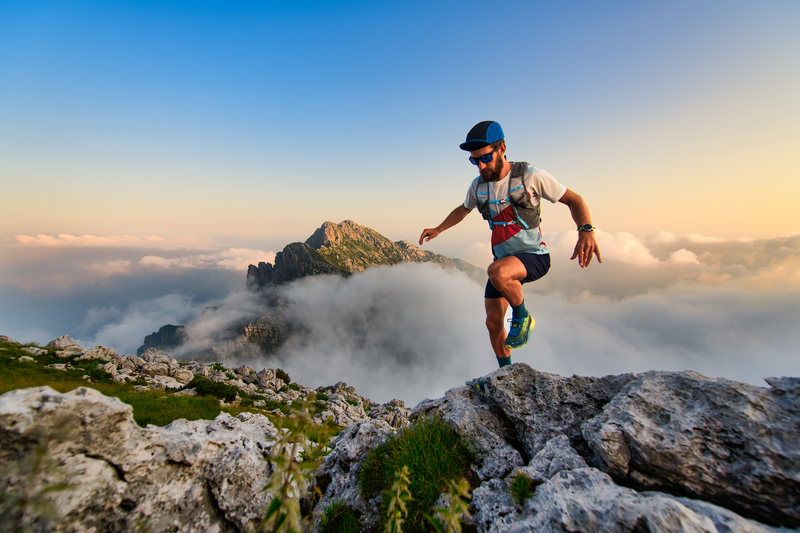
95% of researchers rate our articles as excellent or good
Learn more about the work of our research integrity team to safeguard the quality of each article we publish.
Find out more
EDITORIAL article
Front. Hum. Neurosci. , 05 October 2023
Sec. Brain Imaging and Stimulation
Volume 17 - 2023 | https://doi.org/10.3389/fnhum.2023.1287959
This article is part of the Research Topic Artificial Intelligence Based Diagnostics for Neurological Disorders View all 5 articles
Editorial on the Research Topic
Artificial intelligence based diagnostics for neurological disorders
The future of the world is going to be dominated by the artificial intelligence (AI) viewpoint in all areas of medical research. Neurological disorders are complex types for diagnosis, particularly at the early stage. Early detection can support the patient in innumerable ways to get cured. With these in mind, the topic AI-based diagnostics for neurological disorders was proposed as a special section. A total of 11 articles were received in the special section. After rigorous review, 4 papers were accepted. A short synopsis of these 4 accepted papers is presented as follows:
Zhao et al. presented a mechanical learning model for the prediction of sepsis. They used multivariable logistic regression analysis by employing more than 2,000 pieces of data from sepsis patients. Nine clinically significant features were extracted and analyzed. They obtained a value of 0.743 for the area under the curve (AUC) related to the AI-based detection of sepsis. This could provide a significant application for a clinical set-up in the future.
Kim et al. aided a machine learning model to predict brain amyloid pathology in pre-dementia Alzheimer's disease. They employed the EEG and extracted and analyzed 152 features through a genetic algorithm to identify optimal combinations of features. Using appropriate standard classifiers, they achieved a highest accuracy of 88.6%.
Sun et al. demonstrated a novel deep learning approach for Alzheimer's disease (AD) diagnosis based on eye-tracking data. Eye-tracking-based diagnosis is a recent trend that presents an easy method for diagnosis in the future. They used a nested autoencoder network to extract the eye movement features and a weighted adaptive network layer for feature fusion to feed them to a binary classifier with four-fold validation. They achieved a highest classification accuracy of 85% in AD detection, which is higher than the methods available in the present literature.
Finally, Hsieh et al. administered a seed correlation analysis for ADHD diagnosis. A two-step hierarchical analysis method was used to extract the functional connectivity features. Furthermore, they were evaluated by linear classifiers. The features proposed resulted in a success rate of 83.24% in detecting ADHD.
EA: Writing—original draft, Writing—review and editing. OF: Writing—review and editing. GR: Writing—review and editing.
The authors declare that the research was conducted in the absence of any commercial or financial relationships that could be construed as a potential conflict of interest.
All claims expressed in this article are solely those of the authors and do not necessarily represent those of their affiliated organizations, or those of the publisher, the editors and the reviewers. Any product that may be evaluated in this article, or claim that may be made by its manufacturer, is not guaranteed or endorsed by the publisher.
Keywords: artificial intelligence, diagnostics, neurological disorders, features, deep learning
Citation: Abdulhay E, Faust O and Ramirez G (2023) Editorial: Artificial intelligence based diagnostics for neurological disorders. Front. Hum. Neurosci. 17:1287959. doi: 10.3389/fnhum.2023.1287959
Received: 03 September 2023; Accepted: 05 September 2023;
Published: 05 October 2023.
Edited and reviewed by: Mingzhou Ding, University of Florida, United States
Copyright © 2023 Abdulhay, Faust and Ramirez. This is an open-access article distributed under the terms of the Creative Commons Attribution License (CC BY). The use, distribution or reproduction in other forums is permitted, provided the original author(s) and the copyright owner(s) are credited and that the original publication in this journal is cited, in accordance with accepted academic practice. No use, distribution or reproduction is permitted which does not comply with these terms.
*Correspondence: Enas Abdulhay, ZXdhYmR1bGhheUBqdXN0LmVkdS5qbw==
Disclaimer: All claims expressed in this article are solely those of the authors and do not necessarily represent those of their affiliated organizations, or those of the publisher, the editors and the reviewers. Any product that may be evaluated in this article or claim that may be made by its manufacturer is not guaranteed or endorsed by the publisher.
Research integrity at Frontiers
Learn more about the work of our research integrity team to safeguard the quality of each article we publish.