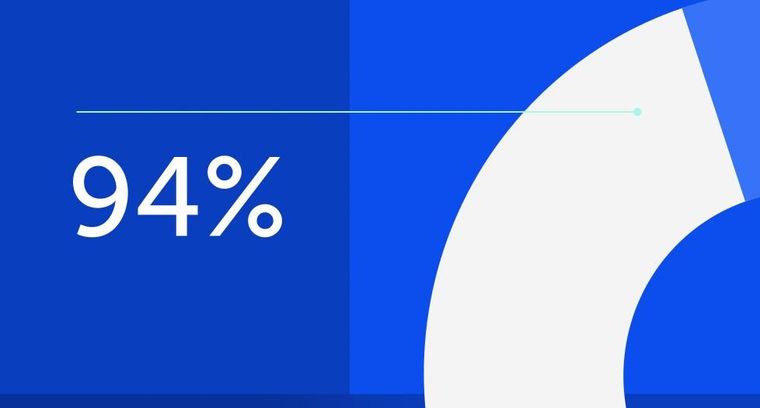
94% of researchers rate our articles as excellent or good
Learn more about the work of our research integrity team to safeguard the quality of each article we publish.
Find out more
ORIGINAL RESEARCH article
Front. ICT, 26 February 2015
Sec. Digital Education
Volume 2 - 2015 | https://doi.org/10.3389/fict.2015.00002
Distance learning is used in medical education, even if some recent meta-analyses indicated that it is no more effective than traditional methods. To exploit the technological capabilities, adaptive distance learning systems aim to bridge the gap between the educational offer and the learner’s need. A decrease of lexical competence has been noted in many western countries, so lexical competence could be a possible target for adaptation. The “Adaptive message learning” project (Am-learning) is aimed at designing and implementing an adaptive e-learning system, driven by lexical competence. The goal of the project is to modulate texts according to the estimated skill of learners, to allow a better comprehension. LexMeter is the first of the four modules of the Am-learning system. It outlines an initial profile of the learner’s lexical competence and can also produce cloze tests, a test based on a completion task. A validation test of LexMeter was run on 443 medical students of the first, third, and sixth year at the University “Sapienza” of Rome. Six cloze tests were automatically produced, with 10 gaps each. The tests were different for each year and with varying levels of difficulty. A last cloze test was manually created as a control. The difference of the mean score between the easy tests and the tests with a medium level of difficulty was statistically significant for the third year students but not for first and sixth year. The score of the automatically generated tests showed a slight but significant correlation with the control test. The reliability (Cronbach alpha) of the different tests fluctuated under and above 0.60, as an acceptable level. In fact, classical item analysis revealed that the tests were on the average too simple. Lexical competence is a relevant outcome and its assessment allows an early detection of students at risk. Cloze tests can also be used to assess specific knowledge of technical jargon and to train reasoning skill.
The use of information and communication technology to support education of healthcare professionals is increasingly growing and different approaches aimed at improving learning have been designed and tested (Mattheos et al., 2001; Sandars, 2012; Du et al., 2013). Distance learning programs have been addressed not only to cognitive but also to affective outcomes, including personal and professional growth, satisfaction, and connectedness (Patterson et al., 2012). Despite the large number of published articles, a clear evidence of the effectiveness of distance learning is still lacking (Tomlinson et al., 2013). A meta-analysis (Cook et al., 2008a) found and reviewed 201 eligible studies, to quantify the association of Internet-based instruction and educational outcomes for student and practicing physicians, nurses, pharmacists, dentists, and other health care professionals. The selected studies compared Internet-based instruction with a no-intervention or non-Internet control group. The results showed that Internet-based learning in the health professions is associated with large positive effects if compared with no intervention. In contrast, the difference of effect compared with non-Internet instructional methods is generally small, suggesting according to the authors that Internet-based learning has an effectiveness similar to traditional methods.
