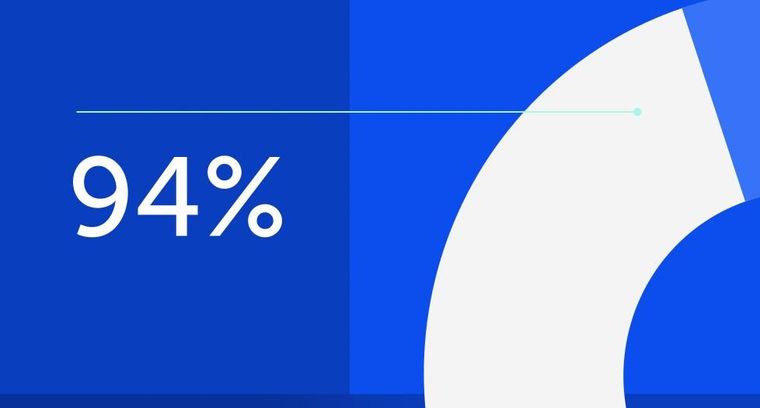
94% of researchers rate our articles as excellent or good
Learn more about the work of our research integrity team to safeguard the quality of each article we publish.
Find out more
ORIGINAL RESEARCH article
Front. Hum. Neurosci., 02 April 2025
Sec. Brain-Computer Interfaces
Volume 19 - 2025 | https://doi.org/10.3389/fnhum.2025.1539081
This article is part of the Research TopicNon invasive BCI for communicationView all 3 articles
Brain Computer Interface spellers offer a promising alternative for individuals with Amyotrophic Lateral Sclerosis (ALS) by facilitating communication without relying on muscle activity. This study assessed the feasibility of using movement related cortical potentials (MRCPs) as a control signal for a Brain-Computer Interface speller in an offline setting. Unlike motor imagery-based BCIs, this study focused on executed movements. Fifteen healthy subjects performed three spelling tasks that involved choosing specific letters displayed on a computer screen by performing a ballistic dorsiflexion of the dominant foot. Electroencephalographic signals were recorded from 10 sites centered around Cz. Three conditions were tested to evaluate MRCP performance under varying task demands: a control condition using repeated selections of the letter “O” to isolate movement-related brain activity; a phrase spelling condition with structured text (“HELLO IM FINE”) to simulate a meaningful spelling task with moderate cognitive load; and a random condition using a randomized sequence of letters to introduce higher task complexity by removing linguistic or semantic context. The success rate, defined as the presence of an MRCP, was manually determined. It was approximately 69% for both the control and phrase conditions, with a slight decrease in the random condition, likely due to increased task complexity. Significant differences in MRCP features were observed between conditions with Laplacian filtering, whereas no significant differences were found in single-site Cz recordings. These results contribute to the development of MRCP-based BCI spellers by demonstrating their feasibility in a spelling task. However, further research is required to implement and validate real-time applications.
In recent decades, the field of Brain Computer Interface (BCI) technology has undergone remarkable advancements, transitioning from theoretical concepts to practical applications (Aricò et al., 2020; Xu et al., 2024). A BCI is defined as a system in which commands are sent to external devices using only brain signals and without passing through the brain’s normal output pathways (Wolpaw et al., 2002). This innovative technology holds significant potential to empower individuals with motor disabilities, such as patients with Amyotrophic lateral sclerosis (ALS) (Masrori and Van Damme, 2020), as it could provide them with a means to communicate and engage with the world.
The basic structure of a BCI includes signal acquisition, feature extraction, classification or feature translation, and output or command (Cecotti, 2011; Shih et al., 2012; Wolpaw et al., 2002). During signal acquisition, brain signals are collected and used to extract specific features that are translated into commands capable of operating a device through mathematical algorithms (Shih et al., 2012; Wolpaw et al., 2002).
The efficacy of a BCI is linked to technological progress and understanding the basic underlying neuroscience, as well as the interaction between the user and the system. The features extracted are commonly individualized for the specific user to allow accurate and efficient translation into device commands (Abiri et al., 2019; Wolpaw et al., 2002; Xu et al., 2024).
One promising application of BCI technology is the development of “BCI Spellers, “which have gained substantial attention for their potential to allow communication for individuals with loss of voluntary motor control due to neurological conditions (Chaudhary et al., 2021; Rezeika et al., 2018).
BCI speller systems fall into two main acquisition method groups: invasive and non-invasive. Invasive methods involve surgical procedures to implant electrodes, providing high spatial and temporal resolution but requiring a potentially risky procedure, making them a less approachable solution (Chaudhary et al., 2022; Oh et al., 2024; Xu et al., 2024). On the other hand, non-invasive methods acquire signals from the scalp which introduces a loss in signal quality though it makes them easier to use and more user friendly (Abiri et al., 2019; Oh et al., 2024; Xu et al., 2024).
Traditional non-invasive BCI spellers and virtual menus rely on control signals such as the P300, steady-state visually evoked potentials (SSVEPs), and event-related spectral perturbations (ERSPs) (Abiri et al., 2019; Cecotti, 2011; Gannouni et al., 2017; Rezeika et al., 2018; Savić et al., 2013). These paradigms have achieved considerable success, with P300 and SSVEP-based BCIs demonstrating high accuracy and generally requiring minimal user training. However, the performance of P300 and SSVEP BCIs can be influenced by factors like stimulus presentation and individual user characteristics. ERSPs, while offering a different approach, can involve more extensive user training. These factors are important considerations when evaluating the suitability of different BCI paradigms for specific applications (Table 1).
In this context, movement-related cortical potentials (MRCPs) as a control signal may provide a feasible alternative to counteract some of the aforementioned limitations. MRCPs are low-frequency negative shifts in the EEG that occur approximately 2 s before the initiation of voluntary movement and can also be induced through motor imagery (Shakeel et al., 2015; Shibasaki and Hallett, 2006; Wright et al., 2011). The MRCP consists of various components associated with motor preparation and execution, including the Bereitschaftspotential (BP), an early negative shift, the negative slope (0.5 prior to movement onset) and motor potential (Crammond and Kalaska, 2000; Jochumsen et al., 2017; Shibasaki and Hallett, 2006).
