- 1Automatic Control and Systems Engineering, Neuroscience Institute, Insigneo Institute, University of Sheffield, Sheffield, United Kingdom
- 2Department of Brain Sciences, Imperial College London, London, United Kingdom
- 3School of Psychology, University College Dublin, Dublin, Ireland
- 4Department of Experimental Psychology, University of Oxford, Oxford, United Kingdom
- 5Oxford Centre for Human Brain Activity, Wellcome Centre for Integrative Neuroimaging, Department of Psychiatry, University of Oxford, Oxford, United Kingdom
- 6Turner Institute for Brain and Mental Health and School of Psychological Sciences, Monash University, Melbourne, VIC, Australia
Non-invasive brain stimulation (NIBS) is a complex and multifaceted approach to modulating brain activity and holds the potential for broad accessibility. This work discusses the mechanisms of the four distinct approaches to modulating brain activity non-invasively: electrical currents, magnetic fields, light, and ultrasound. We examine the dual stochastic and deterministic nature of brain activity and its implications for NIBS, highlighting the challenges posed by inter-individual variability, nebulous dose-response relationships, potential biases and neuroanatomical heterogeneity. Looking forward, we propose five areas of opportunity for future research: closed-loop stimulation, consistent stimulation of the intended target region, reducing bias, multimodal approaches, and strategies to address low sample sizes.
1 Introduction
The human brain, comprising approximately 80 billion neurons and a similar number of glial cells (Herculano-Houzel, 2009), is a highly complex organ. The brain consumes 20% of the body's metabolic budget while constituting only 2% of its mass (Sokoloff, 1960). The outsized cardiovascular intake of the brain sustains its many parallel processes, estimated at (0.18–6.4) × 1014 traversed edges per second (Katja, 2015). Neuromodulatory interventions aim to deliver targeted perturbations of these neural processes, identifying the optimal targets and methods poses a significant challenge. Additionally, neuromodulation must take into account the cerebrospinal fluid, sulci and gyri, meninges, skull, and scalp in which the brain is ensconced. Yet the ability to modulate brain activity without surgery, both in the cortex and in deep brain structures, has been demonstrated through a broad spectrum of approaches (Polańıa et al., 2018).
Non-invasive brain stimulation (NIBS) is a promising approach for improving brain health and quality of life. Moreover, when appropriately configured it presents minimal risks and costs when compared to invasive brain stimulation (Najib and Horvath, 2014; Bikson et al., 2016; Cassano et al., 2022; Qin et al., 2023). Greater patient acceptability facilitates the translation of NIBS to a broader range of applications. For instance, patients with moderate Parkinson's disease consider invasive deep-brain stimulation to be a last resort (Sperens et al., 2017). In a survey of the general public, ultrasound and magnetic stimulation were preferred over pharmaceuticals and implants as an intervention for mental health (Atkinson-Clement et al., 2024).
Applications of NIBS include personalized, intermittent theta-burst transcranial magnetic stimulation (TMS) which has been approved by the Federal Drug Administration (Tarr, 2023) and boasts a 79% remission rate for patients with treatment-resistant depression (Cole et al., 2022). Transcranial electrical stimulation (tES) is being trialed by the National Health Service in the United Kingdom for the treatment of depression (Medtech innovation briefing, 2023). The treatment of drug addiction has promise in preliminary trials using low-intensity focused ultrasound stimulation (LIFUS) (Mahoney et al., 2023). NIBS has also been used for the reduction of chronic pain (Che et al., 2021) and the enhancement of attention (Brosnan et al., 2018) and memory (Grover et al., 2022) in the aging brain.
NIBS shows promise as a therapeutic intervention for various neurological and psychiatric conditions for both its wide-ranging neuromodulatory capabilities and the accessibility of large-scale manufacturing. The manufacturing of NIBS devices relies largely on the existing electronics manufacturing industry, as a result, it has the potential to scale quickly. This could potentially facilitate adoption curves akin to those seen for blood oxygen sensors or consumer electronics products within the respective patient groups. At this scale and speed, NIBS could have a substantial, translational impact. However, the realization of NIBS's potential is contingent upon surmounting several significant barriers. In this work, we introduce different approaches to NIBS and their mechanisms of action, describe major challenges facing the field, and explore opportunities to overcome them.
2 Approaches to non-invasive brain stimulation
The four primary approaches to non-invasive brain stimulation are transcranial electrical stimulation (tES), transcranial magnetic stimulation (TMS), transcranial photobiomodulation (tPBM) and transcranial ultrasound stimulation (TUS). Figure 1 provides an overview of their respective characteristics.
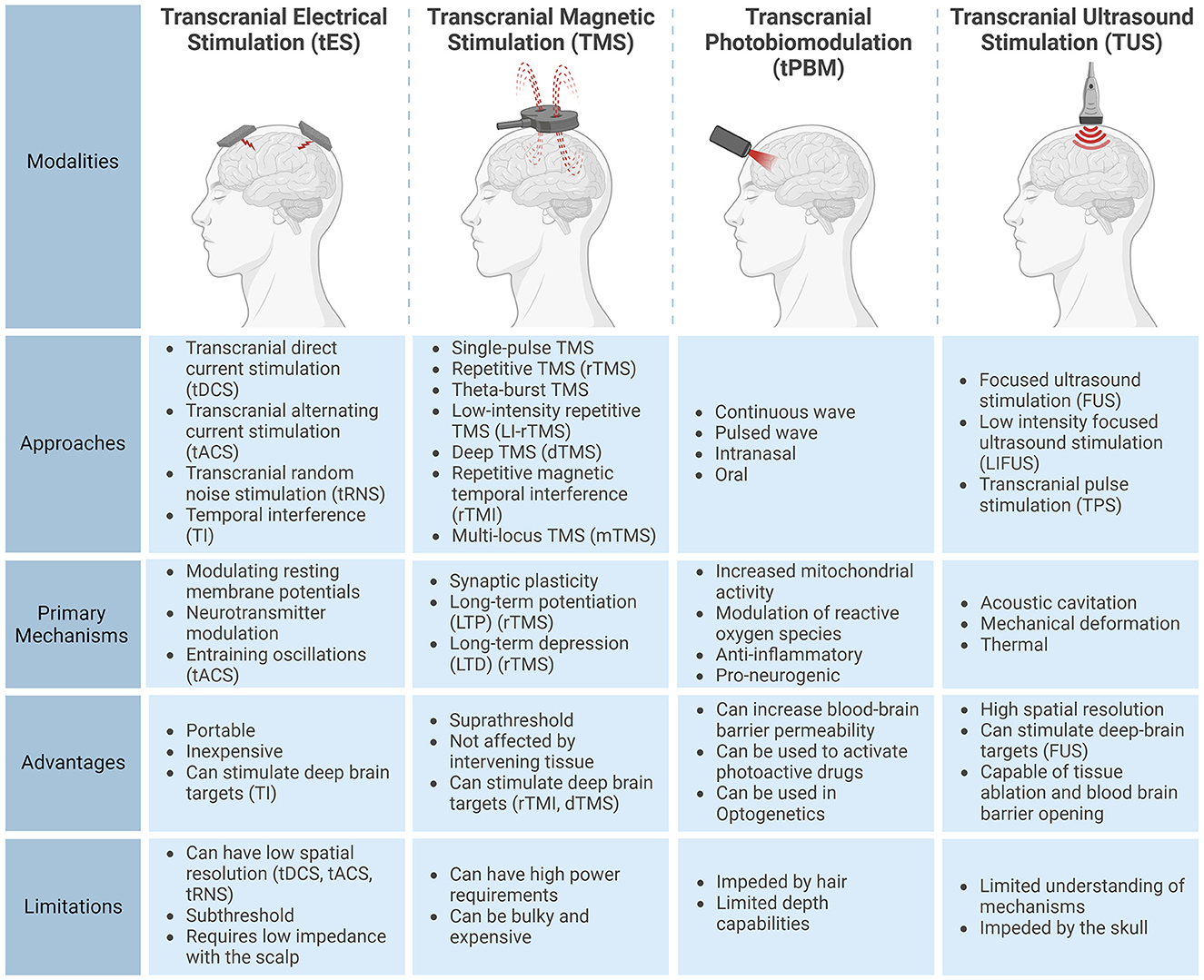
Figure 1. Characteristics of the four non-invasive brain stimulation modalities: transcranial electrical stimulation (tES), transcranial magnetic stimulation (TMS), transcranial photobiomodulation (tPBM), and transcranial ultrasound stimulation (TUS). This figure outlines common subtypes, primary mechanisms, advantages, and limitations for each modality. Created with BioRender.com.
