Ever since the surface recordings of electrical brain activity were introduced as electroencephalography (EEG) by
Berger (1929)
, oscillatory patterns in the brain’s electrical activity have been investigated intensively. In recent years, a special interest for oscillations in the gamma frequency range (40–80 Hz) has emerged in the neurosciences (
Basar-Eroglu et al., 1996
;
Canolty et al., 2006
;
Edwards et al., 2005
). Oscillatory neuronal activity in the gamma frequency range accompanies a wide range of cognitive and perceptual operations. Processes typically related to gamma oscillations include binding (
Muller et al., 1997
;
Tallon-Baudry et al., 1996
), meaningful object perception (
Keil et al., 1999
;
Tallon-Baudry et al., 1997
), memory (
Gruber and Muller, 2005
;
Gruber et al., 2004
;
Herrmann et al., 2004b
;
Howard et al., 2003
), and attention (
Debener et al., 2003
;
Herrmann and Mecklinger, 2000
;
Karns and Knight, 2009
;
Tiitinen et al., 1993
).
Gamma phenomena can be grouped into at least three categories: (i) spontaneous gamma oscillations, (ii) induced gamma oscillations, and (iii) evoked gamma responses (
Basar-Eroglu et al., 1996
). Spontaneous oscillations occur without correlation to sensory stimulation. Induced gamma activity consists of oscillatory bursts whose latency jitters from trial to trial. Thus, the temporal relationship with the stimulus onset is loose. In contrast, evoked gamma-band responses (eGBRs), most consistently recorded from human scalp, occur in an earlier time window than induced gamma oscillations and are phase-locked to the stimulus, i.e. show inter-trial phase synchrony. Simple sensory stimuli evoke gamma responses in the cortex and subcortical structures (
Basar, 1980
;
Demiralp et al., 1996
). The strength of the eGBR is influenced by several physical features of visual stimuli such as size and eccentricity (
Busch et al., 2004
), visual contrast (
Schadow et al., 2007
), or spatial frequency (
Frund et al., 2007a
). Furthermore, the eGBR is modulated by basic cognitive processes such as memory matching (
Herrmann et al., 2004b
), attention (
Busch et al., 2006
;
Debener et al., 2003
;
Fell et al., 2003
), and visual awareness (
Ohla et al., 2007
). Induced gamma-band responses (iGBRs), on the other hand have been associated with a wide range of cognitive processes including learning (
Axmacher et al., 2006
;
Gruber and Muller, 2006
;
Gruber et al., 2001
;
Keil et al., 2001
), perceptual binding (
Singer et al., 1997
), representation of objects (
Lachaux et al., 2005
;
Tallon-Baudry and Bertrand, 1999
;
Tallon-Baudry et al., 2005
), memory encoding and retrieval (
Gruber et al., 2004
;
Sederberg et al., 2003
), and working memory (
Howard et al., 2003
;
Tallon-Baudry et al., 1998
). It has been suggested that synchronous oscillations in the gamma range could group action potentials from different neurons. Such grouping could be used to solve the binding problem (
Singer and Gray, 1995
), facilitate attentional processing (
Fries et al., 2001a
,
b
), or enable hippocampal learning (
Axmacher et al., 2006
). Thus, while early eGBRs might reflect the encoding of physical attributes of the stimulus perceived, iGBRs could play a crucial role in binding together brain areas performing the task (
Tallon-Baudry and Bertrand, 1999
). However, the cognitive correlates of evoked and induced gamma responses widely overlap (
Debener et al., 2003
;
Engel et al., 2001
;
Fries et al., 2001b
;
Herrmann et al., 2004a
,
b
;
Tiitinen et al., 1993
), and therefore, it seems plausible to assume that similar brain regions are responsible for the generation of both types of gamma activity (
Herrmann and Demiralp, 2005
).
Interestingly, it has been consistently demonstrated, that the power of LFP oscillations in the gamma range closely corresponds to the blood oxygen level dependent (BOLD)-signal monitored by functional magnetic resonance imaging (fMRI). This tight relation of intracranially recorded LFPs and hemodynamic responses has been observed in the visual cortex of cat (
Niessing et al., 2005
) and monkey (
Logothetis, 2002
,
2003
;
Logothetis et al., 2001
), as well as in the human auditory cortex (
Mukamel et al., 2005
). Gamma oscillations in the human visual cortex measured with non-invasive electrophysiological measurements (EEG and MEG) resemble intracranial LFP measurements with respect to spatial, temporal, and functional properties (
Hall et al., 2005
). These non-invasive scalp MEG/EEG measures as well as intracranial EEG recordings of oscillatory gamma activity show high spatial correspondence with hemodynamic responses in the human visual cortex as well (
Brookes et al., 2005
;
Brovelli et al., 2005
;
Lachaux et al., 2007
;
Muthukumaraswamy and Singh, 2008
;
Winterer et al., 2007
). Beside this spatial correspondence, also a functional similarity between GBR and hemodynamic response has been reported. In a recent study,
Fiebach et al. (2005)
used a visual repetition priming paradigm and showed that induced GBRs in scalp recorded EEG and hemodynamic measures of brain activity exhibit a similar response pattern. Analogously,
Foucher et al. (2003)
demonstrated functionally corresponding iGBRs and BOLD activations in a visual target detection task.
Taken together, these observations suggest a close anatomical and functional correspondence between activation networks revealed by fMRI and EEG GBRs. However fMRI and EEG data each necessarily provide evidence of the underlying brain activity, although the extend of which they are measuring the same signal, or even signals from the same process, is indeterminate.
In humans, it has been demonstrated that GBRs show strong inter-individual variations in frequency and amplitude (
Busch et al., 2004
;
Demiralp et al., 2007
) and at the same time display considerable intra-individual reliability (
Frund et al., 2007c
). This suggests an individual gamma disposition as a personal trait. Accordingly, the gamma-trait has been associated with variations in individual performance in behavioral tasks. In particular, the stable inter-individual variations in the EEG gamma-band activity reliably separate individuals with a high rate of perceptual switches in a multistable perception task from individuals with a low rate (
Struber et al., 2000
). The individual GBR is also selective for individual reaction times (
Jokeit and Makeig, 1994
) and the ability of an individual to be hypnotizable (
De Pascalis et al., 1987
). Thus, the individual eGBR to a given visual stimulus displays inter-individual variability, intra-individual reliability and is valid in terms of a behavioral relevance fulfilling the minimal requirements for a psychological trait.
In the present study, we investigated the relation between hemodynamic responses and evoked GBRs in response to visual stimulation. We focused our investigation on eGBR, since iGBRs could be confounded with saccadic eye movement artifacts when using moving stimuli (
Yuval-Greenberg et al., 2008
), while eGBRs are unaffected by these ocular muscle activity (
Pope et al., 2009
). We used moving stimuli, because the human area MT can reliably be stimulated by these stimuli and shows inter-individual variation in functional imaging studies (
Malikovic et al., 2007
).
Results of this investigation will extend our knowledge about the functional architecture of the human brain and will shed further light onto our understanding of the dynamic relation between the BOLD signal and the properties of the electrical activity recorded on the scalp. In particular, we tested the hypotheses that human GBRs and BOLD responses covary intra-individually as a function of stimulation as well as inter-individually as a function of gamma-trait.