To achieve a better understanding of the variability of the observed outcomes in distance learning, the outcome has been correlated to students’ learning style, either automatically detected (González et al., 2012) or measured with manual methods (Cook et al., 2009; Cook, 2012; Groenwold and Knol, 2013), but a correlation was not observed. On the contrary, time spent working with the learning system was associated with improved learning efficiency (Cook et al., 2008b, 2010). A review of qualitative studies on health professionals’ experience of e-learning found five key themes as perceived elements of quality: effective peer communication, flexibility, learner’s support, knowledge validation, and course presentation and design focused on learners (Carroll et al., 2009).
With the aim to produce theory driven criteria to guide the development and evaluation of Internet-based medical courses, Wong et al. (2010) identified two main theories that explained variation in learners’ satisfaction and outcomes: Davis’s technology acceptance model (Davis, 1989) and Laurillard’s model of interactive dialog (Laurillard, 2002). Wong argued that learners were more likely to accept a course if it offered a perceived advantage over available non-Internet alternatives, was technically easy to use, and compatible with their values and norms. Interactivity led to effective learning only if learners were able to enter a dialog with a tutor, fellow students, or virtual tutorials and gain formative feedback.
Despite these considerations, according to Vertecchi (2010), at present distance education programs are still too focused on message communication, with the aim to overcome the time and space limits of traditional face-to-face education. On the contrary, it would be necessary to move from the simple transmission of the educational contents to the elaboration of the message, in order to make it coherent with the learning requirements of students.
Adaptive distance learning systems aim to bridge the gap between the educational offer and the learner’s need (Hodgins, 2007). In a comprehensive survey of adaptive methods, Knutov et al. (2009) proposed a model of adaptivity based on three component: the domain model (DM), the user model (UM), and the adaptivity model (AM). The DM is usually a system of concepts and relationships, representing the information of the knowledge domain addressed by the learning system, the UM represents user’s characteristics and goals, the AM represents the teaching model and the rules, which drive the adaptation of the system when reacting to the student’s behavior. In discussing the different methods of adaptivity used in the AM, Knutov identified five classes of adaptation (Table A1 in Appendix).
Until now, adaptation in the medical education domain has been mainly based on the enhancement of tutoring and cooperative aspects (Legg et al., 2009), adapting courses to learner’s cognitive and learning styles (Cook, 2012), or adapting information to students’ knowledge (Pagesy et al., 2002; Romero et al., 2006; Cook et al., 2008b). Contrasting effects were observed with the use of adaptive systems: a systematic review could not find any advantage of adaptive methods over traditionally based educational programs in changing dietary behavior (Harris et al., 2011), a randomized controlled trial showed instead a positive effect in efficiency for an adaptive system taking into account the learner’s prior knowledge (Cook et al., 2009).
Lexical competence is a possible target for adaptivity. It is defined as the ability to recognize and use words in a language, including the knowledge of the relationships among classes of words. Lexical competence is needed to comprehend texts presented in a course and it is one of the major factors in determining the efficacy of an educational message (Agrusti and Harb, 2013). There is evidence that in many industrialized countries adult literacy is regressing, a phenomenon, which is gradually increasing in spite of the considerable number of years of formal education during childhood and adolescence (Vertecchi, 2010)1. In USA, the last national survey on adult literacy, despite the effort put in this field, showed only some upward movement of low end (basic and below to intermediate) adult literacy levels together with a decline in the full proficiency group2. In Italy, the mean literacy score is low compared to other countries participating in the Survey of Adult Skills 2012 (see text footnote 1) and a study on a group of academic students between 20 and 30 years old showed that they knew approximately half of the words they were expected to know (Sobrero, 2009).
The “Adaptive message learning” project (Am-learning) is a project aimed at designing and implementing an adaptive e-learning system, whose adaptivity is based on lexical competence. The goal of the project is to bridge the gap between the skill required for the complete and correct comprehension of the educational contents in their initial formulation (undifferentiated) and the estimated skill of learners. This last variable was called the “word box,” intended as the estimated number of words a user knows and can use. The system is based on a two-step process, which starts with the assessment of lexical competence. In the second step, the estimate of the lexical competence is used to modulate the text of the course. The aim is to obtain the best possible match between the course text and the ability of the learners to understand it, based on their word box.
