- 1Rehabilitation Medicine College, Henan University of Chinese Medicine, Zhengzhou, China
- 2School of Traditional Chinese Medicine, Henan University of Chinese Medicine, Zhengzhou, China
- 3School of Exercise and Health, Shanghai University of Sport, Shanghai, China
Introduction: Several clinical studies have demonstrated that brain-computer interfaces (BCIs) controlled functional electrical stimulation (FES) facilitate neurological recovery in patients with stroke. This review aims to evaluate the effectiveness of BCI-FES training on upper limb functional recovery in stroke patients.
Methods: PubMed, Embase, Cochrane Library, Science Direct and Web of Science were systematically searched from inception to October 2023. Randomized controlled trials (RCTs) employing BCI-FES training were included. The methodological quality of the RCTs was assessed using the PEDro scale. Meta-analysis was conducted using RevMan 5.4.1 and STATA 18.
Results: The meta-analysis comprised 290 patients from 10 RCTs. Results showed a moderate effect size in upper limb function recovery through BCI-FES training (SMD = 0.50, 95% CI: 0.26–0.73, I2 = 0%, p < 0.0001). Subgroup analysis revealed that BCI-FES training significantly enhanced upper limb motor function in BCI-FES vs. FES group (SMD = 0.37, 95% CI: 0.00–0.74, I2 = 21%, p = 0.05), and the BCI-FES + CR vs. CR group (SMD = 0.61, 95% CI: 0.28–0.95, I2 = 0%, p = 0.0003). Moreover, BCI-FES training demonstrated effectiveness in both subacute (SMD = 0.56, 95% CI: 0.25–0.87, I2 = 0%, p = 0.0004) and chronic groups (SMD = 0.42, 95% CI: 0.05–0.78, I2 = 45%, p = 0.02). Subgroup analysis showed that both adjusting (SMD = 0.55, 95% CI: 0.24–0.87, I2 = 0%, p = 0.0006) and fixing (SMD = 0.43, 95% CI: 0.07–0.78, I2 = 46%, p = 0.02). BCI thresholds before training significantly improved motor function in stroke patients. Both motor imagery (MI) (SMD = 0.41 95% CI: 0.12–0.71, I2 = 13%, p = 0.006) and action observation (AO) (SMD = 0.73, 95% CI: 0.26–1.20, I2 = 0%, p = 0.002) as mental tasks significantly improved upper limb function in stroke patients.
Discussion: BCI-FES has significant immediate effects on upper limb function in subacute and chronic stroke patients, but evidence for its long-term impact remains limited. Using AO as the mental task may be a more effective BCI-FES training strategy.
Systematic review registration: Identifier: CRD42023485744, https://www.crd.york.ac.uk/prospero/display_record.php?ID=CRD42023485744.
1 Introduction
Over the past three decades, there has been a significant increase in stroke incidence and mortality rates (GBD 2019 Stroke Collaborators, 2021). Approximately 80% of stroke patients experience upper limb dysfunction, greatly impacting daily activities and limiting social participation (Zhang et al., 2020; Feigin, 2008). Upper limb recovery is a fundamental aspect of stroke rehabilitation and is crucial for mitigating disability (Pollock et al., 2014). Technological advancements in neuroscience research have expanded rehabilitation options. Brain-computer interfaces (BCIs), transcranial magnetic stimulation, and robotic training have emerged as potential approaches for post-stroke rehabilitation (Vink et al., 2023; Zhang et al., 2022; Takebayashi et al., 2022; Frisoli et al., 2022; Guo et al., 2022; Caria et al., 2020). Numerous studies have confirmed that BCI-based training significantly improves upper limb motor function in stroke patients and the combination of BCI with functional electrical stimulation (BCI-FES) may be more effective (Nojima et al., 2022; Peng et al., 2022; Bai et al., 2022).
The BCI-FES system typically consists of a BCI unit, a BCI-FES interface assembly and an FES module (Khan et al., 2023). Pre-programmed therapeutic sessions are tailored to target specific movements for stroke patients, and researchers will adjust the BCI threshold based on patient performance. Within these sessions, patients are instructed to engage in motor imagery (MI) or action observation (AO), both of which effectively induce Electroencephalogram (EEG) changes. Subsequently, through training and decoding algorithms, these EEG signals are matched to specific imagined or observed movements, enabling control of the FES. Finally, the FES device delivers electrical stimulation to the affected muscles to perform the desired movement. Compared to other BCI training systems, BCI-FES directly stimulates muscle movement through the FES, which simultaneously provides proprioceptive feedback. This sensory input plays a vital role in neurorehabilitation by promoting neural reorganization (Remsik et al., 2022).
In 2009, Daly et al. first demonstrated the feasibility of BCI-FES for treating upper limb motor impairments in stroke patients (Daly et al., 2009). Since then, numerous innovations have broadened the applicability and effectiveness of BCI-FES systems in stroke rehabilitation. Rehtian-Romagosa and colleagues designed a recoveriX system, which integrates BCI-FES with virtual reality (Sebastián-Romagosa et al., 2020). This integration highlights the potential for combining BCI-FES with innovative technologies to further improve rehabilitation outcomes. Zhang et al. developed an adaptive BCI-FES system that dynamically adjusts task difficulty based on stroke severity, demonstrating the promise of personalized treatment strategies (Zhang et al., 2023). In future research, it may be feasible to customize specific BCI-FES systems based on the different stages and severities of stroke. Personalized services can be provided by understanding individual needs and capabilities.
Although BCI-FES training shows promise for enhancing motor function recovery in stroke patients, its widespread clinical application lacks substantial evidence (Simon et al., 2021). Variations in BCI-FES training strategies across studies, such as mental tasks, BCI threshold adjustments and intervention duration, have posed challenges in establishing its clinical efficacy. Therefore, this study aims to assess the immediate efficacy of BCI-FES training in improving upper limb recovery in stroke patients and to explore the impact of adjusting the BCI threshold and employing different mental tasks on its effectiveness. Additionally, the study used meta-regression analysis to explore factors that may influence effect size. The findings can provide valuable insights for refining treatment strategies.
2 Materials and methods
We followed the PRISMA guidelines for reporting systematic literature review and meta-analysis (Moher et al., 2009). The protocol has been registered on PROSPERO (CRD42023485744).
2.1 Data sources and search strategy
A comprehensive literature search of PubMed, Embase, Cochrane Library, Science Direct, and Web of Science was conducted from inception through October 2023. The keywords included “brain-computer interface OR brain-machine interface OR BCI OR BMI” and “stroke OR cerebral infarction OR cerebral hemorrhage OR cerebral vascular accident,” Additionally, we conducted a manual search by screening the reference lists of previous systematic reviews and meta-analysis to identify additional relevant articles for inclusion in our analysis.
2.2 Inclusion and exclusion criteria
The systematic review focused on studies in English, using the PICOS framework to set the inclusion criteria:
Participants: Adults (≥18 years) who had experienced a stroke, regardless of clinical variables like gender, stroke severity, nationality, or education level.
Intervention: The experimental group received BCI-FES training.
Comparison: The control group received sham BCI-FES training, conventional rehabilitation, or no intervention.
Outcomes: At least one outcome measure related to upper limb motor function was reported.
Study Design: Randomized controlled trials (RCTs).
Exclusion criteria comprised: (1) Duplicate studies. (2) Non-RCTs. (3) Used BCI-FES training in both intervention and control groups. (4) Studies with unavailable full texts or missing relevant outcome data.
2.3 Data extraction
Two reviewers independently evaluated the titles and abstracts of the retrieved articles, followed by assessment of the full-text articles. Data from these articles were then validated and extracted based on predetermined criteria, including study characteristics, participant profiles, interventions, control interventions, outcome measures, intervention dosages, BCI threshold adjustment, and types of mental tasks. Post-intervention assessments were collected as parameters for analysis of the immediate clinical effects.