ERSPs accompany voluntary movements and motor imagery as well but can also be induced or modulated by various cognitive tasks or affected by parallel streams of sensory inputs or the user’s mental state (Savić et al., 2020). Moreover, ERSPs exhibit variability in their patterns among individuals, in terms of frequency bands of interest and spatio-temporal dynamics. As a result, extensive user training and calibration of both subjects and the BCI are required to operate the system using motor imagery-induced ERSPs (Savić et al., 2020).
In contrast, MRCPs are direct and reproducible signatures of motor preparation, execution, or imagery, exhibit low user training requirements, are adaptable through training and detectable before movement onset (Jochumsen et al., 2018; Savić et al., 2020, 2021; Wright et al., 2011, 2012). However, due to their low signal-to-noise ratio, they have been less commonly used for BCI control. Additionally, MRCPs have shown particular sensitivity to specific visual cues, which can disrupt their morphological features and may limit their use in cue-based applications such as menus and spelling (Savić et al., 2014).
Despite this, recent studies have demonstrated the concept and feasibility of single-trial MRCP detection using novel feature extraction and machine learning techniques (Jiang et al., 2015; Savić et al., 2021). These advances in MRCP detection and classification for BCI control purposes, they have not yet been specifically applied to BCI spelling applications.
This study investigates the feasibility of using MRCPs as control signals for a BCI speller system. While other speller approaches are mentioned as reference points, a direct comparison goes beyond the scope of this study. Our primary goal is to evaluate whether MRCPs can be effectively utilized in this specific framework. Unlike motor imagery-based BCIs, we examine MRCPs elicited by executed movements, where participants actively performed dorsiflexion. To establish a foundational understanding of MRCP behavior in this context, we conducted the experiments in an offline setting, collecting and analyzing MRCP data post-hoc without real-time detection. Instead of implementing machine learning classification or automated MRCP detection, we established predefined criteria to assess the presence of MRCPs, evaluating their reliability as the success rate. Additionally, we explored how MRCP features are influenced by the cognitive load, visual cues, and stimuli imposed by the spelling task.
Fifteen healthy young adults (seven females, eight males; mean age = 24.38 ± 2.98 years) without any neurological disorders or other impairments voluntarily participated in this study. Due to a technical issue with the EEG system during data acquisition, specifically the failure of a EEG or EMG electrode, data from two subjects were excluded from further analysis. This malfunction resulted in unusable data for those channels, rendering the entire dataset for those participants unusable. This exclusion was based on the objective criterion of complete electrode failure. Consequently, the final dataset comprised 13 participants (seven females, six males). All participants provided informed consent prior to their inclusion in the experiment. The study protocol was conducted according to the Declaration of Helsinki.
During all sessions, monopolar EEG signals were recorded using an active EEG electrode system (g.GAMMAcap; g.tec Medical Engineering, GmbH, Austria) connected to a g.USBamp amplifier (g.tec Medical Engineering, GmbH, Austria). Ten electrodes were placed over the motor cortex according to the standard international 10–20 system: FP1, Fz, FC1, FC2, C3, Cz, C4, CP1, CP2, and Pz. The ground electrode was placed centrally on the frontopolar region (FPz), and the contralateral earlobe was used for reference. Surface EMG electrodes were placed on the musculus tibialis anterior (TA) to determine movement onset (Figure 1). All data were sampled at a rate of 1,200 Hz.
Figure 1. The experimental set up for the experiment with the keyboard on the screen and EEG and EMG electrodes connected to the g.USBamp system.
During the actual experiment, subjects interacted with a custom-made speller interface consisting of a 6 × 6 matrix design (Farwell and Donchin, 1988) with characters to choose from and a continuously moving selector (Figure 2). The selector consists of a gray bar that moves horizontally (x-axis) until a selection is made, then changes direction to move vertically (y-axis).
Figure 2. The speller with custom designed interface. The grey bar is the moving selector and phrase area is where chosen characters would appear. The selector first scanned the speller matrix from left to right to identify the column containing the target letters, predefined by the condition (control, phrase spelling, or random). Once the column was reached, the selector changed direction and scanned from bottom to top to locate the target letter’s row. After selecting the row, the selector restarted its position to search for the next target letter, following the same column-first, then row-selection process.
The BCI speller operates as a simulation, where letter selections were predefined, and the system automatically pauses at the corresponding column or row, assuming that the subject would initiate movement during the designated time. Each column and row selection lasted for 2 s. After each selection, the bar paused for 2 s before resuming movement for the next selection. The speller includes a blank area at the top displaying the selected letters or a predefined phrase, with the last selected letter highlighted.
For each condition, the target phrase was presented at the beginning of the experiment in the Phrase Area of the speller interface (Figure 2). The selector moved from left to right, sliding over the columns (which were internally divided within the speller matrix). Once the selector reaches the end of the predefined column of the target letter, it automatically changed direction and moved from bottom to top to stop at the end of the corresponding row. The rows and columns were visually marked with dividers to aid user orientation (Figure 2). Once a letter was selected the bar reset its position at the left of the screen to restart the sliding. Participants were cued to execute a movement when the selector reached the target location (column/row) of the letter. This ensured that MRCPs were aligned with voluntary movement at the desired letter.
All measurements were conducted at the neurophysiological laboratory of the Department of Sports and Sports Science of the University Freiburg.
The subjects were instructed to perform a ballistic dorsiflexion of the dominant leg, executing the movement when the bar was around the midpoint of the column or row containing the desired character. Each subject completed three different phrases, each consisting of 13 characters, resulting in 26 trials or 26 possible MRCPs per phrase (Table 2).
To evaluate MRCP performance in conjunction with a simulated spelling task, three conditions were tested. These conditions were designed to assess how MRCPs are affected by the varying demands of a spelling task, ranging from minimal cognitive load to more complex scenarios involving memory and letter selection. Cognitive load, in this study, refers to the mental effort imposed on participants by the task demands, including factors such as memory, attention, and decision-making. The three conditions were: a control condition using repeated selections of the letter “O” to isolate movement-related brain activity with minimal cognitive load; a phrase spelling condition with structured text (“HELLO IM FINE”) to simulate a meaningful spelling task with moderate cognitive load, reflecting the memory and planning involved in spelling a familiar phrase; and a random condition using a randomized sequence of letters to introduce higher task complexity, as participants needed to process and select letters without the aid of linguistic or semantic context, thus increasing demands on attention and working memory.