The hypothesis of the project was that if students pass through several cycles of reading of modulated texts they will expand their word box and – ultimately – improve their learning with respect to the study of undifferentiated texts.
This article briefly describes the overall architecture of the system, with a special attention to the module assessing lexical competence (LexMeter module) and reports about the first validation experiment of the LexMeter module.
In Am-learning project, the lexical competence was estimated through cloze tests.
The cloze test was created by Taylor (1956) in 1956 as an alternative to traditional tests used for the assessment of reading comprehension skills. It consisted of a completion task in which the subject had to complete the missing words in a text by choosing the correct ones. The test could include a list of words containing the correct answers or have no suggestions. In this last case, the subjects have to rely completely on their lexical knowledge.
The result of a cloze test depends on three specific skills:
(a) basic knowledge of words (vocabulary knowledge);
(b) ability to recall context-related meaning of words;
(c) prediction of the missing word through the use of domain specific knowledge for the interpretation of the meaning of a sentence. Choosing, for example, the appropriate logical connector or adverb to fill a blank in the text (e.g., together/instead, many/few, …) is not a matter of grammatical correctness but it relies on domain knowledge.
The system developed in Am-learning project is based on four modules:
• LexMeter module: it outlines an initial profile estimating the learner’s characteristics depending on his/her lexical competence.
• ProgressMeter module: using the same method of the LexMeter module, it creates tests to monitor the learner’s gradual improvement through the learning path, adapting the level of difficulty of tests to the estimated “word box” of the learner and increasing the difficulty as the learner progresses.
• Adapter module: it uses the results obtained by the two first modules and automatically adjusts the course contents (message).
• A learning management system (LMS): it hosts the three specific modules and support all the usual functionalities of a LMS.
Figure 1 shows the architecture of the system, technical details about the modules can be found in (Agrusti, 2010; Agrusti and Harb, 2011).
Lexmeter is not only a tool to assess lexical competence but also an automated system to create cloze tests with a user-defined level of lexical difficulty. It relies on a lexicon of words and on a database, called “the library,” which is a collection of texts produced and stored by the user. These texts are used to produce cloze tests. It is possible to retrieve texts from the library and group them into virtual archives to have collections grouped for domain or topic.
The lexicon is composed of words derived from many different sources: textbooks of medicine, monographs, and scientific articles. Also, the library contributes to the production of the lexicon. Texts are chunked in single words and words are automatically tagged with their type as part of speech (noun, verb, adjective, …) by computational linguistics open source routines. Each single occurrence of word is tagged also with its canonical form (lemma), to take into account the inflections of a term (singular/plural, different moods of a verb, …). Lemmatization allows LexMeter to group together the different inflected forms of a word so that they can be analyzed as a single item. The list of common words (LCW) contains the terms defined as empty of meaning and which primarily have only a grammatical meaning (auxiliary, conjunction, preposition).
When a user-defined text is considered to be transformed into a cloze test, the lexical frequency of its words is computed, discarding all the words contained in the LCW. A word not contained in this list is considered as eligible. LexMeter also allows to consider only a specific part of speech (noun, verb, adjective) to be selected for the creation of gaps and to mark some parts of a text as invariant, not to be considered for the creation of gaps.
We can now summarize the process of production of a cloze test by the LexMeter module:
1. The teacher selects a series of suitable and coherent texts from the library (an archive).
2. LexMeter assigns a lexical frequency to all the eligible words in the selected texts.
3. A specific text used as the basis of the cloze test is selected by the teacher (original text). The user selects a valid range of frequency of eligible words, setting the lower and the upper threshold of frequency. The wider the gap, the larger the range of eligible words, either very frequent or very unusual. Decreasing the lower threshold produces a more easy cloze test, in which more frequently used words are more likely to be selected and deleted in the text to produce the gaps and vice versa (Figure 2).
