- Division of Sciences, School of Interwoven Arts and Sciences, Krea University, Sri City, India
Traumatic brain injury (TBI) is associated with an increased risk of long-lasting health-related complications. Survivors of brain trauma often experience comorbidities which could further dampen functional recovery and severely interfere with their day-to-day functioning after injury. Of the three TBI severity types, mild TBI constitutes a significant proportion of total TBI cases, yet a comprehensive study on medical and psychiatric complications experienced by mild TBI subjects at a particular time point is missing in the field. In this study, we aim to quantify the prevalence of psychiatric and medical comorbidities post mild TBI and understand how these comorbidities are influenced by demographic factors (age, and sex) through secondary analysis of patient data from the TBI Model Systems (TBIMS) national database. Utilizing self-reported information from National Health and Nutrition Examination Survey (NHANES), we have performed this analysis on subjects who received inpatient rehabilitation at 5 years post mild TBI. Our analysis revealed that psychiatric comorbidities (anxiety, depression, and post-traumatic stress disorder (PTSD)), chronic pain, and cardiovascular comorbidities were common among survivors with mild TBI. Furthermore, depression exhibits an increased prevalence in the younger compared to an older cohort of subjects whereas the prevalence of rheumatologic, ophthalmological, and cardiovascular comorbidities was higher in the older cohort. Lastly, female survivors of mild TBI demonstrated increased odds of developing PTSD compared to male subjects. The findings of this study would motivate additional analysis and research in the field and could have broader implications for the management of comorbidities after mild TBI.
Introduction
Traumatic brain injury (TBI) constitutes one of the major global health challenges across the world (Gururaj, 2002; Langlois et al., 2006; Lawrence et al., 2016; Rao et al., 2018). TBI is known to be associated with several health-related complications both in the acute and chronic phases and progressively affects the quality of life of the patient population (Brooks et al., 1986; Shoumitro et al., 1999; Hibbard et al., 2000). Treating TBI and TBI-related comorbidities are challenging to the healthcare systems given the number of people affected in civilian and military settings and the myriad of symptoms and comorbidities that ensue following the injury (Brooks et al., 1986; Hibbard et al., 2000; Gururaj, 2002; Langlois et al., 2006; Dean and Sterr, 2013; Lawrence et al., 2016; Danna-Dos-Santos et al., 2018). As a result, TBI and associated comorbidities could impose a severe financial burden on both the patient population and governments worldwide (Gururaj, 2002; Langlois et al., 2006; Kayani et al., 2009; Lee et al., 2021). Furthermore, numerous clinical trials to find a cure for TBI have failed to produce the desired benefit in the patient population (Ye et al., 2009; Stein, 2015; Sudhakar et al., 2019; Sudhakar, 2023). Existing treatment options focus on preventing secondary brain damage through careful clinical management of patients (Jacobi et al., 2002; Armitage-Chan et al., 2007; Flower and Hellings, 2012; Vella et al., 2017; Dash and Chavali, 2018; Ripley et al., 2019).
TBI can be categorized as mild, moderate, and severe injury types based on the scores of the patients reported using the Glasgow coma scale (GCS) (Robert Laskowski et al., 2015; Jain and Iverson, 2022). Mild TBI can occur as a result of concussion in sports activities, falls in elderly subjects, injuries on the battlefield, and whiplash injuries (Kraus and McArthur, 1996; Cassidy et al., 2004; Rutland-Brown et al., 2006; Robert Laskowski et al., 2015). While moderate-severe TBIs are responsible for a majority of death and disability (Kraus and McArthur, 1996; Cassidy et al., 2004; Rutland-Brown et al., 2006; Robert Laskowski et al., 2015), it has been reported that the incidence of mild TBI is higher compared to moderate-severe ones (Langlois et al., 2006). In addition to that, the incidence of mild TBI cases is often underreported in the literature because these estimates often do not encompass subjects who don’t seek medical attention or in-patient hospitalization (Robert Laskowski et al., 2015). Mild TBI has often been termed a “silent epidemic” because the sign and symptoms of the injury don’t usually manifest immediately leading to difficulties in diagnosis. Understanding the long-term health complications (Dean and Sterr, 2013; Danna-Dos-Santos et al., 2018) in the mild TBI population could lead to better disease management in the absence of any visible problems immediately after the injury (Robert Laskowski et al., 2015). Further adding to the woes of clinical TBI is the heterogeneity of injury types. Heterogeneity is an inherent component of TBI owing to several factors including the type and severity of the injury, the affected brain region(s), different pathoanatomical correlates, illness history, age, and sex, etc (Saatman et al., 2008; Maas, 2016). Heterogeneity is also widespread in mild TBI where distinct clusters of the patient population were reported across multiple datasets based on demographics, injury-related information, and laboratory metrics (Si et al., 2018a,b; Pugh et al., 2021). In addition to an incomplete understanding of the activation of cellular and molecular cascades post mild TBI (Werner and Engelhard, 2007; Schwab et al., 2022), the presence of widespread heterogeneity (Saatman et al., 2008; Maas, 2016; Si et al., 2018a,b) has made it quite challenging to come up with a drug to stop secondary brain injuries from happening (Ye et al., 2009) resulting in low success rates of numerous clinical trials (Ye et al., 2009; Stein, 2015; Sudhakar, 2023). When effective therapeutic options become limited for TBI, properly planned patient care (Vella et al., 2017; Dash and Chavali, 2018) along with proactive disease prevention measures could vastly improve patient experiences.
Several research studies have indicated that individuals with TBI exhibit an increased risk of developing a plethora of medical and mental-health comorbidities (Bryant et al., 2010; Bryant, 2011; Yeh et al., 2013; Jain et al., 2014; Katzenberger et al., 2015; Hammond et al., 2019; Stein et al., 2019). These conditions which severely affect the life of the patients fall under a wide umbrella of diseases that include the following: psychiatric and neurological impairments (Bryant et al., 2010; Bryant, 2011; Yeh et al., 2013; Jain et al., 2014; Hammond et al., 2019; Stein et al., 2019), cardiovascular ailments (Liao et al., 2014; Hammond et al., 2019), gastrointestinal (Katzenberger et al., 2015), and lastly those diseases that interfere with endocrine functioning (Tanriverdi et al., 2008). While these studies are very informative in providing information about the post-traumatic development of comorbidities, systematic studies exploring the prevalence of various health-related comorbidities at a discrete time point(s) post injury are required in the field. A previous TBIMS study quantified the prevalence of TBI comorbidities at a 10-year follow-up time period following the index injury (Hammond et al., 2019). This study elegantly reports the prevalence of 44 comorbidities collected using a survey method called Medical and Mental Health Comorbidities Interview (MMHCI) (Hammond et al., 2019). MMHCI was modelled based on National comorbidity survey replication for the mental health conditions and National Health and Nutrition Examination Survey (NHANES) (Center for Disease Control, National Center for Health Statistics, 1999) and was administered to a section of subjects in the TBI Model Systems (TBIMS) national database (Traumatic Brain Injury Model Systems Program, 2021), a neurotrauma registry consisting of longitudinal patient data collected from multiple trauma centres in the United States. While the study was very informative in reporting the prevalence of a list of 44 comorbidities for individuals with moderate-severe TBI, there is a larger need in the field to perform a similar analysis for subjects with mild TBI and understand how the prevalence of comorbidities post brain trauma depends on demographics and injury-specific variables. Our view was emphasized in a recent review of research studies (Tso et al., 2021) that have utilized the TBIMS database (Traumatic Brain Injury Model Systems Program, 2021), where the authors have pointed out that the comorbidity analyses that were performed using the database are quite sparse and the subject remains relatively unexplored.
We in this study employ a multidimensional approach in quantifying the prevalence of 26 comorbidities at 5- years post mild TBI. Using TBIMS national database (Traumatic Brain Injury Model Systems Program, 2021), we estimated the prevalence of comorbidities as a function of demographic variables (age and sex) from the data of subjects who received inpatient rehabilitation. Our results indicate individuals with mild TBI are confronted with various health-related ailments the prevalence of which is also influenced by age and sex, the two demographic variables included in the analysis.