Transcranial electrical stimulation (tES) passes a current (typically 1-4mA) through active and return electrodes (Thair et al., 2017). Stimulation is typically delivered via two electrodes, although more electrodes can enhance stimulation focality (Datta et al., 2009). Transcranial direct current stimulation (tDCS), a type of tES, modulates neuronal excitability without reaching the threshold necessary to trigger neuronal depolarizations (Bikson et al., 2004). This alteration of membrane potentials has been shown to occur through several mechanisms, including decreased γ-aminobutyric acid (GABA) concentrations (Stagg et al., 2011) and increased glutamate and glutamine concentrations (Hunter et al., 2015) with effects modulated by sodium channels (Nitsche et al., 2003; Alagapan et al., 2016; Reed and Cohen Kadosh, 2018).
tES also comes in other forms, such as Transcranial Alternating Current Stimulation (tACS) which utilizes sinusoidal currents thought to entrain brain oscillations (Antal et al., 2008; Kanai et al., 2008). Additionally, Transcranial Random Noise Stimulation (tRNS) is the random sampling of currents from a bell-shaped curve (Terney et al., 2008). The mechanisms for tRNS are unclear, but they may involve stochastic resonance (Stacey and Durand, 2000). In contrast to tDCS, aftereffects of tRNS are not NDMA receptor-dependent and can be suppressed by benzodiazepines, suggesting an alternative mechanism (Chaieb et al., 2015).
Temporal Interference (TI) stimulation employs the convergence of two or more high-frequency (kHz) currents to create a low-frequency envelope that selectively modulates brain activity. Importantly, the high-frequency carrier currents, though present, do not impact neuronal activity due to the low-pass filtering effect of neuronal membranes (Hutcheon and Yarom, 2000). This generates a focused electric field (E-field) that oscillates at the difference in frequency of these high-frequency waves (Grossman et al., 2017). Notably, recent human studies have successfully demonstrated TI in humans, including the targeted modulation of the hippocampus (Violante et al., 2023).
Transcranial magnetic stimulation (TMS) uses electromagnets to induce currents within the brain, producing supra-threshold E-fields on the order of hundreds of V/m (Bijsterbosch et al., 2012) compared to less than 1V/m with tES (Evans et al., 2020). Approaches to TMS includes single-pulse TMS for the exploration of brain function and repetitive TMS (rTMS) used to induce effects lasting longer than the stimulation period (Klomjai et al., 2015). TMS can induce long-term potentiation (LTP) through the unblocking of post-synaptic NDMA receptors blocked by magnesium ions resulting in an influx of calcium ions in post-synaptic neurons (Cooke and Bliss, 2006). Additionally, long-term depression (LTD) can be induced through the slow flow of calcium ions from lower-frequency stimulation (Chervyakov et al., 2015). TMS can also alter gene expression and enzyme production along with a multitude of other effects (Chervyakov et al., 2015). Notably, rTMS effects are frequency-dependent, with inhibition below 1Hz and facilitation above 5Hz, although this generalization is disputed (Prei et al., 2023).
The application of TMS involves diverse pulse patterns, tailored to specific purposes. One notable example is theta-burst TMS, showing promise in treating major depressive disorder. This method employs short bursts of 50Hz stimulation to mimic the effects of LTP and LTD, thought to induce synaptic plasticity while offering shorter, lower-intensity procedures than traditional rTMS (Chung et al., 2015). Low-Intensity Repetitive TMS (LI-rTMS) uses an order of magnitude weaker magnetic field strengths (1-150mT) and remains subthreshold, unlike typical TMS that can evoke motor potentials (Barker et al., 1985; Moretti and Rodger, 2022). Furthermore, deep brain regions can be stimulated with deep TMS (dTMS) using a specialized coil known as the Hesed coil (Zangen et al., 2005). Additionally, repetitive magnetic temporal interference (rTMI), which utilizes the electrotemporal interference of high-frequency TMS holds promise for selectively modulating deep-brain targets (Khalifa et al., 2023). Finally, multi-locus TMS (mTMS) is an approach that uses an array of electronically controlled coils, allowing for the shifting and re-orienting of the induced E-field (Koponen et al., 2018).
Transcranial Photobiomodulation (tPBM) commonly employs near-infrared light (600 nm–1,100 nm) to modulate brain activity (Salehpour et al., 2018). Light that reaches the brain is absorbed by chromophores, particularly Cytochrome c oxidase, increasing mitochondrial activity and Adenosine triphosphate production. This also modulates reactive oxygen species and the release of calcium ions, activating transcription factors for long-term cellular effects (Caldieraro and Cassano, 2019). Resultant biological responses include improved oxidative metabolism and increased blood flow (Caldieraro and Cassano, 2019). tPBM is also thought to have anti-inflammatory and pro-neurogenic effects (Cassano et al., 2016). However, the absorption of light by hair limits tPBM applications outside of the forehead region, intranasally or through the oral cavity (Salehpour et al., 2018; Askalsky and Iosifescu, 2019). While tPBM traditionally uses a continuous wave, recent work explores pulsed wave tPBM, allowing for short, high-power pulses (Tang et al., 2023).
Thus far conclusive evidence regarding the efficacy of tPBM for depression and dementia is yet to emerge (Salehpour et al., 2021; Vieira et al., 2023). However, there are encouraging results regarding the effects of tPBM on cognitive abilities and brain function during aging (Dole et al., 2023). Aside from modulating neural activity directly, a picosecond 532nm pulse has been observed as temporarily improving blood-brain barrier permeability (Li et al., 2021), enhancing the delivery and efficacy of paclitaxel in mice with glioblastoma (Cai et al., 2023). Additionally, advances in photopharmacology offer the potential to precisely control pharmaceuticals within the brain using light (Velema et al., 2014).
Transcranial ultrasound stimulation (TUS) uses high-frequency sound waves for non-invasive neural modulation. It is employed in various applications, including the increase of blood-brain barrier permeability via cavitation (Hynynen et al., 2001) and tissue ablation through heating (Martin et al., 2009). Alternatively, low-intensity ultrasound has been demonstrated to modulate brain activity. Examples include the attenuation of thermal pain sensitivity (Badran et al., 2020) and sensory-evoked brain oscillations (Legon et al., 2014). Focused ultrasound stimulation (FUS) uses out-of-phase high-frequency waves to stimulate deep brain regions with millimeter precision (Ghanouni et al., 2015). Low-intensity focused ultrasound (LIFUS) is the application of ultrasound stimulation at low intensities to modulate neuronal activity without causing tissue damage. Thermal effects are thought to be negligible from LIFUS as tissue temperatures rise by less than 0.1°C. Non-thermal effects from LIFUS include stable acoustic cavitation, which involves pressure-induced oscillations of gas-filled microbubbles and influencing neuron excitability via membrane deformation. Additionally, LIFUS can alter ion channel permeability and membrane characteristics through mechanical energy, impacting ion flux and neuronal membrane discharge from mechanosensitive ion channels. These complex interactions are thought to contribute to the neuromodulatory effects of LIFUS (Fomenko et al., 2018). Transcranial pulsed stimulation (TPS) employs short pulses of ultrasound lasting 3μs. These short pulses mitigate brain heating and preliminary evidence suggests cognitive benefits in Alzheimer's patients (Beisteiner et al., 2020).
