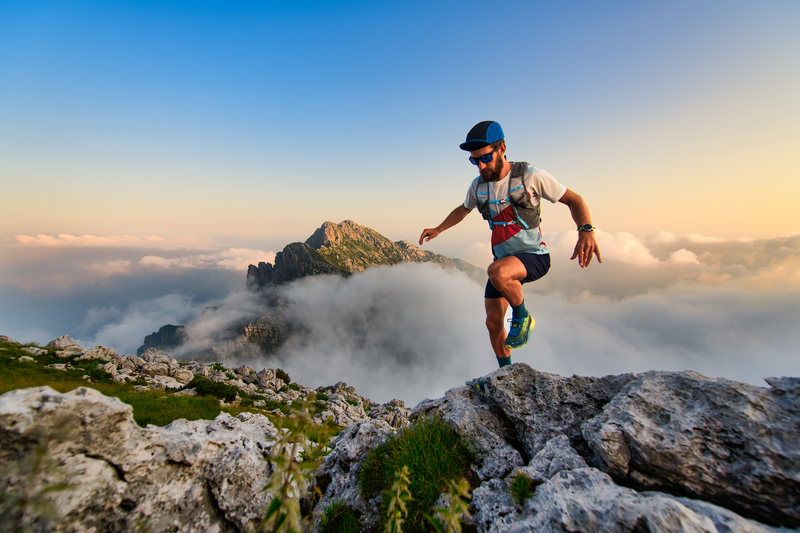
94% of researchers rate our articles as excellent or good
Learn more about the work of our research integrity team to safeguard the quality of each article we publish.
Find out more
ORIGINAL RESEARCH article
Front. Hum. Neurosci.
Sec. Brain-Computer Interfaces
Volume 19 - 2025 | doi: 10.3389/fnhum.2025.1551214
This article is part of the Research Topic Non invasive BCI for communication View all articles
The final, formatted version of the article will be published soon.
You have multiple emails registered with Frontiers:
Please enter your email address:
If you already have an account, please login
You don't have a Frontiers account ? You can register here
A significant challenge in developing reliable Brain-Computer Interfaces (BCIs) is the presence of artifacts in the acquired brain signals. These artifacts may lead to erroneous interpretations, poor fitting of models, and subsequent reduced online performance. Furthermore, BCIs in a home or hospital setting are more susceptible to environmental noise. Artifact handling procedures aim to reduce signal interference by filtering, reconstructing, and/or eliminating unwanted signal contaminants. While straightforward conceptually and largely undisputed as essential, suitable artifact handling application in BCI systems remains unsettled and may reduce performance in some cases. A potential confound that remains unexplored in the majority of BCI studies using these procedures is the lack of parity with online usage (e.g. online parity). This manuscript compares classification performance between frequently used offline digital filtering, using the whole dataset, and an online digital filtering approach where the segmented data epochs that would be used during closed-loop control are filtered instead. In a sample of healthy adults (n=30) enrolled in a BCI pilot study to integrate new communication interfaces, there were significant benefits to model performance when filtering with online parity. While online simulations indicated similar performance across conditions in this study, there appears to be no drawback to the approach with greater online parity.
Keywords: EEG, N200 and P300 potentials, Artifact handling, Signal proccessing, CBCI, BCI - Brain Computer Interface, Online Parity, Signal filtering
Received: 25 Dec 2024; Accepted: 18 Feb 2025.
Copyright: © 2025 Memmott, Klee, Smedemark-Margulies and Oken. This is an open-access article distributed under the terms of the Creative Commons Attribution License (CC BY). The use, distribution or reproduction in other forums is permitted, provided the original author(s) or licensor are credited and that the original publication in this journal is cited, in accordance with accepted academic practice. No use, distribution or reproduction is permitted which does not comply with these terms.
* Correspondence:
Tab Memmott, Oregon Health and Science University, Portland, United States
Disclaimer: All claims expressed in this article are solely those of the authors and do not necessarily represent those of their affiliated organizations, or those of the publisher, the editors and the reviewers. Any product that may be evaluated in this article or claim that may be made by its manufacturer is not guaranteed or endorsed by the publisher.
Research integrity at Frontiers
Learn more about the work of our research integrity team to safeguard the quality of each article we publish.