Participants
Seventeen neurologically healthy (right handed) adults participated in this study (10 female). After a full explanation of the nature and risks of the study, all participants gave their informed consent according to a protocol approved by the local ethics committee. All participants had normal or corrected-to-normal vision and were free of neurological and psychiatric disorders. The selected sample group was homogeneous with respect to age (mean age 23.71 years, SD: 2.97).
Stimuli
All participants underwent separate EEG and fMRI experimental sessions. In each session, participants viewed circular sine wave gratings (diameter 15°; spatial frequency of 0.67 cycles/degree; contrast 50%) either moving outward (standard, 75%) or inward (target, 25%). Gratings were presented for 660 ms, during which a full cycle of movement occurred (cf. Figure
1
).
Figure 1. Schematic description and timing of the experimental trial for Condition 1 (left) and Condition 2 (right).
The participants were to indicate an inward moving grating (target stimulus) by pressing a button with their right index finger. Within each session (EEG and fMRI), two experimental conditions were performed. In Condition 1, a static grating remained visible during a variable interstimulus interval (ISI) of 1000–4000 ms. Thus, at the onset of a stimulus only a motion onset occurred. In Condition 2, a grey screen was presented during ISI. Thus, at stimulus onset both the stimulus and the motion set on. Timing was identical for the fMRI and EEG recording. Despite the advantages associated with parallel acquisition protocols (
Herrmann and Debener, 2008
), in the present study we utilized a serial EEG–fMRI measurement design to rule out any possible confounds of the evoked gamma response measurements with the acoustic and electromagnetic emissions of the MR system.
Procedure and Data Analysis
EEG
The EEG session was performed in an electrically shielded, sound-attenuated, and dimly lit cabin (IAC, Niederkruchten, Germany). The stimulation monitor was placed outside the cabin behind an electrically shielded window. All devices inside the cabin were battery-operated to avoid line frequency interference (50 Hz in Germany). The EEG was measured from 31 scalp locations according to an extended 10–20 system and amplified using a BrainAmp amplifier (Brain Products, Munich, Germany). An electrode placed on the nose served as reference. In order to detect artifacts due to eye movements, an electrode placed below the orbital rim was used to record the electrooculogram (EOG). Activity was recorded using sintered Ag/AgCl electrodes mounted in an elastic cap (Easycap, Falk Minow, Munich, Germany). Electrode impedances were kept below 5 kΩ. EEG data were acquired with a band-pass filter of 0.016–250 Hz and a sampling rate of 500 Hz. A fiber-optic cable transferred the digitized EEG to a computer outside the recording cabin. A digital high-pass filter with a cutoff frequency of 0.5 Hz was applied offline in order to reduce slow shifts in the baseline. If participants moved their eyes away from the fixation cross, it was detected by measurements of EOG activity and the trial was discarded. For this purpose, an automatic artifact detection was computed, which excluded trials from further analysis if the standard deviation within a moving 200 ms window exceeded 40 μV in any channel. The automatic artifact rejection was supplemented by visual inspection of every trial to ensure that only trials without artifacts were included in the subsequent analysis.
Event-related potentials (ERPs) were computed as averages of all artifact-free trials of a given condition. GBRs were derived from the EEG by means of the wavelet transform (Morlet wavelet, time–frequency localization at 40 Hz with a time–frequency resolution of 2σt ≈ 50 ms and 2σf ≈ 13 Hz). The wavelet transform was evaluated for center frequencies from 1 to 90 Hz in steps of 1 Hz. If the grid, on which a wavelet had been evaluated, extended over the borders of a trial, the signal was padded with zeros. The convolutions required to evaluate the wavelet transform were performed using custom-made software written in C. Amplitudes were scaled to conserve the amplitudes of sine wave test signals, rather than total signal energy. Thus, the wavelet transform at a single frequency corresponded to a filtered analytical version of the original signal. At each frequency point, the amplitude for that frequency in a 200- to 100-ms prestimulus baseline period was subtracted. The evoked activity, which is the mean amplitude of the wavelet transform of the ERP, was extracted from the wavelet transform. In order to perform statistical analyses, mean time courses of the frequency band of the response in the time range between 80 and 120 ms after stimulus onset and a frequency range between 45 and 55 Hz were selected for further analysis. To avoid loss of statistical power, electrodes were pooled into a region of interest (ROI). Responses were evaluated from a posterior ROI (electrodes PO7 PO3 POz PO4 PO8 O1 Oz O2). The ROI was chosen from those electrodes that displayed a strong signal change after stimulation. Repeated measurements analysis of variance was used to judge the statistical significance of the factor Stimulation (Condition 1 vs. Condition 2).
For illustration of individual data, low-resolution brain electromagnetic tomography (LORETA) was performed to model the cortical sources of the individual gamma-band activity (
Pascual-Marqui et al., 2002
). For each participant, the ERP for Condition 2 was band-pass filtered corresponding to the individual eGBR frequency and LORETA current density values (μA/mm
2) were calculated within the 3D voxel space. The maxima of the current density distributions were displayed on a cortical surface model and transformed in stereotactic Talairach space (
Talairach and Tournoux, 1988
).
fMRI
In the fMRI experiment, images were acquired using a 3-T whole body scanner (Siemens TRIO) equipped with an eight-channel head coil, radiofrequency transmission and signal reception. Using a midsagittal scout image, 32 axial slices (slice thickness 4 mm) of a T2* weighed gradient echo EPI sequence were acquired parallel to the bicommissural plane and covering the whole brain. The following acquisition parameters were used: repetition time: 2 s, echo time: 30 ms, flip angle: 80°, voxel size: 3.5 × 3.5 × 4 mm3. A total of 600 volumes divided into two runs each lasting 10 min were acquired in the course of the experiment. Additionally, a high-resolution anatomical scan was acquired for each participant.
The fMRI data analysis was performed using MATLAB 7 (Mathworks Inc., Natick, MA, USA), the SPM5 software package, and the MarsBar toolbox for SPM. Preprocessing of functional MR images included movement correction and coregistration with the anatomical data. The scans were then normalized to a standardized brain space (defined by the Montreal Neurological Institute) using a non-linear transformation matrix (voxel size 2 mm × 2 mm × 2 mm) and smoothed using a Gaussian kernel with 8-mm full-width at half-maximum (FWHM). The EPI time series was analyzed using a general linear model (GLM) analysis (
Friston et al., 1995
). Separate regressors were defined for each condition using the canonical hemodynamic response function as the reference waveform. We used separate regressors for the two standard stimuli of each condition (standard of Condition 1 and standard of Condition 2), and also modeled the two target stimuli of each condition as effects of no interest. After estimation of the model parameters for each subject, an analysis of variance was calculated for the whole group, using the individual contrast images for the main effects. In a first step, we compared the two experimental conditions separately with the resting baseline. The results of these statistics were thresholded using an extent threshold of
p-value = 0.001 [family-wise error rate (FWE) adjusted,
k = 85 voxels], and
t = 4.5,
p = 0.005 [false discovery rate (FDR) adjusted]. To investigate within-subject effects, we directly compared the two experimental conditions. Results of the statistic were thresholded by
p = 0.001 (FWE adjusted,
k = 156 voxels), and
t = 3.7,
p = 0.001. To investigate between-subject effects, we performed a correlation analysis using the mean amplitude of the individual eGBR as a regressor. Here, only hemodynamic and electrophysiological data corresponding to Condition 2 were assessed since Condition 1 failed to elicit stable evoked GBR. Results of these statistics were thresholded by
p = 0.001 (
k = 259 voxels) and a
t-value of 2.6.