3 Obstacles to effective non-invasive brain stimulation
The brain can be considered a dynamical, emergent complex system (Turkheimer et al., 2022). Put simply, ongoing brain function is a result of the interplay between exogenous stimuli/perturbations and endogenous processes and goals. The brain presents both stochastic and deterministic characteristics, underpinning the difficulty in developing a mechanistic understanding of brain function and behavior. While the causal perturbations of NIBS facilitate the characterization of neural mechanisms, the complex, individual nature of brain structure and function makes the optimization of NIBS challenging. These sources of variation are illustrated in Figure 2.
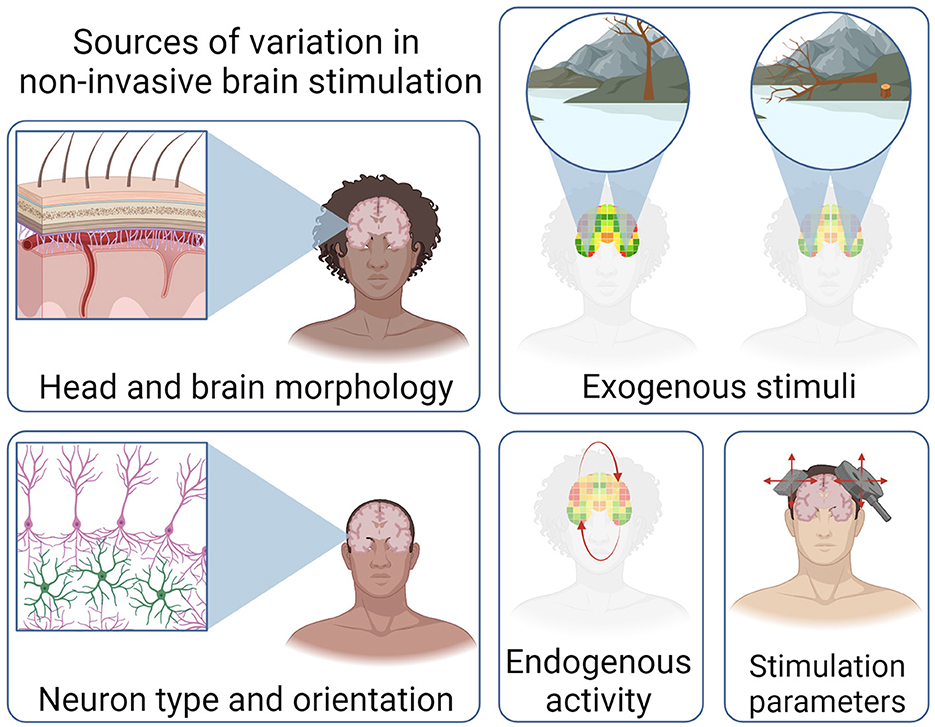
Figure 2. Illustration of the sources of variation that influence the efficacy of non-invasive brain stimulation. Variations in head and brain morphology, exogenous stimuli, neuron type and orientation, as well activity and stimulation parameters. Created with BioRender.com.
One source of complexity is the inter-individual variation in head and brain morphology. For instance, skull thickness (Lillie et al., 2016) and cortical thickness, volume, and surface area are negatively correlated with age (Lemaitre et al., 2012). In adults, ventricles within the brain become larger with age and both white and gray matter volumes are reduced (Bethlehem et al., 2022). These inter-individual and intra-individual variations are particularly consequential in tES. For example, current flow modeling has demonstrated that identical tES parameters result in an over 100% difference in the intensity of the E-field generated in the motor cortex (Evans et al., 2020). There is also the potential for unintentional stimulation of off-target regions, especially when individualized modeling is not performed (Soleimani et al., 2023).
The correlation between the applied stimulation dose and the resulting response remains unclear. In tPBM, a biphasic dose-response curve is suggested (Yang M. et al., 2021). Conversely, a positive relationship between the dose of TUS applied to the lateral geniculate nucleus and the subsequent suppression of visually evoked potentials has been observed (Mohammadjavadi et al., 2022). Regarding tDCS, a simple linear dose-response relationship may be an oversimplification (Esmaeilpour et al., 2018). Dose-response relationships across NIBS remain unclear, likely due to a complex interplay of factors including the functional state of the brain during stimulation.
The functional state of the brain during stimulation is an important area of consideration when optimizing NIBS (Bradley et al., 2022). For instance, responses to tES are associated with preexisting concentrations of the neurotransmitters GABA and glutamate (Filmer et al., 2019). However, the specific nature of the brain-state dependent dose-response relationship in tES remains inadequately understood (Lee et al., 2021). Similarly, FUS differentially affects active and resting neurons (Yang P.-F. et al., 2021). The use of electroencephalography (EEG) for measuring TMS evoked potentials has proven effective in optimizing stimulation orientation in the left pre-supplementary motor area (Tervo et al., 2022). Additionally, it enables real-time adjustment of stimulation protocols to control the efficacy of plasticity induction in the motor cortex (Zrenner et al., 2018). As with NIBS more broadly, applying these state-dependent techniques beyond the motor system is an additional challenge.
The type and orientation of neurons being stimulated is another complicating factor in elucidating dose-response relationships. For instance, when FUS is applied, neurons exhibit varying responses to different pulse frequencies, influenced by their distinct action potential waveforms and genotypes (Yu et al., 2021). An important factor of the efficacy of tES is the orientation of neurons relative to the E-field generated. Additionally, neurons with highly-branched axons are less sensitive to the relative direction of the E-field (Chung et al., 2022). Interestingly, stimulation itself can also change structural components of the brain. For instance, subthreshold rTMS alters the dendritic spine structure in pyramidal neurons in mice (Tang et al., 2021) and static magnetic stimulation through the use of a permanent magnet has been shown to decrease the length of the axon initial segment in cell cultures (Beros et al., 2022). In the development of stimulation configurations, modeling current flow allows for a comprehensive understanding of the interplay between stimulation montage, E-field, and neural orientation (Lee et al., 2021). Further developments in multi-scale modeling may elucidate these relationships.
It is common practice in NIBS to focus on stimulating a single brain region, even though many targeted behaviors involve a distributed network of activity. For instance, in depressed populations, the prefrontal cortex is often targeted, but depression symptoms are linked to activity changes in various brain regions including the thalamus and hippocampus among others (Zhang et al., 2018). Consequently, achieving optimal stimulation parameters may necessitate simultaneous stimulation of multiple targets. Although progress has been made in this direction (Fischer et al., 2017; Corlier et al., 2021), it remains a relatively unexplored area.
There are a multitude of other factors that may impact the efficacy of NIBS, from circadian rhythms (Vergallito et al., 2022) to caffeine (Zulkifly et al., 2021) and broader genetic predispositions (Hasan et al., 2013). Brain temperature variations linked to age, sex, and menstrual cycle (Rzechorzek et al., 2022) may also impact NIBS. As with all experimental studies, excluding common disorders like dyslexia or ADHD limits the generalizability of findings from healthy controls, given that 15-20% of the population is neurodivergent (Doyle, 2020). Further, underdiagnosis in certain groups, such as females with ADHD (Young et al., 2020), may result in the inadvertent inclusion of undiagnosed neurodivergent individuals. Overly broad exclusion criteria can introduce population bias; insufficient accommodations for individuals who wear head coverings or exhibit sensory processing sensitivity may hinder diverse participation. Many studies, excluding those focusing on disorders prevalent in older adults, predominantly involve young participants and are conducted in a limited number of countries (Sun et al., 2022; Medeiros et al., 2023; Wang et al., 2023).
