Mankind’s greatest scientific instrument is arguably in our pockets. In this short article, we point out that their popularity, flexibility, and behavioral sensing capabilities make them ideal for studying humanity at various levels of complexity, in ways that were previously not possible.
Popularity and Economies of Scale
Mobile phones overtook personal computers in popularity in 2013. Today, an estimated 22% of the world’s population owns a mobile phone, while 20% owns a personal computer. In western societies, mobile phones are used by more than 90% of the population, and developing nations are quickly catching up. The economies of scale involved in manufacturing billions of smartphones every year have reduced their cost and dramatically increased the scientific potential of their hardware.
These economic, engineering, and scientific advances are rapidly turning smartphones into compelling scientific instruments. For instance, in the last decade smartphones have repeatedly been used to study the spread of disease (Wang et al., 2009; Wesolowski et al., 2012). Increasingly, however, this type of post hoc modeling overlooks many of the scientific opportunities afforded by modern devices and their sensing capabilities. Because smartphones have become ubiquitous, much more than personal computers, their instrumentation can now provide scientists unparalleled insight into humanity. Therefore, it is now possible to run studies and experiments that previously were simply impossible.
Flexibility
Researchers are using smartphones to study humanity at the micro, meso, and macro scales. At the microscale, scientists use smartphones to instrument everyday environments of individuals and monitor behavior or physiology over time. For example, a smartphone-controlled digital pancreas is helping fight type 1 diabetes (Clery, 2014), smartphone “labs-on-a-chip” can provide cancer diagnosis within an hour (Haun et al., 2011), and tetraplegics are using smartphones to control their wheelchair (Kim et al., 2013). At the mesoscale, scientists are leveraging the capacity of smartphones to collect data, and seek to reveal patterns of group behavior in communication (Malmgren et al., 2008) and social interaction (Lazer et al., 2009). At the macroscale, smartphones can be used as a comprehensive proxy for societal-level behavior, for example, in terms of HIV spreading (Wesolowski et al., 2012) and social diversity (Eagle et al., 2010).
These three approaches resonate with visions for computational behavioral science (Lightfoot, 2007), computational social science (Lazer et al., 2009), and engineering of social systems (Eagle and Greene, 2013), respectively. Common to these approaches is the use of personal technology as a lens through which to study humanity. They mostly rely on rich hardware sensors: smartphones now come equipped with GPS, magnetometers, barometers, accelerometers, and even photometers. Yet, it is the recent emergence of two new types of behavior sensing on smartphones – using software sensors and human sensors – that have the potential to revolutionize science since they allow us to conduct behavioral sensing.
Behavioral Sensing
Software sensors on smartphones conceptualize human actions through data. These sensors do not measure physical properties, but behavioral properties. For example, one’s calendar activities indicate routine and personal-work balance. Other software sensors include the amount of messages one sends or receives, or how many friends one has in their address book, as an indicator of one’s social engagement and support. There is a potential myriad of software sensors due to (i) our increasing use of smartphones to manage everyday activities, (ii) the maturity and diversity of application programing interfaces (APIs) available on smartphones and online, and (iii) ongoing efforts at harmonizing sensing on smartphones such as the AWARE framework. Thus, while much ongoing research focuses on the analysis of big data collected by the likes of Facebook or Google, software sensors are shifting the spotlight toward a decentralized model whereby personal devices can sense and react to smaller yet richer data in real-time.
Human sensors take advantage of smartphones’ ubiquity and interactive capabilities to collect data by querying humans. This resembles crowdsourcing, but with a rather real-time and localized perspective. Smartphones have been used to collect text and photo reports of earthquake aftermath or other crises (Rogstadius et al., 2013), but at the same time they can be used to collect qualitative data on a large scale, for example, comfort levels during heat waves and happiness levels at the workplace. Human sensors can be triggered in response to hardware and software sensor readings. They encapsulate our ability to collect in real-time data from large numbers of humans, and prompt a shift away from static data sets to live data sources in the study of humanity.
