- 1BrainNet, Health and Technology District, Surrey, BC, Canada
- 2Faculty of Individualized Interdisciplinary Studies, Simon Fraser University, Burnaby, BC, Canada
- 3Faculty of Science, Simon Fraser University, Burnaby, BC, Canada
- 4Djavad Mowafaghian Center for Brain Health, Faculty of Medicine, University of British Columbia, Vancouver, BC, Canada
- 5Department of Sport, Exercise and Rehabilitation, Faculty of Health and Life Sciences, Northumbria University, Newcastle upon Tyne, Tyne and Wear, United Kingdom
- 6Brain Behavior Laboratory, Department of Physical Therapy, Faculty of Medicine, University of British Columbia, Vancouver, BC, Canada
- 7Institute of Psychology, BioTechMed Graz, University of Graz, Graz, Austria
- 8Faculty of Applied Sciences, Simon Fraser University, Burnaby, BC, Canada
Introduction: In past work we demonstrated different patterns of white matter (WM) plasticity in females versus males associated with learning a lab-based unilateral motor skill. However, this work was completed in neurologically intact older adults. The current manuscript sought to replicate and expand upon these WM findings in two ways: (1) we investigated biological sex differences in neurologically intact young adults, and (2) participants learned a dynamic full-body balance task.
Methods: 24 participants (14 female, 10 male) participated in the balance training intervention, and 28 were matched controls (16 female, 12 male). Correlational tractography was used to analyze changes in WM from pre- to post-training.
Results: Both females and males demonstrated skill acquisition, yet there were significant differences in measures of WM between females and males. These data support a growing body of evidence suggesting that females exhibit increased WM neuroplasticity changes relative to males despite comparable changes in motor behavior (e.g., balance).
Discussion: The biological sex differences reported here may represent an important factor to consider in both basic research (e.g., collapsing across females and males) as well as future clinical studies of neuroplasticity associated with motor function (e.g., tailored rehabilitation approaches).
1 Introduction
The human brain can be categorized into two main tissue types: gray matter (GM: cell bodies (somas) that functions to receive and process information), and white matter (WM: axon projections that functions to transmit nerve signals between GM regions or between GM and the spinal cord). Neuroplasticity-related changes in these tissues can occur due to development, disease, degeneration, or be associated with learning and experiences. In essence, neuroplasticity can be operationally defined as a lifelong process where the brain reorganizes neural networks (Sampaio-Baptista and Johansen-Berg, 2017; Fields, 2010; Guglielman, 2012; Kesselring, 2015). Historically, activity-dependent changes in these tissues have mainly focused on changes at the synapse of neurons, located in GM. However, our group and others have demonstrated that neuroplasticity can occur beyond the synapse and along the axon in WM (Sampaio-Baptista and Johansen-Berg, 2017; Frizzell et al., 2021; Kirby et al., 2022; Reid et al., 2017; Weber et al., 2019).
Magnetic resonance imaging (MRI) derived techniques are commonly used to non-invasively examine and track structural and functional changes in the brain (Hamaide et al., 2016; Sale et al., 2017; Reid et al., 2017; Tardif et al., 2016). More specifically, MRI-derived techniques such as diffusion-weighted imaging (DWI) and myelin-water imaging (MWI) have been used extensively to focus MRI-based research on WM characterization, development, degeneration, and neuroplasticity (Kirby et al., 2022; Frizzell et al., 2021; Reid et al., 2017; Sale et al., 2017; Scholz et al., 2009; Islam et al., 2020; Weber et al., 2019; Lakhani et al., 2016).
Much of this previous literature acquires these in-vivo microstructural measures from each voxel of an MRI image. Averages of these values are usually taken across multiple voxels making up regions of interests [ROIs; (Lakhani et al., 2016)]. However, these ROI analyses may be skewed by non-population-specific atlases or by the inclusion of multiple fiber pathways within a voxel or ROI. Additionally, tract-based analyses that determine a whole tract mean measure may lead to less specificity and diminish sensitivity to detect structural properties and change. Previous literature has used tractography, computing individual’s WM tracts to then determine tract profiles (Kirby et al., 2022; Deng et al., 2018; Dayan et al., 2015; Yeatman et al., 2012; Baumeister et al., 2020). Tract profiles are helpful in determining the potential locus of change along a tract. New insights into WM change can be revealed using tract profiles that are not obvious from mean measures of that tract (Yeatman et al., 2012). These techniques have been implemented in neurologically intact individuals (Baumeister et al., 2020; Kirby et al., 2022), and in pathological populations, such as those effected by multiple sclerosis [MS; (Reich et al., 2007; Dayan et al., 2015)], Alzheimer’s disease (Zhang et al., 2019), and Parkinson’s disease (Cousineau et al., 2017). While this technique is a step in the right direction, it inherently relies on voxel-wise measurements and averages along fiber pathways, potentially diminishing sensitivity to detect change.
A tractography modality termed correlational tractography [grounded in connectometry (Yeh et al., 2016)] has been recently introduced to investigate WM tracts. This technique is based on a model-free tractography method that tracks connectivity with quantitative anisotropy (QA: the amount of anisotropic spins that diffuse along the fiber orientation) using the local connectome [the degree of connectivity between adjacent voxels in WM (Yeh et al., 2016)]. The core hypothesis in connectometry is that the associations between local connectomes and study variables tend to propagate along a common fiber pathway. Accordingly, this method focuses on tracking the difference instead of finding the difference in the tract (Yeh et al., 2016). Its primary measure, QA, is associated with axonal density (Yeh et al., 2010; Yeh et al., 2013b) and has majorly focused on neurological injury, showing decreases in QA associated with axonal loss or damage (Yeh et al., 2019b; Shen et al., 2015). Tractography based on QA is robust against peritumoral edema which contributes to more reliable results (Zhang et al., 2013) and is less sensitive to partial volume effects when compared to tractography techniques using FA, generalized FA (GFA), and anatomy in both phantom and in-vivo analyses (Yeh et al., 2013b). Subsequently, correlational tractography has been applied to cross-sectional analyses of WM tracts to correlate findings in neuropsychological disorders (Hula et al., 2020; Yeh et al., 2013a; Delaparte et al., 2017; Wang et al., 2019; Dresang et al., 2021; Brownsett et al., 2024) and neurodegenerative diseases (Sanchez-Catasus et al., 2021, 2022; Ghazi Sherbaf et al., 2018; Mojtahed Zadeh et al., 2018). This new method has also tracked longitudinal WM alterations at a group level, achieving higher sensitivity and specificity than conventional tractography (Yeh et al., 2016). Regarding longitudinal tracking, correlational tractography has been used primarily to index degeneration and neuronal damage from mild traumatic brain injury (Huang et al., 2023; Li et al., 2022). To our knowledge, this technique has not been used to study longitudinal changes facilitated by motor skill acquisition and learning.
Biological sex differences may influence the mechanisms that underly neuroplasticity (Marrocco and McEwen, 2016; McEwen and Milner, 2017). Differences in overall brain size, as well as in focused major brain structures was a popular area of research in early neuroimaging studies comparing females and males (Agartz et al., 1992; Coffey et al., 1998; Allen et al., 2002; Luders and Kurth, 2020). More specifically, microstructural values of WM tissue, such as DWI-derived fractional anisotropy (FA: a measure of anisotropy of water molecules’ diffusion in the brain), have been used to study microstructural differences in females and males (Shin et al., 2005; Westerhausen et al., 2003; Schmithorst et al., 2008; Liu et al., 2010; Björnholm et al., 2017; Hasan et al., 2008; Ritchie et al., 2018; Takao et al., 2014). These findings include female and male differences in developmental, degenerative, and experience-dependent changes in microstructural measures (Schmithorst et al., 2008; Toschi et al., 2020; Kirby et al., 2024).
Functionally, sex differences have also been noted in GM activation [i.e. the blood oxygen-level dependent (BOLD) signal] during voluntary motor tasks with males showing a larger surface area and higher variability in the BOLD signal during voluntary motor tasks (Andrushko et al., 2023). We have also shown differences between female and male-specific changes in WM following complex motor skill acquisition, despite comparable change in motor behavior (Kirby et al., 2024). Taken together, this work suggested biological sex is an important variable with demonstrable differences on both GM and WM during motor tasks and learning, which are not explained by differences in performance.
In our previous work, we studied a cohort of older adults [mean age (standard deviation): 64.2 (8.5) years] in a lab-based setting where they performed a unilateral reaching task (Kirby et al., 2024); as such it is unclear whether similar effects apply to different ages and/or motor learning tasks. While past work has shown relationships between DWI-derived measures in WM, with measures of motor learning (Frizzell et al., 2020, 2021; Kirby et al., 2022; Sale et al., 2017; Reid et al., 2017; Lakhani et al., 2016; Azzarito et al., 2021; Moore et al., 2017; Taubert et al., 2010; Borich et al., 2014) very little work has considered how biological sex impacts this relationship. Filling this knowledge gap will improve our understanding of how biological sex impacts neuroplastic change during motor learning and increase awareness of how WM disease might impact females versus males differently. A better understanding can improve monitoring of disease progressions that show differences between females and males (DeCasien et al., 2022; Ferretti et al., 2018). Moving forward with rehabilitation involves understanding the differences of pharmaceutical drug benefits and risks between females and males (Wierenga et al., 2024; Carey et al., 2017), as hormonal or sex-specific genotype interactions may affect drug metabolism and treatment response (Shulman, 2007; Abel et al., 2010; DeCasien et al., 2022; Ferretti et al., 2018).
Accordingly, the current study sought to extend work investigating differences in WM plasticity to consider younger adults learning a complex, whole body balance task. Given prior uses of correlational tractography and previous neuroimaging characterization of WM in females and males, our primary hypothesis was that females and males would show different patterns of WM change in widespread WM tracts, specifically reflected in greater increases in QA in females as compared to males.
2 Materials and methods
2.1 Experimental paradigm
The current study analyzed data from OpenNeuro [OpenNeuro Dataset ds003138, “Tidying Up White Matter: Neuroplastic Transformations in Sensorimotor Tracts following Slackline Skill Acquisition” (Koschutnig et al., 2023)]. A total of 60 participants were originally recruited for the study. After dropouts (7) and noisy data (1), a total of 52 participants [24 balance training (14 female) and 28 controls (16 female)] were included [mean age (standard deviation): 23.88 (3.48) years]. A fuller demographic breakdown is included in Table 1. Participants engaged in a slackline motor skill acquisition paradigm. Briefly, this began with an MRI scan before their first training session. Participants then worked with a professional slackline trainer for 90-minute sessions spaced approximately one week apart until they had reached a specified skill level. All participants reached the specified skill level in three or fewer sessions [range = 2–3 sessions; mean (standard deviation) = 2.29 (0.45)]. This included balancing on the slackline for one minute, followed by walking forward one meter and then backwards one meter. Once this skill level was reached, participants took part in a second MRI scan within 24 hours. The current study focused on pre- and post-training timepoints. All participants also underwent a follow-up MRI scan three weeks after training, but this was not included in the current analysis. All control participants were scanned following a similar timetable but did not undergo balance training.
2.2 MRI acquisition
MRI data were acquired at the MRI-Lab Graz, Austria on a 3-Tesla (3T) Magnetom Skyra scanner (Siemens Healthineers, Erlangen, Germany) using a 32-channel head coil. All scans included a T1-weighted MPRage-Sequence (TR = 2400ms, TE = 2.26ms, 288 slices, thickness 0.8 mm, flip angle = 8°). Multi-shell DWI data were acquired with a multi-band accelerated echo-planar imaging sequence protocol (TR = 3500 ms, 68 slices, voxel size = 2 mm isotropic, multiband factor 4) with an anterior-posterior phase encoding direction for three b-values (b-values = 1000, 2000, and 3000 s/mm2; TE = 104ms, 113ms, 125ms; number of directions = 20, 30, and 64). Additionally, one b0-image and one extra b0-image in the reverse phase-encoding direction for each b-value was also acquired to correct for magnetic susceptibility-induced distortions. Overall, the total time of acquisition was about 14 minutes.