Materials and methods
We performed an extensive analysis of patient data using the TBIMS national database (Traumatic Brain Injury Model Systems Program, 2021) and quantified the prevalence of medical and mental health comorbidities at 5-years post mild TBI. In addition to that, we quantify the prevalence of comorbidities as a function of the age and biological sex of the subjects. The analysis of various psychiatric and medical comorbidities was performed using the public version of the TBIMS national database (Traumatic Brain Injury Model Systems Program, 2021). A request to access the public version of the database was placed in November 2021 and the request was approved a few days later (Traumatic Brain Injury Model Systems Program, 2021). Altogether, the TBIMS database contains information about 17,932 subjects (Traumatic Brain Injury Model Systems Program, 2021). Information about the comorbidities was collected using the National Health and Nutritional Examination Survey (NHANES) (Center for Disease Control, National Center for Health Statistics, 1999) where the subjects were asked a series of questions regarding the presence/absence of each comorbidity and their onset (before, at the same time, and after the index injury) at multiple follow up time point (1, 2, 5, 10, 15, 20, and 25 years). If a particular condition was found to be positive at the previous administration, the same item was refrained from asking again. Self-diagnosis was not accepted and only diagnosis originating from a doctor or a certified healthcare professional was included in the database. More information on NHANES administration can be found in the TBIMS data dictionary (Traumatic Brain Injury Model Systems Program, 2021). The public version of the database (Traumatic Brain Injury Model Systems Program, 2021) has information about 26 medical and mental health comorbidities of the subjects at multiple follow-up time points (Traumatic Brain Injury Model Systems Program, 2021). In this study, only data collected at 5-year follow-up was considered for analysis.
The database (Traumatic Brain Injury Model Systems Program, 2021) consists of subjects with a minimum age of 16 years and hence the same inclusion criteria were applied to our analysis. We grouped the subjects into three categories based on the severity of the index TBI: mild, moderate, and severe. The severity of TBI was determined using the Glasgow coma scale (GCS) (Jain and Iverson, 2022). All analyses in this study were performed on mild TBI subjects whose GCS scores fell between 13 and 15 (Jain and Iverson, 2022). Data analysis was performed using the Python programming language. Custom Python scripts were written and executed using the Google Collaboratory application (https://colab.research.google.com/). Patient data was read using the pandas python library (https://pandas.pydata.org/) and analysis of the prevalence of the comorbidities was performed thereafter. All scripts used in the study and data generated as a result of this study will be made available to the scientific community upon request.
Statistical analysis
All statistical analyses in this study were performed on mild TBI subjects. For each comorbidity, we computed the total number of mild TBI subjects who have the condition with onset at the same time or after the index injury at 5-year follow-up time point. Next, the percentage of subjects with a specific comorbidity was computed as the ratio of the number computed in the previous step to the total number of subjects with and without the condition. This number represents the observed prevalence of each comorbidity at a given follow-up time point (Hammond et al., 2019). We also computed the percentage of subjects with the condition and onset before the index TBI in the same manner. We computed the prevalence using two different descriptions of onset so as to provide maximum information to the medical practitioners as each of these definitions could provide vital insights for designing foresighted patient care programs.
We established statistical significance between the prevalence of comorbidities for young vs old subjects, and male vs female subjects. Subjects whose age at follow-up (5-years post TBI) is less than or equal to 50 years were categorized as young and those with age at follow-up greater than 50 years were categorized as old. For estimating the statistical significance of young vs old subjects, we first computed the odds ratio (McHugh, 2009; Steven and Hoffman, 2022) using the following formula,
where ‘a’ represents the percentage of cases (prevalence) with the comorbidity in the older cohort at 5-year follow-up, ‘b’ represents the percentage of cases in the older cohort without the comorbidity at the same follow-up time period. Similarly, ‘c’ represents the percentage of cases (prevalence) with the comorbidity in the younger cohort at 5-year follow-up and ‘d’ represents the percentage of cases in the younger cohort without the comorbidity at the same follow-up time period. This ratio represents the odds of observing a comorbidity in older subjects compared to the odds in the younger cohort. Similarly, the odds ratio capturing the relative prevalence in female vs male subjects in the cohort was computed to determine the sex dependence of post-traumatic prevalence of comorbidities. After computing the odds ratio, statistical significance was established using Fisher’s-Exact test (95% confidence interval) (McHugh, 2009). The probability ‘p’ for Fisher’s-Exact test was calculated using the following formula,
where a, b, c, and d represent the same metrics as mentioned above for the odds ratio and n represents the sum of all four variables. Confidence intervals (McHugh, 2009; Steven and Hoffman, 2022) for the odds ratio were computed using the below formula,
where u and l are upper and lower bounds of the confidence interval.
To determine if a confounding effect (Jager et al., 2008; Jose et al., 2008) of age at follow-up or effect modification was present in the association between the prevalence and biological sex of the subjects, the following procedure was followed: Two strata were created representing subjects <= 50 years of age and subjects > 50 years of age at 5-year follow-up corresponding to two different values of the confounder. For each stratum, we calculated the odds ratio of the relative prevalence of comorbidities in female subjects compared to male subjects (OR1 and OR2). We next determined if the stratum-specific odds ratios (OR1 and OR2) were similar to each other using the Breslow-Day-Tarone test (BDTT) for homogeneity (95% confidence interval) (Breslow and Day, 1980; Breslow, 1996). If the odds ratios were found to be significantly different from each other (p < 0.05), we report the stratum-specific odds ratio for the comorbidity in question (effect modification).
If the odds ratios were similar (p >= 0.05), we utilized the Mantel-Haenszel test (MHT) (Kuritz et al., 1988; Jose et al., 2008; Tripepi et al., 2010) to determine if there is an association between sex and prevalence of the comorbidity in question (95% confidence interval). Additionally, we report Mantel-Haenszel odds ratio (ORMH) (Kuritz et al., 1988; Jose et al., 2008; Tripepi et al., 2010) adjusted for age at follow-up (and magnitude of confounding) which is calculated from the stratum-specific numbers using the following formula,
where ai, bi, ci, and di represent the same as explained above (for odds ratio) in the ith stratum of the confounder (age at follow-up) and ni is computed by adding all four variables ai, bi, ci, and di in the same stratum.
Lastly, the magnitude of confounding (CM) was calculated using the following formula,
where ORMH represents the adjusted odds ratio obtained using the Mantel-Haenszel formula (Kuritz et al., 1988) and ORcrude represents the crude (unadjusted) odds ratio.
Results
From the TBIMS national database, we first grouped the subjects into mild, moderate, and severe TBI groups based on the GCS scores collected at the time of admission to the hospital (see section “Materials and methods”). Out of the 17,932 subjects in the TBIMS national database, 4,915 subjects belong to the mild category, 2,642 subjects belong to the moderate group and 3,007 subjects are severe TBI subjects. The remaining subjects were either chemically sedated or paralyzed or intubated or their GCS score was missing or unknown. Out of the 10,564 subjects from whom the GCS score was measured, 46.5 % belong to the mild category. We then computed the mean values of age at onset, GCS, and post-traumatic amnesia (PTA) for mild TBI subjects. The average age at injury onset for subjects with mild TBI is 55.47 ± 0.28 (mean ± sem) years. The mean values of PTA and GCS for the same group are 10.38 ± 0.21 days, 14.6 ± 0.007 respectively.
Prevalence of comorbidities at 5 years following mild TBI
We next sought to understand the prevalence of various comorbidities at 5 years following the index TBI. The presence or absence of various medical and behavioural comorbidities was collected through a patient survey modelled based on NHANES (Center for Disease Control, National Center for Health Statistics, 1999). The 26 comorbidities that were covered in the NHANES interview were grouped under the following 8 categories: cardiovascular, neurological, musculoskeletal and rheumatologic, ophthalmological, endocrine, gastrointestinal, respiratory and psychiatric/mental health (Table 1). For each condition, we estimated the prevalence by counting the total number of subjects with the condition at the same time or after acquiring the index TBI and dividing this number by the total number of subjects who responded to the question (those with the presence and absence of the condition). We did the same to obtain the numbers for the prevalence of each condition with onset before acquiring TBI.