4 Areas of opportunity and future research directions
The effects of NIBS are state-dependent, making adaptive stimulation attuned to brain dynamics crucial for maximizing the potential of NIBS (Bergmann, 2018). In a closed-loop NIBS system, brain activity is continuously monitored and compared to a desired state, and stimulation parameters are adjusted in real-time to optimize the therapeutic effects. To facilitate a greater understanding of the mechanisms and predictors of NIBS efficacy, multi-scale models will be informed by multi-scale imaging from synapse to lobe (D'Angelo and Jirsa, 2022). Ultimately, state-dependent neuromodulation could be delivered in more wearable form. EEG captures ongoing brain states non-invasively and has enabled tACS delivered to match the phase and frequency of slow-wave oscillations to enhance long-term generalized memory consolidation post-sleep (Ketz et al., 2018). Additionally, high-definition diffuse optical tomography provides high spatial resolution capture of hemodynamics in the cortex (Vidal-Rosas et al., 2021) and Optically Pumped Magnetometers-Magnetoencephalography (OPM-MEG) enables high spatial and temporal resolutions and sensitivity (Brookes et al., 2022). Optimizing stimulation parameters based on real-time brain state is both necessary and attainable.
Another central limitation is ensuring consistent stimulation of the intended target. Electrode montages are chosen to stimulate a target region, in practice the position and intensity of stimulation in the brain varies between individuals and sessions. Precise sensor and stimulator positioning is crucial for accuracy (Shirazi and Huang, 2019; Caulfield et al., 2022). Achieving such precision involves structural imaging, simulation, and neuronavigation, a resource-intensive process. More scalable approaches are needed, potentially combining subject-specific MRI approximated with generative modeling (Tudosiu et al., 2022) and utilizing smartphone-based 3D head scanning (Everitt et al., 2023). Additionally, techniques such as electrical impedance tomography and pulse-echo ultrasound can estimate the electrical and acoustic properties of the head, respectively (Fernández-Corazza et al., 2017; He et al., 2021). Notably, advances in low-field MRI may offer more accessible structural imaging with 64mT mobile scans approaching the quality of 3T scans (Lucas et al., 2023). Statistical techniques that account for inter-individual variability, such as mixed-model analyses or cluster analysis, may also be used as opposed to the standard population-based approaches (Vergallito et al., 2022).
One source of bias in NIBS research is rooted in the challenges associated with gaining access to the scalp, especially when dealing with long, thick, and/or curly hair (Louis et al., 2022). There is a recognized bias in neuroscience concerning race and sex (Kwasa et al., 2023). Addressing these biases could involve implementing inclusive training and recruitment procedures. Technological solutions, such as 3D-printed electrodes designed for various hair types, may also contribute to mitigating these challenges (Xing and Casson, 2023). Additionally, offering additional remuneration for participants with hair that requires more time for preparation and washing is a consideration. To foster more representative research samples, further open-sourcing of datasets containing demographic information is recommended for the benefit of future researchers.
The efficacy of NIBS could potentially be enhanced by combining stimulation modalities as each approach offers unique benefits and mechanisms of action. For instance, priming with tDCS before applying TMS may produce more robust effects (Hurley and Machado, 2017). Similarly, concerning closed-loop NIBS, multi-modal approaches for reading brain activity can be more effective at classifying brain states as has been demonstrated by the combination of EEG and functional Near Infrared Spectroscopy to improve seizure detection accuracy (Sirpal et al., 2019). Multi-modality may potentially extend to non-invasive optogenetics, with recent work showing the delivery of viral vectors facilitated by FUS to increase blood-brain barrier permeability, followed by channel activation using light in mice (Pouliopoulos et al., 2022). As such, it may be pertinent for further advancement of multi-modal sensing and stimulation.
Finally, statistical power emerges as a noteworthy concern in NIBS research. The average sample size in studies involving tDCS and TMS is 22, leading to a situation where NIBS studies may miss 50% of true positive results (Mitra et al., 2019). While practical constraints often limit the sample size of initial studies, enhancing statistical power through adequately powered studies is crucial. In instances where inter-individual variability is not accounted for through brain imaging and simulation, broader utilization of “N-of-1” trials with a single individual with openly available data, might be a pertinent approach for NIBS research as it is with personalized medicine more broadly (Schork, 2015). The aggregation of such studies has the potential to offer valuable insights beyond the conventional population-based approach as the inter-individual source of variability is not a concern. This could also allow for greater use of longitudinal study designs, that investigate the long-term effects of multi-session stimulation.
5 Discussion
In this paper, we have explored the multifaceted landscape of NIBS, delving into the four main modalities: tES, TMS, tPBM, and TUS. Each modality possesses unique attributes, mechanisms and challenges, contributing differently to the field of neurostimulation. Yet all must deal with the inherent complexities and challenges associated with individual morphological and physiological variations, as well as the dynamic nature of the brain and the subsequent state-dependent nature of stimulation.
Looking forward, this paper highlights several avenues for advancing NIBS. The development of closed-loop systems that integrate real-time brain state monitoring with stimulation holds the promise of enhancing efficacy. Overcoming the challenge of consistently stimulating the intended brain regions across individuals necessitates innovative approaches, potentially leveraging advances in imaging technologies and computational modeling. Addressing biases in NIBS research and its applications, including those related to race, sex, and neurodiversity, is critical for ensuring the broad applicability and effectiveness of these technologies. Furthermore, exploring the synergistic potential of multi-modal NIBS approaches may improve outcomes. Finally, tackling the issue of underpowered studies through adequately sized trials, personalization and appropriate statistical methods. Implementing these recommendations could help propel NIBS toward achieving its full therapeutic potential across diverse populations.
Data availability statement
The original contributions presented in the study are included in the article/supplementary material, further inquiries can be directed to the corresponding author.
Author contributions
JT: Conceptualization, Visualization, Writing – original draft, Writing – review & editing. DK: Writing – review & editing. MB: Supervision, Writing – review & editing. MA: Supervision, Writing – review & editing.
Funding
The author(s) declare that financial support was received for the research, authorship, and/or publication of this article. This research was supported by the University of Sheffield, United Kingdom and the Engineering and Physical Sciences Research Council (EPSRC) Doctoral Training Partnership [grant number EP/M508135/1].
Conflict of interest
The authors declare that the research was conducted in the absence of any commercial or financial relationships that could be construed as a potential conflict of interest.
Publisher's note
All claims expressed in this article are solely those of the authors and do not necessarily represent those of their affiliated organizations, or those of the publisher, the editors and the reviewers. Any product that may be evaluated in this article, or claim that may be made by its manufacturer, is not guaranteed or endorsed by the publisher.