2.3 MRI processing
DWI data were corrected for echo planar imaging (EPI) distortions (Andersson et al., 2012), as well as motion and eddy corrected (Andersson and Sotiropoulos, 2016) using FSL’s Top-up and Eddy tools. Correlational tractography was prepared and carried out following DSI Studio (version: December 14, 2023). This technique is based on a model-free tractography method that calculates the spin distribution function (SDF: an orientation distribution function defined as the density of diffusing spins that have a displacement oriented at a direction during the diffusion time) (Yeh et al., 2010; Yeh and Tseng, 2011) on a local connectome scale (Yeh et al., 2016). By considering the possibility of more than one principal diffusion direction, model-free tractography methods are less susceptible to the partial volume of crossing fibers. The measurement at the base of connectometry, QA, is robust, specifically, to the free water effect (Yeh et al., 2013b). The difference between QA and regular orientation distribution function-based measures is that QA scales with spin density and the isotropic component is discarded (regular orientation distribution function is often min-max scaled to 0–1) (Yeh et al., 2013b). QA is a metric for each resolved fiber population, unlike FA or generalized FA (GFA), which is a metric for each voxel (Yeh et al., 2010). These attributes of QA, specifically the consideration of restricted diffusion, make it less affected by edema than FA and axial diffusivity (Yeh et al., 2013b).
A connectrometry database was created in DSI Studio with 52 subjects (104 total scans – 52 benchmark and 52 post-training scans). The diffusion data were reconstructed in the MNI space using q-space diffeomorphic reconstruction (Yeh and Tseng, 2011) to obtain the spin distribution function (Yeh et al., 2010). A diffusion sampling length ratio of 1.25 was used. The output resolution in diffeomorphic reconstruction was 2 mm isotropic. The restricted diffusion was quantified using restricted diffusion imaging (Yeh et al., 2017). QA (Yeh et al., 2013b) was extracted as the local connectome fingerprint (Yeh et al., 2016) to be used in the connectometry analysis. The difference between longitudinal scans were calculated by post-training minus pre-training scans. Only increased longitudinal changes were used in the analysis. Diffusion MRI connectometry (Yeh et al., 2016) was used to derive the correlational tractography (a deterministic tractography method) that has longitudinal change of QA correlated with the desired variable.
2.4 Statistical analysis
As baseline motor skills and age may represent additional factors affecting WM neuroplasticity [these variables impact motor skill learning in general (Guadagnoli and Lee, 2004; Lillard and Erisir, 2011)], an analysis to determine the relationship between motor performance and neuroimaging results included a two-tailed independent samples t-test between female and male groups to test for significant pre-existing slackline motor skill differences, age differences, and for final slackline skill. The final slackline skill was assessed by the professional trainer from 1 (very bad) to 10 (excellent). Additionally, Spearman’s correlational analysis of mean QA change in the three major highlighted tracts (outlined below) with pre-existing slackline practice (ranked on a 5-point scale: 1 = never attempted, 2 = < 15 min, 3 = 15–30 min, 4 = 30–45 min, 5 = 45–60 min) and Pearson’s correlational analysis of mean QA change in the three major highlighted tracts (outlined below) with age were completed (Supplementary Data 3).
Our correlational tractography analysis used different t-statistic thresholds (2.5, 3.0, and 3.5). The t-statistic is determined and evaluated to pass the chosen threshold from a non-parametric Spearman partial correlation analysis. This analysis is done at each local connectome to investigate weaker and stronger correlations. Using a range of t-thresholds led to higher sensitivity (high true positives at low thresholds) and to higher specificity (high true negatives at high thresholds). Additionally, based on the variety of length thresholds used in previous research (Wang et al., 2019; Hula et al., 2020; Delaparte et al., 2017; Dresang et al., 2021), different length thresholds [15, 20, 25 voxel distances (30, 40, 50 mm)] were used at each t-threshold to remove fragmented findings (similar to cluster size in cluster-based analyses). The full in-depth explanation of connectometry as a deterministic tractography method is described in Yeh et al. (2016) (additional explanation of threshold parameters is included in Supplementary Data 5). The cerebellum region was removed from analysis as lower slices in the cerebellum are an area of high level noise in DWI, that is suggested to be removed in DSI Studio tutorials (Li et al., 2022). Additionally, sex focused analyses in the balance group were rerun with the highest thresholds including the cerebellum, showing similar final results (Supplementary Data 4). As the cerebellum is a major area responsible for postural adjustments, future work can focus on the complex WM fiber structure of the cerebellum. Tracts were filtered and selected by topology-informed pruning with 16 iterations (Yeh et al., 2019a). A randomly permuted null distribution is also created with this t-threshold. A total of 4000 randomized permutations were applied to the group label to obtain the null distributions of the track length. A false discovery rate (FDR) less than 0.05 indicated a highly confirmative finding. Therefore, only tracts with an FDR < 0.05 were kept. Three different correlational tractography analyses were run. To initially determine differences between the control and intervention group, the correlation between increase in QA and group was determined using a nonparametric Spearman partial correlation, while the effects of age and sex were removed using a multiple regression model. Subsequently, to analyze the effect of biological sex on WM neuroplasticity, an additional interaction variable of Group × Sex was determined for each individual. The correlation between increase in QA and this Group × Sex interaction was then determined, while the effects of age were removed using a multiple regression model. Lastly, to further analyze the effect of biological sex, only individuals in the intervention group (n = 24) were used to determine correlation between increase in QA and sex, while the effects of age were removed using a multiple regression model. Results of the sex effect analysis on the balance group for the highest thresholds [t-threshold = 3.5 & length threshold = 25 voxels (50mm)] were clustered by their respective tracts by DSI Studio and only tracts making up more than 10% of the total association of sex and QA increase were highlighted.
3 Results
3.1 Behavioral data
All subjects taking part in balance training reached the specified skill level. There were no significant differences between female and male pre-existing slackline skills (t-statistic = 1.405, p = 0.17, Figure 1). There were also no significant differences between female and male slackline rating after training completion (t-statistic = 0.684, p = 0.50, Figure 2). Additionally, there were no significant differences between female and male age in the intervention group (t-statistic = 0.260, p = 0.80).
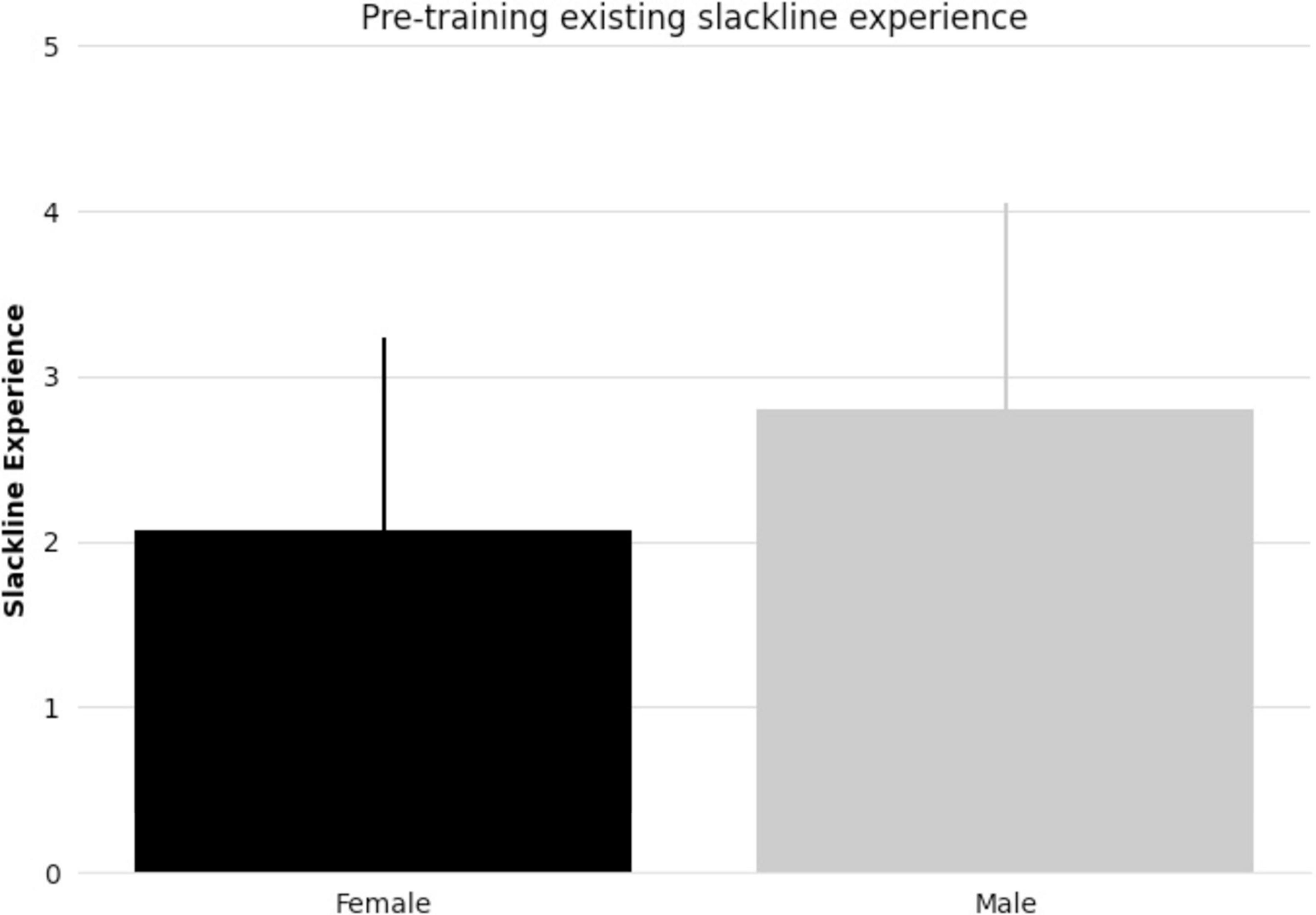
Figure 1. Bar graph comparing female and male mean pre-existing slackline experience (5-point scale: 1 = never attempted, 2 = < 15 min, 3 = 15–30 min, 4 = 30–45 min, 5 = 45–60 min) and standard deviation.
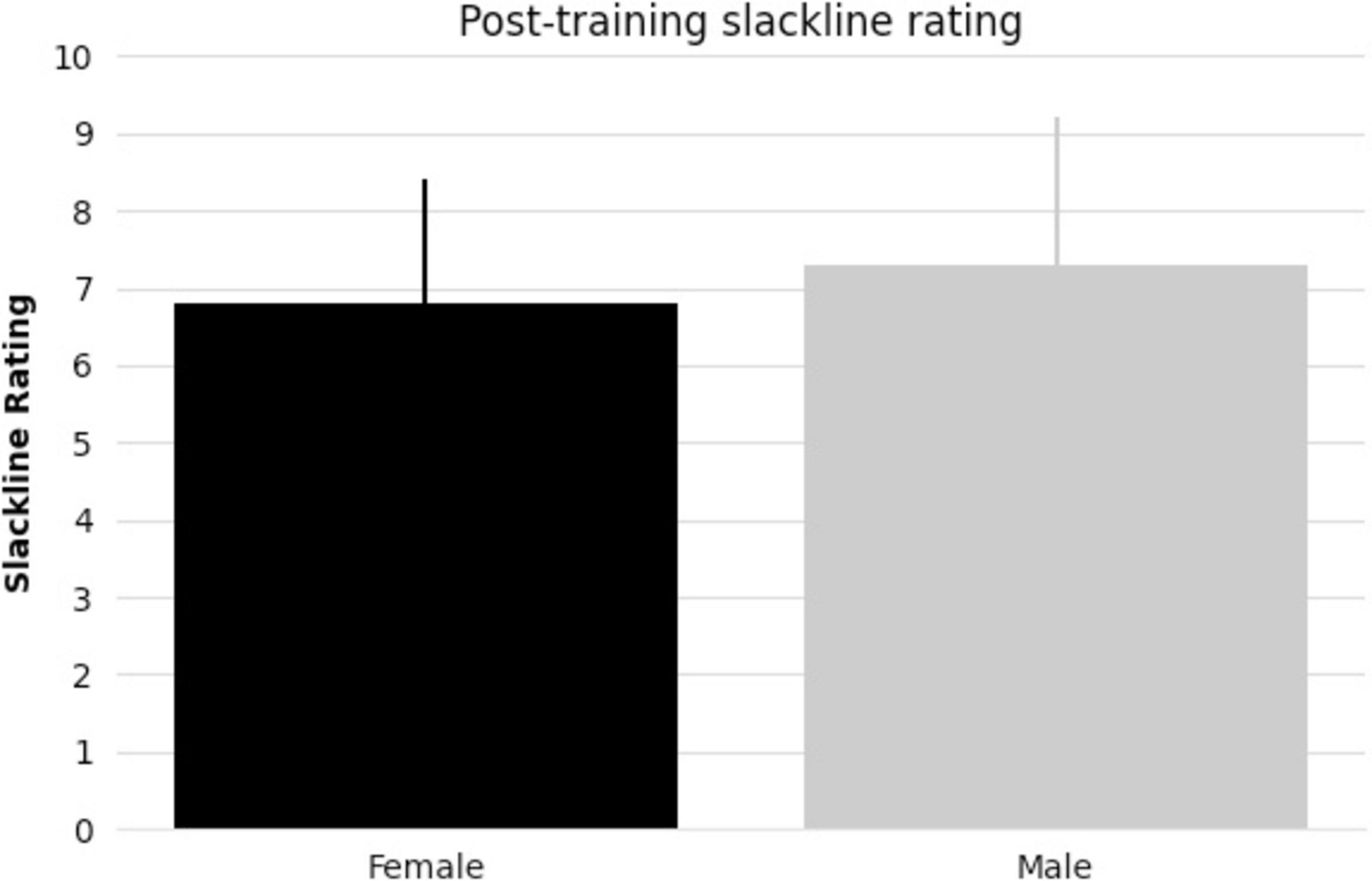
Figure 2. Bar graph comparing female and male mean post-training slackline rating [1 (very bad) to 10 (excellent)] and standard deviation.
