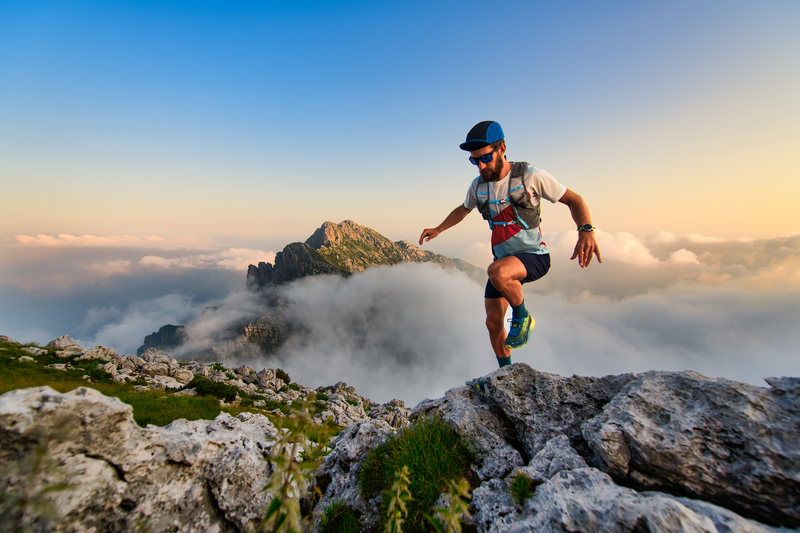
94% of researchers rate our articles as excellent or good
Learn more about the work of our research integrity team to safeguard the quality of each article we publish.
Find out more
ORIGINAL RESEARCH article
Front. Hum. Neurosci.
Sec. Brain Imaging and Stimulation
Volume 19 - 2025 | doi: 10.3389/fnhum.2025.1539222
The final, formatted version of the article will be published soon.
You have multiple emails registered with Frontiers:
Please enter your email address:
If you already have an account, please login
You don't have a Frontiers account ? You can register here
To explore the impact of different breathing patterns on brain connectivity and emotional states.We recruited 31 participants with an average age of 19 years. They were instructed to perform controlled breathing, including calm, shallow, deep, and alternating deep and shallow breathing patterns. We employed functional near-infrared spectroscopy (fNIRS) to investigate disparities in the effects of multiple breathing patterns on the brain. Meanwhile, we captured the participants' facial expressions and vital signs. There were significant variations in the effects of four breathing patterns on functional connectivity between brain regions, facial expressions, and vital signs. The four breathing patterns impacted six brain regions. Among them, alternating deep and shallow breathing had a particularly pronounced effect, and there was robust functional connectivity in different brain regions. Additionally, this breathing pattern elevated autonomic nervous system activity, which contributed to achieving a more tranquil state. Furthermore, alternating deep and shallow breathing had a more positive influence on the changes in oxyhaemoglobin concentration (Δ [HbO2]) of the brain compared with deep breathing.
Keywords: functional connectivity, facial expressions, functional near-infrared spectroscopy (fNIRS), respiratory rehabilitation training, respiratory pattern functional near-infrared spectroscopy, HbO2: oxyhaemoglobin, SPO2: peripheral capillary oxygen saturation, SDNN: standard deviation of the NN intervals
Received: 12 Dec 2024; Accepted: 21 Mar 2025.
Copyright: © 2025 Duan, Guo, Ren, Liu, Li, Liu, Xu and Huang. This is an open-access article distributed under the terms of the Creative Commons Attribution License (CC BY). The use, distribution or reproduction in other forums is permitted, provided the original author(s) or licensor are credited and that the original publication in this journal is cited, in accordance with accepted academic practice. No use, distribution or reproduction is permitted which does not comply with these terms.
* Correspondence:
Min Huang, Chengdu Medical College, Chengdu, China
Disclaimer: All claims expressed in this article are solely those of the authors and do not necessarily represent those of their affiliated organizations, or those of the publisher, the editors and the reviewers. Any product that may be evaluated in this article or claim that may be made by its manufacturer is not guaranteed or endorsed by the publisher.
Research integrity at Frontiers
Learn more about the work of our research integrity team to safeguard the quality of each article we publish.