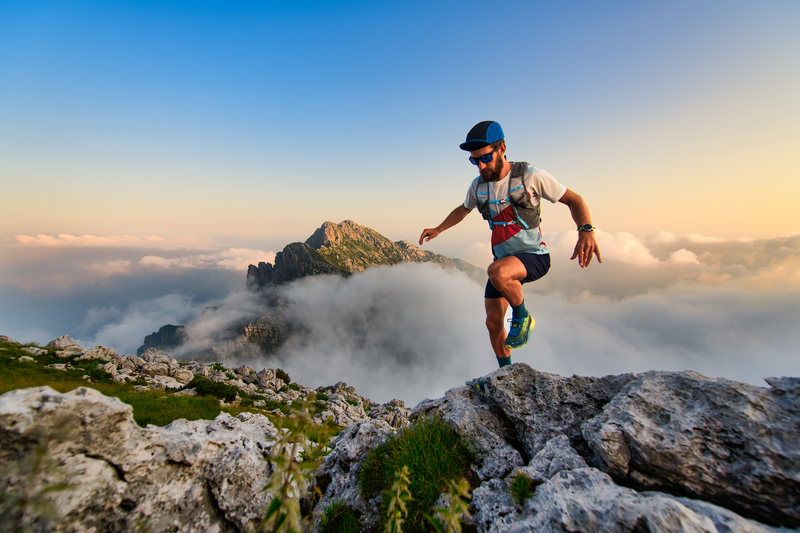
94% of researchers rate our articles as excellent or good
Learn more about the work of our research integrity team to safeguard the quality of each article we publish.
Find out more
HYPOTHESIS AND THEORY article
Front. Hum. Neurosci.
Sec. Motor Neuroscience
Volume 19 - 2025 | doi: 10.3389/fnhum.2025.1525403
This article is part of the Research Topic How cognitive functions interact with the motor system to shape motor behavior View all articles
The final, formatted version of the article will be published soon.
You have multiple emails registered with Frontiers:
Please enter your email address:
If you already have an account, please login
You don't have a Frontiers account ? You can register here
Fibonacci sequences are sequences of numbers whose first two elements are 0, 1 and such that, starting from the third number, every element of the sequence is the sum of the previous two. They are of finite length when the number of elements of the sequence is finite. Furthermore, Fibonacci sequences are named generalized Fibonacci sequences when they are generated by two positive integers that not necessarily equal 0 and 1. By taking inspiration from their use in symmetric walking, generalized Fibonacci sequences of finite length have been very recently adopted to extend the resulting original walking gait characterization to completely automatized gestures in elite swimmers and tennis-players, by accordingly associating the durations of the subphases of the gesture to the elements of such sequences. This can happen within movement-automatization-allowable scenarios, namely, within scenarios in which no external disturbances or additional constraints affect the natural repeatability of movements: at a comfortable speed in walking, at a medium pace in swimming, and under no need of lateral/frontal movements of the entire body in tennis forehand execution or no wind in the serve shot. Now, in such sequences of subphase durations of a physical movement or gesture, the golden ratio has been further found to characterize hidden self-similar patterns, namely patterns in which all the ratios between two consecutive elements of the sequence are surprisingly equal, thus representing a harmonic and mostly aesthetical gesture that admits a perfectly self-similar subphase partition in terms of time-durations. In such a case, the larger-scale structure within the gesture resembles the smaller-scale structure so that the brain can aesthetically resort to the minimum amount of information for the movement temporal design.} In the framework of how cognitive factors like working memory and executive control facilitate motor learning and adaptation, this paper addresses, for the first time in the literature, the open problem of providing a complete mathematical understanding of the automatic generation process at the root of such hidden Fibonacci-sequence-based and self-similar patterns appearing in the aforementioned cyclic human movements. Data referring to walking and tennis-playing are used to illustrate the effectiveness of the proposed approach.
Keywords: Generalized Fibonacci sequence, Symmetry, Automatized Movement, self-similarity, golden ratio, Neuroscience, Physiological systems
Received: 09 Nov 2024; Accepted: 10 Mar 2025.
Copyright: © 2025 Verrelli, Caprioli and Iosa. This is an open-access article distributed under the terms of the Creative Commons Attribution License (CC BY). The use, distribution or reproduction in other forums is permitted, provided the original author(s) or licensor are credited and that the original publication in this journal is cited, in accordance with accepted academic practice. No use, distribution or reproduction is permitted which does not comply with these terms.
* Correspondence:
Cristiano Maria Verrelli, Department of Electronic Engineering, University of Rome Tor Vergata, Roma, Italy
Disclaimer: All claims expressed in this article are solely those of the authors and do not necessarily represent those of their affiliated organizations, or those of the publisher, the editors and the reviewers. Any product that may be evaluated in this article or claim that may be made by its manufacturer is not guaranteed or endorsed by the publisher.
Research integrity at Frontiers
Learn more about the work of our research integrity team to safeguard the quality of each article we publish.