3.2 Neuroimaging
The effect of taking part in balance training was significantly associated with an increase in QA at lower thresholds [(t = 2.5; Length = 30mm, 40mm, 50mm; FDR < 0.05) & (t = 3.0; Length = 30mm; FDR < 0.05)], but this effect was not significantly associated at higher thresholds (Supplementary Data 1). The Interaction effect of Sex × Group was highly associated with increased QA from pre- to post-training across all thresholds (Supplementary Data 2). Results were found in all three fiber types (association, projection, and commissure fibers–Figure 3).
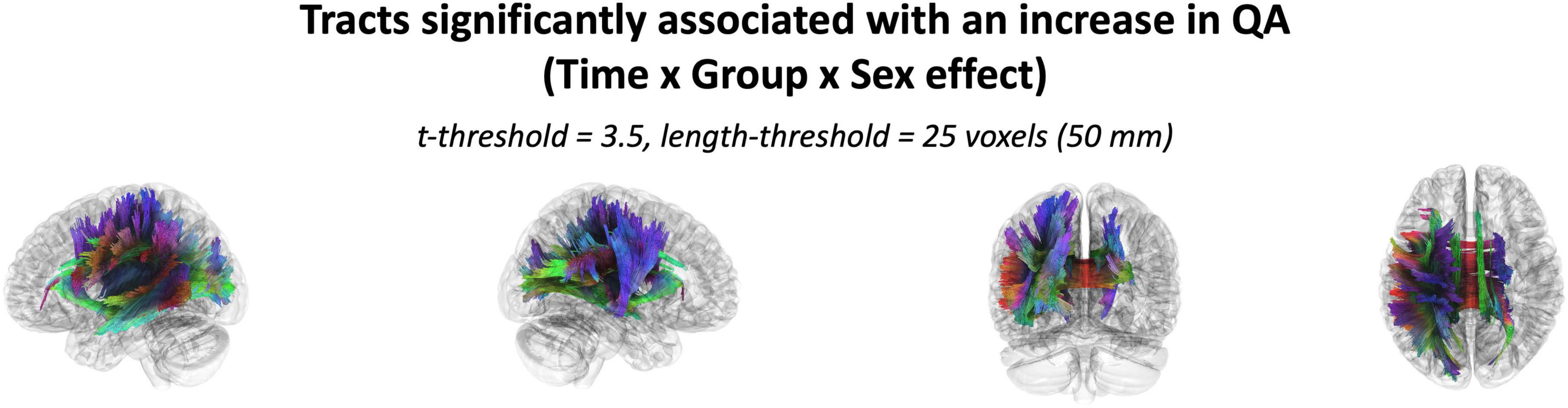
Figure 3. Tracts with increased QA significantly associated with the interaction of Sex and Intervention at a t-threshold of 3.5 and length threshold of 25 voxels (50mm). Tractography is rendered by directional colors (red: left-right; green: anterior-posterior; blue: superior-inferior).
3.3 Intervention sex effect
The effect of participating in balance training was highly associated with an increase in QA for females only, as there were no tracts significantly associated with the increases in QA for males across any thresholds (Table 2). Tracts significantly associated with increased QA in females were similar to the Sex × Group interaction results above (Figure 3 visually compared to Figure 4). Mean QA increase along all the fibers in the cluster of significant tracts from Figure 4 on the individual level is visualized in Figure 5. Tracts having a significant association with an increase in QA in females for the highest thresholds (t-threshold = 3.5 & length threshold = 25 voxels; Figure 4) were used for the following tract breakdowns and results (Figures 6, 7 and Table 2).
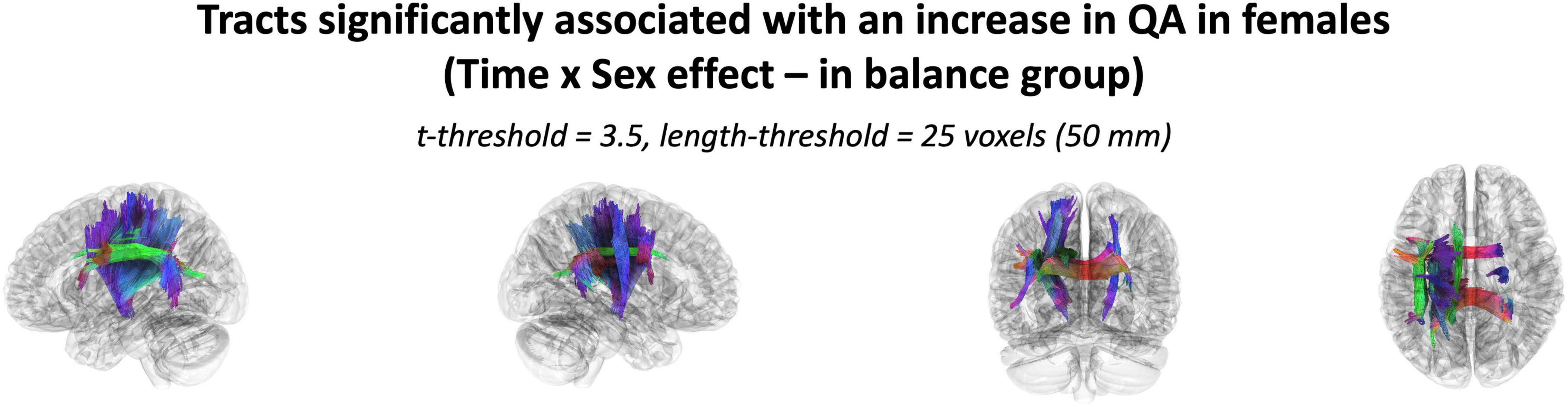
Figure 4. The cluster of tracts with increased QA significantly associated with females in the balance group (Analysis: main effect = biological sex, covariate = age, cohort = balance group). Note only tracts significantly associated with QA increase is shown for females as there were no tracts significantly associated with QA increase in males. Results shown for t-threshold of 3.5 and length threshold of 25 voxels (50 mm). Tractography is rendered by directional colors (red: left-right green: anterior-posterior blue: superior-inferior).
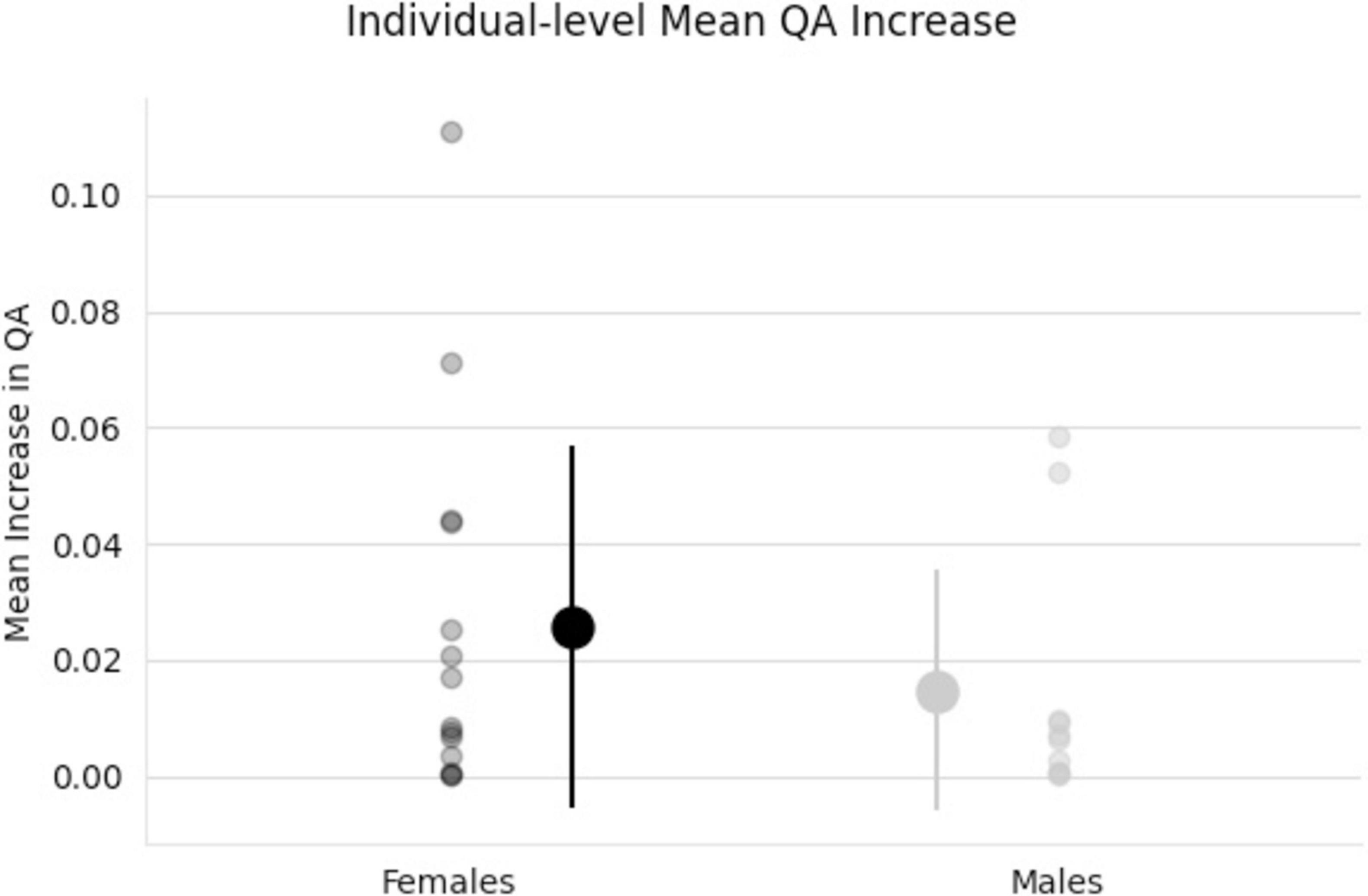
Figure 5. Individual scatter plot data of QA increases. Tracts used for this mean QA comparison are all tracts that showed a significant association with the increase in QA for females from Figure 4. Group means and standard deviations included (middle, larger points) showing larger QA increases for females (black) than males (gray) tracts.