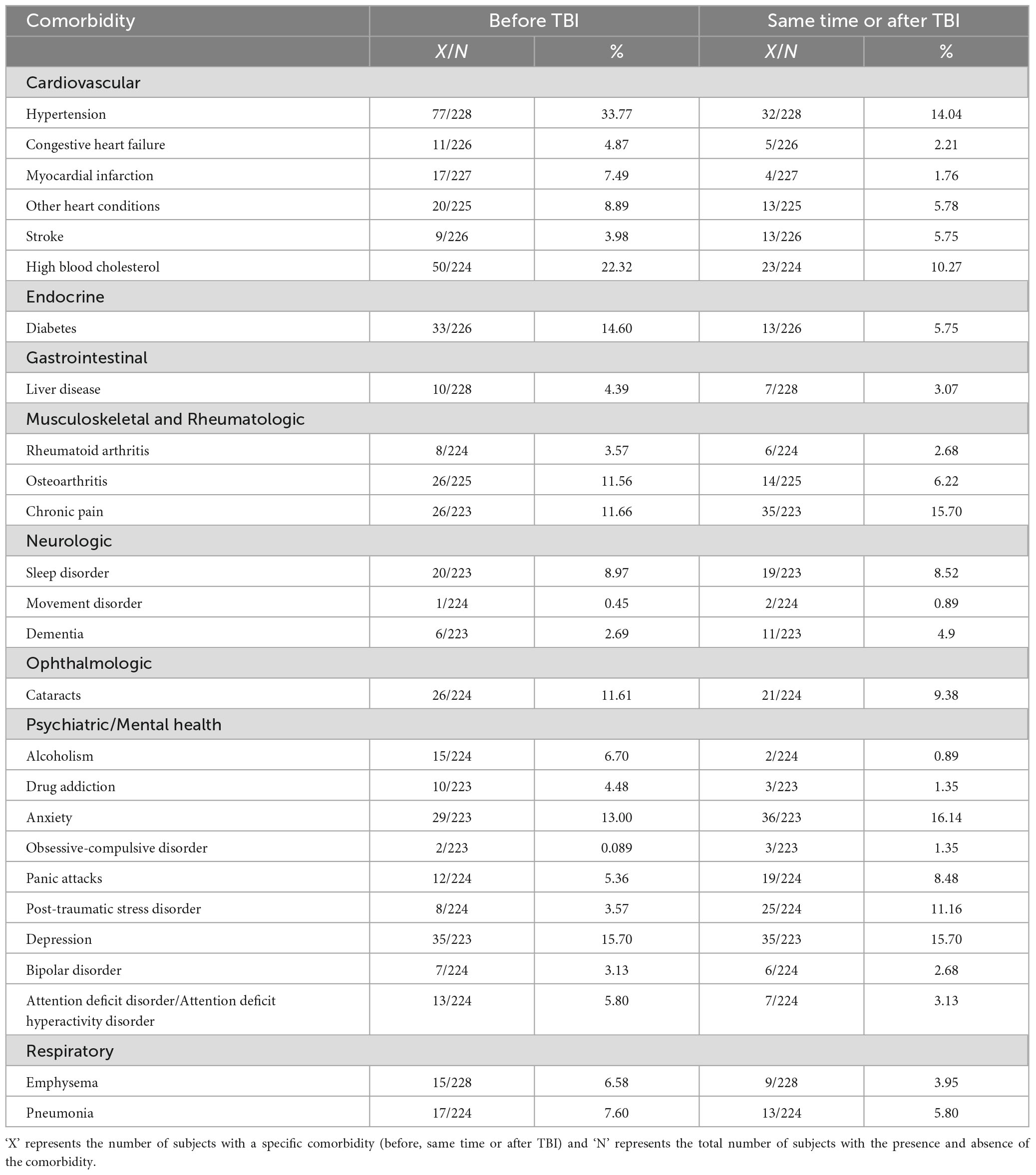
Table 1. Prevalence of psychiatric and medical comorbidities for mild TBI patients at 5-year follow-up time point.
The final number of mild TBI subjects that took part in the NHANES survey at 5-years post injury is 223-228. Out of this, 149-153 belong to the older cohort of patients and 69-71 belong to the younger cohort. 163-166 are males and 59-62 are female subjects. The prevalence of all 26 comorbidities for individuals with mild TBI at 5-year follow-up time point is given in Table 1. Using this data, we compiled a list of the top 10 comorbidities ranked according to their prevalence (at the same time or after TBI) at 5-year follow-up time point for subjects with mild TBI (Table 2). All further analyses in the study (old vs young and female vs male subjects) were performed for this list of highly prevalent comorbidities on subjects with mild TBI. A close inspection of Table 2 reveals that individuals with mild TBI are confronted with psychiatric conditions which include anxiety, depression, post-traumatic stress disorder (PTSD), and panic attacks (PA) at 5 years post injury. In addition to psychiatric comorbidities, cardiovascular comorbidities (hypertension (HT) and high blood cholesterol (HBC)) were also prevalent among subjects with mild TBI in the TBIMS national database. Lastly, the list includes other comorbidities like chronic pain (CP), osteoarthritis (OA), cataracts, and sleep disorder (SD). From these results, it becomes clear that subjects with mild TBI are confronted with a myriad of health-related issues at 5 years following the injury.
Prevalence of comorbidities in young and old subjects
We next sought to determine if the prevalence of the top 10 medical and mental health comorbidities at the same time or after TBI differ between young and old subjects with mild TBI. Subjects were classified as young or old based on an age threshold of 50 years (see section “Materials and methods”). We asked the question if older subjects in the cohort exhibited increased odds of developing a particular set of comorbidities compared to younger subjects. To do this, we computed the OR for the top 10 prevalent comorbidities (compiled in the previous step) and established its significance using the Fisher’s-Exact test (see section “Materials and methods”). Our results indicate that psychiatric comorbidities (depression, anxiety, PTSD, and PA) exhibit an increased prevalence in the younger compared to an older cohort of subjects at the same time or after TBI. However, statistical significance was obtained only for the odds ratio of depression (OR = 0.37 and p = 0.026, Table 3). The prevalence of all other comorbidities (rheumatologic, ophthalmological, and cardiovascular) at the same time or after TBI was higher in the older cohort compared to the younger cohort. For example, subjects with age at follow-up > 50 years were 9.79 times more likely to experience OA compared to subjects with age at follow-up <= 50 years. Altogether, our results reveal a unique pattern of comorbidity dynamics in younger vs older individuals with mild TBI.
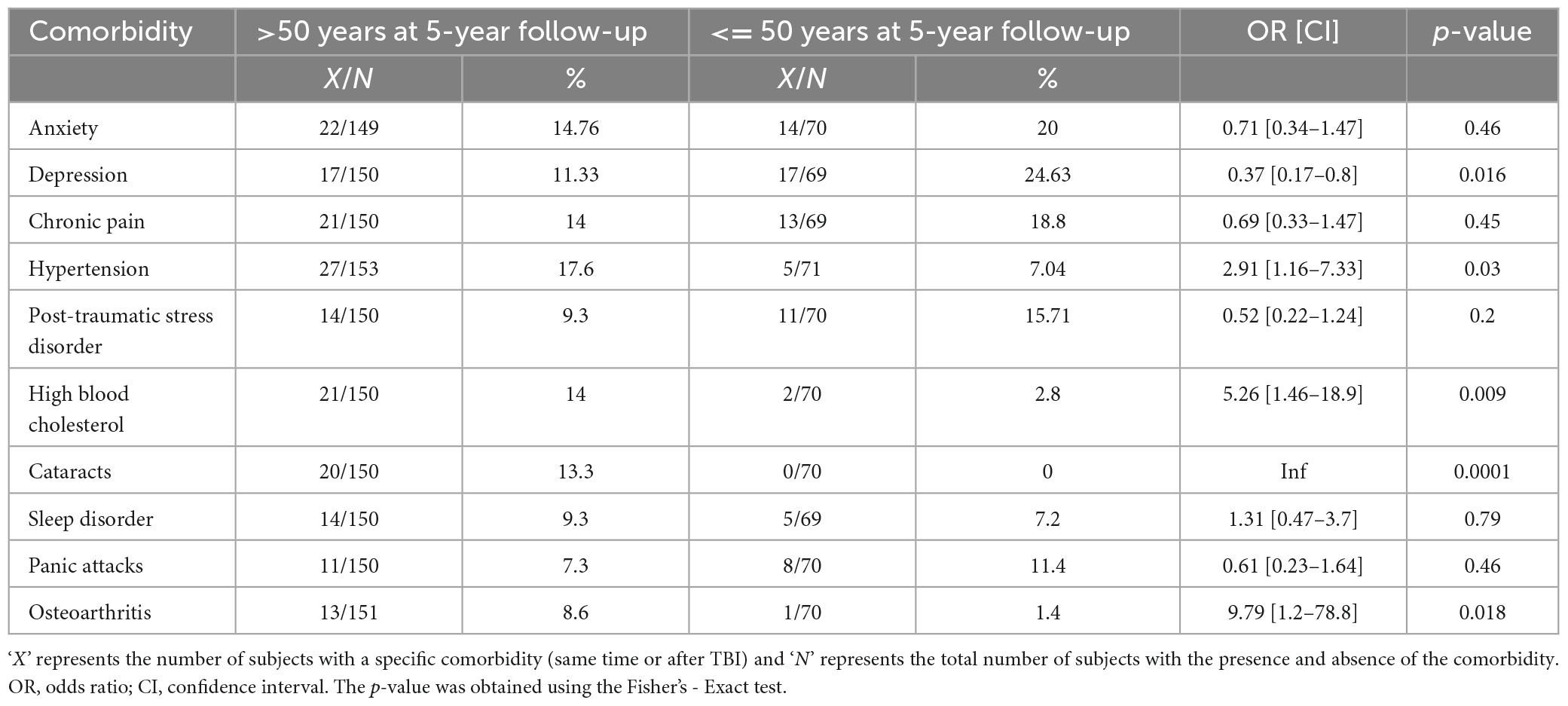
Table 3. Comparison of the prevalence of comorbidities according to the age of the subjects at 5-year follow-up.