2.4 Quality assessment
Two independent evaluators conducted a comprehensive assessment of the methodological quality of the controlled studies using the Physiotherapy Evidence Database (PEDro) scale (Moseley et al., 2002). To ensure consistency, any discrepancies between the evaluators were resolved through discussion and, when necessary, consultation with a third independent reviewer. After a thorough examination of the studies, the evaluators reached a unanimous decision. The PEDro scale comprises 11 criteria, which encompass various factors, including randomization, blinding, dropout rates, intention to treat, allocation concealment, and data reporting. These criteria are used to assess potential bias in clinical trials. Each of the remaining 10 criteria is awarded one point if met by the study except for the first criterion. The cumulative score is determined by summing these points. Studies scoring 9–10 on the PEDro scale are categorized as “excellent” quality, 6–8 as “good” quality, 4–5 as “fair” quality, and those scoring below 4 are deemed “poor” quality (Foley et al., 2003). We performed the quality assessment strictly according to the PEDro assessment guidelines.1
2.5 Data analysis
The Fugl-Meyer Motor Function of Upper Extremity Scale (FMA-UE) is a widely recognized stroke-specific impairment index with excellent properties (Hernández et al., 2019). However, one study employed the Manual Function Test as the primary outcome measure (Jang et al., 2016). To include this measure in our meta-analysis, we utilized the standardized mean difference (SMD) and 95% confidence interval (CI) as the pooled effect size estimators. The effect size was determined from the mean post-treatment scores of the experimental and control groups, along with a 95% confidence interval. Statistical significance was defined as p-values <0.05. Effect sizes were classified as large (SMD = 0.8), medium (SMD = 0.5) and small (SMD = 0.2). In instances where mean or standard deviation data were absent in the selected paper, we either contacted the corresponding author or computed them using available data. Heterogeneity was evaluated using the I2 statistic, with I2 ≥ 50% indicating significant heterogeneity. If I2 was less than 50%, the fixed effects model was applied; otherwise, the random effects model was used (Borenstein et al., 2010). We conducted sensitivity analysis using two approaches to assess the robustness of our meta-analysis: by including only high-quality studies, and by sequentially removing one study. Egger’s test was used to quantify publication bias. Subgroup analysis was performed to investigate the impact of variables such as the stroke stage, BCI threshold adjustment, and different mental tasks. Univariate meta-regression analysis was performed to explore associations between baseline patient age, session duration, cumulative training time and effect size. All data analysis were executed using RevMan 5.4.1 and STATA 18.
3 Results
3.1 Screening process and results of studies
After retrieving 6,360 articles from 5 database and eliminating 953 duplicates, 5,407 records underwent screening based on title and author names. Finally, 10 articles meeting the selection criteria were included in this meta-analysis. The screening process is depicted in Figure 1.
3.2 Characteristics of the included studies
The characteristics of RCTs are summarized in Table 1. The meta-analysis involved 290 patients from 10 studies. Among these, 5 studies focused on chronic patients (Biasiucci et al., 2018; Miao et al., 2020; Lee et al., 2020; Kim et al., 2015; Zhan et al., 2022), 5 on subacute patients (Zhang et al., 2023; Jang et al., 2016; Li et al., 2014, Liu et al., 2023, Liao et al., 2023). In 5 of the 10 studies, researchers adjusted the BCI threshold before each treatment session (Biasiucci et al., 2018; Zhang et al., 2023; Liu et al., 2023; Lee et al., 2020; Li et al., 2014), while in the remaining 5 studies, the BCI threshold was fixed (Jang et al., 2016; Miao et al., 2020; Kim et al., 2015, Zhan et al., 2022, Liao et al., 2023). Six studies utilized MI (Jang et al., 2016; Liu et al., 2023; Miao et al., 2020; Li et al., 2014, Zhan et al., 2022, Liao et al., 2023), 3 used AO (Jang et al., 2016; Lee et al., 2020; Kim et al., 2015), and 1 employed motor attempt (Biasiucci et al., 2018) as mental tasks in BCI-FES training. Treatment sessions ranged from 20 to 60 min, with total treatment duration varying from 4 to 24 h. Among the 10 studies included, 2 were rated as excellent (Biasiucci et al., 2018; Zhang et al., 2023), 4 as good (Liu et al., 2023; Lee et al., 2020; Kim et al., 2015; Li et al., 2014, Liao et al., 2023), and 3 as fair in quality (Jang et al., 2016; Miao et al., 2020; Zhan et al., 2022). The PEDro scores ranged from 5 to 10, with an average score of 7.00 (1.76). The methodological quality of the included studies is detailed in Table 2.
3.3 The effects of BCI-FES training on upper limb function
Across the 10 studies, 146 patients were allocated to the BCI-FES group, while 144 patients were assigned to the control group. Pooled results indicated that BCI-FES training (SMD = 0.50, 95% CI: 0.26–0.73, I2 = 0%, p < 0.0001) significantly enhanced upper limb motor function compared to the control intervention (Figure 2). No evidence of publication bias was observed according to the Egger’s test (β = −0.625; se = 1.529; p = 0.693). In the sensitivity analysis, we sequentially removed each study and reassessed the pooled results. It was observed that both heterogeneity and significance varied only within a narrow range. When only studies of good methodological quality or higher (PEDro score > 5) were included, the effect of BCI-FES training on upper limb motor function increased (SMD = 0.64, 95% CI: 0.37–0.90, I2 = 0%, p < 0.0001) (Figure 3). The Egger’s test also showed no evidence of publication bias (β = 1.146, se = 0.936, p = 0.276).
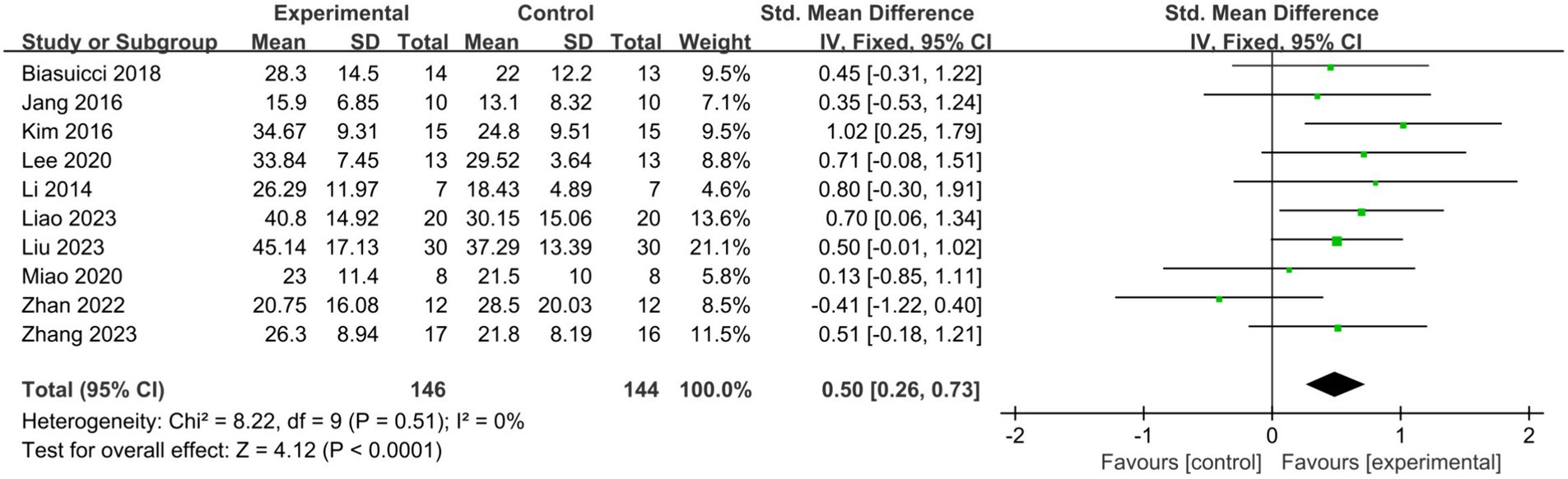
Figure 2. Comparison of the effects of immediate effects of the BCI-FES group and control group on the upper limb recovery in stroke patients.