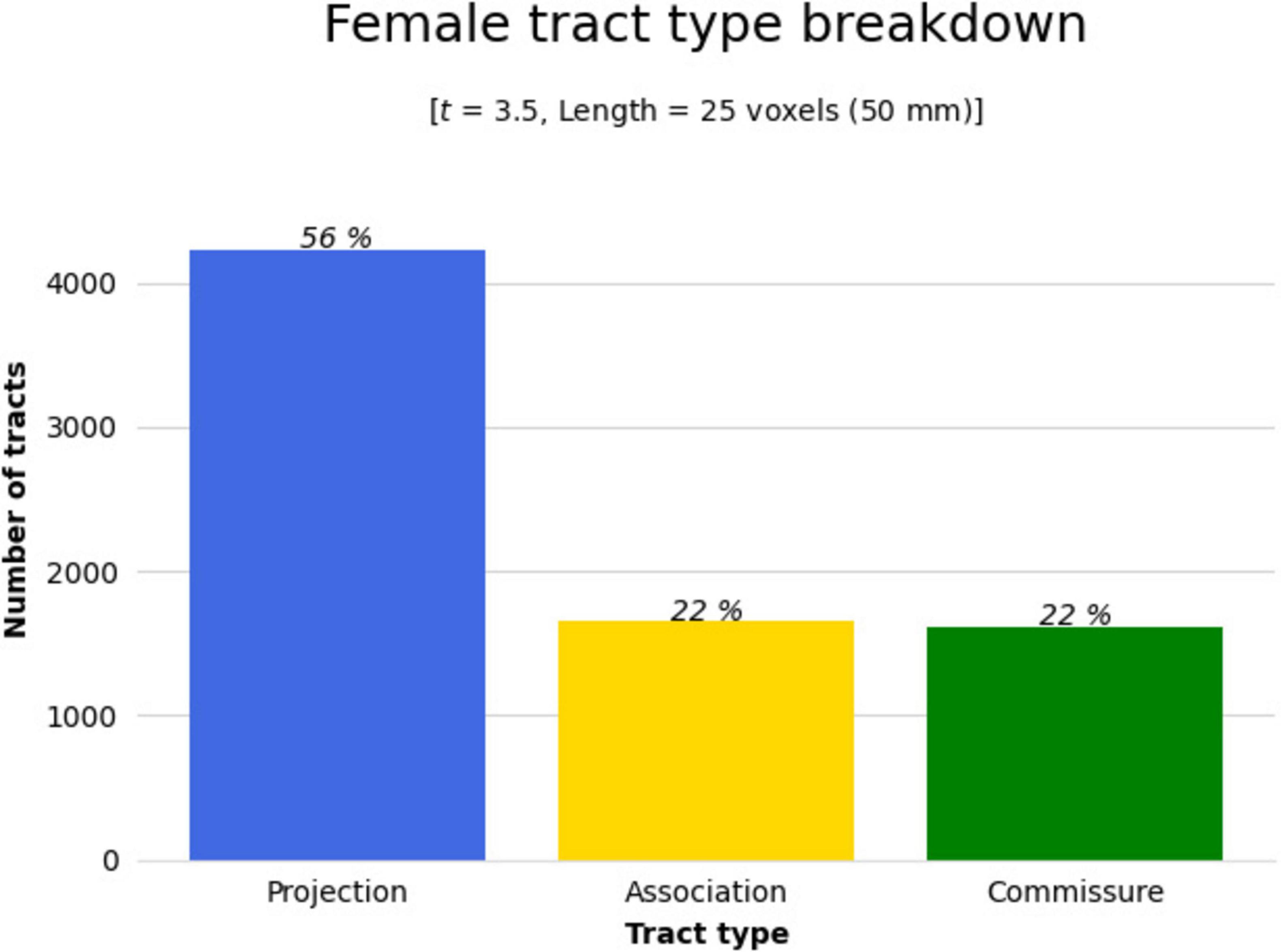
Figure 6. The significant cluster of tracts in Figure 4 (tracts showing a significant association with QA increase in females in the balance group) were clustered by their respective tract types (projection, association, or commissure) by DSI Studio to better determine areas of change. Bar graph breaking down the type of tracts that were shown in Figure 4. Results were majorly found in projection fibers, with significant tracts also noted in association and commissure fibers.
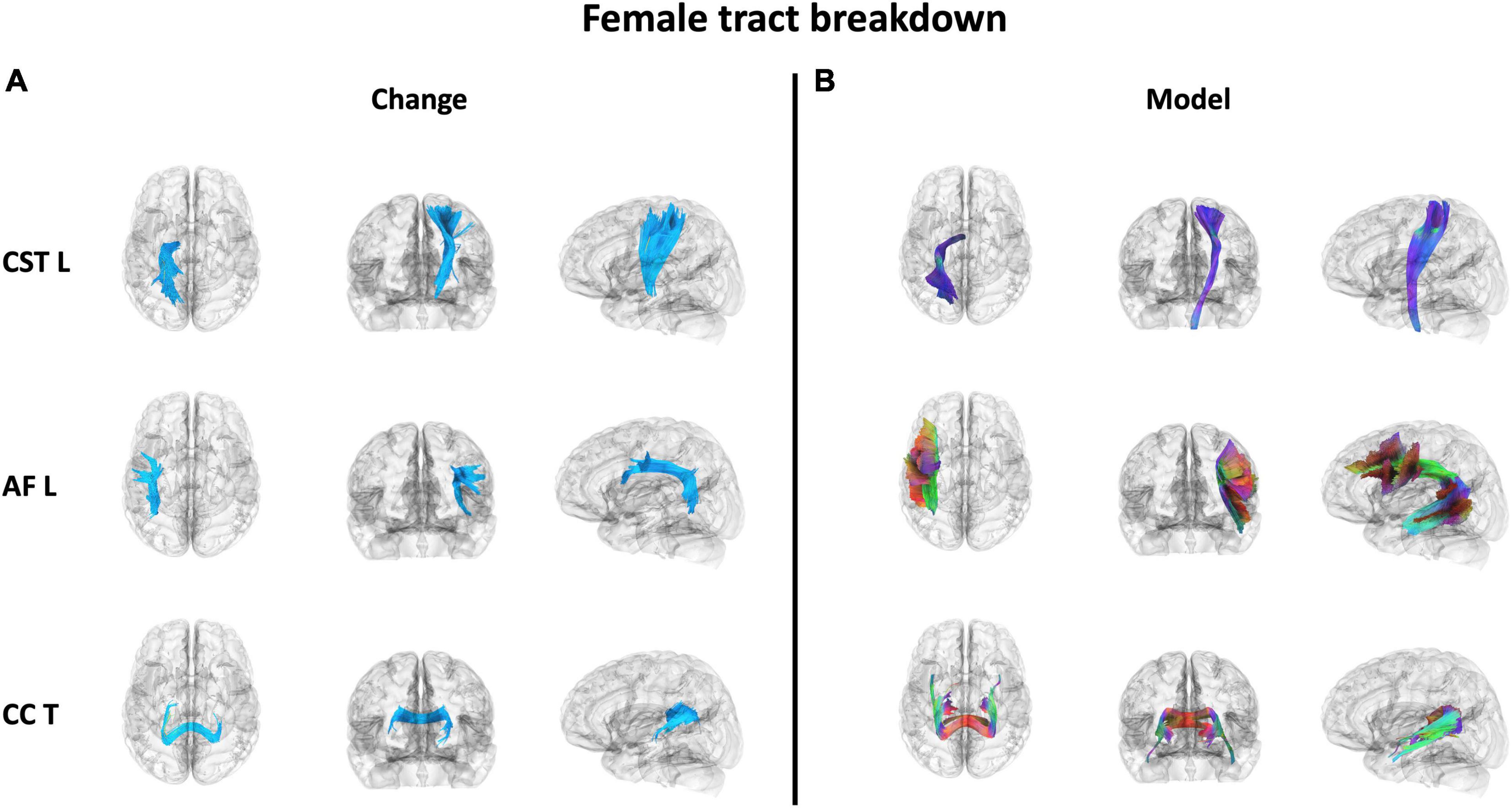
Figure 7. The 3 WM tracts with the highest percentage of tracts associated with increased QA in females with balance training [t = 3.5, Length = 25 voxels (50 mm)]. The portion of the tracts associated with the QA increase associated with females (A) and the full model tract (B). The model tracts are derived from Yeh et al. (2018) to better portray the full WM tract of interest. CST L = left cortico-spinal tract, AF L = left arcuate fasiculus, CC T = tapetum of corpus callosum.
Results were clustered by their respective tracts, and majorly found in projection fibers, with significant tracts also noted in association and commissure fibers (Figure 6). Only tracts making up more than 10% of the total tracts significantly associated with QA increase for females at the highest thresholds [t-threshold = 3.5 & length threshold = 25 voxels (50mm)] were highlighted [the left cortico-spinal tract (CST), arcuate fasciculus (AF), and tapetum of the corpus callosum (CC)] (Table 3 and Figure 7).
3.4 Correlations
Spearman’s correlation analysis showed no significant correlations between mean QA increase in the three highlighted WM tracts and pre-existing slackline skill (Supplementary Data 3). Pearson’s correlation analysis showed no significant correlations between mean QA increase in the three highlighted WM tracts and age (Supplementary Data 3).
4 Discussion
4.1 Summary
The current study characterized widespread QA increases in female human brains associated with balance skill acquisition. This is the first study to our knowledge that employed correlational tractography to investigate WM anisotropy increases associated with motor skill acquisition. The results of our analyses in this younger cohort (mean age = 23.88 years) support our past findings of structural differences between female and male neuroplasticity reported in our previous study with an older cohort (Kirby et al., 2024), similarly showing females exhibiting greater anisotropy increases than males.
4.2 Behavioral changes
All subjects that took part in balance training reached the specified skill level in 2 or 3 90-minute sessions. Notably, in line with our previous research findings (Kirby et al., 2024), there were no discernible differences in performance measures between females and males (pre-existing slackline experience, number of sessions to reach skill level, or post-training slackline skill rating). While performance assessments reveal comparable outcomes between females and males in the current study, neuroimaging provides insights into the differences that may underlie these observed behaviors.
4.3 Neuroimaging
There were no discernible differences in performance measures between females and males even though QA increases showed differences. This structural evidence aligns with functional studies highlighting variations in fMRI activation patterns between females and males during similar task performance (Hugdahl et al., 2006; Lissek et al., 2007; Gorbet and Sergio, 2007; Andrushko et al., 2023). For instance, Gorbet and Sergio (2007) found no performance discrepancies between females and males in a visuo-motor task of increasing complexity, yet identified differences in fMRI activity. Lissek et al. (2007) showed higher activation in females during simple and complex finger tapping tasks, while both groups achieved comparable error rates. These current findings further suggest that females and males may rely on distinct brain regions or tissue types to achieve comparable outcomes.
Correlational tractography identified several tracts that were identified in the Group × Sex interaction across all connectometry threshold values. However, group effects were only noted at lower thresholds. The importance of biological sex in these results was highlighted by the increase in QA for females across all threshold values, while males failed to show any change. The current study supports the importance of including data that are disaggregated by biological sex in analyses and builds on prior work in this research area (Tannenbaum et al., 2019; White et al., 2021; Kirby et al., 2024; Andrushko et al., 2023; Rechlin et al., 2022; Arnegard et al., 2020). Differences in biological sex in neuroimaging analyses have been shown across diverse techniques (Toschi et al., 2020; Schmithorst et al., 2008; Liu et al., 2010; Björnholm et al., 2017; Gorbet and Sergio, 2007). On a large scale, males demonstrate greater variability in cortical surface area measures, subcortical volume, and cortical thickness, across the brain (Wierenga et al., 2020). Additionally, males have a larger cortical surface area, while females show a greater cortical thickness for many regions (Wierenga et al., 2020; Ritchie et al., 2018). Functionally, females and males show different patterns in GM activation during voluntary motor tasks. Similar to the structural results, functional MRI shows that males demonstrate a larger surface area and higher variability of BOLD signal (Andrushko et al., 2023).
While cross-sectional studies are important, identifying different patterns of change can aid in further understanding neural development, degeneration, and plasticity. Previously, we had suggested possible female versus male differences in WM neuroplasticity during motor learning of a unilateral task (Kirby et al., 2024). The current study builds on this past work in two ways. First, we considered change in young, healthy individuals as they completed balance training. Second, we investigated change across a broad network of WM including projection, association, and commissural tracts. It is important to show these patterns across the lifespan in both younger and older cohorts because of the effect age has on WM (Peters, 2002), as well as the previous MRI work that has been done to identify female and male differences in development and degeneration. Toschi et al. (2020) used advanced multi-shell diffusion MRI to suggest that age-related brain changes related to degeneration begin up to 14 years earlier in males than females. They also suggested that the promyelinating effects of female hormones may protect from age-related vulnerabilities in the myelination process (Bartzokis, 2004). Other work demonstrated sex-specific WM development, with females showing a faster rate to matured WM levels (Wang et al., 2012; Schmithorst et al., 2008). While studies relating WM structural and functional measures to motor performance and skills in females and males are scarce (Schmithorst, 2009; Schmithorst and Holland, 2006, 2007; Schmithorst et al., 2008) determined differing structural and functional neural correlates of cognition between females and males during development. The culmination of studies suggested a greater dependance on WM by females during development, potentially moderated by specific information processing demands. On a more macroscopic scale, it has been reported that females show a greater association of intelligence to WM volume, while GM volume correlates more strongly with intelligence in men on both a global (Gur et al., 1999) and regional scale (Haier et al., 2005) in the brain. Outside of DWI and functional MRI, magnetic resonance spectroscopy studies have also demonstrated WM regions in which N-acetyl-aspartate concentrations correlate with intelligence in females but not in males (Jung et al., 2005; Pfleiderer et al., 2004).