References
Alagapan, S., Schmidt, S. L., Lefebvre, J., Hadar, E., Shin, H. W., and Fröhlich, F. (2016). Modulation of cortical oscillations by low-frequency direct cortical stimulation is state-dependent. PLoS Biol. 14:e1002424. doi: 10.1371/journal.pbio.1002424
Antal, A., Boros, K., Poreisz, C., Chaieb, L., Terney, D., and Paulus, W. (2008). Comparatively weak after-effects of transcranial alternating current stimulation (tacs) on cortical excitability in humans. Brain Stimul. 1, 97–105. doi: 10.1016/j.brs.2007.10.001
Askalsky, P., and Iosifescu, D. V. (2019). Transcranial photobiomodulation for the management of depression: current perspectives. Neuropsychiatr. Dis. Treatm. 15, 3255–3272. doi: 10.2147/NDT.S188906
Atkinson-Clement, C., Junor, A., and Kaiser, M. (2024). A large-scale online survey of patients and the general public: preferring safe and noninvasive neuromodulation for mental health. medRxiv [Preprint] 2024–01. doi: 10.1101/2024.01.10.24301043
Badran, B. W., Caulfield, K. A., Stomberg-Firestein, S., Summers, P. M., Dowdle, L. T., Savoca, M., et al. (2020). Sonication of the anterior thalamus with mri-guided transcranial focused ultrasound (tfus) alters pain thresholds in healthy adults: A double-blind, sham-controlled study. Brain Stimul. 13, 1805–1812. doi: 10.1016/j.brs.2020.10.007
Barker, A. T., Jalinous, R., and Freeston, I. L. (1985). Non-invasive magnetic stimulation of human motor cortex. The Lancet 325, 1106–1107. doi: 10.1016/S0140-6736(85)92413-4
Beisteiner, R., Matt, E., Fan, C., Baldysiak, H., Schönfeld, M., Philippi Novak, T., et al. (2020). Transcranial pulse stimulation with ultrasound in alzheimer's disease a new navigated focal brain therapy. Adv. Sci. 7:1902583. doi: 10.1002/advs.201902583
Bergmann, T. O. (2018). Brain state-dependent brain stimulation. Front. Psychol. 9:2108. doi: 10.3389/fpsyg.2018.02108
Beros, J. L., King, E. S., Clarke, D., Jaeschke-Angi, L., Rodger, J., and Tang, A. D. (2024). Static magnetic stimulation induces structural plasticity at the axon initial segment of inhibitory cortical neurons. Sci. Rep. 14:1479. doi: 10.1038/s41598-024-51845-7
Bethlehem, R. A., Seidlitz, J., White, S. R., Vogel, J. W., Anderson, K. M., Adamson, C., et al. (2022). Brain charts for the human lifespan. Nature 604, 525–533. doi: 10.1038/s41586-022-04554-y
Bijsterbosch, J. D., Barker, A. T., Lee, K.-H., and Woodruff, P. W. (2012). Where does transcranial magnetic stimulation (tms) stimulate? Modelling of induced field maps for some common cortical and cerebellar targets. Med. Biol. Eng. Comput. 50, 671–681. doi: 10.1007/s11517-012-0922-8
Bikson, M., Grossman, P., Thomas, C., Zannou, A. L., Jiang, J., Adnan, T., et al. (2016). Safety of transcranial direct current stimulation: evidence based update 2016. Brain Stimul. 9, 641–661. doi: 10.1016/j.brs.2016.06.004
Bikson, M., Inoue, M., Akiyama, H., Deans, J. K., Fox, J. E., Miyakawa, H., et al. (2004). Effects of uniform extracellular dc electric fields on excitability in rat hippocampal slices in vitro. J. Physiol. 557, 175–190. doi: 10.1113/jphysiol.2003.055772
Bradley, C., Nydam, A. S., Dux, P. E., and Mattingley, J. B. (2022). State-dependent effects of neural stimulation on brain function and cognition. Nat. Rev. Neurosci. 23, 459–475. doi: 10.1038/s41583-022-00598-1
Brookes, M. J., Leggett, J., Rea, M., Hill, R. M., Holmes, N., Boto, E., et al. (2022). Magnetoencephalography with optically pumped magnetometers (OPM-MEG): the next generation of functional neuroimaging. Trends Neurosci. 45, 621–634. doi: 10.1016/j.tins.2022.05.008
Brosnan, M. B., Arvaneh, M., Harty, S., Maguire, T., O'Connell, R., Robertson, I. H., et al. (2018). Prefrontal modulation of visual processing and sustained attention in aging, a tdcs-eeg coregistration approach. J. Cogn. Neurosci. 30, 1630–1645. doi: 10.1162/jocn_a_01307
Cai, Q., Li, X., Xiong, H., Fan, H., Gao, X., Vemireddy, V., et al. (2023). Optical blood-brain-tumor barrier modulation expands therapeutic options for glioblastoma treatment. Nat. Communic. 14:4934. doi: 10.1038/s41467-023-40579-1
Caldieraro, M. A., and Cassano, P. (2019). Transcranial and systemic photobiomodulation for major depressive disorder: a systematic review of efficacy, tolerability and biological mechanisms. J. Affect. Disor. 243, 262–273. doi: 10.1016/j.jad.2018.09.048
Cassano, P., Norton, R., Caldieraro, M. A., Vahedifard, F., Vizcaino, F., McEachern, K. M., et al. (2022). “Tolerability and safety of transcranial photobiomodulation for mood and anxiety disorders,” in Photonics (MDPI), 507. doi: 10.3390/photonics9080507
Cassano, P., Petrie, S. R., Hamblin, M. R., Henderson, T. A., and Iosifescu, D. V. (2016). Review of transcranial photobiomodulation for major depressive disorder: targeting brain metabolism, inflammation, oxidative stress, and neurogenesis. Neurophotonics 3, 031404–031404. doi: 10.1117/1.NPh.3.3.031404
Caulfield, K. A., Fleischmann, H. H., Cox, C. E., Wolf, J. P., George, M. S., and McTeague, L. M. (2022). Neuronavigation maximizes accuracy and precision in tms positioning: Evidence from 11,230 distance, angle, and electric field modeling measurements. Brain Stimul. 15, 1192–1205. doi: 10.1016/j.brs.2022.08.013
Chaieb, L., Antal, A., and Paulus, W. (2015). Transcranial random noise stimulation-induced plasticity is nmda-receptor independent but sodium-channel blocker and benzodiazepines sensitive. Front. Neurosci. 9:125. doi: 10.3389/fnins.2015.00125
Che, X., Cash, R. F., Luo, X., Luo, H., Lu, X., Xu, F., et al. (2021). High-frequency rtms over the dorsolateral prefrontal cortex on chronic and provoked pain: a systematic review and meta-analysis. Brain Stimul. 14, 1135–1146. doi: 10.1016/j.brs.2021.07.004
Chervyakov, A. V., Chernyavsky, A. Y., Sinitsyn, D. O., and Piradov, M. A. (2015). Possible mechanisms underlying the therapeutic effects of transcranial magnetic stimulation. Front. Hum. Neurosci. 9:303. doi: 10.3389/fnhum.2015.00303
Chung, H., Im, C., Seo, H., and Jun, S. C. (2022). Key factors in the cortical response to transcranial electrical stimulations a multi-scale modeling study. Comput. Biol. Med. 144:105328. doi: 10.1016/j.compbiomed.2022.105328
Chung, S. W., Hoy, K. E., and Fitzgerald, P. B. (2015). Theta-burst stimulation: A new form of tms treatment for depression? Depr. Anxiety 32, 182–192. doi: 10.1002/da.22335
Cole, E. J., Phillips, A. L., Bentzley, B. S., Stimpson, K. H., Nejad, R., Barmak, F., et al. (2022). Stanford neuromodulation therapy (snt): a double-blind randomized controlled trial. Am. J. Psychiat. 179, 132–141. doi: 10.1176/appi.ajp.2021.20101429
Cooke, S. F., and Bliss, T. V. (2006). Plasticity in the human central nervous system. Brain 129, 1659–1673. doi: 10.1093/brain/awl082
Corlier, J., Wilson, A., Tadayonnejad, R., Ginder, N., Levitt, J., Krantz, D., et al. (2021). Multi-target repetitive transcranial magnetic stimulation (rtms) protocol for the treatment of comorbid depression and chronic pain. Brain Stimul. 14, 1729–1730. doi: 10.1016/j.brs.2021.10.468
D'Angelo, E., and Jirsa, V. (2022). The quest for multiscale brain modeling. Trends Neurosci. 45, 777–790. doi: 10.1016/j.tins.2022.06.007
Datta, A., Bansal, V., Diaz, J., Patel, J., Reato, D., and Bikson, M. (2009). Gyri-precise head model of transcranial direct current stimulation: improved spatial focality using a ring electrode versus conventional rectangular pad. Brain Stimul. 2, 201–207. doi: 10.1016/j.brs.2009.03.005
Dole, M., Auboiroux, V., Langar, L., and Mitrofanis, J. (2023). A systematic review of the effects of transcranial photobiomodulation on brain activity in humans. Rev. Neurosci. 34:3. doi: 10.1515/revneuro-2023-0003
Doyle, N. (2020). Neurodiversity at work: a biopsychosocial model and the impact on working adults. Br. Med. Bull. 135:108. doi: 10.1093/bmb/ldaa021
Esmaeilpour, Z., Marangolo, P., Hampstead, B. M., Bestmann, S., Galletta, E., Knotkova, H., et al. (2018). Incomplete evidence that increasing current intensity of tdcs boosts outcomes. Brain Stimul. 11, 310–321. doi: 10.1016/j.brs.2017.12.002