All signal analysis was done with a custom MATLAB script and GUI (version 2022a, MathWorks, Natick, USA). The CZ electrode was chosen for the subsequent analysis, as it is the electrode that is highly associated with lower limb movement (Do Nascimento et al., 2006; Shibasaki et al., 1986; Shibasaki and Hallett, 2006). The EMG data was used as supplementary data for the detection of the movement and as part of the criteria for selecting the MRCPs.
A simplified signal processing pipeline was implemented, avoiding computationally expensive techniques such as Independent Component Analysis (ICA) for artifact detection. Instead, we employed filtering and epoch-based processing techniques that are compatible with real-time BCI applications. Both the MRCP traces and their features were extracted and analyzed (Figure 3), including peak negativity, readiness potential slopes, negative slope, motor potential, and overall MRCP amplitude (PN, NS1, NS2, rebound, and PP). The preprocessing steps are described as follows (Figures 4, 5):
Figure 3. MRCP features. The black trace represents an example of an MRCP, with dotted lines indicating the time intervals used to compute the slopes (NS1, NS2, and rebound). The PN (Peak Negativity) is identified as the minimum value on the trace, while the PP (Peak-to-Peak) represents the overall amplitude of the MRCP (the difference between the minimum and maximum value of the signal from 2 s before PN to 2 s after).
Line noise was removed by applying a 50 Hz notch filter using a 2nd order Butterworth filter. EEG channels were then filtered using a 2nd order Butterworth band-pass filter ranging from 0.05 to 3 Hz. Next, EEG and EMG data were segmented into epochs 4 s before to 5 s after column/row onsets (Figure 6). A column/row onset was defined as the moment the selector bar intersected the column or row containing the target letter. Offset correction was applied per epoch by demeaning – that is, by calculating the mean for each of them and subtracting it. To improve spatial resolution, a large Laplacian transformation was applied to the Cz electrode. EMG onset detection was performed using an adapted version of Yang’s algorithm, which identifies movement initiation by detecting deviations from baseline activity and image processing techniques (Yang et al., 2017). This adapted version included modifications to the threshold levels for detecting EMG activity and adjustments to the window length for morphological image processing, such as opening and closing techniques. The window duration was set to 1.5 s, based on the participants’ intent to sustain movement for approximately 2 s. This method was used solely for confirming movement execution and not for training or classifying MRCPs in real-time.
Figure 6. MATLAB custom made GUI used for the preprocessing, segmentation, and movement verification.
Data analysis focused on the Cz electrode and the Laplacian result of this electrode for each trial. Column/row onsets were used to segment the data and define individual trials. The EMG signal was then analyzed to confirm that the subject performed the intended movement within the appropriate time window. Both segmentation and movement verification were performed using a custom MATLAB graphical user interface (GUI).
After confirming the movement, the segmented data (trials) were inspected with the GUI to verify the presence of MRCPs. The GUI was also used to identify the Peak Negativity (PN) within each trial. A trial was considered successful, indicating the presence of an MRCP, if it met all the following criteria: (1) PN and EMG onset occurred within the time window of −0.5–0.5 s relative to the column/row onset; (2) the MRCP exhibited two distinct negative slopes followed by a rebound phase; (3) PN corresponded to a local minimum in the MRCP; or (4) PN occurred more than 1 s before the column/row onset (Figure 3).
This stepwise approach ensured that data segmentation, movement verification, MRCP validation, and PN identification were carried out systematically, resulting in the reliable selection of valid MRCPs across all trials.
The number of successful trials per phrase, condition, and subject was calculated as a percentage of the total trials (n = 26). In this study, this metric is referred to as the success rate, which indicates the number of times a subject successfully elicited an MRCP, as determined based on the inclusion criteria outlined in the previous paragraph. Success rates were calculated separately for both Cz and Laplacian-processed data. To standardize onset, three datasets per phrase were created, with MRCPs aligned based on three different time points: the column/row onset, the EMG onset (defined as the start of EMG activity), and the Peak Negativity (PN) timing. Each dataset was centered around the corresponding time point (column/row, EMG, or PN) and consisted of epochs extending 2 s before and 1.5 s after the specified time point. The PN-shifted dataset was selected for further analysis due to phase cancelation observed in other shifts.
Grand averages were computed per condition by grouping selected trials by condition, while Statistical Parametric Mapping (SPM) was conducted by dividing selected trials by subject and condition. For each subject-condition combination, a mean was calculated, yielding 39 average MRCPs (13 subjects * 3 conditions). These were analyzed using one-way ANOVA in MATLAB’s SPM toolbox (Pataky, 2022), with post-hoc paired t-tests and Bonferroni correction if significant results (p < 0.05) were found.
Descriptive features (PN, NS1, NS2, rebound, PP) were extracted (Figure 3), per trial and condition. Finally, statistical analysis involved computing the mean of each feature per subject and condition. Organized arrays were created with columns representing conditions and rows representing subjects. Five arrays, each representing a feature from both preprocessing methods (non-Laplacian and Laplacian), were analyzed using Friedman’s test. If significance was detected, a Wilcoxon signed-rank post-hoc test with Bonferroni correction was applied for the feature.
In order to identify the MRCPs, the continuous data was segmented according to the expected column/row onsets. Subsequently, two onset-shifting techniques, PN shifting and EMG onset shifting, were applied. For each subject and condition, the mean MRCP per subject across conditions and shifting methods were computed (Figure 7), to determine the best onset for feature extraction. The analysis concluded that PN shifting provided better results, making it the chosen reference point for both non-Laplacian and Laplacian data.
Figure 7. Laplacian vs. non-Laplacian on different onsets shifts. The means MRCP for subject 03 across conditions and shifting methods. The figure illustrates the mean and standard deviation of all the MRCPs, with (orange) and without (blue) Laplacian, considering shifting and condition. The graphs are organized in columns and rows. Columns represent different conditions: letter (left), phrase (middle), random (right). Rows indicate various shifting methods: no shifting or column/row (top), based on the PN (middle) and based on the EMG (bottom).