It was important to investigate if the differences in WM neuroplasticity during motor learning occurred outside of the CST, as neuronal activity that is needed to perform a task usually depends on the task demands and increases with parametric changes in task difficulty (Prvulovic et al., 2005; Peters et al., 2020; Wnuk et al., 2018; Andrushko et al., 2021; Danielson et al., 2024; Van Ruitenbeek et al., 2023). Therefore, a full body balance task may recruit more widespread neural activity than the unilateral task from Kirby et al. (2024). Different tasks may lead to more or less observed differences between females and males. Tracts only associated with increases in females were found in all three fiber types. Similar to our previous work (Kirby et al., 2024), females showed an increase in QA associated with motor skill acquisition in projection fibers, mainly the CST (Figures 5, 6). Similarly, select neuroplasticity research during motor learning has focused on unilateral training primarily associated with projection fibers resulting in contralateral brain changes (Lakhani et al., 2016; Kirby et al., 2022; Frizzell et al., 2020, 2021; Sale et al., 2017; Reid et al., 2017). For example, Reid et al. trained participants in a motor task with their left hands and reported increases in FA along the right contralateral CST (Reid et al., 2017). In addition to projection tracts, association tracts showed significant QA increases in females. While most commonly associated with language, the AF (specifically the portion of the AF associated with QA increase in females in the current study – Figure 6) does include connections to the ventral premotor cortex (vPMC). This is a region within the broader premotor cortex, which plays a key role in motor planning and execution (Binkofski and Buccino, 2006; Purves et al., 2001; Kakei et al., 2001). In addition to projection and association tracts, our previous work highlighted the CC (a major commissural tract) as a potential area of significance in motor skill acquisition (Frizzell et al., 2021). This has built on past work associating the CC with motor and visuomotor task activation (Weber et al., 2005; Tettamanti et al., 2002; Fabri et al., 2014; Gawryluk et al., 2011; Mazerolle et al., 2008; D’Arcy et al., 2006; Gawryluk et al., 2014b; Mazerolle et al., 2010; Gawryluk et al., 2014a), as well as, FA measures in this region positively correlating with performance during a motor learning session (Vien et al., 2016).
4.4 Clinical relevance
While adding to the female/male neuroscience literature is generally important on a research-level, this is also very important for understanding disease and optimizing rehabilitation. A more focal and consistent neural processing by females would not need an increased speed of signal transmission from a large cortical surface area, but instead would be focused on neural transmission across WM structures for efficient signal transmission (Andrushko et al., 2023; Kirby et al., 2024). As identified in this research, females show a greater increase in anisotropy values in WM during motor learning. This has direct connection to similar results in an older cohort taking part in a different task (Kirby et al., 2024), as well as a more indirect connection (motor research compared to cognition research) to research showing a higher WM dependence by females during development (Schmithorst and Holland, 2006, 2007; Schmithorst, 2009). Speculatively, the current study adds to this by potentially suggesting a higher WM dependence in motor skill acquisition as well. Although this may be beneficial in healthy, neurologically intact populations, to facilitate efficient nervous signal transmission, this approach may be detrimental in the presence of a neurological injury. For example, with stroke and MS, injury to WM interrupts axon signal transmission, resulting in impairment. As the incidence and presentation of many neurological conditions and movement disorders differ between sexes (Meoni et al., 2020), a higher WM dependance may be the reason behind more severe result of WM injury and disease in females (Tomita et al., 2015; Kim et al., 2010; Phan et al., 2019; Persky et al., 2010; Golden and Voskuhl, 2017; Voskuhl, 2020; Koch-Henriksen and Sørensen, 2010).
Along with WM disease, traumatic brain injury (TBI) commonly causes WM damage (Guerrero-Gonzalez et al., 2022; Wright et al., 2021; Narayana, 2017). Studies have highlighted varying recovery trajectories and outcomes for females vs. males following TBI (Gupte et al., 2019; Mikolić et al., 2021; Haynes and Goodwin, 2023). Furthermore, hormonal influences, particularly progesterone which is commonly associated with females, may be neuroprotective when used as a TBI treatment (Wright et al., 2007). As hormonal fluctuations have been shown to effect neuroplasticity (Catenaccio et al., 2016; Pawluski et al., 2016; Barha and Galea, 2010; Saleki et al., 2022), common sex-related hormones like testosterone, estrogen, and progesterone may be the basis for observed female and male differences. Understanding WM changes is important for exploring targeted therapeutic strategies that harness neuroplasticity for improved recovery. This also extends to sex differences in neonatal brain injury and repair, as infant sex can play a role in disease onset, course and resolution (Rosenkrantz et al., 2019). Although many explanatory factors have been identified in sex-specific WM injury outcomes, incorporating learning-induced WM changes into this phenomenon should be discussed.
4.5 Future work and limitations
The current study focused on identifying greater anisotropy increases in females over males in a motor skill acquisition paradigm. This was mainly because previous connectometry analyses focused on QA decreases associated with degeneration (Moshchin et al., 2023; Huang et al., 2023) and increases in QA associated with cognitive rehabilitation (Brownsett et al., 2024). However, prior work identified decreases in anisotropy as helpful for neuroplasticity. For example, our past work identified a decrease in anisotropy in older males during motor skill acquisition (Kirby et al., 2024). Additionally, Taubert et al. (2010) investigated neuroplasticity during balance training, observing reductions in FA associated with learning. FA and other anisotropy measures have only demonstrated low sensitivity to a single neurobiological process (Jones et al., 2013) making the ability to characterize the exact mechanism behind neuroplasticity changes a limitation of the current study. Future work can include both traditional diffusion tensor imaging-based metrics along with complex metrics such as QA (tractography comparison in Yeh et al. (2013b)) to aid in better understanding neurophysiology associated with motor skill acquisition changes. As Fields (2015) has pointed out, conduction velocity along WM is changed to optimize timing of information transmission through neural circuits. Therefore, attenuated increases in myelination or anisotropy values of a certain WM path may occur to increase efficiency of a network. Future work should better elucidate what increase/decrease in anisotropy is specifically showing in regard to neuroplasticity as decreases may also be positive in some instances.
The current study highlights the importance of different brain tissue types in females and males. As training-induced alterations in different types of brain tissue likely operate on different time-scales and may further change in follow-up assessments (Weber et al., 2019; Zatorre et al., 2012), the focused hypothesis on the effect of motor skill acquisition at two timepoints is a limitation of the current study. It is important to better understand the timing of these changes, especially in both GM and WM regions. Speculatively, females and males may take part in similar neurophysiological changes, but at different timescales. These suggestions warrant further investigation into the biological implications underlying these observations.
As this study used publicly available data, our analysis was restricted to the previously collected data. The current study dataset was unique in terminating training and initiating the post-training timepoint once an individual passed a specified behavioural performance test, indicating they had reached a measurable skill level. However, the nature of the task makes it difficult to quantitatively measure pre-existing skills or comparison of final skill level outside of the passing test, reflected in the discrete behavioral measures used. Greater challenges in a training paradigm may lead to greater changes in WM measures (Lakhani et al., 2016). Therefore, it is important for future work to measure a wide variety of pre-existing motor skill competencies and to note that complexity, task-specific goals, and baseline motor skill competency represent additional factors that may affect WM neuroplasticity, as these have previously been shown to impact motor skill learning in general (Guadagnoli and Lee, 2004; Wulf et al., 2010).
Finally, as mentioned above, the most studied sex-based hormones, estrogen and testosterone, are known to affect neuroplasticity (Catenaccio et al., 2016; Pawluski et al., 2016; Barha and Galea, 2010; Saleki et al., 2022). Therefore, it is important for future work not only to include the effect of biological sex, but also to define how hormones effect WM changes. There are other data suggesting that hormonal balance can affect neuroplasticity even at the cellular level (Catenaccio et al., 2016; Laube et al., 2020). However, given the current analysis, consideration of these effects is beyond our scope. Future work could consider how these factors interact.
5 Conclusion
The current study suggests that female and male differences in WM neuroplasticity during motor skill acquisition may be detectable using MRI-derived DWI measures and connectometry. The current study builds on past work (Kirby et al., 2024) by considering change in young, healthy individuals as they completed balance training and by investigating change across a broad network of WM including projection, association, and commissural tracts. Importantly, we discovered differences in WM changes for females versus males despite both groups reaching a similar level of balance motor skill. These findings suggest that there are distinct patterns of change that take place in female versus male brains to support motor skill acquisition. We provide further support for the importance of including sex-based analyses, as females and males may exhibit differences in neural mechanisms (Toschi et al., 2020; Kopec et al., 2018; Rogojin et al., 2023). As pathophysiology of many illnesses and their resulting rehabilitation differ between females and males, our data suggest that future work should consider how differences in biological sex affect patterns of both structural and functional change associated with motor learning. These differences in tissue dependance likely have important clinical implications for understanding impairment severity and rehabilitation strategies for each sex independently.
Data availability statement
Publicly available datasets were analyzed in this study. This data can be found here: https://doi.org/10.18112/openneuro.ds003138.v1.0.1.
Ethics statement
Ethical approval was not required for the studies involving humans because the study used openly available data from OpenNeuro, which had already received ethics approvals to be made openly available. The studies were conducted in accordance with the local legislation and institutional requirements. Written informed consent for participation was not required from the participants or the participants’ legal guardians/next of kin in accordance with the national legislation and institutional requirements because the study used openly available data from OpenNeuro, which had already received informed consent to be made openly available.
Author contributions
EK: Conceptualization, Data curation, Formal analysis, Investigation, Methodology, Visualization, Writing – original draft, Writing – review and editing. JA: Conceptualization, Formal analysis, Writing – original draft. LB: Conceptualization, Supervision, Visualization, Writing – original draft. KK: Data curation, Project administration, Resources, Writing – review and editing. RD’A: Funding acquisition, Investigation, Supervision, Writing – original draft, Writing – review and editing.
Funding
The author(s) declare financial support was received for the research, authorship, and/or publication of the article. This work has been supported in part by funding from the Natural Sciences and Engineering Research Council of Canada (Discovery Grant, PI R. C. N. D’Arcy; Discovery Grant, PI L.A. Boyd; CGS M, E.D. Kirby), Canadian Institutes of Health Research (Operating Grant, PI L. A. Boyd). JA is supported by a Vice-Chancellor Fellowship at Northumbria University, and was previously supported by Canadian Institutes of Health Research (CIHR) and Michael Smith Foundation for Health Research (MSFHR) postdoctoral fellowships during the early phases of this work.
Acknowledgments
We would like to acknowledge the original authors. A special acknowledgment to Dr. Fang-Cheng Yeh, as the creator of DSI Studio, who is always working hard to update the software and answer any questions relating to it.
Conflict of interest
The authors declare that the research was conducted in the absence of any commercial or financial relationships that could be construed as a potential conflict of interest.
Publisher’s note
All claims expressed in this article are solely those of the authors and do not necessarily represent those of their affiliated organizations, or those of the publisher, the editors and the reviewers. Any product that may be evaluated in this article, or claim that may be made by its manufacturer, is not guaranteed or endorsed by the publisher.
Supplementary material
The Supplementary Material for this article can be found online at: https://www.frontiersin.org/articles/10.3389/fnhum.2024.1432830/full#supplementary-material
References
Abel, K., Drake, R., and Goldstein, J. (2010). Sex differences in schizophrenia. Int. Rev. Psychiatry 22, 417–428. doi: 10.3109/09540261.2010.515205
Agartz, I., Sääf, J., Wahlund, L., and Wetterberg, L. (1992). Quantitative estimations of cerebrospinal fluid spaces and brain regions in healthy controls using computer-assisted tissue classification of magnetic resonance images: Relation to age and sex. Magn. Reson. Imaging 10, 217–226. doi: 10.1016/0730-725x(92)90482-f
Allen, J., Damasio, H., and Grabowski, T. (2002). Normal neuroanatomical variation in the human brain: An MRI-volumetric study. Am. J. Phys. Anthropol. 118, 341–358. doi: 10.1002/ajpa.10092
Andersson, J. L. R., Xu, J., Yacoub, E., Auerbach, E., Moeller, S., and Ugurbil, K. (2012). A comprehensive gaussian process framework for correcting distortions and movements in diffusion images. Proc. Intl. Soc. Mag. Reson. Med. 20:2426.