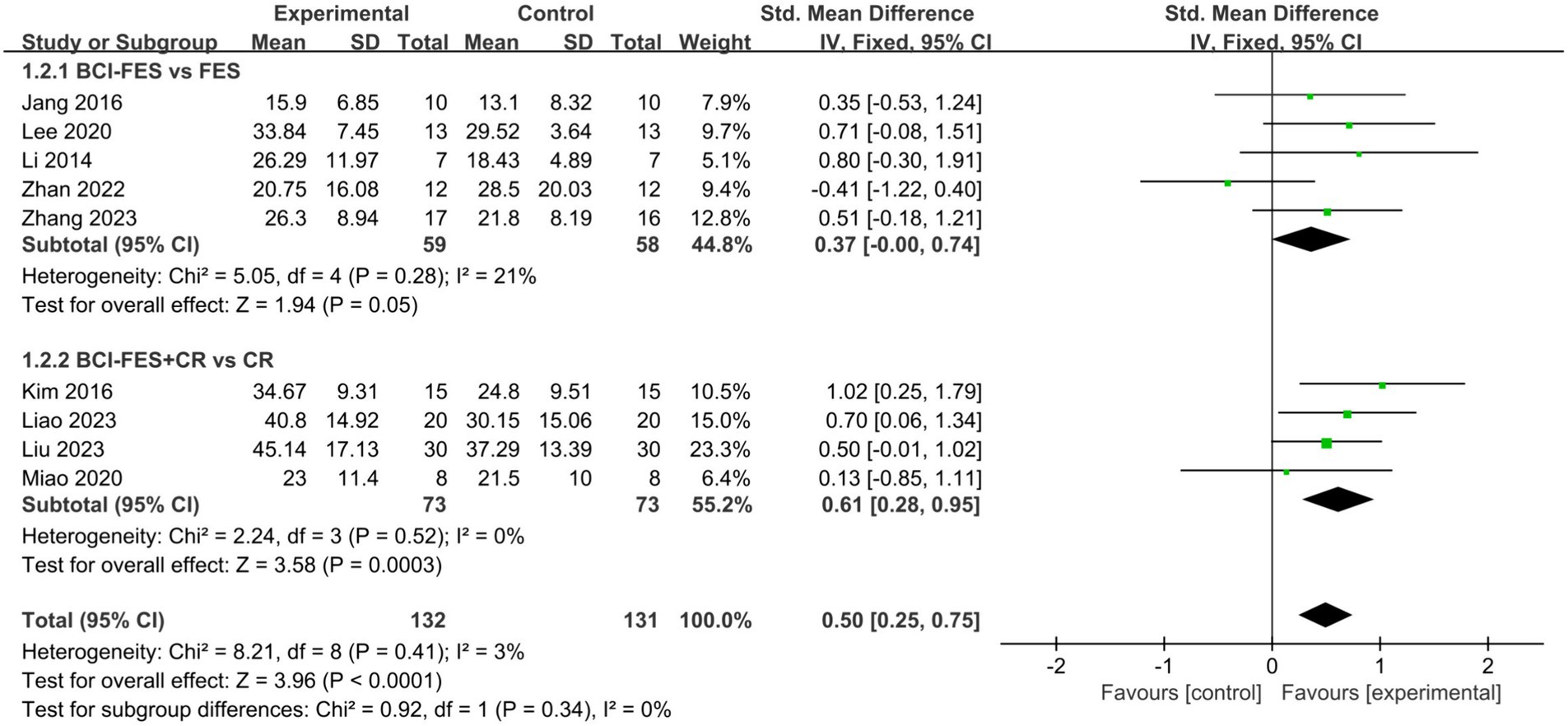
Figure 3. Comparison of the effects of BCI-FES vs. FES and BCI-FES + CR vs. CR on upper Limb recovery in stroke patients.
3.4 Subgroup analysis
3.4.1 Different rehabilitation groups
To explore the effects of BCI-FES interventions across different control groups, we categorized the studies into two subgroups: BCI-FES vs. FES and BCI-FES + CR vs. CR. Subgroup analysis revealed that BCI-FES training significantly enhanced upper limb motor function in BCI-FES vs. FES group (SMD = 0.37, 95% CI: 0.00–0.74, I2 = 21%, p = 0.05), and the BCI-FES + CR vs. CR group (SMD = 0.61, 95% CI: 0.28–0.95, I2 = 0%, p = 0.0003) (Figure 3). The difference between the two subgroups was not statistically significant (p = 0.34). When only studies of good methodological quality and higher were included, both groups remained significant with increased effect sizes: BCI-FES vs. FES group (SMD = 0.64, 95% CI: 0.16–1.11, I2 = 0%, p = 0.008) and the BCI-FES + CR vs. CR group (SMD = 0.67, 95% CI: 0.32–1.03, I2 = 0%, p = 0.0002). There was no statistically significant difference between the two groups (p = 0.90).
3.4.2 Stroke stages
To explore variations in the effectiveness of BCI-FES training among patients at different stages of stroke. Patients within 6 months post-stroke were classified as subacute, while those beyond 6 months were classified as chronic. Subgroup analysis revealed that BCI-FES training significantly enhanced upper limb motor function in both the chronic group (SMD = 0.42, 95% CI: 0.05–0.78, I2 = 45%, p = 0.02) and the subacute group (SMD = 0.56, 95% CI: 0.25–0.87, I2 = 0%, p = 0.0004), with no statistically significant difference between the two groups (p = 0.57) (Figure 4). When only studies of good methodological quality and higher were included, both groups remained significant with increased effect sizes: the chronic group (SMD = 0.73, 95% CI: 0.28–1.18, I2 = 0%, p = 0.001) and the subacute group (SMD = 0.58, 95% CI: 0.25–0.92, I2 = 0%, p = 0.0006). There was no statistically significant difference between the two groups (p = 0.61).