Evans, C., Bachmann, C., Lee, J. S., Gregoriou, E., Ward, N., and Bestmann, S. (2020). Dose-controlled tdcs reduces electric field intensity variability at a cortical target site. Brain Stimul. 13, 125–136. doi: 10.1016/j.brs.2019.10.004
Everitt, A., Richards, H., Song, Y., Smith, J., Kobylarz, E., Lukovits, T., et al. (2023). Eeg electrode localization with 3d iphone scanning using point-cloud electrode selection (pc-es). J. Neural Eng. 20:066033. doi: 10.1088/1741-2552/ad12db
Fernández-Corazza, M., Turovets, S., Luu, P., Price, N., Muravchik, C. H., and Tucker, D. (2017). Skull modeling effects in conductivity estimates using parametric electrical impedance tomography. IEEE Trans. Biomed. Eng. 65, 1785–1797. doi: 10.1109/TBME.2017.2777143
Filmer, H. L., Ehrhardt, S. E., Bollmann, S., Mattingley, J. B., and Dux, P. E. (2019). Accounting for individual differences in the response to tdcs with baseline levels of neurochemical excitability. Cortex 115, 324–334. doi: 10.1016/j.cortex.2019.02.012
Fischer, D. B., Fried, P. J., Ruffini, G., Ripolles, O., Salvador, R., Banus, J., et al. (2017). Multifocal tdcs targeting the resting state motor network increases cortical excitability beyond traditional tdcs targeting unilateral motor cortex. Neuroimage 157, 34–44. doi: 10.1016/j.neuroimage.2017.05.060
Fomenko, A., Neudorfer, C., Dallapiazza, R. F., Kalia, S. K., and Lozano, A. M. (2018). Low-intensity ultrasound neuromodulation: an overview of mechanisms and emerging human applications. Brain Stimul. 11, 1209–1217. doi: 10.1016/j.brs.2018.08.013
Ghanouni, P., Pauly, K. B., Elias, W. J., Henderson, J., Sheehan, J., Monteith, S., et al. (2015). Transcranial mr-guided focused ultrasound: a review of the technology and neuro applications. AJR 205:150. doi: 10.2214/AJR.14.13632
Grossman, N., Bono, D., Dedic, N., Kodandaramaiah, S. B., Rudenko, A., Suk, H.-J., et al. (2017). Noninvasive deep brain stimulation via temporally interfering electric fields. Cell 169, 1029–1041. doi: 10.1016/j.cell.2017.05.024
Grover, S., Wen, W., Viswanathan, V., Gill, C. T., and Reinhart, R. M. (2022). Long-lasting, dissociable improvements in working memory and long-term memory in older adults with repetitive neuromodulation. Nat. Neurosci. 25, 1237–1246. doi: 10.1038/s41593-022-01132-3
Hasan, A., Misewitsch, K., Nitsche, M. A., Gruber, O., Padberg, F., Falkai, P., et al. (2013). Impaired motor cortex responses in non-psychotic first-degree relatives of schizophrenia patients: a cathodal tdcs pilot study. Brain Stimul. 6, 821–829. doi: 10.1016/j.brs.2013.03.001
He, C., Jing, Y., and Han, A. (2021). Human skull profile and speed of sound estimation using pulse-echo ultrasound signals with deep learning. J. Acoustical Soc. Am. 150, A33–A33. doi: 10.1121/10.0007527
Herculano-Houzel, S. (2009). The human brain in numbers: a linearly scaled-up primate brain. Front. Hum. Neurosci. 3:31. doi: 10.3389/neuro.09.031.2009
Hunter, M. A., Coffman, B. A., Gasparovic, C., Calhoun, V. D., Trumbo, M. C., and Clark, V. P. (2015). Baseline effects of transcranial direct current stimulation on glutamatergic neurotransmission and large-scale network connectivity. Brain Res. 1594, 92–107. doi: 10.1016/j.brainres.2014.09.066
Hurley, R., and Machado, L. (2017). Using tdcs priming to improve brain function: Can metaplasticity provide the key to boosting outcomes? Neurosci. Biobehav. Rev. 83, 155–159. doi: 10.1016/j.neubiorev.2017.09.029
Hutcheon, B., and Yarom, Y. (2000). Resonance, oscillation and the intrinsic frequency preferences of neurons. Trends Neurosci. 23, 216–222. doi: 10.1016/S0166-2236(00)01547-2
Hynynen, K., McDannold, N., Vykhodtseva, N., and Jolesz, F. A. (2001). Noninvasive mr imaging-guided focal opening of the blood-brain barrier in rabbits. Radiology 220, 640–646. doi: 10.1148/radiol.2202001804
Kanai, R., Chaieb, L., Antal, A., Walsh, V., and Paulus, W. (2008). Frequency-dependent electrical stimulation of the visual cortex. Current. Biol. 18, 1839–1843. doi: 10.1016/j.cub.2008.10.027
Katja, G. (2015). AI Impacts - Brain performance in TEPS. Available online at: https://aiimpacts.org/brain-performance-in-teps/ (accessed February 11, 2024).
Ketz, N., Jones, A. P., Bryant, N. B., Clark, V. P., and Pilly, P. K. (2018). Closed-loop slow-wave tacs improves sleep-dependent long-term memory generalization by modulating endogenous oscillations. J. Neurosci. 38, 7314–7326. doi: 10.1523/JNEUROSCI.0273-18.2018
Khalifa, A., Abrishami, S. M., Zaeimbashi, M., Tang, A. D., Coughlin, B., Rodger, J., et al. (2023). Magnetic temporal interference for noninvasive and focal brain stimulation. J. Neural Eng. 20:016002. doi: 10.1088/1741-2552/acb015
Klomjai, W., Katz, R., and Lackmy-Vallée, A. (2015). Basic principles of transcranial magnetic stimulation (TMS) and repetitive tms (RTMS). Ann. Phys. Rehabilit. Med. 58, 208–213. doi: 10.1016/j.rehab.2015.05.005
Koponen, L. M., Nieminen, J. O., and Ilmoniemi, R. J. (2018). Multi-locus transcranial magnetic stimulation theory and implementation. Brain Stimul. 11, 849–855. doi: 10.1016/j.brs.2018.03.014
Kwasa, J., Peterson, H. M., Karrobi, K., Jones, L., Parker, T., Nickerson, N., et al. (2023). Demographic reporting and phenotypic exclusion in fnirs. Front. Neurosci. 17:1086208. doi: 10.3389/fnins.2023.1086208
Lee, J., Bestmann, S., and Evans, C. (2021). A future of current flow modelling for transcranial electrical stimulation? Curr. Behav. Neurosci. Rep. 8, 150–159. doi: 10.1007/s40473-021-00238-5
Legon, W., Sato, T. F., Opitz, A., Mueller, J., Barbour, A., Williams, A., et al. (2014). Transcranial focused ultrasound modulates the activity of primary somatosensory cortex in humans. Nat. Neurosci. 17, 322–329. doi: 10.1038/nn.3620
Lemaitre, H., Goldman, A. L., Sambataro, F., Verchinski, B. A., Meyer-Lindenberg, A., Weinberger, D. R., et al. (2012). Normal age-related brain morphometric changes: nonuniformity across cortical thickness, surface area and gray matter volume? Neurobiol. Aging 33, 617–e1. doi: 10.1016/j.neurobiolaging.2010.07.013
Li, X., Vemireddy, V., Cai, Q., Xiong, H., Kang, P., Li, X., et al. (2021). Reversibly modulating the blood-brain barrier by laser stimulation of molecular-targeted nanoparticles. Nano Lett. 21, 9805–9815. doi: 10.1021/acs.nanolett.1c02996
Lillie, E. M., Urban, J. E., Lynch, S. K., Weaver, A. A., and Stitzel, J. D. (2016). Evaluation of skull cortical thickness changes with age and sex from computed tomography scans. J. Bone Mineral Res. 31, 299–307. doi: 10.1002/jbmr.2613
Louis, C. C., Webster, C. T., Gloe, L. M., and Moser, J. S. (2022). Hair me out: Highlighting systematic exclusion in psychophysiological methods and recommendations to increase inclusion. Front. Hum. Neurosci. 16:1058953. doi: 10.3389/fnhum.2022.1058953
Lucas, A., Arnold, T. C., Okar, S. V., Vadali, C., Kawatra, K. D., Ren, Z., et al. (2023). Multi-contrast high-field quality image synthesis for portable low-field mri using generative adversarial networks and paired data. medRxiv 2023–12. doi: 10.1101/2023.12.28.23300409
Mahoney, J. J., Thompson-Lake, D. G., Ranjan, M., Marton, J. L., Carpenter, J. S., Zheng, W., et al. (2023). Low-intensity focused ultrasound targeting the bilateral nucleus accumbens as a potential treatment for substance use disorder: a first-in-human report. Biol. Psychiat. 94, e41–e43. doi: 10.1016/j.biopsych.2023.06.031
Martin, E., Jeanmonod, D., Morel, A., Zadicario, E., and Werner, B. (2009). High-intensity focused ultrasound for noninvasive functional neurosurgery. Ann. Neurol. 66, 858–861. doi: 10.1002/ana.21801
Medeiros, W., Barros, T., and Caixeta, F. V. (2023). Bibliometric mapping of non-invasive brain stimulation techniques (nibs) for fluent speech production. Front. Hum. Neurosci. 17:1164890. doi: 10.3389/fnhum.2023.1164890
Medtech innovation briefing (2023). Flow transcranial direct current stimulation for treating depression [NICE 2023]. Available obnline at: https://www.nice.org.uk/advice/mib324/ (accessed February 11, 2024).