To evaluate whether MRCP elicitation can be effectively used with the speller, the success rates of participants were calculated. Results are presented for each subject (S01–S15, excluding S10 and S11), detailing the number of elicited MRCPs and corresponding success rates across different conditions. This analysis was conducted both without and with Laplacian filtering, the results are shown in Table 3, 4 correspondingly.
For the analysis without Laplacian filtering, the mean number of elicited MRCPs of the control condition and the spelling phrase was similar (17.3 ± 4.06 and 17.31 ± 3.67) and were not significantly different (Table 3). In contrast, a slight decrease in the mean was observed for the random characters condition (16.26 ± 4.79). Success rates equal or below 50% occurred only six times for various conditions and subjects. On the other hand, 11 times different subjects, and across various conditions, achieved percentages equal to or higher than 80%.
Implementing Laplacian filtering did not significantly improve the mean success rate (Table 4) of the control condition or the spelling phrase (17.38 ± 3.30 and 17.28 ± 3.60). Similarly, in the processing without Laplacian, these means did not exhibit significant differences between the two conditions. The most notable difference was observed in the random characters condition, where the mean decreased to almost 50% success rate (13.64 ± 5.01).
The implementation of the Laplacian filter enhanced or matched the success rate to the non-Laplacian results, for six subjects in at least two out of the three conditions-specifically, S01, S02, S03, S05, S06, and S15.
Additionally, there was a consistent or increased success rate observed from the spelling phrase condition compared to the control condition in eight participants:
• Non-Laplacian: S01, S02, S03, S04, S05, S06, S12, S13.
• Laplacian: S01, S02, S03, S07, S09, S12, S14, S15.
In contrast, when comparing the control condition to the random one, a decrease in success rate was noted for eight subjects in both the non-Laplacian and the Laplacian results.
• Non-Laplacian: S02, S03, S07, S08, S12, S13, S14, S15.
• Laplacian: S01, S02, S04, S05, S07, S09, S13, S14.
To assess whether the interface and spelling task affect the morphology of the MRCPs, features were extracted from all selected trials for each subject and condition. The mean of each feature was computed for each subject and condition, and repeated for all features. These means were then statistically compared for both processing methods and the results are shown in Table 5 (non-Laplacian) and Table 6 (Laplacian).
From the Friedman test results, only one feature showed significance (p < 0.05). This feature was the PP in the Laplacian dataset (p = 0.0231). A Wilcoxon rank-sum post-hoc test was performed for pairwise comparisons with a Bonferroni correction (adjusted significance level: p < 0.0167), with results presented in Table 7. No significant differences were found in this test. The comparisons of the features between the control condition and the phrase (p = 0.1272) and against the random condition (p = 0.0574) were the smallest, but neither showed significant differences.
To compare the three conditions, we calculated the grand average of the MRCPs for each condition and conducted an SPM analysis. The grand averages were obtained by averaging the individual MRCP traces over three conditions (Figure 8). The resulting grand averages across conditions exhibit similar morphology, with overlapping confidence intervals.
Figure 8. Cz grand average MRCP data. The top panel displays the grand averages of the 12 participants (trials mean of 17.30—letter, 17.31—phrase 16.24 -random) for each condition, the dashed line over the y axis represents the PN of the grand averages. The lower trace presents the SPM analysis results. Both indicate that there are no differences across the three conditions.
To further investigate these similarities, an SPM analysis was conducted using a one-way ANOVA. For this analysis, MRCPs were first averaged per subject for each condition, resulting in 39 averaged MRCPs (13 subjects * 3 conditions). The SPM analysis confirmed that there were no statistically significant differences between the three conditions, consistent with the observed grand averages.
The Laplacian data showed significant differences across conditions except between −1.56 s and −1.3 s (Figure 9). Post-hoc paired t-tests with Bonferroni correction (Supplementary Figure 1) revealed the following: For the control vs. phrase condition, only the interval from −1.55 s to −1.0 s was not significant (p ≤ 0.2). For the letter vs. random condition, significant differences were found between −2.0 s and −1.88 s, −0.53 s and −0.36 s, and −0.17 s and 1.5 s, with the letter showing the greatest difference. The phrase vs. random condition had a significant difference only between −0.94 s and −0.4 s (p ≤ 0.2).
Figure 9. The Laplacian data. The top panel displays the grand averages of the 12 participants (trials mean of 17.38—letter, 17.28—phrase 13.64 -random) for each condition, the dashed line over the y axis represents the PN of the grand averages, while the black traces show the times where there was significance between conditions. The lower trace presents the SPM analysis results, where the grey shaded area represents the significance.
The aim of the current study was to assess the feasibility of using MRCPs as a control signal for a BCI-speller system. Specifically, we focused on analyzing the MRCP and its features under the cognitive load imposed by the simulation of a spelling task generated by the BCI speller that was designed for this purpose. Our findings indicate that participants successfully generated MRCPs during the tasks, and no significant differences were observed across conditions for the MRCP features. The SPM analysis revealed some differences between conditions only for the Laplacian channel/data. In the following subsections we will provide a detailed discussion of the results.
In the field of BCI, selecting an appropriate control signal that accurately reflects the user’s intent is critical for the system’s effectiveness (Abiri et al., 2019; Krusienski et al., 2012; Wolpaw et al., 2002). In this study, we chose MRCPs as a potential control signal for BCI spellers.
We implemented the success rate, defined as the occurrence of an MRCP, as a metric to evaluate the correlation between the user’s intent and the actual brain output measured by EEG. High success rates suggest that the MRCP has the potential to serve as a reliable control signal, capable of effectively capturing the user’s intent.
Compared to the control condition, the success rates of the phrase spelling condition met expectations, with 9 out of 12 subjects achieving at least 69% success. Notably, six participants were novices, suggesting that MRCPs can be elicited without extensive user training (Jochumsen et al., 2018; Niazi et al., 2013). This trend persisted in the phrase spelling condition, where 10 participants matched or exceeded their performance, supporting the idea that MRCP-based control can be trainable (Jochumsen et al., 2017). While this may appear lower than classification accuracies reported in P300 and SSVEP studies (Abiri et al., 2019; Rezeika et al., 2018), it is important to note that participants in this study had no prior experience or training in eliciting MRCPs. Despite this lack of user training, MRCPs were successfully elicited, suggesting that performance could improve with additional practice and adaptation.