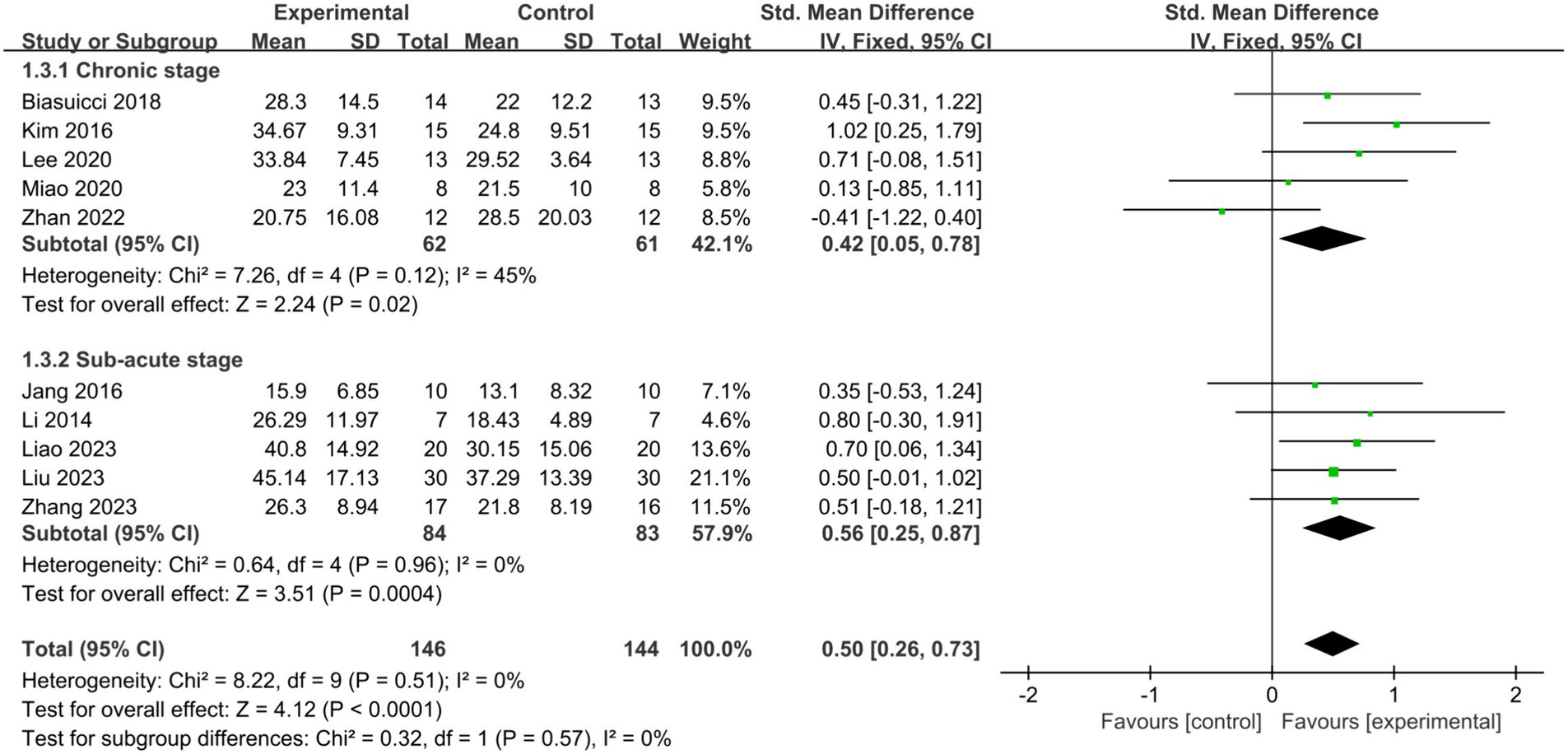
Figure 4. Comparison of the effects of BCI-FES training among patients in different stages of stroke.
3.4.3 BCI threshold adjustment or fixation
Among the 10 studies included in our analysis, 5 adjusted the BCI threshold before each treatment session, while the remaining 5 maintained a fixed threshold. Subgroup analyses showed that both adjusting the BCI threshold before training (SMD = 0.55, 95% CI: 0.24–0.87, I2 = 0%, p = 0.0006) and maintaining a fixed BCI threshold (SMD = 0.43, 95% CI: 0.07–0.78, I2 = 46%, p = 0.02) significantly improved upper limb motor function in stroke patients. There was no statistically significant difference between the two approaches (p = 0.59) (Figure 5). All three studies of fair quality were in the group that maintained a fixed BCI threshold. When only studies of good methodological quality and higher were included, the fixed BCI threshold group remained significant, with an increased effect size (SMD = 0.83, 95% CI: 0.34–1.32, I2 = 0%, p = 0.0009). There was no statistically significant difference between the two approaches (p = 0.36).
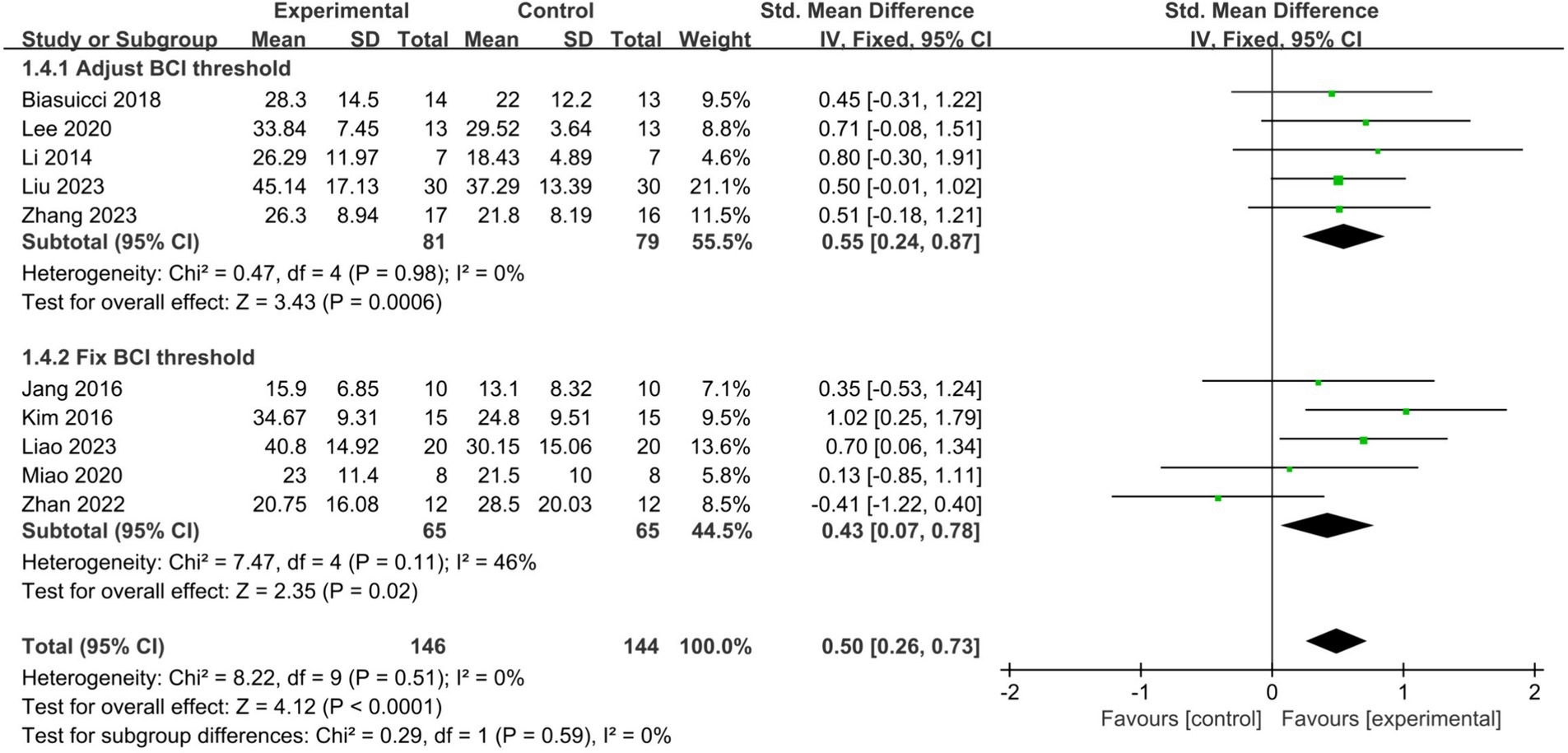
Figure 5. Comparison of the impact of adjusting the threshold before each treatment session or fixing it on upper limb functional recovery in stroke patients.