Prevalence of comorbidities according to the biological sex of the subjects
We next sought to understand if the prevalence of comorbidities at the same time or after TBI was dependent on the biological sex of the subjects in the cohort. For this, we first computed the prevalence for the set of top 10 medical and psychiatric comorbidities at the same time or after TBI for male and female subjects in the cohort (Table 4). Since female subjects have a longer lifespan and tend to outlive men (Ginter and Simko, 2013), we reasoned that age could be a confounding factor in the association between sex and the prevalence of comorbidities. In order to control for confounding, we resorted to stratified analysis (see section “Materials and methods”) (Jager et al., 2008; Jose et al., 2008). Briefly, we created two strata of the confounder (age at follow-up): subjects with age at follow-up > 50 years and subjects with age at follow-up <= 50 years. Stratum-specific odds ratio was computed for each stratum of the confounder. Based on the results from BDTT (Breslow and Day, 1980; Breslow, 1996), either effect modification or possible confounding was established. If effect modification was observed, stratum-specific odds ratios were reported while if confounding was observed unconfounded (adjusted) odds ratio (ORMH) (Kuritz et al., 1988; Jose et al., 2008; Tripepi et al., 2010) and magnitude of confounding (CM) was reported. Odds ratios reflect the odds of observing the comorbidity in female subjects compared to the odds in male subjects.
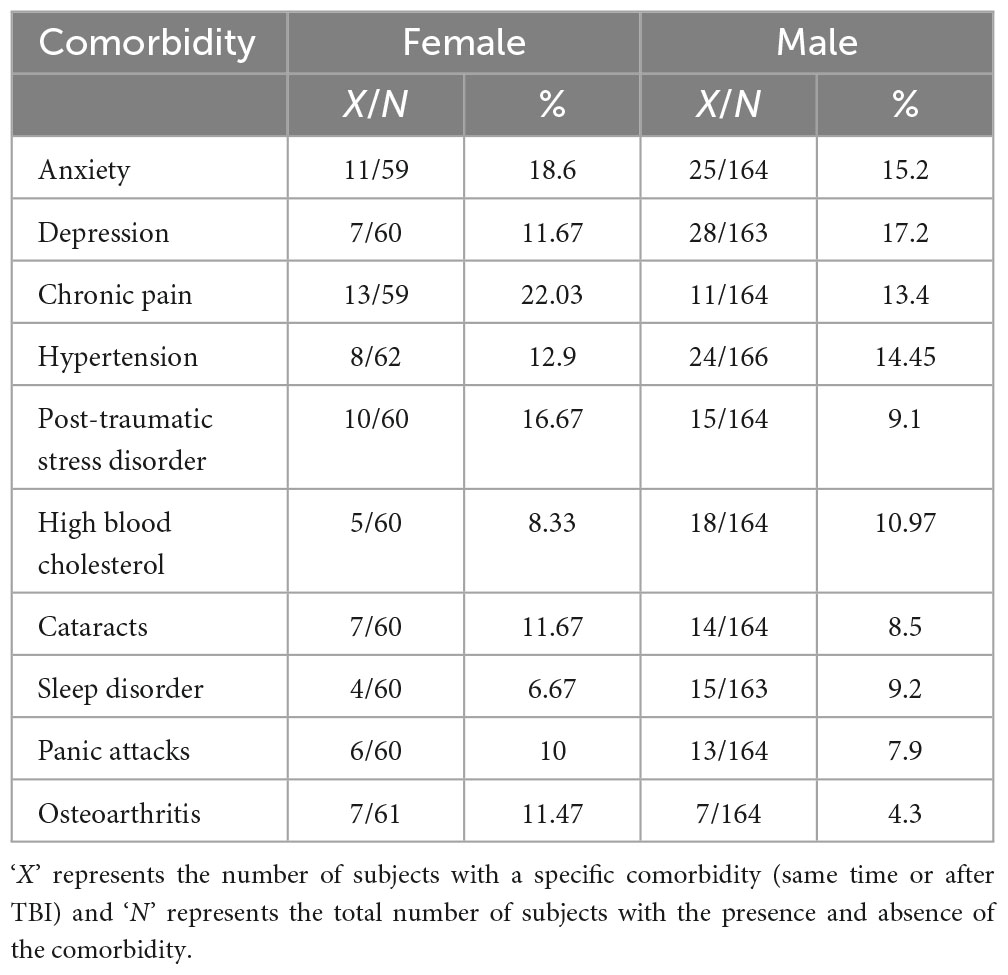
Table 4. Comparison of the prevalence of comorbidities according to the biological sex of the cohort at 5-year follow-up.
Strong effect modification was observed for comorbidities such as depression, CP, SD and OA (p < 0.005 in the BDTT). Female subjects with age at follow-up <= 50 years exhibited decreased odds for depression compared to male subjects at 5-year follow-up (OR2 = 0.18, Table 5). Additionally, female subjects with age at follow-up > 50 years exhibited increased odds of developing CP compared to male subjects at 5-year follow-up (OR1 = 2.84, Table 5).
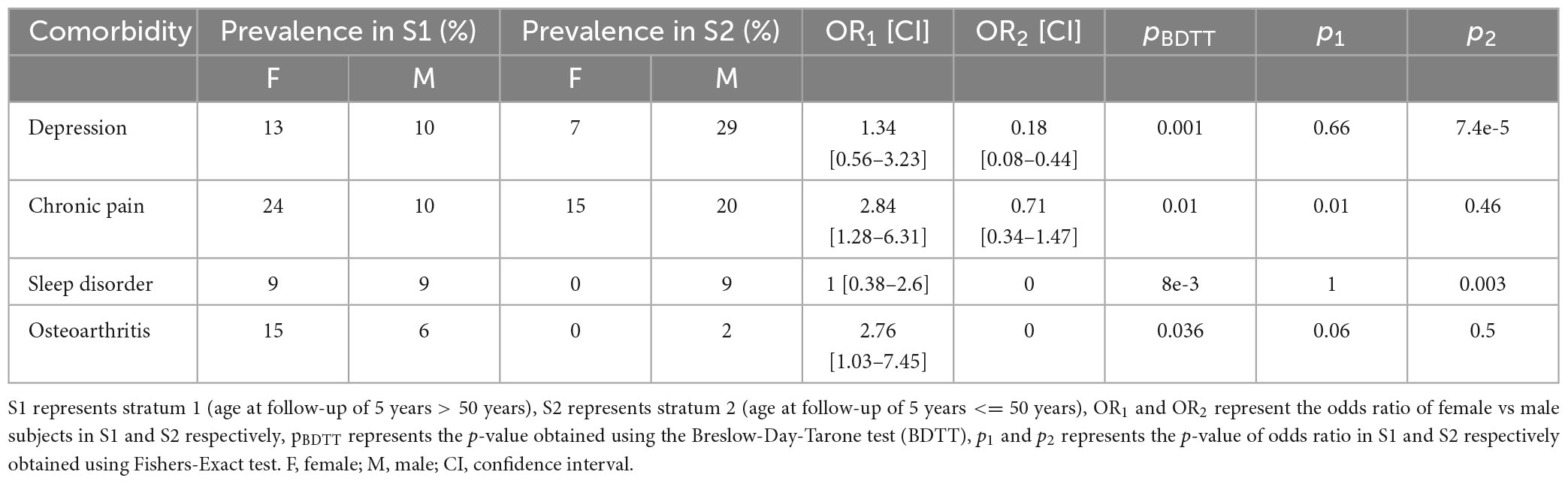
Table 5. Comorbidities for which effect modification was observed in female vs male subjects stratified by age at follow-up of 5-years.