On the other hand, the random condition revealed that just seven subjects achieved an equal or higher success rate than 69%. This decrease could be attributed to the diversion of attention (Aliakbaryhosseinabadi et al., 2017a; Aliakbaryhosseinabadi et al., 2017b), or the complexity of selecting random characters due to the lack of goal-oriented spelling (Frömer et al., 2012; Olsen et al., 2021).
The Laplacian filter improved or maintained the success rate in six subjects across at least two of three conditions but decreased in the others. As a spatial filter, the Laplacian reduces spatial noise by emphasizing local signal sources and minimizing distant ones. While effective in subjects with well-localized MRCPs, the filter can introduce distortions in cases where signals are more diffuse. Additionally, the Laplacian has been shown to counteract attention diversion during tasks, potentially explaining its improved performance in some subjects (Aliakbaryhosseinabadi et al., 2017a; McFarland, 2015).
The grand average MRCPs were computed based on three different onsets (column/ row, PN and EMG), revealing a phase-canceling effect for the column/row and EMG onset. The PN serves as the crucial point marking the end of the negative shift and the beginning of the positive shift (Do Nascimento et al., 2006; Shibasaki and Hallett, 2006). Therefore, it proves to be an excellent reference point for extracting the MRCP features with consistency. Additionally, this selection aligns with the goal of enabling non-muscular control signals as it remains present during motor imagery (Cunnington et al., 1996), which are essential for BCI applications, especially in patients with motor impairments.
Once an onset was selected the MRCPs were analyzed with and without Laplacian filtering. In the non-Laplacian data, MRCPs behaved as expected, showing no statistically significant differences across conditions, either in feature extraction or SPM analysis. Prior research indicates that MRCP morphology, particularly in features like NS1 and NS2, can change with attention diversion (Aliakbaryhosseinabadi et al., 2017a; Aliakbaryhosseinabadi et al., 2017b; Wright et al., 2012). The absence of significant changes here suggests that the spelling task did not impose a cognitive load that interfered with the movement timing or focus.
The use of the Laplacian filter in processing MRCP signals presents both benefits and challenges, particularly when comparing tasks with different cognitive demands. During spelling tasks, which are likely to engage broader cortical regions due to the increased complexity, the Laplacian filter may show amplitude differences in the MRCP. This occurs because the filter enhances localized neural activity but may smooth out signals spread across multiple channels, which is more common in cognitively demanding tasks. In contrast, monotonic tasks such as the control condition, are less affected by the Laplacian because they involve more focused neural activity that tends to produce clearer MRCP signals (Aliakbaryhosseinabadi et al., 2017a; Aliakbaryhosseinabadi et al., 2017b; McFarland, 2015).
Interestingly, in our analysis, while no significant differences were found in the extracted MRCP features, the SPM analysis of the Laplacian-processed data did show differences across conditions. This suggests that the Laplacian filter, while effective at reducing noise, might amplify specific aspects of the MRCPs and reduce their overall amplitude. This suggests that while Laplacian filtering can refine the signal by reducing noise, it may also alter the MRCP morphology, warranting careful consideration in future studies.
P300 and SSVEP spellers have established themselves as reliable BCI paradigms, consistently achieving accuracy rates of 80–95% with information transfer rates of 20–25 bits/min (Gannouni et al., 2022; Kundu and Ari, 2022; Rezeika et al., 2018). However, they require multiple stimulus presentations for each selection and can cause visual fatigue during extended use (Abiri et al., 2019; Gannouni et al., 2022; Rezeika et al., 2018).
In contrast, MRCP-based BCIs offer single-trial detection capability with accuracy rates of 75–85%, though their inherent low-frequency characteristics (~2 s per decision) may result in lower information transfer rates depending on the temporal analysis window (Jochumsen et al., 2020; Olsen et al., 2021; Savić et al., 2021). Recent advances in MRCP detection, including predictive algorithms for low-latency detection and hybrid approaches combining MRCP with ERD/ERS features to increase detection accuracy, show promise in reducing these speed and accuracy limitations (Savić et al., 2014; Savić et al., 2020).
MRCPs offer several advantages for BCI control, including reduced dependence on external stimulation, real-time feedback, and short latency, which could enhance usability and effectiveness in real-world applications (Savić et al., 2020, 2021). However, unlike SSVEP- and P300-based BCIs, which achieve high classification accuracy with minimal user training, MRCP-based systems may require further refinement and validation to reach comparable reliability. Although this study did not implement an online algorithm or automated classification, previous research has demonstrated the successful integration of MRCPs into BCI systems, highlighting their potential as a viable control signal (Savić et al., 2021).
While this study offers promising insights into the development of an MRCP-based BCI speller, several limitations should be considered for future research. Participants performed the spelling task without any prior training. Additional training could improve the reliability of MRCPs elicitation, as some participants exhibited increased success after just one session (Jochumsen et al., 2017). Furthermore, the experimental sessions lasted only 5–10 min, which does not reflect the real-world use of a BCI speller. Investigating MRCP viability for longer periods, accounting for fatigue, is essential for practical applications.
This study demonstrated that MRCPs can be elicited in BCI-naïve users during a simulated BCI-speller task. However, a crucial limitation is that the analyzed MRCPs were derived from *executed* movements, not imagined or attempted ones. This may constrain the interpretation of our results, as they primarily reflect the feasibility of MRCP-based BCIs in scenarios where voluntary motor execution is possible. While MRCPs can be elicited by both executed movements and motor imagery (Do Nascimento et al., 2006; Gu et al., 2013; Shakeel et al., 2015), MRCPs elicited during imagined movements are often weaker and exhibit different characteristics (Do Nascimento et al., 2006; Gu et al., 2013). However not so much is known with regards to attempted movement – thus where patients unable to move due to paralysis attempt to perform the task. Previous research has demonstrated the feasibility of using MRCPs elicited by motor intention (i.e., motor imagery) (Aliakbaryhosseinabadi et al., 2020; Jiang et al., 2015; Niazi et al., 2013; Savić et al., 2021), however, the inherent differences in MRCP characteristics between executed and imagined movements, particularly the potentially weaker signal strength during motor imagery, are critical considerations for future development of MRCP-based BCIs for communication and control. Future research must validate the applicability of this approach in individuals with limited motor abilities.