To further explore the results of these statistics, a post-hoc ROI analysis was performed. ROIs were functionally defined using the peak activated cortical voxel as the center and a sphere with a radius of 6 mm for the correlation of eGBR and BOLD. For each individual subject, averaged beta-values were collected from each ROI. Generally, beta values are estimates of the relationship between a covariate (e.g. experimental events) and data (e.g. fMRI data). They are the regression coefficients for the GLM and can be interpreted as the amplitude of the hemodynamic response function. Thus, beta values represent a numerical estimate of the effect size in the fMRI analysis.
Within-Subject Effects
Figure
2
shows hemodynamic (fMRI) and electrophysiological (evoked GBR) data for Condition 1 and Condition 2. Compared to resting baseline, bilateral hemodynamic responses of area V5/MT were observed for both conditions, and in the V1/V2 complex for Condition 2 (cf. Table
1
). The right panel of Figure
2
displays time–frequency representations of evoked oscillatory activity. Averages across all participants are plotted separately for Condition 1 (top) and Condition 2 (bottom). A clear eGBR at approximately 100 ms after stimulus onset and a mean frequency of 50 Hz emerged in Condition 2. In Condition 1, no clear eGBR was observed.
Figure 2. Hemodynamic and electrophysiological activations for Condition 1 (top) and Condition 2 (bottom). The left column shows hemodynamic activation pattern for the comparison for each condition with the resting baseline. Results are superimposed onto horizontal sections (z = 2, z = 10) of the standard MNI template. Data are plotted in neurological convention. The right column depicts time–frequency representations of oscillatory activity. Plots show evoked activity averaged across all participants. Data have been obtained by averaging time–frequency planes from the posterior ROI. Stimulus onset is at 0 ms. Amplitudes are in μV relative to a baseline from 200 up to 100 ms before stimulus onset (not shown).
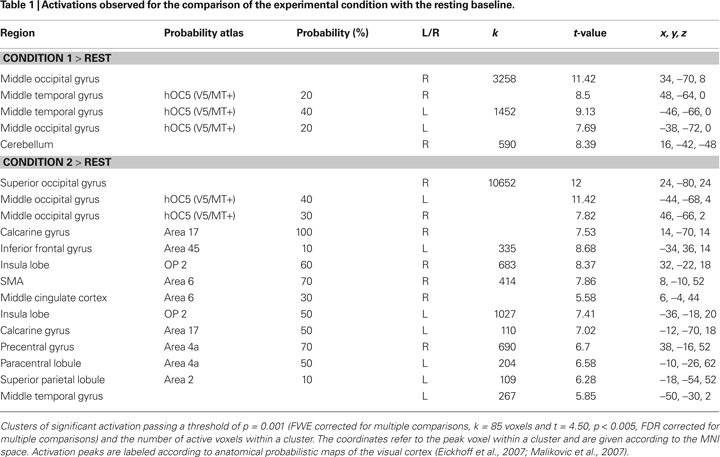
While the focus of the present study is eGBR, we also analyzed lower frequency activity in order to provide a means of comparison to other studies. Figure
3
shows the evoked (phase-locked) and induced activity in a 1- to 40 Hz frequency range for Condition 2. The figure shows an early amplitude increase (event related synchronization, ERS) as well as a later decrease (event related desynchronization, ERD) in the alpha/beta range as would be expected in a visual task.
Figure 3. Time–frequency representations of evoked and induced oscillatory activity for Condition 2 averaged across all participants. Stimulus onset is at 0 ms. Amplitudes are in μV relative to a baseline from 200 up to 100 ms before stimulus onset (not shown).
Figure
4
depicts results for the comparison of Condition 1 and Condition 2 (cf. Table
2
). As shown in the left panel of Figure
4
, the direct comparison of experimental conditions revealed stronger hemodynamic responses of the bilateral area V5/MT for Condition 1. In contrast, the bilateral V1/V2 complex showed significantly stronger hemodynamic responses during Condition 2. The precentral gyri and the Heschl’s gyri displayed bilateral enhanced responses during Condition 2. The right panel of Figure
4
shows the corresponding electrophysiological data. Mean eGBR in Condition 2 was significantly stronger than in Condition 1 (
t = −4.48,
p < 0.001). Thus, within-subject effects analysis demonstrated that intra-individually, the amplitude of the eGBR correlated with hemodynamic response in the visual domain. Visual stimuli that evoked strong GBRs also elicited strong BOLD responses in the human primary visual cortex, and less BOLD in the bilateral area MT.
Figure 4. Differential hemodynamic and electrophysiological activations. Activations observed for the direct comparison of conditions. The left column shows results of the hemodynamic data. Cortical areas showing stronger responses during Condition 1 are color-coded at a blue-petrol scale, brain regions showing stronger responses during Condition 2 are color-coded at a red–yellow scale. Activations are overlaid onto horizontal sections (z = 2, z = 10) of the standard MNI template. The right column shows averaged evoked gamma-band responses for Condition 1 (blue) and Condition 2 (red). Evoked GBR time courses were determined from frequency range from 45 to 55 Hz and were taken from the posterior ROI.
Between-Subject Effects
Figure
5 and Table
3
summarize the results of the correlation analysis of evoked gamma-band and BOLD responses during Condition 2. The analysis demonstrated a significant positive correlation between individual eGBRs and hemodynamic responses to visual standard stimuli in the V1/V2 complex and area V5/MT bilaterally. Furthermore, hemodynamic responses were significantly positively correlated with eGBRs in the cerebellum and right hippocampal formation. Significant negative correlations could be observed in the supplementary motor area and left middle and superior frontal region.
Figure 5. Activation pattern for the correlation analysis between individual hemodynamic and electrophysiological data for Condition 2. Results for the correlation of individual eGBR and BOLD are superimposed onto horizontal sections (z = 6, z = 10) of a standard anatomical template. Data are plotted in neurological convention. Plots illustrate the correlations of the BOLD responses and the individual eGBR in four regions of interest (ROI) in the visual cortex : left A17, right A17, left V5/MT, and right V5/MT. Graphs show the individual hemodynamic signal increases (BOLD) plotted against the individual eGBR together with the related regression line, R2- and p-value.