Andersson, J., and Sotiropoulos, S. (2016). An integrated approach to correction for off-resonance effects and subject movement in diffusion MR imaging. Neuroimage 125, 1063–1078. doi: 10.1016/j.neuroimage.2015.10.019
Andrushko, J., Gould, L., Renshaw, D., Ekstrand, C., Hortobágyi, T., Borowsky, R., et al. (2021). High force unimanual handgrip contractions increase ipsilateral sensorimotor activation and functional connectivity. Neuroscience 452, 111–125. doi: 10.1016/j.neuroscience.2020.10.031
Andrushko, J., Rinat, S., Kirby, Dahlby, J., Ekstrand, C., and Boyd, L. (2023). Females exhibit smaller volumes of brain activation and lower inter-subject variability during motor tasks. Sci. Rep. 13:17698. doi: 10.1038/s41598-023-44871-4
Arnegard, M., Whitten, L., Hunter, C., and Clayton, J. (2020). Sex as a biological variable: A 5-year progress report and call to action. J. Womens Health (Larchmt) 29, 858–864. doi: 10.1089/jwh.2019.8247
Azzarito, M., Ziegler, G., Huber, E., Grabher, P., Callaghan, M. F., Thompson, A., et al. (2021). Coherent waves of myelin plasticity during motor-skill learning. bioRxiv [Preprint]. doi: 10.1101/2021.04.13.439402
Barha, C., and Galea, L. (2010). Influence of different estrogens on neuroplasticity and cognition in the hippocampus. Biochim. Biophys. Acta 1800, 1056–1067. doi: 10.1016/j.bbagen.2010.01.006
Bartzokis, G. (2004). Quadratic trajectories of brain myelin content: Unifying construct for neuropsychiatric disorders. Neurobiol. Aging 25, 49–62. doi: 10.1016/j.neurobiolaging.2003.08.001
Baumeister, T., Kolind, S., MacKay, A., and McKeown, M. (2020). Inherent spatial structure in myelin water fraction maps. Magn. Reson. Imaging 67, 33–42. doi: 10.1016/j.mri.2019.09.012
Binkofski, F., and Buccino, G. (2006). The role of ventral premotor cortex in action execution and action understanding. J. Physiol. Paris 99, 396–405. doi: 10.1016/j.jphysparis.2006.03.005
Björnholm, L., Nikkinen, J., Kiviniemi, V., Nordström, T., Niemelä, S., Drakesmith, M., et al. (2017). Structural properties of the human corpus callosum: Multimodal assessment and sex differences. Neuroimage 152, 108–118. doi: 10.1016/j.neuroimage.2017.02.056
Borich, M., Brown, K., and Boyd, L. (2014). Motor skill learning is associated with diffusion characteristics of white matter in individuals with chronic stroke. J. Neurol. Phys. Ther. 38, 151–160. doi: 10.1097/NPT.0b013e3182a3d353
Brownsett, S., Carey, L., Copland, D., Walsh, A., and Sihvonen, A. (2024). Structural brain networks correlating with poststroke cognition. Hum. Brain Mapp. 45:e26665. doi: 10.1002/hbm.26665
Carey, J., Nader, N., Chai, P., Carreiro, S., Griswold, M., and Boyle, K. (2017). Drugs and medical devices: Adverse events and the impact on women’s health. Clin. Ther. 39, 10–22. doi: 10.1016/j.clinthera.2016.12.009
Catenaccio, E., Mu, W., and Lipton, M. (2016). Estrogen- and progesterone-mediated structural neuroplasticity in women: Evidence from neuroimaging. Brain Struct. Funct. 221, 3845–3867. doi: 10.1007/s00429-016-1197-x
Coffey, C., Lucke, J., Saxton, J., Ratcliff, G., Unitas, L., Billig, B., et al. (1998). Sex differences in brain aging: A quantitative magnetic resonance imaging study. Arch. Neurol. 55, 169–179. doi: 10.1001/archneur.55.2.169
Cousineau, M., Jodoin, P., Morency, F., Rozanski, V., Grand’Maison, M., Bedell, B., et al. (2017). A test-retest study on Parkinson’s PPMI dataset yields statistically significant white matter fascicles. Neuroimage Clin. 16, 222–233. doi: 10.1016/j.nicl.2017.07.020
Danielson, T., Gould, L., DeFreitas, J., MacLennan, R., Ekstrand, C., Borowsky, R., et al. (2024). Activity in the pontine reticular nuclei scales with handgrip force in humans. J. Neurophysiol. 131, 807–814. doi: 10.1152/jn.00407.2023
D’Arcy, R., Hamilton, A., Jarmasz, M., Sullivan, S., and Stroink, G. (2006). Exploratory data analysis reveals visuovisual interhemispheric transfer in functional magnetic resonance imaging. Magn. Reson. Med. 55, 952–958. doi: 10.1002/mrm.20839
Dayan, M., Monohan, E., Pandya, S., Kuceyeski, A., Nguyen, T., Raj, A., et al. (2015). Profilometry: A new statistical framework for the characterization of white matter pathways, with application to multiple sclerosis. Hum. Brain Mapp. 37, 989–1004. doi: 10.1002/hbm.23082
DeCasien, A., Guma, E., Liu, S., and Raznahan, A. (2022). Sex differences in the human brain: A roadmap for more careful analysis and interpretation of a biological reality. Biol. Sex Differ. 13:43. doi: 10.1186/s13293-022-00448-w
Delaparte, L., Yeh, F., Adams, P., Malchow, A., Trivedi, M., Oquendo, M., et al. (2017). A comparison of structural connectivity in anxious depression versus non-anxious depression. J. Psychiatr. Res. 89, 38–47. doi: 10.1016/j.jpsychires.2017.01.012
Deng, F., Zhao, L., Liu, C., Lu, M., Zhang, S., Huang, H., et al. (2018). Plasticity in deep and superficial white matter: A DTI study in world class gymnasts. Brain Struct. Funct. 223, 1849–1862. doi: 10.1007/s00429-017-1594-9
Dresang, H., Hula, W., Yeh, F., Warren, T., and Dickey, M. (2021). White-matter neuroanatomical predictors of aphasic verb retrieval. Brain Connect. 11, 319–330. doi: 10.1089/brain.2020.0921
Fabri, M., Pierpaoli, C., Barbaresi, P., and Polonara, G. (2014). Functional topography of the corpus callosum investigated by DTI and fMRI. World J. Radiol. 6, 895–906. doi: 10.4329/wjr.v6.i12.895
Ferretti, M., Iulita, M., Cavedo, E., Chiesa, P., Schumacher Dimech, A., Santuccione Chadha, A., et al. (2018). Sex differences in Alzheimer disease - the gateway to precision medicine. Nat. Rev. Neurol. 14, 457–469. doi: 10.1038/s41582-018-0032-9
Fields, R. (2010). Neuroscience. Change in the brain’s white matter. Science 330, 768–769. doi: 10.1126/science.1199139
Fields, R. D. (2015). A new mechanism of nervous system plasticity: Activity-dependent myelination. Nat. Rev. Neurosci. 16, 756–767. doi: 10.1038/nrn4023
Frizzell, T., Grajauskas, L., Liu, C., Ghosh Hajra, S., Song, X., and D’Arcy, R. (2020). White matter neuroplasticity: Motor learning activates the internal capsule and reduces hemodynamic response variability. Front. Hum. Neurosci. 14:509258. doi: 10.3389/fnhum.2020.509258
Frizzell, T., Phull, E., Khan, M., Song, X., Grajauskas, L., Gawryluk, J., et al. (2021). Imaging functional neuroplasticity in human white matter tracts. Brain Struct. Funct. 227, 381–392. doi: 10.1007/s00429-021-02407-4
Gawryluk, J., D’Arcy, R., Mazerolle, E., Brewer, K., and Beyea, S. (2011). Functional mapping in the corpus callosum: A 4T fMRI study of white matter. Neuroimage 54, 10–15. doi: 10.1016/j.neuroimage.2010.07.028
Gawryluk, J., Mazerolle, E., Beyea, S., and D’Arcy, R. (2014a). Functional MRI activation in white matter during the symbol digit modalities test. Front. Hum. Neurosci. 8:589. doi: 10.3389/fnhum.2014.00589
Gawryluk, J., Mazerolle, E., and D’Arcy, R. (2014b). Does functional MRI detect activation in white matter? A review of emerging evidence, issues, and future directions. Front Neurosci. 8:239. doi: 10.3389/fnins.2014.00239
Ghazi Sherbaf, F., Same, K., and Aarabi, M. (2018). High angular resolution diffusion imaging correlates of depression in Parkinson’s disease: A connectometry study. Acta Neurol. Belg. 118, 573–579. doi: 10.1007/s13760-018-0937-5
Golden, L., and Voskuhl, R. (2017). The importance of studying sex differences in disease: The example of multiple sclerosis. J. Neurosci. Res. 95, 633–643. doi: 10.1002/jnr.23955
Gorbet, D., and Sergio, L. (2007). Preliminary sex differences in human cortical BOLD fMRI activity during the preparation of increasingly complex visually guided movements. Eur. J. Neurosci. 25, 1228–1239. doi: 10.1111/j.1460-9568.2007.05358.x
Guadagnoli, M., and Lee, T. (2004). Challenge point: A framework for conceptualizing the effects of various practice conditions in motor learning. J. Mot. Behav. 36, 212–224. doi: 10.3200/JMBR.36.2.212-224
Guerrero-Gonzalez, J., Yeske, B., Kirk, G., Bell, M., Ferrazzano, P., and Alexander, A. (2022). Mahalanobis distance tractometry (MaD-Tract) - a framework for personalized white matter anomaly detection applied to TBI. Neuroimage 260:119475. doi: 10.1016/j.neuroimage.2022.119475
Guglielman, E. (2012). The ageing brain: Neuroplasticity and lifelong learning. eLearning Papers 29, 1–7.
