int. J. Hum. Comput. Stud. 71, 335–349. doi:10.1016/j.ijhcs.2012.09.006
Kühne, V., von der Pütten, A. M. R., and Krämer, N. C. (2013). “Using linguistic alignment to enhance learning experience with pedagogical agents: the special case of dialect,” in Proceedings of the 13th International Conference on Intelligent Virtual Agents (Edinburgh: Springer), 149–158.
Lakin, J., and Chartrand, T. (2003). Using nonconscious behavioral mimicry to create affiliation and rapport. Psychol. Sci. 14, 334–339. doi:10.1111/1467-9280.14481
Lee, C.-C., Black, M., Katsamanis, A., Lammert, A., Baucom, B., Christensen, A., et al. (2010). “Quantification of prosodic entrainment in affective spontaneous spoken interactions of married couples,” in Proceedings of the 11th Annual Conference of the International Speech Communication Association. Makuhari.
Leiser, R. (1989). Exploiting convergence to improve natural language understanding. Interact. Comput. 1, 284–298. doi:10.1016/0953-5438(89)90016-7
Levitan, R., and Hirschberg, J. (2011). “Measuring acoustic-prosodic entrainment with respect to multiple levels and dimensions,” in Proceedings of the 12th Annual Conference of the International Speech Communication Association. Florence.
Likowski, K. U., Mühlberger, A., Gerdes, A. B., Wieser, M. J., Pauli, P., and Weyers, P. (2012). Facial mimicry and the mirror neuron system: simultaneous acquisition of facial electromyography and functional magnetic resonance imaging. Front. Hum. Neurosci. 6:214. doi:10.3389/fnhum.2012.00214
Louwerse, M. M., Dale, R., Bard, E. G., and Jeuniaux, P. (2012). Behavior matching in multi-modal communication is synchronized. Cogn. Sci. 36, 1404–1420. doi:10.1111/j.1551-6709.2012.01269.x
Maurer, R. E., and Tindall, J. H. (1983). Effect of postural congruence on clients perception of counselor empathy. J. Couns. Psychol. 30, 158–163. doi:10.1037/0022-0167.30.2.158
McDonough, K. (2006). Interaction and syntactic priming: english L2 speakers’ production of dative constructions. Stud. in Second Lang. Acquis. 28, 179–207. doi:10.1017/S0272263106060098
McLean, J., Pickering, M., and Branigan, H. (2004). “Lexical repetition and syntactic priming in dialogue,” in Approaches to Studying World-Situated Language Use: Bridging the Language-as-Product and Language-as-Action Traditions, eds J. C. Trueswell and M. Tanenhaus (Cambridge, MA: MIT Press), 193–208.
Messenger, K., Branigan, H., and MacLean, J. (2012). Is young children’s passive syntax semantically constrained? Evidence from syntactic priming. J. Mem. Lang. 66, 568–587. doi:10.1016/j.jml.2012.03.008
Mol, L., Krahmer, E., Maes, A., and Swerts, M. (2012). Adaptation in gesture: converging hands or converging minds? J. Mem. Lang. 66, 249–264. doi:10.1016/j.jml.2011.07.004
Nass, C., and Moon, Y. (2000). Machines and mindlessness: social responses to computers. J. Soc. Issues 56, 81–103. doi:10.1111/0022-4537.00153
Nass, C., Moon, Y., Morkes, J., Kim, E.-Y., and Fogg, B. (1997). “Computers are social actors: a review of current research,” in Moral and Ethical Issues in Human-Computer Interaction, ed. B. Friedman (Stanford, CA: CSLI Press), 137–162.
Nass, I., and Lee, K. M. (2001). Does computer-synthesized speech manifest personality? Experimental tests of recognition, similarity-attraction, and consistency-attraction. J. Exp. Psychol. 7, 171–181. doi:10.1037//1076-898X.7.3.171
Nilsenová, M., and Nolting, P. (2010). “Linguistic adaptation in semi-natural dialogues: age comparison,” in Proceedings of the 13th International Conference on Text, Speech and Dialogue (Berlin: Springer), 531–538.
Nishimura, R., Kitaoka, N., and Nakagawa, S. (2008). “Analysis of relationship between impression of human-to-human conversations and prosodic change and its modeling,” in Proceedings of the 9th Annual Conference of the International Speech Communication Association. Brisbane.
Oviatt, S., Darves, C., and Coulston, R. (2004). Toward adaptive conversational interfaces: modeling speech convergence with animated personas. Trans. Comput. Hum. Interact. 11, 300–328. doi:10.1145/1017494.1017498
Pardo, J. S., Gibbons, R., Suppes, A., and Krauss, R. M. (2012). Phonetic convergence in college roommates. J. Phon. 40, 190–197. doi:10.1016/j.wocn.2011.10.001
Parrill, F., and Kimbara, I. (2006). Seeing and hearing double: the influence of mimicry in speech and gesture on observers. J. Nonverbal Behav. 30, 157–166. doi:10.1007/s10919-006-0014-2
Pearson, J., Hu, J., Branigan, H. P., Pickering, M. J., and Nass, C. I. (2006). “Adaptive language behavior in hci: how expectations and beliefs about a system affect users’ word choice,” in Proceedings of the SIGCHI Conference on Human Factors in Computing Systems (Montréal: ACM), 1177–1180.