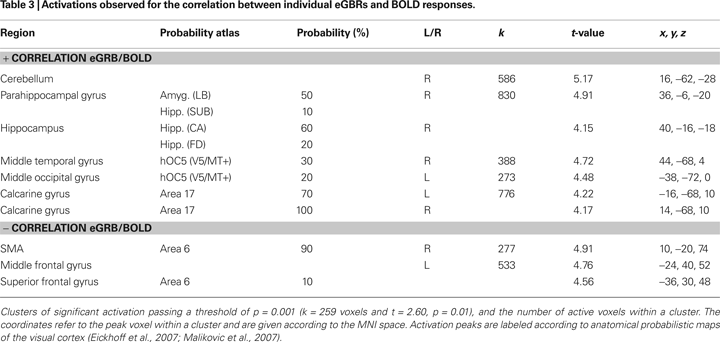
Graphs in Figure
5
show results of the ROI analysis. The BOLD responses in visual cortex areas (left A17, right A17, left V5/MT, and right V5/MT) correlated with the individual eGBRs. Post-hoc test confirmed significant positive correlation of the individual eGBR and BOLD response in all selected areas (left A17:
r = 0.67,
p < 0.01; right A17:
r = 0.6,
p < 0.01; left V5/MT:
r = 0.64,
p < 0.01; right V5/MT:
r = 0.74,
p < 0.001). Thus, between-subject effects analysis showed that inter-individually, the participants’ gamma-trait and BOLD responses in the visual cortex positively correlated. Participants with strong eGBR during a visual target detection task also showed strong BOLD responses in the primary (Area 17) and secondary (Area MT) visual cortex. The iGBR explained 35–55% of the inter-individual variance in the BOLD data of the visual areas. Additionally, the individual eGBRs also positively correlated with intra-individual variations of the BOLD responses in the right hippocampal formation. In order to additionally verify homogeneity within the sample of participants and to exclude an effect of aging on the inter-individual hemodynamic and oscillatory brain responses, brain activation data were correlated with the subjects age. Results showed neither significant correlation of eGBR and the subjects age (
r = −0.18,
p = 0.5), nor of BOLD and age (left A17:
r = −0.13,
p = 0.6; right A17:
r = −0.11,
p = 0.7; left V5/MT:
r = 0.03,
p = 0.9; right V5/MT:
r = −0.26,
p = 0.3).
To further evaluate the impact of positive eGBR values only, an additional analysis was performed. The eGBR-BOLD correlation on the ROI data for those subjects which exhibited a positive GBR value (N = 8) confirmed significant correlations for the left and right A17 (left A17, r = 0.73, p < 0.05; right A17, r = 0.74, p < 0.05). The analysis for the left and right V5/MT area did not reach statistical significance (left V5/MT, r = 0.43; right V5/MT, r = 0.2). However, given the limitations in detecting a significant correlation due to the strong reduction in sample size, it is difficult to draw conclusions from this discrepancy. We expect that with more data, a correlation would emerge with the positive data points in area MT as well. However, an alternative explanation might be that positive GBR values could be associated with different hemodynamic responses than negative GBR values, or that the BOLD response saturates at some point, resulting in a flattening of the curve at higher GBR amplitudes.
Figure
6
illustrates individual data of two representative participants for Condition 2. The left column shows data for a participant with a high amplitude of eGBR, the right column shows data for a participant with a low eGBR amplitude. Both participants showed an early eGBR in the time–frequency representation and exhibited bilateral hemodynamic activations in the visual areas V1/V2 and V5/MT. The LORETA source localization of the eGBRs revealed activations over the occipital cortex covering areas V1/V2 and V5/MT.
Figure 6. Two representative participants (high eGBR subject left and low eGBR subject right) for Condition 2. (A) Time–frequency representations of oscillatory activity. Plots show individual evoked activity for Condition 2. Stimulus onset is at 0 ms. Amplitudes are in μV relative to a baseline 200 up to 100 ms before stimulus onset (not shown). (B) Individual hemodynamic activation pattern for the comparison of Condition 2 with the resting baseline. Data are plotted in neurological convention and superimposed onto horizontal sections (z = 1, z = 8) of the individual anatomical reference image. (C) Low-resolution brain electromagnetic tomography (LORETA) solutions modeling the cortical sources for the individual gamma-band activity.
We performed an identical fMRI/EEG experiment in 17 participants to investigate the content of gamma-related hemodynamic changes in the human cerebral cortex. We observed that eGBRs covaried intra- as well as inter-individually with BOLD responses in the visual cortex. In general, our results are in line with former investigations showing a close spatial correspondence between electrophysiological responses in the gamma range and hemodynamic activations of the visual cortex. Neural oscillations in the gamma-band in response to visual stimulation have been linked to neural processing of the primary and secondary visual cortex (
Cardin et al., 2005
;
Gray and McCormick, 1996
;
Lachaux et al., 2000
,
2005
).
By comparing the processing of visual stimuli differentially evoking gamma-band activity in a within-subject approach, we demonstrate strong correspondence of BOLD and GBRs in the human primary visual cortex and the area MT. Visual stimuli evoking strong GBRs also evoke strong BOLD responses in the primary visual cortex. The observation of eGBR–BOLD relation is consistent with recent research in animals and humans demonstrating a close spatial and temporal correspondence between BOLD signal and LFP oscillations in the gamma range (
Mukamel et al., 2005
;
Niessing et al., 2005
) as well as high spatial correspondence between BOLD signal and GBRs using MEG (
Brookes et al., 2005
;
Hoogenboom et al., 2006
;
Muthukumaraswamy and Singh, 2008
).
Analysis of the stimulus effect also revealed that stimuli evoking weaker GBRs resulted in stronger BOLD responses in area MT. This sounds counter-intuitive at first glance. However, positive BOLD responses do not exclusively correspond to cortical synchronization. For the alpha and beta range hemodynamic activity has been associated with desynchronization of the oscillatory activity (
Singh et al., 2002
;
Winterer et al., 2007
). Thus, the effect of eGBRs and BOLD responses in area MT further suggests that the relation between BOLD and electrical brain activity might be more complex.
By investigating the between-subject effects, our data show that variations in the individual eGBRs to visual stimuli are accompanied by variations in the hemodynamic activity in the bilateral primary (Area 17) and secondary (Area V5/MT) visual cortex and the right hippocampal formation. This analysis of inter-individual variation of brain responses was partly motivated by the frequent observations that participants display a considerable amount of inter-individual variation in their BOLD responses (e.g.
Costafreda et al., 2007
;
Handwerker et al., 2004
;
Miller et al., 2002
), as well as highly inter-individually variable eGBRs during a variety of tasks (
Frund et al., 2007c
).
Factors that can influence the BOLD response are diverse. They include individual differences in size and location of veins (
Cohen et al., 2004
), extracranial arterial diseases (
Hamzei et al., 2003
), pulse or respiration differences (
Dagli et al., 1999
), hematocrit concentration (
Levin et al., 2001
) and baseline cerebral blood flow (
Cohen et al., 2002
). Also food intake, medication, alcohol, and caffeine consumption can affect the BOLD signal (
Levin et al., 1998
;
Mulderink et al., 2002
;
Noseworthy et al., 2003
). On the other hand, only few studies investigated individual parameters of inter-individual eGBR variations. It has been shown that alcoholism modulates the eGBR in visual oddball tasks (
Padmanabhapillai et al., 2006
). Generally, inter-individual differences in both measurements – the oscillatory EEG and the BOLD data – could emerge through differences in the functional architecture of the cortex as described in the cat visual system (
Kaschube et al., 2002
).
Also, behavioral factors have been linked to altered electrophysiological and hemodynamic responses. The evoked GBR as well as the BOLD signal are modulated by the reaction time of the subject in response to a given stimulus (
Frund et al., 2007b
;
Yarkoni et al., 2009
). Due to the experimental design used in the present study, we are not able to perfectly control for this factor. However, accuracy (detection of the target stimuli) of all participants was consistently high during both experimental sessions. Furthermore, only hemodynamic as well as electrophysiological activity in response to standard stimuli were analyzed. These standard stimuli did not require a behavioral response.
Aging constitutes a further considerable factor influencing both the strength of the BOLD response (
D’Esposito et al., 2003
), as well as the amplitude of the evoked GBR (
Bottger et al., 2002
). However, this factor can largely be excluded since we kept the age of the sample homogeneous and additionally, statistically demonstrated that aging was neither influencing the electrophysiological nor the hemodynamic data.