Gupte, R., Brooks, W., Vukas, R., Pierce, J., and Harris, J. (2019). Sex differences in traumatic brain injury: What we know and what we should know. J. Neurotrauma 36, 3063–3091. doi: 10.1089/neu.2018.6171
Gur, R., Turetsky, B., Matsui, M., Yan, M., Bilker, W., Hughett, P., et al. (1999). Sex differences in brain gray and white matter in healthy young adults: Correlations with cognitive performance. J. Neurosci. 19, 4065–4072. doi: 10.1523/JNEUROSCI.19-10-04065.1999
Haier, R., Jung, R., Yeo, R., Head, K., and Alkire, M. (2005). The neuroanatomy of general intelligence: Sex matters. Neuroimage 25, 320–327. doi: 10.1016/j.neuroimage.2004.11.019
Hamaide, J., De Groof, G., and Van der Linden, A. (2016). Neuroplasticity and MRI: A perfect match. Neuroimage 131, 13–28. doi: 10.1016/j.neuroimage.2015.08.005
Hasan, K., Ewing-Cobbs, L., Kramer, L., Fletcher, J., and Narayana, P. (2008). Diffusion tensor quantification of the macrostructure and microstructure of human midsagittal corpus callosum across the lifespan. NMR Biomed. 21, 1094–1091. doi: 10.1002/nbm.1286
Haynes, N., and Goodwin, T. (2023). Literature review of sex differences in mTBI. Mil. Med. 188, e978–e984. doi: 10.1093/milmed/usab472
Huang, S., Li, M., Yeh, F., Huang, C., Zhang, H., and Liu, J. (2023). Differential and correlational tractography as tract-based biomarkers in mild traumatic brain injury: A longitudinal MRI study. NMR Biomed. 36:e4991. doi: 10.1002/nbm.4991
Hugdahl, K., Thomsen, T., and Ersland, L. (2006). Sex differences in visuo-spatial processing: An fMRI study of mental rotation. Neuropsychologia 44, 1575–1583. doi: 10.1016/j.neuropsychologia.2006.01.026
Hula, W., Panesar, S., Gravier, M., Yeh, F., Dresang, H., Dickey, M., et al. (2020). Structural white matter connectometry of word production in aphasia: An observational study. Brain 143, 2532–2544. doi: 10.1093/brain/awaa193
Islam, M., Luo, R., Valaris, S., Haley, E., Takase, H., Chen, Y., et al. (2020). Diffusion tensor-MRI detects exercise-induced neuroplasticity in the hippocampal microstructure in mice. Brain Plast. 5, 147–159. doi: 10.3233/BPL-190090
Jones, D., Knösche, T., and Turner, R. (2013). White matter integrity, fiber count, and other fallacies: The do’s and don’ts of diffusion MRI. Neuroimage 73, 239–254. doi: 10.1016/j.neuroimage.2012.06.081
Jung, R., Haier, R., Yeo, R., Rowland, L., Petropoulos, H., Levine, A., et al. (2005). Sex differences in N-acetylaspartate correlates of general intelligence: An 1H-MRS study of normal human brain. Neuroimage 26, 965–972. doi: 10.1016/j.neuroimage.2005.02.039
Kakei, S., Hoffman, D., and Strick, P. (2001). Direction of action is represented in the ventral premotor cortex. Nat. Neurosci. 4, 1020–1025. doi: 10.1038/nn726
Kesselring, J. (2015). Neuroplasticity – basis for lifelong learning. Eur. Neurol. Rev. 9:143. doi: 10.17925/ENR.2014.09.02.143
Kim, J., Lee, K., Roh, H., Ahn, M., and Hwang, H. (2010). Gender differences in the functional recovery after acute stroke. J. Clin. Neurol. 6, 183–188. doi: 10.3988/jcn.2010.6.4.183
Kirby, E. D., Andrushko, J., Rinat, S., D’Arcy, R., and Boyd, L. (2024). Investigating female versus male differences in white matter neuroplasticity associated with complex visuo-motor learning. Sci. Rep. 14:5951. doi: 10.1038/s41598-024-56453-z
Kirby, E. D., Frizzell, T., Grajauskas, L., Song, X., Gawryluk, J., Lakhani, B., et al. (2022). Increased myelination plays a central role in white matter neuroplasticity. Neuroimage 263:119644. doi: 10.1016/j.neuroimage.2022.119644
Koch-Henriksen, N., and Sørensen, P. (2010). The changing demographic pattern of multiple sclerosis epidemiology. Lancet Neurol. 9, 520–532. doi: 10.1016/S1474-4422(10)70064-8
Kopec, A., Smith, C., Ayre, N., Sweat, S., and Bilbo, S. (2018). Microglial dopamine receptor elimination defines sex-specific nucleus accumbens development and social behavior in adolescent rats. Nat. Commun. 9:3769. doi: 10.1038/s41467-018-06118-z
Koschutnig, K., Weber, B., and Fink, A. (2023). Tidying up white matter: Neuroplastic transformations in sensorimotor tracts following slackline skill acquisition. OpenNeuro doi: 10.18112/openneuro.ds003138.v1.0.1
Lakhani, B., Borich, M., Jackson, J., Wadden, K., Peters, S., Villamayor, A., et al. (2016). Motor skill acquisition promotes human brain myelin plasticity. Neural Plast. 2016:7526135. doi: 10.1155/2016/7526135
Laube, C., van den Bos, W., and Fandakova, Y. (2020). The relationship between pubertal hormones and brain plasticity: Implications for cognitive training in adolescence. Dev. Cogn. Neurosci. 42:100753. doi: 10.1016/j.dcn.2020.100753
Li, M., Yeh, F., Huang, S., Huang, C., Zhang, H., and Liu, J. (2022). Differential tractography and correlation tractography findings on patients with mild traumatic brain injury: A pilot Study. Front. Hum. Neurosci. 16:751902. doi: 10.3389/fnhum.2022.751902
Lillard, A., and Erisir, A. (2011). Old dogs learning new tricks: Neuroplasticity beyond the juvenile period. Dev. Rev. 31, 207–239. doi: 10.1016/j.dr.2011.07.008
Lissek, S., Hausmann, M., Knossalla, F., Peters, S., Nicolas, V., Güntürkün, O., et al. (2007). Sex differences in cortical and subcortical recruitment during simple and complex motor control: An fMRI study. Neuroimage 37, 912–926. doi: 10.1016/j.neuroimage.2007.05.037
Liu, F., Vidarsson, L., Winter, J., Tran, H., and Kassner, A. (2010). Sex differences in the human corpus callosum microstructure: A combined T2 myelin-water and diffusion tensor magnetic resonance imaging study. Brain Res. 1343, 37–45. doi: 10.1016/j.brainres.2010.04.064
Luders, E., and Kurth, F. (2020). Structural differences between male and female brains. Handb. Clin. Neurol. 175, 3–11. doi: 10.1016/B978-0-444-64123-6.00001-1
Marrocco, J., and McEwen, B. (2016). Sex in the brain: Hormones and sex differences. Dialogues Clin. Neurosci. 18, 373–383. doi: 10.31887/DCNS.2016.18.4/jmarrocco
Mazerolle, E., Beyea, S., Gawryluk, J., Brewer, K., Bowen, C., and D’Arcy, R. (2010). Confirming white matter fMRI activation in the corpus callosum: Co-localization with DTI tractography. Neuroimage 50, 616–621. doi: 10.1016/j.neuroimage.2009.12.102
Mazerolle, E., D’Arcy, R., and Beyea, S. (2008). Detecting functional magnetic resonance imaging activation in white matter: Interhemispheric transfer across the corpus callosum. BMC Neurosci. 9:84. doi: 10.1186/1471-2202-9-84
McEwen, B., and Milner, T. (2017). Understanding the broad influence of sex hormones and sex differences in the brain. J. Neurosci. Res. 95, 24–39. doi: 10.1002/jnr.23809
Meoni, S., Macerollo, A., and Moro, E. (2020). Sex differences in movement disorders. Nat. Rev. Neurol. 16, 84–96. doi: 10.1038/s41582-019-0294-x
Mikolić, A., van Klaveren, D., Groeniger, J., Wiegers, E., Lingsma, H., Zeldovich, M., et al. (2021). Differences between men and women in treatment and outcome after traumatic brain injury. J. Neurotrauma 38, 235–251. doi: 10.1089/neu.2020.7228
Mojtahed Zadeh, M., Ashraf-Ganjouei, A., Ghazi Sherbaf, F., Haghshomar, M., and Aarabi, M. (2018). White matter tract alterations in drug-naïve parkinson’s disease patients with impulse control disorders. Front. Neurol. 9:163. doi: 10.3389/fneur.2018.00163
Moore, E., Schaefer, R., Bastin, M., Roberts, N., and Overy, K. (2017). Diffusion tensor MRI tractography reveals increased fractional anisotropy (FA) in arcuate fasciculus following music-cued motor training. Brain Cogn. 116, 40–46. doi: 10.1016/j.bandc.2017.05.001
Moshchin, M., Cheng, K., Osting, S., Laluzerne, M., Hurley, S., Singh, A., et al. (2023). Quantifying changes in local basal ganglia structural connectivity in the 6-hydroxydopamine model of Parkinson’s Disease using correlational tractography. Int. IEEE EMBS Conf. Neural Eng. 2023:10123839. doi: 10.1109/ner52421.2023.10123839
Narayana, P. (2017). White matter changes in patients with mild traumatic brain injury: MRI perspective. Concussion 2:CNC35. doi: 10.2217/cnc-2016-0028
Pawluski, J., Lambert, K., and Kinsley, C. (2016). Neuroplasticity in the maternal hippocampus: Relation to cognition and effects of repeated stress. Horm. Behav. 77, 86–97. doi: 10.1016/j.yhbeh.2015.06.004
Persky, R., Turtzo, L., and McCullough, L. (2010). Stroke in women: Disparities and outcomes. Curr. Cardiol. Rep. 12, 6–13. doi: 10.1007/s11886-009-0080-2
Peters, A. (2002). The effects of normal aging on myelin and nerve fibers: A review. J. Neurocytol. 31, 581–593. doi: 10.1023/a:1025731309829
Peters, S., Lim, S., Louie, D., Yang, C., and Eng, J. (2020). Passive, yet not inactive: Robotic exoskeleton walking increases cortical activation dependent on task. J. Neuroeng. Rehabil. 17:107. doi: 10.1186/s12984-020-00739-6
Pfleiderer, B., Ohrmann, P., Suslow, T., Wolgast, M., Gerlach, A., Heindel, W., et al. (2004). N-acetylaspartate levels of left frontal cortex are associated with verbal intelligence in women but not in men: A proton magnetic resonance spectroscopy study. Neuroscience 123, 1053–1058. doi: 10.1016/j.neuroscience.2003.11.008
Phan, H., Reeves, M., Blizzard, C., Thrift, A., Cadilhac, D., Sturm, J., et al. (2019). Sex differences in severity of stroke in the INSTRUCT study: A meta-analysis of individual participant data. J. Am. Heart Assoc. 8:e010235. doi: 10.1161/JAHA.118.010235
Prvulovic, D., Van de Ven, V., Sack, A., Maurer, K., and Linden, D. (2005). Functional activation imaging in aging and dementia. Psychiatry Res. 140, 97–113. doi: 10.1016/j.pscychresns.2005.06.006
Purves, D., Augustine, G. J., Fitzpatrick, D., Katz, L. C., LaMantia, A.-S., McNamara, J. O., et al. (2001). The premotor cortex: Neuroscience, 2nd Edn. Sunderland, MA: Sinauer Associates.