Possible confounding was observed for anxiety, HT, PTSD, HBC, and PA (Table 6). However, the magnitude of confounding was less than 10% for the above-mentioned comorbidities except for HBC. Female subjects demonstrated increased odds of developing PTSD compared to male subjects at 5-year follow-up (ORMH = 1.88, Table 6). Our results, therefore, revealed interesting patterns in the association between biological sex and the prevalence of comorbidities post mild brain trauma.
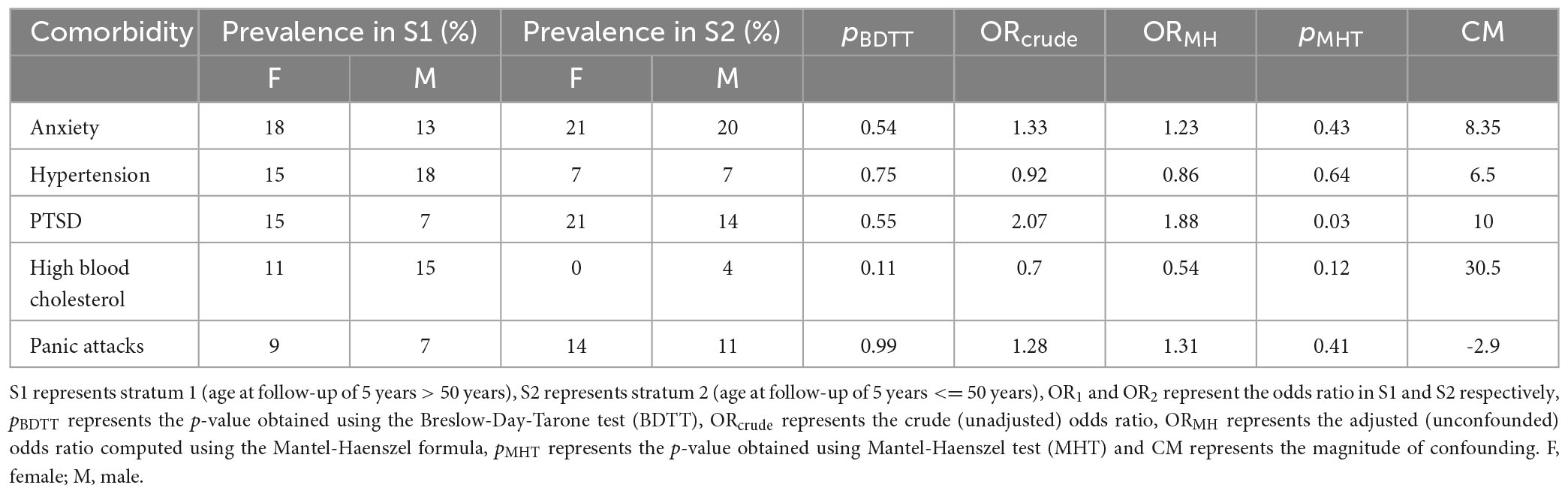
Table 6. Comorbidities effect modification was not observed in female vs male subjects stratified by age at follow-up of 5-years.
Discussion
We studied the longstanding comorbidities of acquiring mild TBI using patient data from the TBIMS national database. We did so by computing the prevalence of medical and mental health comorbidities at 5 years post index TBI. Our secondary aim is to understand how the prevalence of various comorbidities post mild TBI is influenced by a subset of patient demographics (sex, and age). Our analysis indicates the presence of interesting dynamics in the development of medical and mental-health comorbidities post TBI which could be useful to devise foresighted programs for the patient community. Our work hopes to contribute to the sparse body of literature concerning the prevalence of various comorbidities post mild TBI.
The findings in this study are similar to those that have been previously reported in the literature. A number of studies have reported that individuals with TBI exhibit an increased risk of developing psychiatric problems (Schwarzbold et al., 2008; Bryant et al., 2010; Bryant, 2011; Gould et al., 2011; Mallya et al., 2015; Stein et al., 2019) and demonstrate an impaired cognitive and oculomotor function (Dean and Sterr, 2013; Danna-Dos-Santos et al., 2018) compared to the general population. In our study, the most commonly manifested psychiatric disorders for individuals with mild TBI were anxiety and depression. Other frequently reported psychiatric conditions were PTSD, and PA. The prevalence of obsessive-compulsive disorder (OCD), bipolar disorder (BD), and attention deficit disorder/attention deficit hyperactivity disorder (ADD/ADHD) following TBI were far less common compared to other psychiatric conditions in our analysis. Confirming our results, depression, and generalized anxiety disorder were the two most common psychiatric conditions reported in a cohort of subjects with mild TBI from trauma centres in Australia (Bryant et al., 2010). Similarly, an increased risk prevalence of depression (and PTSD) was reported in a previous prospective study (Stein et al., 2019) of more than 1,000 individuals with mild TBI. Increased prevalence of anxiety and comorbid anxiety with depression have also been reported following TBI in the same study (Stein et al., 2019). These results have enormous clinical implications for the mild TBI community in post-traumatic disease management. For example, foresighted clinical programs could be implemented to screen the presence of depression, anxiety, and PTSD at multiple time points post acquiring mild TBI. Further, relatives of mild TBI survivors could be involved to watch out for symptoms of the above-mentioned psychiatric conditions in their family members.
We found that PTSD is highly prevalent in individuals with mild TBI (Table 2) and more so in female subjects (Tables 4, 6). Interestingly, in a previous TBIMS study (Hammond et al., 2019) that quantified the prevalence of comorbidities in individuals with moderate-severe TBIs, PTSD did not find itself in the list of top 10 comorbidities that were highly prevalent. Although the existence of PTSD after both mild (Middelboe et al., 1992; Bryant and Harvey, 1998; Bryant, 2011) and severe (Mcmillan, 1991, 1996; Bryant, 1996, 2011; Bryant et al., 2000) forms of TBI has been established in the literature, it’s possible that stronger PTA and loss of consciousness could retard the encoding of trauma-related experiences and prevent them from being re-experienced later (Bryant, 2011; Mallya et al., 2015). As a consequence, the risk of developing PTSD could be higher in mild cases of TBI compared to severe ones (Bryant, 2011; Mallya et al., 2015). Confirming this observation, a few studies have reported that the risk of developing PTSD is elevated in mild TBI compared to severe cases (Bombardier et al., 2006; Zatzick et al., 2010; Mallya et al., 2015).
With respect to cardiovascular comorbidities, we find that HT and HBC are quite common after mild TBI (Tables 1, 2). In line with our results, there are a few studies in the literature that associate TBI with an increased risk of cardiovascular comorbidities (Ahmadi et al., 2015; Wang et al., 2018; Nyam et al., 2019; Turner et al., 2021; Izzy et al., 2022). In a longitudinal study of individuals with TBI up to 10 years post injury, the authors found that cardiovascular and neurological comorbidities (Izzy et al., 2022) are highly prevalent in mild TBI as well as moderate-severely injured subjects. According to another study (Nyam et al., 2019) involving 16211 subjects who were followed up to 5 years post injury, TBIs are associated with an increased risk of developing cardiovascular comorbidities. In addition to that, TBIs are associated with an elevated risk of developing cardiovascular conditions such as arrhythmias and cardiomyopathies, and atherosclerosis (Ahmadi et al., 2015; Wang et al., 2018).
We also determined if the prevalence of comorbidities at the same time or after TBI exhibits differential patterns as a function of sex and the age of the participants at follow-up. Younger subjects (<= 50 years at follow-up) in our study were prone to developing depression compared to older participants who exhibited increased odds of developing cardiovascular (HT and HBC) comorbidities, cataracts, and OA (Table 3). Similar results were also seen in a previous study (Chan et al., 2017) where the younger cohort (<65 years old) was characterized by a high prevalence of psychiatric comorbidities whereas the older cohort (>65 years old) was characterized by the presence of cardiovascular, metabolic, and endocrine-related comorbidities. Such differential presence of comorbidities post TBI was also confirmed in a previous TBIMS study (Hammond et al., 2019).