Genetic polymorphisms of neurotransmission components form a further mechanism that has been identified to underlie variable brain responses. Multiple gene variants have an impact on hemodynamic responses in several discrete brain circuits during special forms of information processing (
Goldberg and Weinberger, 2004
;
Hariri and Weinberger, 2003
). The impact of a series of polymorphisms on evoked potentials has also been described. Specific cognitive processes and their underlying neurophysiological activity have been associated with common gene variations. Variations of the nicotinic receptor system seem to modulate early attention processes like sensory gating and variations of the P50 component of the ERP (
Freedman et al., 2000
;
Leonard et al., 2002
). Similarly, alterations of the brain-derived neurotrophic factor functioning have been linked to variations in memory functions (
Wilson et al., 1996
) and BOLD signal modulations during visual scene encoding and retrieval in the hippocampal formation (
Hariri et al., 2003
). Variation of the dopamine type-4 receptor gene has an impact on attentional processing and its underlying hemodynamic responses (
Fan et al., 2003
) as well as on the eGBRs (
Demiralp et al., 2007
). A analogous effect on eGBRs has been shown for a polymorphism of the gene encoding the dopamine transporter (
Demiralp et al., 2007
). Similarly, polymorphisms of the catechol-O-methyltransferase have been associated with altered BOLD responses in the prefrontal cortex during memory tasks (
Egan et al., 2001
) and reduced P300 component of the ERP over frontal regions (
Gallinat et al., 2003
). The present results indicate that the inter-individual variation in eGBRs and BOLD responses are mirroring similar inter-individual differences in the underlying neural processing. This variability might, at least partly, be caused by common genetic variations in the neurotransmitter system of the participants.
In the present study, we also revealed significant correlation of hemodynamic responses in the hippocampal formation with the individual eGBR. This might come as a surprise at first sight. However, the two main cortical areas discussed in the context of cerebral gamma oscillations are the primary sensory cortices and the hippocampus, analogous to the two main functions of gamma oscillation in humans: sensory perception and memory processing.
Using scalp EEG, it is not possible to directly assess electrophysiological responses from the medial temporal cortex. Thus, the observed inter-individual relation must be indirect and might be caused by a common underlying mechanism modifying both, GBR and BOLD response. Generally, gamma oscillations are prevalent in the hippocampal formation, where they have been proposed to assist in encoding and retrieval of memory traces (
Bragin et al., 1995
;
Chrobak and Buzsaki, 1998
;
Lisman and Idiart, 1995
;
Sederberg et al., 2007
). Analogously, increased BOLD activity in the hippocampus has been associated with different components of the memory process (
Eldridge et al., 2005
;
Gold et al., 2006
). In the context of a target detection task, the hippocampus might be involved in the detection of deviant stimuli by maintaining a template of previous stimuli for comparison with incoming sensory stimuli (
Knight and Nakada, 1998
).
A recent hypothesis assumes that the early gamma-band activity occurring in EEG and MEG measurements before 150 ms after stimulus presentation reflects a match of the sensory information with the memory content (“match-and-utilization model”). The hypothesis proposes that such matching operations are carried out in primary cortices (
Herrmann et al., 2004b
). One might speculate that the observed eGBR–BOLD relation reflects a coactivity of the hippocampus and the sensory visual cortices. The hippocampus might maintain a stimulus template for deviant detection that elicits BOLD responses, whereas the visual cortex performs the match with the incoming sensory information evoking a GBR. Participants with strong eGBRs in the visual cortex also have strong BOLD responses in the hippocampus. Unfortunately, the direction of this interaction remains unresolved. In addition, our suggestion is speculative and the observed effect is currently difficult to explain and needs to be further addressed in the future.
Taken together, the present study demonstrated covariance of individual eGBRs and BOLD responses in the human visual system. This effect might be related to inter-individual variations in the underlying cortical morphology and individual genotype. The majority of former studies utilized various approaches to localize the GBR and related this anatomical origin to the BOLD measurements. In the present study, we used a correlation approach that identified within- and between-subject BOLD–eGBR correspondence and thus provides further support for the idea that gamma frequency oscillations are involved in the cascade of neural processes underlying the hemodynamic responses measured with fMRI.
Conflict of Interest Statement
The authors declare that the research was conducted in the absence of any commercial or financial relationships that could be construed as a potential conflict of interest.
This research was supported by the state of Saxony – Anhalt (Grant XN 36101A/0705 M) and the BMBF (Center for Advanced Imaging and Bernstein Group for Computational Neuroscience).
Axmacher, N., Mormann, F., Fernandez, G., Elger, C. E., and Fell, J. (2006). Memory formation by neuronal synchronization.
Brain Res. Rev. 52, 170–182.
Basar, E. (1980). EEG-Brain Dynamics Relation Between EEG and Brain Evoked Potentials. Amsterdam, Elsevier.
Basar-Eroglu, C., Struber, D., Schurmann, M., Stadler, M., and Basar, E. (1996). Gamma-band responses in the brain: a short review of psychophysiological correlates and functional significance.
Int. J. Psychophysiol. 24, 101–112.
Berger, H. (1929). über das Elektrenkephalogramm des Menschen.
Arch. Psychiatr. Nervenkr. 87, 527–570.
Bottger, D., Herrmann, C. S., and von Cramon, D. Y. (2002). Amplitude differences of evoked alpha and gamma oscillations in two different age groups.
Int. J. Psychophysiol. 45, 245–251.
Bragin, A., Jando, G., Nadasdy, Z., Hetke, J., Wise, K., and Buzsaki, G. (1995). Gamma (40–100 Hz) oscillation in the hippocampus of the behaving rat.
J. Neurosci. 15, 47–60.
Brookes, M. J., Gibson, A. M., Hall, S. D., Furlong, P. L., Barnes, G. R., Hillebrand, A., Singh, K. D., Holliday, I. E., Francis, S. T., and Morris, P. G. (2005). GLM-beamformer method demonstrates stationary field, alpha ERD and gamma ERS co-localisation with fMRI BOLD response in visual cortex.
Neuroimage 26, 302–308.
Brovelli, A., Lachaux, J. P., Kahane, P., and Boussaoud, D. (2005). High gamma frequency oscillatory activity dissociates attention from intention in the human premotor cortex.
Neuroimage 28, 154–164.
Busch, N. A., Debener, S., Kranczioch, C., Engel, A. K., and Herrmann, C. S. (2004). Size matters: effects of stimulus size, duration and eccentricity on the visual gamma-band response.
Clin. Neurophysiol. 115, 1810–1820.
Busch, N. A., Schadow, J., Frund, I., and Herrmann, C. S. (2006). Time–frequency analysis of target detection reveals an early interface between bottom-up and top-down processes in the gamma-band.
Neuroimage 29, 1106–1116.
Canolty, R. T., Edwards, E., Dalal, S. S., Soltani, M., Nagarajan, S. S., Kirsch, H. E., Berger, M. S., Barbaro, N. M., and Knight, R. T. (2006). High gamma power is phase-locked to theta oscillations in human neocortex.
Science 313, 1626–1628.
Cardin, J. A., Palmer, L. A., and Contreras, D. (2005). Stimulus-dependent gamma (30–50 Hz) oscillations in simple and complex fast rhythmic bursting cells in primary visual cortex.