Even though MRCPs have the potential to be used in BCIs without external stimulation, the specific implementation in this study used a visual speller interface with moving selectors to guide letter selection. This visual interface, while different from flashing stimuli, could also potentially contribute to visual fatigue during extended use, although this was not directly assessed in the present study.
In this study, the presence of the MRCPs was determined through manual inspection of the EEG data using exclusion criteria predefined by the researchers. Each trial was evaluated based on these criteria, and only trials meeting the specified conditions were counted toward the success rate. This systematic approach ensured consistency in MRCP identification. To advance the practical application of MRCP-based BCIs, future work will focus on developing and implementing machine learning algorithms for automated MRCP detection and classification, which could improve accuracy and efficiency in both executed and imagined movement scenarios.
Additionally, while high-pass filters have been used to reduce eye and head movement artifacts, MRCPs are in the frequency range of these artifacts meaning the filters may not be fully effective. Future research should integrate eye tracking and head movement measurements to improve signal quality. The current study was conducted offline, and the next step is to perform online trials to assess real-time performance. In an online setting, MRCPs may be influenced by factors like fatigue, feedback and user errors, making another metrics such as false positives, precision, and accuracy crucial for evaluating system effectiveness.
Significant progress has been made in non-invasive BCI spellers using P300, Steady-State Visual Evoked Potentials (SSVEP), and Motor Imagery (MI) paradigms, each offering advantages such as rapid response (P300), high information transfer rates (SSVEP), and well-established control schemes (MI induced ERSPs) (Cecotti, 2011; Rezeika et al., 2018). However, these approaches also face challenges including reliance on external stimulation (P300, SSVEP), which can lead to fatigue, and extensive user training requirements (MI) (Abiri et al., 2019; Kundu and Ari, 2022; Nicolas-Alonso and Gomez-Gil, 2012; Rezeika et al., 2018). Here we analyzed the potential of MRCPs as an alternative approach, focusing on their elicitation during a simulated spelling task.
This study explored the feasibility of MRCPs as an alternative control signal for BCI spellers, focusing on their elicitation during a simulated spelling task with untrained participants. While potential advantages of MRCP-based BCIs have been suggested in prior research (Aliakbaryhosseinabadi et al., 2020; Aliakbaryhosseinabadi et al., 2017a; Aliakbaryhosseinabadi et al., 2017b; Savić et al., 2021) our study did not directly assess them but demonstrated that MRCPs can be reliably elicited with motor execution in an offline BCI-spelling simulation.
The Laplacian filter’s impact was mixed—enhancing performance in some cases while reducing it in others—yet MRCPs consistently proved to be a promising and practical alternative for BCI spellers, which may enhance their usability and effectiveness in specific users and application scenarios. These findings suggest that MRCPs could address some of the limitations of existing paradigms, providing a feasible solution for future BCI applications.
While our findings provide an initial assessment of MRCPs for BCI spellers, direct generalization to populations with neurological conditions like ALS requires further investigation. This will be an area of focus in our upcoming research.
The raw data supporting the conclusions of this article will be made available by the authors, without undue reservation.
The studies were conducted in accordance with local legislation and institutional requirements. Participants provided written informed consent to participate in this study. Additionally, written informed consent was obtained from the individual(s) for the publication of any potentially identifiable images or data included in this article.
JH-G: Conceptualization, Data curation, Formal analysis, Investigation, Methodology, Project administration, Software, Validation, Visualization, Writing – original draft. AJ-G: Conceptualization, Data curation, Formal analysis, Methodology, Supervision, Validation, Writing – review & editing. AS: Conceptualization, Methodology, Supervision, Validation, Writing – review & editing. NM-K: Conceptualization, Formal analysis, Methodology, Resources, Supervision, Validation, Writing – review & editing.
The author(s) declare that financial support was received for the research and/or publication of this article. Author AS was financially supported by the Ministry of Science, Technological Development and Innovation of the Republic of Serbia under contract number: 451-03-136/2025-03/200103. The authors acknowledge the support of the Open Access Publication Fund of the University of Freiburg.
We thank all participants for their time and effort, which was crucial to this study.
The authors declare that the research was conducted in the absence of any commercial or financial relationships that could be construed as a potential conflict of interest.
The authors declare that Gen AI was used in the creation of this manuscript. This manuscript was prepared with the assistance of ChatGPT for tasks limited to grammar correction and vocabulary enhancement.
All claims expressed in this article are solely those of the authors and do not necessarily represent those of their affiliated organizations, or those of the publisher, the editors and the reviewers. Any product that may be evaluated in this article, or claim that may be made by its manufacturer, is not guaranteed or endorsed by the publisher.
The Supplementary material for this article can be found online at: https://www.frontiersin.org/articles/10.3389/fnhum.2025.1539081/full#supplementary-material
Supplementary Figure 1 | The Laplacian data - post-hoc tests. The figure shows the grand averages of the compared conditions and the corresponding post-hoc tests with a Bonferroni correction. The left trace shows the comparison between the control and the phrase condition. The middle trace shows the comparison between the letter and the random condition. The right trace shows the phrase vs. the random condition. The black lines on the top plots indicate where in time there was significance between the conditions. The grey shaded areas in the bottom plots represent the significance.