With respect to the association between sex and prevalence of comorbidities, our results revealed that for a certain set of comorbidities, the association depends on the age at follow-up of the participants (effect modification) (Tables 5, 6). While depression was highly prevalent in young males compared to young females, the latter group exhibited increased odds of developing post-traumatic chronic pain (Table 5). Also, female subjects with mild TBI were more prone to developing PTSD compared to their male counterparts (Table 6). Women in general are more susceptible to developing PTSD (Olff, 2017), musculoskeletal conditions like OA (Zhang and Jordan, 2010), and anxiety disorders (McLean et al., 2011). In the TBI population, prior results have established that musculoskeletal (OA) and cardiovascular comorbidities are common in females compared to male subjects irrespective of age (Chan et al., 2017). Similarly, women TBI survivors are at an increased risk of developing PTSD, and depression (Kim et al., 2018) and were shown to exhibit an elevated severity in anxiety and depression (Mikolić et al., 2021).
Limitations of the study and future work
The subjects in this study were those who received in-patient rehabilitation for mild TBI in one of the TBIMS centres (Traumatic Brain Injury Model Systems Program, 2021). These subjects were typically characterized by the presence of intracranial pathology and/or PTA (Traumatic Brain Injury Model Systems Program, 2021). Sufficient caution should be exercised while generalizing the results of the study to the mild TBI population as a whole since this population might also comprise subjects who were treated in less sophisticated hospitals/outpatient clinics or may even include subjects who may not seek medical attention. Further, our aim in this study is not to establish any sort of causal relationship but to elucidate the most frequently manifested psychiatric and medical problems in individuals with mild TBI. Future studies could incorporate a control group of non-TBI subjects for comparison and establishing causal inference. Lastly, the study could potentially suffer from ascertainment bias as it’s possible that some comorbidities that were diagnosed post TBI could have been persistent and undiagnosed prior to acquiring the injury due to lack of medical attention or some other reasons.
Another potential limitation of our study could be related to smaller sample sizes at each follow-up time point. For example, even though the TBIMS database consists of information pertaining to 4,915 mild TBI subjects, only 223-228 subjects enrolled in the NHANES study at 5-year follow-up time period. This could become problematic especially when comparing the prevalence between different patient subgroups where sample size could further diminish. As the TBIMS national database expands in the future with an increasing number of subjects, the current analysis could be repeated to see if there is any effect of sample size on the prevalence and statistical significance. Lastly, future administration of patient surveys/questionnaires (NHANES) could be expanded to cover other medical conditions.
This study is a preliminary excursion into the various factors that influence the development of comorbidities post mild TBI. In the future, we intend to build upon this work to study pairwise disease co-occurrences at scale using graph networks and network analysis (Fotouhi et al., 2018; Ljubic et al., 2020; Lee and Park, 2021). In doing so, a larger avenue of analysis is opened to visualize and understand disease comorbidities as differences in network structures across scales (Fotouhi et al., 2018; Ljubic et al., 2020; Lee and Park, 2021). Comorbidities are understood as unique spatial organisations of statistically significant interconnected disease nodes with varying depths of connectedness (Fotouhi et al., 2018; Kim et al., 2018; Lee and Park, 2021). Network structural properties will be compared across time, TBI severity, and demographic conditions. The significance of this approach is an intuitive visualisation of diseases in terms of their co-occurrences, and clusters of diseases can be identified for unique TBI severity and demographic conditions that could inform proactive post-TBI healthcare. The current literature on using disease networks to study comorbidity relationships in subjects with TBI is sparse and we seek to contribute to that body of knowledge. Finally, as the incidence of mild TBI is frequent in the general population, future excursions of the work could include understanding the comorbidity dynamics as a function of the number of impacts experienced by the subjects.
Conclusion
Our study indicates that individuals who have sustained mild TBI could develop serious long-term medical and psychiatric comorbidities which could affect their pace of recovery. The results of this study could motivate further investigation regarding the prevalence of health-related problems in the mild TBI population and encourage the development of foresighted programs and patient care for post-traumatic management of comorbidities. The findings reported in the paper have wider implications for prognosis, patient management, and treatment of various comorbidities after TBI.
Data availability statement
Publicly available datasets were analyzed in this study. This data can be found here: https://www.tbindsc.org.
Ethics statement
This study is secondary data analysis of a publicly available dataset. The original studies involving human participants were reviewed and approved by Krea Institutional Review Board. Written informed consent to participate in the original study was provided by the participants’ legal guardian/next of kin.
Author contributions
SS: conceptualization, methodology, investigation, writing—review and editing. SC: Conceptualization, investigation, writing—review and editing. KP: investigation, writing—review and editing. KM: methodology, writing—review and editing. SKS: conceptualization, methodology, software, validation, formal analysis, investigation, resources, data curation, writing—original draft, writing—review and editing, visualization, supervision, project management, and funding acquisition. All authors contributed to the article and approved the submitted version.
Funding
This work was supported by faculty grant from Krea University.
Acknowledgments
The TBI Model Systems National Database is a multicenter study of the TBI Model Systems Centers Program, and is supported by the National Institute on Disability, Independent Living, and Rehabilitation Research (NIDILRR), a center within the Administration for Community Living (ACL), U.S. Department of Health and Human Services (HHS). However, these contents do not necessarily reflect the opinions or views of the TBI Model Systems Centers, NIDILRR, ACL or HHS.
Conflict of interest
The authors declare that the research was conducted in the absence of any commercial or financial relationships that could be construed as a potential conflict of interest.
Publisher’s note
All claims expressed in this article are solely those of the authors and do not necessarily represent those of their affiliated organizations, or those of the publisher, the editors and the reviewers. Any product that may be evaluated in this article, or claim that may be made by its manufacturer, is not guaranteed or endorsed by the publisher.
References
Ahmadi, N., Hajsadeghi, F., Yehuda, R., Anderson, N., Garfield, D., Ludmer, C., et al. (2015). Traumatic brain injury, coronary atherosclerosis and cardiovascular mortality. Brain Inj. 29, 1635–1641. doi: 10.3109/02699052.2015.1075149
Armitage-Chan, E., Wetmore, L., and Chan, D. (2007). Anesthetic management of the head trauma patient. J. Vet. Emerg. Crit. Care 17, 5–14.
Bombardier, C., Fann, J., Nancy Temkin, M., Esselman, P., Pelzer, E., Meghan Keough, B., et al. (2006). Posttraumatic stress disorder symptoms during the first six months after traumatic brain injury. J. Neuropsychiatry Clin. Neurosci. 18, 501–508.
Breslow, N. (1996). Statistics in epidemiology: The case-control study. J. Am. Stat. Assoc. 91, 14–28.
Breslow, N., and Day, N. (1980). The analysis of case control studies. Statistical methods in cancer research. Lyon: International Agency for Research on Cancer Scientific Publications.
Brooks, N., Campsie, L., Symington, C., Beattie, A., and McKinlay, W. (1986). The five year outcome of severe blunt head injury: A relative’s view. J. Neurol. Neurosurg. Psychiatry 49, 764–770. doi: 10.1136/jnnp.49.7.764
Bryant, R. (1996). Posttraumatic stress disorder, flashbacks, and pseudomemories in closed head injury. J. Trauma Stress 9, 621–629. doi: 10.1007/BF02103671
Bryant, R. (2011). Post-traumatic stress disorder vs traumatic brain injury. Dialogues Clin. Neurosci. 13, 251–262.
Bryant, R., Creamer, M., McFarlane, A., Richard, C. C., and Silove, D. (2010). The psychiatric sequelae of traumatic injury. Am. J. Psychiatry 167, 312–320.
Bryant, R., and Harvey, A. (1998). Relationship between acute stress disorder and posttraumatic stress disorder following mild traumatic brain injury. Am. J. Psychiatry 155, 625–629.
Bryant, R., Marosszeky, J., Crooks, J., and Gurka, J. (2000). Posttraumatic stress disorder after severe traumatic brain injury. Am. J. Psychiatry 157, 629–631.
Cassidy, J., Carroll, L., Peloso, P., Borg, J., von Holst, H., Holm, L., et al. (2004). Incidence, risk factors and prevention of mild traumatic brain injury: Results of the WHO collaborating centre task force on mild traumatic brain injury. J. Rehabil. Med. Suppl. 43, 28–60. doi: 10.1080/16501960410023732
Center for Disease Control, National Center for Health Statistics (1999). National health and nutrition examination survey (NHANES). Available online at: https://health.gov/healthypeople/objectives-and-data/data-sources-and-methods/data-sources/national-health-and-nutrition-examination-survey-nhanes (accessed January 7, 2023).