J. Neurosci. 25, 5339–5350.
Chrobak, J. J., and Buzsaki, G. (1998). Gamma oscillations in the entorhinal cortex of the freely behaving rat.
J. Neurosci. 18, 388–398.
Cohen, E. R., Rostrup, E., Sidaros, K., Lund, T. E., Paulson, O. B., Ugurbil, K., and Kim, S. G. (2004). Hypercapnic normalization of BOLD fMRI: comparison across field strengths and pulse sequences.
Neuroimage 23, 613–624.
Cohen, E. R., Ugurbil, K., and Kim, S. G. (2002). Effect of basal conditions on the magnitude and dynamics of the blood oxygenation level-dependent fMRI response.
J. Cereb. Blood Flow Metab. 22, 1042–1053.
Costafreda, S. G., Brammer, M. J., Vencio, R. Z., Mourao, M. L., Portela, L. A., de Castro, C. C., Giampietro, V. P., and Amaro, E. Jr (2007). Multisite fMRI reproducibility of a motor task using identical MR systems.
J. Magn. Reson. Imaging 26, 1122–1126.
D’Esposito, M., Deouell, L. Y., and Gazzaley, A. (2003). Alterations in the BOLD fMRI signal with ageing and disease: a challenge for neuroimaging.
Nat. Rev. Neurosci. 4, 863–872.
Dagli, M. S., Ingeholm, J. E., and Haxby, J. V. (1999). Localization of cardiac-induced signal change in fMRI.
Neuroimage 9, 407–415.
De Pascalis, V., Marucci, F. S., Penna, P. M., and Pessa, E. (1987). Hemispheric activity of 40 Hz EEG during recall of emotional events: differences between low and high hypnotizables.
Int. J. Psychophysiol. 5, 167–180.
Debener, S., Herrmann, C. S., Kranczioch, C., Gembris, D., and Engel, A. K. (2003). Top-down attentional processing enhances auditory evoked gamma band activity.
Neuroreport 14, 683–686.
Demiralp, T., Basar-Eroglu, C., and Basar, E. (1996). Distributed gamma band responses in the brain studied in cortex, reticular formation, hippocampus and cerebellum.
Int. J. Neurosci. 84, 1–13.
Demiralp, T., Herrmann, C. S., Erdal, M. E., Ergenoglu, T., Keskin, Y. H., Ergen, M., and Beydagi, H. (2007). DRD4 and DAT1 polymorphisms modulate human gamma band responses.
Cereb. Cortex 17, 1007–1019.
Edwards, E., Soltani, M., Deouell, L. Y., Berger, M. S., and Knight, R. T. (2005). High gamma activity in response to deviant auditory stimuli recorded directly from human cortex.
J. Neurophysiol. 94, 4269–4280.
Egan, M. F., Goldberg, T. E., Kolachana, B. S., Callicott, J. H., Mazzanti, C. M., Straub, R. E., Goldman, D., and Weinberger, D. R. (2001). Effect of COMT Val108/158 Met genotype on frontal lobe function and risk for schizophrenia.
Proc. Natl. Acad. Sci. U. S. A. 98, 6917–6922.
Eickhoff, S. B., Paus, T., Caspers, S., Grosbras, M. H., Evans, A. C., Zilles, K., and Amunts, K. (2007). Assignment of functional activations to probabilistic cytoarchitectonic areas revisited.
Neuroimage 36, 511–521.
Eldridge, L. L., Engel, S. A., Zeineh, M. M., Bookheimer, S. Y., and Knowlton, B. J. (2005). A dissociation of encoding and retrieval processes in the human hippocampus.
J. Neurosci. 25, 3280–3286.
Engel, A. K., Fries, P., and Singer, W. (2001). Dynamic predictions: oscillations and synchrony in top-down processing.
Nat. Rev. Neurosci. 2, 704–716.
Fan, J., Fossella, J., Sommer, T., Wu, Y., and Posner, M. I. (2003). Mapping the genetic variation of executive attention onto brain activity.
Proc. Natl. Acad. Sci. U. S. A. 100, 7406–7411.
Fell, J., Fernandez, G., Klaver, P., Elger, C. E., and Fries, P. (2003). Is synchronized neuronal gamma activity relevant for selective attention?
Brain Res. Brain Res. Rev. 42, 265–272.
Fiebach, C. J., Gruber, T., and Supp, G. G. (2005). Neuronal mechanisms of repetition priming in occipitotemporal cortex: spatiotemporal evidence from functional magnetic resonance imaging and electroencephalography.
J. Neurosci. 25, 3414–3422.
Foucher, J. R., Otzenberger, H., and Gounot, D. (2003). The BOLD response and the gamma oscillations respond differently than evoked potentials: an interleaved EEG-fMRI study.
BMC Neurosci. 4, 22.
Freedman, R., Adams, C. E., Adler, L. E., Bickford, P. C., Gault, J., Harris, J. G., Nagamoto, H. T., Olincy, A., Ross, R. G., Stevens, K. E., Waldo, M., and Leonard, S. (2000). Inhibitory neurophysiological deficit as a phenotype for genetic investigation of schizophrenia.
Am. J. Med. Genet. 97, 58–64.
Fries, P., Neuenschwander, S., Engel, A. K., Goebel, R., and Singer, W. (2001a). Rapid feature selective neuronal synchronization through correlated latency shifting.
Nat. Neurosci. 4, 194–200.
Fries, P., Reynolds, J. H., Rorie, A. E., and Desimone, R. (2001b). Modulation of oscillatory neuronal synchronization by selective visual attention.
Science 291, 1560–1563.
Friston, K. J., Holmes, A. P., Worsley, K. P., Poline, J. B., Frith, C. D., and Frackowiak, R. S. (1995). Statistical parameter maps in functional imaging: a general linear approach.
Hum. Brain Mapp. 2, 189–210.
Frund, I., Busch, N. A., Korner, U., Schadow, J., and Herrmann, C. S. (2007a). EEG oscillations in the gamma and alpha range respond differently to spatial frequency.
Vision Res. 47, 2086–2098.
Frund, I., Busch, N. A., Schadow, J., Korner, U., and Herrmann, C. S. (2007b). From perception to action: phase-locked gamma oscillations correlate with reaction times in a speeded response task.
BMC Neurosci. 8, 27.
Frund, I., Schadow, J., Busch, N. A., Korner, U., and Herrmann, C. S. (2007c). Evoked gamma oscillations in human scalp EEG are test-retest reliable.
Clin. Neurophysiol. 118, 221–227.
Gallinat, J., Bajbouj, M., Sander, T., Schlattmann, P., Xu, K., Ferro, E. F., Goldman, D., and Winterer, G. (2003). Association of the G1947A COMT (Val(108/158)Met) gene polymorphism with prefrontal P300 during information processing.
Biol. Psychiatry 54, 40–48.
Gold, J. J., Smith, C. N., Bayley, P. J., Shrager, Y., Brewer, J. B., Stark, C. E., Hopkins, R. O., and Squire, L. R. (2006). Item memory, source memory, and the medial temporal lobe: concordant findings from fMRI and memory-impaired patients.
Proc. Natl. Acad. Sci. U. S. A. 103, 9351–9356.
Goldberg, T. E., and Weinberger, D. R. (2004). Genes and the parsing of cognitive processes.
Trends Cogn. Sci. 8, 325–335.
Gray, C. M., and McCormick, D. A. (1996). Chattering cells: superficial pyramidal neurons contributing to the generation of synchronous oscillations in the visual cortex.