Opportunities and Challenges
What makes smartphones ideal scientific instruments? We argue that their ever-increasing sensing and computational capabilities, coupled with their popularity, makes them ideal for “peaking” into humanity in ways that existing scientific protocols cannot. They provide a means to complement lab and clinical studies, and ground scientific progress in naturalistic settings. Global application stores (i.e., appstores) now enable us to link smartphones into one collective, widely adopted scientific instrument, that records and reacts to data streams and enables different disciplines to collaborate on global challenges (Lang, 2011). Fundamentally, each one of us is personally motivated to maintain and protect their device, fix it when broken, and keep it alive with enough battery power. Unlike conventional scientific instruments that often require substantial investment by governments or institutions, smartphone maintenance is democratized.
These developments highlight the rise of what we call “ubiquitous instrumentation.” This trend is likely to see smartphones and other personal devices become increasingly valuable scientific instruments for studying humanity, enabling real-time public health studies, large-scale behavioral monitoring, and million-size cohort studies to name a few (Figure 1). Ironically, we argue that this trend will shift the focus from “big data” to “small data for the big picture,” and from data sets to data sources. For science, this means that methods dealing with big data sets will have to be complemented with methods for dealing with small, diverse, and intermittent data sources, while experiments that take data snapshots for analysis will require novel data dashboards for real-time monitoring. And maybe someday, a visit to the doctor may even entail a prescription for your phone.
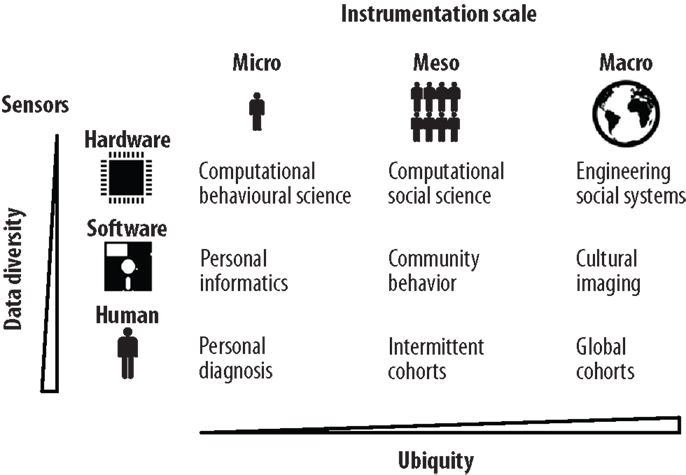
Figure 1. Smartphones enable instrumentation at the micro, meso, and macroscale. Orthogonally, there are three types of sensors that smartphones can provide: hardware sensors (to measure the environment), software sensors (to measure behavior), and human sensors (to query humans). Researchers are already using hardware sensors to address visions for computational behavioral science (Lightfoot, 2007), computational social science (Lazer et al., 2009), and engineering of social systems (Eagle and Greene, 2013). Software sensors allow for personal informatics (reflecting on one’s own behavior), community behavior (measuring the behavior of groups), and cultural imaging (global studies contrasting behavior). Human sensors allow for personal diagnosis (targeting queries to an individual’s needs), intermittent cohorts (querying multiple individuals based on their context), and global cohorts (engaging million-size groups across continents).
Of course, many challenges lie along the way. Crucially, not everyone has a mobile phone at the moment, with certain vulnerable populations (elders, children, poor) not having equal access to this technology. This can greatly affect the validity and reliability of any study, and care needs to be taken to ensure that representative samples are used. Furthermore, privacy is a major obstacle to this kind of work. To address this, we need ways to ensure that sensitive data remains on individuals’ devices, and only sanitized data are ever shared. Finally, the question of value is crucial: what is in it for users? We need to ensure that any type of ubiquitous instrumentation provides fair value to its users, either through the provision of useful information and services, empowerment, personalized advice, and the ability to self-reflect on ones’ own behavior.