4. LexMeter compares any eligible word of the original text with those included in the chosen range of lexical frequency and searches for correspondences.
5. When a correspondence is found in the selected range of frequency, the word is extracted from the text, put into the Solutions List of the test and its place in the text is substituted with a gap. LexMeter flags the word as the correct solution for that gap.
6. At the end, the program shows the resulting modified text. The teacher can accept the proposed test or modify it, asking the system to produce a different choice of gaps. The final accepted version can be printed to be used for an at-presence test of delivered by an e-learning system. If LexMeter is embedded in an e-learning platform, automated correction, and scoring of tests is provided.
Figure 2. The mechanism of selection of the thresholds of difficulty of words to be selected for the creation of gaps in the cloze tests. The word “paziente” (patient) has 1717 instances in the lexicon. It is then more frequent and – in principle – more easy to be guessed than the word “atriale” (atrial: 481 instances).
The Am-learning project was presented during three plenary meetings and students were invited to participate on a voluntary basis. Only 9% of the students attending the presentation meetings left the lecture hall without completing the test. A convenience sample of 443 medical students from the first (n. 230), third (n. 130), and sixth (n. 83) year was then recruited. The difference in numbers among years reflects the difference in attending students: the high number of first year students was due to a change in academic policy, resulting in an increase in students admitted to the curriculum, sixth year students were less because some of them were in clinical rotation at the time of the experiment and then not available to join the experimental activity.
We assessed the reliability of the cloze tests automatically generated by LexMeter and tested their efficacy in a reading comprehension task by comparing them with a similar test manually generated by the researchers.
Two different cloze tests, with 10 gaps each, were automatically generated by LexMeter (tests A and B). Test A was generated with a low threshold of difficulty, while test B with a medium level. A control cloze test (test C) was manually produced by a member of the research team. Tests A and B were different for each year, test C was the same for all years. All tests consisted of 500–600 words. Students had to complete the text identifying the missing word out of a list of 15 words: 10 valid options and 5 distractors.
In order to assure that all the students who accepted to join the experiment fulfilled the task, we did not deliver the comprehension tasks through the e-learning platform but we printed them on paper and the test was run during the plenary meeting, after the presentation of the project. The score was computed manually by the research team. The highest possible score was 10 points.
Classical item analysis was carried out, to describe the features of the tests. Reliability was expressed as internal consistency by the Cronbach alpha index, the correlation between the automatically generated tests and the control test was expressed as correlation coefficient. A value of Cronbach alpha >0.6 was considered acceptable for an experimental testing environment (Streiner and Norman, 1995).
The topic of all the texts for the comprehension tasks was the cardiovascular system. The texts were part of the “cardiology archive,” which contained 65 texts in.txt format (from books and papers or purposively produced by the project team), with a size of about 2.2 Mb. The lexicon consisted of 17,561 unique words, corresponding to 13,267 lemmas (6,145 lemmas of nouns and adjectives). The topics of A and B texts were coherent with the expected level of knowledge for the three different years: method of measurement of arterial blood pressure and functional anatomy of vessels for the first year, platelet aggregation and thrombogenesis for the third year, thrombosis and laboratory tests for thrombofilia for the sixth year. The topic of the C text was the physiology of coagulation. The three tests were administered in the same order for the three years: test A first, then B, and finally C.
The measures of the distribution of the score of tests are described in Table 1.
Figures 3–5 summarize the distribution of scores for the 3 years.
Figure 3. The distribution of the score for the two cloze tests of the first year students. On the vertical axis the number of students, on the horizontal axis the scores.
Figure 4. The distribution of the score for the two cloze tests of the third year students. On the vertical axis the number of students, on the horizontal axis the scores.
Figure 5. The distribution of the score for the two cloze tests of the sixth year students. On the vertical axis the number of students, on the horizontal axis the scores.