Science 274, 109–113.
Gruber, T., Keil, A., and Muller, M. M. (2001). Modulation of induced gamma band responses and phase synchrony in a paired associate learning task in the human EEG.
Neurosci. Lett. 316, 29–32.
Gruber, T., and Muller, M. M. (2005). Oscillatory brain activity dissociates between associative stimulus content in a repetition priming task in the human EEG.
Cereb. Cortex 15, 109–116.
Gruber, T., and Muller, M. M. (2006). Oscillatory brain activity in the human EEG during indirect and direct memory tasks.
Brain Res. 1097, 194–204.
Gruber, T., Tsivilis, D., Montaldi, D., and Muller, M. M. (2004). Induced gamma band responses: an early marker of memory encoding and retrieval.
Neuroreport 15, 1837–1841.
Hall, S. D., Holliday, I. E., Hillebrand, A., Singh, K. D., Furlong, P. L., Hadjipapas, A., and Barnes, G. R. (2005). The missing link: analogous human and primate cortical gamma oscillations.
Neuroimage 26, 13–17.
Hamzei, F., Knab, R., Weiller, C., and Rother, J. (2003). The influence of extra- and intracranial artery disease on the BOLD signal in FMRI.
Neuroimage 20, 1393–1399.
Handwerker, D. A., Ollinger, J. M., and D’Esposito, M. (2004). Variation of BOLD hemodynamic responses across subjects and brain regions and their effects on statistical analyses.
Neuroimage 21, 1639–1651.
Hariri, A. R., Goldberg, T. E., Mattay, V. S., Kolachana, B. S., Callicott, J. H., Egan, M. F., and Weinberger, D. R. (2003). Brain-derived neurotrophic factor val66met polymorphism affects human memory-related hippocampal activity and predicts memory performance.
J. Neurosci. 23, 6690–6694.
Hariri, A. R., and Weinberger, D. R. (2003). Imaging genomics.
Br. Med. Bull. 65, 259–270.
Herrmann, C. S., and Debener, S. (2008). Simultaneous recording of EEG and BOLD responses: a historical perspective.
Int. J. Psychophysiol. 67, 161–168.
Herrmann, C. S., and Demiralp, T. (2005). Human EEG gamma oscillations in neuropsychiatric disorders.
Clin. Neurophysiol. 116, 2719–2733.
Herrmann, C. S., Lenz, D., Junge, S., Busch, N. A., and Maess, B. (2004a) Memory-matches evoke human gamma-responses.
BMC Neurosci. 5, 13.
Herrmann, C. S., Munk, M. H., and Engel, A. K. (2004b). Cognitive functions of gamma-band activity: memory match and utilization.
Trends Cogn. Sci. 8, 347–355.
Herrmann, C. S., and Mecklinger, A. (2000). Magnetoencephalographic responses to illusory figures: early evoked gamma is affected by processing of stimulus features.
Int. J. Psychophysiol. 38, 265–281.
Hoogenboom, N., Schoffelen, J. M., Oostenveld, R., Parkes, L. M., and Fries, P. (2006). Localizing human visual gamma-band activity in frequency, time and space.
Neuroimage 29, 764–773.
Howard, M. W., Rizzuto, D. S., Caplan, J. B., Madsen, J. R., Lisman, J., Aschenbrenner-Scheibe, R., Schulze-Bonhage, A., and Kahana, M. J. (2003). Gamma oscillations correlate with working memory load in humans.
Cereb. Cortex 13, 1369–1374.
Jokeit, H., and Makeig, S. (1994). Different event-related patterns of gamma-band power in brain waves of fast- and slow-reacting subjects.
Proc. Natl. Acad. Sci. U. S. A. 91, 6339–6343.
Karns, C. M., and Knight, R. T. (2009). Intermodal auditory, visual, and tactile attention modulates early stages of neural processing.
J. Cogn. Neurosci. 21, 669–683.
Kaschube, M., Wolf, F., Geisel, T., and Lowel, S. (2002). Genetic influence on quantitative features of neocortical architecture.
J. Neurosci. 22, 7206–7217.
Keil, A., Muller, M. M., Gruber, T., Wienbruch, C., and Elbert, T. (2001). Human large-scale oscillatory brain activity during an operant shaping procedure.
Brain Res. Cogn. Brain Res. 12, 397–407.
Keil, A., Muller, M. M., Ray, W. J., Gruber, T., and Elbert, T. (1999). Human gamma band activity and perception of a gestalt.
J. Neurosci. 19, 7152–7161.
Knight, R. T., and Nakada, T. (1998). Cortico-limbic circuits and novelty: a review of EEG and blood flow data.
Rev. Neurosci. 9, 57–70.
Lachaux, J. P., Fonlupt, P., Kahane, P., Minotti, L., Hoffmann, D., Bertrand, O., and Baciu, M. (2007). Relationship between task-related gamma oscillations and BOLD signal: new insights from combined fMRI and intracranial EEG.
Hum. Brain Mapp. 28, 1368–1375.
Lachaux, J. P., George, N., Tallon-Baudry, C., Martinerie, J., Hugueville, L., Minotti, L., Kahane, P., and Renault, B. (2005). The many faces of the gamma band response to complex visual stimuli.
Neuroimage 25, 491–501.
Lachaux, J. P., Rodriguez, E., Martinerie, J., Adam, C., Hasboun, D., and Varela, F. J. (2000). A quantitative study of gamma-band activity in human intracranial recordings triggered by visual stimuli.
Eur. J. Neurosci. 12, 2608–2622.
Leonard, S., Gault, J., Hopkins, J., Logel, J., Vianzon, R., Short, M., Drebing, C., Berger, R., Venn, D., Sirota, P., Zerbe, G., Olincy, A., Ross, R. G., Adler, L. E., and Freedman, R. (2002). Association of promoter variants in the alpha7 nicotinic acetylcholine receptor subunit gene with an inhibitory deficit found in schizophrenia.
Arch. Gen. Psychiatry 59, 1085–1096.
Levin, J. M., Frederick, B. B., Ross, M. H., Fox, J. F., von Rosenberg, H. L., Kaufman, M. J., Lange, N., Mendelson, J. H., Cohen, B. M., and Renshaw, P. F. (2001). Influence of baseline hematocrit and hemodilution on BOLD fMRI activation.
Magn. Reson. Imaging 19, 1055–1062.
Levin, J. M., Ross, M. H., Mendelson, J. H., Kaufman, M. J., Lange, N., Maas, L. C., Mello, N. K., Cohen, B. M., and Renshaw, P. F. (1998). Reduction in BOLD fMRI response to primary visual stimulation following alcohol ingestion.
Psychiatry Res. 82, 135–146.
Lisman, J. E., and Idiart, M. A. (1995). Storage of 7+/− 2 short-term memories in oscillatory subcycles.
Science 267, 1512–1515.
Logothetis, N. K. (2002). The neural basis of the blood-oxygen-level-dependent functional magnetic resonance imaging signal.
Philos. Trans. R. Soc. Lond. B Biol. Sci. 357, 1003–1037.
Logothetis, N. K. (2003). The underpinnings of the BOLD functional magnetic resonance imaging signal.
J. Neurosci. 23, 3963–3971.
Logothetis, N. K., Pauls, J., Augath, M., Trinath, T., and Oeltermann, A. (2001). Neurophysiological investigation of the basis of the fMRI signal.