Conflict of Interest Statement
The authors declare that the research was conducted in the absence of any commercial or financial relationships that could be construed as a potential conflict of interest.
References
Eagle, N., and Greene, K. (2013). Reality Mining: Using Big Data to Engineer a Better World. Cambridge MA: MIT Press.
Eagle, N., Macy, M., and Claxton, R. (2010). Network diversity and economic development. Science 328, 1029–1031. doi:10.1126/science.1186605
Haun, J. B., Castro, C. M., Wang, R., Peterson, V. M., Marinelli, B. S., Lee, H., et al. (2011). Micro-NMR for rapid molecular analysis of human tumor samples. Sci. Transl. Med. 3, ra16–ra71. doi:10.1126/scitranslmed.3002048
Pubmed Abstract | Pubmed Full Text | CrossRef Full Text | Google Scholar
Kim, J., Park, H., Bruce, J., Sutton, E., Rowles, D., Pucci, D., et al. (2013). The tongue enables computer and wheelchair control for people with spinal cord injury. Sci. Transl. Med. 5, ra166–ra213. doi:10.1126/scitranslmed.3006296
Pubmed Abstract | Pubmed Full Text | CrossRef Full Text | Google Scholar
Lang, T. (2011). Advancing global health research through digital technology and sharing data. Science 331, 714–717. doi:10.1126/science.1199349
Pubmed Abstract | Pubmed Full Text | CrossRef Full Text | Google Scholar
Lazer, D., Pentland, A., Adamic, L., Aral, S., Barabási, A.-L., Brewer, D., et al. (2009). Computational social science. Science 323, 721–723. doi:10.1126/science.1167742
Lightfoot, D. W. (2007). Social and behavioral scientists building cyber infrastructure. First Monday 12. doi:10.5210/fm.v12i6.1907
Malmgren, R. D., Stouffer, D. B., Motter, A. E., and Amaral, L. A. N. (2008). A Poissonian explanation for heavy tails in e-mail communication. Proc. Natl. Acad. Sci. U.S.A. 105, 18153–18158. doi:10.1073/pnas.0800332105
Pubmed Abstract | Pubmed Full Text | CrossRef Full Text | Google Scholar
Rogstadius, J., Teixeira, C., Vukovic, M., Kostakos, V., Karapanos, E., and Laredo, J. (2013). CrisisTracker: crowdsourced social media curation for disaster awareness. IBM J. Res. Dev. 57, 4:1–4:13. doi:10.1147/JRD.2013.2260692
Wang, P., González, M. C., Hidalgo, C. A., and Barabási, A.-L. (2009). Understanding the spreading patterns of mobile phone viruses. Science 324, 1071–1076. doi:10.1126/science.1167053
Pubmed Abstract | Pubmed Full Text | CrossRef Full Text | Google Scholar
Wesolowski, A., Eagle, N., Tatem, A. J., and Smith, D. L. (2012). Quantifying the impact of human mobility on malaria. Science 338, 267–270. doi:10.1126/science.1223467
Pubmed Abstract | Pubmed Full Text | CrossRef Full Text | Google Scholar
Keywords: smartphones, sensing, community, instrumentation, humans, ubiquitous computing
Citation: Kostakos V and Ferreira D (2015) The rise of ubiquitous instrumentation. Front. ICT 2:3. doi: 10.3389/fict.2015.00003
Received: 04 January 2015; Accepted: 10 February 2015;
Published online: 23 February 2015.
Edited by:
Alessandro Vinciarelli, University of Glasgow, UKReviewed by:
Yolanda Vazquez-Alvarez, University of Glasgow, UKCopyright: © 2015 Kostakos and Ferreira. This is an open-access article distributed under the terms of the Creative Commons Attribution License (CC BY). The use, distribution or reproduction in other forums is permitted, provided the original author(s) or licensor are credited and that the original publication in this journal is cited, in accordance with accepted academic practice. No use, distribution or reproduction is permitted which does not comply with these terms.
*Correspondence:dmFzc2lsaXNAZWUub3VsdS5maQ==