Mitra, S., Mehta, U. M., Binukumar, B., Venkatasubramanian, G., and Thirthalli, J. (2019). Statistical power estimation in non-invasive brain stimulation studies and its clinical implications: An exploratory study of the meta-analyses. Asian J. Psychiat. 44, 29–34. doi: 10.1016/j.ajp.2019.07.006
Mohammadjavadi, M., Ash, R. T., Li, N., Gaur, P., Kubanek, J., Saenz, Y., et al. (2022). Transcranial ultrasound neuromodulation of the thalamic visual pathway in a large animal model and the dose-response relationship with mr-arfi. Sci. Rep. 12:19588. doi: 10.1038/s41598-022-20554-4
Moretti, J., and Rodger, J. (2022). A little goes a long way: neurobiological effects of low intensity RTMS and implications for mechanisms of rtms. Curr. Res. Neurobiol. 3:100033. doi: 10.1016/j.crneur.2022.100033
Najib, U., and Horvath, J. C. (2014). “Transcranial magnetic stimulation (tms) safety considerations and recommendations,” in Transcranial Magnetic Stimulation. Neuromethods, eds. A. Rotenberg, J. Horvath, A. Pascual-Leone (New York, NY: Humana Press). doi: 10.1007/978-1-4939-0879-0_2
Nitsche, M., Fricke, K., Henschke, U., Schlitterlau, A., Liebetanz, D., Lang, N., et al. (2003). Pharmacological modulation of cortical excitability shifts induced by transcranial direct current stimulation in humans. J. Physiol. 553, 293–301. doi: 10.1113/jphysiol.2003.049916
Polanía, R., Nitsche, M. A., and Ruff, C. C. (2018). Studying and modifying brain function with non-invasive brain stimulation. Nat. Neurosci. 21, 174–187. doi: 10.1038/s41593-017-0054-4
Pouliopoulos, A. N., Murillo, M. F., Noel, R. L., Batts, A. J., Ji, R., Kwon, N., et al. (2022). Non-invasive optogenetics with ultrasound-mediated gene delivery and red-light excitation. Brain Stimul. 15, 927–941. doi: 10.1016/j.brs.2022.06.007
Prei, K., Kanig, C., Osnabruegge, M., Langguth, B., Mack, W., Abdelnaim, M., et al. (2023). Limited evidence for reliability of low and high frequency rtms over the motor cortex. Brain Res. 1820:148534. doi: 10.1016/j.brainres.2023.148534
Qin, P. P., Jin, M., Xia, A. W., Li, A. S., Lin, T. T., Liu, Y., et al. (2023). The effectiveness and safety of low-intensity transcranial ultrasound stimulation: A systematic review of human and animal studies. Neurosci. Biobehav. Rev. 140:105501. doi: 10.1016/j.neubiorev.2023.105501
Reed, T., and Cohen Kadosh, R. (2018). Transcranial electrical stimulation (tes) mechanisms and its effects on cortical excitability and connectivity. J. Inheri. Metab. Dis. 41, 1123–1130. doi: 10.1007/s10545-018-0181-4
Rzechorzek, N. M., Thrippleton, M. J., Chappell, F. M., Mair, G., Ercole, A., Cabeleira, M., et al. (2022). A daily temperature rhythm in the human brain predicts survival after brain injury. Brain 145, 2031–2048. doi: 10.1093/brain/awab466
Salehpour, F., Khademi, M., and Hamblin, M. R. (2021). Photobiomodulation therapy for dementia: A systematic review of pre-clinical and clinical studies. J. Alzheimer's Dis. 83, 1431–1452. doi: 10.3233/JAD-210029
Salehpour, F., Mahmoudi, J., Kamari, F., Sadigh-Eteghad, S., Rasta, S. H., and Hamblin, M. R. (2018). Brain photobiomodulation therapy: a narrative review. Molec. Neurobiol. 55, 6601–6636. doi: 10.1007/s12035-017-0852-4
Schork, N. J. (2015). Personalized medicine: time for one-person trials. Nature 520, 609–611. doi: 10.1038/520609a
Shirazi, S. Y., and Huang, H. J. (2019). More reliable eeg electrode digitizing methods can reduce source estimation uncertainty, but current methods already accurately identify brodmann areas. Front. Neurosci. 13:1159. doi: 10.3389/fnins.2019.01159
Sirpal, P., Kassab, A., Pouliot, P., Nguyen, D. K., and Lesage, F. (2019). fnirs improves seizure detection in multimodal eeg-fnirs recordings. J. Biomed. Opt. 24, 051408–051408. doi: 10.1117/1.JBO.24.5.051408
Sokoloff, L. (1960). The metabolism of the central nervous system in vivo. Handb. Physiol. Neurophysiol. 3, 1843–1864.