Nature 412, 150–157.
Malikovic, A., Amunts, K., Schleicher, A., Mohlberg, H., Eickhoff, S. B., Wilms, M., Palomero-Gallagher, N., Armstrong, E., and Zilles, K. (2007). Cytoarchitectonic analysis of the human extrastriate cortex in the region of V5/MT+: a probabilistic, stereotaxic map of area hOc5.
Cereb. Cortex 17, 562–574.
Miller, M. B., Van Horn, J. D., Wolford, G. L., Handy, T. C., Valsangkar-Smyth, M., Inati, S., Grafton, S., and Gazzaniga, M. S. (2002). Extensive individual differences in brain activations associated with episodic retrieval are reliable over time.
J. Cogn. Neurosci. 14, 1200–1214.
Mukamel, R., Gelbard, H., Arieli, A., Hasson, U., Fried, I., and Malach, R. (2005). Coupling between neuronal firing, field potentials, and FMRI in human auditory cortex.
Science 309, 951–954.
Mulderink, T. A., Gitelman, D. R., Mesulam, M. M., and Parrish, T. B. (2002). On the use of caffeine as a contrast booster for BOLD fMRI studies.
Neuroimage 15, 37–44.
Muller, M. M., Teder, W., and Hillyard, S. A. (1997). Magnetoencephalographic recording of steady-state visual evoked cortical activity.
Brain Topogr. 9, 163–168.
Muthukumaraswamy, S. D., and Singh, K. D. (2008). Spatiotemporal frequency tuning of BOLD and gamma band MEG responses compared in primary visual cortex.
Neuroimage 40, 1552–1560.
Niessing, J., Ebisch, B., Schmidt, K. E., Niessing, M., Singer, W., and Galuske, R. A. (2005). Hemodynamic signals correlate tightly with synchronized gamma oscillations.
Science 309, 948–951.
Noseworthy, M. D., Alfonsi, J., and Bells, S. (2003). Attenuation of brain BOLD response following lipid ingestion.
Hum. Brain Mapp. 20, 116–121.
Ohla, K., Busch, N. A., and Herrmann, C. S. (2007). Early electrophysiological markers of visual awareness in the human brain.
Neuroimage 37, 1329–1337.
Padmanabhapillai, A., Porjesz, B., Ranganathan, M., Jones, K. A., Chorlian, D. B., Tang, Y., Kamarajan, C., Rangaswamy, M., Stimus, A., and Begleiter, H. (2006). Suppression of early evoked gamma band response in male alcoholics during a visual oddball task.
Int. J. Psychophysiol. 60, 15–26.
Pascual-Marqui, R. D., Esslen, M., Kochi, K., and Lehmann, D. (2002). Functional imaging with low-resolution brain electromagnetic tomography (LORETA): a review.
Methods Find. Exp. Clin. Pharmacol. 24(Suppl. C), 91–95.
Pope, K. J., Fitzgibbon, S. P., Lewis, T. W., Whitham, E. M., and Willoughby, J. O. (2009). Relation of gamma oscillations in scalp recordings to muscular activity.
Brain Topogr. doi: 10.1007/s10548-009-0081-x. [Epub ahead of print].
Schadow, J., Lenz, D., Thaerig, S., Busch, N. A., Frund, I., Rieger, J. W., and Herrmann, C. S. (2007). Stimulus intensity affects early sensory processing: visual contrast modulates evoked gamma-band activity in human EEG.
Int. J. Psychophysiol. 66, 28–36.
Sederberg, P. B., Kahana, M. J., Howard, M. W., Donner, E. J., and Madsen, J. R. (2003). Theta and gamma oscillations during encoding predict subsequent recall.
J. Neurosci. 23, 10809–10814.
Sederberg, P. B., Schulze-Bonhage, A., Madsen, J. R., Bromfield, E. B., Litt, B., Brandt, A., and Kahana, M. J. (2007). Gamma oscillations distinguish true from false memories.
Psychol. Sci. 18, 927–932.
Singer, W., Engel, A. K., Kreiter, A. K., Munk, M. H. J., Neuenschwander, S., and Roelfsema, P. R. (1997). Neuronal assemblies: necessity, signature and detectability.
Trends Cogn. Sci. 1, 252–261.
Singer, W., and Gray, C. M. (1995). Visual feature integration and the temporal correlation hypothesis.
Annu. Rev. Neurosci. 18, 555–586.
Singh, K. D., Barnes, G. R., Hillebrand, A., Forde, E. M., and Williams, A. L. (2002). Task-related changes in cortical synchronization are spatially coincident with the hemodynamic response.
Neuroimage 16, 103–114.
Struber, D., Basar-Eroglu, C., Hoff, E., and Stadler, M. (2000). Reversal-rate dependent differences in the EEG gamma-band during multistable visual perception.
Int. J. Psychophysiol. 38, 243–252.
Talairach, J., and Tournoux, P. (1988). Co-palanar Stereotaxis Atlas of the Human Brain. New York, Thieme.
Tallon-Baudry, C., and Bertrand, O. (1999). Oscillatory gamma activity in humans and its role in object representation.
Trends Cogn. Sci. 3, 151–162.
Tallon-Baudry, C., Bertrand, O., Delpuech, C., and Pernier, J. (1996). Stimulus specificity of phase-locked and non-phase-locked 40 Hz visual responses in human.
J. Neurosci. 16, 4240–4249.
Tallon-Baudry, C., Bertrand, O., Delpuech, C., and Pernier, J. (1997). Oscillatory gamma-band (30–70 Hz) activity induced by a visual search task in humans.
J. Neurosci. 17, 722–734.
Tallon-Baudry, C., Bertrand, O., Henaff, M. A., Isnard, J., and Fischer, C. (2005). Attention modulates gamma-band oscillations differently in the human lateral occipital cortex and fusiform gyrus.
Cereb. Cortex 15, 654–662.
Tallon-Baudry, C., Bertrand, O., Peronnet, F., and Pernier, J. (1998). Induced gamma-band activity during the delay of a visual short-term memory task in humans.
J. Neurosci. 18, 4244–4254.
Tiitinen, H., Sinkkonen, J., Reinikainen, K., Alho, K., Lavikainen, J., and Naatanen, R. (1993). Selective attention enhances the auditory 40-Hz transient response in humans.
Nature 364, 59–60.
Wilson, R. S., Sullivan, M., Toledo-Morrell, L., Stebbins, G. T., Bennett, D. A., and Morrell, F. (1996). Association of Memory and cognition in Alzheimer’s disease with volumetric estimates of temporal lobe structures.
Neuropsychology 10, 459–463.
Winterer, G., Carver, F. W., Musso, F., Mattay, V., Weinberger, D. R., and Coppola, R. (2007). Complex relationship between BOLD signal and synchronization/desynchronization of human brain MEG oscillations.
Hum. Brain Mapp. 28, 805–816.
Yarkoni, T., Barch, D. M., Gray, J. R., Conturo, T. E., and Braver, T. S. (2009). BOLD correlates of trial-by-trial reaction time variability in gray and white matter: a multi-study fMRI analysis.
PLoS ONE 4, e4257.
Yuval-Greenberg, S., Tomer, O., Keren, A. S., Nelken, I., and Deouell, L. Y. (2008). Transient induced gamma-band response in EEG as a manifestation of miniature saccades.
Neuron 58, 429–441.