3.4.4 Different mental tasks
MI and AO represent the two primary mental tasks utilized in BCI-FES training. Subgroup analysis demonstrated that employing MI (SMD = 0.41, 95% CI: 0.12–0.71, I2 = 13%, p = 0.006) or AO (SMD = 0.73, 95% CI: 0.26–1.20, I2 = 0%, p = 0.002) significantly improved upper limb motor function in stroke patients, with no statistically significant difference observed between the two methods (p = 0.27) (Figure 6). When only studies of good methodological quality and higher were included, both groups remained significant with increased effect sizes: the MI group (SMD = 0.54, 95% CI: 0.16–0.93, I2 = 0%, p = 0.006) and the AO group (SMD = 0.87, 95% CI: 0.32–1.43, I2 = 0%, p = 0.002). There was no statistically significant difference between the two tasks (p = 0.34).
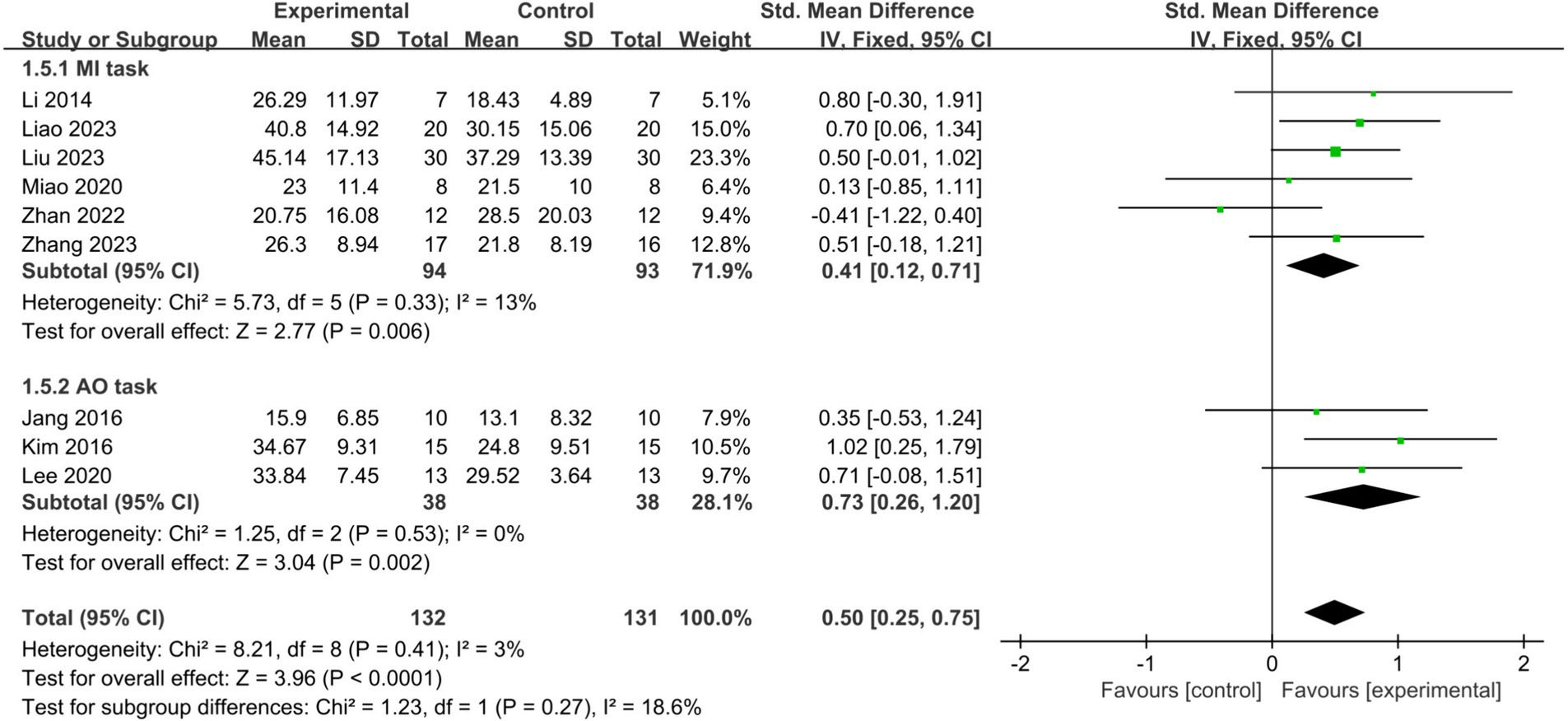
Figure 6. Comparison of the effects of the MI tasks group and AO tasks group on the upper limb in stroke patients.
3.5 Meta-regression
To assess the potential influence of other clinical factors, we performed meta-regression analysis on baseline patient age and the duration of interventions. Single-factor analysis revealed that baseline patient age (coefficient = 0.0159, se = 0.1822, p = 0.384), session duration (coefficient = 0.0026, se = 0.0075, p = 0.733), and cumulative training time (coefficient = 0.0004, se = 0.0004, p = 0.271) were not significant predictors of effect size.
4 Discussion
This study aims to evaluate the immediate effects of BCI-FES training on upper limb recovery in stroke patients and to analyze the impact of BCI threshold adjustments and different mental tasks on its effectiveness. Additionally, meta-regression analysis was performed to identify factors that may influence the overall effect size. These findings may provide valuable insights for optimizing BCI-FES treatment strategies in stroke rehabilitation.
The quality of the included studies was assessed using the PEDro scale, a validated tool widely employed in rehabilitation and physical therapy research to evaluate the methodological rigor of randomized controlled trials. The 10 studies included had an average score of 7.00 (1.76), indicating generally good methodological quality. The meta-analysis of 10 studies demonstrated that BCI-FES training had a favorable medium effect on upper limb function (SMD = 0.50). Heterogeneity analysis and sensitivity analysis confirmed the robustness of the result. When restricting the meta analysis to studies with good methodological quality and higher, the effect of BCI-FES training increased (SMD = 0.64). No heterogeneity was obversed after excluding studies of fair quality (I2 = 0) and Egger’s test showed no evidence of publication bias (p = 0.276). This suggested that the 3 fair-quality studies slightly underestimated the effect size. Similarly, in all subgroup analysis, limiting the analysis to good quality and higher studies consistently showed that BCI-FES remained effective in improving upper limb motor function, with increased effect sizes. In conclusion, although variations in study quality have influence on the effect sizes, they do not significantly affect the overall assessment of BCI-FES effectiveness in improving upper limb motor function in stroke patients.
The adjustment of the BCI threshold aims to improve the accuracy of mental tasks, which has been shown to be associated with better functional recovery (Myrden and Chau, 2015). Among the 10 studies included, nearly all studies with good and higher quality implemented BCI threshold adjustments. This suggests that researchers recognized the link between BCI threshold adjustments and improved clinical outcomes. However, subgroup analysis revealed that no statistically significant difference between the two approaches. Further studies are required to validate these findings and to clarify the specific role of BCI threshold adjustments in enhancing upper extremity functional recovery in stroke patients.
AO and MI serve as primary mental tasks capable of eliciting EEG signal alterations during BCI-FES training. The subgroup analysis demonstrated that both AO and MI, when used as mental tasks during BCI-FES training, significantly enhance upper limb motor function in stroke patients. However, AO appears to yield greater improvements in upper limb function compared to MI. AO involves activating the brain’s motor areas by observing specific movements performed by others. Based on mirror neuron theory, observing such movements can trigger the activation of the neural network associated with them (Garrison et al., 2013). Conversely, MI entails mentally simulating movements to activate the brain’s motor areas without physical execution (Jackson et al., 2001). Neuroimaging studies have extensively explored the neural substrates of MI, revealing significant overlap with the neural network responsible for motor execution (Hétu et al., 2013). However, approximately 20–30% of stroke patients, due to impaired sensory-motor areas or advanced age, fail to produce Event-Related Desynchronization through MI (Tani et al., 2018; Ahn, 2013). Research by Allison and Neuper reported that even in healthy individuals, around 15–30% fail to produce distinct ERD (Allison and Neuper, 2010). Furthermore, studies by Kübler et al. have shown that despite several months of MI training, performance improvements for certain participants remain limited (Kübler et al., 1999). In contrast to MI, AO does not necessitate initial skill proficiency; even passive observation by individuals with limited proficiency can activate the brain’s motor areas (Binks et al., 2023). MI may be suboptimal for stroke patients learning complex movements beyond their repertoire. Further research is warranted to directly compare the roles of these two mental tasks in BCI-FES training.