Chan, V., Mollayeva, T., Ottenbacher, K., and Colantonio, A. (2017). Clinical profile and comorbidity of traumatic brain injury among younger and older men and women: A brief research notes. BMC Res. Notes 10:371. doi: 10.1186/s13104-017-2682-x
Danna-Dos-Santos, A., Mohapatra, S., Santos, M., and Degani, A. (2018). Long-term effects of mild traumatic brain injuries to oculomotor tracking performances and reaction times to simple environmental stimuli. Sci. Rep. 8:4583. doi: 10.1038/s41598-018-22825-5
Dash, H., and Chavali, S. (2018). Management of traumatic brain injury patients. Korean J. Anesthesiol. 71, 12–21. doi: 10.4097/kjae.2018.71.1.12
Dean, P., and Sterr, A. (2013). Long-term effects of mild traumatic brain injury on cognitive performance. Front. Hum. Neurosci. 7:30. doi: 10.3389/fnhum.2013.00030
Flower, O., and Hellings, S. (2012). Sedation in traumatic brain injury. Emerg. Med. Int. 2012, 1–11. doi: 10.1155/2012/637171
Fotouhi, B., Momeni, N., Riolo, M., and Buckeridge, D. (2018). Statistical methods for constructing disease comorbidity networks from longitudinal inpatient data. Appl. Netw. Sci. 3:46. doi: 10.1007/s41109-018-0101-4
Ginter, E., and Simko, V. (2013). Women live longer than men. Bratislava Med. J. 114, 45–49. doi: 10.4149/bll_2013_011
Gould, K., Ponsford, J., Johnston, L., and Schönberger, M. (2011). The nature, frequency and course of psychiatric disorders in the first year after traumatic brain injury: A prospective study. Psychol. Med. 41, 2099–2109. doi: 10.1017/S003329171100033X
Gururaj, G. (2002). Epidemiology of traumatic brain injuries: Indian scenario. Neurol. Res. 24, 24–28. doi: 10.1179/016164102101199503
Hammond, F., Corrigan, J., Ketchum, J., Malec, J., Dams-O’Connor, K., Hart, T., et al. (2019). Prevalence of medical and psychiatric comorbidities following traumatic brain injury. J. Head Trauma Rehabil. 34, E1–E10. doi: 10.1097/HTR.0000000000000465
Hibbard, M., Bogdany, J., Uysal, S., Kepler, K., Silver, J., Gordon, W. A., et al. (2000). Axis II psychopathology in individuals with traumatic brain injury. Brain Inj. 13, 45–61. doi: 10.1080/0269905001209161
Izzy, S., Chen, P., Tahir, Z., Grashow, R., Radmanesh, F., Cote, D., et al. (2022). Association of traumatic brain injury with the risk of developing chronic cardiovascular, endocrine, neurological, and psychiatric disorders. JAMA Netw. Open 5:E229478. doi: 10.1001/jamanetworkopen.2022.9478
Jacobi, J., Fraser, G., Coursin, D., Riker, R., Fontaine, D., Wittbrodt, E., et al. (2002). Clinical practice guidelines for the sustained use of sedatives and analgesics in the critically ill adult. Crit. Care Med. 30, 119–141. doi: 10.1097/00003246-200201000-00020
Jager, K., Zoccali, C., MacLeod, A., and Dekker, F. (2008). Confounding: What it is and how to deal with it. Kidney Int. 73, 256–260. doi: 10.1038/sj.ki.5002650
Jain, A., Mittal, R., Sharma, A., Sharma, A., and Gupta, I. (2014). Study of insomnia and associated factors in traumatic brain injury. Asian J. Psychiatr. 8, 99–103. doi: 10.1016/j.ajp.2013.12.017
Jose, S., George, P., and Mathew, A. (2008). Assessment of confounding and interaction using the mantel-haenszel risk estimation method. Asian Pacific J. Cancer Prev. 9, 323–326.
Katzenberger, R., Ganetzky, B., and Wassarman, D. (2015). The gut reaction to traumatic brain injury. Fly 9, 68–74. doi: 10.1080/19336934.2015.1085623
Kayani, N., Homan, S., Yun, S., and Zhu, B. (2009). Health and economic burden of traumatic brain injury: Missouri, 2001-2005. Public Health Rep. 124, 551–560. doi: 10.1177/003335490912400412
Kim, L., Quon, J., Sun, F., Wortman, K., Adamson, M., and Harris, O. (2018). Traumatic brain injury among female veterans: A review of sex differences in military neurosurgery. Neurosurg. Focus 45:E16. doi: 10.3171/2018.9.FOCUS18369
Kraus, J., and McArthur, D. (1996). Epidemiologic aspects of brain injury. Neurol. Clin. 14, 435–450. doi: 10.1016/S0733-8619(05)70266-8
Kuritz, S., Landis, R., and Koch, G. (1988). A general overview of mantel-haenszel methods: Applications and recent developments. Annu. Rev. Public Health 9, 123–160. doi: 10.1146/annurev.pu.09.050188.001011
Langlois, J., Rutland-Brown, W., and Wald, M. (2006). The epidemiology and impact of traumatic brain injury A brief overview. J. Head Trauma Rehabil. 21, 375–378.
Lawrence, T., Helmy, A., Bouamra, O., Woodford, M., Lecky, F., and Hutchinson, P. (2016). Traumatic brain injury in England and Wales: Prospective audit of epidemiology, complications and standardised mortality. BMJ Open 6:12197. doi: 10.1136/bmjopen-2016
Lee, H., and Park, H. (2021). Comorbidity network analysis related to obesity in middle-Aged and older adults: Findings from Korean population-based survey data. Epidemiol. Health 43:e2021018. doi: 10.4178/EPIH.E2021018
Lee, Y., Lee, H., Leigh, J., Choi, Y., Kim, H., and Oh, B. (2021). The Socioeconomic Burden of Acquired Brain Injury among the Korean Patients over 20 Years of Age in 2015–2017: A Prevalence-Based Approach. Brain Neurorehabil. 14:e24. doi: 10.12786/bn.2021.14.e24
Liao, C., Chou, Y., Yeh, C., Hu, C., Chiu, W., and Chen, T. (2014). Stroke risk and outcomes in patients with traumatic brain injury: 2 Nationwide studies. Mayo Clin. Proc. 89, 163–172. doi: 10.1016/j.mayocp.2013.09.019
Ljubic, B., Pavlovski, M., Alshehri, J., Roychoudhury, S., Bajic, V., van Neste, C., et al. (2020). Comorbidity network analysis and genetics of colorectal cancer. Inform Med. Unlocked 21:100492. doi: 10.1016/j.imu.2020.100492
Maas, A. (2016). Traumatic brain injury: Changing concepts and approaches. Chin. J. Traumatol. 19, 3–6. doi: 10.1016/j.cjtee.2016.01.001
Mallya, S., Sutherland, J., Pongracic, S., Mainland, B., Ornstein, T., and Psych, C. (2015). The manifestation of anxiety disorders after traumatic brain injury: A review. J. Neurotrauma 32, 411–421.
McHugh, L. M. (2009). The odds ratio: Calculation, usage, and interpretation. Biochem. Med. 19, 120–126.
McLean, C., Asnaani, A., Litz, B., and Hofmann, S. (2011). Gender differences in anxiety disorders: Prevalence, course of illness, comorbidity and burden of illness. J. Psychiatr. Res. 45, 1027–1035. doi: 10.1016/j.jpsychires.2011.03.006
Mcmillan, T. (1991). Post-traumatic stress disorder and severe head injury. Br. J. Psychiatry 159, 431–433.
Mcmillan, T. (1996). Post-traumatic stress disorder following minor and severe closed head injury: 10 single cases. Brain Inj. 10, 749–758. doi: 10.1080/026990596124016
Middelboe, T., Andersen, H., Birket-Smith, M., and Friis, M. (1992). Psychiatric sequelae of minor head injury. A prospective follow-up study. Eur. Psychiatry 7, 183–189.