Abiri, R., Borhani, S., Sellers, E. W., Jiang, Y., and Zhao, X. (2019). A comprehensive review of EEG-based brain-computer interface paradigms. J. Neural Eng. 16:011001. doi: 10.1088/1741-2552/aaf12e
Aliakbaryhosseinabadi, S., Farina, D., and Mrachacz-Kersting, N. (2020). Real-time neurofeedback is effective in reducing diversion of attention from a motor task in 1 healthy individuals and patients with amyotrophic lateral sclerosis 2. J. Neural Eng. 17:036017. doi: 10.1088/1741-2552/ab909c
Aliakbaryhosseinabadi, S., Kamavuako, E. N., Jiang, N., Farina, D., and Mrachacz-Kersting, N. (2017a). Influence of dual-tasking with different levels of attention diversion on characteristics of the movement-related cortical potential. Brain Res. 1674, 10–19. doi: 10.1016/j.brainres.2017.08.016
Aliakbaryhosseinabadi, S., Kostic, V., Pavlovic, A., Radovanovic, S., Nlandu Kamavuako, E., Jiang, N., et al. (2017b). Influence of attention alternation on movement-related cortical potentials in healthy individuals and stroke patients. Clin. Neurophysiol. 128, 165–175. doi: 10.1016/j.clinph.2016.11.001
Aricò, P., Sciaraffa, N., and Babiloni, F. (2020). Brain–computer interfaces: toward a daily life employment. Brain Sci. 10:157. doi: 10.3390/brainsci10030157
Cecotti, H. (2011). Spelling with non-invasive brain-computer interfaces - current and future trends. J. Physiol. 105, 106–114. doi: 10.1016/j.jphysparis.2011.08.003
Chaudhary, U., Mrachacz-Kersting, N., and Birbaumer, N. (2021). Neuropsychological and neurophysiological aspects of brain-computer-interface (BCI) control in paralysis. J. Physiol. 599, 2351–2359. doi: 10.1113/JP278775
Chaudhary, U., Vlachos, I., Zimmermann, J. B., Espinosa, A., Tonin, A., Jaramillo-Gonzalez, A., et al. (2022). Spelling interface using intracortical signals in a completely locked-in patient enabled via auditory neurofeedback training. Nat. Commun. 13:28859. doi: 10.1038/s41467-022-28859-8
Crammond, D. J., and Kalaska, J. F. (2000). Prior information in motor and premotor cortex: activity during the delay period and effect on pre-movement activity. J. Neurophysiol. 84, 986–1005. doi: 10.1152/jn.2000.84.2.986
Cunnington, R., Iansek, R., Bradshaw, J. L., Phillips, J. G., Cunnington, R., Bradshaw, J. L., et al. (1996). Movement-related potentials associated with movement preparation and motor imagery. Exp. Brain Res. 111:8732. doi: 10.1007/BF00228732
Do Nascimento, O. F., Nielsen, K. D., and Voigt, M. (2006). Movement-related parameters modulate cortical activity during imaginary isometric plantar-flexions. Exp. Brain Res. 171, 78–90. doi: 10.1007/s00221-005-0247-z
Farwell, L., and Donchin, E. (1988). Talking off the top of your head: Toward a mental prosthesis utilizing event-related brain potentials. Electroencephalography and Clinical Neurophysiology 70, 510–523.
Frömer, R., Hafner, V., and Sommer, W. (2012). Aiming for the bull’s eye: preparing for throwing investigated with event-related brain potentials. Psychophysiology 49, 335–344. doi: 10.1111/j.1469-8986.2011.01317.x
Gannouni, S., Alangari, N., Mathkour, H., Aboalsamh, H., and Belwafi, K. (2017). BCWB: a p300 brain-controlled web browser. Int. J. Semant. Web Inf. Syst. 13, 55–73. doi: 10.4018/IJSWIS.2017040104
Gannouni, S., Belwafi, K., Al-Sulmi, M. R., Al-Farhood, M. D., Al-Obaid, O. A., Al-Awadh, A. M., et al. (2022). A brain controlled command-line Interface to enhance the accessibility of severe motor disabled people to personnel computer. Brain Sci. 12:926. doi: 10.3390/brainsci12070926
Gu, Y., Farina, D., Murguialday, A. R., Dremstrup, K., and Birbaumer, N. (2013). Comparison of movement related cortical potential in healthy people and amyotrophic lateral sclerosis patients. Front. Neurosci. 7:65. doi: 10.3389/fnins.2013.00065
Jiang, N., Gizzi, L., Mrachacz-Kersting, N., Dremstrup, K., and Farina, D. (2015). A brain-computer interface for single-trial detection of gait initiation from movement related cortical potentials. Clin. Neurophysiol. 126, 154–159. doi: 10.1016/j.clinph.2014.05.003
Jochumsen, M., Knoche, H., Kjaer, T. W., Dinesen, B., and Kidmose, P. (2020). EEG headset evaluation for detection of single-trial movement intention for brain-computer interfaces. Sensors 20:804. doi: 10.3390/s20102804
Jochumsen, M., Niazi, I. K., Nedergaard, R. W., Navid, M. S., and Dremstrup, K. (2018). Effect of subject training on a movement-related cortical potential-based brain-computer interface. Biomed. Signal Proc. Control 41, 63–68. doi: 10.1016/j.bspc.2017.11.012
Jochumsen, M., Rovsing, C., Rovsing, H., Cremoux, S., Signal, N., Allen, K., et al. (2017). Quantification of movement-related EEG correlates associated with motor training: a study on movement-related cortical potentials and sensorimotor rhythms. Front. Hum. Neurosci. 11:604. doi: 10.3389/fnhum.2017.00604
Krusienski, D. J., McFarland, D. J., and Principe, J. C. (2012). “BCI Signal processing: feature extraction” in Brain–computer interfaces principles and practice. eds. D. J. McFarland and D. J. Krusienski (Oxford: Oxford University Press), 124–145.