In the meta-regression analysis, baseline age was not identified as a significant predictor of effect size. This suggests that BCI-FES provides similar benefits across different age groups. Session duration and cumulative training time were also not significant predictors of effect size. These findings are similar to previous studies (Nojima et al., 2022; Bai et al., 2022). We speculate that the effectiveness of BCI-FES training may rely more on the accuracy of mental task. As reported by Miao et al., stroke patients with the highest mental task accuracy achieved the greatest improvements in upper limb function (Miao et al., 2020).
Based on previous studies, two hypotheses were proposed regarding the mechanisms by which BCI-FES training facilitates the recovery of motor function after stroke: enhancing the excitability of the ipsilateral M1 and rectifying interhemispheric imbalance. Numerous studies have shown that BCI-FES training not only significantly enhances connections between neurons in existing neural pathways but also fosters the formation of new neural connections. These developments facilitate the activation of both the motor cortex and PM in the lesioned hemisphere (Min et al., 2020). Functionally, BCI-FES training induces noteworthy changes in cortical activation patterns. Initially, there is substantial activation observed in the contralateral motor cortex post-stroke. As recovery progresses, activation in the motor cortex of the lesioned hemisphere gradually intensifies, signifying the process of rectifying hemispheric imbalance (Ramos-Murguialday et al., 2013).
Increased excitability within the ipsilesional motor cortex is considered symbol of motor rehabilitation in the affected upper limb. Studies by Biasiucci et al. and Zhang et al. observed heightened connectivity between the ipsilesional primary M1 and PM following training (Biasiucci et al., 2018; Zhang et al., 2023). Additionally, these studies indicated varied μ, α, and beta rhythm activity across brain regions, with notable activation of μ and beta rhythms in the M1 (Zhang et al., 2023; Liu et al., 2023; Lee et al., 2020). Numerous pre-and post-single-group design studies with healthy subjects have also demonstrated that BCI significantly activates the prefrontal cortex, PMC, and posterior parietal cortex. This increased connectivity and rhythmic activity in ipsilesional areas hold significant implications for motor rehabilitation (Zhang et al., 2014; Nicolo et al., 2015; Schulz, 2012). In summary, BCI-FES training may enhance connectivity along existing neural pathways, facilitate the formation of new neural connections, and promote Hebbian-like plasticity through synchronized activation of cerebral motor areas and peripheral effectors (Behboodi et al., 2022).
Another mechanism involves addressing interhemispheric imbalances. Miao et al. and Li et al. conducted a study examining changes in patients’ brain topography before and after BCI-FES training. Their findings suggested that during BCI-FES training, cortical activation initially spreads across broad areas before concentrating in motor or adjacent cortical areas in the lesioned hemisphere (Miao et al., 2020; Li et al., 2014). Initially, due to significant brain function impairment, there was a notable increase in activation in motor areas bilaterally, particularly in the non-lesioned hemisphere, which is crucial for stroke recovery (Serrien et al., 2004; Rehme et al., 2011; Ward et al., 2003). However, sustained activation of the contralateral prefrontal and parietal cortex may indicate slower and incomplete recovery (Murphy and Corbett, 2009). Over time, activation in the non-lesioned hemisphere diminishes, and neural activation gradually shifts back to the diseased hemisphere, suggesting the correction of interhemispheric imbalances (Park et al., 2011; Markram et al., 2011). However, a review has highlighted the lack of compelling evidence to support the existence of interhemispheric inhibitory imbalance (McDonnell and Stinear, 2017). Caution is warranted when considering the repair of interhemispheric imbalance as a mechanism for BCI-FES training to promote stroke rehabilitation.
5 Limitations
The limitation of this study is the small sample size, which may affect the reliability of the results. Although we employed various methods to explore heterogeneity, differences in study methodologies could still have a potential impact on the findings. Additionally, previous clinical trials have rarely addressed the long-term follow-up effects of BCI-FES training. Future research should focus on increasing sample sizes and conducting extended follow-up to more accurately assess the clinical value of BCI-FES training.
6 Conclusion
BCI-FES training has a significant immediate impact on enhancing upper limb function in both subacute and chronic stroke patients. Adjusting the threshold before each BCI-FES session did not result in significantly better upper limb motor function recovery compared to fixing the threshold. Using the AO as mental task may be a better BCI-FES training strategy. However, future larger trials are needed to validate these results and explore the long-term sustained effects of BCI-FES.
Data availability statement
The original contributions presented in the study are included in the article/supplementary material, further inquiries can be directed to the corresponding author.
Author contributions
CR: Writing – original draft, Conceptualization, Methodology. XL: Writing – original draft. QG: Methodology, Writing – original draft. MP: Writing – original draft. JW: Writing – original draft. FY: Writing – original draft. ZD: Writing – original draft. PG: Writing – original draft. YZ: Writing – original draft.
Funding
The author(s) declare that financial support was received for the research, authorship, and/or publication of this article. This work was funded by 2023 Henan Special Research Project of TCM on “Double First-Class” Construction (No. HSRP-DFCTCM-2023-1-21), the Science and Technology Project of Henan Province (No. 232102310466), and Henan University of Chinese Medicine 2024 Education and Teaching Reform Research and Practice Projects (No. 2024JX62).
Acknowledgments
We extend our gratitude to the authors of the research studies included in this systematic review for their invaluable support and contributions.
Conflict of interest
The authors declare that the research was conducted in the absence of any commercial or financial relationships that could be construed as a potential conflict of interest.
Publisher’s note
All claims expressed in this article are solely those of the authors and do not necessarily represent those of their affiliated organizations, or those of the publisher, the editors and the reviewers. Any product that may be evaluated in this article, or claim that may be made by its manufacturer, is not guaranteed or endorsed by the publisher.