Pickering, M., and Garrod, S. (2004). Toward a mechanistic psychology of dialogue. Behav. Brain Sci. 27, 169–226. doi:10.1017/S0140525X04000056
Pickering, M., and Garrod, S. (2006). Alignment as the basis for successful communication. Res. Lang. Comput. 4, 203–228. doi:10.1007/s11168-006-9004-0
Reidsma, D., and van Welbergen, H. (2013). AsapRealizer in practice – a modular and extensible architecture for a BML realizer. Entertain. Comput. 4, 157–169. doi:10.1016/j.entcom.2013.05.001
Reitter, D., and Moore, J. D. (2014). Alignment and task success in spoken dialogue. J. Mem. Lang. 76, 29–46. doi:10.1016/j.jml.2014.05.008
Rosenthal-von der Pütten, A., Wiering, L., and Krämer, N. (2013). Great minds think alike. Experimental study on lexical alignment in human-agent interaction. i-com 1, 32–38. doi:10.1524/icom.2013.0005
Rowland, C. F., Chang, F., Ambridge, B., Pine, J. M., and Lieven, E. V. (2012). The development of abstract syntax: evidence from structural priming and the lexical boost. Cognition 125, 49–63. doi:10.1016/j.cognition.2012.06.008
Schröder, M., and Trouvain, J. (2003). The german text-to-speech synthesis system MARY: a tool for research, development and teaching. Int. J. Speech Technol. 6, 365–377. doi:10.1023/A:1025708916924
Scissors, L., Gill, A., and Gergle, D. (2008). “Linguistic mimicry and trust in text-based cmc,” in Proceedings of CSCW (New York, NY: ACM Press), 277–280.
Staum Casasanto, L., Jasmin, K., and Casasanto, D. (2010). “Virtually accommodating: speech rate accommodation to a virtual interlocutor,” in Proceedings of the 32nd Annual Conference of the Cognitive Science Society, eds S. Ohlsson and R. Catrambone (Austin, TX: Cognitive Science Society), 127–132.
Suzuki, N., and Katagiri, Y. (2007). Prosodic alignment in human-computer interaction. Connect. Sci. 19, 131–141. doi:10.1080/09540090701369125
Tai, Y. F., Scherfler, C., Brooks, D. J., Sawamoto, N., and Castiello, U. (2004). The human premotor cortex is ‘mirror’ only for biological actions. Curr. Biol. 14, 117–120. doi:10.1016/j.cub.2004.01.005
Truong, K. P., and Heylen, D. (2012). “Measuring prosodic alignment in cooperative task-based conversations,” in Proceedings of the 13th Annual Conference of the International Speech Communication Association (Portland, OR: International Speech Communication Association), 1085–1089.
Van Baaren, R. B., Holland, R. W., Kawakami, K., and Van Knippenberg, A. (2004). Mimicry and prosocial behavior. Psychol. Sci. 15, 71–74. doi:10.1111/j.0963-7214.2004.01501012.x
Van Baaren, R. B., Holland, R. W., Steenaert, B., and van Knippenberg, A. (2003). Mimicry for money: behavioral consequences of imitation. J. Exp. Soc. Psychol. 39, 393–398. doi:10.1016/S0022-1031(03)00014-3
van Welbergen, H., Yaghoubzadeh, R., and Kopp, S. (2014). “AsapRealizer 2.0: the next steps in fluent behavior realization for ECAs,” in Intelligent Virtual Agents (Berlin: Springer).
Vaughan, B. (2011). “Prosodic synchrony in co-operative task-based dialogues: a measure of agreement and disagreement,” in Proceedings of the 12th Annual Conference of the International Speech Communication Association. Florence.
Vilhjálmsson, H., Cantelmo, N., Cassell, J., Chafai, N., Kipp, M., Kopp, S., et al. (2007). “The behavior markup language: recent developments and challenges,” in Proceedings of the 7th International Conference on Intelligent Virtual Agents (Berlin: Springer), 99–111.
Von der Pütten, A., Hoffmann, L., Klatt, J., and Krämer, N. (2011). “Quid pro quo? Reciprocal self-disclosure and communicative accomodation towards a virtual interviewer,” in Proceedings of the 11th International Conference on Intelligent Virtual Agents (Berlin), 183–194.
Von der Pütten, A., Krämer, N., Gratch, J., and Kang, S.-H. (2010). “It doesen’t matter what you are!” Explaining social effects of agents and avatars. Comput. Human Behav. 26, 1641–1650. doi:10.1016/j.chb.2010.06.012
Keywords: alignment, virtual agents, human–computer interaction, gestures
Citation: Bergmann K, Branigan HP and Kopp S (2015) Exploring the alignment space – lexical and gestural alignment with real and virtual humans. Front. ICT 2:7. doi: 10.3389/fict.2015.00007
Received: 19 December 2014; Accepted: 07 April 2015;
Published: 18 May 2015
Edited by:
Nadia Berthouze, University College London, UKReviewed by:
Gualtiero Volpe, Università degli Studi di Genova, ItalyCopyright: © 2015 Bergmann, Branigan and Kopp. This is an open-access article distributed under the terms of the Creative Commons Attribution License (CC BY). The use, distribution or reproduction in other forums is permitted, provided the original author(s) or licensor are credited and that the original publication in this journal is cited, in accordance with accepted academic practice. No use, distribution or reproduction is permitted which does not comply with these terms.
*Correspondence: Stefan Kopp, Social Cognitive Systems Group, CITEC, Faculty of Technology, Bielefeld University, P.O. Box 100 131, Bielefeld 33501, Germany,c2tvcHBAdGVjaGZhay51bmktYmllbGVmZWxkLmRl
Disclaimer: All claims expressed in this article are solely those of the authors and do not necessarily represent those of their affiliated organizations, or those of the publisher, the editors and the reviewers. Any product that may be evaluated in this article or claim that may be made by its manufacturer is not guaranteed or endorsed by the publisher.
Research integrity at Frontiers
Learn more about the work of our research integrity team to safeguard the quality of each article we publish.