The difference of the mean score between test A (easy level of difficulty) and test B (medium level of difficulty) was statistically significant for the third year students (t-test <0.001) but not for first and sixth year.
The cloze tests showed different measures of reliability as shown in Table 2.
Table 2. Internal consistency of the seven different cloze tests, expressed as Cronbach alpha index.
The Cronbach alpha for the three tests of the first year students was 0.63, 0.68 for the third year students, and 0.41 for the sixth year students.
Table 3 shows the overall difficulty index for the items of the three tests.
Table 3. Difficulty index for the items of the three tests: no. of wrong answers/total number of respondents.
Overall, the A cloze tests had a correlation coefficient with the C tests of 0.32, which was statistically significant (p < 0.01), while the B tests had only a 0.17 correlation coefficient, although statistically significant as well (p < 0.01). The highest correlation was showed by the third year tests, with a r = 0.582 between A and C and r = 0.48 between B and C (both p < 0.01).
This experiment was meant to test the ability of the LexMeter module to produce cloze tests from a library of texts, by assessing the reliability of the produced tests and by comparing them to a manually produced cloze test.
Our results show that the LexMeter module generated cloze tests whose reliability was comparable with the manually generated tests. Overall, the reliability of the six tests generated by LexMeter fluctuated under and above the boundary of acceptability and items analysis showed that the tests were probably too simple to be more reliable. In fact, only 3 out of the total 30 items displayed a difficulty index >0.30 (number of wrong answers/total answers). This was particularly evident for the sixth year students, who had very high scores and whose tests had a very low reliability. When the variance of the score of a test is too small, the resulting reliability is low. Nevertheless, as shown in Figures 3–5, it was possible to identify a subset of students of the first and third year, who had a lower, potentially problematic level of lexical competence.
Furthermore, setting the threshold in order to have more infrequent words selected for the gaps would lead LexMeter to choose words from the technical jargon. In this case, the cloze test would assess the specific terminological knowledge and this is particularly valuable in the first 3 years.
This study has some limitations. The first one is due to the selection of a single cohort of students from a single institution. Although the overall sample was rather wide, it was unbalanced among years. A second possible limitation is linked to the motivation of students. They knew they were cooperating to an experiment and that they were not assessed in a formal way. This could have caused some of the low scores, especially among the sixth year students. Finally, since the tests were administered in the same order for the 3 years, a fatigue effect could have hampered the results of C test, which was the last student faced. Nevertheless, in our opinion the experiment gave relevant information about the effectiveness of the LexMeter module and about the best way to use lexical competence as an indicator.
The identification of medical students at risk of failure is a diffuse problem and many faculties are devoting more and more resources to remediation paths (Cleland et al., 2013). As discussed in the Section “Introduction,” there is a global trend in many western countries toward a decrease of lexical competence and a level under the mean for this competence could be a good early indicator of risk of failure of a student. A set of repeated cloze tests of different level of difficulty could be useful to draw the profile of lexical competence of each student and this could be easily done through an e-learning platform, as a mandatory assignment of formative assessment.
A recent review of the literature led to the formulation of twelve recommendations to develop the students’ clinical reasoning skills (Rencic, 2011). The authors suggested to capitalize every clinical experience to increase the stock of situations on which the recognition of disease may be based and to train the patho-physiological reasoning, namely, that form of deductive reasoning that starts from biological facts to interpret clinical facts. Attention was also devoted to the development of reasoning on the basis of epidemiological and probabilistic arguments. The Am-learning system could be used to propose reading and completion exercises of argued clinical texts. These texts may be composed of sections that expose clinical facts through short narratives and lists of clinical data, interspersed with sections of arguments, in which the narrative and laboratory-instrumental data are interpreted and discussed in their patho-physiological and epidemiological meaning. If the author of the exercise selected a high frequency range for the terms to be eliminated to create the gaps and marked as invariant the sections with clinical facts – which represent the data of the problem – it is very likely that verbs and connectors would be selected, rather than words of the technical jargon. This would require the students to read the text in a particularly careful and active way, in order to reconstruct the logic of sentences, and this could be helpful in developing their reasoning skill.