Footnotes
References
Ahn, M. (2013). Gamma band activity associated with BCI performance: simultaneous MEG/EEG study. Front. Hum. Neurosci. 7:848. doi: 10.3389/fnhum.2013.00848
Allison, B. Z., and Neuper, C. (2010). Could anyone use a BCI? Brain-Comput. Interfaces 58, 35–54. doi: 10.1007/978-1-84996-272-8_3
Bai, Z., Fong, K. N. K., Zhang, J. J., Chan, J., and Ting, K. H. (2022). Immediate and long-term effects of BCI-based rehabilitation of the upper extremity after stroke: a systematic review and meta-analysis. J. Neuroeng. Rehabil. 17:57. doi: 10.1186/s12984-020-00686-2
Behboodi, A., Lee, W. A., Hinchberger, V. S., and Damiano, D. L. (2022). Determining optimal mobile neurofeedback methods for motor neurorehabilitation in children and adults with non-progressive neurological disorders: a scoping review. J. Neuroeng. Rehabil. 19:104. doi: 10.1186/s12984-022-01081-9
Biasiucci, A., Leeb, R., Iturrate, I., Perdikis, S., al-Khodairy, A., Corbet, T., et al. (2018). Brain-actuated functional electrical stimulation elicits lasting arm motor recovery after stroke. Nat. Commun. 9:2421. doi: 10.1038/s41467-018-04673-z
Binks, J. A., Emerson, J. R., Scott, M. W., Wilson, C., van Schaik, P., and Eaves, D. L. (2023). Enhancing upper-limb neurorehabilitation in chronic stroke survivors using combined action observation and motor imagery therapy. Front. Neurol. 14:1097422. doi: 10.3389/fneur.2023.1097422
Borenstein, M., Hedges, L. V., Higgins, J. P. T., and Rothstein, H. R. (2010). A basic introduction to fixed and random effects models for meta-analysis. Res. Synth. Methods 1, 97–111. doi: 10.1002/jrsm.12
Caria, A., da Rocha, J. L. D., Gallitto, G., Birbaumer, N., Sitaram, R., and Murguialday, A. R. (2020). Brain-machine interface induced morpho-functional remodeling of the neural motor system in severe chronic stroke. Neurotherapeutics 17, 635–650. doi: 10.1007/s13311-019-00816-2
Daly, J. J., Cheng, R., Rogers, J., Litinas, K., Hrovat, K., and Dohring, M. (2009). Feasibility of a new application of noninvasive brain computer Interface (BCI): a case study of training for recovery of volitional motor control after stroke. J. Neurol. Phys. Ther. 33, 203–211. doi: 10.1097/NPT.0b013e3181c1fc0b
Foley, N. C., Teasell, R. W., Bhogal, S. K., and Speechley, M. R. (2003). Stroke rehabilitation evidence-based review: methodology. Top. Stroke Rehabil. 10, 1–7. doi: 10.1310/Y6TG-1KQ9-LEDQ-64L8
Frisoli, A., Barsotti, M., Sotgiu, E., Lamola, G., Procopio, C., and Chisari, C. (2022). A randomized clinical control study on the efficacy of three-dimensional upper limb robotic exoskeleton training in chronic stroke. J. Neuroeng. Rehabil. 19:14. doi: 10.1186/s12984-022-00991-y
Garrison, K. A., Aziz-Zadeh, L., Wong, S. W., Liew, S. L., and Winstein, C. J. (2013). Modulating the motor system by action observation after stroke. Stroke 44, 2247–2253. doi: 10.1161/STROKEAHA.113.001105
GBD 2019 Stroke Collaborators (2021). Global, regional, and national burden of stroke and its risk factors, 1990-2019: a systematic analysis for the global burden of disease study 2019. Lancet Neurol. 20, 795–820. doi: 10.1016/S1474-4422(21)00252-0
Guo, N., Wang, X., Duanmu, D., Huang, X., Li, X., Fan, Y., et al. (2022). SSVEP-based brain computer Interface controlled soft robotic glove for post-stroke hand function rehabilitation. IEEE Trans. Neural Syst. Rehabil. Eng. 30, 1737–1744. doi: 10.1109/TNSRE.2022.3185262
Hernández, E. D., Galeano, C. P., Barbosa, N. E., Forero, S. M., Nordin, Å., Sunnerhagen, K. S., et al. (2019). Intra-and inter-rater reliability of Fugl-Meyer assessment of upper extremity in stroke. J. Rehabil. Med. 51, 652–659. doi: 10.2340/16501977-2590
Hétu, S., Grégoire, M., Saimpont, A., Coll, M. P., Eugène, F., Michon, P. E., et al. (2013). The neural network of motor imagery: an ALE meta-analysis. Neurosci. Biobehav. Rev. 37, 930–949. doi: 10.1016/j.neubiorev.2013.03.017
Jackson, P. L., Lafleur, M. F., Malouin, F., Richards, C., and Doyon, J. (2001). Potential role of mental practice using motor imagery in neurologic rehabilitation. Arch. Phys. Med. Rehabil. 82, 1133–1141. doi: 10.1053/apmr.2001.24286
Jang, Y. Y., Kim, T. H., and Lee, B. H. (2016). Effects of brain-computer Interface-controlled functional electrical stimulation training on shoulder subluxation for patients with stroke: a randomized controlled trial. Occup. Ther. Int. 23, 175–185. doi: 10.1002/oti.1422
Khan, M. A., Fares, H., Ghayvat, H., Brunner, I. C., Puthusserypady, S., Razavi, B., et al. (2023). A systematic review on functional electrical stimulation based rehabilitation systems for upper limb post-stroke recovery. Front. Neurol. 14:1272992. doi: 10.3389/fneur.2023.1272992
Kim, T., Kim, S., and Lee, B. (2015). Effects of action observational training plus brain-computer Interface-based functional electrical stimulation on paretic arm motor recovery in patient with stroke: a randomized controlled trial. Occup. Ther. Int. 23, 39–47. doi: 10.1002/oti.1403
Kübler, A., Kotchoubey, B., Hinterberger, T., Ghanayim, N., Perelmouter, J., Schauer, M., et al. (1999). The thought translation device: a neurophysiological approach to communication in total motor paralysis. Exp. Brain Res. 124, 223–232. doi: 10.1007/s002210050617
Lee, S. H., Kim, S. S., and Lee, B. H. (2020). Action observation training and brain-computer interface controlled functional electrical stimulation enhance upper extremity performance and cortical activation in patients with stroke: a randomized controlled trial. Physiother. Theory Pract. 38, 1126–1134. doi: 10.1080/09593985.2020.1831114
Li, M., Liu, Y., Wu, Y., Liu, S., Jia, J., and Zhang, L. (2014). Neurophysiological substrates of stroke patients with motor imagery-based brain-computer interface training. Int. J. Neurosci. 124, 403–415. doi: 10.3109/00207454.2013.850082
Liao, W., Li, J., Zhang, X., and Li, C. (2023). Motor imagery brain-computer interface rehabilitation system enhances upper limb performance and improves brain activity in stroke patients: A clinical study. Front. Hum. Neurosci. 14, 17:1117670. doi: 10.3389/fnhum.2023.1117670
Liu, X., Zhang, W., Li, W., Zhang, S., Lv, P., and Yin, Y. (2023). Effects of motor imagery based brain-computer interface on upper limb function and attention in stroke patients with hemiplegia: a randomized controlled trial. BMC Neurol. 23:136. doi: 10.1186/s12883-023-03150-5
Markram, H., Gerstner, W., and Sjöström, P. J. (2011). A history of spike-timing-dependent plasticity. Front. Synaptic. Neurosci. 3:4. doi: 10.3389/fnsyn.2011.00004
McDonnell, M. N., and Stinear, C. M. (2017). TMS measures of motor cortex function after stroke: a meta-analysis. Brain Stimul. 10, 721–734. doi: 10.1016/j.brs.2017.03.008
Miao, Y., Chen, S., Zhang, X., Jin, J., Xu, R., Daly, I., et al. (2020). BCI-based rehabilitation on the stroke in sequela stage. Neural Plast. 2020:8882764. doi: 10.1155/2020/8882764
Min, Y., Park, J. W., Park, E., Kim, A. R., Cha, H., Gwak, D. W., et al. (2020). Interhemispheric functional connectivity in the primary motor cortex assessed by resting-state functional magnetic resonance imaging aids long-term recovery prediction among subacute stroke patients with severe hand weakness. J. Clin. Med. 9:975. doi: 10.3390/jcm9040975