Soleimani, G., Kuplicki, R., Camchong, J., Opitz, A., Paulus, M. P., Lim, K. O., et al. (2023). Are we really targeting and stimulating dlpfc by placing transcranial electrical stimulation (tes) electrodes over f3/f4? Technical report, Wiley Online Library. doi: 10.1101/2022.12.01.22282886
Sperens, M., Hamberg, K., Hariz, G.-M., et al. (2017). Are patients ready for “earlystim”? attitudes towards deep brain stimulation among female and male patients with moderately advanced parkinson's disease. Parkinson's Dis. 2017:1939831. doi: 10.1155/2017/1939831
Stacey, W. C., and Durand, D. M. (2000). Stochastic resonance improves signal detection in hippocampal ca1 neurons. J. Neurophysiol. 83, 1394–1402. doi: 10.1152/jn.2000.83.3.1394
Stagg, C. J., Bachtiar, V., and Johansen-Berg, H. (2011). The role of gaba in human motor learning. Curr. Biol. 21, 480–484. doi: 10.1016/j.cub.2011.01.069
Sun, W., Song, J., Dong, X., Kang, X., He, B., Zhao, W., et al. (2022). Bibliometric and visual analysis of transcranial direct current stimulation in the web of science database from 2000 to 2022 via citespace. Front. Hum. Neurosci. 16:1049572. doi: 10.3389/fnhum.2022.1049572
Tang, A. D., Bennett, W., Bindoff, A. D., Bolland, S., Collins, J., Langley, R. C., et al. (2021). Subthreshold repetitive transcranial magnetic stimulation drives structural synaptic plasticity in the young and aged motor cortex. Brain Stimul. 14, 1498–1507. doi: 10.1016/j.brs.2021.10.001
Tang, L., Jiang, H., Sun, M., and Liu, M. (2023). Pulsed transcranial photobiomodulation generates distinct beneficial neurocognitive effects compared with continuous wave transcranial light. Lasers in Medical Science 38:203. doi: 10.1007/s10103-023-03865-4
Tarr, P. (2023). Fda clears saint rapid-acting brain stimulation approach for those suffering from resistant major depression. Available online at: https://bbrfoundation.org/content/fda-clears-saint-rapid-acting-brain-stimulation-approach-those-suffering-resistant-major (accessed January 17, 2023).
Terney, D., Chaieb, L., Moliadze, V., Antal, A., and Paulus, W. (2008). Increasing human brain excitability by transcranial high-frequency random noise stimulation. J. Neurosci. 28, 14147–14155. doi: 10.1523/JNEUROSCI.4248-08.2008
Tervo, A. E., Nieminen, J. O., Lioumis, P., Metsomaa, J., Souza, V. H., Sinisalo, H., et al. (2022). Closed-loop optimization of transcranial magnetic stimulation with electroencephalography feedback. Brain Stimul. 15, 523–531. doi: 10.1016/j.brs.2022.01.016
Thair, H., Holloway, A. L., Newport, R., and Smith, A. D. (2017). Transcranial direct current stimulation (tdcs): a beginner's guide for design and implementation. Front. Neurosci. 11:641. doi: 10.3389/fnins.2017.00641
Tudosiu, P.-D., Pinaya, W. H. L., Graham, M. S., Borges, P., Fernandez, V., Yang, D., et al. (2022). “Morphology-preserving autoregressive 3d generative modelling of the brain,” in International Workshop on Simulation and Synthesis in Medical Imaging (Springer), 66–78. doi: 10.1007/978-3-031-16980-9_7
Turkheimer, F. E., Rosas, F. E., Dipasquale, O., Martins, D., Fagerholm, E. D., Expert, P., et al. (2022). A complex systems perspective on neuroimaging studies of behavior and its disorders. Neuroscientist 28, 382–399. doi: 10.1177/1073858421994784
Velema, W. A., Szymanski, W., and Feringa, B. L. (2014). Photopharmacology: beyond proof of principle. J. Am. Chem. Soc. 136, 2178–2191. doi: 10.1021/ja413063e
Vergallito, A., Feroldi, S., Pisoni, A., and Romero Lauro, L. J. (2022). Inter-individual variability in tdcs effects: a narrative review on the contribution of stable, variable, and contextual factors. Brain Sci. 12:522. doi: 10.3390/brainsci12050522
Vidal-Rosas, E. E., Zhao, H., Nixon-Hill, R. W., Smith, G., Dunne, L., Powell, S., et al. (2021). Evaluating a new generation of wearable high-density diffuse optical tomography technology via retinotopic mapping of the adult visual cortex. Neurophotonics 8, 025002–025002. doi: 10.1117/1.NPh.8.2.025002
Vieira, W. F., Gersten, M., Caldieraro, M. A. K., and Cassano, P. (2023). Photobiomodulation for major depressive disorder: Linking transcranial infrared light, biophotons and oxidative stress. Harvard Rev. Psychiat. 31, 124–141. doi: 10.1097/HRP.0000000000000363
Violante, I. R., Alania, K., Cassarà, A. M., Neufeld, E., Acerbo, E., Carron, R., et al. (2023). Non-invasive temporal interference electrical stimulation of the human hippocampus. Nat. Neurosci. 26, 1994–2004. doi: 10.1038/s41593-023-01456-8
Wang, T., Huang, X., Zhao, L., Wang, Y., Zhang, S., Fu, X., et al. (2023). A bibliometric analysis of global publication trends on rtms and aphasia. Medicine 102:e33826. doi: 10.1097/MD.0000000000033826
Xing, L., and Casson, A. J. (2023). 3d-printed, directly conductive and flexible electrodes for personalized electroencephalography. Sensors Actuat. A: 349:114062. doi: 10.1016/j.sna.2022.114062
Yang, M., Yang, Z., Wang, P., and Sun, Z. (2021). Current application and future directions of photobiomodulation in central nervous diseases. Neur. Regener. Res. 16:1177. doi: 10.4103/1673-5374.300486
Yang, P.-F., Phipps, M. A., Jonathan, S., Newton, A. T., Byun, N., Gore, J. C., et al. (2021). Bidirectional and state-dependent modulation of brain activity by transcranial focused ultrasound in non-human primates. Brain Stimul. 14, 261–272. doi: 10.1016/j.brs.2021.01.006
Young, S., Adamo, N., Ásgeirsdóttir, B. B., Branney, P., Beckett, M., Colley, W., et al. (2020). Females with adhd: An expert consensus statement taking a lifespan approach providing guidance for the identification and treatment of attention-deficit/hyperactivity disorder in girls and women. BMC Psychiat. 20, 1–27. doi: 10.1186/s12888-020-02707-9
Yu, K., Niu, X., Krook-Magnuson, E., and He, B. (2021). Intrinsic functional neuron-type selectivity of transcranial focused ultrasound neuromodulation. Nat. Commun. 12:2519. doi: 10.1038/s41467-021-22743-7
Zangen, A., Roth, Y., Voller, B., and Hallett, M. (2005). Transcranial magnetic stimulation of deep brain regions: evidence for efficacy of the h-coil. Clin. Neurophysiol. 116, 775–779. doi: 10.1016/j.clinph.2004.11.008
Zhang, F.-F., Peng, W., Sweeney, J. A., Jia, Z.-Y., and Gong, Q.-Y. (2018). Brain structure alterations in depression: Psychoradiological evidence. CNS Neurosci. Therap. 24, 994–1003. doi: 10.1111/cns.12835
Zrenner, C., Desideri, D., Belardinelli, P., and Ziemann, U. (2018). Real-time eeg-defined excitability states determine efficacy of tms-induced plasticity in human motor cortex. Brain Stimul. 11, 374–389. doi: 10.1016/j.brs.2017.11.016
Keywords: brain stimulation, tES, TMS, tPBM, TUS, tDCS, FUS, neurotechnology
Citation: Toth J, Kurtin DL, Brosnan M and Arvaneh M (2024) Opportunities and obstacles in non-invasive brain stimulation. Front. Hum. Neurosci. 18:1385427. doi: 10.3389/fnhum.2024.1385427
Received: 12 February 2024; Accepted: 05 March 2024;
Published: 18 March 2024.
Edited by:
Reza Kazemi, Institute for Cognitive Science Studies (ICSS), IranReviewed by:
Abed L. Hadipour, University of Messina, ItalyCopyright © 2024 Toth, Kurtin, Brosnan and Arvaneh. This is an open-access article distributed under the terms of the Creative Commons Attribution License (CC BY). The use, distribution or reproduction in other forums is permitted, provided the original author(s) and the copyright owner(s) are credited and that the original publication in this journal is cited, in accordance with accepted academic practice. No use, distribution or reproduction is permitted which does not comply with these terms.
*Correspondence: Jake Toth, anRvdGgxJiN4MDAwNDA7c2hlZmZpZWxkLmFjLnVr