All the authors of this article gave together a substantial contribution to the design of the experimental work as members of the Am-learning project team, to the draft and to the revised version of the article. More in particular, FC did statistic calculations and drafted the article, SB critically revised the article, MP and ET acquired the experimental data, AL revised the second draft of the article and the texts for the production of cloze tests. All the authors read and approved the final version of the article and are accountable for all aspects of the work in ensuring that questions related to the accuracy or integrity of any part of the work are appropriately investigated and resolved.
The authors declare that the research was conducted in the absence of any commercial or financial relationships that could be construed as a potential conflict of interest.
Am-Learning project was funded by the Ministry for Education University and Research (MIUR) and carried out by the Department of Educational Design – University of Roma Tre (Scientific Director Prof. B. Vertecchi), the Department of Experimental Medicine – “Sapienza” University of Rome (Scientific Director Prof. AL) and Social, Cognitive, and Quantitative Science Department – University of Modena e Reggio Emilia (Scientific Director Prof. L. Cecconi).
Agrusti, F. (2010). From LexMeter to adapter. Towards a match up between the virtual and the real reader. CADMO 1, 97–108. doi: 10.3280/CAD2010-001010
Agrusti, F., and Harb, N. (2011). Progressmeter. A transitional step-by-step solution aiding the evaluation of learners’ gradual progress. CADMO 19, 118–120. doi:10.3280/CAD2011-001012
Agrusti, F., and Harb, N. (2013). “A new individualized on-line learning experience,” in Proceedings of the European Distance and E-Learning Network; 2013 Annual Conference, Oslo, 12-15 June, ed. M. F. Paulsen (Budapest: European Distance and E-Learning Network).
Carroll, C., Booth, A., Papaioannou, D., Sutton, A., and Wong, R. (2009). UK health-care professionals’ experience of on-line learning techniques: a systematic review of qualitative data. J. Contin. Educ. Health Prof. 29, 235–241. doi:10.1002/chp.20041
Pubmed Abstract | Pubmed Full Text | CrossRef Full Text | Google Scholar
Cleland, J., Leggett, H., Sandars, J., Costa, M. J., Patel, R., and Moffat, M. (2013). The remediation challenge: theoretical and methodological insights from a systematic review. Med. Educ. 47, 242–251. doi:10.1111/medu.12052
Pubmed Abstract | Pubmed Full Text | CrossRef Full Text | Google Scholar
Cook, D. A. (2012). Revisiting cognitive and learning styles in computer-assisted instruction: not so useful after all. Acad. Med. 87, 778–784. doi:10.1097/ACM.0b013e3182541286
Pubmed Abstract | Pubmed Full Text | CrossRef Full Text | Google Scholar
Cook, D. A., Levinson, A. J., and Garside, S. (2010). Time and learning efficiency in Internet-based learning: a systematic review and meta-analysis. Adv. Health Sci. Educ. Theory Pract. 15, 755–770. doi:10.1007/s10459-010-9231-x
Pubmed Abstract | Pubmed Full Text | CrossRef Full Text | Google Scholar
Cook, D. A., Levinson, A. J., Garside, S., Dupras, D. M., Erwin, P. J., and Montori, V. M. (2008a). Internet-based learning in the health professions: a meta-analysis. JAMA 300, 1181–1196. doi:10.1001/jama.300.10.1181
Pubmed Abstract | Pubmed Full Text | CrossRef Full Text | Google Scholar
Cook, D. A., Beckman, T. J., Thomas, K. G., and Thompson, W. G. (2008b). Adapting web-based instruction to residents’ knowledge improves learning efficiency: a randomized controlled trial. J. Gen. Intern. Med. 23, 985–990. doi:10.1007/s11606-008-0541-0