Mikolić, A., van Klaveren, D., Groeniger, J., Wiegers, E., Lingsma, H., Zeldovich, M., et al. (2021). Differences between men and women in treatment and outcome after traumatic brain injury. J. Neurotrauma 38, 235–251. doi: 10.1089/neu.2020.7228
Nyam, T., Ho, C., Chio, C., Lim, S., Wang, J., Chang, C., et al. (2019). Traumatic brain injury increases the risk of major adverse cardiovascular and cerebrovascular events: A 13-year, population-based study. World Neurosurg. 122, e740–e753. doi: 10.1016/j.wneu.2018.10.130
Olff, M. (2017). Sex and gender differences in post-traumatic stress disorder: An update. Eur. J. Psychotraumatol. 8:1351204. doi: 10.1080/20008198.2017.1351204
Pugh, M., Kennedy, E., Prager, E., Humpherys, J., Dams-O’connor, K., Hack, D., et al. (2021). Phenotyping the spectrum of traumatic brain injury: A review and pathway to standardization. J. Neurotrauma 38, 3222–3234. doi: 10.1089/neu.2021.0059
Rao, D., McFaull, S., Thompson, W., and Jayaraman, G. (2018). Traumatic brain injury management in canada: Changing patterns of care. Health Promot. Chronic Dis. Prev. Can. 38, 147–150. doi: 10.24095/hpcdp.38.3.05
Ripley, D., Driver, S., Stork, R., and Maneyapanda, M. (2019). Pharmacologic management of the patient with traumatic brain injury. rehabilitation after traumatic brain injury. Amsterdam: Elsevier.
Robert Laskowski, A., Creed, J., and Raghupathi, R. (2015). “Pathophysiology of Mild TBI Implications for Altered Signaling Pathways,” in Brain neurotrauma: Molecular, neuropsychological, and rehabilitation aspects, ed. F. Kobeissy (Boca Raton, FL: CRCPress).
Rutland-Brown, W., Langlois, J., Thomas, K., and Xi, Y. (2006). Incidence of traumatic brain injury in the United States, 2003. J. Head Trauma Rehabil. 21, 544–548.
Saatman, K., Duhaime, A., Bullock, R., Maas, A., Valadka, A., and Manley, G. (2008). Classification of traumatic brain injury for targeted therapies. J. Neurotrauma 25, 719–738. doi: 10.1089/neu.2008.0586
Schwab, N., Taskina, D., Leung, E., Innes, B., Bader, G., and Hazrati, L. (2022). Neurons and glial cells acquire a senescent signature after repeated mild traumatic brain injury in a sex-dependent manner. Front. Neurosci. 16:1027116. doi: 10.3389/fnins.2022.1027116
Schwarzbold, M., Diaz, A., Martins, E., Rufino, A., Amante, L., Thais, M., et al. (2008). Psychiatric disorders and traumatic brain injury. Neuropsychiatr. Dis. Treat. 4, 797–816.
Shoumitro, D., Lyons, I., Koutzoukis, C., Ali, I., and Mccarthy, G. (1999). Rate of psychiatric illness 1 year after traumatic brain injury. Am. J. Psychiatry 156:3.
Si, B., Dumkrieger, G., Wu, T., Zafonte, R., Dodick, D., Schwedt, T., et al. (2018a). A cross-study analysis for reproducible sub-classification of traumatic brain injury. Front. Neurol. 9:606. doi: 10.3389/fneur.2018.00606
Si, B., Dumkrieger, G., Wu, T., Zafonte, R., Valadka, A., Okonkwo, D., et al. (2018b). Sub-classifying patients with mild traumatic brain injury: A clustering approach based on baseline clinical characteristics and 90-day and 180-day outcomes. PLoS One 13:e0198741. doi: 10.1371/journal.pone.0198741
Stein, D. (2015). Embracing failure: What the Phase III progesterone studies can teach about TBI clinical trials. Brain Inj. 29, 1259–1272. doi: 10.3109/02699052.2015.1065344
Stein, M., Jain, S., Giacino, J., Levin, H., Dikmen, S., Nelson, L., et al. (2019). Risk of posttraumatic stress disorder and major depression in civilian patients after mild traumatic brain injury: A TRACK-TBI study. JAMA Psychiatry 76, 249–258. doi: 10.1001/jamapsychiatry.2018.4288
Sudhakar, S. (2023). Are GABAergic drugs beneficial in providing neuroprotection after traumatic brain injuries? A comprehensive literature review of preclinical studies. Front. Neurol. 14:1109406. doi: 10.3389/fneur.2023.1109406
Sudhakar, S., Choi, T., and Ahmed, O. (2019). Biophysical modeling suggests optimal drug combinations for improving the efficacy of GABA agonists after traumatic brain injuries. J. Neurotrauma 36, 1–14. doi: 10.1089/neu.2018.6065
Tanriverdi, F., Ulutabanca, H., Unluhizarci, K., Selcuklu, A., Casanueva, F., and Kelestimur, F. (2008). Three years prospective investigation of anterior pituitary function after traumatic brain injury: A pilot study. Clin. Endocrinol. 68, 573–579. doi: 10.1111/j.1365-2265.2007.03070.x
Traumatic Brain Injury Model Systems Program (2021). Traumatic brain injury model systems national database. Traumatic Brain Injury Model Systems National Data and Statistical Center, doi: 10.17605/OSF.IO/A4XZB
Tripepi, G., Jager, K., Dekker, F., and Zoccali, C. (2010). Stratification for confounding-part 1: The mantel-haenszel formula. Nephron. Clin. Pract. 116, c317–c321. doi: 10.1159/000319590
Tso, S., Saha, A., and Cusimano, M. (2021). The traumatic brain injury model systems national database: A review of published research. Neurotrauma Rep. 2, 149–164. doi: 10.1089/neur.2020.0047
Turner, G., McMullan, C., Aiyegbusi, O., Bem, D., Marshall, T., Calvert, M., et al. (2021). Stroke risk following traumatic brain injury: Systematic review and meta-analysis. Int. J. Stroke 16, 370–384. doi: 10.1177/17474930211004277
Vella, M., Crandall, M., and Patel, M. (2017). Acute management of traumatic brain injury. Surg. Clin. North Am. 97, 1015–1030. doi: 10.1016/j.suc.2017.06.003
Wang, J., Su, E., Wang, H., Guo, C., Lawrence, D., and Eitzman, D. (2018). Traumatic brain injury leads to accelerated atherosclerosis in apolipoprotein e deficient mice. Sci. Rep. 8:5639. doi: 10.1038/s41598-018-23959-2
Werner, C., and Engelhard, K. (2007). Pathophysiology of traumatic brain injury. Br. J. Anaesth. 99, 4–9. doi: 10.1093/bja/aem131
Ye, X., Asim, M., and Michael, C. (2009). Emerging treatments for traumatic brain injury. Expert Opin. Emerg. Drugs 14, 67–84. doi: 10.1517/14728210902769601.Emerging
Yeh, C., Chen, T., Hu, C., Chiu, W., and Liao, C. (2013). Risk of epilepsy after traumatic brain injury: A retrospective population-based cohort study. J. Neurol. Neurosurg. Psychiatry 84, 441–445. doi: 10.1136/jnnp-2012-302547
Zatzick, D., Rivara, F., Jurkovich, G., Hoge, C., Wang, J., Fan, M., et al. (2010). Multisite investigation of traumatic brain injuries, posttraumatic stress disorder, and self-reported health and cognitive impairments. Arch. Gen. Psychiatry 67, 1291–1300. doi: 10.1001/archgenpsychiatry.2010.158
Keywords: traumatic brain injury, comorbidities, prevalence, psychiatric, mild TBI, rehabilatation, medical complications
Citation: Sudhakar SK, Sridhar S, Char S, Pandya K and Mehta K (2023) Prevalence of comorbidities post mild traumatic brain injuries: a traumatic brain injury model systems study. Front. Hum. Neurosci. 17:1158483. doi: 10.3389/fnhum.2023.1158483
Received: 03 February 2023; Accepted: 26 May 2023;
Published: 15 June 2023.
Edited by:
Fernando Zanela Arêas, Federal University of Espirito Santo, BrazilReviewed by:
Carmen M. Cirstea, University of Missouri, United StatesAnthony P. Salvatore, University of Louisiana at Lafayette, United States
Copyright © 2023 Sudhakar, Sridhar, Char, Pandya and Mehta. This is an open-access article distributed under the terms of the Creative Commons Attribution License (CC BY). The use, distribution or reproduction in other forums is permitted, provided the original author(s) and the copyright owner(s) are credited and that the original publication in this journal is cited, in accordance with accepted academic practice. No use, distribution or reproduction is permitted which does not comply with these terms.
*Correspondence: Shyam Kumar Sudhakar, c2h5YW1rdW1hci5zdWRoYWthckBrcmVhLmVkdS5pbg==
†These authors share first authorship