Kundu, S., and Ari, S. (2022). Brain-computer Interface speller system for alternative communication: a review. IRBM 43, 317–324. doi: 10.1016/j.irbm.2021.07.001
Li, M., He, D., Li, C., and Qi, S. (2021). Brain–computer interface speller based on steady-state visual evoked potential: a review focusing on the stimulus paradigm and performance. Brain Sci. 11:0450. doi: 10.3390/brainsci11040450
Masrori, P., and Van Damme, P. (2020). Amyotrophic lateral sclerosis: a clinical review. Eur. J. Neurol. 27, 1918–1929. doi: 10.1111/ene.14393
McFarland, D. J. (2015). The advantages of the surface Laplacian in brain-computer interface research. Int. J. Psychophysiol. 97, 271–276. doi: 10.1016/j.ijpsycho.2014.07.009
Niazi, I. K., Jiang, N., Jochumsen, M., Nielsen, J. F., Dremstrup, K., and Farina, D. (2013). Detection of movement-related cortical potentials based on subject-independent training. Med. Biol. Eng. Comput. 51, 507–512. doi: 10.1007/s11517-012-1018-1
Nicolas-Alonso, L. F., and Gomez-Gil, J. (2012). Brain computer interfaces, a review. Sensors 12, 1211–1279. doi: 10.3390/s120201211
Oh, E., Shin, S., and Kim, S. P. (2024). Brain–computer interface in critical care and rehabilitation. Acute Critic. Care 39, 24–33. doi: 10.4266/ACC.2023.01382
Olsen, S., Alder, G., Williams, M., Chambers, S., Jochumsen, M., Signal, N., et al. (2021). Electroencephalographic recording of the movement-related cortical potential in ecologically valid movements: a scoping review. Front. Neurosci. 15:721387. doi: 10.3389/fnins.2021.721387
Pataky, T. (2022). spm1D. Available online at: https://spm1d.org/index.html (Accessed July 15, 2024).
Rezeika, A., Benda, M., Stawicki, P., Gembler, F., Saboor, A., and Volosyak, I. (2018). Brain–computer interface spellers: a review. Brain Sci. 8:57. doi: 10.3390/brainsci8040057
Savić, A. M., Aliakbaryhosseinabadi, S., Blicher, J. U., Farina, D., Mrachacz-Kersting, N., and Došen, S. (2021). Online control of an assistive active glove by slow cortical signals in patients with amyotrophic lateral sclerosis. J. Neural Eng. 18:046085. doi: 10.1088/1741-2552/ac0488
Savić, A., Lontis, R., Jiang, N., Popović, M., Farina, D., Dremstrup, K., et al. (2014). Movement related cortical potentials and sensory motor rhythms during self initiated and cued movements. Biosyst. Biorobotics 7, 701–707. doi: 10.1007/978-3-319-08072-7_98
Savić, A. M., Lontis, E. R., Mrachacz-Kersting, N., and Popović, M. B. (2020). Dynamics of movement-related cortical potentials and sensorimotor oscillations during palmar grasp movements. Eur. J. Neurosci. 51, 1962–1970. doi: 10.1111/ejn.14629
Savić, A. M., Malešević, N. B., and Popović, M. B. (2013). Motor imagery driven BCI with cue-based selection of FES induced grasps. Biosyst. Biorobotics 1, 513–516. doi: 10.1007/978-3-642-34546-3_82
Shakeel, A., Navid, M. S., Anwar, M. N., Mazhar, S., Jochumsen, M., and Niazi, I. K. (2015). A review of techniques for detection of movement intention using movement-related cortical potentials. Comput. Math. Methods Med. 2015:346217. doi: 10.1155/2015/346217
Shibasaki, H., Barrett, G., Neshige, R., Hirata, I., and Tomoda, H. (1986). Volitional movement is not preceded by cortical slow negativity in cerebellar dentate lesion in man. Brain Res. 368, 361–365. doi: 10.1016/0006-8993(86)90582-2
Shibasaki, H., and Hallett, M. (2006). What is the Bereitschaftspotential? Clin. Neurophysiol. 117, 2341–2356. doi: 10.1016/j.clinph.2006.04.025
Shih, J. J., Krusienski, D. J., and Wolpaw, J. R. (2012). Brain-computer interfaces in medicine. Mayo Clinic Proc. 87, 268–279. doi: 10.1016/j.mayocp.2011.12.008
Wolpaw, J. R., Birbaumer, N., Mcfarland, D. J., Pfurtscheller, G., and Vaughan, T. M. (2002). Brain-computer interfaces for communication and control. Clin. Neurophysiol. 113, 767–791. doi: 10.1016/S1388-2457(02)00057-3
Wright, D. J., Holmes, P. S., Di Russo, F., Loporto, M., and Smith, D. (2012). Differences in cortical activity related to motor planning between experienced guitarists and non-musicians during guitar playing. Hum. Mov. Sci. 31, 567–577. doi: 10.1016/j.humov.2011.07.001
Wright, D. J., Holmes, P. S., and Smith, D. (2011). Using the movement-related cortical potential to study motor skill learning. J. Mot. Behav. 43, 193–201. doi: 10.1080/00222895.2011.557751
Xu, S., Liu, Y., Lee, H., and Li, W. (2024). Neural interfaces: bridging the brain to the world beyond healthcare. Exploration 4:146. doi: 10.1002/EXP.20230146
Keywords: movement-related cortical potentials, brain-computer interface speller, control signal, amyotrophic lateral sclerosis, electroencephalography
Citation: Hernández-Gloria JJ, Jaramillo-Gonzalez A, Savić AM and Mrachacz-Kersting N (2025) Toward brain-computer interface speller with movement-related cortical potentials as control signals. Front. Hum. Neurosci. 19:1539081. doi: 10.3389/fnhum.2025.1539081
Received: 03 December 2024; Accepted: 07 March 2025;
Published: 02 April 2025.
Edited by:
Eduardo Santamaría-Vázquez, University of Valladolid, SpainReviewed by:
Monika Błaszczyszyn, Opole University of Technology, PolandCopyright © 2025 Hernández-Gloria, Jaramillo-Gonzalez, Savić and Mrachacz-Kersting. This is an open-access article distributed under the terms of the Creative Commons Attribution License (CC BY). The use, distribution or reproduction in other forums is permitted, provided the original author(s) and the copyright owner(s) are credited and that the original publication in this journal is cited, in accordance with accepted academic practice. No use, distribution or reproduction is permitted which does not comply with these terms.
*Correspondence: Natalie Mrachacz-Kersting, bmF0YWxpZS5tcmFjaGFjei1rZXJzdGluZ0BzcG9ydC51bmktZnJlaWJ1cmcuZGU=
Disclaimer: All claims expressed in this article are solely those of the authors and do not necessarily represent those of their affiliated organizations, or those of the publisher, the editors and the reviewers. Any product that may be evaluated in this article or claim that may be made by its manufacturer is not guaranteed or endorsed by the publisher.
Research integrity at Frontiers
Learn more about the work of our research integrity team to safeguard the quality of each article we publish.