Pubmed Abstract | Pubmed Full Text | CrossRef Full Text | Google Scholar
Cook, D. A., Thompson, W. G., Thomas, K. G., and Thomas, M. R. (2009). Lack of interaction between sensing-intuitive learning styles and problem-first versus information-first instruction: a randomized crossover trial. Adv. Health Sci. Educ. Theory Pract. 14, 79–90. doi:10.1007/s10459-007-9089-8
Pubmed Abstract | Pubmed Full Text | CrossRef Full Text | Google Scholar
Davis, F. D. (1989). Perceived usefulness, perceived ease of use, and user acceptance of information technology. MIS Q. 13, 319–340. doi:10.2307/249008
Du, S., Liu, Z., Liu, S., Yin, H., Xu, G., Zhang, H., et al. (2013). Web-based distance learning for nurse education: a systematic review. Int. Nurs. Rev. 60, 167–177. doi:10.1111/inr.12015
Pubmed Abstract | Pubmed Full Text | CrossRef Full Text | Google Scholar
González, C., Blobel, B., and López, D. M. (2012). Adaptive intelligent systems for pHealth – an architectural approach. Stud. Health Technol. Inform. 177, 170–175. doi:10.3233/978-1-61499-069-7-170
Pubmed Abstract | Pubmed Full Text | CrossRef Full Text | Google Scholar
Groenwold, R. H., and Knol, M. J. (2013). Learning styles and preferences for live and distance education: an example of a specialisation course in epidemiology. BMC Med. Educ. 13:93. doi:10.1186/1472-6920-13-93
Pubmed Abstract | Pubmed Full Text | CrossRef Full Text | Google Scholar
Harris, J., Felix, L., Miners, A., Murray, E., Michie, S., Ferguson, E., et al. (2011). Adaptive e-learning to improve dietary behaviour: a systematic review and cost-effectiveness analysis. Health Technol. Assess. 15, 1–160. doi:10.3310/hta15370
Pubmed Abstract | Pubmed Full Text | CrossRef Full Text | Google Scholar
Hodgins, W. (2007). Distance education but beyond: “meLearning” – what if the impossible isn’t? J. Vet. Med. Educ. 34, 325–329. doi:10.3138/jvme.34.3.325
Pubmed Abstract | Pubmed Full Text | CrossRef Full Text | Google Scholar
Knutov, E., De Bra, P., and Pechenizkiy, M. (2009). AH 12 years later: a comprehensive survey of adaptive hypermedia methods and techniques. New Rev. Hypermedia Multimedia 15, 5–38. doi:10.1080/13614560902801608
Laurillard, D. (2002). Rethinking University Teaching: A Conversational Framework for the Effective Use of Learning Technologies, Second Edn. London: Routledge.
Legg, T. J., Adelman, D., Mueller, D., and Levitt, C. (2009). Constructivist strategies in online distance education in nursing. J. Nurs. Educ. 48, 64–69. doi:10.3928/01484834-20090201-08
Pubmed Abstract | Pubmed Full Text | CrossRef Full Text | Google Scholar
Mattheos, N., Schittek, M., Attström, R., and Lyon, H. C. (2001). Distance learning in academic health education. Eur. J. Dent. Educ. 5, 67–76. doi:10.1034/j.1600-0579.2001.005002067.x
Pagesy, R., Soula, G., and Fieschi, M. (2002). Enhancing a medical e-learning environment: the adaptive DI2@DEM approach. Stud. Health Technol. Inform. 90, 745–751. doi:10.3233/978-1-60750-934-9-745
Pubmed Abstract | Pubmed Full Text | CrossRef Full Text | Google Scholar
Patterson, B. J., Krouse, A. M., and Roy, L. (2012). Student outcomes of distance learning in nursing education: an integrative review. Comput. Inform. Nurs. 30, 475–488. doi:10.1097/NXN.0b013e3182573ad4
Pubmed Abstract | Pubmed Full Text | CrossRef Full Text | Google Scholar
Rencic, J. (2011). Twelve tips for teaching expertise in clinical reasoning. Med. Teach. 33, 887–892. doi:10.3109/0142159X.2011.558142
Pubmed Abstract | Pubmed Full Text | CrossRef Full Text | Google Scholar