Rechlin, R., Splinter, T., Hodges, T., Albert, A., and Galea, L. (2022). An analysis of neuroscience and psychiatry papers published from 2009 and 2019 outlines opportunities for increasing discovery of sex differences. Nat. Commun. 13:2137. doi: 10.1038/s41467-022-29903-3
Reich, D., Smith, S., Zackowski, K., Gordon-Lipkin, E., Jones, C., Farrell, J., et al. (2007). Multiparametric magnetic resonance imaging analysis of the corticospinal tract in multiple sclerosis. Neuroimage 38, 271–279. doi: 10.1016/j.neuroimage.2007.07.049
Reid, L., Sale, M., Cunnington, R., Mattingley, J., and Rose, S. (2017). Brain changes following four weeks of unimanual motor training: Evidence from fMRI-guided diffusion MRI tractography. Hum. Brain Mapp. 38, 4302–4312. doi: 10.1002/hbm.23514
Ritchie, S., Cox, S., Shen, X., Lombardo, M., Reus, L., Alloza, C., et al. (2018). Sex Differences in the Adult Human Brain: Evidence from 5216 UK Biobank Participants. Cereb. Cortex. 28, 2959–2975. doi: 10.1093/cercor/bhy109
Rogojin, A., Gorbet, D., and Sergio, L. (2023). Sex differences in the neural underpinnings of unimanual and bimanual control in adults. Exp. Brain Res. 241, 793–806. doi: 10.1007/s00221-023-06561-5
Rosenkrantz, T., Hussain, Z., and Fitch, R. (2019). Sex differences in brain injury and repair in newborn infants: Clinical evidence and biological mechanisms. Front. Pediatr. 7:211. doi: 10.3389/fped.2019.00211
Sale, M., Reid, L., Cocchi, L., Pagnozzi, A., Rose, S., and Mattingley, J. (2017). Brain changes following four weeks of unimanual motor training: Evidence from behavior, neural stimulation, cortical thickness, and functional MRI. Hum. Brain Mapp. 38, 4773–4787. doi: 10.1002/hbm.23710
Saleki, K., Banazadeh, M., Saghazadeh, A., and Rezaei, N. (2022). Aging, testosterone, and neuroplasticity: Friend or foe? Rev. Neurosci. 34, 247–273. doi: 10.1515/revneuro-2022-0033
Sampaio-Baptista, C., and Johansen-Berg, H. (2017). White matter plasticity in the adult brain. Neuron 96, 1239–1251. doi: 10.1016/j.neuron.2017.11.026
Sanchez-Catasus, C., Bohnen, N., D’Cruz, N., and Müller, M. (2022). Striatal acetylcholine-dopamine imbalance in Parkinson disease: In vivo neuroimaging study with dual-tracer PET and dopaminergic PET-informed correlational tractography. J. Nucl. Med. 63, 438–445. doi: 10.2967/jnumed.121.261939
Sanchez-Catasus, C., Bohnen, N., Yeh, F., D’Cruz, N., Kanel, P., and Müller, M. (2021). Dopaminergic nigrostriatal connectivity in early Parkinson disease: In vivo neuroimaging study of 11C-DTBZ PET combined with correlational tractography. J. Nucl. Med. 62, 545–552. doi: 10.2967/jnumed.120.248500
Schmithorst, V. J. (2009). DEVELOPMENTAL SEX DIFFERENCES IN THE RELATION OF NEUROANATOMICAL CONNECTIVITY TO INTELLIGENCE. Intelligence 37, 164–173. doi: 10.1016/j.intell.2008.07.001
Schmithorst, V., and Holland, S. (2006). Functional MRI evidence for disparate developmental processes underlying intelligence in boys and girls. Neuroimage 31, 1366–1379. doi: 10.1016/j.neuroimage.2006.01.010
Schmithorst, V., and Holland, S. (2007). Sex differences in the development of neuroanatomical functional connectivity underlying intelligence found using Bayesian connectivity analysis. Neuroimage 35, 406–419. doi: 10.1016/j.neuroimage.2006.11.046
Schmithorst, V., Holland, S., and Dardzinski, B. (2008). Developmental differences in white matter architecture between boys and girls. Hum. Brain Mapp. 29, 696–710. doi: 10.1002/hbm.20431
Scholz, J., Klein, M., Behrens, T., and Johansen-Berg, H. (2009). Training induces changes in white-matter architecture. Nat. Neurosci. 12, 1370–1371. doi: 10.1038/nn.2412
Shen, C., Tyan, Y., Kuo, L., Wu, C., and Weng, J. (2015). Quantitative evaluation of rabbit brain injury after cerebral hemisphere radiation exposure using generalized q-sampling imaging. PLoS One 10:e0133001. doi: 10.1371/journal.pone.0133001
Shin, Y., Kim, D., Ha, T., Park, H., Moon, W., Chung, E., et al. (2005). Sex differences in the human corpus callosum: Diffusion tensor imaging study. Neuroreport 16, 795–798. doi: 10.1097/00001756-200505310-00003
Shulman, L. M. (2007). Gender differences in Parkinson’s disease. Gender Med. 4, 8–18. doi: 10.1016/S1550-8579(07)80003-9
Takao, H., Hayashi, N., and Ohtomo, K. (2014). Sex dimorphism in the white matter: Fractional anisotropy and brain size. J. Magn. Reson. Imaging 39, 917–923. doi: 10.1002/jmri.24225
Tannenbaum, C., Ellis, R., Eyssel, F., Zou, J., and Schiebinger, L. (2019). Sex and gender analysis improves science and engineering. Nature 575, 137–146. doi: 10.1038/s41586-019-1657-6
Tardif, C., Gauthier, C., Steele, C., Bazin, P., Schäfer, A., Schaefer, A., et al. (2016). Advanced MRI techniques to improve our understanding of experience-induced neuroplasticity. Neuroimage 131, 55–72. doi: 10.1016/j.neuroimage.2015.08.047
Taubert, M., Draganski, B., Anwander, A., Müller, K., Horstmann, A., Villringer, A., et al. (2010). Dynamic properties of human brain structure: Learning-related changes in cortical areas and associated fiber connections. J. Neurosci. 30, 11670–11677. doi: 10.1523/JNEUROSCI.2567-10.2010
Tettamanti, M., Paulesu, E., Scifo, P., Maravita, A., Fazio, F., Perani, D., et al. (2002). Interhemispheric transmission of visuomotor information in humans: fMRI evidence. J. Neurophysiol. 88, 1051–1058. doi: 10.1152/jn.2002.88.2.1051
Tomita, H., Hagii, J., Metoki, N., Saito, S., Shiroto, H., Hitomi, H., et al. (2015). Impact of sex difference on severity and functional outcome in patients with cardioembolic stroke. J. Stroke Cerebrovasc. Dis. 24, 2613–2618. doi: 10.1016/j.jstrokecerebrovasdis.2015.07.016
Toschi, N., Gisbert, R., Passamonti, L., Canals, S., and De Santis, S. (2020). Multishell diffusion imaging reveals sex-specific trajectories of early white matter degeneration in normal aging. Neurobiol. Aging 86, 191–200. doi: 10.1016/j.neurobiolaging.2019.11.014
Van Ruitenbeek, P., Santos Monteiro, T., Chalavi, S., King, B., Cuypers, K., Sunaert, S., et al. (2023). Interactions between the aging brain and motor task complexity across the lifespan: Balancing brain activity resource demand and supply. Cereb. Cortex 33, 6420–6434. doi: 10.1093/cercor/bhac514
Vien, C., Boré, A., Lungu, O., Benali, H., Carrier, J., Fogel, S., et al. (2016). Age-related white-matter correlates of motor sequence learning and consolidation. Neurobiol. Aging 48, 13–22. doi: 10.1016/j.neurobiolaging.2016.08.006
Voskuhl, R. (2020). The effect of sex on multiple sclerosis risk and disease progression. Mult. Scler. 26, 554–560. doi: 10.1177/1352458519892491
Wang, H., Wang, N., and Yeh, F. (2019). Specifying the diffusion MRI connectome in Chinese-speaking children with developmental dyslexia and auditory processing deficits. Pediatr. Neonatol. 60, 297–304. doi: 10.1016/j.pedneo.2018.07.016
Wang, Y., Adamson, C., Yuan, W., Altaye, M., Rajagopal, A., Byars, A., et al. (2012). Sex differences in white matter development during adolescence: A DTI study. Brain Res. 1478, 1–15. doi: 10.1016/j.brainres.2012.08.038
Weber, B., Koschutnig, K., Schwerdtfeger, A., Rominger, C., Papousek, I., Weiss, E., et al. (2019). learning unicycling evokes manifold changes in gray and white matter networks related to motor and cognitive functions. Sci. Rep. 9:4324. doi: 10.1038/s41598-019-40533-6
Weber, B., Treyer, V., Oberholzer, N., Jaermann, T., Boesiger, P., Brugger, P., et al. (2005). Attention and interhemispheric transfer: A behavioral and fMRI study. J. Cogn. Neurosci. 17, 113–123. doi: 10.1162/0898929052880002
Westerhausen, R., Walter, C., Kreuder, F., Wittling, R., Schweiger, E., and Wittling, W. (2003). The influence of handedness and gender on the microstructure of the human corpus callosum: A diffusion-tensor magnetic resonance imaging study. Neurosci. Lett. 351, 99–102. doi: 10.1016/j.neulet.2003.07.011
White, J., Tannenbaum, C., Klinge, I., Schiebinger, L., and Clayton, J. (2021). The integration of sex and gender considerations into biomedical research: Lessons from international funding agencies. J. Clin. Endocrinol. Metab. 106, 3034–3048. doi: 10.1210/clinem/dgab434
Wierenga, L., Doucet, G., Dima, D., Agartz, I., Aghajani, M., Akudjedu, T., et al. (2020). Greater male than female variability in regional brain structure across the lifespan. Hum. Brain Mapp. 43, 470–499. doi: 10.1002/hbm.25204
Wierenga, L., Ruigrok, A., Aksnes, E., Barth, C., Beck, D., Burke, S., et al. (2024). Recommendations for a better understanding of sex and gender in the neuroscience of mental health. Biol. Psychiatry Glob. Open Sci. 4:100283. doi: 10.1016/j.bpsgos.2023.100283
Wnuk, A., Davis, A., Parks, C., Halber, D., Kelly, D., Zyla, G., et al. (2018). BrainFacts: A primer on the brain and nervous system. The society of neuroscience. Washington DC: BrainFacts.
Wright, D., Kellermann, A., Hertzberg, V., Clark, P., Frankel, M., Goldstein, F., et al. (2007). ProTECT: A randomized clinical trial of progesterone for acute traumatic brain injury. Ann. Emerg. Med. 49:e1–e2. doi: 10.1016/j.annemergmed.2006.07.932
Wright, D., Symons, G., O’Brien, W., McDonald, S., Zamani, A., Major, B., et al. (2021). Diffusion imaging reveals sex differences in the white matter following sports-related concussion. Cereb. Cortex 31, 4411–4419. doi: 10.1093/cercor/bhab095
Wulf, G., Shea, C., and Lewthwaite, R. (2010). Motor skill learning and performance: A review of influential factors. Med. Educ. 44, 75–84. doi: 10.1111/j.1365-2923.2009.03421.x
Yeatman, J., Dougherty, R., Myall, N., Wandell, B., and Feldman, H. (2012). Tract profiles of white matter properties: Automating fiber-tract quantification. PLoS One 7:e49790. doi: 10.1371/journal.pone.0049790
Yeh, F., and Tseng, W. Y. (2011). NTU-90: A high angular resolution brain atlas constructed by q-space diffeomorphic reconstruction. Neuroimage 58, 91–99. doi: 10.1016/j.neuroimage.2011.06.021
Yeh, F., Badre, D., and Verstynen, T. (2016). Connectometry: A statistical approach harnessing the analytical potential of the local connectome. Neuroimage 125, 162–171. doi: 10.1016/j.neuroimage.2015.10.053
Yeh, F., Liu, L., Hitchens, T., and Wu, Y. (2017). Mapping immune cell infiltration using restricted diffusion MRI. Magn. Reson. Med. 77, 603–612. doi: 10.1002/mrm.26143
Yeh, F., Zaydan, I., Suski, V., Lacomis, D., Richardson, R., Maroon, J., et al. (2019b). Differential tractography as a track-based biomarker for neuronal injury. Neuroimage 202:116131. doi: 10.1016/j.neuroimage.2019.116131
Yeh, F., Panesar, S., Barrios, J., Fernandes, D., Abhinav, K., Meola, A., et al. (2019a). Automatic removal of false connections in diffusion MRI tractography using topology-informed pruning (TIP). Neurotherapeutics 16, 52–58. doi: 10.1007/s13311-018-0663-y
Yeh, F., Panesar, S., Fernandes, D., Meola, A., Yoshino, M., Fernandez-Miranda, J., et al. (2018). Population-averaged atlas of the macroscale human structural connectome and its network topology. Neuroimage 178, 57–68. doi: 10.1016/j.neuroimage.2018.05.027
Yeh, F., Tang, P., and Tseng, W. (2013a). Diffusion MRI connectometry automatically reveals affected fiber pathways in individuals with chronic stroke. Neuroimage Clin. 2, 912–921. doi: 10.1016/j.nicl.2013.06.014
Yeh, F., Verstynen, T. D., Wang, Y., Fernández-Miranda, J. C., and Tseng, W. Y. I. (2013b). Deterministic diffusion fiber tracking improved by quantitative anisotropy. PLoS One 8:e80713. doi: 10.1371/journal.pone.0080713
Yeh, F., Wedeen, V., and Tseng, W. (2010). Generalized q-sampling imaging. IEEE Trans. Med. Imaging 29, 1626–1635. doi: 10.1109/TMI.2010.2045126
Zatorre, R., Fields, R., and Johansen-Berg, H. (2012). Plasticity in gray and white: Neuroimaging changes in brain structure during learning. Nat. Neurosci. 15, 528–536. doi: 10.1038/nn.3045
Zhang, H., Wang, Y., Lu, T., Qiu, B., Tang, Y., Ou, S., et al. (2013). Differences between generalized q-sampling imaging and diffusion tensor imaging in the preoperative visualization of the nerve fiber tracts within peritumoral edema in brain. Neurosurgery 73, 1044–53; discussion 1053. doi: 10.1227/NEU.0000000000000146
Keywords: white matter, neuroplasticity, motor learning, correlational tractography, MRI
Citation: Kirby ED, Andrushko JW, Boyd LA, Koschutnig K and D’Arcy RCN (2024) Sex differences in patterns of white matter neuroplasticity after balance training in young adults. Front. Hum. Neurosci. 18:1432830. doi: 10.3389/fnhum.2024.1432830
Received: 14 May 2024; Accepted: 08 August 2024;
Published: 27 August 2024.
Edited by:
Ilan Laufer, Ariel University, IsraelReviewed by:
Elif Keles, Northwestern Medicine, United StatesVijay Venkatraman, The University of Melbourne, Australia
Copyright © 2024 Kirby, Andrushko, Boyd, Koschutnig and D’Arcy. This is an open-access article distributed under the terms of the Creative Commons Attribution License (CC BY). The use, distribution or reproduction in other forums is permitted, provided the original author(s) and the copyright owner(s) are credited and that the original publication in this journal is cited, in accordance with accepted academic practice. No use, distribution or reproduction is permitted which does not comply with these terms.
*Correspondence: Ryan C. N. D’Arcy, ryan@healthtechconnex.com