Moher, D., Liberati, A., Tetzlaff, J., and Altman, D. G.PRISMA Group (2009). Preferred reporting items for systematic reviews and meta-analysis: the PRISMA statement. PLoS Med. 6:e1000097. doi: 10.1371/journal.pmed.1000097
Moseley, A. M., Herbert, R. D., Sherrington, C., and Maher, C. G. (2002). Evidence for physiotherapy practice: a survey of the physiotherapy evidence database (PEDro). Aust. J. Physiother. 48, 43–49. doi: 10.1016/s0004-9514(14)60281-6
Murphy, T., and Corbett, D. (2009). Plasticity during stroke recovery: from synapse to behaviour. Nat. Rev. Neurosci. 10, 861–872. doi: 10.1038/nrn2735
Myrden, A., and Chau, T. (2015). Effects of user mental state on EEG-BCI performance. Front. Hum. Neurosci. 9:308. doi: 10.3389/fnhum.2015.00308
Nicolo, P., Rizk, S., Magnin, C., Pietro, M. D., Schnider, A., and Guggisberg, A. G. (2015). Coherent neural oscillations predict future motor and language improvement after stroke. Brain 138, 3048–3060. doi: 10.1093/brain/awv200
Nojima, I., Sugata, H., Takeuchi, H., and Mima, T. (2022). Brain–computer Interface training based on brain activity can induce motor recovery in patients with stroke: a meta-analysis. Neurorehabil. Neural Repair 36, 83–96. doi: 10.1177/15459683211062895
Park, C. H., Chang, W. H., Ohn, S. H., Kim, S. T., Bang, O. Y., Pascual-Leone, A., et al. (2011). Longitudinal changes of resting-state functional connectivity during motor recovery after stroke. Stroke 42, 1357–1362. doi: 10.1161/STROKEAHA.110.596155
Peng, Y., Wang, J., Liu, Z., Zhong, L., Wen, X., Wang, P., et al. (2022). The application of brain-computer Interface in upper limb dysfunction after stroke: a systematic review and Meta-analysis of randomized controlled trials. Front. Hum. Neurosci. 16:798883. doi: 10.3389/fnhum.2022.798883
Pollock, A., Farmer, S. E., Brady, M. C., Langhorne, P., Mead, G. E., Mehrholz, J., et al. (2014). Interventions for improving upper limb function after stroke. Cochrane Database Syst. Rev. 2014:CD010820. doi: 10.1002/14651858.CD010820
Ramos-Murguialday, A., Broetz, D., Rea, M., Läer, L., Yilmaz, O., Brasil, F. L., et al. (2013). Brain-machine interface in chronic stroke rehabilitation: a controlled study. Ann. Neurol. 74, 100–108. doi: 10.1002/ana.23879
Rehme, A. K., Fink, G. R., von Cramon, D., and Grefkes, C. (2011). The role of the contralesional motor cortex for motor recovery in the early days after stroke assessed with longitudinal FMRI. Cereb. Cortex 21, 756–768. doi: 10.1093/cercor/bhq140
Remsik, A. B., van Kan, P., Gloe, S., Gjini, K., Williams, L., Nair, V., et al. (2022). BCI-FES with multimodal feedback for motor recovery Poststroke. Front. Hum. Neurosci. 16:725715. doi: 10.3389/fnhum.2022.725715
Schulz, R. (2012). Assessing the integrity of corticospinal pathways from primary and secondary cortical motor areas after stroke. Stroke 43, 2248–2251. doi: 10.1161/STROKEAHA.112.662619
Sebastián-Romagosa, M., Cho, W., Ortner, R., Murovec, N., von Oertzen, T., Kamada, K., et al. (2020). Brain computer Interface treatment for motor rehabilitation of upper extremity of stroke patients-a feasibility study. Front. Neurosci. 14:591435. doi: 10.3389/fnins.2020.591435
Serrien, D. J., Strens, L. H., Cassidy, M. J., Thompson, A. J., and Brown, P. (2004). Functional significance of the ipsilateral hemisphere during movement of the affected hand after stroke. Exp. Neurol. 190, 425–432. doi: 10.1016/j.expneurol.2004.08.004
Simon, C., Bolton, D. A. E., Kennedy, N. C., Soekadar, S. R., and Ruddy, K. L. (2021). Challenges and opportunities for the future of brain-computer Interface in neurorehabilitation. Front. Neurosci. 15:699428. doi: 10.3389/fnins.2021.699428
Takebayashi, T., Takahashi, K., Amano, S., Gosho, M., Sakai, M., Hashimoto, K., et al. (2022). Robot-assisted training as self-training for upper-limb hemiplegia in chronic stroke: a randomized controlled trial. Stroke 53, 2182–2191. doi: 10.1161/STROKEAHA.121.037260
Tani, M., Ono, Y., Matsubara, M., Ohmatsu, S., Yukawa, Y., Kohno, M., et al. (2018). Action observation facilitates motor cortical activity in patients with stroke and hemiplegia. Neurosci. Res. 133, 7–14. doi: 10.1016/j.neures.2017.10.002
Vink, J. J. T., van Lieshout, E., Otte, W. M., van Eijk, R., Kouwenhoven, M., Neggers, S. F. W., et al. (2023). Continuous Theta-burst stimulation of the Contralesional primary motor cortex for promotion of upper limb recovery after stroke: a randomized controlled trial. Stroke 54, 1962–1971. doi: 10.1161/STROKEAHA.123.042924
Ward, N. S., Brown, M. M., Thompson, A. J., and Frackowiak, R. S. (2003). Neural correlates of motor recovery after stroke: a longitudinal fMRI study. Brain 126, 2476–2496. doi: 10.1093/brain/awg245
Zhan, G., Chen, S., Ji, Y., Xu, Y., Song, Z., Wang, J., et al. (2022). EEG-based brain network analysis of chronic stroke patients after BCI rehabilitation training. Front. Hum. Neurosci. 16:909610. doi: 10.3389/fnhum.2022.909610
Zhang, J. J., Bai, Z., and Fong, K. N. K. (2022). Priming intermittent Theta burst stimulation for Hemiparetic upper limb after stroke: a randomized controlled trial. Stroke 53, 2171–2181. doi: 10.1161/STROKEAHA.121.037870
Zhang, J., Meng, L., Qin, W., Liu, N., Shi, F. D., and Yu, C. (2014). Structural damage and functional reorganization in ipsilesional m1 in well-recovered patients with subcortical stroke. Stroke 45, 788–793. doi: 10.1161/STROKEAHA.113.003425
Zhang, R., Wang, C., He, S., Zhao, C., Zhang, K., Wang, X., et al. (2023). An adaptive brain-computer Interface to enhance motor recovery after stroke. IEEE Trans. Neural Syst. Rehabil. 31, 2268–2278. doi: 10.1109/TNSRE.2023.3272372
Zhang, T., Zhao, J., Li, X., Bai, Y., Wang, B., Qu, Y., et al. (2020). Chinese Stroke Association stroke council guideline writing committee. Chinese Stroke Association guidelines for clinical management of cerebrovascular disorders: executive summary and 2019 update of clinical management of stroke rehabilitation. Stroke Vasc. Neurol. 5, 250–259. doi: 10.1136/svn-2019-000321
Keywords: stroke, brain-computer interface, functional electrical stimulation, upper limb function, meta-analysis
Citation: Ren C, Li X, Gao Q, Pan M, Wang J, Yang F, Duan Z, Guo P and Zhang Y (2024) The effect of brain-computer interface controlled functional electrical stimulation training on rehabilitation of upper limb after stroke: a systematic review and meta-analysis. Front. Hum. Neurosci. 18:1438095. doi: 10.3389/fnhum.2024.1438095
Edited by:
Noman Naseer, Air University, PakistanCopyright © 2024 Ren, Li, Gao, Pan, Wang, Yang, Duan, Guo and Zhang. This is an open-access article distributed under the terms of the Creative Commons Attribution License (CC BY). The use, distribution or reproduction in other forums is permitted, provided the original author(s) and the copyright owner(s) are credited and that the original publication in this journal is cited, in accordance with accepted academic practice. No use, distribution or reproduction is permitted which does not comply with these terms.
*Correspondence: Yasu Zhang, zhangyasu16@163.com