Romero, C., Ventura, S., Gibaja, E. L., Hervás, C., and Romero, F. (2006). Web-based adaptive training simulator system for cardiac life support. Artif. Intell. Med. 38, 67–78. doi:10.1016/j.artmed.2006.01.002
Pubmed Abstract | Pubmed Full Text | CrossRef Full Text | Google Scholar
Sandars, J. (2012). Technology and the delivery of the curriculum of the future: opportunities and challenges. Med. Teach. 34, 534–538. doi:10.3109/0142159X.2012.671560
Pubmed Abstract | Pubmed Full Text | CrossRef Full Text | Google Scholar
Sobrero, A. A. (2009). L’incremento della competenza lessicale, con particolare riferimento ai linguaggi scientifici. Italiano Lingua Due 1, 211–225. doi:10.13130/2037-3597/441
Streiner, D. L., and Norman, G. R. (1995). Health Measurement Scales. A practical Guide to Their Development and Use. Oxford: Oxford Medical Publications.
Taylor, W. L. (1956). Recent developments in the use of cloze procedure. Journalism Q. 33, 42–99. doi:10.1177/107769905603300106
Tomlinson, J., Shaw, T., Munro, A., Johnson, R., Madden, D. L., Phillips, R., et al. (2013). How does tele-learning compare with other forms of education delivery? A systematic review of tele-learning educational outcomes for health professionals. NSW Public Health Bull. 24, 70–75. doi:10.1071/NB12076
Pubmed Abstract | Pubmed Full Text | CrossRef Full Text | Google Scholar
Vertecchi, B. (2010). New hypotheses for the development of e-learning. J. e Learn. Knowl. Soc. 6, 29–38.
Wong, G., Greenhalgh, T., and Pawson, R. (2010). Internet-based medical education: a realist review of what works, for whom and in what circumstances. BMC Med. Educ. 10:12. doi:10.1186/1472-6920-10-12
Pubmed Abstract | Pubmed Full Text | CrossRef Full Text | Google Scholar
Keywords: adaptive system, cloze test, distance learning, lexical competence, clinical reasoning
Citation: Consorti F, Basili S, Proietti M, Toscano E and Lenzi A (2015) LexMeter: validation of an automated system for the assessment of lexical competence of medical students as a prerequisite for the development of an adaptive e-learning system. Front. ICT 2:2. doi: 10.3389/fict.2015.00002
Received: 03 December 2014; Accepted: 08 February 2015;
Published online: 26 February 2015.
Edited by:
Xiaoxun Sun, Australian Council for Educational Research, AustraliaReviewed by:
Anthony Philip Williams, Avondale College of Higher Education, AustraliaCopyright: © 2015 Consorti, Basili, Proietti, Toscano and Lenzi. This is an open-access article distributed under the terms of the Creative Commons Attribution License (CC BY). The use, distribution or reproduction in other forums is permitted, provided the original author(s) or licensor are credited and that the original publication in this journal is cited, in accordance with accepted academic practice. No use, distribution or reproduction is permitted which does not comply with these terms.
*Correspondence: Fabrizio Consorti, Department of Surgical Sciences, Sapienza University of Rome, Viale del Policlinico, Rome 00161, Italy e-mail:ZmFicml6aW8uY29uc29ydGlAdW5pcm9tYTEuaXQ=
Disclaimer: All claims expressed in this article are solely those of the authors and do not necessarily represent those of their affiliated organizations, or those of the publisher, the editors and the reviewers. Any product that may be evaluated in this article or claim that may be made by its manufacturer is not guaranteed or endorsed by the publisher.
Research integrity at Frontiers
Learn more about the work of our research integrity team to safeguard the quality of each article we publish.